Psychometric assessment of organizational readiness scale for digital innovations and antecedents of organizational readiness
Abstract
BACKGROUND:
This article aims to perform a psychometric assessment of the scale of organizational readiness for digital innovations in a transition economy and to examine the antecedents of organizational readiness for digital innovations.
METHODOLOGY:
The study employed a quantitative research method to analyze data collected from a sample of 1236 health professionals. The scale secondary confirmatory factor and linear regression analysis were employed to verify organizational readiness and test the respective hypotheses about organizational readiness for digital innovation, respectively.
RESULTS/CONCLUSIONS:
The research findings show that the organizational readiness scale for digital innovations is valid and reliable in transition economies. Findings show that the relationship between variables such as adaptation of human resources (AHR), cognitive readiness (COR), planning for new telehealth and e-health (PNTH), IT readiness (ITR), resource readiness (RR), partnership readiness (PR), and cultural readiness (CUR) are correlated with the innovations implementation effectiveness (IIE), and organizational readiness for digital innovation is positive statistically significant. Findings also suggest that Integration of old technologies (IoT) and organizational readiness for digital innovation is statistically significant and have negative relationship.
Besarta Taganoviq is a Ph. D candidate in Nursing Care program in University of Maribor, Slovenia. Her research interests are in field of women care, emotional intelligence and labour, digital nursing services, caesarean sections, e-health, evidence-based practice and evidence informed practice. She is the dean of Nursing program, bachelor and master level in Heimerer College.
Mehmet Bağış received his Ph.D. from Sakarya University Business Institute in 2018. Since 2022 he has been an Associate Professor at Sakarya University of Applied Sciences, Faculty of Applied Sciences, Department of International Trade and Finance. His professional interests include strategic management, entrepreneurship, resource-based view, dynamic capabilities, behavioral strategy, and competitive strategy.
Mehmet Nurullah Kurutkan (MNK) graduated from Sakarya University, Labour Economics and Industrial Relations, Sakarya, Turkey in 2000; received his Master’s degree in Labour Economics and Industrial Relations from Sakarya University, Turkey in 2003, and his Ph.D. degree in Labour Economics and Industrial Relations Sakarya University, Sakarya, Turkey in 2010. He authored 50 articles, 11 books, and five chapters in Turkish and English. MNK is a full-time academic member of Duzce University, Faculty of Healthcare Management (2011– present). His professional interests mainly include Risk Management, Health Economics, Professionalism, Patient Safety, and Lean Healthcare.”
Adnan Hoxha acts as Vice Chancellor for Projects and International Cooperation. He is a PhD candidate in Economics, majoring in Entrepreneurship. Previously, he completed his master’s studies in Business Sciences and Economics at SKEMA Business School, Sofia Antipolis, France, as a researcher of the “Young Cell” Program of the European Commission and the Government of Kosovo, and his bachelor’s studies with a major in Economics and Management, and majoring in Public Policy at the Rochester Institute of Technology (RIT), NY, USA.
Arben Hysenaj is a Ph D candidate at Bamberg University, Germany. His intrests areas are: Migration and mental health Trauma Depression Cognition Salutogenesis. He is working as Dean of Faculty of Social and Psychological Science at Heimerer College, Kosovo.
Blerta Kryeziu is a Ph D candidate in Nursing program at University of Maribor, Slovenia. Her interests’ areas are: education in health care, evidence-based practice, women’s care. She is engaged as coordinator for teaching in Nursing program at Heimerer college and at the same time she is teaching assistant in Nursing program in University of Hasan Prishtina, Kosovo.
Linda Ukimeraj Harris has obtained her PhD in Ljubljana University, Slovenia. She is working as Vice Rector for Quality Office in Heimerer College. Her interests’ fields are: Education Management, Quality Assurance in Higher Education.
Erza Haxhijakupi has obtained her bachelor degree in Speech therapy at Heimerer College. Her interests’ areas are: dyslexia, e-health, multilinguist, VR and AR education and services. Erza’s working position is assistant for international cooperation and projects in Heimerer College.
Altina Bimbashi has a bachelor’s degree in the field of Speech and Language Therapy. She is currently pursuing a master’s degree in Cognitive Neuropsychology at CITY College, University of York Europe Campus. Altina was previously engaged as a research assistant at Heimerer College, where she currently also serves as an external research associate. While at ISEE-HSP, she is engaged in the role of Program Officer for Training and Research.
Blerte Hyseni studies Ph D in Nursing Program in Maribor University, Slovenia. She is working as teaching assistant in Nursing program University of Fehmi Agani Gjakova, Kosovo. Her areas of interests are: nurses’ burnout, job satisfaction...
Arta Dalipi studies PhD in Nursing program in Maribor University, Slovenia. She is working as teaching assistant in Nursing program University of Fehmi Agani Gjakova, Kosovo. Her areas of interests are: geriatric care, daily activities of elderly people, quality of live...
1Introduction
Healthcare organizations have launched organizational changes to reduce costs, improve quality and market share, increase efficiency and patient satisfaction, maintain valuable employees [3]. Among the changes that healthcare organizations have been focused on is adapting technology by maximizing the advantages that digitalization and the internet have brought to provide better health services [4]. E-health readiness refers to the extent to which healthcare organizations and health professionals are ready for the anticipated change through employing Information and Communications Technology (ICT) [1, 67].
E-health organizational readiness has attracted the attention of scholars and put the need for developing readiness assessment tools regarding e-health [5]. There are numerous advantages derived from e-health, both for health institutions and professionals [6], focusing on organizational readiness related to health services [7] and health professionals’ readiness [8]. Hence, e-health represents a significantly more efficient way of delivering healthcare services and accessing information regarding patients’ past and current levels of well-being. Undeniably, when converting from face-to-face to digital health (telemedicine, e-Health) services [9], the digital competencies of health professionals are essential to lower the damage to patients and errors [10]. However, there is limited research regarding organizational readiness in healthcare organizations, particularly in transition and low-income countries [11].
E-health is a new platform that aims to solve many health-related challenges by providing many advanced technological tools and devices [11]. The process of adoption and improvement of e-Health has significant policy [12] and managerial implications [13]. Organizational readiness for digital innovation refers to organizations’ readiness to innovate using the latest technology [14]. Healthcare professionals argue that organizational readiness is crucial for successful change implementation [3]. Organizational readiness refers to the changes in the health system that lead to digital innovation [4]. Furthermore, the extent to which shared beliefs for digital readiness among employees and managers determines the implementation process [13].
Organizational readiness has been on the agenda of different studies. Past research has provided a comprehensive organizational readiness framework by analyzing the literature on the necessary readiness factors for successful lean implementation in healthcare [65]. Researchers dealing with the subject in different contexts designed an e-health readiness assessment tool by performing an application in Iranian hospitals and used this tool to evaluate the readiness of employees for e-health applications [67]. The results of a study conducted in India identified, analyzed, and developed a model to measure the interactions between different factors of organizational readiness in the healthcare industry-specific service sector [69]. In a study conducted in Pakistan, e-health readiness assessment tools were developed for healthcare providers and managers in developing countries [1]. Research conducted in developed countries covering different sectors has developed a measurement tool for digital innovation consisting of seven structures (resource readiness, IT readiness, cognitive readiness, partnership readiness, innovation valance, cultural readiness, and strategic readiness) [2]. When these studies are examined, an organizational readiness scale and antecedents of organizational readiness are not found in transition economies.
The development and diffusion of digital technologies are assumed to improve and increase the innovation potential of most organizations. However, due to the lack of organizational readiness, it is tough for innovative activities to transform into new products and services [2, 58, 59]. Therefore, considering the effects of Covid-19 on health organizations and other organizations [60–64, 66, 99], it becomes more meaningful to examine whether health institutions are ready for innovation, especially in the post-Covid period. In addition, as a result of the calls made by the World Health Organization on adaptation to digital innovations in the e-health framework, practical tools are needed to measure the organizational readiness of the relevant countries. However, a standard tool has not been developed in this regard, and different tools are used in other countries for organizational readiness for e-health-related digital innovations [1, 2, 67, 68]. In particular, differences such as technological infrastructure, education level of employees, and cultural characteristics in developed, developing, and transition economies may not be appropriate to use tools developed in other countries.
This article aims to perform a psychometric assessment of the scale of organizational readiness for digital innovations in a transition economy and to examine the antecedents of organizational readiness for digital innovations. This study is carried out in the case of Kosovo, a low-income country characterized by weak institutional settings due to the slow process of institutional reforms [15, 47]. These countries face a lack of capabilities to manage and analyze technology. As a result, organizational readiness for e-health is influenced by factors at all levels [11]. In this framework, we have built the research on four parts, apart from the introduction. The second section covers the literature review and hypothesis development. The third section consists of the method. In the fourth section, the results of the research are presented. Finally, in the discussion section, administrative implications, policy recommendations, limitations of the study, and suggestions for future research are given.
2Literature review and hypothesis development
2.1Organizational readiness theory
Organizational readiness theory is based on: change valence, change efficacy, and factors related to the context [2, 3, 16]. Change valence refers to employees’ commitment to change. Change efficacy is how healthcare organizations perceive their ability to change [2, 17]. Contextual factors are related to the organizational atmosphere in the healthcare organization facilitating innovation-related change. Organizational readiness theory considers organizational readiness a shared psychological state where the employees feel committed to implementing organizational change and have the required collective skills and abilities [16]. Organizational readiness for change is a multi-level and multi-faceted construct, where readiness is evident depending on the nature of change at the individual, group, unit, department, or organizational level [16]. Thus, the extent these are crucial for health organizations to increase their e-health readiness refers to how healthcare organizations are prepared to anticipate change through programs related to ICT [1].
