Relocation trends determined by increasing risks in Eastern Europe: An ANP-TOPSIS approach
Abstract
BACKGROUND:
The COVID-19 pandemic has changed companies’ perspective on relocation and brought uncertainty into people’s lives. Uncertainty, a decisive factor in today’s global environment, requires new research about human resources and companies. The COVID-19 pandemic has caused an unexpected need for change within organizations, especially in terms of human resources management, creating a complex and challenging environment that interferes with business continuity, forcing employees to cope with this challenging situation.
OBJECTIVE:
The article aims identifying the changes generated by the COVID 19 pandemic in the relocation of businesses from the European Union, assessing the relocation trends of companies around the world in European countries in this volatile macroeconomic environment. The sustainability of companies, the way they can overcome the crises generated by the pandemic depend mainly on economic, social, financial, political factors and human resource involved in the relocation process. Studying the influence of the pandemic on relocation decision contributes to better management of crises in the future and to reducing risks.
METHODS:
The study proposes an integrated ANP-TOPSIS (Analytic Network Process- Technique for Order of Preference by Similarity to Ideal Solution) for ordering preference according to the ideal solution framework. Priority should be given to solutions that consider the interactions between factors involved in decision-making. The proposed model will increase the efficiency of the transfer decision-making process and help managers choose solutions effectively based on their importance and impact on the company and the human resources involved.
RESULTS:
The synthesis of the indicators and methods used, in addition to the factors that affect relocation, complements the specialized literature. The results showed a shift in business relocation options from east to west, demonstrating the current trend in the relocation issue associated with the COVID-19 virus. Eastern European countries are no longer as attractive for companies relocating compared to the pre-pandemic period. The countries with more stable economies, characterized by lower risks, seem to become more attractive to companies that relocate their facilities.
CONCLUSIONS:
The strategic positioning of the firm, its growth or adaptation to the present environment, and its geographic focus are fundamental components of a company’s migration. The selection of an ideal site is a research problem; not only to find a place where firms will have access to qualified human resources, to lower their costs, to be close to raw materials or the market, but also to prevent associated relocation hazards. According to research, today’s reality necessitates a risk-focused strategy.
Oana Panazan graduated Faculty of Technological Engineering, Transylvania University of Brasov, Romania in 2017. Now, she is Ph.D. student in the research field of Engineering and Management, at the same institution.
Catalin Gheorghe graduated Faculty of Technological Engineering, Transylvania University of Brasov, Romania in 1995. Five years later he graduated Bucharest University of Economic Studies (specialization Finance, Insurance, Banking and Stock Exchange), Romania. In 2006 he was awarded with Ph.D. in Industrial Management by Transylvania University of Brasov, Romania. Actually, he acts as lecturer at the Faculty of Technological Engineering and Industrial Management, Department of Engineering and Industrial Management. His research’s fields of interest include industrial businesses, finance analysis, financial management and capital market.
Gavrila Calefariu is full professor and Ph.D. supervisor in the field of Engineering and Management, at Faculty of Technological Engineering and Industrial Management, Department of Engineering and Industrial Management, Transylvania University of Brasov, Romania. His research fields of interest are: technology management, industrial engineering and business management.
1Introduction
Relocation is one of the most debated features of economic globalization. Until the COVID-19 pandemic, companies were choosing sites to relocate for benefits in terms of profits, costs, and economies of scale. The major events in recent times have determined the company’s orientation according to political stability and a priority health system with a significant share in GDP.
In recent decades, relocation has been an important factor that has influenced economic development. During this period, companies have integrated their business into a global value system. Pandemic waves have affected economic sectors, thus developing new approaches to crisis management. Studies about social distancing have established the effects generated by the COVID-19 pandemic on people, economies, and states [1]. Global economies can lead to the revitalization of contributions, scientific collaborations, and specific strategies, thus generating the adoption of new technologies against the background of the COVID-19 pandemic with the help of government support [2].
Governments have a key role to play in supporting SMEs and have an indispensable role in stimulating business activities, providing a major share of GDP that drives the economy [3].
In this research, 34 European states were considered relocation options for companies in Europe The relocations factors and supporting indicators demonstrated by various researchers have been identified in the literature. After their identification and grouping, they resulted in 8 criteria and 34 sub-criteria. The criteria pursued were human resources, infrastructure, education, environmental factors, science and technology, poverty, and social exclusion. The aim of the research is to build a decision-making model for companies, countries and authorities on the criteria that determine the relocation, measurement indicators and possible locations within European countries. The research consists of five parts. In the next section, a synthesis of the literature was made, of the most relevant works explicitly focused on relocations. The third section outlines a theoretical framework of the methodology, followed by database study. The fourth section contains the empirical findings, and the implications followed by limitation. In the last section, a summary of the findings was provided and a discussion of their implications.
2Literature review
2.1Relocation literature
The broad relocation context was able to be defined through the study of literature, limits of the subject, the current trends, factors determining relocation, and the methods used by different researchers. The general theoretical framework of relocation is the result of location theory, [4]. According to this theory, the main forces that lead to the relocation of companies are the expansion and the need for more appropriate premises. Another reason is that companies seek to take advantage of lower production costs, specific to other markets. Due to the economic downturn, organizations are facing challenges in making investment portfolio decisions. When resources have dwindled, decision-makers need to manage risk, considering the current economic environment.
Spatial flow models were used and the impact of economic and labour structures was considered to draw conclusions using the traditional theory of migration [5]. A general analysis of the research indicates that the geographical location of economic activity and its importance to companies, have been the subject of several studies [6–11]. The decision-making context is related to political, environmental, legal, administrative and market aspects [12]. Political pressure and government subsidies play a significant role in relocating the company. Marginal income is an objective of organizations, relocation being a solution for them, to achieve favourable cost conditions [9]. To give decision makers more flexibility, a new model for uncertainty conditions was proposed, consisting of an algorithmic methodology [13]. Organizations tend to gain sustainable advantages to strengthen their market position. BSC (Balanced Scorecard) is a systematically methodology considering non-financial indicators in assessing company’s performance. In this way a hybrid approach was applied based on DEMATEL (Decision Making Trial and Evaluation Laboratory) and ANP (Analytical Network Process) under conditions of uncertainty [23].
2.2Methods used in relocation problem
Which is the best location? This problem-solving approach starts with the data collection from the countries considered to have a real potential for relocation. If we consider their unpredictability and how sensitive they are to any event, we will reach a typical MCDM problem under conditions of uncertainty.
The evaluation and prioritization of feasible alternatives, depending on their preference, can be achieved by applying a model that is developing a decision-making problem using, fuzzy ARAS (Additive Ratio Assessment) and ANP approaches [15]. An overview of the international location decision, which includes a set of factors in the relocation process, was analysed throught the Delphi approach which provides a methodology for obtaining information from a group of individuals, who have relevant knowledge and experience. The results reflect opinions and views on location decisions [16]. Applying an ANP-TOPSIS analysis method, the five most important indicators for the choice of location: diversity of transport services, availability of human resources, shipping services, distance to suppliers and markets, [17].
