The impact of ICT characteristics on students’ learning outcomes during COVID-19 lockdown period: The crucial role of techno eustress perception
Abstract
BACKGROUND:
The COVID-19 crisis led to an unprecedented acceleration of digital learning. It pushed many institutions to abruptly switch to fully online learning modes from face-to-face learning. Prior studies show that higher IT demands can cause challenge or hindrance stressors, depending on how the digital technology characteristics are perceived by the end-user. However, there is a gap in our knowledge regarding how ICT characteristics can lead to positive stress appraisals in a remote learning environment.
OBJECTIVE:
This paper leverages the person-environment fit and technostress literature to examine how usefulness and reliability as demand-ability stressors of ICT tools can positively impact learning outcomes among remote learning students. Techno eustress perceptions are evaluated as a crucial mechanism for theorizing the positive impact.
METHODS:
We used the survey method, sampling students (N = 82) during the lockdown period to test this model.
RESULTS:
Our findings highlight the ICT characteristic of usefulness as salient in contributing to student learning outcomes as it promotes techno eustress.
CONCLUSIONS:
This is the first study to demonstrate a positive impact of ICT characteristics on student learning outcomes via techno eustress perceptions.
Anuragini Shirish is an Associate Professor at Institute Mines-Télécom Business Ecole, France. She leads the Smart BIS research team within the research lab LITEM, University of Paris-Saclay, France. Her research interests includes management of digital work, digital society and digital innovation. Her research has been published in international referred journals including European Journal of Information Systems (EJIS), Information Systems Journal (ISJ), Communications of the Association of the Information Systems (CAIS) and International Journal of Information and Management (IJIM). She has also presented her work in several premier IS and management conferences. Recently, she won the “Outstanding Educator Award” by the AIS women’s network
Nina Verstraete is an alumnus of Institut Mines Telecom Business School, Evry, France. She has undertaken her Master’s in Business Information Systems for the Digital Era in 2020. She is currently working as a SAP consultant at Accenture. Her research interests include examining the transformative capabilities of technology in organizations and educational institutions.
Olfa Chourabi Tantan is an Associate Professor at Mines-Telecom Business School. She co-coordinates a specialized major program in IS consulting since September 2014. His research interests are related to the engineering and management of information systems, educational innovations in IS (Gamification, Mooc . . .). She is the author of several articles published in national and international journals and conferences of engineering and management of information systems.
1Introduction
Digital learning environments open up new pedagogical opportunities to educational institutions. Many studies have shown that the use of these technologies in education can bring multiple benefits [1–3], such as enhanced learning outcomes, increased learner engagement, and more efficient management and organization of learning [4]. These environments impact learning positively and have long been understood as a way “to think” [5], “to represent"[7], “to communicate” [7] or “to teach” [8].
Although digital technology undoubtedly motivates learners of all ages, including younger learners [9], there is gap in the literature regarding identifying the extant drivers influencing students’ learning performance during a situation of forced remote learning, as experienced during the COVID-19 pandemic [1]. Recent editorials and a number of scholars have explicitly called for a better understanding of this virtual transition for learners during the pandemic [86]. Some prior work claims that the relationship between the use of digital learning environments and academic performance is unclear, and conflicting results are presented in the literature [10, 11]. Similarly, another study has called for the development of a nuanced understanding of the use of digital technologies in education [12]. Researchers are urged to look into ways to ameliorate the negative aspects of digital technology usage, such as technology addiction, digital distraction, or cyberbullying in order to make digital education sustainable. Some researchers posit that the learner’s appraisal of the features of a digital learning system can have a positive influence on learning [1, 13]. However, the relationship between information communication technology characteristics (1) and students’ appraisals in a digital learning environment is yet to be empirically examined. Filling this research gap will help us to better understand how human systems perceive digital learning environments that have become a norm in many educational institutions around the world.
Recent studies have also called for a more integrative and holistic understanding of digital learning environments [14]. Specifically, understanding the affordances of various technologies (both functional and non-functional), their appraisal by learners, and their impact on learning is an important avenue for further theorization [14]. In the context of both positive and negative impacts of digital technology usage, it becomes interesting to develop a holistic approach on student learning outcomes and examine the conditions leading to positive impacts of digital learning environments on students’ performance during COVID lockdowns [87]. The findings of our study offer solutions for improving the effectiveness of educational programs, products, and learning outcomes through the successful appropriation of the digital learning environment by human systems.
Past research has shown a negative impact of digital technologies on individuals but few studies have analyzed the positive appraisal of technology stressors [15], apart from one study that addresses this aspect in the educational domain [1]. We attempt to contribute to this emerging stream of literature. In order to address the research gaps identified, we pose the following research questions:
To what extent were ICT characteristics positively appraised by students during remote digital learning during the pandemic and what might the impact of this appraisal be on student learning?
We leverage person-environment fit theory to study these questions, examining the influence of common ICT features that are often considered as demand stressors, namely usefulness and reliability of technology on student remote learning outcomes [16]. The learning outcomes we study in this research are digital learning environment-enabled productivity and innovation [1, 17]. To the best of our knowledge, this paper is the first to attempt to theorize the indirect role of ICT characteristics on student learning outcomes. Our conclusions will help us to understand how to foster positive appraisals during digital learning experiences and thereby better understand how human systems can be engaged as educational institutions invest in digital transformation initiatives. In the following sections, we elaborate on our theoretical framework and hypothesis development.
2Background literature and theory
Tarafdar, Cooper, and Stich (2019) provide an excellent review of past research on techno distress situations [15]. Therefore, due to space constraints, we will not elaborate on the negative aspects of increasing IT demands here. In this paper, we focus on how and when challenge stress perceptions, also known as techno eustress perceptions, may be experienced by remote learners. In particular, we address the call to identify the technological features amenable to a positive appraisal [15]. Positive appraisals are humanistic intermediary outcomes that can lead to instrumental learning goals. Past research has looked at the relationship between the technological characteristics of ICTs and stressors. Ayyagiri, Grover, and Purvis (2011) has identified three categories of ICT characteristics viewed as antecedents for techno distress perception among end-users: usability features, dynamic features, and intrusive features [16]. Usability features are those characteristics related to the use and adoption of ICTs, including usefulness, reliability, and complexity. Dynamic features are those characteristics related to the pace of change and refer to the way in which ICTs are constantly evolving. Intrusive features refer to the invasiveness of ICTs, and include anonymity and presenteeism. Technology characteristics can influence the person-technology gap by manipulating the individual’s ability to deal with the demands of ICTs. They can also generate supply attributes that contradict the individual’s values, preferences, needs, or expectations. Usability features enhance an individual’s capabilities for dealing with technological challenges and results in reducing strain [11].
Based on person-environment fit theory, this paper argues that usability features, unlike dynamic and intrusive features, can contribute to creating a better fit between the learner and the digital learning environment, as they are viewed as demand-enhancing stressors. Thus, in this study we have incorporated two of the demand-enhancing stressors from the usability features, namely the usefulness characteristics of the ICT tools and the reliability characteristics of the ICT tools as antecedents to techno eustress perceptions.
