Factors affecting social network use by students in Tunisia
Abstract
BACKGROUND:
Several theories have developed instruments for assessing the acceptance of using technologies. This work is a continuation of the unified theory of acceptance and use of technology (UTAUT) research for the review of the factors affecting the acceptance of digital social networks (DSN) among Tunisian students.
OBJECTIVE:
We aim to bring a better understanding of technology use in the educational context. More specifically, we investigate the determinants of digital social network (DSN) acceptance by Tunisian students and the effects of this acceptance on students’outcomes in the presence of social isolation as a mediating variable and fear of COVID-19 to ensure the continuity of university pedagogy.
METHOD:
We used the survey method, sampling stutents (N = 100) during the COVID-19 pandemic to test this model. For the data analysis, this study employed structural equation modeling through the Partial Least Squares Structural Equation Modeling (PLS-SEM) and condition process modeling.
RESULTS:
The research found that Social Influence (SI); Effort Expectancy (EE), Facilitating Conditions (FC), and Performance Expectancy (PE) have a significant and affirmative effect on the behavioral intention (BI) and influence of behavioral intention on digital social network (DSN) user behavior in learning (UBL). Furthermore, Social Isolation (SIS) as a mediating variable and fear of COVID-19 (FOC) has a significant effect between dimensions of adjusted unified theory of acceptance and use of technology (UTAUT) model and behavioural intention of learning by digital social network (DSN) applications.
CONCLUSION:
The new adjusted conceptual model is a significant contribution to the current knowledge, which will enhance the dimensions of the actual unified theory of acceptance and use of technology (UTAUT) model. Moreover, the findings have significant implications for educators and decision-makers involved in designing learning through digital social network (DSN) applications for implementation in universities.
Dr. Hanen Khanchel is an Assistant Professor within the management department at the Institute of Advanced Business Studies (IHEC), University of Carthage, Tunisia. She is an active member and researcher of LIGUE (University of Manouba, Tunisia). Dr. Khanchel is passionate about multi-disciplinary approaches for complex problem solving. Her main research interest includes: Human Resource Management, Strategic Management, Leadership, and Learning. Dr. Khanchel is a highly regarded author, with many research articles published in international journals.
1Introduction
Information and Communication Technologies (ICT) are more and more present in all spheres of life [1]. Computers, laptops and mobile phones are generalized worldwide. The International Union of Telecommunications (IUT) estimates in its 2020 report that 51.2% of the global population has a mobile phone. It also estimates in its 2021 report that 95% of the global population is covered by mobile internet. The International Union of Telecommunications (IUT) also estimates that 71% of the African population is covered by the Internet. The International Union of Telecommunications (IUT) estimates also that there was a total of 16.39 millions connections in 2021 in Tunisia among them were 14.85 million mobile phones, which corresponds to an average of 1.3 per person. Unpredictably, the percentage of people who own telephones in some disadvantaged neighborhoods is higher than the average in the capital of Tunisia. Investigations by the International Union of Telecommunications (IUT) during these recent years have also shown an important evolution in the penetration rate of mobile phones in urban areas such as rural areas [2].
The development of mobile technologies and mobile Internet has largely favored the use of mobile applications such as Facebook, WhatsApp, Snapchat, Instagram, Twitter and Viber. Despite the development of these technologies and perspectives on apprenticeship education which they offer to learners, they are considered as a source of distraction [3]. The educational resources contained in these mobile technologies are considered to have a significant contribution to learn [4]. This consideration of these digital tools constitutes a pretext for their use in university.
Preventive measures are taken in Tunisia with a view to slow down the spread of the pandemic. It is in this context of health crisis that the country has proceeded to the closure of higher education establishments for reduce the contagion. In view of this situation, the Ministry of higher education have proposed the use of mobile technologies in particular the Facebook to ensure pedagogical continuity in universities. Several research studies [5, 6] have shown that digital social networks such as Facebook, Instagram, WhatsApp Snapchat, YouTube, Twitter and Messenger are attracting more and more attention from researchers in the humanities and social sciences [5]. Several research studies are interested in friendly bonds [6], private life and digital identity [7], professional life, digital social capital in communities of practice [8, 25]. Researchers are interested in accepting Information and Communication Technologies (ICT) to learn [9–11]. Rarely have research studies agreed on the intention to use digital social networks in Tunisian university [12]. Subsequently, the present research aims to study the determining factors for the use of social networks in digital education of students with a view to ensure pedagogical continuity beyond Tunisian university infrastructures during the COVID-19 pandemic.
The closure of universities and large schools, following the health crisis [4], has prompted the commitment of Tunisian authorities to resort to digital education. The aim is to ensure pedagogical continuity beyond university infrastructures thanks to a digital education facility. This health crisis has also allowed for an update on the reflection on digital education devices and more particularly on the use of social networks to provide short-term solutions to students. Instructions are given in this sense, in particular to appeal to social networks for educational continuity. However, this decision is a matter of unanimity among all the actors in education for a certain number of reasons why they can be retained: the absence of free and accessible digital educational resources on university sites. It is in this context that voluntary teachers from Tunisian universities have taken the initiative to ensure pedagogical continuity with the help of social networks.
In the study, we aim to bring a better understanding of the use of technologies in the educational context. More specifically, we investigate the determinants of social networks acceptance by university learners and the effects of this acceptance on learners’ outcomes in the presence of social isolation as a mediating variable and fear of COVID-19. The specific technology under consideration here is digital social networks. Social networks are technologies that Tunisian universities use to support online courses and to ensure pedagogical continuity during the COVID-19 pandemic. They allow synchronous interactions between students and teachers, and asynchronous communication through audios, videos, whiteboards, shared applications and images. Social networks enable learners to follow the course in live from outside the classroom anywhere and anytime or to listen to the recordings later at home. These facilities are advantageous to busy learners who are in the labor market or to foreign learners unable to travel at a specific time during the COVID-19 pandemic to attend a classroom session online. The aim of this study is three fold:
– The first aim is to identify the determinants of social networks intention to use and effective use by learners. We believe it is fundamental to know why learners accept or reject the adoption of technology as it represents a benefic investment in an economic stringency climate.
– The second aim is to investigate the effect of social networks use on learners’ outcomes. As stated earlier, understanding factors of use can help ensure the educational continuity during the health crisis, which may enhance knowledge and skills development. The acceptance of a technology would be improved if we focus pedagogical efforts on these determinants.
– The third aim is to consider the influence of the health crisis on social networks use and on learners’ outcomes. On one hand, the propensity of learners to use a certain kind of applications is dependent on mediating variables such as social isolation and fear of COVID-19. On the other hand, we believe that learners’ performance expectancy are dependent not only upon social network applications use, but on many other determinants derived from the context of use. Therefore, we are not able to control the technology as a complex contextual factors, we focused on personal factors that could be influenced by this context [34].
The article is organized as follows:
In the conceptual framework, we define the digital social networks concept, we present some recent research studies dealing with the use of social network applications and sites, and we introduce the theoretical framework of this study. Then, we define the different variables, describe the unified theory of acceptance and use of technology (UTAUT) model and propose the hypotheses derived from the adjusted model. Consequently, we present the procedure we used to collect and to analyze data. We present and discuss results in four steps: 1) descriptive statistics; 2) reliability and validity of the model; 3) hypotheses testing and interpretation; and 4) explained variances findings. We end the study with a conclusion where we summarize the results, expose contributions, debate limitations, and provide recommendations for future research.
2Conceptual framework
The conceptual framework of this study is constituted by the theoretical approach of digital social networks for learning and the theory of acceptance and use of technology.
2.1Digital social networks (DSN)
The notion of social network was introduced by several research studies [13, 24] to study the properties of social structures of a community. It designates a group of individuals and organizations that are connected by different social relationships and that interact between them to share resources [14]. From this definition, the link between social network and social capital is apparent. The notion of social capital thus refers to the terms of access and use of the resources contained in the social network. Bourdieu seems to have been one of the first to use the term social capital which he defines as “the set of potential resources related to the possession of a network lasting relationship of more or less institutionalized knowledge and mutual recognition, belonging to a group” [24]. He also defines three other types of capital:
– cultural capital, a concept close to that of human capital, whether incorporated or institutionalized.
– economic capital linked to heritage resources or income.
– symbolic capital, designating any form of capital having special recognition within society.