Moreover, health organizational readiness is related to three levels; macro-level (e.g., infrastructure, development policies), meso-level (organizational aspects), and micro-level (e.g., professionals) [18]. Digital readiness refers to a particular change where digital technologies play a crucial role. Yet, this may be interpreted based on context-specific organizations related to tech-specific barriers, attitudes, and capabilities [13]. The lack of readiness for digitalization in healthcare organizations is due to the weak health system at the national level, the lack of capability building, infrastructure for coordination, and increasing collaborations and commitment of policymakers [19], as well as the resistance of health professionals and their lack of readiness towards technology due to their lack of skills [4, 20]. In this regard, an investigation shows Kosovo health professionals show low readiness and trust in the digitalization of healthcare services [57].
2.2Hypothesis development
Integration of information technology in healthcare organizations improves efficiency and the quality of services [21], which process is influenced by the motivation of human resources and capabilities to implement organizational change [3]. Therefore, the adaptability of human resources for technical and organizational change is essential in delivering digital innovation [2]. Studies show the importance of HR adaptation to technology [22–24], which is influenced by technological literacy [25], the personality of human resources [13, 26, 47, 67], the role of managers [13, 27] the ability of benefit from technology depends on the extent HR use their technology as well [21, 28, 29]. Besides, HR that focuses on flexibility and cognitive readiness focuses on extended knowledge are found to be conducive factors for digital innovation [2, 14]. Subsequently, we propose the following hypotheses:
H1: Adapting human resources to technology (AHR) in healthcare organizations positively affects organizational readiness for digital innovation (ORFDI).
H2: In healthcare organizations, cognitive readiness (COR) positively affects organizational readiness for digital innovation.
Organizational readiness for digital innovation is influenced by resource readiness, IT readiness, combining existing technologies with new ones, and planning for new telehealth and e-health. Resource readiness refers to the organization’s flexibility to facilitate its digital innovation needs [14]. At the same time, IT readiness refers to the ability of healthcare organizations to foster digital innovation through IT infrastructure [2]. As for combining existing and new technologies, we define the increase in the speed and efficiency of healthcare organizations’ readiness for digital innovation. In addition, planning for new telehealth and e-health, ranging from simple to sophisticated bio-monitoring systems, refers to providing an additional information source about patients and better services [28].
Studies on resource readiness show relationships between resource readiness and organizational readiness for digital innovations. Lokuge [2, 14] argue that resource readiness influences organizational readiness for digital innovations. In another study, Hussain [17] supported the hypothesis that resource readiness affects digital financial innovation through its change efficacy. While studies on IT readiness suggest that IT readiness positively influences digital financial innovation [17], and implementing hardware and software positively influences e-health systems [11]. Other studies show that the barrier to IT readiness is the IT infrastructure [18, 31], while other studies show that the IT skills of health professionals may hinder IT readiness [11, 18], which then influences organizational readiness for digital innovation.
Planning new telehealth and e-health (PNTH) is crucial for health organizations which may lead to organizational readiness for digital innovation. Telehealth is essential to increase the monitoring of patients using devices that capture vital signs and other related healthcare information transmitted via technology [28]. Scholars maintain that the decision of managers to plan and adopt new telehealth technologies and e-health in which the adaptation of human resources is crucially essential in implementing telehealth [28]. This includes well-trained employees in healthcare information technology in increasing the ability to benefit from it [21]. The extent that human resources adapt to technology and the role that managers play in health organizations influence the extent that organization combines existing technologies with new ones. For example, if employees in health organizations adopt old technology, managers need to build a strategy to prepare the readiness of human resources for this. Thus, shared beliefs on digital readiness in management and employees are the source to guide and implement organizational change [13] by combining existing technologies with new ones that will affect organizational readiness for digital innovation. Based on the discussion above, we recommend the following hypothesis:
H3: Planning new telehealth and e-health (PNTH) technologies in healthcare organizations positively affects organizational readiness for digital innovation.
H4: Integrating old technologies (IOT) with new technologies in healthcare organizations positively affects organizational readiness for digital innovation.
H5: IT readiness (ITR) in healthcare organizations positively affects organizational readiness for digital innovation.
H6: Resource readiness (RR) in healthcare organizations positively affects organizational readiness for digital innovation.
Partnership readiness, cultural readiness, and effective implementation of innovations are also among the factors that affect the organizational readiness of healthcare organizations for digital innovation. Partnership readiness is the affiliation of stakeholders outside the organization who support digital innovation [2]. Studies acknowledge the importance of building partnerships and influencing organizational readiness for digital innovation [30, 32]. For example, Hussain’s [17] study shows that digital financial innovation is affected positively by partnership readiness. However, according to Lokuge [14], creating and preserving innovation readies are challenging for health organizations and external partners. To respond to such a challenge, before focusing on digital innovation, it is critical to ensure the acceptance of patterns and their engagement in ensuring e-health readiness [33]. In addition, studies show that mutual trust between client consultants helps improve project outputs and the knowledge transfer process [34]. Similarly, another study shows how organizations improve their knowledge pool through knowledge transfer using clients to improve IT performance and build organizational readiness [32].
Cultural readiness can be defined as health organizations’ advantages on their central values that lead to digital innovation [2, 65]. Scholars argue that organizational readiness is higher among organizations when all members have the culture to focus on implementing organizational change and the confidence to do so [3, 16]. It is related to the extent to which organization members share beliefs on digital empowerment and involvement, which is influenced by how managers act as role models to lead the organization toward digital readiness [13]. Thus, concerning change built within the organization and collective involvement, coordinated behavior change is found to have a crucial role [16]. The extent managers have decentralized the decision-making influences organizational readiness for digital innovation [14]. Studies show that cultural readiness positively influences digital financial innovation [17].
There is a significant correlation between organizational culture and digital capabilities and innovation [35], and organizational culture is crucial to developing the absorptive capacity that influences the implementation of new technologies [36]. The organizational readiness for digital innovation depends on the effectiveness of health organizations in implementing innovations, as 90% of ideas never come into implementation face due to the lack of readiness [14]. Increasing the effectiveness of organizational readiness is crucial that health organizations managers understand contextual factors within the organization [37], such as; resources, human resources, culture, decision-making, communication, and reward system [14]. Also, to increase healthcare organizations’ effectiveness, it is crucial to focus on developing knowledge management, coordinating and collaborating at inter-functional and inter-organizational levels, and building innovation infrastructure [38]. Based on the discussion above, we recommend the following hypothesis:
H7: Healthcare organizations’ partnership readiness (PR) positively affects organizational readiness for digital innovation.
H8: Healthcare organizations, cultural readiness (CUR) positively affects organizational readiness for digital innovation.
H9: Healthcare organizations’ innovations implementation effectiveness (IIE) positively affects organizational readiness for digital innovation.
3Methodology
We reviewed previous research to create and test the organizational readiness model. At the end of that phase, we identified eight critical dimensions of Khoja’s [1] study on e-health readiness assessment tools for healthcare institutions in developing countries (This scale consists of 21 questions). Then, we adopted the eight dimensions of the Lokuge [2] scale regarding organizational readiness for digital innovation, which consists of 26 questions. Therefore, the model we created consisted of a total of 16 dimensions. In this study, we combined the analysis of Khoja et al. [1] and Lokuge et al. [2]. The combined version of these two scales examines the organizational preparation process more broadly. Therefore, we performed a second-level confirmatory factor analysis.
Also, we added new questions of our own to each dimension. While creating the questions, we received help from experts operating in clinical and academic specializing in health and digitalization. All of the respective scales were translated from English to Albanian by the authors, and these translations were checked by translation and back-translation methods. Thus, the total number of questions in the survey was 54. Lastly, we arranged the survey questions on a 5-point Likert scale (from 1. Strongly Agree with 5. Strongly Disagree).
There are 7 Regional hospitals and 1 Kosovo University Clinical Center (UCCK) in Kosovo. The seven regional hospitals of Kosovo are Peja regional hospital, Gjakova Regional Hospital, Ferizaj Regional Hospital, Vushtri Regional Hospital, Mitrovica Regional Hospital, Gilan Regional Hospital, and Prizren Regional Hospital. The study occurred at the Kosovo University Clinical Center, the leading tertiary healthcare institution in Pristina (the capital), providing the most specialized medical services. This hospital consists of an Emergency Care Center and 12 Clinics. The hospital stands out for researching readiness for digital innovations, as it is the largest hospital in Kosovo, and the country’s health resources are directed to this hospital. For this reason, the study was conducted in this hospital, which is assumed to represent the general sample well.
Employees in Kosovo University Clinical Center were determined as the target group, and employees who could be reached by random sampling method were included in the analysis. Initially, we piloted the questionnaires. At this stage, we collected 50 questionnaires between 20.11.2021 and 30.11.2021 using the Google form. Then, we checked whether there were deficiencies in the collected data, whether data were entered other than the minimum and maximum values, and finally, whether all expressions were given the same points. After that, we eliminated the unsuitable ones. Then, we examined the factor loads of the data. After we saw that the factor loads were suitable for the analysis, we moved on to the second data collection stage.