We will now elaborate on our selected theoretical framework, the Person-Environment (P-E) Fit model. This model is widely used in stress research [18–20]. The P-E Fit model [21–23] defines stress as “a negative discrepancy between an individual’s perceived situation and desired state, provided that this imbalance is significant for the individual” [20] p. 242. The central hypothesis of this model is that a misfit between a person and their environment can produce psychological, physiological, and behavioral strains, e.g. irritation, dissatisfaction, boredom, anxiety, depression, whereas fitness perception should lead to positive cognitive and behavioral outcomes.
This model is based on the principle of a balance between people and their environment. When this balance is upset, it results in strain. The P-E Fit model explains the state of stress as the result of a mismatch between the demands and rewards of the work environment and the expectations and abilities of the people. P-E Fit can take two forms: either the environment provides the rewards and resources to meet the individual’s needs and preferences, or the individual’s skills, abilities, and aptitudes are adapted to the requirements and constraints of the job or position [23]. According to this model, a person-environment imbalance threatens well-being. In response to this tension-inducing imbalance, the individual can implement a range of adjustment strategies. If these adjustment behaviours are ineffective, the additive effects of stress can lead to problems in the long term, such as work dissatisfaction and disturbances in physical and mental health.
Selye has identified two types of responses to stress namely distress and eustress [24]. Distress is the negative outcome of all forms of stressors, physical or psychological, that exceed an individual’s capabilities. Eustress is the positive reactions an individual adopts when exposed to a stressor. Recently, by introducing challenge techno-stressors and challenge appraisal into the technostress literature, researchers have claimed that an individual’s use of ICTs can enhance and improve their skills by pushing them to learn how to use them and by improving their flexibility in different contexts [25, 26].
Further, ICT characteristics can introduce changes that can be perceived as a challenge, i.e. the opportunity to enhance skills, tasks, and work-life activities leading to positive outcomes, or as a threat, subjecting the individual to pressure and an inability to carry out the task leading to negative outcomes [15]. We can therefore perceive certain ICT characteristics as an opportunity in some contexts to enhance flexibility and productivity by enabling users to access and process information [26, 27]. The organizational literature suggests that types of stressors such as time pressure and job control are perceived as challenge stressors and they give rise to challenge appraisal, which is then linked to daily creativity and proactive behaviour [28]. Using a similar logic, we posit that certain ICT characteristics can be perceived as challenge stressors giving rise to challenge appraisals.
2.1Digital learning environments and student learning performance
The COVID-19 lockdowns of 2020-21 gave new urgency to the need to switch from a fully face-to-face mode of learning to online learning environments. These new digital environments open up possibilities for the creation of new learning opportunities for both on-campus and online learners. There is therefore a need to understand the impact of students’ use of and their assessment of digital learning environments on their learning outcomes.
Prior research has looked at stress appraisals and mental health among higher education students populations. Higher education students face several types of stressors: their ongoing academic studies, financial worries, balancing their personal life and university work, potential lack of support and feedback, competition between peers, being evaluated by their supervisors [29], the fear of having made a mistake in their choice of subject [30], academic deadlines [31], having to alternate between different roles, such as tutoring research, taking part in seminars, acting as an assistant, and being supervised [29]. Depending on the intensity, frequency, duration, and students’ subjective interpretation of these stressors, a range of affects can occur. The consequences can be academic burnout [32], decreasing physical health [33], decreasing academic performance, increased plagiarism, increasing errors in professional practice, decreased empathy, increasing dropout levels [34], or development of symptoms of anxiety or depression [35]. We provide specific information on research studies into digital learning during the pandemic in Table 1 below.
Table 1
Recent research on digital learning environments and positioning of this research
Recent research perspectives concerning digital learning during the COVID-19 pandemic | Sources |
Perspectives of teachers, teaching structure, and teaching effectiveness | [42–46] |
Institutional resources, policies, and IT tool adoption | [47–54] |
Learner-centric responsibility for effective learning | [1, 55, 56] |
Negative emotional impacts on learners | [45, 54, 57] |
Stressors, learner traits, techno eustress, and holistic learning | [1] |
Role of demand stressors (ICT characteristics) on techno eustress and holistic learning | This study |
To the best of our knowledge, only one study has addressed techno eustress and its impact on students’ performance in digital learning environments [1]. One research stream shows that digital learning environments do not play a key role in students’ performance in higher education [36, 37]. Another demonstrates that digital learning environments have positive impacts on students’ achievement [38, 39]. On the other hand, some studies show that students score higher in a traditional class format than in an online format [40]. Digital learning environments are closely related to self-regulatory learning, making learner-centric learning a key factor in our understanding of the success of such environments [41]. From [1] we can see that when students are IT mindful, they adapt positively to stressors in digital learning environments, leading to enhanced academic creativity and performance. However, we need more research that helps us understand the impact of technological characteristics on positive appraisal and student learning and learning processes [41].
Thus, this research paper aims to examine the role of IT characteristics in digital learning environments and the subjective assessment or challenge appraisal of IT characteristics by students during the lockdown period in France. We believe that a positive perception of technological characteristics will lead to better student outcomes. Indeed, digital learning technologies, which are increasingly valued by students and integrated into higher education, represent a major challenge. It is therefore necessary to understand the context in which they are effective for students so that educational institutions can be better informed about levers for improvement. They will then be in a position to address the inhibitors of performance improvement and put in place measures (human, technical, material, or pedagogical) to strengthen their effectiveness.
3Hypothesis development
In this section, we explain how digital learning environments and their usability features can reduce the misfit described in the Person-Environment Fit model that is shown to cause stress among students. Reducing the gaps will lessen stress levels and will produce greater productivity and innovation in learning. We chose to focus on two learning outcomes –productivity and innovation in learning –as they offer a holistic examination of learning. Our research examines both task-focused (ICT-enabled productivity) and learning-focused outcomes (ICT-enabled innovation in learning process), which allows us to measure them from a learner’s perspective [58]. These two outcomes are also salient student outcomes when it comes to the use of technology for non-routine tasks [17, 59]. Figure 1 presents our research model with corresponding hypotheses, which will be further developed in this section.
Fig. 1
Research Model.
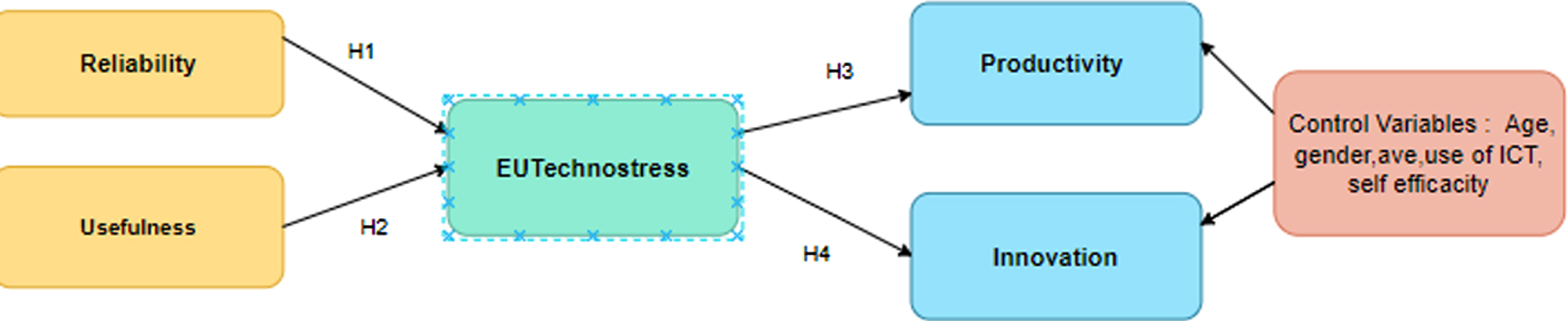
The technostress literature associates three ICT characteristics with ICT use and adoption: usefulness, complexity, and reliability. A lack of choice in the use and adoption of technologies at work [60] is common and is intrinsically linked to the characteristics and requirements of the job position [40, 61]. Despite having a low perception of their usability features, individuals would still have to use ICTs due to the requirements of their particular job. This contributes to a feeling of having to work harder [62]. One study suggests that using technologies for compliance reasons rather than through voluntary adoption creates additional conflicts, which result in more stress [63]. However, reliability and usability features increase the demand perceptions of users rather than contributing to supply-value incongruence [16].