According to Bourdieu’s theory [24], the volume of social capital held by an individual varies according to the number of people belonging to his personal network and the volume of cultural, economic and symbol they hold. Social capital is, like symbolic capital, a meta-capital, it has no content. He considers him a multiplier of other capitals. Social capital is perceived as an instrument of domination mobilized by a social group and only takes on its meaning in the exercise of a power relationship. Relationships are traditionally constructed by a physical community that maintains face-to-face interactions. The arrival of the Internet has allowed the emergence of new forms of communication, exchange and virtual communities. However, the virtual communities of social networks are not distinguished from traditional communities that are assisted by communication and exchange modes using digital tools (computers, laptops, smart phones, applications, web sites, etc.). In fact, this community that utilizes digital social networks is called the virtual community [15]. Social networks have long been considered as an exchange space for the members of a community, but this community can also be grouped together to learn [16]. The generalization of mobile telephony, the multiplication of electronic communication platforms, the success of social networking services has offered to virtual communities, in addition to the opportunities to communicate and share dynamically, learning cells [17]. According to this author, the use of digital social networks for learning is simply a return to the sources for those who know the work by psychologist Albert Bandura on “Social learning”. Similarly, social networks are spaces that allow for the development of collaborative learning with fruitful exchanges between individuals within the community [17]. Thus, social networks for learning offer opportunities to communities of practice to develop very active exchanges in real time, to have returns and proceeds as well on their learning [25]. They offer above all the opportunities for learners to continue learning activities outside of class thanks to web tools, such as Facebook, YouTube, WhatsApp, Instagram, Twitter and Viber. These virtual learning communities are recognizable by the digital tools they use [18]. The possibility of creating virtual communities of learners through the use of digital social networks has been retained by those responsible for the educational policy of Tunisia, to ensure the continuity of education in the university during the COVID-19 pandemic. The present study will analyze the acceptance and use of digital social networks to learn among students of universities in Tunisia having experienced the e-learning device.
2.2Model of the unified theory of acceptance and use of technology (UTAUT)
The unified theory of acceptance and use of technology (UTAUT) model is a unified theory of several models providing the theoretical bases for a better underestanding of the different factors affecting the use of ICT [19]. The unified theory of acceptance and use of technology (UTAUT) model, proposed by several research studies has integrated several theories to predict and explain the intention to use a technology by users. Performance expectancy and effort expectancy are taken directly from the TAM Model (Technology Acceptance Model); social influence and enabling conditions are taken from social learning theories; four variables are then considered to have a moderating influence on behavioral intention: gender, age, experience with technology and voluntary or involuntary use. Thus, taking into account the context of use and time makes it possible to understand that the performance expectancy and the effort expectancy may in fact become insignificant in the case of use supported. This theory has been tested and validated empirically by transversal and longitudinal studies on the adoption of technologies in different contexts [19, 31]. That justifie our choice of this model in this research. However, the most widely used model is undoubtedly the Technology Acceptance Model (TAM) developed by researcher [19] and its variants (TAM 2, 3, UTAUT 1 & 2 and versions) with nearly 2000 referenced citations [20]. Based on the psychosocial theories of Reasoned Action [21] and planned behavior (PBT) [22], this Technology Acceptance Model (TAM) proposes to explain the acceptability process by two main subjective factors: perceived usefulness and perceived ease of use. These two factors would thus influence attitudes, which influence intentions to use the technology in question. The work carried out attempts to identify the variables that would affect these representations, the organization (support from managers, communication policy, social influence, etc.), the characteristics of the system itself [19]. Some approaches also propose combining the Technology Acceptance Model (TAM) with other theoretical paradigms to overcome its shortcomings. This is for certain authors who criticize the Technology Acceptance Model (TAM) for its lack of interest in the tasks performed or to be performed. These authors propose to articulate it with another theoretical approach; the Task Technology Fit (TTF) model [23]. The Task Technology Fit (TTF) thus seeks to assess whether the functionalities of the technologies are well “aligned”, that is to say compatible with the work of the users. Their work shows that technology would be more accepted and have more positive effects on individual performance if the technological attributes correspond well to the expected tasks. The traditional theories, in particular the unified theory of acceptance and use of technology (UTAUT) model, contain six dimensions such as Social Influence (SI); Effort Expectancy (EE), Facilitating Conditions (FC), and Performance Expectancy (PE), Behavioral intention (BI) and User behavior (UB). Nevertheless, there are some criticisms adressed to these theories because they don’t take into consideration the attitude, anxiety, and self-efficacy, which are the natural aspects of intention, and attitude concerning using technology does not have a direct effect on intention. For several research studies [24, 25], Bourdieu found that habitus, which describes an individual’s disposition, attitude, and expected benefits about using technology, affects actual practices. He suggested that individual dispositions are important psychological resources. To capture this psychological capital, we adapt the habitus concept to the educational context and define it as an individual’s disposition toward using digital social network applications.
2.3Research hypotheses
In order to study the acceptance of digital social networks (DSN) by students as a learning device in an emergency pandemic settings, we use all the constructs of the unified theory of acceptance and use of technology (UTAUT) model [19]. Although the traditional unified theory of acceptance and use of technology (UTAUT) model explained a considerable variety of behavioural intentions and behavioural options, the model theorized some relationships that may not be applicable in all contexts, omitted some relationships that may be fundamental, and also singled out some dimensions that may be crucial to know why learners accept or reject the adoption of technology. Therefore, we add additional constructs corresponding to fear of COVID-19 (FOC), Social isolation (SIS), Perceived enjoyment (PRE), Mobile self-efficacy (MSE) discussed below and reformulate some of the hypotheses derived from the adjusted unified theory of acceptance and use of technology (UTAUT) model.
The behavioral intention (BI) is defined by several research studies [19, 32] as the degree to which a user has formulated conscious plans to execute or does not execute any future behaviors for the use of a technology. This variable has become the determinant of the most widely used in studies and models of individual-level technology adoption and use.
Use behavior in learning (UBL) are defined as the subjective probability of executing or not executing a future behavior for the intent to adopt a technology [19, 33]. According to this research, the performance expectancy has a positive and significant association with behavioral intention (BI). We formulate the H1 hypothesis that, the performance expectancy has a positive and significant association with behavioral intention in learning by digital social network (DSN) applications.
Effort Expectancy (EE) is defined by several research studies [19, 35] as the degree of facility associated with the use of a technology. According to this research, the effort expectancy affects behavioral intention. We formulate the following hypotheses:
H2: The effort expectancy (EE) has a significant and affirmative relationship with behavioral intention (BI) in learning by digital social network (DSN) applications.
Social Influence (SI) was defined by several research studies [19, 36] as the degree that an individual perceives, other important individuals within the community think he should use a technology. It is also widely accepted that important referents’ behavioral expectations, or subjective norms, will influence one’s technology use. Bourdieu found that social influence refers to social capital factor [24] that appears to be especially relevant to our educational context: support from acquaintances and perceived expectations from family, relatives, peers, and friends. Also, having access to acquaintances that can provide information and knowledge about technology usage represents not only instrumental assistance but also emotional support that can encourage adoption. According to this research [19], the social influence has a significant and affirmative relationship with behavioral intention (BI). We formulate the following hypotheses:
H3: The social influence (SI) has a significant and affirmative relationship with behavioral intention (BI) in learning by digital social network (DSN) applications.
The Facilitating Conditions (FC) were also introduced in the unified theory of acceptance and use of technology (UTAUT) model and this variable was defined by several research studies [19, 37] as the degree to which an individual believes that the necessary infrastructures exist to support the use of a technology. According to this research, the facilitating conditions have a significant and affirmative relationship with behavioral intention. Bourdieu found that economic capital refers to the Facilitating Conditions (FC) means to acquire and access to technology [24]. Obviously, learners with high socioeconomic status tend to possess more information technology access, contact and use experience, and thus more likely to use digital social network applications. We formulate the H4 hypothesis that, facilitating conditions (FC) have a significant and affirmative relationship with behavioral intention (BI) in learning by digital social network (DSN) applications.
Self-efficacy is an important part of psychological capital. It is defined as “it is learner’s assessments of their effectiveness to perform a specific task well; it is related to how he uses skills’ [17]. Self-efficacy is an individual’s trust that he retains the skills to thrive while mastering the tasks associated with digital social networks (DSN). Meanwhile, self-efficacy describes the belief in one’s ability to perform a behavior. Without sufficient self-efficacy, or confidence, even a learner with adequate knowledge may not achieve intended outcomes. Therefore, some view self-efficacy as a student’s believed competencies for task performance. Similarly, scholars have also conceptualized knowledge and self-efficacy as two different aspects of user competence. Bourdieu conceives that cultural capital can manifest itself in three forms [24], including 1) objectified cultural capital, such as pictures and books; 2) institutional cultural capital, such as educational credentials; and 3) embodied cultural capital, or the internal competencies needed to appropriate, understand, and use cultural artifacts [24]. Mobile self-efficacy (MSE) is described as “an individual’s perceptions of his capability to use digital social network (DSN) applications to achieve distinct tasks’ [17]. The research has used mobile self-efficacy as an external stimulus in digital education that supports the learners’ knowledge of digital social networks (DSN). Mobile self-efficacy has been proven in several research studies that showed an affirmative and cogent influence on behavioral intention [17]. Thus, we have framed the following hypothesis:
H5: Mobile self-efficacy (MSE) has an affirmative association with behavioral intention (BI).