The population of Kosovo is 1.66 million. Therefore, we collected 1,272 questionnaires assuming this population would be representative and using the hard copy method. The questionnaires were distributed to the relevant hospital employees and then collected back. Upon reviewing the questionnaires, we determined that 36 were unsuitable for analysis and did not include them in the study. We analyzed the data with SPSS 23 and AMOS 23. We performed demographic analysis, descriptive statistics, reliability analysis (Cronbach alpha), secondary confirmatory factor analysis, and linear regression analysis. Many publications inspired this study in the interpretation of method and analysis results [66, 82–84]. Therefore, we benefited from the above articles in this study, especially in interpreting methods and results (tables and graphs).
4Results
4.1Demographic characteristics of participants
As a result of the research, frequency, and percentage analysis were applied to the data, and findings related to the demographic characteristics of the participants were obtained. Table 1 shows that most participants were men (63.3). Approximately two-thirds of the participants declared they had completed at least undergraduate education. While 50.6% of the participants have a monthly income of 500 Euros or less, 38.9% have an income of 500–1000 Euros. As for the working experience, 38.7% were 0–5 years, and 18.1% had 21 years or more of work experience. As for occupation, 16.1% are non-nurse health personnel, 19.5% are doctors, 51% are nurses, and 13.4% are other personnel. 28.7% are between the ages of 17–26.
Table 1
Demographic information of the participants
Gender | Frequency | % | Work experience | Frequency | % |
Female | 453 | 36,7 | 0–5 year | 478 | 38,7 |
Male | 783 | 63,3 | 6–10 year | 240 | 19,4 |
Total | 1236 | 100 | 11–15 year | 165 | 13,3 |
Level of education | Frequency | % | 16–20 year | 129 | 10,4 |
High school | 298 | 24,1 | 21 and up | 224 | 18,1 |
License | 546 | 44,2 | Total | 1236 | 100 |
Master | 257 | 20,8 | Profession | Frequency | % |
Doctorate | 135 | 10,9 | Non-nurse health personnel | 199 | 16,1 |
Total | 1236 | 100 | Doctor | 241 | 19,5 |
Total monthly income (Euro) | Frequency | % | Nurse | 630 | 51 |
0–500 | 625 | 50,6 | Other | 166 | 13,4 |
501–1000 | 481 | 38,9 | Total | 1236 | 100 |
1001–2000 | 104 | 8,4 | Age | Frequency | % |
2001–4000 | 14 | 1,1 | 17–26 | 355 | 28,7 |
4001 and up | 12 | 1 | 27–35 | 303 | 24,5 |
Total | 1236 | 100 | 36–44 | 242 | 19,6 |
45 and up | 336 | 27,2 | |||
Total | 1236 | 100 |
4.2Descriptive statistics on research data
The skewness and kurtosis coefficients of all expressions in the scale were examined to determine whether the variables included in the study showed a normal distribution. It was observed that the skewness and kurtosis coefficients of the data set were within the expected values (skewness <2, Kurtosis <7) [39, 40] (see Table 2). Accordingly, when the results of the normality test of the data are examined, it can be said that the data show a normal distribution.
Table 2
Factor loads and descriptive statistics
Variables | Factor Loads | Cronbach Alpha | AVE | CR | Mean | SD | Skewness | Kurtosis |
Identification of Needs for future changes Telehealth/e-health | 0,811 | 0,881 | 0,654 | 0,883 | 3,198 | 1,173 | –0,114 | –0,805 |
0,84 | 3,233 | 1,0864 | –0,1 | –0,664 | ||||
0,81 | 3,281 | 1,1739 | –0,17 | –0,797 | ||||
0,772 | 3,277 | 1,168 | –0,217 | –0,756 | ||||
Awareness about telehealth/e-health | 0,83 | 0,865 | 0,686 | 0,867 | 3,216 | 1,1513 | –0,212 | –0,787 |
0,859 | 3,192 | 1,0817 | –0,108 | –0,688 | ||||
0,794 | 3,306 | 1,1088 | –0,199 | –0,654 | ||||
Comfort with technology | 0,774 | 0,838 | 0,638 | 0,841 | 3,186 | 1,1064 | –0,265 | –0,618 |
0,839 | 3,291 | 1,1682 | –0,285 | –0,789 | ||||
0,781 | 3,273 | 1,1915 | –0,265 | –0,799 | ||||
Trust on the use of ICT | 0,793 | 0,82 | 0,608 | 0,823 | 3,371 | 1,1581 | –0,271 | –0,781 |
0,804 | 3,461 | 1,1646 | –0,345 | –0,746 | ||||
0,741 | 3,504 | 1,1628 | –0,461 | –0,58 | ||||
Planning for the new telehealth/e-health | 0,751 | 0,885 | 0,608 | 0,886 | 3,051 | 1,1886 | –0,082 | –0,873 |
0,793 | 3,132 | 1,1818 | –0,105 | –0,796 | ||||
0,816 | 3,189 | 1,1939 | –0,185 | –0,844 | ||||
0,759 | 3,05 | 1,1801 | –0,106 | –0,913 | ||||
0,779 | 3,154 | 1,1907 | –0,214 | –0,742 | ||||
Satisfaction and willingness | 0,701 | 0,841 | 0,58 | 0,846 | 3,258 | 1,1942 | –0,249 | –0,758 |
0,71 | 3,648 | 1,1608 | –0,619 | –0,449 | ||||
0,833 | 3,468 | 1,1395 | –0,397 | –0,595 | ||||
0,794 | 3,505 | 1,2056 | –0,48 | –0,644 | ||||
Integration of technology | 0,746 | 0,836 | 0,562 | 0,837 | 3,318 | 1,1162 | –0,326 | –0,529 |
0,746 | 3,162 | 1,1439 | –0,098 | –0,733 | ||||
0,764 | 3,599 | 1,1563 | –0,506 | –0,601 | ||||
0,742 | 3,729 | 1,1468 | –0,698 | –0,303 | ||||
Resource readiness | 0,84 | 0,863 | 0,684 | 0,866 | 3,108 | 1,1628 | –0,119 | –0,78 |
0,872 | 3,21 | 1,1213 | –0,129 | –0,722 | ||||
0,765 | 3,091 | 1,1614 | –0,152 | –0,74 | ||||
Cultural readiness | 0,825 | 0,858 | 0,668 | 0,858 | 3,174 | 1,0881 | –0,088 | –0,634 |
0,826 | 3,175 | 1,0844 | –0,152 | –0,587 | ||||
0,801 | 3,154 | 1,1408 | –0,189 | –0,685 | ||||
Strategic readiness | 0,776 | 0,841 | 0,641 | 0,843 | 3,357 | 1,1109 | –0,328 | –0,609 |
0,825 | 3,495 | 1,1022 | –0,381 | –0,581 | ||||
0,8 | 3,577 | 1,1548 | –0,482 | –0,577 | ||||
IT readiness | 0,79 | 0,831 | 0,623 | 0,832 | 3,259 | 1,147 | –0,296 | –0,668 |
0,761 | 3,245 | 1,1825 | –0,259 | –0,75 | ||||
0,816 | 3,168 | 1,177 | –0,227 | –0,729 | ||||
Innovation valance | 0,797 | 0,847 | 0,649 | 0,847 | 3,376 | 1,1409 | –0,335 | –0,619 |
0,801 | 3,517 | 1,1401 | –0,41 | –0,673 | ||||
0,819 | 3,429 | 1,1831 | –0,408 | –0,623 | ||||
Cognitive readiness | 0,841 | 0,862 | 0,681 | 0,865 | 3,385 | 1,1707 | –0,348 | –0,701 |
0,849 | 3,397 | 1,1887 | –0,321 | –0,791 | ||||
0,784 | 3,453 | 1,1322 | –0,425 | –0,519 | ||||
Partnership readiness | 0,852 | 0,887 | 0,724 | 0,887 | 3,199 | 1,2099 | –0,139 | –0,867 |
0,866 | 3,266 | 1,1736 | –0,178 | –0,811 | ||||
0,834 | 3,258 | 1,1943 | –0,19 | –0,854 | ||||
Organizational readiness for digital innovation | 0,825 | 0,903 | 0,701 | 0,904 | 3,115 | 1,1664 | –0,145 | –0,76 |
0,852 | 3,131 | 1,1448 | –0,14 | –0,682 | ||||
0,846 | 3,087 | 1,2099 | –0,087 | –0,875 | ||||
0,825 | 3,194 | 1,2082 | –0,207 | –0,821 | ||||
Innovation implementation effectiveness | 0,781 | 0,849 | 0,659 | 0,853 | 3,47 | 1,1992 | –0,456 | –0,66 |
0,797 | 3,32 | 1,194 | –0,265 | –0,796 | ||||
0,856 | 3,183 | 1,2384 | –0,195 | –0,886 | ||||
Q1-Q16 (54 item) | 0,977 |
****A composite reliability of 0.70 is recommended [54]. Collier recommended an AVE greater than 0.5.
4.3Measurement model
Cronbach Alpha is a widely used method to measure the reliability and internal consistency of the construct [76, 78]. Özdamar [41] and Gliem [42] state the criterion values for the reliability coefficient as follows; the scale is unreliable in the range of 0.00 < α< 0.40, low reliable in the range of 0.41 < α< 0.60, average reliable in the range of 0.61 < α< 0.80, 0.81 < α< 1.00 range is quite reliable. The Cronbach Alpha values of the variables in the structure range from 0.831 to 0.903 (see Table 2). According to the relevant authors’ scale, the dimensions’ reliability is relatively high [77, 79]. According to the reliability analysis results, no statement was deleted because the level of reliability did not increase when the statements were deleted. Whether or not this instrument will yield the same findings each time it is used on the same subject in the same context is the subject of the dependability question. Science-related instruments are typically dependable if they yield consistent results regardless of how they are used or who administers them [75].