A decision-making instrument used to determine mission, objectives, strategy, direction and target market, to allocate resources, strategic planning is an essential component of tactics. According to definition, the process of choosing and establishing strategies constitutes strategic planning [18]. The lack of homogeneity of sub-urbanization processes, the efficient use of land, the lack of local practices and standards for the use of space and the shortcomings in its planning, are decision-makers when developing land plans, which consider economic and social policies [19].
MCDM methods should be integrated to adapt to multi criteria problems [20]. The development of plans, projects with other organizations and industries is another aspect of development. In this case, the internal process was identified as the most effective perspective, with a major influence on other aspects [21]. The authors developed a BSC model for universities with the help of two different groups of experts. According to the authors, one of the factors that has a major influence is the budget MCDM methods have extensive applications in solving decision-making problems [22]. An integrated model was applied based on TOPSIS, ANP, and SWOT analysis (strengths, weaknesses, opportunities, and threats). The results show that the production capacity should be improve [22–26]. Starting from the idea that different MDM (master data management methods) for the same problem offers mutually inconsistent solutions,a model was recommended to analyse the consistency of methods [23].
Identifying a suitable relocation alternative involves a complex case that requires that all criterion for making decisions be considered simultaneously. AHP (analytical hierarchy process) is a method for solving multi-criteria decision-making issues that is applied to numerous issues [27]. Selection problems involve the use of different qualitative and quantitative criteria. The adaptation of the Fuzzy-AHP model demonstrated actual organizational issues [28].
There are numerous MCDM techniques that assist decision makers in choosing the location. These include the analytical network process (ANP), the similarity of command preferences to ideal solution (TOPSIS), the weighted aggregate product valuation method (WASPAS), multicriteria optimization and compromise solution (VIKOR), analytical hierarchy process (AHP), multi-attribute decisions and factors (FMAADM).
In the relocation process, the complex interactions between factors amplify the difficulty of the analysis. It was chosen to process the data using the SWOT-AHP method [9]. Decreased competitiveness and increased costs can be the consequences of a poorly chosen location and structured this complex problem using the AHP method. For this reason, the authors opted for a FUZZY-AHP extension, the advantage of this extension was the precision of the analysis, due to the reduction in the degree of inaccuracy [28].
In the case of the fuzzy CODAS (Combinative Distance-Based Assessment) technique, the evaluation process is based only on the ideal negative solution. The advantage of the method is that it involves two types of distance, which increase the results [29]. It was proposed the application of SWOT, FQSPM-Gap, (Fuzzy Quantitative Strategic Planning Matrix-Gap analysis), for the ranking of alternatives integrated with four methods: COPRAS-F, (Fuzzy Complex Proportional Assessment), Fuzzy MOORA, (Fuzzy Multi-objective Optimization by Ratio Analysis), ARAS-F, (Fuzzy Additive Ratio Assessment) and Fuzzy TOPSIS together with the Borda procedure [26] and analyzed the results obtained using MDM methods. The model provided reliable classification information for situations where the application of different MDM methods leads to mutually inconsistent solutions [23].
Popular and ideal for the application of the concept of business process management are micro, small; and medium businesses [30]. To make an action plan for the improvement of location selection, the availability of qualified human resources (HR) is one of the important factors [31]. The study introduced characteristics of factors of human resources. To find a suitable solution, a location assessment model is formed based on 34 indicators.
It was proposed a model developed within the AHP, which has a specific characteristic given by the set of evaluation criteria and the number of alternatives. The idea of α-cut was used in Buckley’s optimization model to represent a certain amount of uncertainty in decision making [32] and used multi-criteria decision analysis (MCDA) to include multiple data, namely fractal size, slope, and flow accumulation [33]. The factors were integrated, and the determination of the weighting coefficients was made based on the AHP method. It was studied the urgent need for decommissioning the facilities due to unforeseen costs, significant danger, and environmental issues [20]. Finally, Table 1 presents a synthesis of the most relevant references and approaches that has been used for the proposed methodology of this study.
Table 1
Synthesis of MCDM methods
Author (year) | Method | Method description approach |
Saaty and Vargas, (2006) | ANP | Site selection |
Nong, (2021) | ANP-TOPSIS | Selection of the distribution centre location |
Zamani et al. (2014) | ARAS-F-ANP | Brand extension |
Azimi et al. (2011) | SWOT-ANP-TOPSIS | The strategies prioritizing |
Nazari-Shirkouhi et al. (2020) | BSC-FDEMATEL-FANP-IPA | Adopting more appropriate educational, research and institutional policies |
Saaty (1987). | AHP | Site selection |
Eslamipoor &Sepehriar, (2014) | SWOT-AHP | Firm relocation |
Kaboli et al. (2007) | FAHP | The location problem |
Pan, (2008) | FAHP | The selection of the suitable construction method. |
Khazaeni et al. (2012) | FAHP | The selection of balanced risk allocation |
Choudhury et al. (2019) | AHP-Gini coefficient method | Optimal location selection |
Marinković et al. (2018) | DELPHI-AHP | Selecting location for a new business |
Kabo-bah et al. (2021); | AHP-FD | Erosion potential mapping |
Yu, (2002) | GP-AHP | Solving group decision-making problems |
Abdelgawad and Fayek, (2010) | FFMEA-FAHP | Support for establishing timely corrective actions |
Pamučar et al. (2017) | TOPSIS-COPRAS-VIKOR-ELECTRE | Site selection |
Roy et al. (2019) | WIRN-COPRAS | The collective decision-making evaluation framework |
MacCarthy &Atthirawong, (2003) | DELPHI | The international location decisions |
Li and Hu, (2021) | AHP-SAW-SMARTS-MAUT | Facility decommissioning study |
3Methodology
Since the AHP method, frequently used by researchers, fails to capture the interdependencies and feedback between levels, the authors opted for the use of the analytical network process (ANP). The hybrid approach of ANP-TOPSIS captures the interdependencies and feedback between levels, leading to the location selection approach simultaneously consider environmental, economic, political and social policies [17]. Applying the TOPSIS method, the locations are ranked. The research model presented in Fig. 1 is structured in six stages: problem identification, factor selection, sub-factor selection, calculation of criteria weights with ANP method, evaluation of alternatives by applying the TOPSIS method and discussions on the ranking of alternatives. Some details of the adopted and applied methodology are presented in the following:
• Data were collected for each country and for 2017 –2020 period. Next, ANP (Analytic network process) and TOPSIS (Technique for Order of Preference by Similarity to Ideal Solution), methods were applied to solve the problem of selecting the optimal variant of relocation. Throughout the work, the authors provide numerical results and graphic representations that support the proposed objectives. The data were collected from the Eurostat database for all the established criterion and sub-criterion for all selected countries and for a period of four consecutive years (2017, 2018, 2019 and 2020) [34]. A summary of the criteria to ensure homogeneity has been proposed. Most of them refer to the HR available in the countries considered in the analysis;
• Regional demographic statistics, related to the population structure data;
• Regional education statistics, gathers data on population participation in education and training, the structure of the population by level of education, dropout from education and training, on young people without employment and on employment rates of young people who do not follow the cycle of education and training;
• Regional science and technology statistics, reflected data on HR in science and technology;
• Regional labour market statistics, gathers data about the active population, unemployment, population structure, long-term employees;
• Regional digital economy and society, collects data on households with internet access, people who have used the internet, frequency of use and activities, interaction with public authorities via the internet, people who have ordered goods or services via the internet;
• Regional environmental and energy statistics, collects data on greenhouse gas emissions and air pollutants;
• Regional poverty and social exclusion statistics, gathers information on people at risk of poverty or social exclusion, living in very low-intensity households, the rate of severe material deprivation and the rate of poverty risk;
• Regional transport statistics, cumulates information about the transported.
