Construction and application of a core competitiveness evaluation index system for public health personnel training based on the CIPP model
Abstract
BACKGROUND:
With the change of human disease spectrum and the increase of public emergencies, the public’s demand for health services and health is gradually increasing and higher expectations are placed on public health personnel.
OBJECTIVE:
Research needs to establish a comprehensive system of evaluation indices for accurate assessment of the core competencies of public health personnel, to enhance their core competitiveness, and introduce novel approaches to evaluate talent development in the field of public health.
METHODS:
The study is based on the CIPP (Context Input Process Product) model and uses literature analysis, semi-structured interviews, and Delphi methods to construct an evaluation index system for the core competitiveness of public health talent cultivation. The entropy method is used to determine the weight of the model evaluation index. Finally, the improved Artistic Be Colony algorithm (ABC) is used to optimize the BP network, and apply it to evaluate the core competitiveness of public health talent cultivation.
RESULTS:
The improved BP network achieved the target accuracy within 11 iterations, with the optimal value observed after 16 iterations, producing an MSE (Mean Square Error) value of 10–13. The evaluation of the index system reported a 97% accuracy, and upon application to the university’s public health training programme, nearly 50% of students and teachers achieved core competitiveness quality scores above 90.
CONCLUSIONS:
The aforementioned method suggests that it can proficiently assess the fundamental competitiveness of training for public health personnel and offer guidance for future development in the domain of public health.
Ma Luo, from Hangzhou, Zhejiang, China. Master’s degree. He is currently a lecturer at Zhejiang Agriculture and Forestry University Jiyang College, Zhuji, China. His research direction is public administration. MaLuo is interested inthe professional capacity building path based on big data analysis for students in the major of public administration.
1Introduction
Against the background of changes in the spectrum of human diseases and an increase in public health emergencies, there is a growing demand from the public for health services and health, with corresponding increasing expectations for public health personnel [1–3]. With the maturation of China’s public health system, the significance of cultivating public health experts has become increasingly prominent [4–6]. Nonetheless, issues such as the slow development of talent and the need to enhance quality persist [7, 8]. The impartial and scientific assessment of the quality of public health talent cultivation has thus become a critical task that requires urgent attention. The study aims to develop a competency assessment index system that is appropriate for the cultivation of public health specialists, as well as to suggest practical and scientific methods for evaluation. The researchers utilized an advanced artificial bee colony algorithm (ABC) to enhance the BP neural network, which was then applied to the evaluation of the core competency of public health specialists. Furthermore, the study employed the entropy method to establish the weights of each indicator, thus augmenting the objectivity and scientific rigor of the evaluation findings. The research provides a scientific and practical evaluation tool for public health talent cultivation by addressing the gap in the evaluation index system and methods. Additionally, the study introduces a novel technique using the improved artificial bee colony (ABC) algorithm to optimize the BP neural network with proven efficiency and accuracy through empirical testing. The investigation utilizes the validated and widely applied CIPP (Context Input Process Product) model, which boasts a robust theoretical basis within educational assessment fields. The objective of the study is to expand the public health talent cultivation core competitiveness evaluation index system from a unidimensional to a multidimensional comprehensive assessment, thereby innovating the study. The aim of this study is to produce objective and scientific talent assessment tools for decision-making departments in education at all levels. These tools will aid in optimizing public health talent cultivation strategies, promoting the integration of industry, academia, and research, advancing public health initiatives, and improving the health of the public.
The main purpose of the study is to construct an evaluation index system for the core competitiveness of public health talent cultivation, effectively and scientifically evaluate the core competitiveness of public health talent cultivation, and provide specific suggestions and guidance for public health talent cultivation. Therefore, the study uses the CIPP model to construct an evaluation index system for the core competitiveness of public health talent cultivation. This model adopts four dimensions of environment, investment, process and product to comprehensively evaluate the project. The CIPP model can be customized based on specific requirements, enhancing its adaptability and suitability across diverse evaluation settings and demands. The upgraded ABC algorithm can enhance the optimization of the BP neural network. The enhanced ABC algorithm plays a valuable role in producing clear and precise evaluation results while tackling vast datasets and complex issues. In addition, the entropy method enables the objective determination of indicator weights, eliminating subjectivity and enhancing objectivity. Meanwhile, this approach is relatively easy, straightforward to use, lowers the threshold for implementation and enhances practicality. To summarize, the techniques utilized in the investigation possess excellent completeness, flexibility, precision, and ease of use. The research topic’s main gap concerns the absence of a scientific and standardized system of evaluation indices for the core competitiveness of public health talent cultivation. Moreover, there is an absence of a comprehensive, accurate, and pragmatic method of appraising the core competitiveness of public health talent cultivation. The study’s proposed method can accurately address the mentioned research gap. Employing the CIPP model to build an indicator system can effectively enhance the comprehensive reflection of the core competitiveness of talent cultivation, thereby addressing the inadequacies of the current evaluation indicator system. By utilizing the entropy method to determine the weight of indicators, the evaluation findings become more objective and scientifically driven, precisely demonstrating the significance of each indicator in the overall evaluation system, consequently addressing the limitations posed by subjective evaluation methods in current evaluating systems. The use of research methods bears practical significance in enhancing evaluation efficiency and accuracy, guiding training strategies and educational decision-making, and fostering the integration of industry, academia, and research. It serves to enhance the quality of public health personnel development, thereby promoting the enhancement of public health standards.
2Literature review
Sukarni S introduced the CIPP evaluation model as a management-oriented evaluation model to help managers make program decisions through evaluation. The model uses a mixed methods approach to analyze data quantitatively and descriptively and has been shown to be effective in addressing potential problems in public health programs [9]. Setyadi F et al. propose a CIPP evaluation model to assess physical education programs in relation to the physical health of teachers and students in schools. The model used an interview instrument as well as a value scale questionnaire to collect relevant data, while quantitative descriptive analysis of these data showed that the mean physical health assessed by this method reached 84 [10]. Rachmaniar R’s team conducted a quantitative analysis using the CIPP assessment model developed by Stufflebeam. Respondents included five department heads, 27 supervisors, 20 industry supervisors and 134 students who participated in the 2017 industry placement class. The results showed that among the respondents who were supervisors of management information systems, this category was followed by a relatively low percentage of 37.03%, thus requiring the development of information systems [11]. Aman A’s team used a mixed method approach, combining qualitative and quantitative methods, to evaluate blended learning in colleges and universities. The chosen evaluation model was Stufflebeam’s CIPP model. The results showed that the blended learning evaluation model had a good load-factor value structure and the overall reliability score was higher than 0.7 [12]. Thaitami S H and other researchers proposed the application of the CIPP model to career information services in response to the current problem of the existence of many cases of unemployment. The model focused on assessing the context, process, input and product of each component to achieve effective delivery of career information services and the results showed that the actual assessment was well accepted by the industry [13].
BP neural networks are now widely used in various fields, and the improvement methods for BP networks can provide methodological references for the construction of evaluation index systems. wu Z J et al. proposed a 4D trajectory prediction model based on BP neural network amount for the problem that the traditional trajectory prediction methods cannot adapt to the requirements of high precision real-time prediction. The model mainly analyzes the total flight time by hierarchical clustering algorithm to predict the future trajectory. And the results showed that its predicted trajectory had high accuracy [14]. Liang Y et al. found that the vegetation changes in certain environments can affect the interferometric reflectometer of the satellite system, so they proposed a BP neural network-based nonlinear soil moisture inversion method. The method is based on a sinusoidal fitting model to obtain signal-to-noise interferograms for GPS data acquisition. The results showed that the model had a strong nonlinear fitting capability and can handle soil moisture well [15]. Xia W et al for solving the problem of low detection rate and detection efficiency of Industrial control system intrusion detection model for various attacks, a method of optimizing BP neural network based on Adaboost algorithm is proposed. The results showed that the Adaboost algorithm can effectively solve intrusion detection problems by optimizing the BP neural network [16]. Abellán García J et al. constructed four models based on BP neural networks to predict the slump flow of concrete to address the problem that the performance of ultra-high performance concrete is difficult to predict. The results showed that the improved BP models can accurately predict the slump flow of UHPC [17]. Samuel O D’s team found that ethanol is highly renewable and can be used as a substitute for methanol, so they used BP neural networks and response surface methodology to model the yield of ethyl ester. And the results showed that the model predicted yields that were consistent with experimental yields [18]. Geng J et al. investigated the impact of the 2019 coronavirus outbreak on household income, sustainable food diversity, sustainable energy consumption and food security. They collected relevant data from 728 households, and analyzed the data using t-tests and logistic regression. It was found that COVID-19 will have a serious impact on households in 2019. Researching and launching nutrition-sensitive programs can help reduce the impact of the virus on society and households, while highlighting the importance of training public health personnel [19]. The Hafeez A team believes that understanding national and subnational health trends and estimating disease burden can help decision-makers track progress and identify disparities in overall health performance. They used the methods and data inputs of GBD 2019 to estimate the social population index, total fertility rate, specific causes of death, years of life lost, years of disability, disability-adjusted life years, healthy life years, and risk factors for 286 causes of death and 369 nonfatal causes of health loss in Pakistan and its four provinces and three regions from 1990 to 2019. The results showed that public health has a significant impact on life, seriously affecting people’s quality of life and threatening their safety. Therefore, research suggests equitable investment in the health system and prioritizing high-impact policy interventions and planning to save lives and improve health outcomes [20].
In summary, many researchers have conducted a lot of studies on the application of CIPP evaluation model and BP neural network model in practice and achieved certain results, but few scholars have applied them to the field of building a core competitiveness evaluation index system for public health personnel training. Therefore, this study uses the CIPP evaluation model as the basis for constructing the corresponding index system and the improved BP neural network for evaluation to provide a basis for the quality of talent training. Through this improvement study, there is a certain gap between the methods of other researchers and their comparison. The study introduces an improved ABC algorithm to optimize the BP network for evaluation. In previous studies, many were based on the original BP neural network without further emphasizing and adopting improved algorithms to optimize the BP network; The study also used the entropy method to determine indicator weights, which helps to improve the accuracy and scientificity of the evaluation, which was not emphasized in previous research.