After the construct validity test, convergent and divergent (discriminant) validity should also be tested [43, 80]. To measure convergent validity, composite reliability (CR) and mean variance AVE (Average Variance Extracted) tests were performed [81]. For convergent validity, all CR values for the scale are expected to be greater than the AVE values and the AVE value to be greater than 0.5. In addition, while the CR value should exceed 0.70 for each construct, for convergent validity, the AVE value exceeding 0.50 is a condition for validity [55]. In the measurement model, the CR value was between 0.823 and 0.904, and the AVE value was between 0.562 and 0.724. These results revealed the reliability and internal consistency of the scale [78]. It is seen that the goodness of fit values provided for each structure are in the range of acceptable or excellent values.
To provide divergent validity, the condition that the square root of the AVE is greater than the correlation between the factors must be met [43]. When the correlation values of each structure with the other structures are examined, it is seen that the divergent validity condition is met for each structure, as it is lower than the said value (see Table 3). The convergent and discriminant validity of the scales used in the study were also evaluated. Convergent validity means that items under the same factor are related to each other and to the factor they belong to, while discriminant validity means that items under a factor are less related to other factors than their factor. Besides the CR coefficient, the AVE value should also be calculated for convergent validity. The AVE value expresses the average squares of the factor loads of the items in a factor. AVE > 0.500, CR > 0.700, and CR > AVE conditions must be met to be able to say that a factor has convergent validity [44, 45]. For discriminant validity, besides the AVE value, the correlation coefficients between the factors, the square of the maximum shared variance (MSV), and the mean of the average shared square variance (ASV) values should be calculated. The MSV value represents the square of the highest correlation coefficient between a factor and other factors. The ASV value represents the average square of the correlation coefficients between a factor and other factors. To say that the factors have discriminant validity, the conditions for the correlation coefficients between the factors MSV < AVE, ASV < AVE, and Square root AVE > must be met [45–47] (see Table 2).
Table 3
Constructive divergent validity
Dimensions | Mean | STD | CA | CR | AVE | MSV | ASV | 1.00 | 2.00 | 3.00 | 4.00 | 5.00 | 6.00 | 7.00 | 8.00 | 9.00 | 10.00 | 11 | 12 | 13 | 14 | 15 | 16 |
Identification of Needs for future changes Telehealth/e-health | 3.25 | 0.98 | 0.88 | 0.88 | 0,65 | 0.40 | 0.25 | (0.81) | |||||||||||||||
Awareness about telehealth/e-health | 3.24 | 0.98 | 0.87 | 0.87 | 0,68 | 0.38 | 0.24 | 0.63** | (0.83) | ||||||||||||||
Comfort with technology | 3.25 | 1.00 | 0.84 | 0.84 | 0,63 | 0.37 | 0.30 | 0.60** | 0.621** | (0.80) | |||||||||||||
Trust on the use of ICT | 3.45 | 0.99 | 0.82 | 0.82 | 0,60 | 0.35 | 0.28 | 0.52** | 0.524** | 0.608** | (0.78) | ||||||||||||
Planning for the new telehealth/e-health | 3.12 | 0.98 | 0.89 | 0.89 | 0,60 | 0.43 | 0.34 | 0.59** | 0.515** | 0.581** | 0.598** | (0.78) | |||||||||||
Satisfaction and willingness | 3.47 | 0.96 | 0.84 | 0.85 | 0,58 | 0.44 | 0.31 | 0.50** | 0.491** | 0.565** | 0.584** | 0.538** | (0.76) | ||||||||||
Integration of technology | 3.45 | 0.93 | 0.84 | 0.84 | 0,56 | 0.39 | 0.33 | 0.53** | 0.542** | 0.572** | 0.587** | 0.578** | 0.666** | (0.75) | |||||||||
Resource readiness | 3.14 | 1.01 | 0.86 | 0.87 | 0,68 | 0.46 | 0.32 | 0.57** | 0.494** | 0.545** | 0.531** | 0.636** | 0.521** | 0.621** | (0.83) | ||||||||
Cultural readiness | 3.17 | 0.97 | 0.86 | 0.86 | 0,66 | 0.41 | 0.36 | 0.56** | 0.518** | 0.561** | 0.50** | 0.597** | 0.534** | 0.587** | 0678** | (0.82) | |||||||
Strategic readiness | 3.48 | 0.97 | 0.84 | 0.84 | 0,64 | 0.36 | 0.34 | 0.54** | 0.517** | 0.563** | 0.526** | 0.516** | 0.629** | 0.625** | 0.560** | 0.618** | (0.80) | ||||||
IT readiness | 3.22 | 1.01 | 0.83 | 0.83 | 0,62 | 0.48 | 0.40 | 0.51** | 0.474** | 0.513** | 0.471** | 0.604** | 0.513** | 0.550** | 0.555** | 0.594** | 0.585** | (0,79) | |||||
Innovation valance | 3.44 | 1.01 | 0.84 | 0.85 | 0,64 | 0.46 | 0.39 | 0.51** | 0.450** | 0.521** | 0.518** | 0.543** | 0.581** | 0.601** | 0.514** | 0.573** | 0.600** | 0.694** | (0,81) | ||||
Cognitive readiness | 3.41 | 1.03 | 0.86 | 0.87 | 0,68 | 0.42 | 0.41 | 0.57** | 0.454** | 0.548** | 0.517** | 0.575** | 0.560** | 0.567** | 0.548** | 0.605** | 0.604** | 0.601** | 0.682** | (0,83) | |||
Partnership readiness | 3.24 | 1.07 | 0.89 | 0.89 | 0,72 | 0.52 | 0.48 | 0.51** | 0454** | 0.528** | 0.494** | 0.578** | 0.507** | 0542** | 0.582** | 0.594** | 0.546** | 0.637** | 0.611** | 0.631** | (0,85) | ||
Organizational readiness for digital innovation | 3.13 | 1.04 | 0.90 | 0.90 | 0,70 | 0.60 | 0.60 | 0.55** | 0.466** | 0.578** | 0.536** | 0.657** | 0.557** | 0.550** | 0.639** | 0.642** | 0.591** | 0.657** | 0.624** | 0.653** | 0.726** | (0,84) | |
Innovation implementation effectiveness | 3.32 | 1.06 | 0.85 | 0.,85 | 0.,65 | 0.66 | 0.66 | 0.53** | 0.445** | 0.520** | 0.544** | 0.615** | 0.574** | 0.549** | 0.575** | 0.601** | 0.595** | 0.619** | 0.643** | 0.643** | 0.694** | 0.771** | (0,80) |
*Values in parentheses indicate square root AVE values. **P < 0,01.
4.3Structural model
Structural Equation Modeling (SEM) is a statistical technique to test and evaluate theoretical models of complex relationship relationships among variables. The assumptions of SEM are as follows: i) Linearity: The relationships between the variables in the model are assumed to be linear. In other words, the changes in one variable are assumed to produce a proportional change in another variable. Ii) Normality: The data distribution is assumed to be normal or approximately normal. This assumption is essential because SEM relies on maximum likelihood estimation, assuming the data are normally distributed. Iii) Independence: The observations used in SEM are assumed to be independent. That is, the values of one observation should not be related to the values of another observation in the sample [86]. Iv) Absence of multicollinearity: Multicollinearity is the presence of high correlations between two or more independent variables in a model. This assumption is important because it can cause problems estimating the model parameters. V) Sample size: The sample size used in SEM should be sufficient to produce stable estimates of the model parameters. The exact sample size required depends on several factors, including the complexity of the model and the number of variables in the model [87]. Vi) Missing data: The presence of missing data can be a problem for SEM. The assumption is that the data are missing at random, which means the missing values are unrelated to the values of other variables in the model. Vii) Model identification: The model should be identified, which means that the number of parameters in the model should be less than the number of observations. If the model is over-identified, it may not be possible to estimate the parameters of the model. Viii) Non-negative variance: The variances of the latent variables should be non-negative. This is because negative variances are not meaningful in SEM [88]. The estimation method followed in this article is the Maximum Likelihood (ML) model. One of the most widely used methods for estimation is the Maximum Likelihood (ML) estimation technique, which assumes multivariate normal data [89–91]. Structural equation modeling is also used to examine correlations between latent (unobserved) variables or constructs that are measured by (a number of) manifest (observable) variables or indicators [85]. Second-level confirmatory factor analysis was performed in this article to reveal latent relationships. SEM is presented in Fig. 1.
Fig. 1
Secondary confirmatory factor analysis.