Information systems are often evaluated according to their reliability and on how dependable and consistent the system is [64–66]. However, ICTs are becoming increasingly more complex and their reliability is difficult to ensure [67]. Reliability problems include software errors, quality problems, and failures [68–70]. This unreliability creates frustration and strain among users [62]. Therefore, from a P-E Fit model perspective, we suggest that reliable technologies would reduce the perceived workload and instead create demand ability fit perceptions; thereby triggering techno eustress, whereas unreliable systems will lead to strain due to the additional efforts required should the system break down. Thus, we hypothesize that:
H1: Reliability is positively associated with techno eustress perceptions
According to the P-E Fit model [16], the usability characteristics of ICTs enhance users’ ability to work better and lower the perceived demand ability gap. The more useful users perceive technologies to be, the more they will be able to use them to improve their performance. The individual will therefore perceive the use of technologies as a means of increasing their ability to cope with their workload. Reducing the perceived workload will reduce the misfit and lead to techno eustress. A recent study has also shown that usefulness perception reduces perceptions of technology spatial intrusion that end-users may feel in a remote working situation [10]. Thus, we hypothesize that:
H2: Usefulness is positively associated with techno eustress perceptions
When faced with technostress creators, the first step is to appraise whether the use of ICTs is useful, challenging, and reliable to users –in our case, students. The second step is to devise a suitable response by assessing the coping resources, options, and efforts needed to manage the technostress creators. In this context, individuals use ICTs to reduce their workload and their stress. Usability features will enable users to work better by reducing their perception of their workload and increasing their ability to cope with new technological challenges. We suggest it will impact positively on their productivity.
H3: Techno eustress is positively associated with students’ productivity
Following the same line of argument for the three prior hypotheses, and based on challenge appraisal, we posit that the use of ICTs will appear as challenging, as individuals will perceive them as a means of increasing their abilities and improving their competences to become more flexible, autonomous, and productive. The perception of ICTs’ usefulness will play a key role in the adoption of digital learning environments by students, thereby increasing their performance in learning tasks and contributing to innovation in learning process. Prior studies have also shown that digital technologies may be appraised as a challenge and contribute to creativity and innovation processes and outcomes [59]. Thus, we hypothesize that:
H4: Techno eustress is positively associated with students’ innovation
4Methodology
We adopted a two-wave survey method to collect data and test our hypotheses. The initial questionnaires were sent to approximately 500 students, who were students from a French business school. Respondents were contacted via their academic email addresses and through the school’s Facebook group. The first survey was sent in early March 2020 and stayed open for two weeks; the second was sent in late April 2020 and also stayed open for two weeks. During this period of time, students were exclusively taught via remote learning and were not allowed to visit the campus. The first phase consisted of collecting data on ICT characteristics, while the second aimed to collect data on techno eustress and the dependent variables of student innovation and productivity. After screening both sets of data collected during the two phases, we found a total of 82 usable responses, 75% of which came from female students with 25% from male students. The distribution regarding the year of study was: 49% of first to third-year students, 22% of fourth-year students, and 29% of fifth-year students. Working students made up 25% of the respondents. Students on average had three years of experience with ICTs and on average used ICTs for six to eight hours per day. We also incorporated suitable control variables, as shown in the research model (Fig. 1). For example, self-efficacy is known to contribute to learning outcomes that occur via ICT tools [1], hence we have incorporated it in our research model to isolate the potential influence of independent and mediator variables on our learning outcomes. Table 2 provides details of the scales used in this study.
Table 2
Key Variables
Self-efficacy [71] |
I could complete a job or task using ICT . . . |
... if there is no one around to tell me what to do as I go (SEFC1) |
... if I could call someone for help. (SEFC2) |
. . . if I have a lot of time to carry out the task for which ICTs are provided (SEFC3) |
...if I have the built-in help facility for assistance (SEFC4) |
Techno Eustress [1] [72] |
ICT use helps me to fulfill the demands of my schoolwork (university) so I can improve my personal growth and well-being (TEU1) |
I feel ICT use helps me to address the demands of my school (university) challenges so I can achieve personal goals and accomplishment (TEU2) |
In general, I feel that ICT use for my school (university) work promotes my personal accomplishments (TEU3) |
ICT-enabled productivity [1][73] |
The ICTs I use for school related work help me to . . . ... |
.... improve the quality of my work (PROD1) |
.... improve my productivity (PROD2) |
.... accomplish more work than would otherwise be possible (PROD3) |
.... to perform my job better (PROD4) |
ICT-enabled innovation [1][73] |
The ICTs I use for school (university) related work help me to . . . |
. . . identify innovative ways of doing my school (university) work.. (INNO1) |
. . . come up with new ideas relating to my school (university) work (INNO2) |
. . . try out innovative ideas (INNO3) |
Reliability [64] |
The features provided by ICTs are dependable (RELI1) |
The capabilities provided by ICTs are reliable (RELI2) |
ICTs behave highly consistently (RELI3) |
Usefulness [74] |
Use of ICTs enables me to accomplish tasks more quickly (USEF1) |
Use of ICTs improves the quality of my work (USEF2) |
Use of ICTs makes it easier to do my job (USEF3) |
Use of ICTs enhances my effectiveness on the job (USEF4) |
As the sample size of this study was 82, we checked the smallest acceptable sample size to detect the effect of regression analysis at p < 0.05 using G*Power [75]. The desired power level is typically 0.80, but the researcher performing power analysis can specify a higher level, such as 0.90. Our study’s power analysis results revealed a smallest acceptable sample size of 74 for a power level of 0.95, so we can be reasonably sure, with a 95% probability, of not committing a type II error in our research with the given sample size. As seen in Fig. 2, the G power plot gives us the confidence that our sample size is sufficient to offer significant results.
Fig. 2
Power Analysis.