3Construction of the core competitiveness evaluation index system of public health personnel training based on CIPP model
3.1The construction of CIPP model in the indicator system
The COVID-19 pandemic has put great strain on the global healthcare system, resulting in the heightened competition for excellence in public health education. Accordingly, the development of an evaluation index system is paramount [21, 22]. The CIPP model is a process-oriented and integrated model of training evaluation. The ultimate goal of the model is not to evaluate a particular behavior, nor is the motivation for evaluation to state or verify a particular conclusion, but rather to identify the problem and continuously improve the talent development process [23]. The CIPP model is one of the most common methods used to provide researchers with a large amount of basic material, but it is also an ongoing process of analyzing existing problems and thus continuously tracking dynamic changes. The CIPP model is actually a combination of four processes: Context, Input, Process and Product, and consists of four main components: Context Evaluation, Input Evaluation, Process Evaluation and Outcome Evaluation [24]. Contextual evaluation is the assessment and analysis of the purpose, implementation conditions and other context. It is a scientific and rigorous analysis and assessment of the participants’ receptiveness and the implementation conditions, both for the overall objectives and for each level of objectives, and provides a reference basis for judging whether the training program is feasible and achieves practical results, as well as a strong support for optimizing the implementation program. The input evaluation is an in-depth assessment of the context, which allows the selection of a training pathway and the selection of a more suitable and efficient implementation program from a practical point of view [25]. Process evaluation is the evaluation of the process, i.e. the implementation of the program, with the main aim of monitoring and testing the implementation process to ensure that it is efficient, stable and reliable. Outcome evaluation is the final part of the CIPP model framework, focusing on the whole implementation process. The basic structure of the CIPP model is shown in Fig. 1.
Fig. 1
Basic framework of CIPP theoretical model.
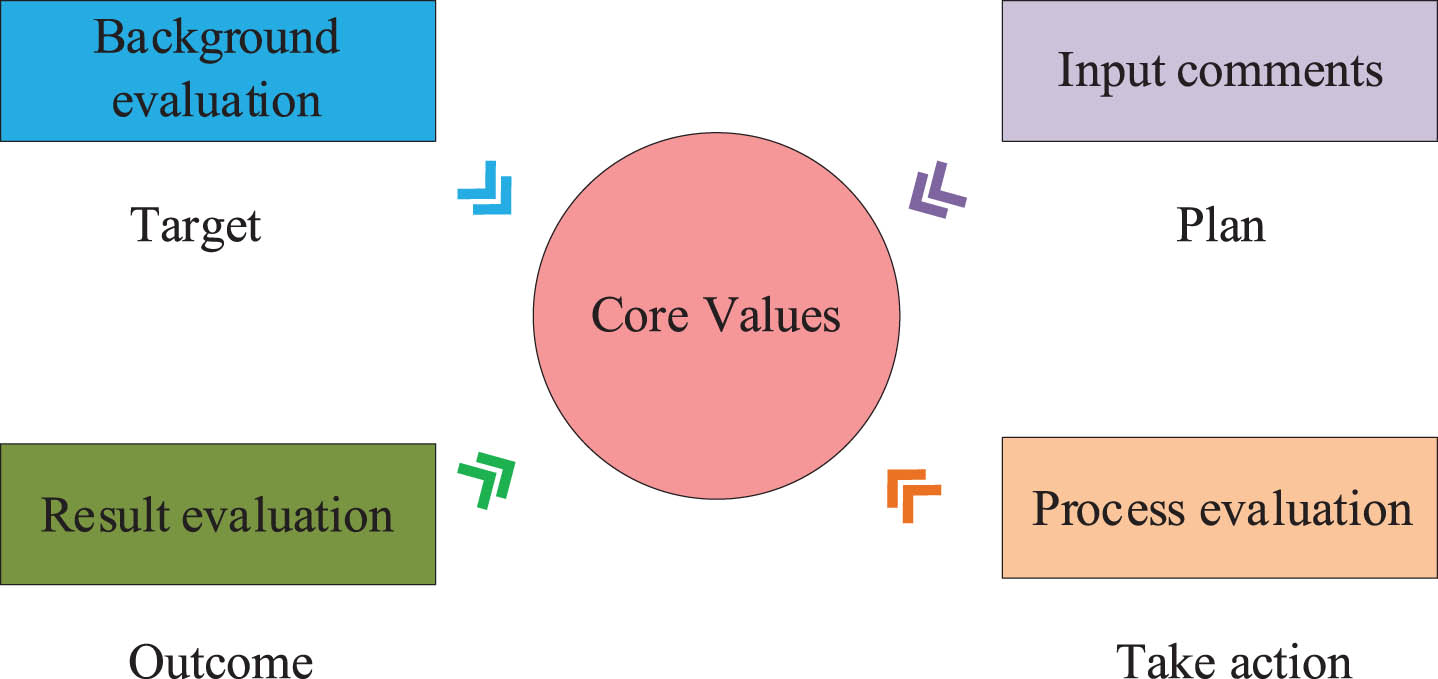
The study was grounded in the CIPP theoretical model and used a combination of semi-structured interviews and literature analysis to first identify the core competitiveness evaluation indicators for public health workforce education. The literature analysis was conducted by searching for keywords such as ‘Delphi, undergraduate, workforce training, quality assessment, core competitiveness, evaluation indicators’, with a time frame of 2017–2022 and excluding full text, duplicate publications, conference abstracts or reports that were not available. Semi-structured interviews were conducted by developing an interview outline, collecting relevant information, collating and analyzing the data. The identity of interviewees was coded to protect their privacy, and changes were made according to the interviewee’s wishes [26]. To ensure the quality of the indicator system, a large amount of national and international literature was searched and rigorously screened and classified during the quality control phase. At the same time, national and international evaluation standards were used based on familiarity with relevant national policies and documents. At the semi-structured interview stage, an outline of the interview was developed through group discussions, and disagreements were resolved through discussion, secondary interviews or tutor judgement as they arose [27, 28]. The literature screening and semi-structured interview process is shown in Fig. 2.
Fig. 2
Literature screening and a semi-structured interview process.
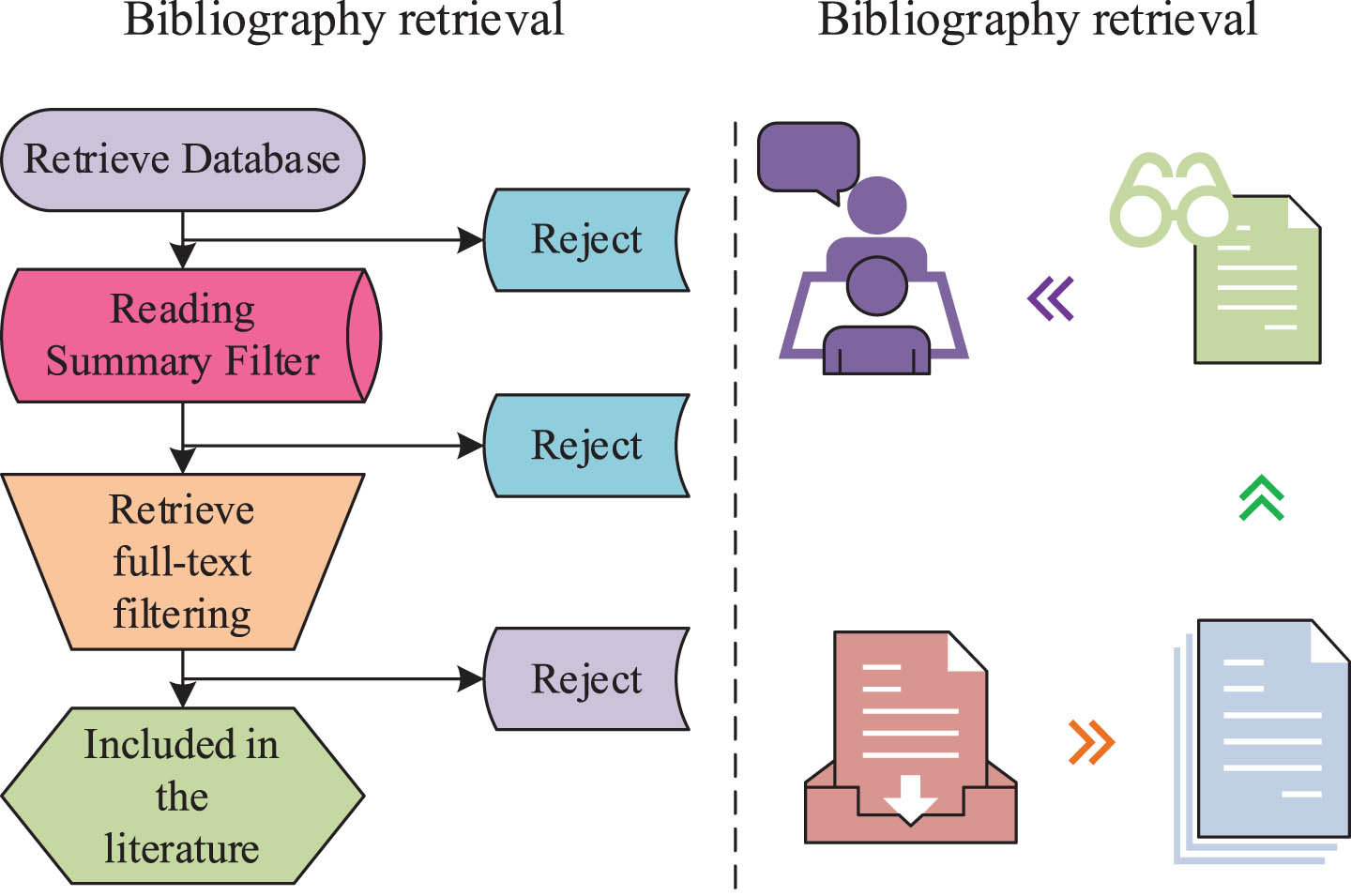
On this basis, experts in the field of public health education, teaching management and other public health fields in China were selected to conduct two rounds of expert consultation using the Delphi method to determine the content of the core competitiveness evaluation indexes for public health personnel training, and to determine the weights of the indexes at all levels using the entropy value method. Table 1 shows the core competitiveness evaluation index system of public health workforce training identified by the proposed method. In Table 1, four first-level indicators are identified, namely background evaluation, input evaluation, process evaluation and outcome evaluation. The background evaluation includes three secondary indicators: training objectives, attractiveness to students and social reputation. The input evaluation includes two secondary indicators: faculty building and educational support. The process evaluation includes three secondary indicators: curriculum design, teaching organization and teaching quality assurance. In the outcome evaluation, it includes three secondary indicators of honors performance, student core quality and graduate quality.
Table 1
Evaluation index system for core competitiveness of public health talent cultivation
Primary indicator | Secondary indicators |
A. Background evaluation | A1. Training objectives |
A2. Student Attraction | |
A3. Social reputation | |
B. Input comments | B1. Teacher construction |
B2. Educational support | |
C. Process evaluation | C1. Curriculum construction |
C2. Teaching organization | |
C3. Teaching quality assurance | |
D. Result evaluation | D1. Honor Striving Power |
D2. Student Core Literacy | |
D3. Graduate quality |
Table 1 identifies the primary and secondary indicators, and further divides the secondary indicators into three levels. The three-level indicators can better optimize the performance of the evaluation model. The three-level indicators are divided into 74 indicators, as shown in Fig. 3.
