Dynamic changes in coagulation, hematological and biochemical parameters as predictors of mortality in critically ill COVID–19 patients: A prospective observational study
Abstract
INTRODUCTION:
This study was created to analyze dynamic alterations in coagulation, hematological and biochemical parameters and their association with mortality of COVID-19 patients. To identify the most sensitive biomarkers as predictors of mortality more research is required.
METHODS:
The present study was a prospective, one-year-long observational study conducted on all critically ill, COVID–19 patients with respiratory failure. The following data were collected: demographic and clinical characteristics of the study population, comorbidities, coagulation, biochemical and hematological parameters. The primary outcome was the proportion of patients who died.
RESULTS:
91 patients with median age 60 (50–67), 76.9% male, met the acute respiratory distress syndrome criteria. It was tested whether dynamic change (delta-Δ) of parameters that were found to be predictors of mortality is independently associated with poor outcome. Adjusted (multivariate) analysis was used, where tested parameters were corrected for basic and clinical patients characteristics. The only inflammatory parameter which dynamic change had statistically significant odds ratio was ΔCRP (p < 0.005), while among coagulation parameters statistically significant OR was found for Δ fibrinogen (p < 0.005) in predicting mortality.
CONCLUSION:
Monitoring of coagulation, hematological and biochemical parameters abnormalities and their dynamical changes can potentially improve management and predict mortality in critically ill COVID –19 patients.
1Introduction
Coronavirus disease (COVID–19) caused by the severe acute respiratory syndrome coronavirus 2 (SARS –CoV-2) [1], was reported for the first time in Wuhan, China in December, 2019, and has become an ongoing global pandemic. By April,2022, there have been over 481 million cases and over 6 million deaths reported globally since the start of the pandemic [2]. COVID-19 is a multisystem inflammatory syndrome with multi - organ involvement and a complex pathogenesis that incorporates hemostasis, the immune system, and the hematopoietic system. COVID-19-associated coagulopathy refers to hemostatic pathway abnormalities which are very common in critically ill COVID-19 patients [3]. Both venous and arterial thrombotic events have been identified as independent risk factors for mortality [4, 5]. In the majority of COVID-19 patients, including critically ill, with severe coagulation abnormalities, disseminated intravascular coagulation (DIC) and thrombotic microangiopathy are common complications [6]. D-dimer is the predominant coagulation biomarker studied in critically ill COVID-19 patients, and its value is significantly increased in 50 percent of COVID-19 patients who are at significantly higher risk of death. Rasyd et al reported that D-dimer can be one predictor of mortality in COVID-19 patients with acute stroke [7–11]. In addition, thrombocytopenia, slight prolongation of the prothrombin time (PT), activated partial tromboplastin time (aPTT), increased plasma fibrinogen levels, and reduction in plasma fibrinogen levels in some patients were also found in critically ill COVID-19 patients. Nugroho et al had similar results; eleveted fibrinogen levels on admission where associated with poor outcome in COVID-19 patients [3, 11–15]. Besides, death has been associated with low levels of natural anticoagulants (antithrombin and protein C) and factor V activity [11, 16, 17]. Many biomarkers, besides coagulation parameters, has been found to be abnormal in critically ill COVID-19 patients. These include inflammatory markers like elevated CRP, erythrocyte sedimentation rate, white blood cell count, fibrinogen, procalcitonin, as well as tissue injury indicators, such as elevated lactic dehydrogenase (LDH), alanine aminotransferase (AST), cardiac troponins. There are multiple reports that CRP, LDH, creatinine, cardiac troponin I (TnI), ALT, AST, leukocytes, neutrophils, were more elevated in critically ill COVID-19 patients [11, 18–20]. Some studies have explored the relationship between mortality and laboratory abnormalities. These investigations showed elevated values of CRP, LDH, D-dimer, creatinine, cardiac troponins, leukocytes, ALT, PT and procalcitonin in non-survivors compared to survivors [7, 11, 18, 21, 22]. Additional studies are needed to select the most sensitive biomarkers or combination of biomarkers as predictors of mortality in critically ill COVID-19 patients. Taking into account the aforementioned, this study was designed to analyze dynamic alterations in coagulation, hematological and biochemical parameters, and their association with mortality of critically ill COVID-19 patients.
2Material and methods
2.1Study design
The present study was a prospective, observational study conducted during an one-year-period (September 1, 2020/August 31, 2021) on selective adults critically ill COVID–19 patients with respiratory failure, admitted to the MICU of the University Clinical Centre of the Republic of Srpska (UCC-RS). The MICU serves as a referral centre of the Republic of Srpska, which is currently the most advanced multidisciplinary MICU in Bosnia and Herzegovina. This study has been performed in accordance with the ethical standards as laid down in the 1964 Declaration of Helsinki and its later amendments or comparable ethical standards and our Institutional Ethics Commitee (Chairperson prof. S. Satara) provided approval for the study (Ethical committee N° 01-19-533-2/20) on 16 october 2020. Written informed consent was signed by all patients or their legal representative. COVID–19 was confirmed by detection of SARS–CoV–2 RNA in nasopharyngeal or throat swab or broncoalveolar lavage semples, using the real time polymerase chain reaction (RT-PCR) method.
2.2Outcomes and predictor variables
The following data were collected: demographic and clinical characteristics of the study population, pre-existing comorbidities (cardiovascular diseases, pulmonary diseases, diabetes mellitus), coagulation, biochemical and hematological parameters. The primary outcome was the proportion of patients who died (non-survivors).
2.3Sample collection
The venous blood samples were collected on day 1 and day 7 of admission to the medical ICU. The following tests were performed:
• Coagulation parameters: D-dimer, fibrinogen, aPTT, INR, coagulation factors II, V, VII, VIII, IX, X, XI, XII, XIII, vWF, protein C and S. Fully automated coagulation analyser was used for performing all the coagulation tests.