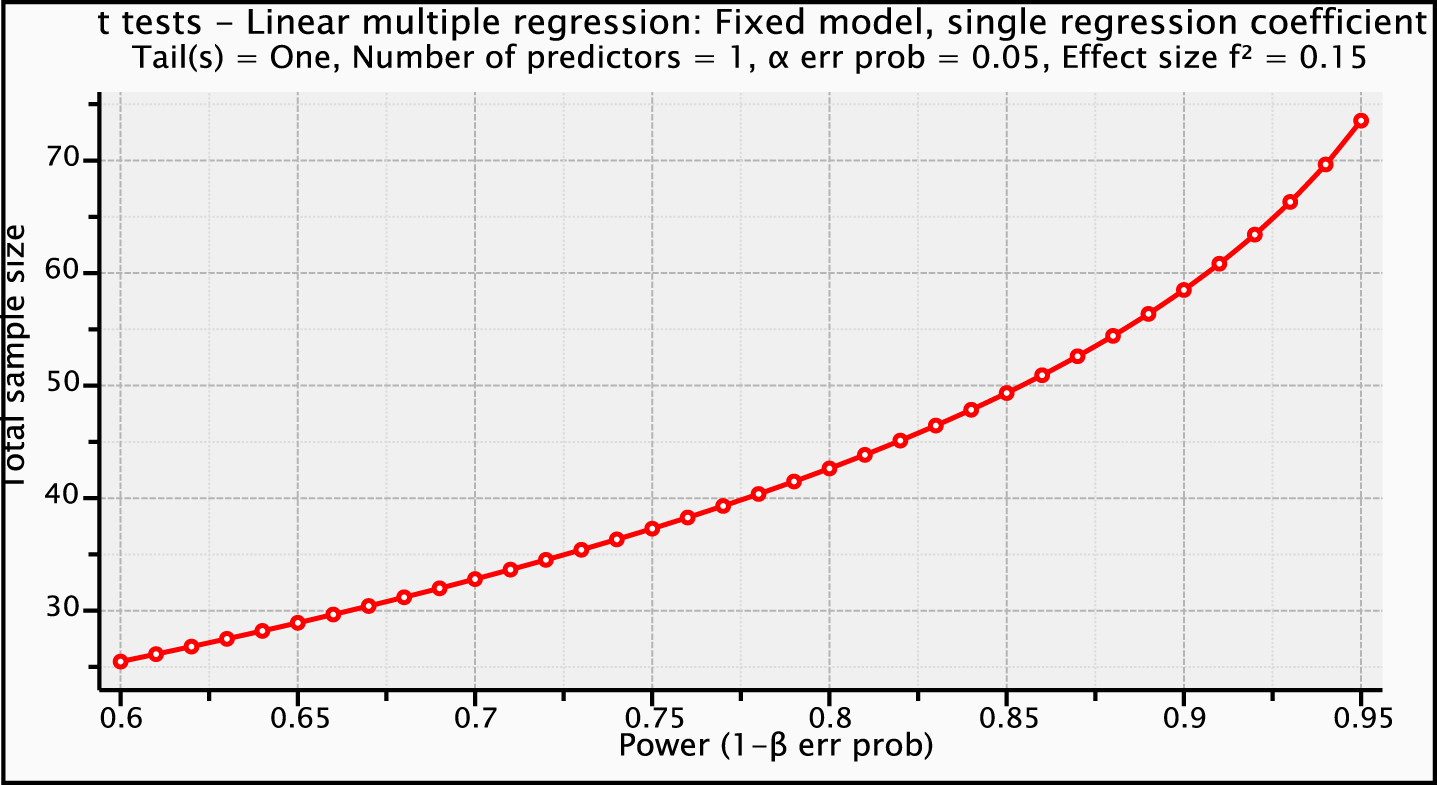
5Results and discussion
5.1Measurement model assessment
To conduct this study, we used Partial Least Squares Structural Equation Modelling (PLS-SEM) [76]. Several IS studies have used the PLS technique and have proved PLS to be an effective method for data analysis [59, 77, 78]. PLS software is known to be appropriate for smaller sample sizes. Moreover, as per [88] PLS allows us to check whether our sample size is adequate depending on the maximum number of arrows pointing at a latent variable as specified in a given structural equation model. These authors recommend that for a sample size of 80 the maximum number of arrows pointing to a latent variable should be 7. In our case, the maximum number of arrows pointing towards our final dependant variable is only 5. Given that our sample size is adequate for the purposes of the PLS method, we went ahead with this method.
We adopted a two-step approach for our analysis [76, 79]. The first step refers to the analysis of the measurement model while the second step tests the structural relationships between the different constructs. For the measurement model, we tested for three types of validity: content validity, convergent validity, and discriminant validity. The values of the square roots of the Average Variance Extracted (see Table 3) are all above the related inter construct correlations, showing a satisfying discriminant validity. The items’ cross loading values were low, showing discriminant validity. Table 4 provides construct reliability and validity measures. Since the measurement model was satisfactory, we proceeded to test the research model using structural model testing.
Table 3
Fornell and Larcker Criterion
Age | TEU | Gender | ICT use | Innovation | Productivity | Reliability | Self efficacy | Usefulness | |
Age | 1.000 | ||||||||
TEU | 0.204 | 0.876 | |||||||
Gender | –0.041 | 0.107 | 1.000 | ||||||
ICT use | 0.085 | 0.139 | –0.012 | 1.000 | |||||
Innovation | –0.068 | 0.622 | 0.012 | 0.264 | 0.904 | ||||
Productivity | 0.080 | 0.621 | 0.028 | 0.231 | 0.570 | 0.818 | |||
Reliability | 0.198 | 0.301 | –0.133 | 0.096 | 0.211 | 0.316 | 0.825 | ||
Self efficacy | 0.104 | 0.262 | 0.086 | 0.079 | 0.274 | 0.515 | 0.077 | 0.735 | |
Usefulness | 0.151 | 0.398 | 0.028 | 0.097 | 0.255 | 0.444 | 0.491 | 0.188 | 0.807 |
Notes: TEU refers to techno eustress.
Table 4
Construct Reliability and Validity
Cronbach’s Alpha | rho_A | Composite Reliability | Average Variance Extracted | |
Age | 1,000 | 1,000 | 1,000 | 1,000 |
Technoeustress | 0,848 | 0,851 | 0.908 | 0,767 |
Gender | 1,000 | 1,000 | 1,000 | 1,000 |
ICT use | 1,000 | 1,000 | 1,000 | 1,000 |
Innovation | 0,888 | 0,888 | 0,930 | 0,817 |
Productivity | 0,835 | 0,838 | 0,890 | 0,669 |
Reliability | 0,773 | 0,797 | 0,864 | 0,680 |
Self-efficacy | 0,707 | 0,732 | 0,821 | 0,540 |
Usefulness | 0,821 | 0,832 | 0,881 | 0,651 |
As a robustness test, we performed the Stone and Geisser Q2 test using the blindfolding procedure to evaluate the predictive relevance of the structural model [2, 3]. The blindfolding test, which was conducted with omission distance equal to 7 (the recommended number), revealed that all Q2 values of the endogenous variables were greater than zero with the two dependent variables. Positive Q2 values provide evidence for the predictive relevance of our theorized research model.