Fig. 1
The proposed research model.
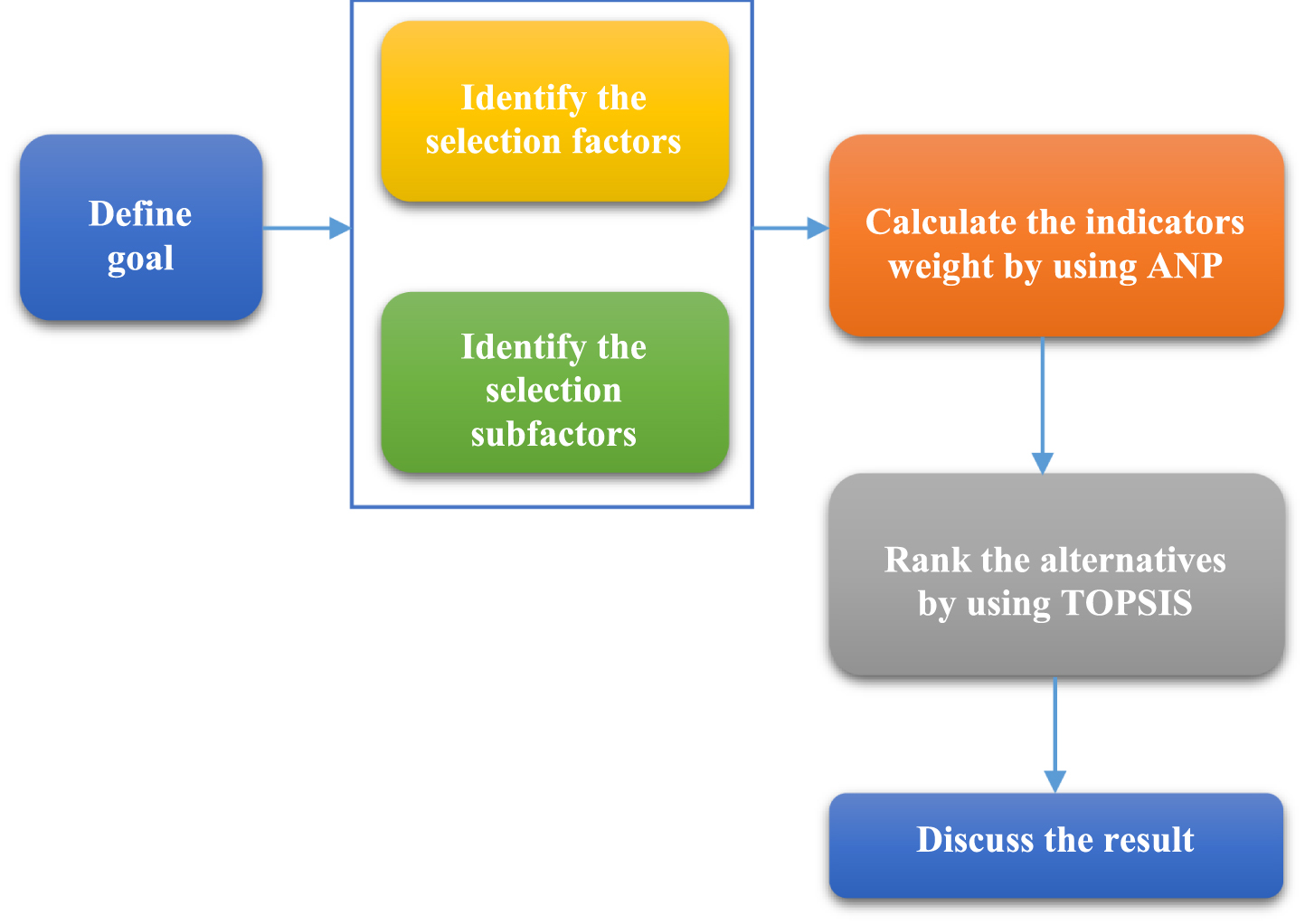
Location selection involves not only technical requirements but also economic, demographic, social, environmental, infrastructure, education data solutions to such challenges often involve complex decision-making processes. The selected criteria reflect the dynamics and structure of the population and the educational level. The selected measurement indicators capture the evolution of these factors, (Table 2). Research and development data are in line with the direction of collecting and reporting experimental research data. Another important aspect is given by the statistics of economy, connection, poverty, and social exclusion. Last, location selection cannot be done without infrastructure information. It can be seen from the list of criteria that most of them refer to the human resources available in the countries considered in the analysis.
Table 2
Criteria and sub criteria considered in the research approach
Criteria | Sub-criteria | |
Regional demographic statistics | C1 | Population structure indicators |
Regional education statistics | C2 | Participation rate in education and training |
C3 | Population by educational attainment level | |
C4 | Early leavers from education and training | |
C5 | Young people neither in employment nor in education and training | |
C6 | Employment rates of young people not in education and training | |
Regional science and technology statistics | C7 | R&D personnel and researchers |
C8 | GERD by sector of performance | |
C9 | HRST by category and NUTS 2 regions | |
C10 | Employed HRST by category, NACE Rev. 2 activity and NUTS 1 regions | |
Regional labour market statistics | C11 | Population by sex, age, citizenship, labour status and NUTS 2 regions |
C12 | Economically active population by sex, age and NUTS 2 regions (1 000) | |
C13 | Economic activity rates by sex, age and NUTS 2 regions (%) | |
C14 | Employment rates by sex, age, educational attainment level, citizenship and NUTS 2 regions | |
C15 | Average number of usual weekly hours of work in main job by sex, age and NUTS 2 regions (hours) | |
C16 | Unemployment rates by sex, age, educational attainment level and NUTS 2 regions (%) | |
C17 | Long-term unemployment (12 months and more) by sex, age, educational attainment level and NUTS 2 regions (%) | |
C18 | Labour market slack by sex, age and NUTS 2 regions | |
Regional digital economy and society | C19 | Households with access to the internet at home |
C20 | Households with broadband access | |
C21 | Individuals who used the internet, frequency of use and activities | |
C22 | Individuals who used the internet for interaction with public authorities | |
C23 | Individuals who ordered goods or services over the internet for private use | |
Regional environmental and energy statistics | C24 | Cooling and heating degree days by NUTS 3 regions - annual data |
Regional poverty and social exclusion statistics | C25 | People at risk of poverty or social exclusion by NUTS regions |
C26 | People living in households with very low work intensity by NUTS regions (population aged 0 to 59 years) | |
C27 | Severe material deprivation rate by NUTS regions | |
C28 | At-risk-of-poverty rate by NUTS regions | |
Regional transport statistics | C29 | Goods transported |
C30 | Summary of annual road freight transport by type of operation and type of transport | |
C31 | National annual road transport by group of goods and type of transport | |
C32 | International annual road freight transport - goods unloaded in reporting country, by group of goods and type of transport | |
C33 | Country level - gross weight of goods handled in all ports | |
C34 | Air transport of freight by NUTS 2 regions |
The aim of the research is to anticipate barriers, the competitiveness of relocation motivations, although in this uncertain context the motivations are clearly defined (Table 2) and the selected states are: Belgium (A1); Bulgaria (A2); Czech Republic (A3); Denmark (A4); Germany (A5); Estonia (A6); Ireland (A7); Greece (A8); Spain (A9); France (A10); Croatia (A11); Italy (A12); Cyprus (A13); Latvia (A14); Lithuania (A15); Luxembourg (A16); Hungary (A17); Malta (A18); Netherlands (A19); Austria (A20); Poland (A21); Portugal (A22); Romania (A23); Slovenia (A24); Slovakia (A25); Finland (A26); Sweden (A27); Iceland (A28); Norway (A29); Switzerland (A30); Montenegro (A31); North Macedonia (A32); Serbia (A33) and Turkey (A34).