• Biochemical parameters: urea, creatinin, bilirubin direct, bilirubin indirect, AST, ALT, LDH, creatine kinase, troponin, albumin, ionized calcium-Ca2 +, CRP, procalcitonin, IL-6, ferritine, lactate.
• Hematological parameters: absolute eritrocyte count, absolute leucocyte counts, absolute lymphocyte counts, platelet count, hemoglobin, iron, UIBC, TIBC.
The samples were analyzed in the biochemical laboratory in the UCC-RS. Fully automated biochemeical analyser was used, according to the manufacturer’s recommendations.
2.4Inclusion criteria
All adult, RT-PCR positive patientswith respiratory failure due to COVID-19 pneumonia and with a need for mechanical ventilation were included in the study.
2.5Exclusion criteria
Patients were excluded from the study if they had: sepsis or septic shock, respiratory failure not casued by COVID-19, thromboembolic disorders not related to COVID-19, cancer, liver or kidney disease, hematological disorders, surgeries or trauma, pregnancy.
2.6Statistical analysis
The study data were analyzed using SPSS version 26.0. All continuous variables were expressed as median (interquartile range [IQR]) and categorical variables as numbers and proportions. The Kolmogorov–Smirnov test was used for testing the normality of data distribution and Mann–Whitney U and Kruskal–Wallis tests were used for comparing continuos variables between the groups. The chi–square/Fisher exact test and Pearson’s tests were used for comparing categorical data. To analyze the efficiency of coagulation, biochemical and hematological parameters in predicting mortality, receiver operation characteristic (ROC) curve was used and the area under the ROC curve (AUC) was designed. For calculated AUC for parameters, non–survivor cases were taken as primary outcome.The multivariate logistic regression analysis was performed and adjusting for age, comorbidity and the coagulation, biochemical and hematological parameters. The odds-ratios (OR) with 95 % confidence intervals were calculated. The P < 0.05 was considered statistically significant.
3Results
There were 91 patients included in this study. The demographic and clinical characteristics of the patients are shown in Table 1. Out of the 91 patients, 46 (50.5%) of them died and 45 (49.5%) recovered. The median age was 60 years (IQR, 50–67) and there was a statistically significant association between their age and mortality. The population in this study consisted mostly of men, (76.9%). A total of 70/91 patients (76.9%) receaved full-dose anticoagulation therapy.
Table 1
The demographic and clinical characteristics of the study population
Parameters | Total | Surviver | Non - surviver | p |
n (%) | 91 (100%) | 45 (49.5%) | 46 (50.5%) | |
Age (years) | 60 (50–67) | 56,5 (46–63) | 61 (56–69) | 0.027 |
Male | 70 (76.9%) | 33 (73.3%) | 37 (80.4%) | 0.464 |
Duration of ICU stay (days) | 12 (8–18) | 12 (8,5–16) | 12.5 (7,75–20) | 0.466 |
Illnes before hospitalization (days) | 6 (5–8) | 7 (4–8) | 6 (5–8.25) | 0.466 |
SOFA score, day 1 | 33 (33–50) | 33 (33–45) | 33 (33–50) | 0.402 |
SOFA score, day 7 | 33 (33–50) | 33 (33–33) | 50 (50–80) | 0.000 |
APACHE score | 15 (8–15) | 10 (8–15) | 15 (9.75–25) | 0.127 |
SAPS II score | 12 (5,80–24) | 11,8 (4.2–24) | 13,4 (6.1–24) | 0.599 |
FiO2 | NA | 70 (60–100) | 80 (70–100) | 0,141 |
PEEP | NA | 10 (8–10) | 10 (9,5–10) | 0,320 |
Respiratory rate,admission | NA | 30 (27–32) | 29 (26–32) | 0,765 |
Heart rate,admission | NA | 90 (80–102) | 97,5 (80–110) | 0,596 |
Smoking | 33 (36.3%) | 25 (54.3%) | 8 (17.8%) | 0,000 |
Cardiovascular desease | 14 (15.4%) | 7 (15.6%) | 7 (15.2%) | 1 |
Hypertension | 44 (48.4%) | 20 (44.4%) | 24 (52.2%) | 0,531 |
Diabetes | 32 (35.2%) | 14 (31.1%) | 18 (39.1%) | 0,512 |
Chronic pulmonary desease | 5 (5.6%) | 4 (9.1%) | 1 (2.2%) | 0,198 |
Chronic kidney desease | 4 (4.4%) | 2 (4.4%) | 2 (4.3%) | 1 |
Charlson index | 90 (53–90) | 90 (53–90) | 78 (53–90) | 0,346 |
ECMO | 6 (6.6%) | 1 (2.2%) | 5 (10.9%) | 0,203 |
CRRT | 15 (16.5%) | 2 (13.3%) | 13 (86.7%) | 0,004 |
AKI | 30 (33%) | 2 (4.4%) | 28 (60.9%) | 0,000 |
Thromboembolic events | 23/91 (25.3%) | 8 (34.8%) | 15 (65.2%) | 0,148 |
Non-invasive mechanical ventilation | 29/91 (31.8%) | 29 (100%) | 0 | 0,000 |
Bleeding events | 7/91 (7.7%) | 0 | 7 (100%) | 0,000 |
Anticoagulation,therapeutic dose | 70/91 (76.9%) | 39 (55.7%) | 31 (44.3%) | 0,072 |
ICU, intensive care medicine; SOFA, sequential organ failure assessment; APACHE, Acute Physiology, and Chronic Health Evaluation II; SAPS II, simplified acute physiology score II; FiO2, a fraction of inspired oxygen; PEEP, positive end-expiratory pressure; ECMO, extracorporeal membrane oxygenation; CRRT, continuous renal replacement therapy; AKI, acute kidney injury. All results are given in median [IQR] or as as numbers and proportions, n(%).