5.2Findings: Structural model assessment
Table 5 presents the results of our structural model with productivity and innovation as student learning
Table 5
Structural Model with Innovation and Productivity Performance
TEU | Productivity | Innovation | ||||
Control Variables | β | t | B | t | β | T |
Age | –0.08 | 1.06 | –0.23** | 2.70 | ||
Gender | –0.06 | 0.82 | –0.07 | 0.87 | ||
Self-Efficacy | 0.38** | 4.66 | 0.13 | 1.18 | ||
ICT use | 0.13** | 2.20 | 0.19** | 2.86 | ||
Independent Variables | ||||||
Reliability | 0.14 | 1.15 | ||||
Usefulness | 0.33** | 2.8 | ||||
Mediator Variable | ||||||
Techno eustress | 0.53** | 6.06 | 0.62** | 7.18 | ||
R square value | 0.17 | 0.48 | 0.55 |
Notes: *p < 0.05, **p < 0.01, N = 82 participants.
6Implications
In this section we provide the implications stemming from our work both for theory and practice respectively.
6.1Theoretical implications
Our study offers several theoretical implications. First, our study addresses calls to explore the role of learners’ perception, more specifically in fostering productivity and innovation in digital learning environments [89, 90]. Although prior research has shown the salience of specific technological and course design elements as well as individual differences in technology-mediated learning outcomes [1, 91], such studies have not focused on how technology features may reduce the negative impact of demand-ability misfit perceptions of learners on their learning outcomes. It is very important to also look at how digital learning technologies can alleviate or enhance the use of digital learning technologies. Our work sheds light on this salient research agenda and is one of the first studies to demonstrate a positive impact of ICT characteristics on student learning outcomes in a pandemic lockdown context.
Specifically, we note that ICT characteristics have been studied as antecedents to techno distress perceptions [16]. Digital learning environments can be a source of stress that is detrimental to well-being but they can also be a source of enhanced learner performance. Thus, our study extends recent prior research that showed how positive student traits such as IT mindfulness created an antecedent for student learning outcomes in the context of remote learning during a pandemic via techno eustress [1, 91]. We are able to now show that the usability characteristics of digital learning tools can also be an antecedent to techno eustress perceptions. Our study provides a theoretical framework for an understanding of the technological antecedents of techno eustress and contributes to this under-studied phenomenon [15]. In our study, we show that techno eustress or challenge appraisals can reduce the misfit perceptions between digital technologies and learners and they can also enhance fitness perceptions between digital technologies and learners.
Second, our study is one of the few IS studies to theorize the role of ICT characteristics as a means of alleviating the proven negative stress responses among students using ICTs for work [92, 93]. This has allowed us to narrow down the usability characteristics of technology to one pivotal feature, i.e., the “usefulness” feature in the context of digital learning. Hence, we contribute to both the technostress and digital learning literature [1, 15, 28, 80, 81]. The usefulness feature enhances techno eustress within the student population in a remote learning situation. Our findings demonstrate that students who perceive digital learning environments and tools to be useful are better prepared to understand and adapt to stressful situations arising from the enhanced IT demands placed on them during the sudden transition to a virtual mode. Our research paves the way for future studies into person-environment fitness perceptions as essential to enhancing the well-being of students in a digital learning context.
Third, a recent review paper on technostress highlighted that IS literature is essentially skewed towards examining distress perceptions with a limited focus on appraising the demands from the technology environment as challenging or motivating [94]. Interestingly, very few IS papers discuss the positive side of IT stressors [17, 81]. Our study adds to this noteworthy stream of research. These findings can be examined alongside other person-specific variables, such as cognitive absorption and self-efficacy in fostering positive stress perceptions while using technology for learning and work [17].
Fourth, this study looks at digital learning from a holistic perspective, as we study person-environmental fitness perceptions and also consider both productivity and innovation in the learning process as learning outcomes. Hence, we contribute to the educational technology literature in general [1, 12–14, 41, 84, 85]. Moreover, the findings of our study extend previous studies that have looked at creativity [1, 28, 80, 81], flexibility [26, 27] and productivity [1] in the technostress literature. Our study also extends the online student performance literature by identifying technological determinants [82, 83] as well as contributing to our understanding of the use of digital technologies in the educational context.
The cumulative building of knowledge about the use of digital learning tools for education purposes by end-users such as learners will further enable educational institutions to plan better digital transition policies and usage practices. It could also enable designers to design digital learning environments that take into account the features that best contribute to a positive experience for learners, including techno eustress perceptions.
6.2Practical implications
In addition to the aforementioned theoretical implications, our study has a number of practical implications. The results of our study provide clear directions for educational institutions regarding the provision of technological infrastructure while allowing them to think about the features of the technology that can be effectively introduced and leveraged from the perspective of learners or other end-users. We believe that these findings have implications for all educational businesses and organizations undergoing rapid digital transformation due to the coronavirus pandemic. Appropriate multi-pronged efforts are required to communicate to end-users to what extent usability features are embedded into digital learning environments and tools so they can appraise these technologies in a positive light, thereby contributing to their effective use. We believe that the usefulness features highlighted in our study could counteract the stressful situations that students often face in fully remote learning settings. In sum, by taking onboard the person-environment fitness perceptions specified in this study practitioners and pedagogical engineers could hone the signaling strategies and design of learning technologies. Such signaling and communication would help learners to appropriate these tools both effectively and positively and would also increase their productivity in learning as well as their innovation outcomes.
7Limitations and future research directions
We acknowledge that our study has several limitations. However, we believe that these limitations provide interesting avenues for future research. First, the COVID-19 pandemic has emphasized the relevance of the IT and digital transformation in all areas of business as well as in our personal lives. However, our study focuses only on the education sector, where there is an ongoing debate about how to increase 21st-century students’ productivity and innovation in learning through IT [95–97]. Future studies could theorize and examine other settings undergoing massive digital transformation because of COVID-19-induced changes. Replication and extension of the current model in non-academic settings that involve digital workforce transformation would help to increase the generalizability of our research findings.
Second, our study uses a two-wave survey method. While collecting the data in two phases mitigates methodological biases, researchers could consider using an experimental method to produce conclusive evidence for the causal impact of perceptions of usability features on learning outcomes. In a similar vein, qualitative studies on the subject could help us to better understand the nuanced processes through which usability features influence learning outcomes.
Third, we tested the theorized model via a unique data set collected during the first pandemic-driven lockdown period (from March to May 2020) in France. However, our sample size was relatively small. This was primarily because the study was designed as a two-wave matched pair survey conducted during a strict COVID-19-induced lockdown period in France. At that time, many students were forced to make an abrupt first time switch to different online learning modes. However, a G Power plot analysis shows that our study had sufficient power to establish conclusive results. Future researchers could conduct similar studies with a larger number of respondents, perhaps from different cultures and countries.
Fourth, in this study, we examined two generic usability features of the digital learning tools, i.e. usefulness and reliability. Examining the role of complexity characterized as a usability feature is a fertile avenue for future research. In addition, issues related to student engagement, mental health conditions (such as depression), and other academic performance outcomes have gained salience, especially among college students [98]. Examining these novel outcome variables could provide interesting directions for future work.