The integrated ANP-TOPSIS model could be useful to model MCDM problems under uncertainty conditions. The logical scheme of the analysis steps is shown in Fig. 2 and the calculation mathematical model will be presented below.
Fig.2
The followed analysis steps (Logical scheme).
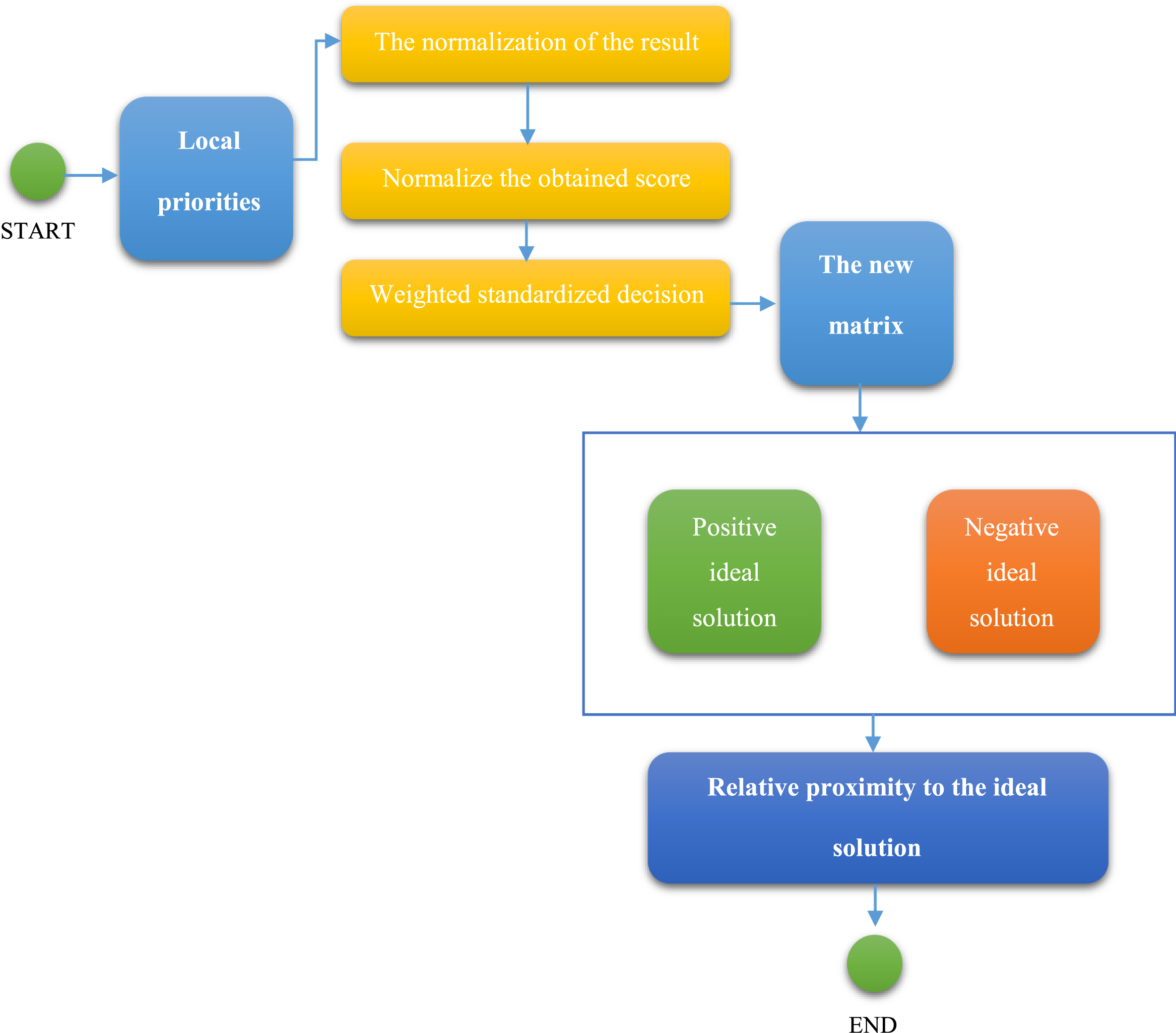
Local priorities are determined by calculating the geometric mean value of relative intensity:
(1)
Further, Appendix 1 presents the normalization of the result done by the normalization score (rij) calculations (for i = 1, …, m ; j = 1, …, n):
(2)
(3)
The process continued with the configuration of the standardized weighted decision matrix.
(4)
The next step is to determined the standardized decision matrix in function of corresponding weight; the new matrix is presented in Appendix 2 and the calculus is based on the following relation:
(5)
Finally for establishing the hierarchy, the TOPSIS method was applied [35]:
• Positive ideal solution is considered with respect to the following relation:
(6)
• Negative solution is determined based on the following relation:
(7)
Each positive and negative ideal alternative were calculated separately and centralized in Table 3 following the mathematical relations (8) and (9).