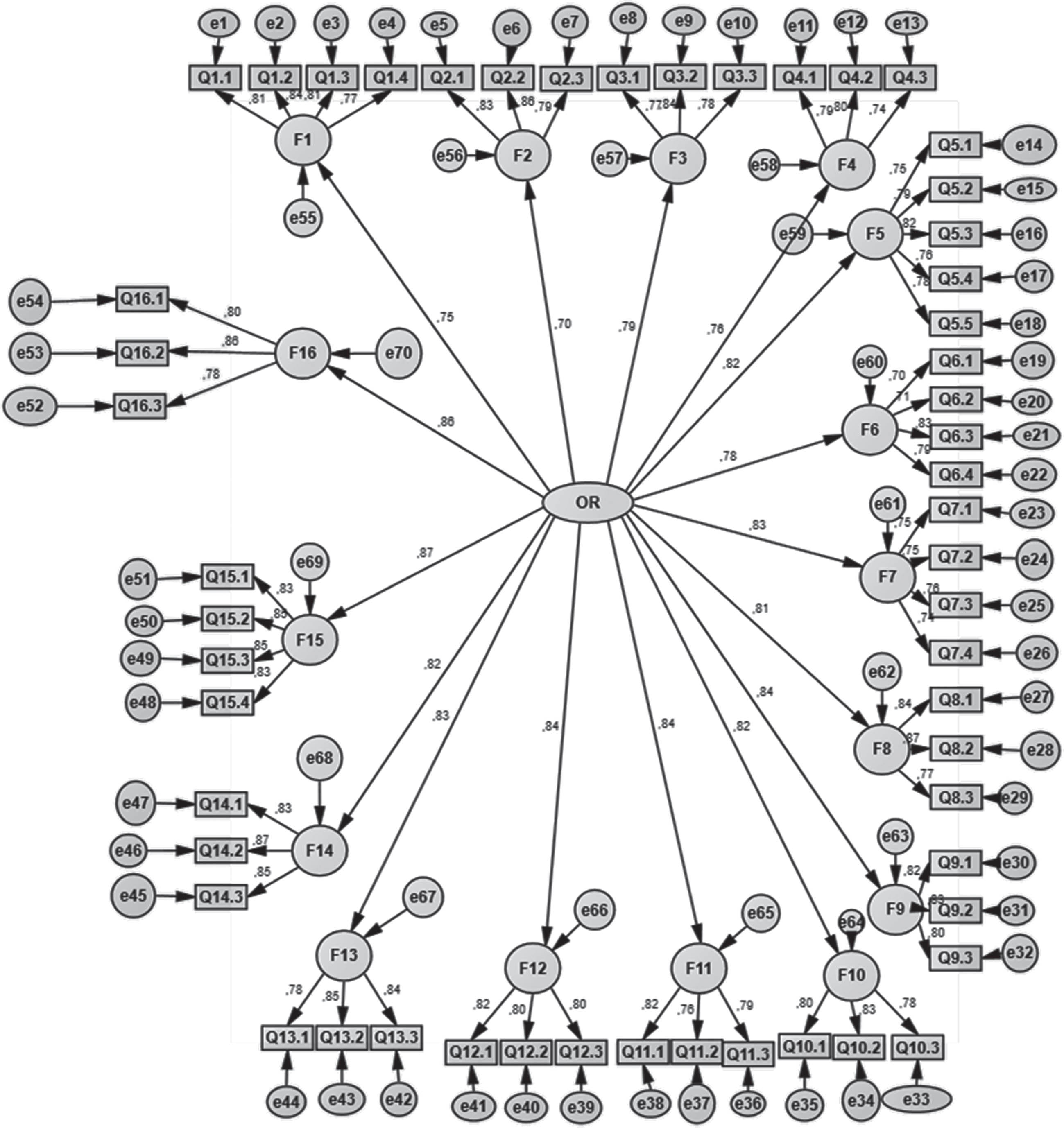
4.5Goodness of fit of the model
The Confirmatory factor analysis (CFA) is used to test the suitability of the factors determined by explanatory factor analysis to the factor structures defined by the hypothesis [48, 49]. While testing the fit between the model and the data, some goodness-of-fit tests may be used, or all may be preferred [50]. The general indicators for model fit are CMIN/DF = x2/df, GFI, CFI, IFI, AGFI, RMR, and RMSEA. The reported values may vary according to the importance the researcher wants to draw attention to. Many goodness-of-fit measures are used in structural equation model evaluations in the literature. It isn’t easy to meet all of these criteria in a model. However, meeting some essential criteria is mandatory for model validity [51, 52]. Therefore, secondary confirmatory factor analysis was performed for the model, and the analysis results are presented in Fig. 1. The required goodness of fit values for the model and the obtained values are given in Table 4.
Table 4
Goodness of fit of the model
Fit Index | Acceptable Range 2 | Perfect Range 1 | Model Value |
χ2/df | 2≤χ2/df≤5 | 0≤χ2/df≤2 | 4 |
GFI | 0.90≤GFI≤0.95 | 0.95≤GFI≤1.00 | 0.848 |
CFI | 0.90≤CFI≤0.95 | 0.95≤CFI≤1.00 | 0.913 |
TLI | 0.90≤TLI≤0.95 | 0.95≤TLI≤1.00 | 0.908 |
RMSEA | 0.05≤RMSEA≤0.10 | 0.00≤RMSEA≤0.05 | 0.05 |
SRMR | 0≤SRMR≤0.05 | 0.05≤SRMR≤0.10 | 0.048 |
Source: Hair et al. (2006: 642-645); Schumacker and Lomax (2016: 112); Kirchoff and Falasca (2022: 5); Collier, (2020: 67).
4.6Linear regression analysis
Although binary outcomes may also be employed, linear regression models frequently examine the relationship between a continuous outcome and independent variables. As arbitrary, biased outcome adjustments are typically unneeded, linear regression models are relatively resilient to breaches of the normality assumption in scenarios with high sample sizes. Instead, researchers should identify model miss-specifications, which may distort results regardless of sample size. They include outlier values, excessive leverage, heteroscedasticity, correlated errors, nonlinearity, and interactions [92]. The regression model is found to be significant (F = 354,042; p = 0.000). Simultaneously, H1-AHR (β= 0.076; t 3,561; p = 0.00), H2-COR (β= 0.072; t 3,158; p = 0.00), H3- PNTH (β= 0.109; t 4,523; p = 0.00), H5-ITR (β= 0.098; t 4,275; p = 0.00), H6-RR (β= 0.112; t 4,668; p = 0.00), H7-PR (β= 0.195; t = 8,57; p = 0.00), H8-CUR (β= 0.066; t 2,635; p = 0.01), H9-IIE (β= 0.354; t 15,169; p = 0.00), and variables have a positive and significant effect on of organizational readiness for digital innovation (β= 0.17; t = 4.662; p = 0.04). IOT (β= –0.063; t –2,6; p = 0.01) variable negatively and significantly affects organizational readiness for digital innovation. The model explains 72,5 percent of the changes in dependent variables organizational readiness for digital innovation (R2 = 0.725). Analysis results are presented in Table 5.
Table 5
Linear regression analysis results
Unstandardized | Standardized | t | Sig. | 95,0% ConfidenceInte | Collinearity | ||||
Coefficients | Coefficients | for B | Statistics | ||||||
B | Std. Error | Beta | LowerBo | UpperBo | Tolerance | VIF | |||
(Constant) | –0.169 | 0.067 | –2.52 | 0.01 | –0.3 | –0.037 | |||
H1-AHR | 0.076 | 0.021 | 0.074 | 3.561 | 0.00 | 0.034 | 0.118 | 0.526 | 1.90 |
H2-COR | 0.072 | 0.023 | 0.071 | 3.158 | 0.00 | 0.027 | 0.117 | 0.44 | 2.27 |
H3-PNTH | 0.109 | 0.024 | 0.103 | 4.523 | 0.00 | 0.062 | 0.157 | 0.431 | 2.318 |
H4-IOT | –0.063 | 0.024 | –0.056 | –2.6 | 0.01 | –0.11 | –0.015 | 0.478 | 2.092 |
H5-ITR | 0.098 | 0.023 | 0.095 | 4.275 | 0.00 | 0.053 | 0.143 | 0.452 | 2.214 |
H6-RR | 0.112 | 0.024 | 0.109 | 4.668 | 0.00 | 0.065 | 0.158 | 0.411 | 2.436 |
H7-PR | 0.195 | 0.023 | 0.202 | 8.57 | 0.00 | 0.151 | 0.24 | 0.403 | 2.484 |
H8-CUR | 0.066 | 0.025 | 0.062 | 2.635 | 0.01 | 0.017 | 0.115 | 0.411 | 2.434 |
H9-IIE | 0.354 | 0.023 | 0.361 | 15.169 | 0.00 | 0.308 | 0.4 | 0.396 | 2.526 |
a Dependent Variable: Organizational readiness for digital innovation.
5Discussion
This study aimed to examine the level of readiness of healthcare institutions for digital innovation by focusing on a transition economy, namely the case of Kosovo. In doing so, we firstly tested the validity of the organizational readiness scale adapted from Khoja [1], and Lokuge [2] validated the scale using secondary confirmatory factor analysis. Secondly, we tested nine hypotheses regarding the following antecedents of organizational readiness for digital innovation in healthcare institutions in Kosovo. Findings show that all hypotheses were statistically positive and had significant relationships, except for H4. In H4, we found a negative and significant relationship. This study adds to the organizational readiness literature [3] and illustrates the different antecedents influencing readiness for digital innovation in healthcare institutions [2]. We have discussed the contribution of this study to the literature under five headings below.
Firstly, the research adds to past research by presenting a psychometrically tested instrument to measure institutional readiness for digital innovation in transition economies [1, 2, 67, 69]. In this respect, in developed countries [2, 69] and developing countries [1, 67], we have presented evidence that measurement tools developed in past research can be applied in the context of transition economies. The results of this research are the scales developed in different countries [1, 2] verified that it is valid in Kosovo. In this respect, the results are interesting that the organizational readiness scale, which has been tested in developed [2] and developing [1] countries, can be used in the context of transition economies, despite differences such as technological infrastructure, education level of employees, and cultural characteristics.