The perceived enjoyment (PRE) is “the extent to which the task of using an application is perceived to be enjoyable in its own right, aside from any performance resulting from use’ [37]. The perceived enjoyment (PRE) is an intrinsic inspiration, which signifies the degree of fun enjoyment through digital social network (DSN) applications. Moreover, the habitus is predisposed to generate line of practice or unconscious strategies to maximize learning enjoyment. It orients learning practices based not on consciousness or intentional aims but on the dispositions built out of a practical, prereflective, corporeal sense of limits and realistic possibilities [24], leading learners to accept or reject the adoption of technology. Several research studies [37] have confirmed the positive impact of perceived enjoyment (PRE) with a behavioral intention other than digital social network (DSN) acceptance. The perceived enjoyment (PRE) is used as an outer technology acceptance model (TAM) element. The perceived enjoyment is a vital variable, which positively impacts the behavioral intention (BI); therefore, the perceived enjoyment (PRE) is being used as an external variable of the unified theory of acceptance and use of technology (UTAUT) model to investigate the behavioral intention (BI) in learning by digital social network (DSN) applications. Consequently, we have framed the following hypothesis:
H6: The perceived enjoyment (PRE) has an affirmative association with behavioral intention (BI).
Several research studies [37] certified that the concept of behavioral intention (BI) is the precursor of action. Behavioral intention measure will predict the performance of any voluntary act unless intent changes before the performance. Thus, we have established an affirmative and significant association between actual user behavior and behavioral intention by applying the unified theory of acceptance and use of technology (UTAUT) model. Consequently, we framed the following hypothesis:
H7: Behavioral intention (BI) has a significant and affirmative influence on the user behavior in learning (UBL) by digital social network (DSN) applications.
Digital social networks (DSN) show more related information about the damage caused by COVID-19 pandemic, netizens were more anxious and refrained from going in face to face interactions. So for the precautionary measures put in place by universities from social distancing to avoid contagion, students have stayed at home in self-isolation around the world. Social isolation is described as “the lack of interaction between agents or intersubjective ties between individuals within the community, thus making them socially isolated” [24]. Therefore, universities have faced similar results, and higher education has gone digital. Socially isolated students are more encouraged to take an online course through DSN. Thus, we have formulated the following hypotheses:
H8: Social isolation (SIS) has a significant mediation in the association between the performance expectancy (PE) and learning by digital social networks (DSN) through behavioral intention (BI).
H8A: Social isolation (SIS) has a significant mediation in the association between the performance expectancy (PE) and learning by digital social networks (DSN) through behavioral intention (BI).
H8B: Social isolation (SIS) has a significant mediation in the association between Effort Expectancy (EE) and learning by digital social networks (DSN) through behavioral intention (BI).
H8C: Social isolation (SIS) has a significant mediation in the association between social influence (SI) and learning by digital social networks (DSN) through behavioral intention (BI).
H8D: Social isolation (SIS) has a significant mediation in the association between facilitating conditions (FC) and learning by digital social networks (DSN) through behavioral intention (BI).
H8E: Social isolation (SIS) has a significant mediation in the association between mobile self-efficacy (MSE) learning by digital social networks (DSN) through behavioral intention (BI).
H8F: Social isolation (SIS) has a significant mediation in the association between perceived enjoyment (PRE) and learning by digital social networks (DSN) through behavioral intention (BI).
H8G: Social isolation (SIS) has a significant mediation in the association between behavioral intention (BI) and user behavior in learning (UBL) by digital social network (DSN) applications.
The digital education by the use of digital social networks (DSN) is suitable for the circumstance of COVID-19 pandemic. Thus, students accept digital social networks (DSN) to resume the development of their learning. Several research studies [30] have proven that fear of COVID-19 (FOC) has a moderating influence between variables. Consequently, the following hypotheses are outlined:
H9A: Fear of COVID-19 (FOC) has a significant moderation in the association of the performance expectancy (PE) and behavioral intention (BI).
H9B: Fear of COVID-19 (FOC) has a significant moderation in the association of effort expectancy (EE) and behavioral intention (BI).
H9C: Fear of COVID-19 (FOC) has a significant moderation in the association of social influence (SI) and behavioral intention (BI)
H9D: Fear of COVID-19 (FOC) has a significant moderation in the association of facilitating conditions (FC) and behavioral intention (BI).
H9E: Fear of COVID-19 (FOC) has a significant moderation in the association of mobile self-efficacy (MSE) and behavioral intention (BI).
H9F: Fear of COVID-19 (FOC) has a significant moderation in the association of perceived enjoyment (PRE) and behavioral intention (BI).
H9G: Fear of COVID-19 (FOC) has a significant moderation in the association of behavioral intention (BI) and user behavior in learning (UBL) by digital social network (DSN) applications.
3Methodology
In this section the design of the research is presented in the following subsections. The questionnaire and data collection, surveyed data, the population and the data analysis are discussed in the end.
3.1Research design
The Unified Theory of Acceptance and Use of Technology (UTAUT) model was employed in this study. The acceptance and use of social networks by students in Tunisian universities is tried to be explained on the basis of unified theory of acceptance and use of technology (UTAUT) determining factors. It is assumed that performance expectancy, social influence effort expectancy and facilitating conditions, which are the determining factors of the unified theory of acceptance and use of technology (UTAUT) model, have a positive effect on behavioral intention [19, 37].
3.2Sample and procedure
The validation work was carried out in two phases with a population aged 20 years or over. In the first phase of this study, we asked 30 participants to assess the clarity of the items. The second phase consisted of an online experiment, it allowed us to collect data from 70 participants to study the psychometric properties of the questionnaire. In sum, 100 students answered our online questionnaire. The socio-demographic characteristics of this sample are given in Table 1.
Table 1
Sample description
Variables | Categorization | Frequency N = 100% | |
Gender | Men | 76 | 76% |
Women | 24 | 24% | |
Universities | Private | 72 | 72% |
Public | 28 | 28% | |
Age | 20 to 25 years | 37 | 37% |
25 to 30 years | 18 | 18% | |
30 to 35 years | 6 | 6% | |
35 to 40 years | 4 | 4% | |
40 to 45 years | 16 | 16% | |
45 to 50 years | 19 | 19% | |
Education level | BTP | 32 | 32% |
2020–2021 | BTS | 13 | 13% |
License | 12 | 12% | |
Master 1 | 31 | 31% | |
Master 2 | 11 | 11% | |
Doctorate | 1 | 1% | |
Use Social networks to | Yes | 80 | 80% |
Networks | No | 20 | 20% |
Source: Author.
Table 1 gives the results of the frequent analysis of socio-demographic variables. These results indicate that there are more male respondents in our sample. More of the respondents are students from Private universities. Respondents are at a young age and are enrolled in the first year of the 2020/2021 university cycle.
3.3Data collection and analysis tools
Data from the present study were collected using a questionnaire developed based on items tested in other studies [9, 19]. Questions were chosen in order to reduce the errors on the measures [19]. A Likert type scale composed of 7 modalities ranging from 1 (total disagreement) to 7 (total agreement) was used [38], to measure the perception of students on the adoption of digital social networks in digital education to ensure pedagogical continuity. The students’ responses are directly recorded online then downloaded to an Excel file for processing and analysis. For the data analysis, this study employed structural equation modeling through Partial Least Squares Structural Equation Modeling (PLS-SEM) and condition process modeling. There is a method for estimating the parameters which is less demanding in its conditions of use (no normality, small samples accepted) [39, 40].
4Results and discussions
In this section we report on the findings of testing the adjusted unified theory of acceptance and use of technology (UTAUT) model. First, a reliability and validity analysis is discussed, and second we report on the results of the adjusted unified theory of acceptance and use of technology (UTAUT) model are presented in Fig. 1, and then the original unified theory of acceptance and use of technology (UTAUT) model test results are compared with those of the adjusted unified theory of acceptance and use of technology (UTAUT) model.