(8)
(9)
Table 3
Positive/negative solutions
Country | 2017 | 2018 | 2019 | 2020 | ||||
Si+ | Si– | Si+ | Si– | Si+ | Si– | Si+ | Si– | |
Belgium | 0.257 | 0.041 | 0.255 | 0.045 | 0.250 | 0.046 | 0.253 | 0.040 |
Bulgaria | 0.276 | 0.012 | 0.275 | 0.012 | 0.273 | 0.009 | 0.271 | 0.011 |
Czech Republic | 0.242 | 0.052 | 0.239 | 0.055 | 0.233 | 0.056 | 0.238 | 0.050 |
Denmark | 0.270 | 0.019 | 0.271 | 0.018 | 0.267 | 0.017 | 0.264 | 0.019 |
Germany | 0.035 | 0.282 | 0.035 | 0.281 | 0.037 | 0.276 | 0.031 | 0.277 |
Estonia | 0.285 | 0.006 | 0.284 | 0.007 | 0.279 | 0.006 | 0.279 | 0.004 |
Ireland | 0.275 | 0.014 | 0.274 | 0.014 | 0.270 | 0.014 | 0.269 | 0.013 |
Greece | 0.251 | 0.040 | 0.248 | 0.046 | 0.244 | 0.046 | 0.252 | 0.034 |
Spain | 0.160 | 0.134 | 0.161 | 0.135 | 0.155 | 0.138 | 0.152 | 0.135 |
France | 0.133 | 0.156 | 0.146 | 0.143 | 0.144 | 0.141 | 0.144 | 0.139 |
Croatia | 0.282 | 0.006 | 0.281 | 0.006 | 0.276 | 0.007 | 0.275 | 0.007 |
Italy | 0.200 | 0.097 | 0.198 | 0.099 | 0.191 | 0.102 | 0.190 | 0.101 |
Cyprus | 0.285 | 0.015 | 0.280 | 0.027 | 0.275 | 0.027 | 0.278 | 0.014 |
Latvia | 0.281 | 0.010 | 0.278 | 0.013 | 0.274 | 0.011 | 0.275 | 0.007 |
Lithuania | 0.281 | 0.009 | 0.278 | 0.014 | 0.274 | 0.013 | 0.274 | 0.010 |
Luxembourg | 0.279 | 0.034 | 0.278 | 0.033 | 0.273 | 0.034 | 0.274 | 0.033 |
Hungary | 0.266 | 0.037 | 0.263 | 0.038 | 0.259 | 0.039 | 0.260 | 0.035 |
Malta | 0.177 | 0.119 | 0.177 | 0.117 | 0.174 | 0.116 | 0.178 | 0.113 |
Netherlands | 0.225 | 0.089 | 0.226 | 0.086 | 0.222 | 0.087 | 0.219 | 0.084 |
Austria | 0.248 | 0.049 | 0.245 | 0.051 | 0.240 | 0.052 | 0.244 | 0.046 |
Poland | 0.169 | 0.124 | 0.174 | 0.119 | 0.166 | 0.122 | 0.166 | 0.120 |
Portugal | 0.273 | 0.017 | 0.273 | 0.016 | 0.269 | 0.015 | 0.269 | 0.014 |
Romania | 0.269 | 0.019 | 0.267 | 0.021 | 0.261 | 0.023 | 0.260 | 0.022 |
Slovenia | 0.281 | 0.007 | 0.280 | 0.007 | 0.276 | 0.007 | 0.275 | 0.007 |
Slovakia | 0.268 | 0.038 | 0.266 | 0.037 | 0.261 | 0.038 | 0.264 | 0.034 |
Finland | 0.261 | 0.028 | 0.260 | 0.027 | 0.257 | 0.027 | 0.257 | 0.026 |
Sweden | 0.244 | 0.045 | 0.241 | 0.047 | 0.240 | 0.044 | 0.237 | 0.046 |
Iceland | 0.177 | 0.119 | 0.177 | 0.116 | 0.173 | 0.115 | 0.170 | 0.116 |
Norway | 0.261 | 0.033 | 0.260 | 0.032 | 0.257 | 0.032 | 0.256 | 0.032 |
Switzerland | 0.256 | 0.043 | 0.253 | 0.047 | 0.250 | 0.044 | 0.244 | 0.050 |
Montenegro | 0.288 | 0.001 | 0.287 | 0.002 | 0.283 | 0.008 | 0.277 | 0.028 |
North Macedonia | 0.171 | 0.121 | 0.176 | 0.118 | 0.173 | 0.118 | 0.165 | 0.118 |
Serbia | 0.165 | 0.124 | 0.168 | 0.121 | 0.164 | 0.120 | 0.169 | 0.117 |
Turkey | 0.163 | 0.000 | 0.171 | 0.124 | 0.167 | 0.125 | 0.160 | 0.128 |
The relative proximity to the ideal solution could be determine based on (10):
(10)
4Results and discussion
4.1.Research results
If before the COVID-19 pandemic, relocation had a negative connotation in developed countries, now the perception is different. The relocation direction is from Eastern to Western Europe, as this research demonstrates. The study captures existing trends in the relocation issue generated by the COVID-19 pandemic. The multi-criteria model provides reliable results to obtain rational decisions and eliminates rank inversion problems [36]. The synthesis of the causes that determine the relocation, of the indicators and methods used contributes to the literature completion. The results obtained with ANP method illustrated the structure of the model and the interdependence of the criteria (Fig. 3). In the first stage the results were normalized (Appendix 1) and the decision matrix was identified (Appendix 2).
Fig. 3
The conceptual model of the research.
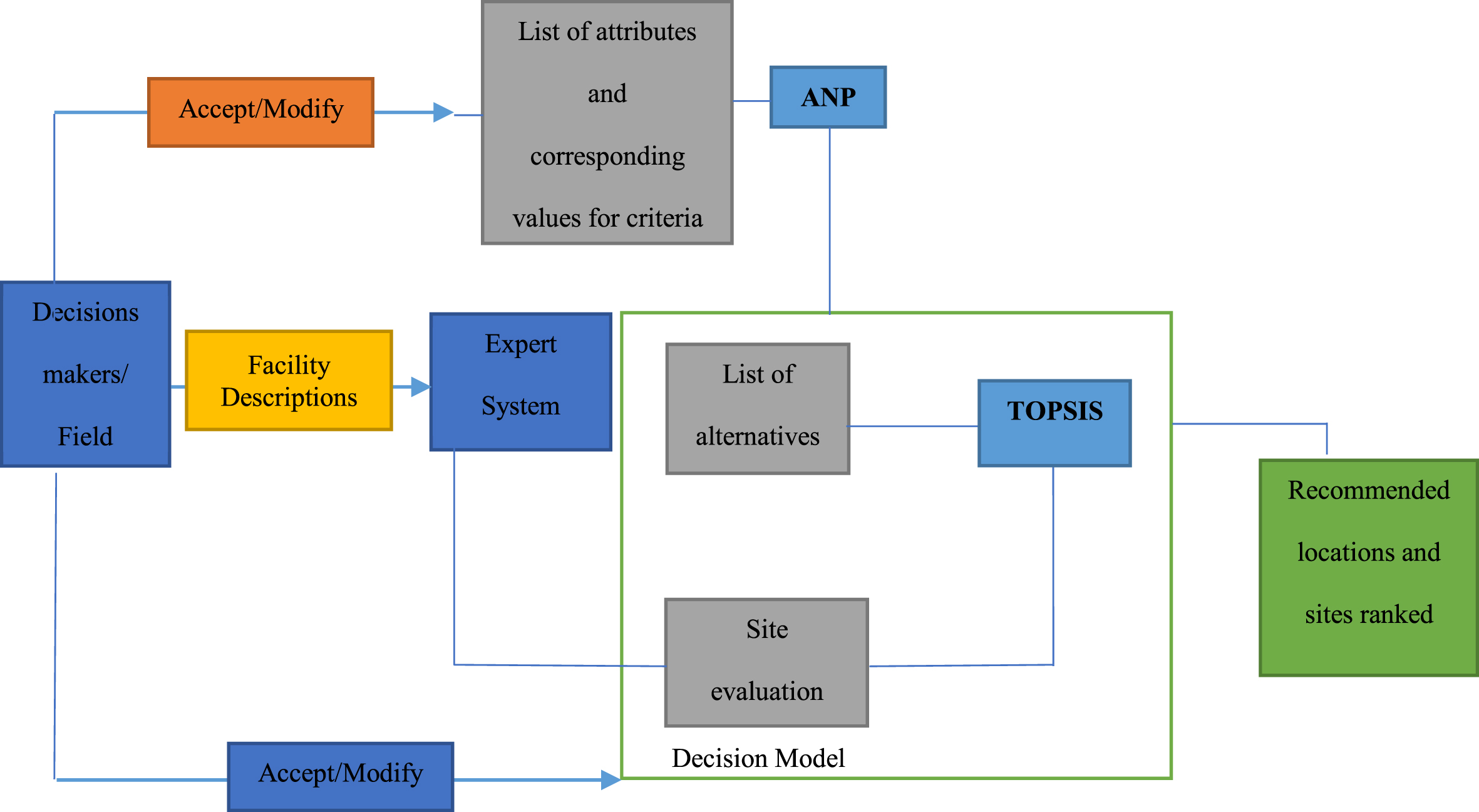
The infrastructure modernization contributes to the change of location selection and, to the stimulation of long-term economic growth. By creating jobs, infrastructure projects help reduce poverty while also improving the quality of life of locals. Infrastructure contributes to the movement of people and goods and influences companies’ revenues, policies, and strategies [37]. It is noted that the infrastructure had the largest share in three of the periods analysed (2017, 2018 and 2020). In 2019, the infrastructure was overtaken by two other criteria (research and development and the labour market).