8Conclusion
The need to better understand the impact of the imposition of digital learning technologies on students during and since the pandemic of 2020/21 is of utmost importance to researchers, pedagogical engineers, practitioners, and education policy makers. Research has made great strides in understanding broader technology design in terms of the selection of collaborative tools such as Zoom and Moodle platforms to quickly navigate the switch to online learning that became necessary during the COVID-19 pandemic. Studies report both negative and positive impacts of digital learning environments on teachers and learners. None of the studies has tried to undertake a nuanced investigation of the impact of technology features perception by learners using various digital learning environments. The theorization undertaken in this study has enabled us to understand under which conditions learners adopt digital learning platforms. Moreover, we are able to better understand how to design tools that generate positive learning outcomes. Since the forced transition has put the onus of remote learning on the student, it is important to study their person-technology fitness perceptions so we can understand how they cope with digital learning tool features that cause demand ability stressors so that they can be productive and creative during the learning process.
Our research provides a novel contribution to theory regarding ICT characteristics as an antecedent to techno eustress perceptions. Our study is one of the first to link ICT characteristics to learners’ learning outcomes via techno eustress perceptions. The ICT characteristic that causes the best person-environment fitness perceptions is the “usability” feature. This study demonstrates that certain usability characteristics, i.e. usefulness of the digital tool, are viewed positively by learners, which then leads to techno eustress perceptions. These techno eustress perceptions propel them to use the digital learning tool effectively for both productive and creative learning processes. Communicating the usefulness of digital learning tools to learners can provide humanistic intermediator outcomes of techno eustress perceptions and can lead to instrumental goals, such as higher productivity in learning and more innovative learning processes. We encourage practitioners and policy makers to incorporate our findings in their design and communication strategies.
Acknowledgments
The authors would like to thank the students who took part in the survey during the pandemic times.
Author contributions
CONCEPTION: Anuragini Shirish
METHODOLOGY: Anuragini Shirish and Nina Verstraete
DATA COLLECTION: Anuragini Shirish and Nina Verstraete
INTERPRETATION OR ANALYSIS OF DATA: Anuragini Shirish and Nina Verstraete
PREPARATION OF THE MANUSCRIPT: Anuragini Shirish, Nina Verstraete and Olfa Chourabi Tantan
REVISION FOR IMPORTANT INTELLECTUAL CONTENT: Anuragini Shirish and Olfa Chourabi Tantan
SUPERVISION: Anuragini Shirish
References
[1] | Shirish A , Chandra S , Srivastava SC , Switching to online learning during COVID- Theorizing the role of IT mindfulness and techno eustress for facilitating productivity and creativity in student learning, International Journal of Information Management.94 (2021) ;61: :102394. DOI: 10.1016/j.ijinfomgt.2021.102394. |
[2] | Agrawal R , Wankhede VA , Nair RS , Analysis of drivers of digital learning in COVID-19 and Post-COVID-19 scenario using an ISM approach, Journal of the Institution of Engineers. Series B (2021) ;(4):1–13. DOI: 10.1007/s40031-020-00528-8. |
[3] | Lin MH , Chen HG , A study of the effects of digital learning on learning motivation and learning outcome, Eurasia Journal of Mathematics, Science and Technology Education (2017) ;13: (7):3553–64. |
[4] | Perrotta C , Do school-level factors influence the educational benefits of digital technology? A critical analysisof teachers’ perceptions, British Journal of Educational Technology (2013) ;44: (2):314–27. |
[5] | Arsac J Les machines à penser, Paris: Éditions du Seuil 1987. |
[6] | Linard M , Les TIC en éducation: un pont possible entre faire et dire Les Jeunes et les médias. Hachette 2000151. |
[7] | Perriault J , La logique de l’usage, essai sur les machines à communiquer, Éditions L’Harmattan. 2008. |
[8] | Bruillard E , Les machines à enseigner, Paris: Hermès 1997. |
[9] | Warschauer M , The paradoxical future of digital learning, Learning Inquiry (2007) ;1: (5):41–49. DOI: org/10.1007/s11519-007-0001-5. |
[10] | Chandra Y Online education during COVID- perception of academic stress and emotional intelligence coping strategies among college students, Asian Education and Development Studies (2020) ;10: (2):229–38. DOI: 10.1108/AEDS-05-2020-0097. |
[11] | Hasan N , Bao Y , Impact of e-Learning crack-up perception on psychological distress among college students during COVID-19 pandemic: A mediating role of fear of academic year loss, Children and Youth Services Review (2020) ;118: :105355. DOI: 10.1016/j.childyouth.2020.105355. |
[12] | Selwyn N , Aagaard J . Banning mobile phones from classrooms— An opportunity to advance understandings of technology addiction, distraction and cyberbullying, British Journal of Educational Technology (2021) ;52: (5):8–19. DOI: org/10.1111/bjet.12943. |
[13] | Kemp A , Palmer E , Strelan P , A taxonomy of factors affecting attitudes towards educational technologies for use with technology acceptance models, British Journal of Educational Technology (2019) ;50: (5):2394–413. |
[14] | Bower M , Technology-mediated learning theory, British Journal of Educational Technology (2019) ;50: (3):1035–48. |
[15] | Tarafdar M , Cooper CL , Stich JF , The technostress trifecta-techno eustress, techno distress and design: Theoretical directions and an agenda for research, Information Systems Journal (2019) ;29: (5):6–42. |
[16] | Ayyagari R , Grover V , Purvis R , Technostress: Technological antecedents and implications, MIS Quarterly (2011) ;35: (5):"831–58. |
[17] | Chandra S , Shirish A , Srivastava SC , Does technostress inhibit employee innovation? Examining the linear and curvilinear influence of technostress creators, Communications of the Association for Information Systems (2019) ;44: (5):1–19. |
[18] | Cooper CL , Cooper CP , Dewe PJ , O’Driscoll MP , O’Driscoll DM Organizational stress:Areviewand critique of theory, research, and applications. Sage. 2001. |
[19] | Edwards JR . Person-job fit: A conceptual integration, literature review, and methodological critique. International Review of Industrial and Organizational Psychology. (1991) ;6: , 283–357. |
[20] | Edwards JR , Cooper CL , The impacts of positive psychological states on physical health: A review and theoretical framework, Social Science & Medicine (1988) ;27: (12):1447–59. |
[21] | French JRP , Rodgers W , Cobb S Adjustment as a Person Environment fit. In G.V. Coelho, D.A. Hanburg, & J.F. Adams. Coping and Adaptation: Interdisciplinary perspectives, Basic Books. 1974. |
[22] | Ivancevich JM , Matteson MT Stress and work: A managerial perspective. Pearson Scott Foresman. 1980. |
[23] | Edwards JR , Caplan RD , Van Harrison R Person-environment fit theory, Theories of Organizational Stress (1998) ;28: (5):67–94. |
[24] | Selye H Stress and the general adaptation syndrome. BMJ. (1950) ;1: (4667):1383–92. DOI: 10.1136/bmj.1.4667.1383. |