Fig. 1
Smart-PLS- Path analyses with R-square values. Source: Figure developed by the author
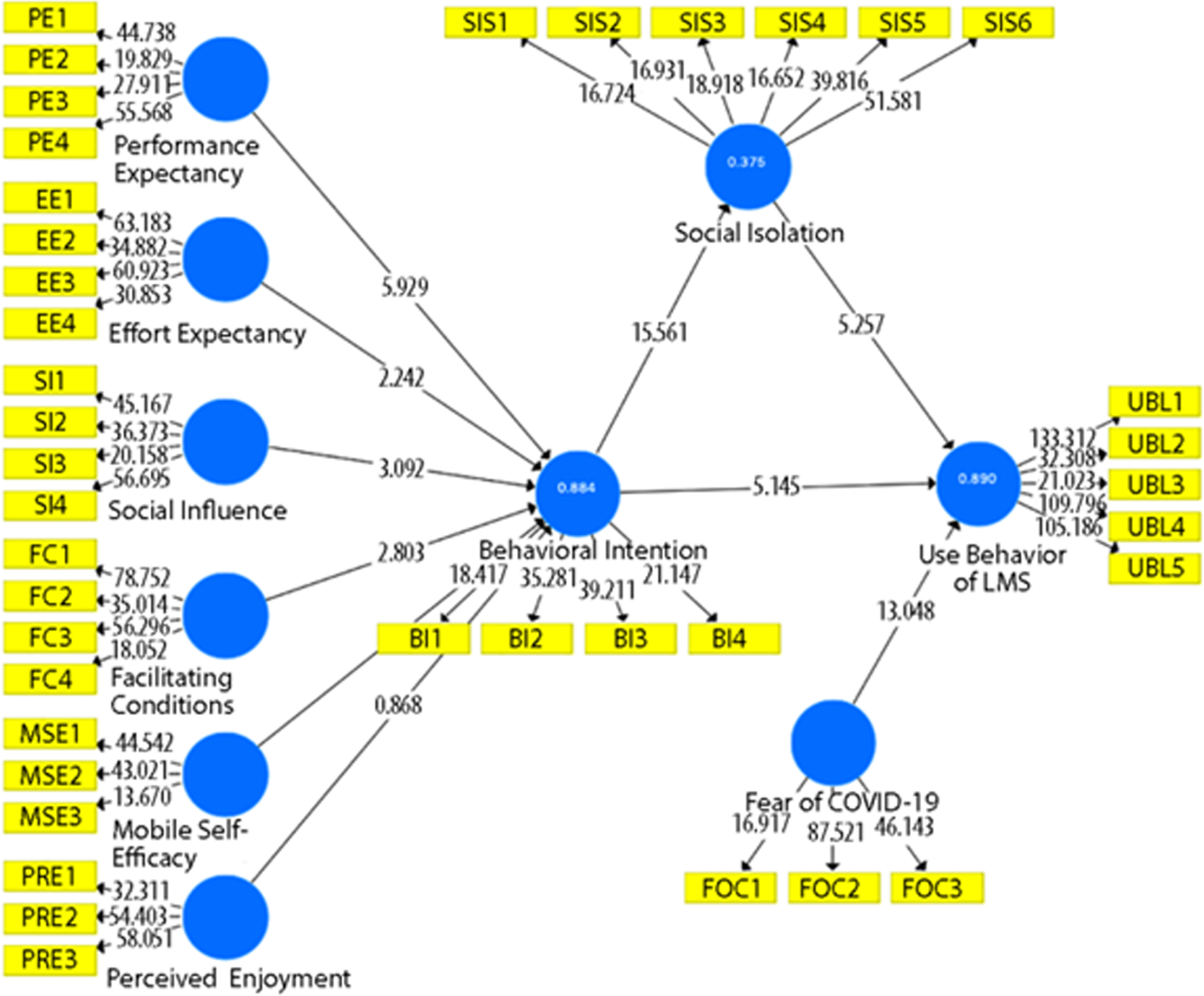
4.1Measurement model analysis
The data was analyzed using Partial Least Squares Structural Equation Modeling (PLS-SEM) through Smart-PLS software version 3.2.3. In Partial Least Squares Structural Equation Modeling (PLS-SEM), the feasibility of the study was verified by several tools such as factor loading, composite reliability average variance extracted, and validity. The calculation of Cronbach’s apha coefficient of the variables studied, in particular the internal consistency of the unified theory of acceptance and use of technology (UTAUT) questionnaire developed. This test allows precisely to reduce the random errors that modify the answers to the questions following a scale. Once the coefficient has been calculated, an estimate is required of the variance of the total score due to all the common factors, based on the items of the scale tested. More precisely, it indicates what is the part of the total score that depends on generic factors based on the set of announcements rather than particular items of the questionnaire. Several research studies [41, 42] allow an internal consistency of a questionnaire in an exploratory research. The structural model is adjusted by evaluating R squared (R2) and path coefficient analyses. R squared (R2) is the proportion of the variation in the dependent variable that is predictable from the independent variable [39]. The structure model is further validated through path co-efficient analysis via direct hypotheses relationship and mediating relationship. The results are consistent with those of previous studies [9, 19] and also the adoption of social networks in digital education of students with a view to ensure a better pedagogical continuity according to the unified theory of acceptance and use of technology (UTAUT) model [11]. The study has evaluated the normality of the data through standard deviation, Kurtosis and skewness since the values of standard deviation and skewness are > ±1.5, and Kurtosis > ±3. Table 2 evaluated the measurement model, which demonstrated that the factor loadings are > 0.70, rho_A > 0.70, CA > 0.70, composite reliability > 0.70, A range of indicators, such as the average variance extracted (AVE), is used to validate constructs [39]. AVE > 0.50 for individual factors.
Table 2
Measurement model
Variables | Items | FL | CA | rho_A | CR | AVE | R Square | SD | SKE | KUR |
Behavioral Intention | BI1 | 0.697 | 0.757 | 0.765 | 0.846 | 0.580 | 0.885 | 1.090 | –0.862 | 0.184 |
BI2 | 0.807 | |||||||||
BI3 | 0.809 | |||||||||
BI4 | 0.727 | |||||||||
Performance Expectancy | PE1 | 0.838 | 0.81 | 0.817 | 0.876 | 0.639 | 1.106 | –0.982 | 0.356 | |
PE2 | 0.718 | |||||||||
PE3 | 0.778 | |||||||||
PE4 | 0.857 | |||||||||
Effort Expectancy | EE1 | 0.853 | 0.849 | 0.853 | 0.898 | 0.689 | 0.977 | –1.039 | 1.379 | |
EE2 | 0.812 | |||||||||
EE3 | 0.855 | |||||||||
EE4 | 0.798 | |||||||||
Social Influence | SI1 | 0.862 | 0.829 | 0.831 | 0.887 | 0.665 | 1.006 | –1.023 | 1.109 | |
SI2 | 0.800 | |||||||||
SI3 | 0.704 | |||||||||
SI4 | 0.883 | |||||||||
Facilitating Conditions | FC1 | 0.904 | 0.842 | 0.857 | 0.894 | 0.681 | 1.076 | –0.89 | 0.266 | |
FC2 | 0.780 | |||||||||
FC3 | 0.883 | |||||||||
FC4 | 0.721 | |||||||||
Mobile Self Efficacy | MSE1 | 0.838 | 0.675 | 0.713 | 0.818 | 0.602 | 1.094 | –1.011 | 0.441 | |
MSE2 | 0.820 | |||||||||
MSE3 | 0.657 | |||||||||
Perceived Enjoyment | PRE1 | 0.775 | 0.843 | 0.841 | 0.906 | 0.764 | 1.051 | –0.966 | 0.603 | |
PRE2 | 0.917 | |||||||||
PRE3 | 0.923 | |||||||||
Fear of COVID-19 | FOCI | 0.714 | 0.791 | 0.847 | 0.876 | 0.703 | 1.061 | –0.964 | 0.52 | |
FOC2 | 0.903 | |||||||||
FOC3 | 0.886 | |||||||||
Social Isolation | SIS1 | 0.718 | 0.854 | 0.89 | 0.887 | 0.569 | 0.378 | 1.072 | –0.823 | 0.255 |
SIS2 | 0.717 | |||||||||
SIS3 | 0.720 | |||||||||
SIS4 | 0.713 | |||||||||
SIS5 | 0.805 | |||||||||
SIS6 | 0.839 | |||||||||
Use Behavior in learning by DSN | UBL1 | 0.952 | 0.934 | 0.944 | 0.951 | 0.796 | 0.890 | 1.082 | –0.924 | 0.309 |
UBL2 | 0.839 | |||||||||
UBL3 | 0.755 | |||||||||
UBL4 | 0.950 | |||||||||
UBL5 | 0.946 |
Source: Author.