The next step was to create the super-matrix that led to the criteria weighting (Appendix 3).
This analysis shows that integrated ANP-TOPSIS model have been applied to evaluate and prioritize relocation strategies in Europe. It was decided to apply the ANP due to the possible dependencies between criteria, indicators, and alternatives. Calculations’ results, the relative proximity of the ideal solution (Ci + related to(10)), are presented in Table 4 (calculation separated from the positive ideal point Si + based on (8) and the negative point Si + based on (9)).
Table 4
Relative proximity to the ideal solution (Ci+)
Country | 2017 | 2018 | 2019 | 2020 |
Belgium | 0.138 | 0.151 | 0.156 | 0.136 |
Bulgaria | 0.044 | 0.041 | 0.034 | 0.039 |
Czech Republic | 0.177 | 0.186 | 0.194 | 0.173 |
Denmark | 0.067 | 0.061 | 0.061 | 0.066 |
Germany | 0.888 | 0.888 | 0.882 | 0.896 |
Estonia | 0.020 | 0.023 | 0.021 | 0.017 |
Ireland | 0.049 | 0.047 | 0.049 | 0.047 |
Greece | 0.137 | 0.157 | 0.158 | 0.120 |
Spain | 0.457 | 0.455 | 0.470 | 0.469 |
France | 0.539 | 0.494 | 0.495 | 0.490 |
Croatia | 0.022 | 0.023 | 0.025 | 0.025 |
Italy | 0.327 | 0.332 | 0.348 | 0.347 |
Cyprus | 0.049 | 0.088 | 0.090 | 0.047 |
Latvia | 0.034 | 0.044 | 0.041 | 0.028 |
Lithuania | 0.033 | 0.046 | 0.047 | 0.037 |
Luxembourg | 0.110 | 0.106 | 0.110 | 0.110 |
Hungary | 0.123 | 0.128 | 0.131 | 0.120 |
Malta | 0.402 | 0.398 | 0.401 | 0.389 |
Netherlands | 0.283 | 0.276 | 0.283 | 0.277 |
Austria | 0.165 | 0.174 | 0.178 | 0.159 |
Poland | 0.424 | 0.405 | 0.424 | 0.420 |
Portugal | 0.059 | 0.055 | 0.055 | 0.052 |
Romania | 0.068 | 0.075 | 0.081 | 0.079 |
Slovenia | 0.025 | 0.026 | 0.027 | 0.025 |
Slovakia | 0.124 | 0.123 | 0.127 | 0.114 |
Finland | 0.097 | 0.096 | 0.095 | 0.092 |
Sweden | 0.158 | 0.164 | 0.155 | 0.163 |
Iceland | 0.401 | 0.397 | 0.400 | 0.406 |
Norway | 0.114 | 0.112 | 0.110 | 0.113 |
Switzerland | 0.146 | 0.156 | 0.151 | 0.171 |
Montenegro | 0.004 | 0.005 | 0.029 | 0.092 |
North Macedonia | 0.416 | 0.401 | 0.404 | 0.417 |
Serbia | 0.429 | 0.418 | 0.423 | 0.410 |
Turkey | 0.445 | 0.419 | 0.428 | 0.444 |
These results have given the countries ranking (Fig. 4) and the dynamics of the hierarchy in the analyzed period as presented in Appendix 4 and Appendix 5 which shows the dynamics of the score for each country considered in the research. The main results are:
• Germany is the best solution for relocation in all periods analyzed, followed by France with a difference of 0.384, Spain with 0.426 and Turkey with 0.454. Therefore, Germany is the best choice for business location due to its modern infrastructure and the stability of its political and economic environment. Eastern Europe has been a favourite area of large companies for the past two decades for relocating facilities [7, 38, 39]. Eastern European countries, with some exceptions, record a decrease in the score, especially after the Covid-19 pandemic;
• Poland, Hungary and Austria have been one of the most attractive countries for companies in the last two decades. However, there is a reduction in the score after 2019;
• The Czech Republic and Slovakia were two favourite destinations for Western European companies. In the case of the Czech Republic, there is a reduction in the score after 2019, while Slovakia registered a sharp decrease in the rating throughout the period;
• In Romania the results show a capping of the score after 2019;
• Bulgaria registered a decrease in the score for the entire analysed period. A similar trend is manifested in Greece, Cyprus, Malta and Serbia especially after 2019;
• In the case of Slovenia, a turning point in 2019 can be identified, materialized by the consistent decrease of the score;
• The Baltic countries have had an approximately similar evolution. If in the case of Estonia and Latvia there is a decrease in the score after 2018, in Lithuania the trend is to cap;
• There are several countries that have had an increase score, such as Croatia, Northern Macedonia and Montenegro;
• Germany, Switzerland, Spain and Italy are characterized by a considerable increase in the score. A similar dynamic, but with a lower growth rate we meet in Iceland;
• Norway, the Netherlands, Belgium had an oscillating evolution but with growth trends after 2019;
• We find a distinct situation in Turkey. Although there was a sharp decrease in the score in 2018 and 2019, there is an increase in 2020. The increase in score can be justified by the geostrategic position that this country has.
Fig. 4
The Rank of the considered countries in the research.