Fig. 3
Evaluation Indicators for Core Competitiveness of Public Health Talent Cultivation.
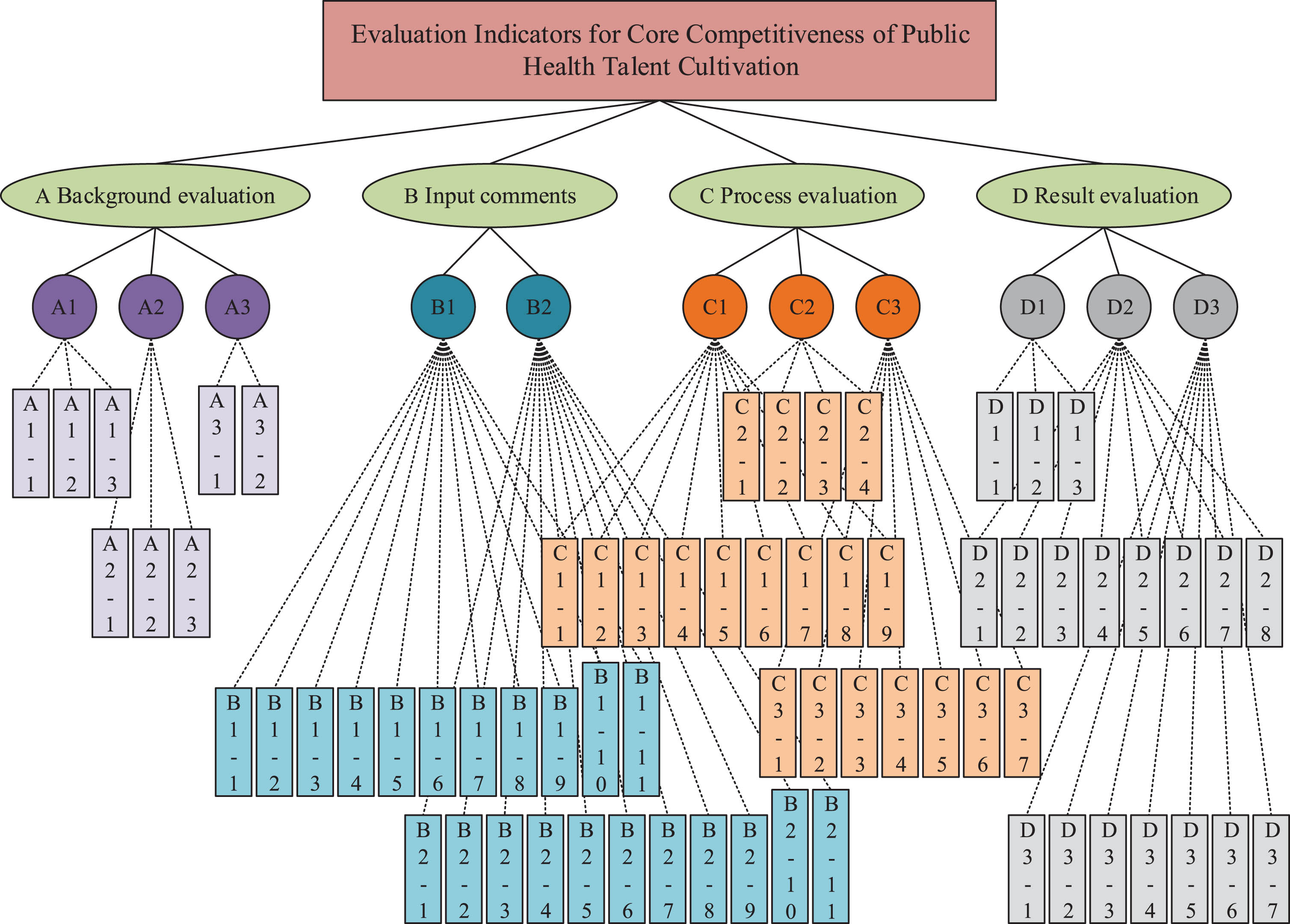
In Fig. 3, A1-1 represents the degree of compliance with professional development laws; A1-2 represents guidance and operability; A1-3 represents the degree to which nursing concepts are reflected; A2-1 represents the enrollment batch; A2-2 represents the average admission score; A3-1 represents the number of nurses; A3-2 represents the medical care ratio; A3-3 represents the demand for highly educated nurses; B1-1 represents the level of reasonableness; B1-2 represents the degree of matching. B1-3 is the support level of the course for graduation requirements; B1-4 to B1-6 are the number of courses, the number of first-class courses, and the number of ideological and political courses; B1-7 is the internship and practice plan; B1-8 is the degree of integration of content courses. B2-1 to B2-9 represent the ratio of professional teachers to students, the structure of teachers’ titles, the age structure of teachers, the structure of teachers’ educational background, the competence of teaching positions, the scientific research and innovation ability of teachers, the cultivation of teachers’ ethics, the development mechanism of teachers, and the proportion of “dual teachers”. B3-1 to B3-11 represent education funds, book resources and electronic information resources, laboratory space, situation of tertiary teaching hospitals, mental health symposiums and sports activities, ideological and political competitions and lectures, interprofessional cooperation projects, proportion of overseas cooperation and educational exchange students, research competitions and academic lectures, community volunteer services and practical activities. The C1-1 value and C1-3 value respectively represent the teacher’s appearance, teaching attitude and teaching investment. C2-1 to C2-5 respectively represent the teaching content and progress, learning environment, teaching form, teaching methods and tools, and teaching informatization construction. C3-1 to C3-7 respectively represent the teacher evaluation and assessment system, the evaluation system and standards for assessing students’ academic performance, the quality monitoring and evaluation of the whole teaching process, the cooperative education and practical teaching mechanism, the clinical teaching base management mechanism, students’ satisfaction with teaching, and the implementation of the continuous tracking and feedback mechanism for graduate quality. D1-1 to D1-7 respectively represent the degree of achievement of talent cultivation goals, the pass rate of course grades, the pass rate of students’ physical fitness tests, the number of awards in national competitions, the number of students participating in scientific research innovation and entrepreneurship projects, the number of papers and patents published by students, and the number of other kinds of awards. D2-1 to D2-9 respectively represent the quality of graduate thesis, graduation rate, enrolment rate, employment rate, industry orientation and stability, one-time pass rate of practicing nurse qualification examination, graduate employment satisfaction, internship and employer satisfaction, and the number of outstanding alumni. D3-1 to D3-7 respectively represent professional humanistic literacy, ideological and moral cultivation, communication ability, self-learning ability, innovation and entrepreneurship ability, foreign language proficiency, and professional competence. After establishing the indicator system, the study carried out the weighting of indicators at all levels using the entropy value method. The entropy value method calculates the evaluation data of the core competitiveness of talent cultivation as shown in Equation (1).
(1)
In Equation (1),
(2)
In Equation (2), Zij is the value to complete the translation, and B is the corresponding length value. Subsequently, the core competency evaluation indicators for public health personnel training are homogenized in Equation (3).
(3)
In Equation (3), pij is the indicator weighting of i for the mid-sample of the j indicator, and Zij corresponds to the panned completed evaluation data.
The entropy values under the j indicator are shown in Equation (4).
(4)
In Equation (4), Ej is the entropy value. The coefficient of variation for this indicator is calculated as shown in Equation (5).
(5)
In Equation (5), Gj denotes the coefficient of variation. The weights of the discrepancy coefficients were obtained by normalization as shown in Equation (6).
(6)
In Equation (6), wj indicates the weight. The final calculation of the specific quality of this indicator evaluation is shown in Equation (7).
(7)
In Equation (7), Fi indicates the quality of the evaluation.
3.2Improvement of BP neural network in evaluating the core competitiveness of talent cultivation
Based on the establishment of the core competitiveness evaluation index system of public health personnel training, the study uses BP neural network to evaluate the core competitiveness of public health personnel training. BP neural network is a multi-layer feed-forward neural network trained according to the error back-propagation algorithm, which is capable of black-box modelling of non-linear problems by training the network parameters, so as to obtain the optimal threshold and weights [29, 30]. The topology of the neural network can be divided into three layers, namely the input layer, the hidden layer and the output layer, and the specific network structure is shown in Fig. 4.
Fig. 4
BP neural network structure.
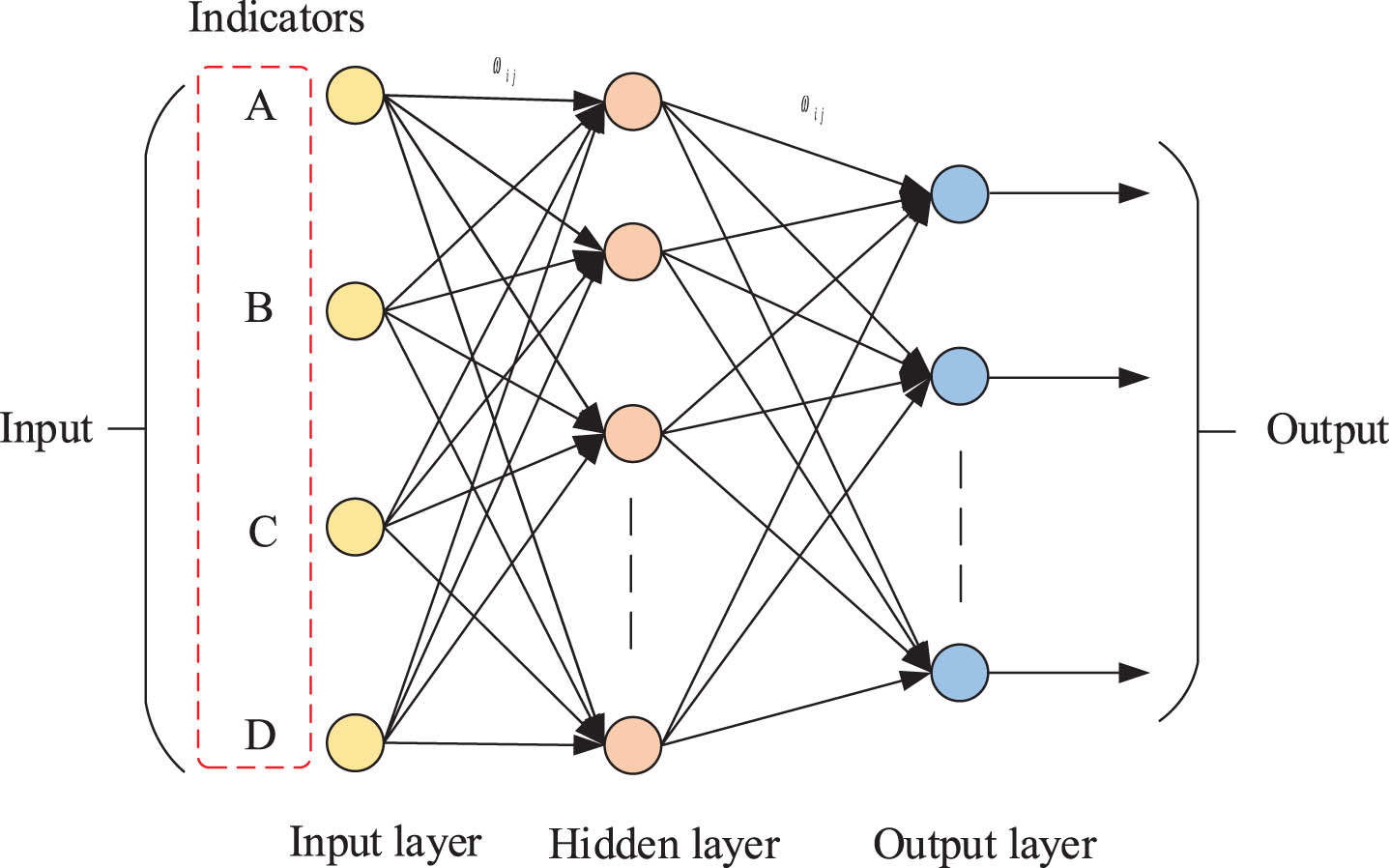
BP neural networks generally use the negative gradient descent method to find the minimum of the error function, which consists of forward propagation between the input layer and the hidden layer and backward propagation of the error [31, 32]. The propagation from the input layer to the hidden layer is linear, while the propagation from the hidden layer to the output layer is achieved by a sigmoid function, which has the advantage of handling the non-linear relationship between the input and output layers to achieve the gradient computation. The output layer excitation function is given by Equation (8).