The convergent validity and reliability of individual scales and variables have been met. This result has found the discriminant validities as represented in Table 3 that the square roots of the average variance extracted (AVE) values are higher than the correlation of variables.
Table 3
Discriminant validity
Variables | BI | EE | FC | FOC | MSE | PE | PRE | SI | SIS | UBL |
BI | 0.762 | |||||||||
EE | 0.671 | 0.830 | ||||||||
FC | 0.725 | 0.754 | 0.825 | |||||||
FOC | 0.703 | 0.693 | 0.785 | 0.839 | ||||||
MSE | 0.650 | 0.756 | 0.797 | 0.760 | 0.776 | |||||
PE | 0.717 | 0.705 | 0.781 | 0.725 | 0.740 | 0.800 | ||||
PRE | 0.588 | 0.567 | 0.648 | 0.626 | 0.668 | 0.613 | 0.874 | |||
SI | 0.693 | 0.746 | 0.814 | 0.728 | 0.753 | 0.702 | 0.623 | 0.815 | ||
SIS | 0.614 | 0.541 | 0.699 | 0.836 | 0.763 | 0.583 | 0.681 | 0.632 | 0.754 | |
UBL | 0.579 | 0.551 | 0.722 | 0.826 | 0.641 | 0.602 | 0.587 | 0.616 | 0.752 | 0.892 |
Source: Author.
The criterion of discriminant validities of variables has been achieved. The outcomes of Table 4 explained that the Heterotrait-Monotrait ratio of correlation is less than 0.90.
Table 4
Heterotrait-Monotrait Ratio
Variables | BI | EE | FC | FOC | MSE | PE | PRE | SI | SIS | UBL |
BI | ||||||||||
EE | 0.881 | |||||||||
FC | 0.809 | 0.868 | ||||||||
FOC | 0.849 | 0.895 | 0.815 | |||||||
MSE | 0.735 | 0.829 | 0.832 | 0.864 | ||||||
PE | 0.870 | 0.894 | 0.853 | 0.857 | 0.873 | |||||
PRE | 0.716 | 0.654 | 0.759 | 0.782 | 0.869 | 0.726 | ||||
SI | 0.723 | 0.701 | 0.881 | 0.832 | 0.828 | 0.802 | 0.726 | |||
SIS | 0.737 | 0.605 | 0.802 | 0.829 | 0.856 | 0.668 | 0.794 | 0.721 | ||
UBL | 0.680 | 0.612 | 0.849 | 0.836 | 0.802 | 0.688 | 0.655 | 0.695 | 0.870 |
Source: Author.
The considered hypothesized measurement model is valid for assessing the unified theory of acceptance and use of technology (UTAUT) dimensions and behavioral intentions and use behavior of learning by digital social networks (DSN).
Figure 1 demonstrated the R2 (R-square values), also known as a coefficient of determination, and goodness of fit measure. The reading of R2 (R-squared) analyzed the collective value of variance in percentage that treats the change of dependent variable due to independent variable. Thus, figure 1 showed that the R-squared (R2) of behavioral intention is 0.884 that explains that a total change of 88.4% of the behavioral intention (BI) is experienced due to the adjusted dimensions of the unified theory of acceptance and use of technology (UTAUT) model. R-squared (R2) of social Isolation (SIS) is 0.375, explaining that a 37.5% chance of social Isolation (SIS) is experienced due to the behavioral intention (BI). R-squared (R2) of UBL (Use Behavior in learning) is 0.890 explains that a total change of 89.0% of UBL (Use Behavior in learning) is experienced due to behavioral intention (BI) and social isolation (SIS).
4.2Stone-Geisser (Q2) and SRMR indicator’s outcomes
The findings of Table 5 treated the predictive relevance of the individual endogenous model and its factors. The Standardized Root Mean Square Residual (SRMR) is a measure of the mean absolute value of the covariance residuals. It explained the saturated model’s value 0.764 and estimated model value 0.103, which further validated the structural model’s goodness of fit.
Table 5
Stone-Geisser (Q2)
Variables | Q2 = 1 — SSE / SSO |
BI | 0.4567 |
PE | |
EE | |
SI | |
FC | |
MSE | |
PRE | |
SIS | 0.2521 |
FOC | |
UBL | 0.5432 |
Source: Author.
Table 5 explained the values of standard regression weights and p-values of direct and mediating relationships that represented the hypothesized structural model’s validity.
4.3Postulated direct association
Table 6 treated the direct hypothesized association between regressors and dependent factors. The outcomes of Table 6 explained that the direct hypotheses H1 to H5 and H7 are reinforced because individual T-values are greater than±1.96, and corresponding probabilities are less than 0.05 except H6. It is found that dimensions of adjusted unified theory of acceptance and use of technology (UTAUT) model such as PE (Performance Expectancy), EE (Effort Expectancy), SI (Social Influence), FC (Facilitating Conditions) and MSE (Mobile Self-Efficacy) significantly impact behavioral intention (BI). Behavioral intention has a significant effect on UBL (Use Behavior in learning). Nevertheless, PRE did not have a cogent influence on BI (Behavioral Intention). The individual influence of dimensions explained that performance expectancy (PE) showed the maximum influence of 0.538 on behavioral intention (BI). Then, the mobile self-efficacy (MSE) has an effect of 0.285 on behavioral intention (BI).
Table 6
Postulated direct association
Direct Path Hypotheses | Standardized Regression Weights | T-Statistics | P-Values Confidence | Interval | Decision | |
2.5% | 97.5% | |||||
Hl: PE ->BI | 0.538 | 5.888 | 0.000** | 0.376 | 0.737 | Supported |
H3: EE ->BI | 0.125 | 2.173 | 0.030* | 0.013 | 0.240 | Supported |
H4: SI ->BI | 0.312 | 3.127 | 0.002** | 0.094 | 0.484 | Supported |
H5: FC ->BI | 0.275 | 2.884 | 0.004** | 0.452 | 0.087 | Supported |
H6: MSE ->BI | 0.285 | 3.273 | 0.001** | 0.113 | 0.454 | Supported |
H7: PRE ->BI | –0.020 | 0.877 | 0.380 I | –0.067 | 0.024 | Not Supported |
H8: BI ->UBL | 0.156 | 5.296 | 0.000** | 0.211 | 0.094 | Supported |
Note: Null hypotheses rejected at: *p < 0.05 and **p < 0.01. Source: Author.
4.4Mediation analyses
The adjusted unified theory of acceptance and use of technology (UTAUT) model included social isolation as mediating variable. Table 7 explained that indirect mediation of social isolation (SIS) has a significant effect between PE (Performance Expectancy) through BI (Behavioral Intention) on UBL (Use Behavior in learning). Social isolation (SIS) has a significant mediation between EE (Effort Expectancy), SI (Social Influence), FC (Facilitating Conditions) and MSE (Mobile Self-Efficacy) through BI (Behavioral Intention) on UBL (Use Behavior in learning). Nevertheless, the adjusted dimension of perceived enjoyment (PRE) does have a significant effect on UBL (Use Behavior in learning). Social isolation (SIS) has a significant moderating impact on the relationship BI (Behavioral Intention) and user behavior of learning (UBL) by digital social networks (DSN). It is found that hypotheses from H8A to H8G, H8B, H8C, H8D, H8E, H8G are supported except H8F because individual T-values are greater than±1.96, and corresponding probabilities are less than 0.05.
Table 7
Mediation assessement
Mediation Path Hypotheses | Path Coefficient | T-Statistics | P-Values | BCCI | Decision | |
2.5% | 97.5% | |||||
H8A: PE ->BI ->SIS ->UBL | 0.090 | 4.013 | 0.000** | 0.054 | 0.143 | Supported |
H8B: EE ->BI ->SIS ->UBL | 0.021 | 1.997 | 0.046* | 0.004 | 0.047 | Supported |
H8C: FC ->BI ->SIS ->UBL | 0.046 | 2.596 | 0.010* | 0.092 | 0.019 | Supported |
H8D: SI ->BI ->SIS ->UBL | 0.052 | 2.636 | 0.008** | 0.017 | 0.097 | Supported |
H8E: MSE ->BI ->SIS ->UBL | 0.048 | 2.822 | 0.005** | 0.020 | 0.087 | Supported |
H8F: PRE ->BI ->SIS ->UBL | –0.003 | 0.872 | 0.383 | –0.012 | 0.003 | Not Supported |
H8G: BI ->SIS ->UBL | 0.167 | 5.142 | 0.000** | 0.109 | 0.234 | Supported |
Note: Null hypotheses rejected at: *p < 0.05 and **p < 0.01. Source: Author.