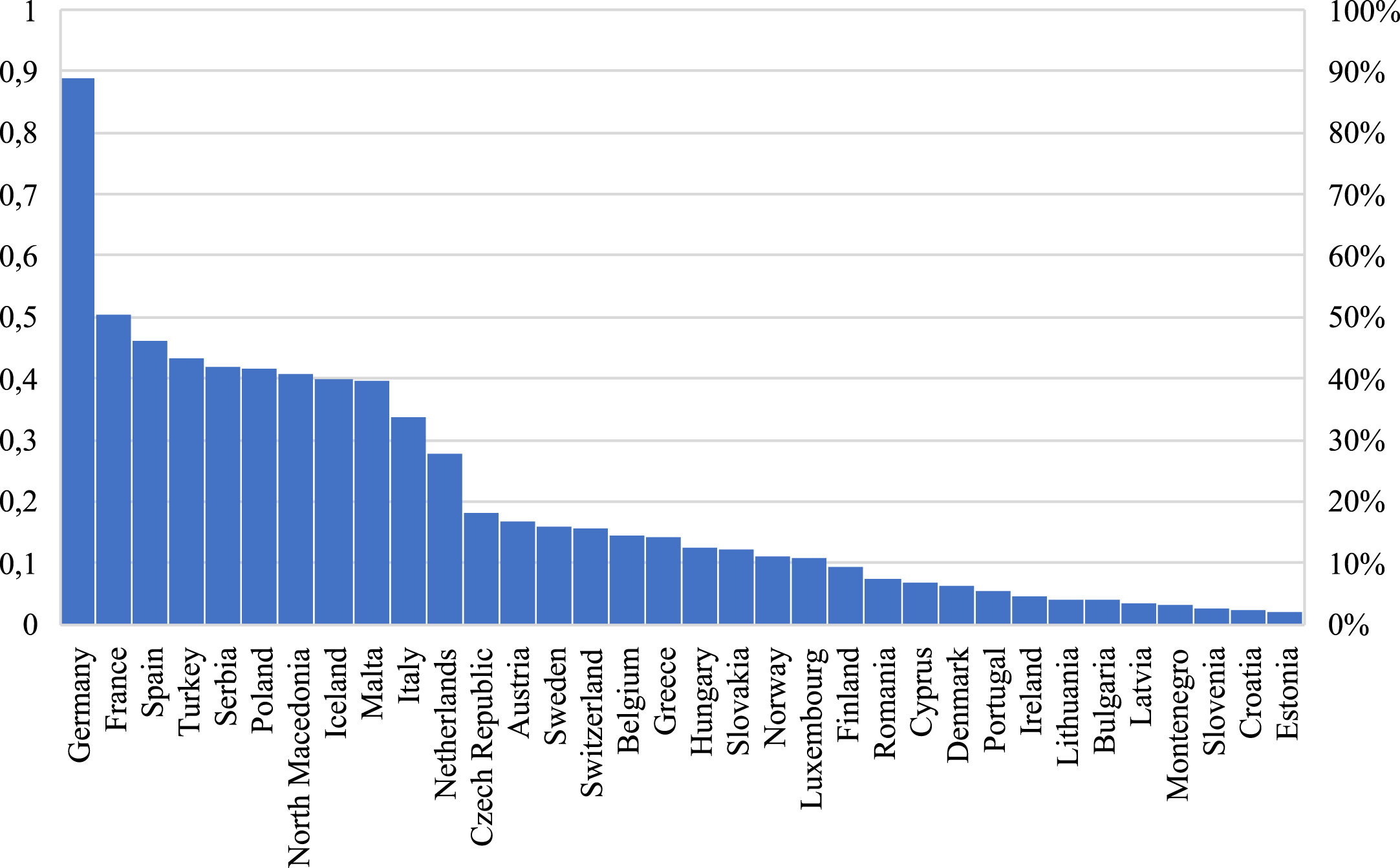
Appendix 4 emphasizes the dynamics of the hierarchy over the studied time periods; Appendix 5 highlights the dynamics of the score and the trend for each country considered in this study.
The criteria and sub-criteria considered in the analysis and their dynamics in the analyzed period were presented by the authors. Such a phenomenon will cause considerable changes in the HR market, related to the geographical areas of the material and financial flows, too.
4.2The research implications
The consequence of this study in the relocation-specific literature is significant. Given that relocation involves financial resources, the choice of location determines the long-term capital savings. Existing trends in national markets have a major role in substantiating the relocation decision. The financial balance of many companies after the COVID-19 pandemic is precarious. Since they cannot afford to skid, the study contributes to their better information. The study results contribute to the success of companies that relocate their business. The results obtained confirm other studies outcomes and implications, demonstrating back-shoring phenomenon imposed by the Industry 4.0 revolution [40–42]. Based on these we can say that military events in Ukraine will amplify these existing trends in the relocation of companies.
4.2Limitations
There are some limitations of the study determined by the field studied, the availability of the data collected, and the methods used. Studies may be limited to a single industry; this may be more significant than the criteria used to choose the place [24]. An optimized strategic alliance can only take place with the agreement of all parties [26].
One limitation of the analyzed methods is the interdependence of the criteria. The study opted for a wide range of locations, and the ANP-TOPSIS analysis reduced the interdependence of the criteria. The share of indicators depends not only on the economic context but also on the object of activity of the organizations. Secondly, it is based on the area of the European space. Restricting it could lead to different results, which requires further development. A significant limitation of the results’ study is given if the different legal system (specific laws and regulations at the national level) in the European Union member states is considered.
These limitations may be removed in future research and may lead to an understanding of the links between uncertainty and business results. Future studies could investigated how government affects relocation, the security provided by the country in which the company will relocate, the cost savings identified, and finally the COVID-19 epidemic, which caused damage to the global economy, led to the discovery of additional elements.
5Conclusions
Choosing an optimal location is a research concern, not only to identify a location that will give organizations the opportunity to reduce their costs, to be close to the raw materials or market, to be able to hire qualified human resources, but to prevent the risks associated with relocation. The current context is characterized by instability and insecurity. If the COVID-19 pandemic led to the reduction of companies’ activity, some industrial sectors were more affected than others, depending on the field in which they carried out their activity. Today’s reality calls for a risk-focused approach. Minimizing the risks of relocation has been an important criterion in research, as it is done with considerable financial effort.
The investigation of location selection as a step in organizational growth includes methods, resources, and mechanisms. The study findings indicate that infrastructure and human resources are key factors in the selection of sites. An essential part of economic operations is the choice of qualified individuals. It is important to remember that human resources play a crucial role in the efficient functioning of any company. Locations with a lower risk become preferred, even if they do not bring cost savings. Another crucial aspect is to keep the company’s assets safe, without exposing them to the risk. After the pandemic, the risk of relocation is a major concern for companies. In this paper, we argue a point of view on this process, offering a combined multi-criteria decision-making (MCDM) approach to evaluate the European countries that offer the most favorable resettlement conditions by interrogating a large database and type of criteria and indicator.
The methodology outlined in the study is based on assessing places with the aid of chosen indicators. Selection is based on the positional classification approach, and the rank of places is determined using the MCDM model. The suggested method enables us to choose a location that meets the specified criteria and has pertinent characteristics. Future research will look at how the hybrid MCDM method is applied for site selection, considering the significance of the criteria and recent developments in Europe.
The performance of the company depends greatly on the evaluation of location choice. The MCDM approach of location evaluation is more rational, practical, objective, and impartial. This approach suggested a new way of thinking to assess the site using HR indicators, which might boost the value of the business. The HR value in the Knowledge Economy Era is undeniable. As a result, it is crucial to consider both the economic state of development and the role HR are playing in this context. Managers now have a simple, scientific, and logical technique thanks to the ANP-TOPSIS. Countries were ranked and the criteria were evaluated using an integrated ANP-TOPSIS model. From the results of the analysis, it can be seen that “infrastructure” is the criterion that can help reduce risks, costs and economic development. The research results reinforce the importance of infrastructure in substantiating the relocation decision. The strategic positioning of the company, the expansion, or following the current context, the restriction of the geographical area are essential aspects in relocating a business.