Secondly, this research contributes to research on the antecedents of readiness for digital innovations. In recent years, an essential current of research has emerged on what the antecedents of innovation might be [93–96]. These studies have revealed the importance of antecedents such as leadership [95], knowledge management [96], R&D and production processes [94], competitive intensity, the technological orientation of the founding team, business orientation, and environmental dynamism [93]. For the sustainability of organizations, innovation and what contributes to innovation are of great importance for organizations. This research presents a combined approach that considers existing theory and literature, measuring organizational readiness for digital innovation and establishing a valid scale in transition economies, and providing evidence of antecedents for organizational readiness for digital innovation.
Thirdly findings suggest that adapting human resources to technology (H1) and cognitive readiness (H2) positively influences organizational readiness for digital innovation. These findings are in line with previous studies [13, 21, 27, 28] and suggest that AHR and COR are crucial for healthcare organizations’ technological readiness. Hence, leaders of (health) organizations need to ensure that human resources are ready to adopt [13] (new) digital technology on the one hand, and another hand to invest in developing the necessary competencies [2] in shifting from change readiness to digital readiness [13].
Fourthly, findings show that planning for new telehealth and e-health (H3), integration of old technologies (H4), IT readiness (H5), and resource readiness (H6) positively influence health organizations’ readiness for digital innovation. Planning for new telehealth and e-health is crucial and depends on preparing human resources and combining existing technologies with new technologies. Therefore, health organizations need to analyze how health organizations have IT readiness. These findings are in line with those of previous studies that show that IT and resource readiness influence organizational readiness [2, 3, 17] and planning for new telehealth and e-health [28]. Likewise, these findings show the importance of resource readiness for health organizations and align with previous studies [2, 14, 69]. It is important to emphasize that health organizations in low-income countries like Kosovo still face challenges regarding the antecedents of organizational readiness that may lead to digital innovation. Those findings align with those of the study conducted by Qureshi [11], acknowledging the challenges organizations from low-income countries most often face regarding organizational readiness for digital innovation.
Lastly, findings show that partnership readiness (H7), cultural readiness (H8), and the effectiveness of healthcare organizations (H9) influence organizational readiness for digital innovation. Similar results were found about the importance of partnership readiness by Lokuge [2, 14, 17], cultural readiness by Weiner [16], Zhen [35], and the effectiveness of healthcare organizations to implement innovations by Williams [38]. Although health organizations in Kosovo have a long way to building cultural readiness concerning technology and organizational change, these findings are crucial. Similarly, alongside the weak institutional environments in Kosovo [15], which are generally recognized as having a significant impact on the effectiveness of healthcare organizations, these findings suggest that effectiveness in healthcare facilities may be related not only to better services to patients but also to organizational readiness for digital innovation. In addition, partnership readiness is of crucial importance. However, it is essential to emphasize that partnership readiness includes patient-clients and partnership readiness with other healthcare institutions at the national and international levels. The extent to which partners are ready to cooperate and share their knowledge and experience is critical for healthcare organizations which may increase the effectiveness and influence cultural readiness within the organization.
5.1Managerial and policy implications
This research’s managerial and policy implications can be evaluated under five headings. Firstly, the tool was tested on organizational readiness for digital innovations, which can help organizations determine their readiness for new technological change [2]. The fact that the survey can be easily applied among all organizations and their stakeholders and takes a short time makes it a valuable tool for organizations. At the very least, instead of getting off to a bad start by applying the tool in readiness for digital innovations, organizations can identify their shortcomings through the tool and make the necessary improvements. Secondly, organizations could identify the readiness of different stakeholders through organizational readiness for digital innovation [2, 98]. In this way, they can prepare organizations and increase innovations’ success by creating mechanisms that will enable all stakeholders to act together. Thirdly, given the critical role of technological literacy, the challenges healthcare professionals face to adapt to technological changes, and the availability of resources, managers in healthcare organizations need to consider the antecedents that affect organizational readiness for digital innovation early in the process. Fourthly, managers need to motivate their employees by collaborating with professional healthcare organizations experiencing organizational change towards digital innovation, organizing training on technology, and focusing on changing the organizational culture in healthcare institutions. Finally, about policymakers, we recommend increasing investment in healthcare institutions, significantly increasing the capabilities of healthcare services to deploy new technologies, and providing financial support for healthcare professionals to develop their technology-related skills and abilities.
5.2Limitations and future suggestions
The limitations of the study can be categorized into four main groups. First, Khoja [1] and Lokuge [2] adapted scale has been tested and validated in a country with relatively weak institutions in the transition economy context. Therefore, the scale must be tested and applied in developing and developed countries. Future research can test the validity of the respective scale by repeating similar analyses in developing and developed countries. Second, the cross-sectional data reflect the current situation but do not reveal long-term developments’ impact on organizational readiness for digital innovation. Therefore, future research can longitudinally examine the organizational readiness of healthcare organizations for digital innovation. Third, the Covid-19 pandemic-related factors may have influenced and directed the organizational readiness of health organizations for digital innovation [53]. For this reason, future research can investigate the issue by conducting in-depth interviews with employees and managers with pre-Covid 19 experience [63, 64] with case studies. Fourth, in the study, we did not touch on the managerial and institutional phenomena that affect the organizational readiness of health organizations for digital innovation. Therefore, future research can examine which organizational and institutional factors facilitate or complicate this process. Fifth, the data used in the research were collected from one university hospital. Therefore, future studies can expand the study’s sample by collecting data from different hospitals. Sixth, although the sample size consisted of 1236 questionnaires, studies with larger samples are likely to yield better results [67]. Finally, the organizational readiness structure for digital innovation in this research can be used to assess readiness from multiple stakeholder perspectives [2]. For example, this study collected data from doctors, nurses, health personnel other than nurses, and other personnel working in a hospital. However, this does not provide information on the readiness of different stakeholders. Therefore, future research can compare stakeholders’ views on organizational readiness for digital innovation by collecting data from samples, including healthcare providers, non-governmental organizations, and policymakers.
Acknowledgments
We would like to emphasize the contributions made by students from the Departments of Nursing, Speech Therapy, and Occupational Therapy. Their efforts in collecting data and making significant contributions to the field are highly appreciated and recognized.
Author contributions
CONCEPTION: Besarta Taganoviq, Arben Hysena and Adnan Hoxha
METHODOLOGY: Mehmet Nurullah Kurutkan, Mehmet Bağış, Arben Hysenaj and Besarta Taganoviq
DATA COLLECTION: Arben Hysenaj, Arta Dalipi, Blerte Hyseni, Erza Haxhijakupi, Altina Bimbashi and Blerta Kryeziu
INTERPRETATION OR ANALYSIS OF DATA: Mehmet Nurullah Kurutkan and Mehmet Bağış
PREPARATION OF THE MANUSCRIPT: Arben Hysenaj and Besarta Taganoviq
REVISION FOR IMPORTANT INTELLECTUAL CONTENT: Adnan Hoxha and Linda Ukimeraj Harris
SUPERVISION: Besarta Taganoviq, Arben Hysenaj, Mehmet Nurullah Kurutkan and Mehmet Bağış
References
[1] | Khoja S , Scott RE , Casebeer AL , Mohsin M , Ishaq AF , Gilani S . e-Health readiness assessment tools for healthcare institutions in developing countries. Telemedicine and e-Health. (2007) ;13: (4):425–32. |
[2] | Lokuge S , Sedera D , Grover V , Dongming X . Organizational readiness for digital innovation: Development and empirical calibration of a construct. Information & Management. (2019) ;56: (3):445–61. |