4.5Moderation analyses using conditional process modeling
The research has analyzed the moderation between variables. This study used conditional process modeling. Table 8 treated the cogent effect of FOC (Fear of COVID-19), FOC (Fear of COVID-19) does not have any moderation between PRE (Perceived Enjoyment) and BI (Behavioral Intention). It is found that H9A, H9B, H9C, H9D, H9E, and H9G are supported except H9F (T > ±1.96 and p < 0.05).
Table 8
Moderation analysis
Hypotheses | Mode rator | Moderation | Coeffident | SE | T | P* | LLCI | ULCI |
Moderating Effect of FOC b/w PE and Behavioral Intention (BI.) | ||||||||
H9A: | FOC | PE×FOC | –0.1059 | 0.0060 | –17.69 | 0.0000 | –0.1176 | –0.0941 |
Moderating Effect of FOC b/w EE and Behavioral Intention (BI.) | ||||||||
H9B: | FOC | EE×FOC | –0.0341 | 0.0049 | –6.95 | 0.0000 | –0.0438 | –0.0245 |
Moderating Effect of FOC b/w SI and Behavioral Intention (BI.) | ||||||||
H9C: | FOC | SI×FOC | –0.0274 | 0.0054 | –5.04 | 0.0000 | –0.0380 | –0.0167 |
Moderating Effect of FOC b/w FC and Behavioral Intention (BI.) | ||||||||
H9D: | FOC | FC×FOC | 0.0177 | 0.0041 | 4.36 | 0.0000 | 0.0097 | 0.0257 |
Moderating Effect of FOC b/w MSE and Behavioral Intention (BI.) | ||||||||
H9E: | FOC | MSE×FOC | –0.0938 | 0.0056 | –16.69 | 0.0000 | –0.1048 | –0.0828 |
Moderating Effect of FOC b/w PRE and Behavioral Intention (BI.) | ||||||||
H9F: | FOC | PRE×FOC | –0.0099 | 0.0062 | –1.60 | 0.1090 | –0.0221 | 0.0022 |
Moderating Effect of FOC b/w BI and Use behavior of DSN | ||||||||
H9G: | FOC | BI×FOC | –0.0420 | 0.0050 | –8.36 | 0.0000 | –0.0518 | –0.0321 |
Note: where “x” denoted for the multiplicative sign; * Indicates rejection of Null Hypotheses at p < 0.05. Source: Author.
4.6Discussions
This study aims to analyze the unified theory of acceptance and use of technology (UTAUT) model in which adjusted two dimensions as predictors perceived enjoyment and mobile self-efficacy, and social isolation as a mediator. The research implications are divided into sub-sections such as following:
4.6.1Theoretical implications
The educational context of Tunisia during the health crisis is specific. Digital Social Network (DSN) learning as a subsystem of society must draw a fine line between two complementary functions. On the one hand, learning means ensuring pedagogical continuity. On the other hand, it is a question of promoting change and creativity, that is to say, of propelling Tunisian students towards the unknown. These two functions relate equally to knowledge and attitudes, understanding and behavior.
Several research studies analyzed the effect of several factors on digital education by the use of digital social networks (DSN) [44, 45]. The results found the similarities due to the use of digital social networks (DSN) in learning, faculty qualification, and learners’ socio-demographics. Consequently, this study analysed the effect of independent variables such as SI (Social Influence), EE (Effort Expectancy), FC (Facilitating Conditions), and PE (Performance Expectancy), mobile self-efficacy (MSE), and perceived enjoyment (PRE) on behavioral intention (BI), and influence of behavioral intention on the user behavior in learning (UBL) by digital social network (DSN) applications. The results are in line with the previous studies that have shown that SI (Social Influence), EE (Effort Expectancy), FC (Facilitating Conditions), and PE (Performance Expectancy) have an affirmative and significant effect on behavioural intention (BI) [44, 45]. Likewise, the effect of mobile self-efficacy (MSE) treats a cogent and positive effect on behavioral intention (BI), and several research studies found similarities [17]. Nevertheless, the perceived enjoyment (PRE) does not significantly effect behavioral intention (BI) there is no role of perceived enjoyment (PRE). Moreover, the analysis was conducted using dimensions of the Performance Expectancy (PE) that were regarded most appropriate for a situation in which the respondents have diverse social and cultural backgrounds and have to base their answers on the impact of hedonic factor (perceived enjoyment) on the intention to use social network applications in learning. According to Bourdieu’s theory [24], the volume of social capital held by an individual varies according to the number of people belonging to his personal network and the volume of cultural, economic and symbol they hold. This study does not deny such a situation, however it admits the existence of subconscious practices and “the control by the will” [46]. The “unchosen principle of all choices” [47], it orients learning practices based not on consciousness or intentional aims but on the dispositions built out of a practical, prereflective, corporeal sense of limits and realistic possibilities [25, 48], leading learners to accept or reject the adoption of technology. Thus, the habitus is predisposed to generate line of practice or unconscious strategies to improve the pleasure of studying, meeting colleagues, and sharing experiences. We chose to use Bourdieu’s theory of social capital for our study because it attends to this potential downside, to the influences of power and social structures on access to and ability to deploy social capital resources [24]. Bourdieu distinguished between the social networks to which people belong and the outcomes of those social relationships [24]. He noted that although individuals benefit from participation in groups, benefits and costs of participation are not equally distributed but rather on the basis of one’s social position. Such power differentials constitute and are constituted by the opportunities and constraints offered various individuals by social institutions that influence both the actions that individuals take and the contexts in which they act [25]. These influences inform one’s ability to make choices and to activate one’s self-efficacy, both consciously and outside of conscious awareness [25]. Besides, this study treats the role of social isolation (SIS) as a mediating variable in an association between regressors and the actual user behavior in learning (UBL) by digital social networks (DSN). The results are in line with the previous studies that have shown that social isolation (SIS) mediates significantly positively in an association between independent variables PE (Performance Expectancy), EE (Effort Expectancy), SI (Social Influence), FC (Facilitating Conditions), MSE (Mobile Self-Efficacy), and UBL (user behavior in learning) [30]. Nevertheless, social isolation (SIS) does not mediate between perceived enjoyment (PRE) and the user behavior in learning (UBL). Furthermore, the research treats the moderating effect of fear of COVID-19 (FOC) between the adjusted unified theory of acceptance and use of technology (UTAUT) model and UBL (user behavior in learning) dimensions. The outcomes are coherent with the previous studies that have shown that fear of COVID-19 (FOC) had a cogent impact between adjusted unified theory of acceptance and use of technology (UTAUT) model PE (Performance Expectancy), EE (Effort Expectancy), SI (Social Influence), FC (Facilitating Conditions), and MSE (Mobile Self-Efficacy), the user behavior in learning (UBL) [49, 50]. Nevertheless, fear of COVID-19 (FOC) does not moderate between PRE (Perceived Enjoyment) and UBL (user behavior in learning).
4.6.2Practical implications
This research was an answer to a need and challenge that the educators experienced to introduce digital social networks (DSN) in Tunisian universities during the COVID-19 pandemic. Their experience has been partially successful, but the road thus far has been more difficult and included an inefficient education system. These experiences glowed an interest opportunity in investigating the acceptance and use of social networks in the university, indirectly leading to this research. Meanwhile, the use of digital social network (DSN) sites in education is also becoming like a tradition, a custom. Digital social network (DSN) use would thus be a practice in accordance with social conventions, observed among members of a given social group belonging to communities of practice [25]. It then corresponds to a set of socially shared practices, constructed by a group of students according to defined social rules. This finding is in line with previous studies that have mentioned these processes in their studies of the social meanings associated with the uses of technology in different contexts [51]. Furthermore, this study has shown how the use of digital social network (DSN) applications in learning stabilized to become a norm for the socio-professional environment of education. However, the educational context imposed by the social isolation appears to be decisive for universities and decision-makers involved in ensuring pedagogical continuity in Tunisia. It determines the social representations mobilized from their insertion in the education field. Social capital is perceived as an instrument of domination mobilized by a group of decision-makers and only takes on its meaning in the exercise of a power relationship. This relationship is the root of the charismatic illusion in which, in extreme cases, the group of decision-makers represents the spokesperson can appear to itself and others as causa sui. Alienation arises from the fact that isolated agents who are not part of the social network of this group, especially since they are symbolically less strong, they cannot constitute as an influence group [24, 52], that is to say as a force capable of making itself heard and expressing their opinions in the field of education.