A receiver-operating characteristics curve analysis was performed since there was a statistically significant difference between the groups of survivors and non-survivors for the parameters years, SOFA score day 7, smoking, AKI, CRRT, and IMV (Fig. 1). The area under the ROC curve for SOFA score day 7 was the largest (AUC = 0.874), followed by the area under the ROC curve for IMV (AUC = 0.814), indicating that both parameters are excellent predictors of mortality. The third highest area under the ROC curve for AKI (AUC = 0.774) was considered an acceptable predictor of mortality.
Fig. 1
Receiver-operating characteristic curves for significant demographical and clinical parameters in the prediction of mortality.
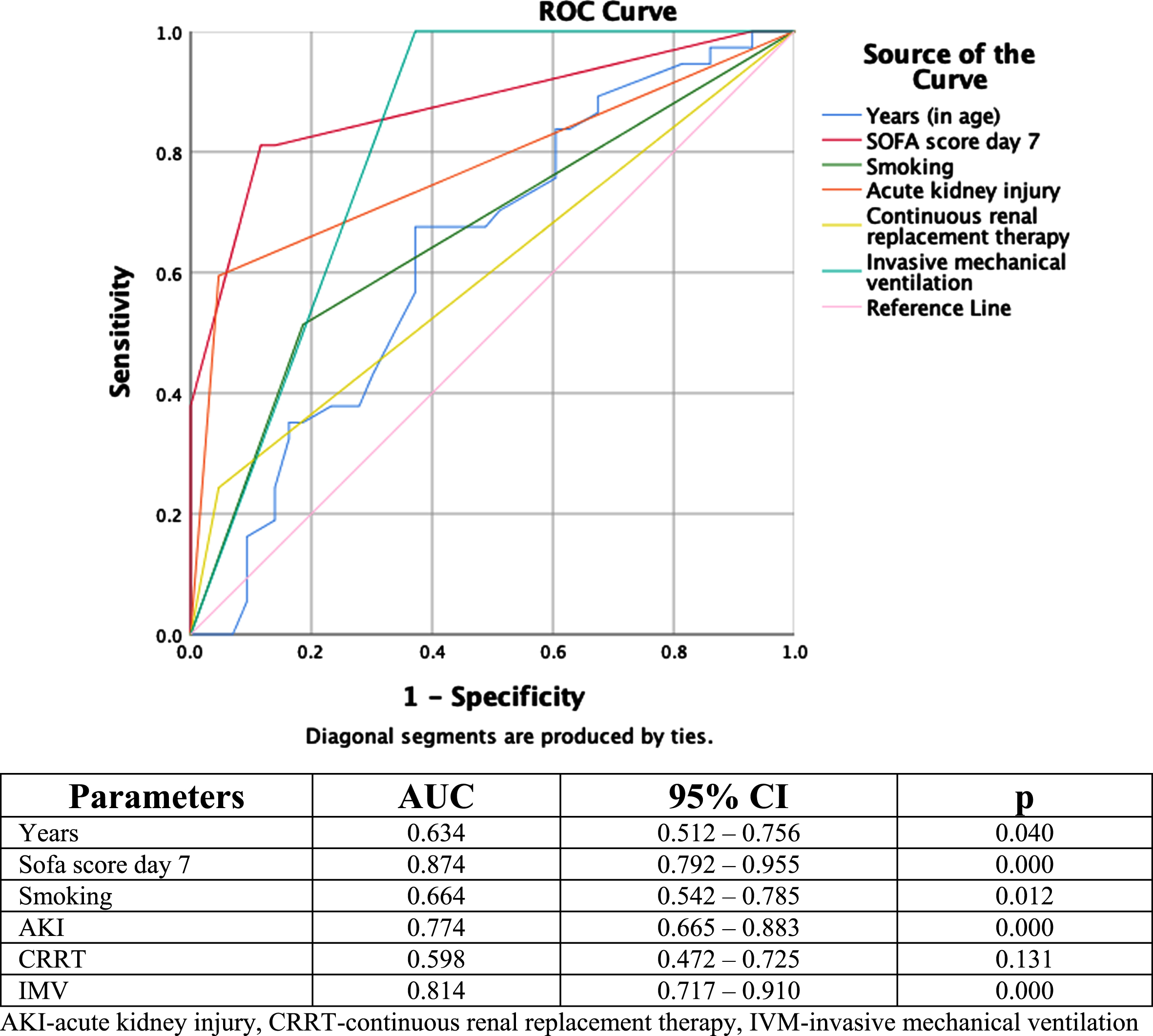
Table 2 shows dynamic changes/delta values for biochemical parameters. The delta (Δ) value represents a dynamic change from biomarker value from day one to day seven. Statistically significant difference between survivors and non-survivors for Dbil Δ (p = 0.030), LDH Δ (p = 0.002), troponin Δ (p = 0.008), CRP Δ (p = 0.004) was found.