[3] | Weiner BJ , Amick H , Lee SY . Conceptualization and measurement of organizational readiness for change: A review of the literature in health services research and other fields. Medical Care Research and Review. (2008) ;65: (4):379–436. |
[4] | Lapão LV . The future impact of healthcare services digitalization on health workforce: The increasing role of medical informatics. Exploring Complexity in Health: An Interdisciplinary Systems Approach. (2016) ;675–9. |
[5] | Jennett P , Jackson A , Healy T , Ho K , Kazanjian A , Woollard R , Haydt S , Bates J . A study of a rural community’s readiness for telehealth. Journal of Telemedicine and Telecare. (2003) ;9: (5):259–63. |
[6] | Demirci Ş . Sağlığın dijitalleşmesi-Digitalization of health. Mehmet Akif Ersoy Üniversitesi Sosyal Bilimler Enstitüsü Dergisi. (2019) ;10: (26):710–21. |
[7] | May C , Mort M , Williams T , Mair F , Gask L . Health technology assessment in its local contexts: Studies of telehealthcare. Social Science & Medicine. (2003) ;57: (4):697–710. |
[8] | Childs S , Blenkinsopp E , Hall A , Walton G . Effective e- learning for health professionals and students— barriers and their solutions. A systematic review of the literature— findings from the HeXL project. Health Information & Libraries Journal. (2005) ;22: :20–32. |
[9] | Muigg D , Duftschmid G , Kastner P , Modre-Osprian R , Haluza D . Telemonitoring readiness among Austrian diabetic patients: A cross-sectional validation study. Health Informatics Journal. (2020) ;26: (4):2332–43. |
[10] | Salahuddin L , Ismail Z . Classification of antecedents towards safety use of health information technology: A systematic review. International Journal of Medical Informatics. (2015) ;84: (11):877–91. |
[11] | Qureshi QA , Shah B , Kundi GM , Nawaz A , Miankhel AK , Chishti KA , Qureshi NA . Infrastructural barriers to e-health implementation in developing countries. European Journal of Sustainable Development. (2013) ;2: (1):163. |
[12] | Khoja S , Durrani H , Nayani P , Fahim A . Scope of policy issues in e Health: Results from a structured literature review. Journal of Medical Internet Research. (2012) ;14: (1):e1633. |
[13] | Gfrerer A , Hutter K , Füller J , Ströhle T . Ready or not: Managers’ and employees’ different perceptions of digital readiness. California Management Review. (2021) ;63: (2):23–48. |
[14] | Lokuge S , Sedera D . Is your IT eco-system ready to facilitate organizational innovation? Deriving an IT eco-system readiness measurement model. In Proceedings of the 37th International Conference on Information Systems (ICIS Association for Information Systems (AIS). (2016) :1–22. |
[15] | Kryeziu L , Coşkun R . Political and economic institutions and economic performance: Evidence from Kosovo. South East European Journal of Economics and Business. (2018) ;13: (2):84–99. |
[16] | McCrae JS , Scannapieco M , Leake R , Potter CC , Menefee D . Who’s on board? Child welfare worker reports of buy-in and readiness for organizational change. Children and Youth Services Review. (2014) ;37: , 28–35. |
[17] | Hussain M , Papastathopoulos A . Organizational readiness for digital financial innovation and financial resilience. International Journal of Production Economics. (2022) ;243: :108326. |
[18] | Lennon MR , Bouamrane MM , Devlin AM , O’connor S , O’donnell C , Chetty U , Agbakoba R , Bikker A , Grieve E , Finch T , Watson N . Readiness for delivering digital health at scale: Lessons from a longitudinal qualitative evaluation of a national digital health innovation program in the United Kingdom. Journal of Medical Internet Research. (2017) ;19: (2):e6900. |
[19] | El-Nour AE , Elnimeiri MK , Abbas AM . Towards a digitized and integrated health information system in Sudan: Assessment of readiness at state level. Sudan Journal of Medical Sciences. (2016) ;11: (2):55–60. |
[20] | Simonova MV , Zabelina OV , Mirzabalaeva FI . Qualified Specialists’ Readiness for Digitalization Risks. InInternational Online Forum named after A. Ya. Kibanov” Innovative Personnel Management” Springer, Cham. (2020) , pp. 364–373. |
[21] | Walston SL , Bennett CJ , Al-Harbi A . Understanding the factors affecting employees’ perceived benefits of healthcare information technology. International Journal of Healthcare Management. (2014) ;7: (1):35–44. |
[22] | Parsell G , Bligh J . The development of a questionnaire to assess the readiness of health care students for interprofessional learning (RIPLS). Medical Education. (1999) ;33: (2):95–100. |
[23] | Nguyen DN , Zierler B , Nguyen HO . A survey of nursing faculty needs for trainingin use of new technologies for education and practice. Journal of Nursing Education. (2011) ;5: (4):181–9. |
[24] | Edwards J , O’Connor PA . Improving technological competency in nursing students: The passport project. Journal of Educators Online. (2011) ;8: (2):n2. |
[25] | Sharp LA . Literacy in the digital age. Language and Literacy Spectrum. (2014) ;24: , 74–85. |
[26] | Samal A , Patra S , Chatterjee D . Impact of culture on organizational readiness to change: Context of bank M&A. Benchmarking: An International Journal. 2019. |
[27] | Ruel H , Rowlands H , Njoku E . Digital business strategizing: The role of leadership and organizational learning. Competitiveness Review: An International Business Journal. 2020. |
[28] | Browning SV , Tullai-McGuinness S , Madigan E , Struk C . Telehealth: Is your staff ready to implement? A descriptive exploratory study of readiness for this technology in home health care. Home Healthcare Now. (2009) ;27: (4):242–8. |
[29] | Bağış M , Karagüzel ES , Kryeziu L , Ardıç K . A Longitudinal analysis on intellectual structure of human resources management: Theoretical foundations and research trends. Mehmet Akif Ersoy Üniversitesi İktisadi ve İdari Bilimler Fakültesi Dergisi. (2019) ;6: (3):796–814. |
[30] | Levin DZ , Cross R . The strength of weak ties you can trust: The mediating role of trust in effective knowledge transfer. Management Science. (2004) ;50: (11):1477–90. |
[31] | Teo TS , Bhattacherjee A . Knowledge transfer and utilization in IT outsourcing partnerships: A preliminary model of antecedents and outcomes. Information & Management. (2014) ;51: (2):177–86. |
[32] | Alami H , Lehoux P , Denis JL , Motulsky A , Petitgand C , Savoldelli M , Rouquet R , Gagnon MP , Roy D , Fortin JP . Organizational readiness for artificial intelligence in health care: Insights for decision-making and practice. Journal of Health Organization and Management 2020. |
[33] | Ko DG . The mediating role of knowledge transfer and the effects of client-consultant mutual trust on the performance of enterprise implementation projects. Information & Management. (2014) ;51: (5):541–50. |
[34] | Ko DG, Kirsch LJ, King WR. Antecedents of knowledge transfer from consultants to clients in enterprise system implementations. MIS quarterly. (2005) ;1: :59–85. |
[35] | Zhen Z , Yousaf Z , Radulescu M , Yasir M . Nexus of digital organizational culture, capabilities, organizational readiness, and innovation: Investigation of SMEs operating in the digital economy. Sustainability. (2021) ;13: (2):720. |
[36] | do Carmo Caccia- Bava M , Guimaraes T , Harrington SJ . Hospital organization culture, capacity to innovate and success in technology adoption. Journal of Health Organization and Management 2006. |
[37] | Armenakis AA , Harris SG , Mossholder KW . Creating readiness for organizational change. Human Relations. (1993) ;46: (6):681. |
[38] | Williams I . Organizational readiness for innovation in health care: Some lessons from the recent literature. Health Services Management Research. (2011) ;24: (4):213–8. |
[39] | West SG, Finch JF, Curran PJ. Structural equation models with nonnormal variables: Problems and remedies. |
[40] | Hong S , Malik ML , Lee MK . Testing configural, metric, scalar, and latent mean invariance across genders in sociotropy and autonomy using a non-Western sample. Educational and Psychological Measurement. (2003) ;63: (4):636–54. |
[41] | Yemez İ . Doğrulayıcı faktör analizi ile sosyal medya reklamlarına yönelik tutum ölçeğinin yapı geçerliliğinin incelenmesi: Cumhuriyet 18 Üniversitesi İİBF’de bir uygulama. Cumhuriyet Üniversitesi İktisadi ve İdari Bilimler Dergisi. (2016) ;17: (2):97–118. |
[42] | Gliem JA , Gliem RR . Calculating, interpreting, and reporting Cronbach’s alpha reliability coefficient for Likert-type scales. Midwest Research-to-Practice Conference in Adult, Continuing, and Community Education. |
[43] | Brahma SS . Assessment of construct validity in management research: A structured guideline. Journal of Management Research. (2009) ;9: (2):59–71. |
[44] | Gürbüz S . AMOS ile yapısal eşitlik modellemesi. Ankara: Seçkin Yayıncılık. 2019. |
[45] | Hair JJr , Black W , Babin B , Anderson R , Tatham R . Multivariate Data Analysis, 6th Education. Inc., Upper Saddle River, New Jersey. 2006. |
[46] | Ali PA. Stressors Affecting Nursing Students in Pakistan Professor Roger Watson. |
[47] | Walczuch R , Lemmink J , Streukens S . The effect of service employees’ technology readiness on technology acceptance. Information & Management. (2007) ;44: (2):206–15. |
[48] | Kryeziu L , Coşkun R , Krasniqi B . Social networks and family firm internationalization: Cases from a transition economy. Review of International Business and Strategy 2021. |
[49] | Yuan KH , Bentler PM , Kano Y . On averaging variables in a confirmatory factor analysis model. Behaviormetrika. (1997) ;24: (1):71–83. |
[50] | Stegmann RB . Review of A Beginner’s Guide to Structural Equation Modeling, by Randall E.Schumacker& Richard G.Lomax: New York, NY: Routledge, (2016) , 351: pp. $65.91 (paperback). |
[51] | Nejatian M , Tehrani H , Momeniyan V , Jafari A . A modified version of the mental health literacy scale (MHLS) in Iranian people. BMC Psychiatry. (2021) ;21: (1):1–1. |