Several aspects that might be taken into consideration by practitioners are provided below:
Firstly, educators should be more vigilant and prepared as compared to the conventional learning. Similarly, educators need support to effectively integrate digital social network (DSN) applications into their innovative teaching practices and methods and to help learners overcome some of the difficulties encountered with this form of dynamic learning environment [53]. Supporting the training of educators in the use of digital social network (DSN) applications and promoting teaching practices adapted to this context of health crisis is fundamental to guarantee the effective use of digital social networks (DSN) in education.
Secondly, parents should monitor their children’ digital social network (DSN) use. They should monitor the types of interactions that learners make and shared documents on social media, as well as in text messages. Make sure that the photos that they post are appropriate, and that they are not on the receiving end of questionable content from other individuals within the community.
Thirdly, universities should invest in quality performance expectancy and impact evaluations of new tools and broadly share the results [19, 33]. They should concentrate on digital social network (DSN) applications in learning and incorporate the modes of digital education imparting devices to enhance efficiency.
Fourthly, the education policies should aim to strengthen engagement between universities and parents to improve parent information and guidance on effective practices to support their children’s digital education [43].
Summing up, parents and educators have a vital role in supporting learners to develop strong attitudes towards digital social network (DSN) applications in learning, they can help learners overcome some of the potential challenges posed by digital education such as remaining focused during online courses or maintaining sufficient enjoyment to learn [37]. They are also crucial in supporting learners using digital social network (DSN) applications and sites effectively and making the most of digital divices for learning. Self-efficacy and perceived enjoyment to learn have an important role in improving performance expectancy at university [17, 37], but may be especially crucial should digital education continue during the COVID-19 pandemic.
5Conclusions and research contributions
The research analyzed the influence of health crisis on behavioral intention and influence of behavioral intention on the user behavior of digital social network (DSN) users to ensure learning continuity. The research treats the influence of social isolation as a mediating factor between variables; this study incorporated the COVID-19 pandemic as a moderating variable and measured the moderator’s effect between dimensions of the adjusted unified theory of acceptance and use of technology (UTAUT) model and the outcome variable. The results found that SI (Social Influence), EE (Effort Expectancy), FC (Facilitating Conditions), and PE (Performance Expectancy) have a significant and affirmative effect on the behavioral intention and influence of behavioral intention on digital social network (DSN) user behavior [44, 45]. Nevertheless, the perceived enjoyment does not significantly effect behavioral intention. The research recommend that social isolation mediates significantly and positively in an association between independent factors SI (Social Influence), EE (Effort Expectancy), FC (Facilitating Conditions), PE (Performance Expectancy) and MSE (Mobile Self-Efficacy), and user behavior in learning by digital social networks (DSN). However, social isolation (SIS) does not mediate between perceived enjoyment (PRE) and the user behavior of digital social networks (DSN). The results found that Fear of COVID-19 (FOC) did not have a cogent impact between adjusted unified theory of acceptance and use of technology (UTAUT) model SI (Social Influence), EE (Effort Expectancy), FC (Facilitating Conditions), PE (Performance Expectancy), and MSE (Mobile Self-Efficacy), and user behavior in learning by digital social networks (DSN). Nevertheless, Fear of COVID-19 (FOC) does not moderate between perceived enjoyment and the user behavior in learning by digital social network (DSN) applications. The effect of mobile self-efficacy showed a cogent and positive effect on behavioral intention [17]. The study concluded that the adjusted unified theory of acceptance and use of technology (UTAUT) model is a valuable instrument to analyze the effect of behavioral intention on the user behavior in learning by digital social network (DSN) applications. This study concluded that social influence (SI); effort expectancy (EE), facilitating conditions (FC), and performance expectancy (PE) have significant effect on the behavioral intention (BI).
Summing up, the new adjusted conceptual model is a significant contribution to the current knowledge, which will enhance the dimensions of the actual unified theory of acceptance and use of technology (UTAUT) model. This research was new and innovative in that there has been very little work done integrating social network with technology acceptance. The modification of the unified theory of acceptance and use of technology (UTAUT) model in an attempt to strengthen the different constructs, added to technology acceptance theory in a number of ways.
Firstly, the application of the unified theory of acceptance and use of technology (UTAUT) model to Tunisian universities in designing learning through digital social network (DSN) applications. Most technology acceptance research has been done on learners in classroom settings and the use of the tool in real-life, professional settings is valuable.
Secondly, the research was conducted at public and private educational institutions in Tunisia, implementing different types of social network applications. This variety of case institutions and applications also adds breadth and depth to the unified theory of acceptance and use of technology (UTAUT) research.
5.1Practical contributions
In addition to the theoretical contributions, the results of this study also provide contributions for practice.
Firstly, the findings have significant contributions for educators and decision-makers involved in designing learning through digital social network (DSN) applications for implementation in universities.
Secondly, the use of digital social network (DSN) applications in education stabilized to become a norm for the socio-professional environment of the Tunisian university. The experience of educators to introduce social networks in Tunisian universities during the COVID-19 pandemic has been partially successful, but the road thus far has been more difficult and included a failed system at one organization. Therefore, appropriate measures will be taken to improve their pedagogy for more effectiveness.
Thirdly, parents and educators have a vital role in supporting learners to develop strong attitudes towards digital social network (DSN) applications in learning, they can help learners overcome some of the potential challenges posed by digital education such as remaining focused during online courses or maintaining sufficient enjoyment to learn [37]. They are also crucial in supporting learners using digital social network (DSN) applications and sites effectively and making the most of digital divices for learning. Self-efficacy and perceived enjoyment to learn have an important role in improving performance expectancy at university [17, 37], but may be especially crucial should digital education continue during the COVID-19 pandemic.
5.2Research limitations and directions for future research
This research has been limited by the following aspects that might be addressed in future research:
The study turned out relatively small in size, it reached what was expected of it, namely to answer questions that were formulated at the start of the research. Armed with the knowledge that the sample population was large and varied, as well as with detailed information about the research itself, readers of research can more generalize and transfer the conclusions to other contexts. Furthermore, we have studied the determinants of the use of social networks in digital education of students with a view to ensure a pedagogical continuity according to the unified theory of acceptance and use of technology (UTAUT) model [19]. These findings allow us to identify that the use of social networks in higher education is not solely determined by the internal factors of the users, but this also implies considering external factors that can facilitate or secure operational use of digital social network (DSN) applications.
The research did not use the full complement of items from the Performance Expectancy (PE) dimension of the unified theory of acceptance and use of technology (UTAUT). The effect of this adaptation of the measurement device was limited as the scale still functioned reliably and drawn significant findings. Accumulating further validity evidence and adding items to measure other factors affecting social network use by students are recommended in future research.
This study provides outlook to future investigations to replicate this adjusted model in perspectives of other educational contexts and analyze the effectiveness of the digital education in other countries.
Acknowledgments
The author thanks the editor and two anonymous reviewers for their constructive comments and help in developing this article and bringing it into the present form.
The author received no financial support for the research, authorship and/or publication of this article.