(8)
In Equation (8), β represents the output layer excitation function. The Sigmoid function is a continuously derivable function, as shown in Equation (9).
(9)
Although BP neural networks have strong self-learning and self-adaptation capabilities, there are still problems such as insufficient accuracy and unstable results. The Artificial Bee Colony (ABC) is a swarm intelligence optimization algorithm designed to simulate the process of a natural bee colony searching for a honey source, with fewer adjustment parameters and a simple structure that can achieve a dynamic balance in global and local search [33, 34]. The ABC algorithm was introduced to improve the BP network by dividing the artificial bee colony into hired bees and non-hired bees, which include scouting bees and following bees. The hired and follower bees are responsible for exploiting the nectar sources, while the scout bees are responsible for randomly finding new nectar sources, mainly by reflecting the merits of the nectar sources through the fitness values. In the process of nectar source exploitation, the update equation is shown in Equation (10).
(10)
In Equation (10), r is a random number between [–1,1], k ∈ (1, 2, …, M), j ∈ (1, 2, …, N) where k ≠ j. Ukj refers to the updated nectar location, Sij is the current location marked by the nectar, and Skj is the randomly selected nectar location in the region.
The value of the degree of adaptation when opening the nectar source is shown in Equation (11).
(11)
In Equation (11), f (θ) represents the fitness value and fi represents the mean absolute error (EMAPE) of the network corresponding to the i-th honey source.
The most desirable value for the fitness value is 1. The following bee selects the nectar source using the roulette wheel method based on information about the nectar source marked by the hiring bee. The probability is calculated as shown in Equation (12).
(12)
In Equation (12), θi is the i-th nectar source, f (θi) is the fitness value of the θi nectar source, i ∈ (1, 2, …, T). TP represents the probability of being selected. It can be seen that the swarm sorts out the optimal nectar source by comparing the size of the nectar adaptation values, which is the optimal solution. The artificial bee colony algorithm is able to achieve global optimization through individual local search, effectively avoiding the convergence of local extrema, and has the advantage of a relatively simple structure, fewer control parameters, and the ability to parallelize global and local search. At the same time, the algorithm can effectively reduce complexity and improve efficiency. However, as the amount of data increases, there are still limits to its search range and the merit-seeking mechanism destroys the diversity of the population, which leads to a reduction in the speed of convergence at a later stage. Therefore, the improved artificial bee colony algorithm (IABC) was developed by introducing the global optimal solution and adaptive inertia factors ω1 and ω2 to speed up the convergence and improve the prediction accuracy. The IABC algorithm is shown in Equation (13).
(13)
ω1 in the calculation is shown in Equation (13). In Equation (7), n and nmax represent the current and maximum number of iterations, ωmax and ωmin represent the maximum and minimum values of the inertia factor, ω1 is the rate at which the new nectar source approaches the original or domain nectar source, and ω2 is the rate at which the new nectar source approaches the optimal nectar source for the population. The calculation procedure is shown in Equation (14).
(14)
(15)
In Equations (14) and (15), ω1 and ω2 take the minimum and maximum values respectively when n = 0 is used, and ω1 and ω2 take the maximum and minimum values respectively when n = nmax is used. Initially, ω2 is large and the search speed is fast and the convergence speed is relatively fast. As the iterations continue, ω1 gradually increases, the search range of random honey sources is expanded and the racial diversity is increased. The IABC algorithm first initializes the colony and the hiring bees search for new nectar sources in the neighborhood, compare the advantages and disadvantages of the old and new sources, mark the source with the higher adaptation value and discard the old one. The following bees then determine the location of the new nectar source by calculating the size of the adaptation. When the number of nectar source updates reaches the limit, the following or hiring bee becomes a scout bee and continues to search for a new source. The algorithm is terminated when the final number of iterations reaches the maximum number of iterations. The schematic diagram is shown in Fig. 5.
Fig. 5
Schematic diagram of IABC algorithm implementation process.
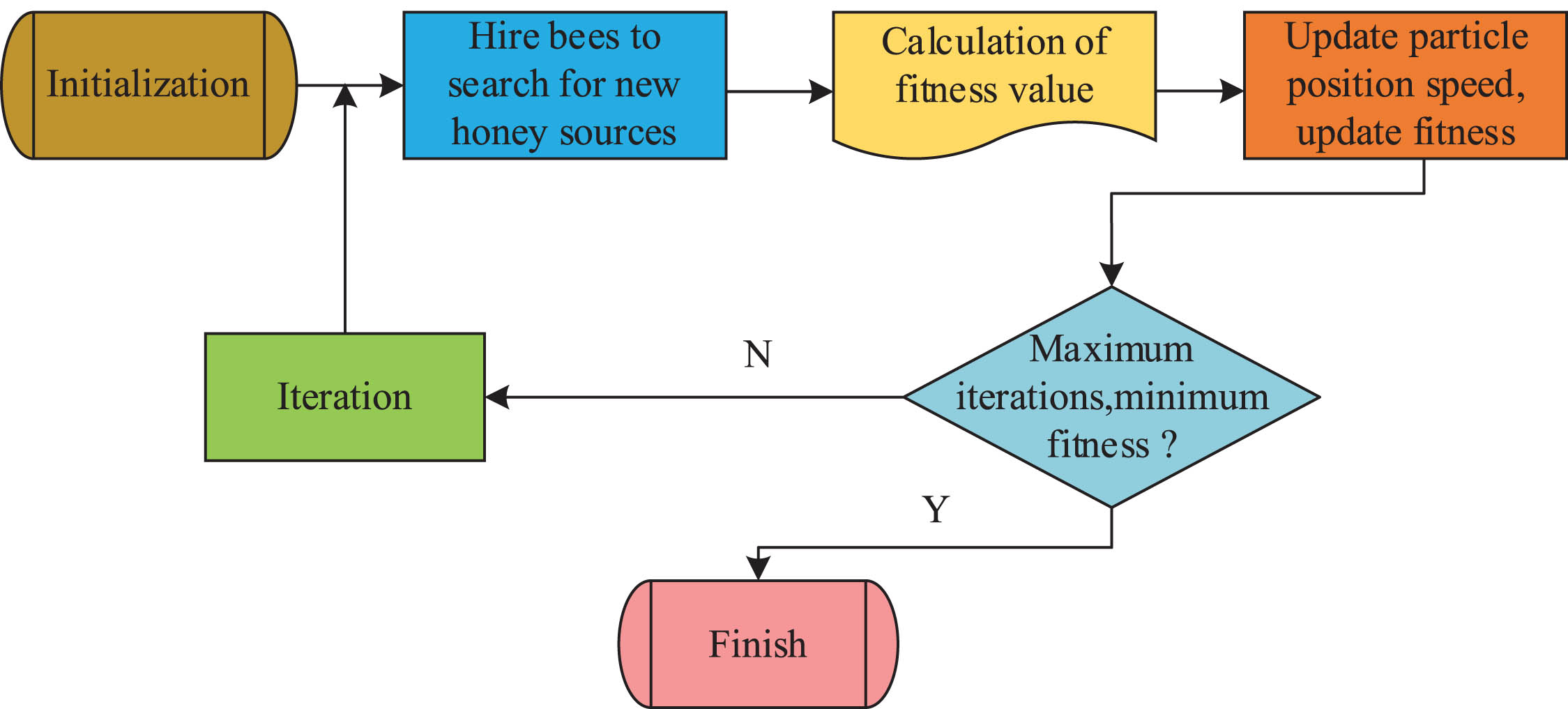
The final IABC-BP algorithm first preprocesses the input data to determine the BP neural network structure. Then the colony parameters are initialized and the hired bees start searching for new nectar sources and calculate the size of the adaptation. If the fitness is greater than the original nectar source, it is converted into a hired bee to continue selecting the nectar source; if the fitness is less than the original nectar source, the source is cancelled. When the number of nectar source updates reaches the limit and is still not optimized, the calculation is stopped. Finally, the data corresponding to the globally optimal honey source is used as the weight and threshold to complete the test. The specific process steps are shown in Fig. 6.
Fig. 6
Specific process of IABC-BP algorithm.