Table 2
Dynamic changes/ delta (Δ) values for biochemical parameters
Parameters (Δ) | Total | Surviver | Non - surviver | p |
Urea delta | 1.20 (4.8) | 1.50 (5.73) | 0.40 (3.70) | 0.273 |
Creatinin delta | –6 (39) | –6 (79.25) | –6 (25) | 0.943 |
Tbil delta | –0.45 (12.68) | 0.15 (11.48) | –0.90 (17.02) | 0.578 |
Dbil delta | 2.10 (5.80) | 0.70 (5.65) | 3 (6.50) | 0.030 |
AST delta | –7 (47) | –7.50 (57.25) | –5 (39) | 0.784 |
ALT delta | –5 (63) | –5 (50.75) | –5 (70) | 0.625 |
LDH delta | –204 (376) | –323 (416) | –155 (301) | 0.002 |
CK delta | –75 (191.75) | –101.50 (184.75) | –4.75 (200.25) | 0.614 |
Alb delta | –2 (4) | –2 (5) | –2 (3.50) | 0.589 |
Ca 2 + delta | –0.02 (0.21) | –0.01 (0.19) | –0.02 (0.21) | 0.515 |
CRP delta | –3 (124) | 31.05 (136.7) | –20.50 (100.65) | 0.004 |
PCT delta | 0.16 (1.97) | 0.46 (5.90) | 0.01 (0.99) | 0.213 |
IL–6 delta | –3.10 (95.1) | –3.07 (129.11) | –7.90 (41.80) | 0.536 |
Ferirtin delta | –82 (693) | –112 (1010) | –46 (533.5) | 0.815 |
Lactate delta | –0.40 (1.22) | –0.50 (1.30) | –0.30 (1.64) | 0.185 |
Tbil, total bilirubin; Dbil, direct bilirubin; AST, aspartate aminitransferase; ALT, alanin aminotransferase; LDH, lactic dehidrogenaase; CK, cretine cinase; Alb, albumin: Ca2+, calcium; CRP, C –reactive protein; PCT, procalcitonin; IL-6, interleukin 6. Delta value, dynamic change from biomarker value from day 1 to day 7.
Table 3 shows dynamic changes/delta (Δ) values for hematological parameters. The Δ value represents a dynamic change from biomarker value from day one to day seven. Statistically significant difference between survivors and non-survivors for RBC Δ (p = 0.023), Ly Δ (p = 0.018), Hb Δ (p = 0.003) was found.
Table 3
Dynamic changes /delta (Δ) value for hematological parameters
Parameters (Δ) | Total | Surviver | Non - surviver | p |
RBC delta | –0.42 (1.07) | –0.60 (0.76) | –0.35 (0.76) | 0.023 |
WBC delta | 1.80 (4.73) | 1.01 (4.08) | 2.40 (4.88) | 0.427 |
Ly delta | 0.22 (0.70) | 0.03 (0.79) | 0.35 (0.67) | 0.018 |
Plt delta | –18 (172) | –57 (172) | –14 (168) | 0.104 |
Hb delta | –13 (32) | –23.50 (44.75) | –6 (18) | 0.003 |
Fe delta | 0.85 (7.75) | 0.85 (7.62) | 0.85 (10.45) | 0.488 |
UIBC delta | –6.20 (13.75) | –8.70 (14.70) | –4.30 (12.82) | 0.325 |
TIBC delta | –1.20 (12.20) | –2.15 (11.47) | 0 (11.52) | 0.208 |
RBC, red blood count,; WBC, white blood count;; Ly, absolute Lymphocyte count; Plt, platelet count; Hb, hemoglobin; Fe, iron; UIBC, unsaturated iron-binding capacity; TIBC, total iron-binding capacity. Δ value, dynamic change from biomarker value from day 1 to day 7.
Table 4 shows dynamic changes/delta (Δ) values for coagulation parameters. The Δ value represents a dynamic change from biomarker value from day one to day seven. There was no statistically significant difference between survivors and non-survivors in Δ values for all measured coagulation parameters, except for fibrinogen (p = 0.048)
Table 4
Dynamic changes/delta (Δ) values for coagulation parameters
Parameters (Δ) | Total | Surviver | Non-surviver | p |
F II delta | –6 (39) | –2.50 (43.25) | –10 (36) | 0.703 |
F V delta | –15 (62) | –1.50 (57) | –18 (51) | 0.144 |
F VII delta | –3 (41) | –6 (45) | –2 (35.50) | 0.565 |
F VIII delta | 0 (0.46) | 0 (4.25) | 0 (0.23) | 0.983 |
F IX delta | –1 (60) | –3 (69.75) | –1 (65.50) | 0.739 |
F X delta | –12 (46) | –0.5 (61.75) | –15 (36.5) | 0.170 |
F XI delta | –14 (51) | –14.5 (53.25) | –14 (51.5) | 0.843 |
F XII delta | –9 (38) | –13.5 (37) | –1 (39) | 0.266 |
F XIII delta | –46 (49.5) | –40.50 (59.25) | –54 (42) | 0.599 |
vWF delta | 0 (0) | 0 (0) | 0 (0) | 0.271 |
protein C delta | 16 (49) | 3.50 (50.25) | 17 (42.50) | 0.086 |
protein S delta | 0 (43) | –8.50 (52.25) | 1 (32) | 0.074 |
Antithrombin delta | –3 (34) | –4 (36.50) | –3 (38) | 0.584 |
Aptt delta | 2.40 (9.40) | 3.20 (10.08) | 2.30 (9.05) | 0.116 |
INR delta | 0.04 (0.30) | 0.03 (0.29) | 0.04 (0.29) | 0.453 |
Parameters ( Δ) | Total | Surviver | Non-surviver | p |
D –dimer delta | 0.20 (11.17) | –1.60 (24.07) | 0.43 (4.11) | 0.066 |
Fibrinogen delta | –1.80 (3) | –1.20 (3.24) | –2.30 (2.70) | 0.048 |
Coagulation cascade factors: F II, factor II; F V, factor V, F X, factor X; F XI, factor XI; F XIII, factor XIII; vWF, von Wilebrand factor; aptt, partial thromboplastin time; INR, international normalized ratio; Delta value, dynamic change from biomarker value from day 1 to day 7.