References
[1] | Ratheeswari K . Information communication technology in education, Journal of Applied and Advanced Research (2018) ;3: :45. doi: 10.21839/jaar.2018.v3iS1.169. |
[2] | IUT, Statistics. [Internet]. Available from: https://www.itu.int. [Accessed 10 February 2022]. |
[3] | Beaune A , Thanh JT About mobile learning in the countries of the south. Adject.net. [Internet]. Available from: https://www.adjectitnet/spip/spip.php?article334. [Accessed 10 February 2022]. |
[4] | UNESCO, Statistics, [Internet]. Available 1187 from: https://en.unesco.org/covid19/educationresponse. [Accessed 10 February 2022]. |
[5] | Stenger T , Coutant A , The social networking networks: Promotional discourse on the definition of an object and aresearch methodology, HERMES Journalof Language and Communication in Business (2010) ;44: :209–28. |
[6] | Affo MIB. Numerical social capital, substitution role, or complementarily? Stress management issues among users of digital social networks. Thesis dissertation, Aix-Marseille; 2017. |
[7] | Capelle RS , Representations and strategies ofyoung teachers face digital social networks, Les Cahiers du Numerique (2018) ;14: (3):13–34. |
[8] | Tobi S , Maon S , Ghazali N The use of online social networking and quality of life. 2013;131-5. doi: 10.1109/TIME-E.2013.6611978. |
[9] | Attuquayefio S , Addo H , Using the UTAUT model to analyze students’ICT adoption, International Journal of Education and Development Using ICT (2014) .10: (3). |
[10] | Ghassoub A , Ahmed Fal M Factor analysis determinant of the acceptance of Moroccan students of a new mode of school support conceived for a mobile learning out of class. EpiNetN°. 2017;199. |
[11] | Kouakou KS The determinants of network adoption Numerical societies in professional situations: Empirical study within the libraries of Ivory universities: Determinants of adoption of social digital networks by Ivory Coast university librarians. Frantice.net. 2014;9. |
[12] | Ben Romdhane E , La question de l’acceptation des outils de e-learning par les apprenants: Quels dimensions et déterminants en milieu universitaire tunisien? Revue Internationale Des Technologies en Pédagogie Universitaire (2013) ;10: :46–57. |
[13] | Barnes JA , Class and committees in a Norwegian island parish, Human Relations (1954) ;7: (1):39–58. |
[14] | Kwon O , Wen Y , An empirical study of the factors affecting social network service use, Computers in Human Behavior (2010) ;26: (2):254–63. |
[15] | Agostini S , Mechant P Towards a definition of virtual community. Signo y Pensamiento. 2019;38. doi: 10.11144/Javeriana.syp38-74.tdvc. |
[16] | Dillenbourg P , Poirier C , Caries L Communities, learning virtual: E-jargon or new paradigm. Dans A. Taurisson et A. Sentini (dir.), Pedagogies. Only. Montreal Presses; 2003, pp. 11-47. |
[17] | Bandura A The stormy decade: Fact or fiction, Psychology in the Schools (2006) ;1: :224–31 doi: 10.1002/1520-6807(196407)1:3 < 224: AID-PITS2310010303 > 3.0.CO;2-I. |
[18] | Dolbeau-Bandin C , Are social media networks reinventing museum education tools? The case of the Suzon Facebook page at the Caen Memorial Museum, Essachess. (2016) ;9: :141–52. |
[19] | Venkatesh V , Brown SA , Maruping LM , Bala H , Predicting different conceptualizations of system use: The competing roles of behavioral intention, facilitating conditions, and behavioral expectation. MIS Quarterly. 2008;483-502. |
[20] | Sandberg K , Wahlberg O Towards a model of the acceptance of information and communication technology in rural small businesses. 2006. |
[21] | Fishbein M , Ajzen I Belief, attitude, intention and behaviour: An introduction to theory and research. 1975. |
[22] | Luzzi L , Spencer A , Factors influencing the use of public dental services: An application of the Theory of Planned Behaviour, BMC Health Services Research (2008) ;8: :93. doi: 10.1186/1472-6963-8-93. |
[23] | Schlagwein D , Daneshgar F , Van Toorn C Theoretical Model of E-Learning Ability to Support Attainment of Students’ Graduate Attributes. Proceedings –Pacific Asia Conference on Information Systems, PACIS, 2012. |
[24] | Bourdieu P The forms of capital. In: Richardson J, Handbook of Theory and Research for the Sociology of Education. Westport, CT: Greenwood, 1986, pp. 241-58. |
[25] | Bourdieu P Outline of a Theory of Practice. Cambridge, UK: Cambridge University Press; (1977) . |
[26] | Venkatesh V , Davis F , A theoretical extension of the technology acceptance model: Four longitudinal field studies, Management Science (2000) ;46: :186–204. doi: 10.1287/mnsc.46.2.186.11926. |
[27] | Venkatesh V , Bala H , , Technology acceptance model 3 and a research agenda on interventions, Decision Sciences - DECISION SCI (2008) ;39: :273–315. doi: 10.1111/j.1540-5915.2008.00192.x. |
[28] | Venkatesh V , Morris M , Davis G , Davis F , User acceptance of information technology: Toward a unified view, MIS Quarterly (2003) ;27: :425–78. doi: 10.2307/30036540. |
[29] | Venkatesh V , Thong JYL , Xu X Consumer acceptance and use of information technology: Extending the unified theory of acceptance and use of technology. MIS Quarterly. 2012;36. |
[30] | Ahmed RR , štreimikiene D , Rolle JA , Due PA , The COVID-19 Pandemic and the antecedents for the Impulse buying behavior of US Citizens, . Journal of Competitiveness (2020) ;12: (3):5–27. doi:10.7441/joc.2020.03.01. |
[31] | Yi M , Jackson J , Park J , Probst J , Understanding information technology acceptance by individual professionals: Toward an integrative view, Information & Management (2006) ;43: (3):350–63. |
[32] | Lee YH , Hsieh YC , Hsu CN , Adding innovation diffusion theory to the technology acceptance model: Supporting employees’ intentions to use E-learning systems, Educational Technology & Society (2011) ;14: (4):124–37. |
[33] | Chechen L , Prashant P , Chen JL , Information technology adoption behavior life cycle: Toward a Technology Continuance Theory (TCT), International Journal of Information Management - INT J INFORM MANAGE (2009) ;29: :309–20. doi: 10.1016/j.ijinfomgt.2009.03.004. |
[34] | Hidoussi K , Dubois M , L’effet du contrôle comportemental, l’auto-efficacité et la charge cognitive de travail sur l’intention d’usage d’un progiciel de gestion intégrée, Psychologie du Travail et Des Organisations 2015. |
[35] | Bobillier Chaumon ME , Cuvillier B , Durif-Bruckert C , Cros F , Vanhille M , Body BS , Concevoir une technologie ambiante pour le maintien à domicile: Une démarche prospective par la prise en compte des systèmes d’activité, Le Travail Humain (2014) ;1: :39–62. doi: 10.3917/th.771.0039. |
[36] | Radovan M , Kristl N , Acceptance of technology and its impact on teacher’s activities in virtual classroom: Integrating UTAUT and CoI into a combined model, Turkish Online Journal of Educational Technology (2017) ;16: :11–22. |
[37] | Zhao H , Khan A The students’ flow experience with the continuous intention of using online english platforms, Front Psychol. (2022) ;12: :807084. doi: 10.3389/fpsyg.2021.807084. |
[38] | Demeuse M , Introduction aux théories et aux méthodes de la mesure en sciences psychologiques et en sciences de l’éducation Éducation 2004. |
[39] | Hair JF , Hult GTM , Ringle C , Sarstedt M A Primer on Partial Least Squares Structural Equation Modeling (PLSSEM). Sage Publications, Inc.; (2013) . |
[40] | Başol O , Yalcın EC , How does the digital economy and society index (DESI) affect labor market indicators in EU countries? 2021:503-12. |
[41] | Berthon PM , Ewing LLH , Captivating company: Dimensions of attractiveness in employer branding, International Journal of Advertising (2005) ;24: (2):151–72. |
[42] | Tavakol M , Dennick R , Making sense of cronbach’s alpha, International Journal of Medical Education (2011) ;2: :53–5. doi: 10.5116/ijme.4dfb.8dfd. |
[43] | Gorica P , Popovski F , Popovska H , Communication strategies for strengthening the parent-teacher relationships in the primary schools, International Journal of Research Studies in Education (2021) ;10: :123–34. doi: 10.5861/ijrse.2021.a076. |
[44] | Kumar V , Nanda P Social media in higher education: A framework for continuous engagement. International Journal of Information and Communication Technology Education. 2018;15. doi: 10.4018/IJICTE.2019010108. |
[45] | Ovadia S ResearchGate and academia.edu: Academic social networks. Behavioral & Social Sciences Librarian. 2014;33. 165-169. doi: 10.1080/01639269.2014.934093. |
[46] | Bourdieu P , Distinction: A Social Critique of the Judgement of Taste. London: Routledge; 1984. |
[47] | Bourdieu P The Logic of Practice. Cambridge, UK: Polity; 1990. |
[48] | Bourdieu P , Wacquant L An Invitation to Reflexive Sociology. Chicago, IL: University of Chicago Press; Cambridge: Polity Press; (1992) . |
[49] | Shahzad A , Hassan R , Aremu AY , Hussain A , Lodhi RN , Effects of COVID-19 in Elearning on higher education institution students: The group comparison between male and female, Quality and Quantity (2021) ;55: :805–26. doi: 10.1007/s11135-020-01028-z. |
[50] | Raza SA , Qazi W , Khan KA , Salam J Social isolation and acceptance of the Learning Management System (LMS) in the time of COVID-19 Pandemic: An expansion of the UTAUT Model, Journal of Educational Computing Research (2021) ;59: (2):183–208. doi: 10.1177/0735633120960421. |
[51] | Bourdieu P Un art moyen essai sur les usages sociaux de la photographie. Paris: Minuit; 1965. |
[52] | Bourdieu P , The social space and the genesis of groups, Theory and Society (1985) ;14: (6):723–44. |
[53] | Franklin H , Harrington I , A review into effective classroom management and strategies for student engagement: Teacher and student roles in today’s classrooms, Journal of Education and Training Studies (2019) ;7: :1. doi: 10.11114/jets.v7i12.4491. |