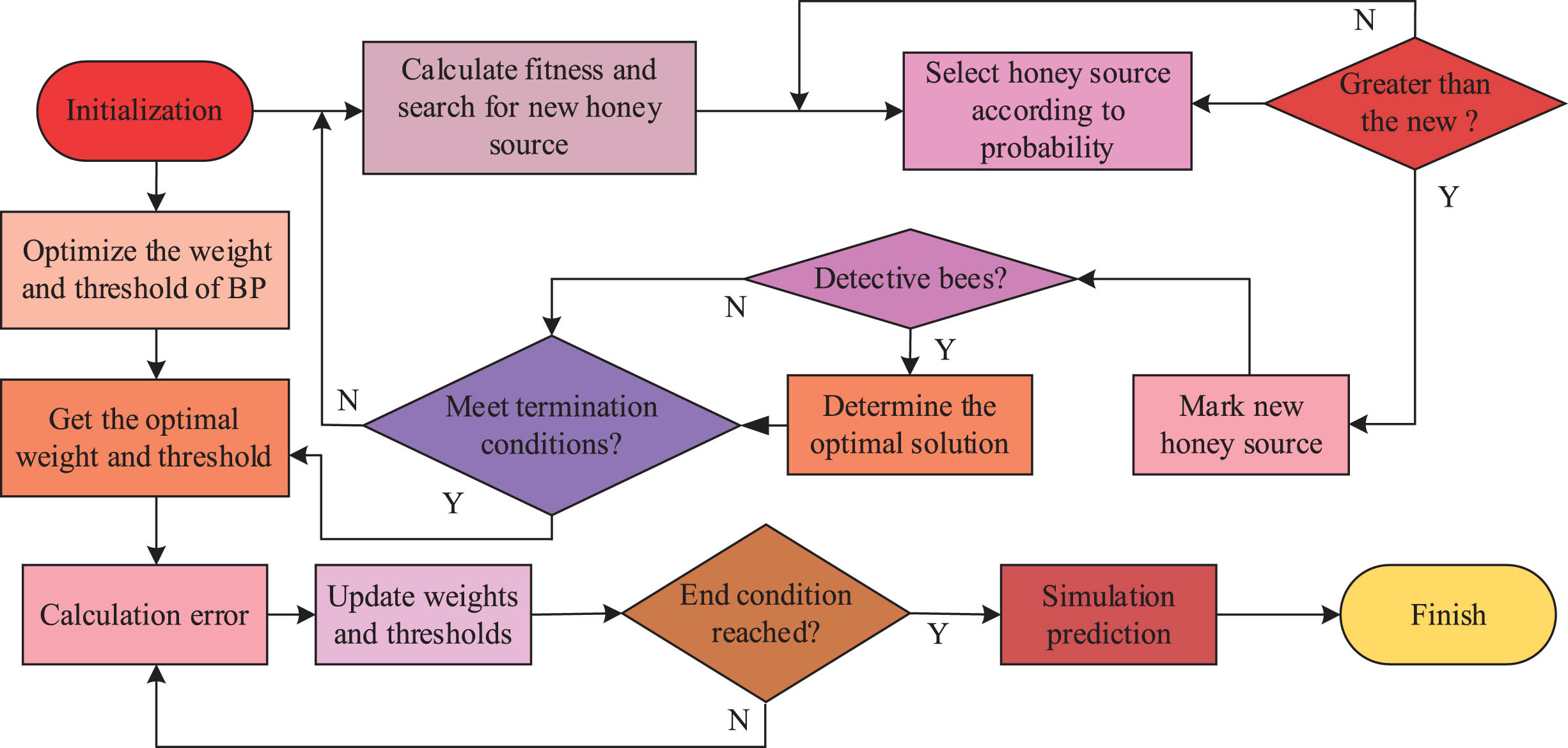
4Analysis of the evaluation results of core competence in talent cultivation based on CIPP model and improved BP neural network
Based on the establishment of the core competitiveness evaluation index system of public health personnel training, the study determines the index weights by the entropy value method and applies the IABC algorithm combined with the BP network in the core competitiveness evaluation of talents. Table 2 shows the weights of each index in the core competitiveness evaluation index system of public health personnel training. The weight of process evaluation is 0.449 and the weight of outcome evaluation is 0.283. Among the secondary indicators, the proportion of course construction and teaching quality assurance in the process evaluation indicators is 0.417, which proves the importance of teaching quality and course construction in the core competitiveness of public health personnel training, which is in line with the current direction of improving the quality of talent training.
Table 2
Weights of various indicators in the evaluation index system for core competitiveness of public health talent cultivation
Primary indicator | Weight | Secondary indicators | Weight |
A. Background evaluation | 0.106 | A1. Training objectives | 0.334 |
A2. Student Attraction | 0.142 | ||
A3. Social reputation | 0.524 | ||
B. Input comments | 0.164 | B1. Teacher construction | 0.748 |
B2. Educational support | 0.252 | ||
C. Process evaluation | 0.449 | C1. Curriculum construction | 0.417 |
C2. Teaching organization | 0.166 | ||
C3. Teaching quality assurance | 0.417 | ||
D. Result evaluation | 0.283 | D1. Honor Striving Power | 0.122 |
D2. Student Core Literacy | 0.320 | ||
D3. Graduate quality | 0.558 |
The study introduces a global optimal solution and an adaptive inertia factor to improve the ABC algorithm, resulting in the IABC algorithm. The performance of the IABC algorithm is first verified. The IABC algorithm was tested using four standard functions, Griewank, Rastrigin, Schaffer and Ackley, and compared with the Particle Swarm Optimization (PSO) algorithm and the ABC algorithm. To eliminate randomness, all four test functions were run 50 times. Fig. 7 displays the outcomes of the three algorithms’ optimization searches for the Griewank and Rastrigin functions. In Fig. 7(a), in the Griewank function, both the PSO and ABC algorithms fall into local extremes around 40 generations and experience a brief stagnation, while the IABC algorithm shows a linear decline within 5 generations and starts to stabilize after 35 iterations with good convergence performance. In Fig. 6(b), all three algorithms converge quickly within 10 generations in the Rastrigin function. Among them, the PSO algorithm leveled off around 20 generations, the ABC algorithm started to level off around 60 generations, while the IABC algorithm entered a stable state at 10 generations, each 10 and 40 generations ahead of the previous two methods, with better performance.
Fig. 7
Optimization Results of Three Algorithms in Griewank and Rastigin Functions.
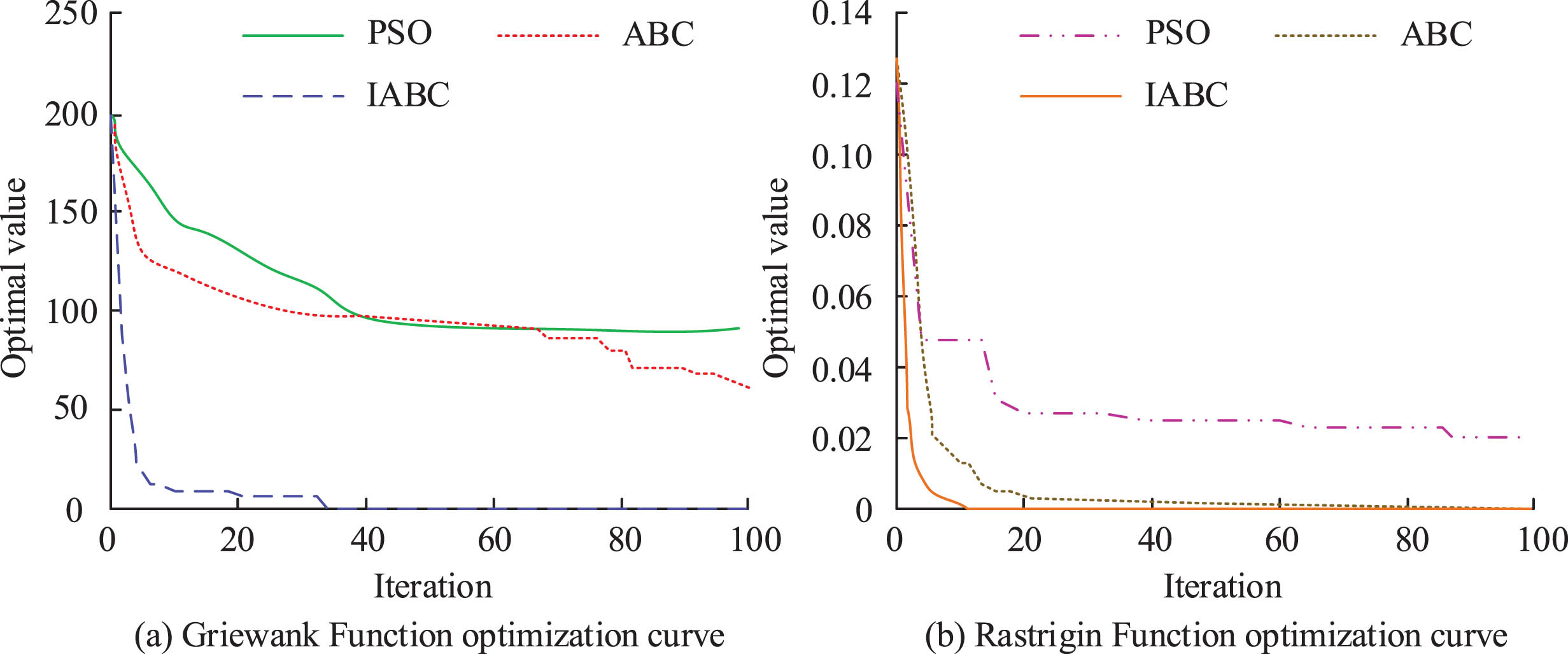
Figure 8 presents the optimization curves for the three techniques in the Schaffer and Ackley functions. Among the three methods, the PSO algorithm has the slowest convergence rate in the Schaffer function and still exhibits non-smoothness after 100 generations. The ABC algorithm converges after approximately 20 generations, while the IABC algorithm has a faster convergence rate in the early stages and reaches complete smoothness at three generations. From Fig. 8(b), it is evident that the Ackley function displays significant convergence in the early stages for all three methods. Notably, the IABC algorithm has a convergence rate that is less different from PSO, and all methods show a smoother convergence after roughly 21 generations. In contrast, the ABC algorithm only converges after 80 generations. Thus, it may be concluded that the IABC algorithm exhibits a more stable convergence rate and clear superior performance.
Fig. 8
Optimization Curves of Three Methods in Schaffer and Ackley Functions.