Table 5 shows the association between demographic/clinical and biochemical/inflammatory parameters and survival in the study cohort. In the unadjusted (univariate) analysis, the OR was statistically significant for CRP day 1 (OR = 1.006; 95% CI 1 to 1.012; p = 0.038), CRP day 7 (OR = 1.016; 95% CI 1.009 to 1.024; p = 0.000), ΔCRP (OR = 1.006; 95% CI 1.001 to 1.011; p = 0.010) and Ly day 7 (OR = 0.126; 95% CI 0.041 to 0.379; p = 0.000) in predicting mortality. Moreover, the same parameters kept a significant association with a poor prognosis even in the adjusted (multivariate) analysis, CRP day 1 (AOR = 1.008; 95% CI 1.002 to 1.015; p = 0.016), CRP day 7 [AOR = 1.017 95% CI 1.009 to 1.024; p = 0.000), ΔCRP (AOR = 1.006; 95% CI 1.001 to 1.011; p = 0.029) and Ly day 7 (AOR = 0.113; 95% CI 0.034 to 0.377; p = 0.000).
Table 5
Association of demographic/clinical and biochemical/inflammatory parameters with mortality
Inflammatory parameters | Unadjusted | Adjusted* | ||||
OR | CI (95%) | p | OR | CI (95%) | p | |
CRP day 1 | 1.006 | 1–1.012 | 0.038 | 1.008 | 1.002–1.015 | 0.016 |
CRP day 7 | 1.016 | 1.009–1.024 | 0.000 | 1.017 | 1.009–1.024 | 0.000 |
CRP Δ | 1.006 | 1.001–1.011 | 0.010 | 1.006 | 1.001–1.011 | 0.029 |
IL-6 day 1 | 1.004 | 0.999–1.008 | 0.086 | 1.004 | 0.999–1.008 | 0.107 |
IL-6 day 7 | 1.003 | 1–1.005 | 0.078 | 1.002 | 1–1.005 | 0.097 |
IL-6 Δ | 1 | 0.999–1.001 | 0.462 | 1 | 0.999–1.002 | 0.477 |
Ly day 1 | 0.333 | 0.097–1.141 | 0.080 | 0.366 | 0.097–1.374 | 0.136 |
Ly day 7 | 0.126 | 0.041–0.379 | 0.000 | 0.113 | 0.034–0.377 | 0.000 |
Ly Δ | 0.656 | 0.341–1.256 | 0.204 | 0.636 | 0.331–1.222 | 0.175 |
OR,odds ratio; CI (95%), 95% confidence interval; CRP, C - reactive protein; IL-6, interleukin 6; Ly, total lymphocyte count. *Adjusted for sequential organ failure assessment (SOFA) score, age, sex, Charlson comorbidity index.
Table 6 shows the association between demographic/clinical and coagulation parameters and survival in the study cohort. In the unadjusted univariate analysis, the OR was statistically significant for F II day 1 (OR = 0.974; 95% CI 0.956 to 0.992; p = 0.005), F II day 7 (OR = 0.982; 95% CI 0.965 to 0.999; p = 0.040), F V day 1 (OR = 0.089; 95% CI 0.977 to 1; p = 0.049) and F XIII day 7 (OR = 0.975; 95% CI 0.952 to 0.998; p = 0.035) in predicting mortality. In the adjusted multivariate analysis only parameters F II day 1 (AOR = 0.978; 955 CI 0.960 to 0.997; p = 0.025) and F XIII day 7 (AOR = 0.975; 95% CI 0.950 to 1; p = 0.048) kept a significant association with a poor prognosis.
Table 6
Association of demographic/clinical and coagulation parameters with mortality
Coagulation parameters | Unadjusted | Adjusted* | ||||
OR | CI (95%) | p | OR | CI (95%) | P | |
F II day 1 | 0.974 | 0.956–0.992 | 0.005 | 0.978 | 0.960–0.997 | 0.025 |
F II day 7 | 0.982 | 0.965–0.999 | 0.040 | 0.985 | 0.967–1.002 | 0.089 |
F II Δ | 1.004 | 0.991–1.017 | 0.536 | 1.003 | 0.989–1.016 | 0.719 |
F V day 1 | 0.089 | 0.977–1 | 0.049 | 0.991 | 0.980–1.003 | 0.132 |
F V day 7 | 0.993 | 0.979–1.007 | 0.358 | 0.991 | 0.976–1.006 | 0.254 |
F V Δ | 1.005 | 0.996–1.015 | 0.265 | 1.003 | 0.993–1.013 | 0.567 |
F X day 1 | 1.001 | 0.996–1.006 | 0.688 | 1.001 | 0.995–1.008 | 0.653 |
F X day 7 | 1.005 | 0.991–1.005 | 0.484 | 1.007 | 0.991–1.023 | 0.388 |
F X Δ | 1 | 0.995–1.004 | 0.860 | 0.999 | 0.994–1.005 | 0.847 |
F XI day 1 | 0.991 | 0.980–1.002 | 0.094 | 0.992 | 0.981–1.003 | 0.137 |
F XI day 7 | 0.987 | 0.973–1.001 | 0.066 | 0.991 | 0.977–1.005 | 0.208 |
F XI Δ | 1.002 | 0.997–1.008 | 0.400 | 1.003 | 0.994–1.011 | 0.530 |
F XIII day 1 | 0.987 | 0.973–1.001 | 0.060 | 0.989 | 0.975–1.003 | 0.134 |
F XIII day 7 | 0.975 | 0.952–0.998 | 0.035 | 0.975 | 0.950–1 | 0.048 |
F XIII Δ | 1.004 | 0.992–1.015 | 0.545 | 1.002 | 0.990–1.014 | 0.744 |
Fibrinogen day 1 | 0.789 | 0.596–1.043 | 0.097 | 0.816 | 0.612–1.087 | 0.165 |
Fibrinogen day 7 | 1.075 | 0.797–1.449 | 0.634 | 1.080 | 0.777–1.500 | 0.647 |
Fibrinogen Δ | 1.193 | 0.959–1.483 | 0.111 | 1.175 | 0.934–1.478 | 0.168 |
OR, odds ratio; CI (95%), 95% confidence interval; Coagulation cascade factors: F II, factor II; F V, factor V, F X, factor X; F XI, factor XI; F XIII, factor XIII; *Adjusted for sequential organ failure assessment (SOFA) score, age, sex, Charlson comorbidity index.