[25] | Diaz I , Chiaburu DS , Zimmerman RD , Boswell WR , Communication technology: Pros and cons of constant connection to work, Journal of Vocational Behavior (2012) ;80: (2):500–8. |
[26] | Ohly S , Latour A , Work-related smartphone use and well-being in the evening, Journal of Personnel Psychology (2014) ;13: (5):174–83. |
[27] | Boswell WR , Olson-Buchanan JB , The use of communication technologies after hours: The role of work attitudes and work-life conflict, Journal of Management (2007) ;33: (5):592–610. |
[28] | Ohly S , Fritz C , Work characteristics, challenge appraisal, creativity, and proactive behavior: A multi-level study, Journal of Organizational Behavior (2010) ;31: (5):543–65. |
[29] | Schwartz-Mette RA , Challenges in adressing graduate student impairment in academic professional psychology programs, Ethics & Behavior (2009) ;19: (2):91–102. DOI: 10.1080/10508420902768973. |
[30] | Grignon C , Gruel L , Bensoussan B , Les conditions de vie des étudiants (pp 1-175), La Documentation Française. Paris: Observatoire de la vie étudiante 1996. |
[31] | Nelson NG , Dell’Oliver C , Koch C , Buckler R Stress, coping, and success among graduate students in clinical psychology, Psychological Reports (2001) ;88: (3):759–67. |
[32] | May RW , Bauer KN , Fincham FD , School burnout: Diminished academic and cognitive performance, Learning and Individual Differences (2015) ;42: :126–31. |
[33] | Watson R , Deary I , Thompson D , Li G , A study of stress and burnout in nursing students in Hong Kong: a questionnaire survey, International Journal of Nursing Studies (2008) ;45: (10):1534–42. |
[34] | Dyrbye LN , Thomas MR , Power DV , Durning S , Moutier C , Massie FS Jr , Shanafelt TD , Burnout and serious thoughts of dropping out of medical school: a multi-institutional study, Academic Medicine (2010) ;85: (5):94–102. |
[35] | Ogden J , Mtandabari T , Examination stress and changes in mood and health related behaviours, Psychology and Health (1997) ;12: (2):289–99. |
[36] | Angrist J , Lavy V . New evidence on classroom computers and pupil learning, The Economic Journal (2002) ;112: (482):735–65. DOI: 10.1111/1468-0297.00068. |
[37] | Banerjee AV , Cole S , Duflo E , Linden L , Remedying education: Evidence from two randomized experiments in India, The Quarterly Journal of Economics (2007) ;122: (3):1235–64. |
[38] | Sosin K , Lecha BJ , Agarwal R , Bartlett RL , Daniel JI ,. Efficiency in the use of technology in economic education: Some preliminary results, American Economic Review (2004) ;94: (2):253–8. |
[39] | Coates D , Humphreys BR , Kane J , Vachris MA , No significant distance between face-to-face and online instruction: Evidence from principles of economics, Economics of Education Review (2004) ;23: (5):533–46. |
[40] | Brown SA , Massey AP , Montoya-Weiss MM , Burkman JR , Do I really have to? User acceptance of mandated technology, European Journal of Information Systems (2002) ;11: (5):283–95. |
[41] | Hooshyar D , Pedaste M , Saks K , Leijen Ä , Bardone E , Wang M , Open learner models in supporting self-regulated learning in higher education: A systematic literature review, Computers & Education (2020) ;154: :103878. DOI: 10.1016/j.compedu.2020.103878. |
[42] | Abcouwer T , Takacs E , Solymosy J , Fine-tuning the evaluation focus in the university cooperative learning model in relation to the pandemic, Communications of the Association for Information Systems (2021) ;48: (5):196–204. |
[43] | Alqabbani S , Almuwais A , Benajiba N , Almoayad F , Readiness towards emergency shifting to remote learning during COVID-19 pandemic among university instructors, E Learning and Digital Media (2020) ;18: (5):460–79. |
[44] | Boor I , Cornelisse S , How to encourage online self-regulation of students, Communications of the Association for Information Systems (2021) ;48: (5):1–27. |
[45] | Carter RA Jr , Rice M , Yang S , Jackson HA , Self-regulated learning in online learning environments: Strategies for remote learning, Information and Learning Sciences (2020) ;121: (6):321–9. |
[46] | Prinsloo T , Singh P , COVID- Leapfrogging 8,000 students from face-to-face to online learning in three weeks, Communications of the Association for Information Systems (2021) ;48: (5):1–9. |
[47] | Barnes SJ , Information management research and practice in the post-COVID-19 world, International Journal of Information Management (2020) ;55: :102175. DOI: 10.1016/j.ijinfomgt.2020.102175. |
[48] | Favale T , Soro F , Trevisan M , Drago I , Mellia M , Campus traffic and E-LEarning during COVID-19 pandemic, Computer Networks (2020) ;176: :107290. DOI: 10.1016/j.comnet.2020.107290. |
[49] | Hvalshagen M , Nittala L , Raman R , Sullivan N , Zolbanin HM , When worlds collide: framing students’ challenges with stay-at-home learning during COVID-19 through the lens of conflicting role identities, Communications of the Association for Information Systems (2021) ;48: (5):227–35. |
[50] | Iyer DG , Chapman TA , Overcoming technological inequity in synchronous online learning, Communications of the Association for Information Systems (2021) ;48: (5):1–26. |
[51] | Li TW , Karahalios K , Sundaram H , “It’s all about conversation” Challenges and concerns of faculty and students in the arts, humanities, and the social sciences about education at scale, In Proceedings of the ACM on Human-Computer Interaction (2021) ;4: :1–37. |
[52] | Lumor T , Pulkkinen M , Hirvonen A The Actual Adoption and Use of Mobile Apps: The Case of a Higher Education Context. In Proceeding of Americas Conference on Information Systems, Association of Information Systems. 2020. |
[53] | Mavengere N , Henriksen-Bulmer J , Passmore D , Mayes H , Fakorede O , Coles M , Atfield-Cutts S , Applying innovative technologies and practices in the rapid shift to remote learning, Communications of the Association for Information Systems (2021) ;48: (5):185–95. |
[54] | Xie X , Siau K , Online education during and after COVID-19 pandemic, Journal of Information Technology Case and Application Research (2020) ;22: (3):175–87. |
[55] | Hwang MI , Windsor JC , Pryor A , Building a knowledge base for MIS research: A meta-analysis of a systems success model, Information Resources Management Journal (2000) ;13: (2):26–32. |
[56] | Mehta N , Chauhan S , Gupta P , Jaiswal MP , Pursuing digital learning platform success: A meta-analytic investigation of user and cultural contingencies, Communications of the Association for Information Systems (2021) ;48: (5):1–34. |
[57] | Mosanya M , Buffering academic stress during the COVID-19 pandemic related social isolation: Grit and growth mindset as protective factors against the impact of loneliness, International Journal of Applied Positive Psychology (2020) ;6: (2):159–74. DOI: 10.1007/s41042-020-00043-7. |
[58] | Rogers PL Designing instruction for technology-enhanced learning. IGI Global. 2003. |
[59] | Shirish A , Boughzala I , Srivastava CS , Does technology enabled design-thinking influence digital innovation ? An innovation affordance perspective. In Proceedings of the 52nd Hawaii International Conference on System Sciences. 2019. |
[60] | Weil MM , Rosen LD Technostress: Coping with technology@ work@ home@ play, New York: JohnWiley&Sons. (1997) . |