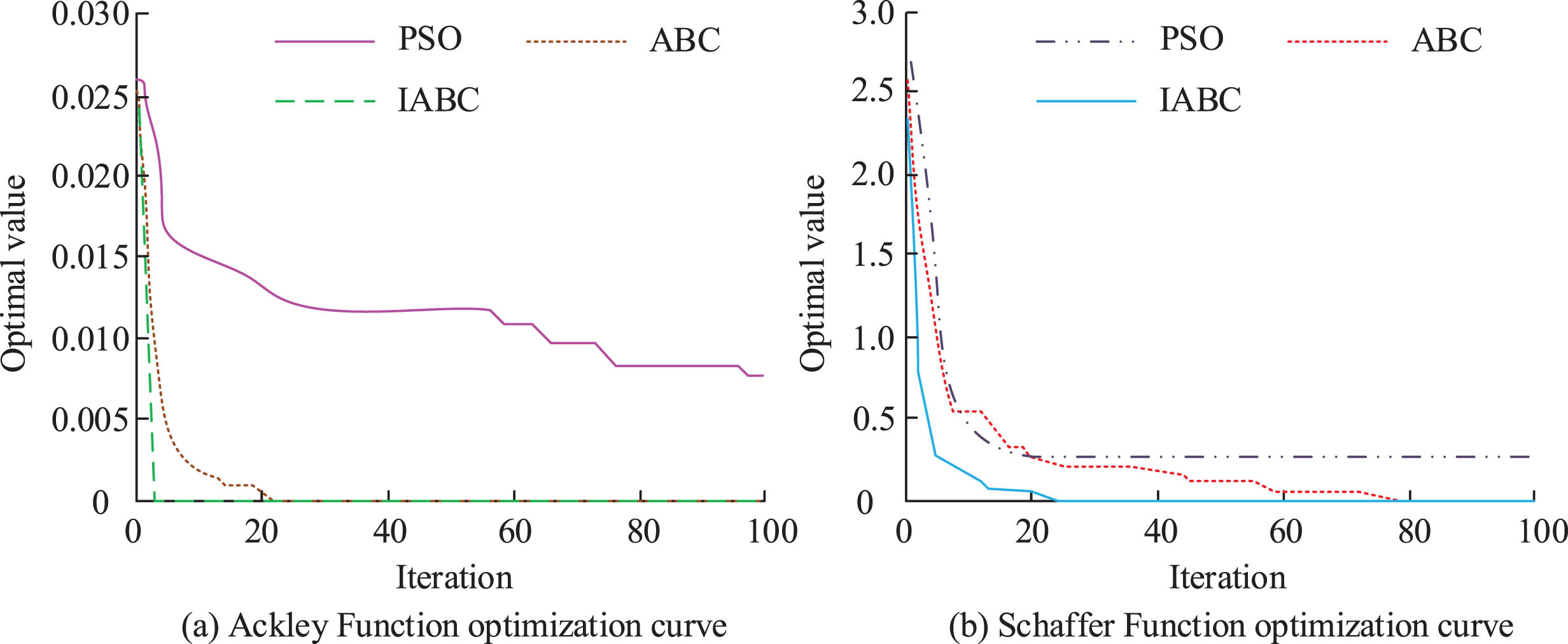
The study applied the improved IABC algorithm to BP network optimization, i.e. the proposed IABC-BP model. To test its performance, it was compared with the GA-BP (BP Neural Network based on Genetic Algorithm) and the mean square error (MSE) obtained is shown in Fig. 9. From Fig. 9(a), the GA-BP converges relatively quickly in the early stage and reaches the target accuracy in 115 iterations with an MSE value of about 10-8. In Fig. 9(b), the proposed IABC-BP model reaches the target accuracy in only about 11 iterations and the optimal value appears in 16 iterations with an MSE value of 10–13. Compared to GA-BP, IABC-BP improves the performance by 99%. IABC-BP improves by 99 iterations and has a significantly lower MSE value with higher accuracy.
Fig. 9
Comparison results of mean square error between the proposed IABC-BP model and GA-BP.
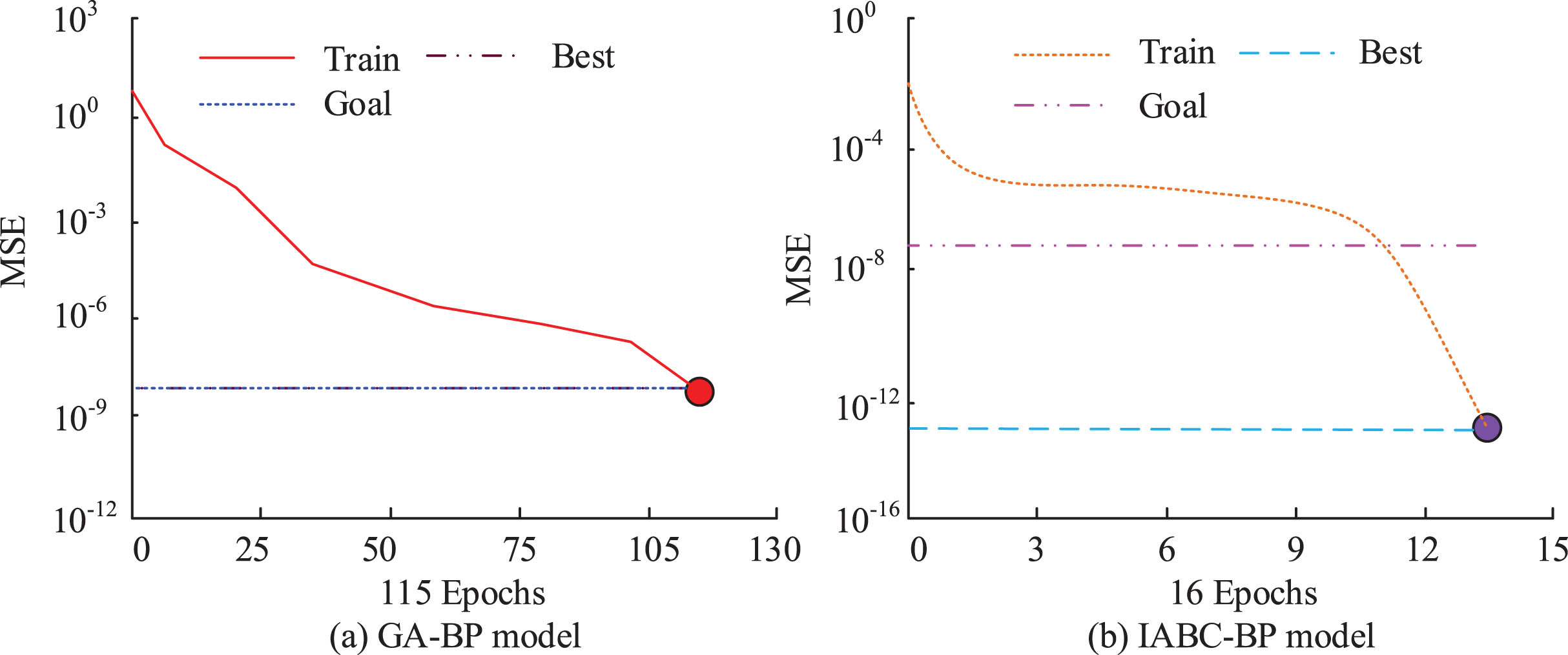
Finally, the proposed IABC-BP model was applied to the core competitiveness evaluation index system of public health personnel training for evaluation and compared with BP network and GA-BP, and the results obtained are shown in Fig. 10. According to Fig. 10, the evaluation accuracy of all three methods fluctuated to different degrees as the sample data of public health personnel increased. The IABC-BP model, on the other hand, was over 90% overall and up to 97%, with an improvement range of 2–14% compared with the GA-BP model, indicating that the proposed IABC-BP model can effectively improve the evaluation accuracy.
Fig. 10
Prediction accuracy of BP network, GA-BP, and IABC-BP models in the core competitiveness evaluation index system of public health talent cultivation.
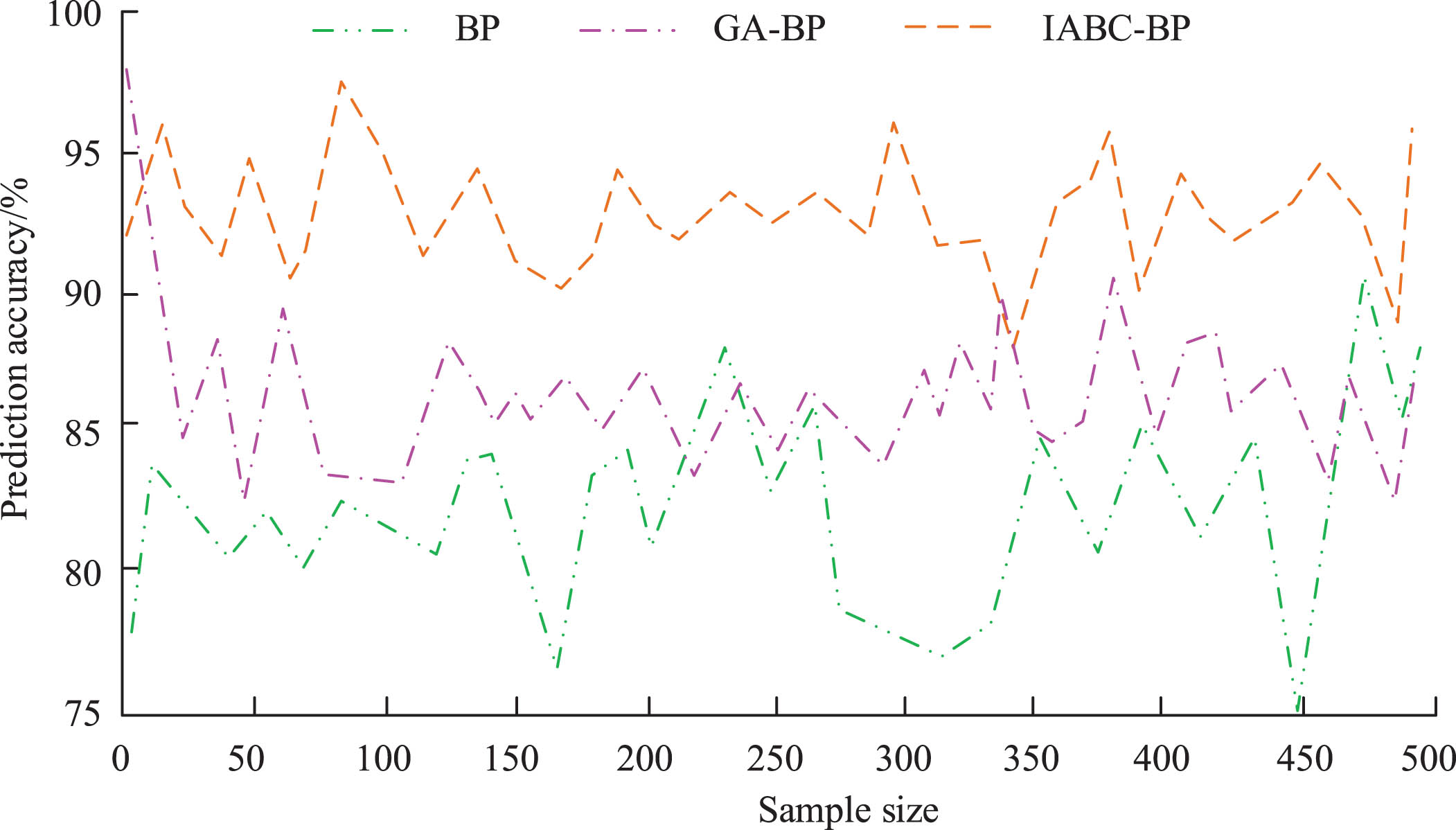
To further verify the practical application effect of the IABC-BP model and the core competitiveness evaluation index system for public health personnel training, it was applied to public health training programs in 10 universities in China and tested through joint evaluation by teachers and students, and the results obtained are shown in Fig. 11. As can be seen from Fig. 11, before the application of the method, the training quality ratings of students and teachers were concentrated between 75 and 85 points, accounting for about 80%. After the application of the method, nearly 50% of the students and teachers scored above 90 in the quality of core skills, and basically exceeded 80, indicating that the method can effectively improve the level of talent training.