Dynamic change (Δ) of parameters for which we found to be predictors of mortality was tested in order to identify those which were independently associated with poor outcome in patients. Adjusted (multivariate) analysis was used, in which parameters were corrected by basic and clinical patients characteristics. The only inflammatory parameter which dynamic change had statistically significant OR was ΔCRP (AOR = 1.015; 95% CI 1.007 to 1.022), p = 0.000) and among coagulation parameters statistically significant OR was found for Δfibrinogen (AOR = 1.371; 95% CI 1.029 to 1.827), p = 0.031), when lethal outcome is concerned, as shown in Table 7.
Table 7
Association of demographic/clinical parameters and dynamic value (Δ value) of inflammatory/coagulation parameters with mortality
Adjusted* | |||
Inflammatory parameters | OR | CI (95%) | p |
CRP Δ | 1.015 | 1.007–1.022 | 0.000 |
IL-6 Δ | 1 | 0.999–1.002 | 0.527 |
Ly Δ | 0.549 | 0.291–1.038 | 0.065 |
Coagulation parameters | OR | CI (95%) | p |
F II Δ | 0.988 | 0.970–1.006 | 0.193 |
F V Δ | 0.998 | 0.986–1.009 | 0.679 |
F X Δ | 0.997 | 0.990–1.005 | 0.481 |
F XI Δ | 1.002 | 0.994–1.010 | 0.661 |
F XIII Δ | 0.993 | 0.979–1.007 | 0.317 |
Fibrinogen Δ | 1.371 | 1.029–1.827 | 0.031 |
OR, odds ratio; CI (95%), 95% confidence interval; CRP, C - reactive protein; IL-6, interleukin 6; Ly, total lymphocyte count; Coagulation cascade factors: F II, factor II; F V, factor V, F X, factor X; F XI, factor XI; F XIII, factor XIII; * Adjusted for SOFA score (sequential organ failure assessment), Age, Sex, CRP-day 1, F II-day 1.
4Discussion
This study showed that dynamic change of coagulation, biochemical and hematological biomarkers can have significant predictive value for mortality of critically ill COVID-19 patients. The C-reactive protein and fibrinogen are the only two biochemical and coagulation parameters which dynamic changes in critically ill COVID-19 patients are independent predictors of mortality. Recent studies have reported that CRP level is elevated in patients with COVID-19 and may correlate with the severity of the disease, disease progression and mortality [23–25]. Sharifpour et al. had similar results to the present ones; patients who died had significantly higher CRP levels than those who survived [26]. Meta-analysis of 20 studies including 4,843 COVID-19 patients provided by the Preeti et al. reported that there was almost four times greater risk of poor outcome for COVID-19 patients with high CRP [27]. It seems that there are no studies that analyzed the dynamic change of this parameter. It was found in this study that many of parameters can potentially be important predictors of mortality, such as CRP, LDH, troponin, PCT, ferritin, what is similar with previous studies [7, 14, 18]. However, when multivariate analysis adjusted for SOFA score, age, gender and Charlson comorbidity index was performed, only the CRP value, static or dynamic, was identified as an independent predictor of mortality. It was found that total red blood count, total lymphocyte count, and hemoglobin can potentially be predictors of mortality, which was also found in other studies [18, 33]. However, multivariate analysis adjusted for SOFA score, age, gender, and Charlson comorbidity index was performed, only the total lymphocyte counts on day seven was identified as a potential independent predictor of mortality. Several studies have associated lymphopenia with poor prognosis of COVID-19 [19, 20, 22, 33]. In available literature no data on lymphocytes dynamic change in COVID-19 patients could be found. Fibrinogen is a well studied biomarker in patients suffering from COVID-19. Some studies have indicated that fibrinogen was associated with the severity of COVID-19 disease, while others indicated that fibrinogen alone was not significant in predicting mortality [11, 30, 31]. It was found in the present that change in fibrinogen dynamics, increased fibrinogen levels, and its evaluation over time is an independent biomarker of mortality in seriously ill COVID-19 patients and may guide physicians in predicting the outcomes. Based on the available information, there are no studies that analyzed the dynamic change of this parameter. This is the first report that studied all coagulation factors (II, V, VII, VIII, IX, X, XI, XII, XII, vWF, protein C, protein S, antithrombin) together with their dynamic changes, in comparison to many published studies that have focused only on few parameters (such as D-dimer, PT, aPTT, factor V, factor VIII) [11, 13, 16, 17, 28, 29]. This study clearly showed that coagulation factors were poor predictors of mortality and monitoring during the time could not predict poor outcome. Additionally, clotting factor tests cannot be easily undertaken in many laboratories and these analyses are very expensive. Therefore, it can be concluded that regular monitoring of these parameters is not always rational in critically ill COVID-19 patients. Consistent with the previous studies, we found reduced production, or more likely, increased consumption of clotting proteins, coagulation factors, in critically ill COVID-19 patients [32], with potential importance of factor II, factor V and factor XIII in the prediction of the mortality. A more detailed evaluation is necessary. There are limitations to this study. First, it is a single-center study and second, there is a limited number of patients, considering the size of the pandemic.