[52] | Baumgartner H , Homburg C . Applications of structural equation modeling in marketing and consumer research: A review. International Journal of Research in Marketing. (1996) ;13: (2):139–61. |
[53] | Krasniqi BA , Kryeziu L , Bağış M , Kurutkan MN , Idrizi S . COVID-19 and SMEs in Kosovo: Assessing effect and policy preferences. Journal of Developmental Entrepreneurshi. (2021) ;26: (01):2150005. |
[54] | Collier JE . Applied structural equation modeling using AMOS: Basic to advanced techniques. Routledge; 2020. |
[55] | Hair JF , Marko S , Christian MR , Jeannette AM . An assessment of the use of partial least squares structural equation modeling in marketing research. Journal of the Academy of Marketing Science. (2012) ;40: (3):414–33. |
[56] | Kirchoff JF , Falasca M . Environmental differentiation from a supply chain practice view perspective. International Journal of Production Economics. (2022) ;244: , 108365. |
[57] | Hoxha A , Taganoviq B , Hysenaj A . Qualitative research: Determinantsand long-term implications of readiness and trust of kosovo healthprofessionals on digital technology. Pielęgniarstwo w Opiece Długoterminowej/Long-Term Care Nursing. (2021) ;6: (4):47–56. |
[58] | Larsen J , Roberts AOH . Effective Use of Mental Health Research Information, Final Report to the National Institute of Mental Health, American Institutes for Research, Palo Alto, California 1971. |
[59] | Gartner, Gartner Reveals Five Business Intelligence Predictors for 2009 and Beyond, 2009. |
[60] | Aqeel M , Rehna T , Shuja KH , Abbas J . Comparison of students’ mental wellbeing, anxiety, depression, and quality of life during COVID-19’s full and partial (Smart) lockdowns: A follow-up study at a 5-month interval. Front Psychiatry. (2022) ;13: :835585.10.3389/fpsyt.2022.83558. |
[61] | Abbas J . The impact of coronavirus (SARS-CoV2) epidemic on individuals mental health: The protective measures of pakistan in managing and sustaining transmissible disease. Psychiatr Danub. (2020) ;32: (3-4):472–7. https://doi.org/10.24869/psyd.2020.472. |
[62] | Ge T , Abbas J , Ullah R , Abbas A , Sadiq I , Zhang R . Women’s entrepreneurial contribution to family income: Innovative technologies promote females’ entrepreneurship amid COVID-19 crisis. Front Psychol. (2022) ;13: :828040.10.3389/fpsyg.2022.828040. |
[63] | Rahmat TE , Raza S , Zahid H , Mohd Sobri F , Sidiki S . Nexus between integrating technology readiness 2.0 index and students’ e-library services adoption amid the COVID-19 challenges: Implications based on the theory of planned behavior. J Educ Health Promot. (2022) ;11: (1):50.10.4103/jehjehp_508_21. |
[64] | Liu Q , Qu X , Wang D , Mubeen R . Product market competition and firm performance: Business survival through innovation and entrepreneurial orientation amid COVID-19 financial crisis. Front Psychol. (2021) ;12: :790923.10.3389/fpsyg.2021.790923. |
[65] | Al-Balushi S , Sohal AS , Singh PJ , Al Hajri A , Al Farsi YM , Al Abri R . Readiness factors for lean implementation in healthcare settings– a literature review. Journal of Health Organization and Management. (2014) ;28: (2):135–53. |
[66] | Shoib S , Gaitan Buitrago JET , Shuja KH , Aqeel M , de Filippis R ,… Arafat SMY . Suicidal behavior sociocultural factors indeveloping countries during COVID-19. L’Encéphale. (2021) ;47.10.1016/j.ence2021.06.011. |
[67] | Gholamhosseini L , Ayatollahi H . The design and application of an e-health readiness assessment tool. Health Information Management Journal. (2017) ;46: (1):32–41. |
[68] | Li J , Land LPW , Chattopadhyay S , et al. E-Health readiness framework from Electronic Health Records perspective. International Journal of Internet and Enterprise Management. (2010) ;6: :326–48. |
[69] | Vaishnavi V , Suresh M , Dutta P . A study on the influence of factors associated with organizational readiness for change in healthcare organizations using TISM. Benchmarking: An International Journal. (2019) ;26: (4):1290–313. https://doi.org/10.1108/BIJ-06-2018-0161. |
[70] | Balasubramanian S , Shukla V , Sethi JS , Islam N , Saloum R . A readiness assessment framework for Blockchain adoption: A healthcare case study. Technological Forecasting and Social Change. (2021) ;165: :120536. |
[71] | Dönmez E , Kitapçı NŞ , Kitapçı OC , Yay M , Aksu PK , Köksal L , Mumcu G . Readiness for health information technology is associated to information security in healthcare institutions. Acta Informatica Medica: AIM: Journal of the Society for Medical Informatics of Bosnia & Herzegovina: Casopis Drustva za Medicinsku Informatiku BiH. (2020) ;28: (4):265–71. |
[72] | Dannapfel P , Thomas K , Chakhunashvili A , Melin J , Trolle Lagerros Y . A self-help tool to facilitate implementation of eHealth initiatives in health care (E-Ready): Formative evaluation JMIR form res. (2022) ;6: (1):1–12. |
[73] | Ghaleb EAA , Dominic PDD , Fati SM , Muneer A , Ali RF . The assessment of big data adoption readiness with a technology-organization-environment framework: A perspective towards healthcare employees. Sustainability. (2021) ;13: (15):1–33. |
[74] | Sharma A , Venkatraman S . Towards a Standard Framework for Organizational Readiness for Technology Adoption. In: AmitRK, PawarKS, SundarrajRP, RatchevS. (eds) Advances in Digital Manufacturing Systems. Springer, Singapore, (2023) , pp. 197–219. https://doi.org/10.1007/978-981-19-7071-9_10. |
[75] | George D , Mallery P . Reliability analysis. In IBM SPSS Statistics 25 Step by Step, Routledge. (2018) , pp. 249–260. |
[76] | Cronbach LJ , Shavelson RJ . My current thoughts on coefficient alpha and successor procedures. Educational and Psychological Measurement. (2004) ;64: (3):391–418. |
[77] | Douglas GB , Wright TA . Cronbach’s alpha reliability: Interval estimation, hypothesis testing, and sample size planning. Journal of Organizational Behavior. (2015) ;36: (1):3–15. |
[78] | Osburn HG . Coefficient alpha and related internal consistency reliability coefficients. Psychological Methods. (2000) ;5: (3):343–55. |
[79] | Taber KS . The use of cronbach’s alpha when developing and reporting research instruments in science education. Research in Science Education. (2018) ;48: (1):1273–96. |
[80] | Strauss ME , Smith GT . Construct validity: Advances in theory and methodology. Annual Review of Clinical Psychology. (2009) ;5: , 1–25. |
[81] | Bendermacher N . Beyond alpha: Lower bounds for the reliability of tests. Journal of Modern Applied Statistical Methods. (2010) ;9: (1):95–102. |
[82] | Hussain T , Abbas J , Li B , Aman J , Ali S . Natural resource management for the world’s highest park: Community attitudes on sustainability for central karakoram national park, pakistan. Sustainability. (2017) ;9: (6):972. |
[83] | Yao J , Ziapour A , Abbas J , Toraji R , NeJhaddadgar N . Assessingpuberty-related health needs among 10–15-year-old boys: Across-sectional study approach. Archives de Pédiatrie. (2022) ;29: (4):307–11. |
[84] | Farzadfar F , Naghavi M , Sepanlou SG , Moghaddam SS , Dangel WJ , Weaver ND , … & Mahdavi MM . Health system performance in Iran: A systematic analysis for the global burden of disease study 2019. The Lancet. (2022) ;399: (10335):1625–45. |
[85] | Hair JF Jr , Hult GTM , Ringle CM , Sarstedt M , Danks NP , Ray S , … & Ray S . An introduction to structural equation modeling. Partial Least Squares Structural Equation Modeling (PLS-SEM) Using R: A Workbook. (2021) , pp. 1–29. |
[86] | Kline RB . Assumptions in structural equation modeling. Handbook of Structural Equation Modeling. (2012) ;111: :125. |
[87] | Chin WW . Commentary: Issues and opinion on structural equation modeling. MIS Quarterly. (1998) ;vii–xvi. |
[88] | Tarka P . An overview of structural equation modeling: Its beginnings, historical development, usefulness and controversies in the social sciences. Quality & Quantity. (2018) ;52: , 313–54. |
[89] | Maydeu-Olivares A . Maximum likelihood estimation of structural equation models for continuous data: Standard errors and goodness of fit. Structural Equation Modeling: A Multidisciplinary Journal. (2017) ;24: (3):383–94. |
[90] | Hox JJ , Bechger TM . An introduction to structural equation modeling, 1998. |
[91] | Crisci A . Estimation methods for the structural equation models: Maximum likelihood, partial least squares E generalized maximum entropy. Journal of Applied Quantitative Methods. (2012) ;7: (2). |
[92] | Schmidt AF , Finan C . Linear regression and the normality assumption. Journal of Clinical Epidemiology. (2018) ;98: :146–51. |
[93] | Saemundsson RJ , Candi M . Antecedents of innovation strategies in new technology-based firms: Interactions between the environment and founder team composition. J Prod Innov Manage. (2013) ;31: (5):939–55. |
[94] | Wan F , Williamson PJ , Yin E . Antecedents and implications of disruptive innovation: Evidence from China. Technovation. (2015) ;39: :94–104. |
[95] | Somech A . The effects of leadership style and team process on performance and innovation in functionally heterogeneous teams. J Manage. (2006) ;32: (1):132–57. |
[96] | Roy R , Sarkar M . Knowledge, firm boundaries, and innovation: Mitigating the incumbent’s curse during radical technological change. Strateg Manage J. (2016) ;37: (5):835–54. |
[97] | Nylén D , Holmström J . Digital innovation strategy: A framework for diagnosing and improving digital product and service innovation. Bus Horiz. (2015) ;58: (1):57–67. |
[98] | Laforet S . Organizational innovation outcomes in SMEs: Effects of age, size, and sector. J World Bus. (2013) ;48: :490–502. |
[99] | Kryeziu L , Bağış M , Kurutkan MN , Krasniqi BA , Haziri A . COVID-19 impact and firm reactions towards crisis: Evidence from a transition economy. Journal of Entrepreneurship, Management, and Innovation. (2022) ;18: (1):169–96. https://doi.org/10.7341/20221816. |