Fig. 11
Application effect of IABC-BP model and evaluation system in public health training programs in 10 domestic universities.
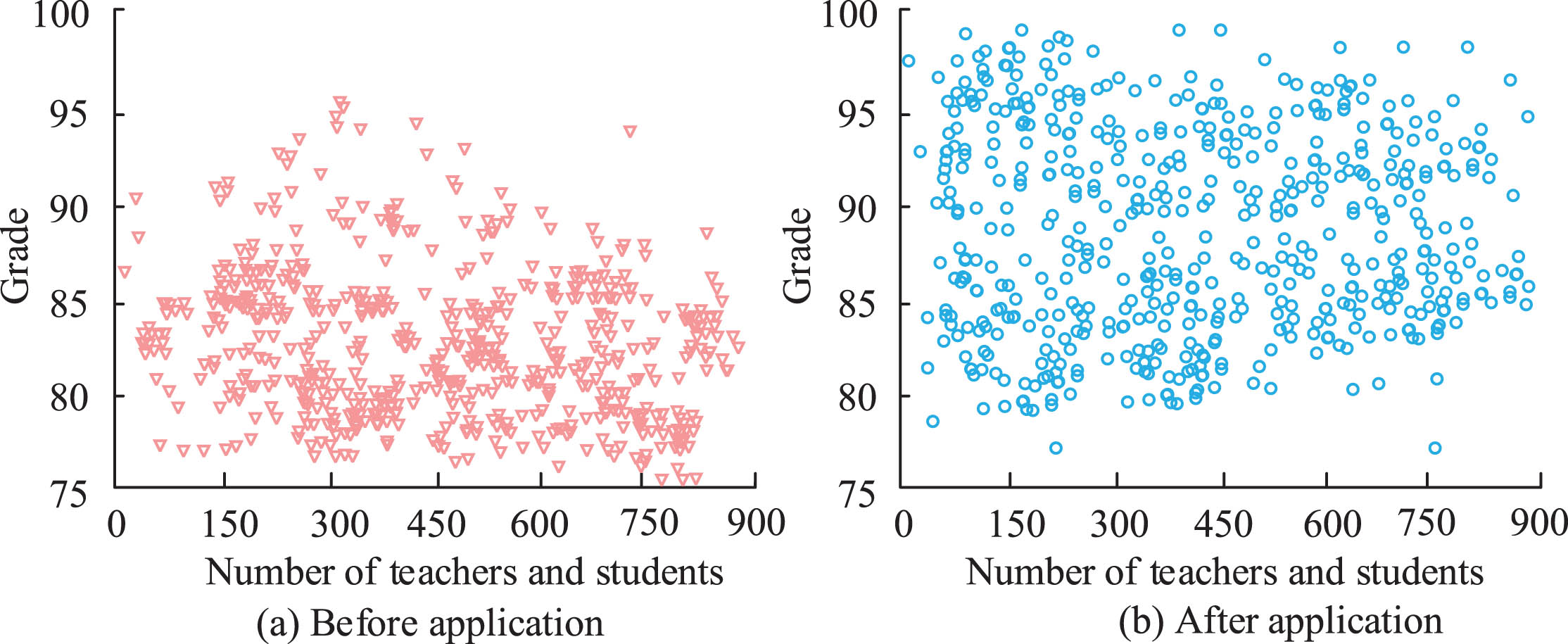
5Discussion
There are numerous gaps in the existing literature concerning the establishment of evaluation index systems for the core competitiveness of public health talent development, including the absence of scientific and standardized evaluation index systems, the completeness and systematization of indicators, and the determination of weights [35]. Therefore, this study selected the CIPP model, improved artificial bee colony (ABC) algorithm, BP neural network, and entropy method as the primary research techniques. The CIPP model ensures a comprehensive evaluation covering key points and steps, reflecting the effectiveness and problems of cultivating. The improved ABC algorithm enhances evaluation accuracy and efficiency, handling multi-dimensional and multi-indicator results. The BP neural network predicts the impact of evaluation indicators on talent cultivation effectiveness. The entropy method eliminates subjectivity, creating objective and fair evaluation results [36, 37].
The model was constructed by selecting environmental, input, process, and product factors as variables through an analysis of experimental results. Additional research has also examined these variables. Environmental factors are crucial determinants of the direction and objectives of cultivating public health talent. They reflect the level of alignment between training efforts and societal demands. The investment factor serves as the cornerstone for achieving high-quality talent cultivation by determining the quality and scale of investment. Process factors play a significant role in the effectiveness and efficiency of training, as they form a critical link connecting inputs to outputs. Finally, the product factor serves as the most direct measure of the effectiveness of public health talent cultivation. It is also an essential benchmark for ultimately assessing the success of cultivating talent.
The aforementioned results demonstrate the validation of the IABC algorithm’s performance across four standard functions and its comparison with both Particle Swarm Optimization (PSO) and ABC algorithms. The IABC algorithm exhibits swift convergence and commendable stability in the Griewank and Rastigin functions. Furthermore, in the Ackley and Schaffer functions, IABC outperforms PSO and ABC in regards to convergence speed and stability. These findings strongly endorse the supremacy of the IABC algorithm in addressing intricate optimization issues. The IAABC-optimized BP neural network also demonstrated remarkable performance while contrasting with the BP neural network based on the genetic algorithm. Particularly regarding MSE, the IABC-BP model accomplishes the intended accuracy within a small number of iterations, and the MSE magnitude is less than GA-BP. The aforementioned demonstrates that the IABC-BP model outperforms in complex problem solving with greater accuracy. Applying the IABC-BP model to assess the fundamental competitiveness of public health talent training yields a higher evaluation accuracy compared to the BP network and GA-BP. As the sample data on public health talent increases, the IABC-BP model demonstrates a consistently high evaluation accuracy, highlighting its proficiency in processing big data. The IABC-BP model and the evaluation index system for the core competitiveness of cultivating public health talent were used in practical scenarios to assess the training programs for public health provided by 10 universities. The outcomes received affirmative feedback too. The application of this method has resulted in significant improvements in both student and teacher scores, highlighting its efficacy in enhancing talent cultivation. The research outcomes underscore the crucial role of the evaluation index system in developing the core competitiveness of public health talent cultivation. The process evaluation received the highest rating, indicating the significance of curriculum development and quality assurance in teaching. These results align with the ongoing effort to enhance educational quality by prioritizing sustained attention and substantial investments in the teaching process.
6Conclusion
Public health training of human resources is an important element in the further optimization of the public health system. This study established the evaluation index system for assessing the core competencies of public health personnel training through the CIPP model. Additionally, the IABC algorithm was developed using the global optimal solution and the adaptive inertia factor, which was later combined with the BP network to assess the core competencies of staff training. The results showed that in the Griewank function, the IABC algorithm showed a linear decreasing trend within 5 generations and started to stabilize after 35 iterations with good convergence performance. In the Rastrigin function, the IABC algorithm entered a stable state at 10 generations, which was 10 and 40 generations ahead of the PSO and ABC algorithms respectively. In the Schaffer and Ackley functions, the IABC algorithm plateaus at 3 and 21 generations respectively. Combined with BP network, it improved by 99 generations compared with GA-BP, and the MSE value decreased significantly, with the lowest MSE value of 10–13. In the talent training core competitiveness evaluation index system, the overall accuracy of the method was above 90%, while the core competitiveness quality scores of students and teachers basically exceeded 80 points, which effectively improved the level of enhancing talent training.
The research results offer three significant contributions to the existing knowledge system. Firstly, a novel evaluation index system for public health talent cultivation’s core competitiveness, encompassing crucial factors like teaching quality and curriculum construction, is proposed. This system provides an innovative viewpoint and tool for evaluating and cultivating talent in the public health sector. The second aspect involves introducing and enhancing the Artificial Bee Colony Algorithm (IABC), which is integrated with the BP network to create a new model: the IABC-BP model. This model outperforms other typical optimization algorithms in numerous standard function tests, offering a fresh approach to tackling intricate problems and optimizing complex networks. The third objective is to conduct empirical testing of the IABC-BP model’s application effect in evaluating the core competitiveness of public health talent development. This provides some evidence of the model’s efficacy and usefulness.
Research offers scientific readers a novel perspective and an array of tools to evaluate and comprehend the quality of public health talent cultivation in the fields of public health and education. Moreover, the study highlights the enhanced IABC algorithm and its optimization performance coupled with BP networks, which presents new avenues for the exploration of optimization techniques for readers in computer science, artificial intelligence, and optimization algorithms.
6.1Implications
The research findings have made a notable contribution to various domains, including education policies and the education of public health professionals. With regards to education policy, the research has emphasized the need for process evaluation to develop competent public health practitioners. This will likely influence education policy makers to increase investment in curriculum design and teaching quality. Regarding education for public health professionals, the IABC-BP model suggests increased accuracy in evaluating processes, leading to changes in educational practices and an improvement in the quality of public health talent development.
6.2Limitations
The study has demonstrated positive research findings, yet some limitations remain. The examination of the key competencies involved in the cultivation of public health personnel utilized a novel IABC-BP model, however, the efficacy of this model is primarily reliant on the testing of limited sample data and algorithm functions. Further research is necessary to explore the performance of this model in dealing with more intricate practical issues and larger sets of data. In the section on empirical validation, the study evaluated only 10 domestic universities with a relatively small sample size. Differences in evaluation results across regions and types of universities may exist. Therefore, future research should focus on improving the IABC-BP model for managing complex issues and large-scale data, while increasing its applicability to evaluating talent cultivation in public health and other domains. To comprehensively evaluate the performance of the IABC-BP model in differing environments, it is recommended that the sample range be expanded to incorporate more regions and types of universities.
6.3Policy recommendations
Based on the results above, the study proposes some feasible policy recommendations. The initial recommendation is to emphasize curriculum development and improve teaching standards. The research indicates that process evaluation holds the greatest weightage, and teaching quality and curriculum development also hold high relative weightages. Therefore, it is imperative for the education sector to prioritize the development of the curriculum and teaching quality in the public health teaching process, with the local government providing the necessary financial support. Additionally, it is recommended to broaden the scope of the pilot program and carry out more extensive trials of the evaluation system and models in the research, incorporating universities of varying levels and types. To enhance the effectiveness and relevance of this approach, this study aims to validate it while identifying potential areas for improvement. Additionally, introducing public evaluation, including students, parents, and various sectors within society, can ensure comprehensive and diverse evaluation of the cultivation of public health talent.
Acknowledgments
None.