5Abbreviation
ICU, intensive care medicine; MICU, Medical Intensive Care Unit; UCC-RS, University Clinical Centre of the Republic of Srpska; ARDS, acute respiratory distress syndrome; SOFA, sequential organ failure assessment; APACHE, Acute Physiology and Chronic Health Evaluation II; SAPS II, simplified acute physiology score II; FiO2, a fraction of inspired oxygen; PEEP, positive end-expiratory pressure; ECMO, extracorporeal membrane oxygenation; CRRT, continuous renal replacement therapy; AKI, acute kidney injury; Tbil, total bilirubin; Dbil, direct bilirubin; AST, aspartate aminitransferase; ALT, alanin aminotransferase; LDH, lactic dehidrogenaase; CK, cretine cinase; Alb, albumin: Ca2+, calcium; CRP, C-reactive protein; PCT, procalcitonin; IL-6, interleukin 6; RBC, red blood count; WBC, white blood count;; Ly, absolute Lymphocyte count; Plt, platelet count; Hb, hemoglobin; Fe, iron; UIBC, unsaturated iron-binding capacity; TIBC, total iron-binding capacity; coagulation cascade factors: F II, factor II, F V, factor V, F X, factor X, F XI, factor XI, F XIII, factor XIII, vWF, von Wilebrand factor; aptt, partial thromboplasin time; INR, international normalized ratio; OR, odds ratio; CI (95%), 95% confidence interval; IQR, interquartile range; ROC, receiver operation characteristic curve; AUC, the area under the ROC curve.
Acknowledgments
The authors want to thank professor Ognjen Gajić for his invaluable contribution to the study.
References
[1] | Wu F , Zhao S , Yu B , Chen Y-M , Wang W , Song Z-G , et al. A new coronavirus associated with human respiratory disease in China. Nature [Internet] ((2020) );579: (7798):265–9. Available from: http://dx.doi.org/10.1038/s41586-020-2008-3 |
[2] | https://www.worldmeters.info./coronavirus as of 27th March 2022 |
[3] | Leentjens J , van Haaps TF , Wessels PF , Schutgens REG , Middeldorp S . COVID-19-associated coagulopathy and antithrombotic agents-lessons after 1 year. Lancet Haematol [Internet]. (2021) ;8: (7):e524–33. Available from: http://dx.doi.org/10.1016/S2352-3026(21)00105-8 |
[4] | Bilaloglu S , Aphinyanaphongs Y , Jones S , Iturrate E , Hochman J , Berger JS . Thrombosis in hospitalized patients with COVID-19 in a New York city health system. JAMA [Internet]. (2020) ;324: (8)799–801. Available from: http://dx.doi.org/10.1001/jama.2020.13372 |
[5] | Koutsiaris AG , Riri K , Boutlas S , Panagiotou TN , Kotoula M , Daniil Z , Tsironi EE . COVID-19 hemodynamic and thrombotic effect on the eye microcirculation after hospitalization: A quantitative case-control study. Clinical Hemorheology and Microcirculation. 2022;1-12. |
[6] | Oudkerk M , Büller HR , Kuijpers D , van Es N , Oudkerk SF , McLoud T , et al. Diagnosis, prevention, and treatment of thromboembolic complications in COVID- Report of the National Institute for Public Health of the Netherlands. Radiology [Internet]. (2020) ;297: (1):E216–22. Available from: http://dx.doi.org/10.1148/radiol.2020201629 |
[7] | Zhou F , Yu T , Du R , Fan G , Liu Y , Liu Z , et al. Clinical course and risk factors for mortality of adult inpatients with COVID-19 in Wuhan, China: a retrospective cohort study. Lancet [Internet]. (2020) ;395: (10229):1054–62. Available from: http://dx.doi.org/10.1016/S0140-6736(20)30566-3 |
[8] | Xing Y , Yang W , Jin Y , Wang C , Guan X . D-dimer daily continuous tendency predicts the short-term prognosis for COVID-19 independently: A retrospective study from Northeast China. Clinical Hemorheology and Microcirculation. (2021) ;79: (2):269–77. |
[9] | Rasyid A , Riyanto DL , Harris S , Kurniawan X , Mesiano M , Hidayat R , Wiyarta E . Association of coagulation factors profile with clinical outcome in patient with COVID-19 and acute stroke: A second wave cohort study. Clin Hemorheol Microcirc. 2022 Jul 16. doi: 10.3233/CH-221546. |
[10] | Rasyid A , Timan IS , Riyanto DL , Harris S , Kurniawan TM , Mesiano T , et al. Coagulation and hemorheology profile of patient with stroke and COVID-19: A case series during second wave pandemic. Clin Hemorheol Microcirc [Internet]. 2022;1-6. Available from: http://dx.doi.org/10.3233/ch-221504 |
[11] | Tang N , Li D , Wang X , Sun Z . Abnormal coagulation parameters are associated with poor prognosis in patients with novel coronavirus pneumonia. J ThrombHaemost [Internet]. (2020) ;18: (4):844–7. Available from: http://dx.doi.org/10.1111/jth.14768 |
[12] | Huang C , Wang Y , Li X , Ren L , Zhao J , Hu Y , et al. Clinical features of patients infected with novel coronavirus in Wuhan, China. Lancet [Internet]. (2020) ;395: (10223):497–506. Available from: http://dx.doi.org/10.1016/S0140-6736(20)30183-5 |
[13] | Christensen B , Favaloro EJ , Lippi G , Van Cott EM . Hematology laboratory abnormalities in patients with Coronavirus Disease (COVID-19). Semin ThrombHemost [Internet]. (2020) ;46: (7):845–9. Available from: http://dx.doi.org/10.1055/s-0040-1715458 |
[14] | Henry BM , de Oliveira MHS , Benoit S , Plebani M , Lippi G . Hematologic, biochemical and immune biomarker abnormalities associated with severe illness and mortality in coronavirus disease (COVID-19): a meta-analysis. Clin Chem Lab Med [Internet]. (2020) ;58: (7):1021–8. Available from: http://dx.doi.org/10.1515/cclm-2020-0369 |