[61] | Mazmanian M , Yates J , Orlikowski W Ubiquitous email: individual experiences and organizational consequences of blackberry use. In Proceeding of 65th Annual Meeting of the Academy of Management, Academy of Management. 2006. DOI: 10.5465/ambpp.2006.27169074. |
[62] | Åborg C , Billing A ,. Health effects of the paperless office–evaluations of the introduction of electronic document handling systems, Behaviour and Information Technology (2003) ;22: (6):389–96. |
[63] | Sami LK , Pangannaiah NB , Technostress: A Literature survey on the effect of information technology on library users, Library Review (2006) ;55: (7):429–39. |
[64] | DeLone WH , McLean ER , Information systems success: The quest for the dependent variable, Information Systems Research (1992) ;3: (5):60–95. |
[65] | DeLone WH , McLean ER , The DeLone and McLean model of information systems success: a ten-year update, Journal of Management Information Systems (2003) ;19: (5):9–30. |
[66] | Jiang JJ , Klein G , Carr CL , Measuring information system service quality: SERVQUAL from the other side, MIS Quarterly (2002) ;26: (2):145–66. |
[67] | Butler BS , Gray PH , Reliability, mindfulness, and information systems, MIS Quarterly (2006) ;30: (2):211–24. |
[68] | Abdel-Hamid TK , Madnick SE ,The elusive silver lining: how we fail to learn from failure in software development, Sloan Management Review (1990) ;32: :1–32. |
[69] | Austin RD , The effects of time pressure on quality in software development: An agency model, Information Systems Research (2001) ;12: (2):195–207. |
[70] | Ba S , Stallaert J , Whinston AB ,. Research commentary: Introducing a third dimension in information systems design—The case for incentive alignment, Information Systems Research (2001) ;12: (3):225–39. DOI: 10.1287/isre.12.3.225.9712. |
[71] | Compeau DR , Higgins CA , Computer self-efficacy: Development of a measure and initial test, MIS Quarterly (1995) ;19: (2):189–211. |
[72] | Le Pine MA , Zhang Y , Crawford ER , Rich BL . Turning their pain to gain: Charismatic leader influence on follower stress appraisal and job performance. Academy of Management Journal (2016) ;59: (3):1036–59 . |
[73] | Tarafdar M , Tu Q , Ragu-Nathan TS , Impact of technostress on end-user satisfaction and performance, Journal of Management Information Systems (2010) ;27: (3):303–34. |
[74] | Moore GC , Benbasat I . Development of an instrument to measure the perceptions of adopting an information technology innovation, Information Systems Research (1991) ;2: (3):192–222. |
[75] | Faul F , Erdfelder E , Buchner A , Lang AG , Statistical power analyses using G* Power 3, Tests for correlation and regression analyses. Behavior Research Methods (2009) ;41: (5):1149–60. |
[76] | Hair JF , Anderson RE , Tatham RL , Black WC , Multivariate data analysis with readings, Industrial Management & Data Systems (1998) ;12: (5):5–11. |
[77] | Srivastava SC , Chandra S , Shirish A , Technostress creators and job outcomes: Theorising the moderating influence of personality traits, Information Systems Journal (2015) ;25: (5):355–401. |
[78] | Teo TS , Srivastava SC , Jiang L , Trust and Electronic Government Success: An Empirical Study, Journal of Management Information Systems (2008) ;25: (3):99–132. |
[79] | Anderson JC , Gerbing DW , Structural equation modeling in practice: A review and recommended two-step approach, Psychological Bulletin (1988) ;103: (3):411–23. |
[80] | Agarwal R , Prasad JA conceptual and operational definition of personal innovativeness in the domain of information technology, Information Systems Research (1998) ;9: (2):204–15. |
[81] | Shirish A , Cognitive-affective appraisal of technostressors by ICT-based mobile workers and their impacts on technostrain, Human Systems Management (2021) ;40: (2):265–85. DOI: 10.3233/hsm-200979. |
[82] | Atchley W , Wingenbach G , Akers C , Comparison of course completion and student performance through online and traditional courses, International Review of Research in Open and Distributed Learning (2013) ;14: (5):104–16. |
[83] | Doris Austin RD , The effects of time pressure on quality in software development: An agency model, Information Systems Research (2001) ;12: (2):195–207. |
[84] | He T , Li SA , Comparative study of digital informal learning: The effects of digital competence and technology expectancy, British Journal of Educational Technology (2019) ;50: (5):1744–58. |
[85] | Gupta S , Bostrom RP , Technology-Mediated Learning: A Comprehensive Theoretical Mode, Journal of the Association for Information Systems (2009) ;10: (5): DOI:10.17705/1jais.00207. |
[86] | Shatat AS , Shatat AS , Virtual migration of higher education institutions in times of crisis: Major challenges and critical success factors, Human Systems Management (2021) ;40: (5):653–67. DOI: 10.3233/HSM-201160. |
[87] | Al-Ghurbani AM , Jazim F , Abdulrab M , Al-Mamary YHS , Khan I , The Impact of Internal Factors on the Use of Technology in Higher Education in Saudi Arabia during the COVID-19 Pandemic, Human Systems Management (2022) ;41: (2):283–302. DOI: 10.3233/HSM-211219. |
[88] | Marcoulides GA , Saunders C , Editor’s comments: PLS: a silver bullet? MIS Quarterly (2006) ;30: (2): DOI: 10.2307/25148727. |
[89] | Michinov N , Primois C , Improving productivity and creativity in online groups through social comparison process: New evidence for asynchronous electronic brainstorming, Computers In Human Behavior (2005) ;21: (1):11–28. |
[90] | Wang K , Nickerson JV , A literature review on individual creativity support systems, Computers in Human Behavior (2017) ;74: , 139–51. |
[91] | Jahnke I , Liebscher J , Three types of integrated course designs for using mobile technologies to support creativity in higher education, Computers & Education (2020) ;146: :103782. DOI: 10.1016/j.compedu.2019.103782. |
[92] | Di Pietro G , Biagi F , Costa P , Karpinski Z , Mazza J The likely impact of COVID-19 on education: Reflections based on the existing literature and recent international datasets. Publications Office of the European Union. 2020; doi:10.2760/126686. |
[93] | Tarafdar M , Tu Q , Ragu-Nathan T , Impact of technostress on end-user satisfaction and performance, Journal of Management Information Systems (2011) ;27: (3):303–34. DOI: 10.2307/29780194. |
[94] | Tarafdar M , Cooper CL , Stich JF , The technostress trifecta-techno eustress, techno distress and design: Theoretical directions and an agenda for research, Information Systems Journal (2019) ;29: (1):6–42. DOI: 10.1111/isj.12169. |
[95] | Massy WF , Zemsky R information technology to enhance academic productivity.Washington, DC: Educom. 1995. |
[96] | Powell LM , Wimmer H , Evaluating the effectiveness of student group work for mobile application development learning, productivity, enjoyment and confidence in quality, Information Systems Education Journal (2015) ;14: (3):85–95. |
[97] | Van Laar E , Van Deursen AJ , Van Dijk JA , De Haan J , The relation between 21st-century skills and digital skills: A systematic literature review, Computers in Human Behavior (2017) ;72: :577–88. DOI: org/10.1016/j.chb.2017.03.010. |
[98] | Yang C , Chen A , Chen Y , College students’ stress and health in the COVID-19 pandemic: The role of academic workload, separation from school, and fears of contagion, PLoS One (2021) ;16: (2):e0246676. DOI: org/10.1371/journal.pone.0246676. |