References
[1] | Asgari I , Karimi S , Charmdooz N , Tahani B . Competencies of dental public health for undergraduate students and their self-perceived achievements: The case of Iran. European Journal of Dental Education. (2022) ;26: (4):767–80. DOI: 10.1111/eje.12759 |
[2] | Dai Y , Wu J , Fan Y , Wang J , Niu J , Gu F , Shen S . MSEva: A musculoskeletal rehabilitation evaluation system based on EMG signals. ACM Transactions on Sensor Networks. (2022) ;19: (1):1–23. DOI: 10.1145/3522739 |
[3] | Jamieson S . State of the science: quality improvement of medical curricula—How should we approach it? Medical Education. (2023) ;57: (1):49–56. DOI: 10.1111/medu.14912 |
[4] | Indriani F , Atiaturrahmaniah A . Evaluation of the implementation of integrative thematic learning: A qualitative research approach phenomenology. Jurnal Penelitian dan Evaluasi Pendidikan. (2019) ;23: (2):184–96. DOI: 10.21831/pev23i2.27431 |
[5] | Liu Q , Qu X , Wang D , Mubeen R . Product Market Competition and Firm Performance: Business Survival Through Innovation and Entrepreneurial Orientation Amid COVID-19 Financial Crisis. Front Psychol. (2021) ;12: :790923. DOI: 10.3389/fpsyg.2021.790923 |
[6] | Ge T , Ullah R , Abbas A , Sadiq I , Zhang R . Women’s Entrepreneurial Contribution to Family Income: Innovative Technologies Promote Females’ Entrepreneurship Amid COVID-19 Crisis. Front Psychol. (2022) ;13: :828040. DOI: 10.3389/fpsyg.2022.828040 |
[7] | Hafeez A , Dangel WJ , Ostrof SM , Kiani AG , Glenn SD , Abbas J , ... Mokdad AH . The state of health in Pakistan and its provinces and territories, 1990-2019: a systematic analysis for the Global Burden of Disease Study 2019. The Lancet Global Health. (2023) ;11: (2):e229–43. DOI: 10.1016/S2214-109X(22)00497-1 |
[8] | Al-Sulaiti K , Lorente DB , Shah SAR , Shahzad U . Reset the industry redux through corporate social responsibility: The COVID-19 tourism impact on hospitality firms through business model innovation. In Economic Growth and Environmental Quality in a Post-Pandemic World. (2022) ;1: :177–201. Routledge. DOI: 10 |
[9] | Sukarni S . The Context, Input, Process, and Product Evaluation Model on English Language Teaching at Public Health Study Program. IJECA (International Journal of Education and Curriculum Application). (2022) ;5: (3):241–9. DOI: 10.31764/ijeca.v5i3.10318 |
[10] | Setyadi F , Hidayatullah MF , Purnama SK . The evaluation of physical health and sport education program at SMA Negeri 2 Ngawi using the CIPP (context, input, process, product) evaluation model. International Journal of Humanities and Education Development (IJHED). (2021) ;3: (6):66–77. DOI: 10.22161/jhed.3.6.8 |
[11] | Rachmaniar R , Yahya M , Lamada M . Evaluation of Learning through Work Practices Industry Program at University with the CIPP Model Approach. International Journal of Environment, Engineering and Education. (2021) ;3: (2):59–68. DOI: 10.55151/ijeedu.v3i2.55 |
[12] | Aman A , Setiawan R , Prasojo LD , Mehta K . Evaluation of hybrid learning in college using CIPP model. Jurnal Penelitian dan Evaluasi Pendidikan. (2021) ;25: (2):218–31. DOI: 10.21831/pev25i2.46348 |
[13] | Thaitami SH , Giatman G , Ambiyar A , Jalinus N , Syahril S , Wakhinuddin W . Model Context, Input, Process, and Product (CIPP) pada Career Development Center (CDC) untuk Evaluasi Program Layanan Informasi Karir SMK. Mimbar Ilmu. (2021) ;26: (2):309–14. DOI: 10.23887/mi.v26i2.36226 |
[14] | Wu ZJ , Tian S , Ma L . A 4D trajectory prediction model based on the BP neural network. Journal of Intelligent Systems. (2019) ;29: (1):1545–57. DOI: 10.1515/jisys-2019-0077 |
[15] | Liang Y , Ren C , Wang HY , Huang YB , Zheng ZT . Research on soil moisture inversion method based on GA-BP neural network model. International journal of remote sensing. (2019) ;40: (5–6):2087–103. DOI: 10.1080/01431161.2018.1484961 |
[16] | Xia W , Neware R , Kumar SD , Karras DA , Rizwan A . An optimization technique for intrusion detection of industrial control network vulnerabilities based on BP neural network. International Journal of System Assurance Engineering and Management. (2022) ;13: (Suppl 1):576–82. DOI: 10.1007/s13198-021-01541-w |
[17] | Abellán García J , Fernandez Gomez J , Torres Castellanos N . Properties prediction of environmentally friendly ultra-high-performance concrete using artificial neural networks. European Journal of Environmental and Civil Engineering. (2022) ;26: (6):2319–43. DOI: 10.1080/19648189.2020.1762749 |
[18] | Samuel OD , Okwu MO . Comparison of Response Surface Methodology (RSM) and Artificial Neural Network (ANN) in modelling of waste coconut oil ethyl esters production. Energy Sources, Part A: Recovery, Utilization, and Environmental Effects. (2019) ;41: (9):1049–61. DOI: 10.1080/15567036.2018.1539138 |
[19] | Geng J , Ul Haq S , Ye H , Shahbaz P , Abbas A , Cai Y . Survival in Pandemic Times: Managing Energy Efficiency, Food Diversity, and Sustainable Practices of Nutrient Intake amid COVID-19 Crisis. Frontiers in Environmental Science. (2022) ;13: :945774. DOI: 10.3389/fenvs.2022.945774 |
[20] | Hafeez A , Dangel WJ , Ostroff SM , Kiani AG , Glenn SD , Abbas J , ... Mokdad AH . The state of health in Pakistan and its provinces and territories, 1990-2019: a systematic analysis for the Global Burden of Disease Study 2019. The Lancet Global Health. (2023) ;11: (2):e229–43. DOI: 10.1016/S2214-109X(22)00497-1 |
[21] | Shah SAR , Zhang Q , Abbas J , Tang H , Al-Sulaiti KI . Waste management, quality of life and natural resources utilization matter for renewable electricity generation: The main and moderate role of environmental policy. Utilities Policy. (2023) ;82: :101584. DOI: 10.1016/j.ju2023.101584 |
[22] | Iorember PT , Iormom B , Jato TP , Abbas J . Understanding the bearable link between ecology and health outcomes: the criticality of human capital development and energy use. Heliyon. (2022) ;8: (12):e12611. DOI: 10.1016/j.heliyon.2022.e12611 |
[23] | Li Y , Al-Sulaiti K , Dongling W , Al-Sulaiti I . Tax Avoidance Culture and Employees’ Behavior Affect Sustainable Business Performance: The Moderating Role of Corporate Social Responsibility. Frontiers in Environmental Science. (2022) ;10: :1081. DOI: 10.3389/fenvs.2022.964410 |
[24] | Yao J , Ziapour A , Toraji R , NeJhaddadgar N . Assessing puberty-related health needs among 10–15-year-old boys: A cross-sectional study approach. Archives de Pédiatrie. (2022) ;29: (4):307–11. DOI: 10.1016/j.arcped.2021.11.018 |
[25] | Li X , Dongling W , Baig NUA , Zhang R . From Cultural Tourism to Social Entrepreneurship: Role of Social Value Creation for Environmental Sustainability. Front Psychol. (2022) ;13: :925768. DOI: 10.3389/fpsyg.2022.925768 |
[26] | Yao J , Ziapour A , Toraji R , NeJhaddadgar N . Assessing puberty-related health needs among 10-15-year-old boys: A cross-sectional study approach. Archives de Pédiatrie. (2022) ;29: (2):307–11. DOI: S0929693X2200001X |
[27] | Lan W , Yang X , Gong T , Xie J . Predicting the shelf life of Trachinotus ovatus during frozen storage using a back propagation (BP) neural network model. Aquaculture and Fisheries. (2023) ;8: (5):544–50. DOI: 10.1016/j.aaf.2021.12.016 |
[28] | Sofiawati ET , Suryadi BS . Development Of Education Quality Assurance In Model School. Journal of Positive School Psychology. (2022) ;6: (8):5802–5810. DOI: 10.1080/14681811.2021.1908984 |
[29] | Li STT , Klein MD , Balmer DF , Gusic ME . Scholarly evaluation of curricula and educational programs: using a systematic approach to produce publishable scholarship. Academic Pediatrics. (2020) ;20: (8):1083–93. DOI: 10.1016/j.aca2020.07.005 |
[30] | Li X , Wang J , Yang C . Risk prediction in financial management of listed companies based on optimized BP neural network under digital economy. Neural Computing and Applications. (2023) ;35: (3):2045–58. DOI: 10.1016/j.uclim.2021.101078 |
[31] | Gao J . Performance evaluation of manufacturing collaborative logistics based on BP neural network and rough set. Neural Computing and Applications. (2021) ;33: (2):739–54. DOI: 10.1007/s00521-020-05099-9 |
[32] | Rocha AC , Silva M , Duarte C . How is sexuality education for adolescents evaluated? A systematic review based on the Context, Input, Process and Product (CIPP) model. Sex Education. (2022) ;22: (2):198–216. DOI: 10.1080/14681811.2021.1908984 |
[33] | Chen L , Jagota V , Kumar A . RETRACTED ARTICLE: Research on optimization of scientific research performance management based on BP neural network. International Journal of System Assurance Engineering and Management. (2023) ;14: (1):489. DOI: 10.1007/s13198-021-01263-z |
[34] | Agustina NQ , Mukhtaruddin F . The CIPP Model-Based Evaluation on Integrated English Learning (IEL) Program at Language Center. English Language Teaching Educational Journal. (2019) ;2: (1):22–31. DOI: 10.12928/eltej.v2i1.1043 |
[35] | Geng J , Ul Haq S , Ye H , Shahbaz P , Abbas A , Cai Y . Survival in Pandemic Times: Managing Energy Efficiency, Food Diversity, and Sustainable Practices of Nutrient Intake amid COVID-19 Crisis. Frontiers in Environmental Science. (2022) ;13: :945774. DOI: 10.3389/fenvs.2022.945774 |
[36] | Zhuang D , Al-Sulaiti K , Fahlevi M , Aljuaid M , Saniuk S . Land-use and food security in energy transition: Role of food supply. Frontiers in Sustainable Food Systems. (2022) ;6. DOI: 10.3389/fsufs.2022.1053031 |
[37] | Iorember PT , Iormom B , Jato TP , Abbas J . Understanding the bearable link between ecology and health outcomes: the criticality of human capital development and energy use. Heliyon. (2022) ;8: (12):e12611. DOI: 10.1016/j.heliyon.2022.e12611 |