[15] | Nugroho J , Wardhana A , Mulia EP , Maghfirah I , Rachmi DA , A’yun MQ , et al. Elevated fibrinogen and fibrin degradation product are associated with poor outcome in COVID-19 patients: A meta-analysis. Clin Hemorheol Microcirc [Internet]. (2021) ;77: (2):221–31. Available from: http://dx.doi.org/10.3233/CH-200978 |
[16] | Stefely JA , Christensen BB , Gogakos T , Cone Sullivan JK , Montgomery GG , Barranco JP , et al. Marked factor V activity elevation in severe COVID-19 is associated with venous thromboembolism. Am J Hematol [Internet]. (2020) ;95: (12):1522–30. Available from: http://dx.doi.org/10.1002/ajh.25979 |
[17] | Helms J , Tacquard C , Severac F , Leonard-Lorant I , Ohana M , Delabranche X et al. High risk of thrombosis in patients with severe SARS-CoV-2 infection: a multicenter prospective cohort study. Intensive Care Med [Internet]. (2020) ;46: (6):1089–98. Available from: http://dx.doi.org/10.1007/s00134-020-06062-x |
[18] | Lippi G , Plebani M . Laboratory abnormalities in patients with COVID-infection. Clin Chem Lab Med [Internet]. (2020) ;58: (7):1131–4. Available from: http://dx.doi.org/10.1515/cclm-2020-0198 |
[19] | Fan BE . Hematologic parameters in patients with COVID-19 infection: a reply. Am J Hematol [Internet]. (2020) ;95: (8):E215. Available from: http://dx.doi.org/10.1002/ajh.25847 |
[20] | Wu C , Chen X , Cai Y , Xia J , Zhou X , Xu S , et al. Risk factors associated with acute respiratory distress syndrome and death in patients with Coronavirus disease pneumonia in Wuhan, China. JAMA Intern Med [Internet]. (2020) ;180: (7):934–43. Available from: http://dx.doi.org/10.1001/jamainternmed.2020.0994 |
[21] | Lippi G , Plebani M , Henry BM . Thrombocytopenia is associated with severe coronavirus disease (COVID-19) infections: A meta-analysis. Clin Chim Acta [Internet]. (2020) ;506: :145–8. Available from: http://dx.doi.org/10.1016/j.cca.2020.03.022 |
[22] | Wu C , Chen X , Cai Y . Risk factors associated with acute respiratory distress syndrome and death in patients with coronavirus disease 2019 pneumonia in Wuhan, China JAMA Intern Med. 2020. China JAMA Intern. 2020; |
[23] | Gong J , Dong H , Xia Q , Huang Z , Wang D , Zhao Y , et al. Correlation analysis between disease severity and inflammation-related parameters in patients with COVID-19 pneumonia [Internet]. 2020. Available from: http://dx.doi.org/10.1101/2020.02.25.20025643 |
[24] | Ahnach M , Zbiri S , Nejjari S , Ousti F , Elkettani C . C-reactive protein as an early predictor of COVID-19 severity. J Med Biochem [Internet]. (2020) ;39: (4):500–7. Available from: http://dx.doi.org/10.5937/jomb0-27554 |
[25] | Liu W , Tao Z-W , Wang L , Yuan M-L , Liu K , Zhou L , et al. Analysis of factors associated with disease outcomes in hospitalized patients with novel coronavirus disease. Chin Med J (Engl) [Internet]. (2020) ;133: (9):1032–8. Available from: http://dx.doi.org/10.1097/cm9.0000000000000775 |
[26] | Sharifpour M , Rangaraju S , Liu M , Alabyad D , Nahab FB , Creel-Bulos CM , et al. C-Reactive protein as a prognostic indicator in hospitalized patients with COVID-19. PLoS One [Internet]. (2020) ;15: (11):e4000242. Available from: http://dx.doi.org/10.1371/journal.pone.0242400 |
[27] | Malik P , Patel U , Mehta D , Patel N , Kelkar R , Akrmah M , et al. Biomarkers and outcomes of COVID-19 hospitalisations: systematic review and meta-analysis. BMJ Evid Based Med [Internet]. (2021) ;26: (3):107–8. Available from: http://dx.doi.org/10.1136/bmjebm-2020-111536 |
[28] | Becker RC . COVID-19 update: Covid-19-associated coagulopathy. J Thromb Thrombolysis [Internet]. (2020) ;50: (1):54–67. Available from: http://dx.doi.org/10.1007/s11239-020-02134-3 |
[29] | Thachil J , Tang N , Gando S , Falanga A , Cattaneo M , Levi M , et al. ISTH interim guidance on recognition and management of coagulopathy in COVID-19. J Thromb Haemost [Internet]. (2020) ;18: (5):1023–6. Available from: http://dx.doi.org/10.1111/jth.14810 |
[30] | Thachil J , Tang N , Gando S , Falanga A , Cattaneo M , Levi M , et al. ISTH interim guidance on recognition and management of coagulopathy in COVID-19. J Thromb Haemost [Internet]. (2020) ;18: (5):1023–6. Available from: http://dx.doi.org/10.1111/jth.14810 |
[31] | Short SAP , Gupta S , Brenner SK , Hayek SS , Srivastava A , Shaefi S , et al. D-dimer and death in critically ill patients with Coronavirus disease 2019. Crit Care Med [Internet]. (2021) ;49: (5):e500–11. Available from: http://dx.doi.org/10.1097/ccm.0000000000004917 |
[32] | Lichter Y , Badelbayov T , Shalev I , Schvartz R , Szekely Y , Benisty D , et al. Low FXIII activity levels in intensive care unit hospitalized COVID-19 patients. Thromb J [Internet] (2021) ;19: (1). Available from: http://dx.doi.org/10.1186/s12959-021-00333-3 |
[33] | Ziadi A , Hachimi A , Admou B , Hazime R , Brahim I , Douirek F , et al. Lymphopenia in critically ill COVID-19 patients: A predictor factor of severity and mortality. Int J Lab Hematol [Internet] (2021) ;43: (1). Available from: http://dx.doi.org/10.1111/ijlh.13351 |