Future research can be an important support for managers in terms of the risk of relocation decisions, in selecting the most appropriate strategy in function of the economic context and the specifics of organizations and finally for an assessment of future returns. Data collection can lead to inconclusive results depending on the database used and their timeliness.
Acknowledgments
The authors have no acknowledgments.
Author contributions
CONCEPTION: Catalin Gheorghe and Oana Panazan
METHODOLOGY: Catalin Gheorghe
DATA COLLECTION: Catalin Gheorghe and Oana Panazan
INTERPRETATION OR ANALYSIS OF DATA: Catalin Gheorghe and Oana Panazan
PREPARATION OF THE MANUSCRIPT: Catalin Gheorghe and Oana Panazan
REVISION FOR IMPORTANT INTELLECTUAL CONTENT: Catalin Gheorghe and Gavrila Calefariu
SUPERVISION: Catalin Gheorghe and Gavrila Calefariu
Supplementary material
[1] The supplementary material is available in the electronic version of this article: https://dx.doi.org/10.3233/HSM-220062.
References
[1] | Yu S , Draghici A , Negulescu OH , Ain NU , Social media application as a new paradigm for business communication: The role of COVID-19 knowledge, social distancing, and preventive attitudes, Front Psychol (2022) ;13: :1664–078. DOI: 10.3389/fpsyg.2022.903082. |
[2] | Zhou Y , Draghici A , Abbas J , Mubeen R , Boatca ME , Salam MA , Social media efficacy in crisis management: Effectiveness of non-pharmaceutical interventions to manage COVID-19 challenges, Front Psychiatry, (2022) ;12: (1099):626134. DOI: 10.3389/fpsyt.2021.626134. |
[3] | Liu Q , Qu X , Wang D , Abbas J Mubeen R , Product market competition and firm performance: Business survival through innovation and entrepreneurial orientation amid COVID-19 financial crisis, Front Psychol (2022) ;12: :790923. DOI: 10.3389/fpsyg.2021.790923. |
[4] | Pellenbarg PH , Wissen LJG , Van Dijk J , Firm relocation: State of the art and research prospects. Research Report 02D31, University of Groningen, Research Institute SOM (Systems, Organisations and Management), 2002. |
[5] | Murayama K , Nagayasu J , Bazzaoui L , Spatial dependence, social networks, and economic structures in Japanese regional labor migration, Sustainability (2022) ;14: :1865. DOI: 10.3390/su14031865. |
[6] | Conroy T , Deller S , Tsvetkova A , Regional business climate and interstate manufacturing relocation decisions, Reg Sci Urban Econ (2015) ;60: :155–68. DOI: 10.1016/j.regsciurbeco.2016.06.009. |
[7] | Kapitsinis N , The impact of economic crisis on firm relocation: Greek SME movement to Bulgaria and its effects on business performance, Geoj (2019) ;84: :321–43. DOI: 10.1007/s10708-018-9863-6. |
[8] | Ferdows K , Making the most of foreign factories, HBR (1997) ;75: :73–88. |
[9] | Eslamipoor R , Sepehriar A , Firm relocation as a potential solution for environment improvement using a SWOT-AHP hybrid method, Process Saf Environ Prot (2014) ;92: (3):269–76. DOI: 10.1016/j.pse2013.02.003. |
[10] | Brouwer AE , Mariotti I , Van Ommeren JN , The firm relocation decision: An empirical investigation, The Annals of Reg Science (2004) ;38: :335–47. DOI: 10.1007/s00168-004-0198-5. |
[11] | Lampón J , The impact of uncertainty on production relocation: Implications from a regional perspective, Pap Reg Sci (2019) ;94: :127–39. DOI: 10.1111/pirs.12493. |
[12] | Marinković S , Nikolić I , Rakićević J , Selecting location for a new business unit in ICT industry, ZBEFRI (2018) ;36: :801–25. DOI: 10.18045/zbefri.2018.2.801. |
[13] | Roy J , Sharma HK , Kar S , Zavadskas EK , Saparauskas J , An extended COPRAS model for multi-criteria decision-making problems and its application in web-based hotel evaluation and selection, Ec Res Ekonomska Istrazivanja (2019) ;32: (1):219–53. DOI: 10.1080/1331677X.2018.1543054. |
[14] | Nazari-Shirkouhi S , Mousakhani S , Tavakoli M , Dalvand MR , Saparauskas J , Antucheviciene J Importance-performance analysis based balanced scorecard for performance evaluation in higher education institutions: An integrated fuzzy approach., J Bus Econ Manag (2020) ;21: (3):647–78. DOI: 10.3846/jbem.2020.11940. |
[15] | Zamani M , Rabbani A , Yazdani-Chamzini A , Turskis Z , An integrated model for extending brand based on fuzzy ARAS and ANP methods, J Bus Econ Manag (2014) ;15: (3):403–23. DOI: 10.3846/16111699.2014.923929. |
[16] | MacCarthy BL , Atthirawong W , Factors affecting location decisions in international operations –a Delphi study, Int J Oper Prod Manag (2003) ;23: (7):794–818. DOI: 10.1108/01443570310481568. |
[17] | Nong TN-M , A hybrid model for distribution center location selection, Asian J Shipp Logist (2021) ;38: :40–9. DOI: 10.1016/j.ajsl.2021.10.003. |
[18] | Zakeri S , Yang Y , Zakeri M , Grey strategies interaction model, Strateg Manag J (2018) ;12: (1):30–60. DOI: 10.1108/JSMA-06-2018-0055. |
[19] | Martyniuk-Pęczek J , Gierusz A , Peczek G , Determinants of SME location in a suburban area: Evidence from the Gdańsk–Gdynia–Sopot metropolitan area, Urbani Izziv (2017) ;28: (1):122–34. DOI: 10.5379/urbani-izziv-en-2017-28-01-004. |
[20] | Li Y , Hu Z , A review of multi-attributes decision-making models for offshore oil and gas facilities decommissioning, JOES (2021) ;7: (1):58–74. DOI: 10.1016/j.joes.2021.05.002. |
[21] | Zolfani SH , Ghadikolaei AS , Performance evaluation of private universities based on balanced scorecard: Empirical study based on Iran, J Bus Econ Manag (2013) ;14: (4):696–714. DOI: 10.3846/16111699.2012.665383. |
[22] | Azimi R , Yazdani-Chamzini A , Fouladgar MM , Zavadskas EK , Basiri MH , Ranking the strategies of mining sector through ANP and TOPSIS in a SWOT framework, J Bus Econ Manag (2011) ;12: (4):670–89. DOI: 10.3846/16111699.2011.626552. |
[23] | Pamucar DS , Bozanić D , Rand A , Multi-criteria decision making: An example of sensitivity analyses. , Serb J Manag (2017) ;12: (1):1–27. DOI: 10.5937/sjm12-9464. |
[24] | Feldmann A , Olhager J , Fleet D . Shi Y , Linking networks and plant roles: The impact of changing a plant role, Int J Prod Res (2013) ;51: (19):5696–710. DOI: 10.1080/00207543.2013.778429. |
[25] | Popovic G , Stanujkic D , Karabasevic D , A framework for the evaluation of hotel property development projects, Int J Strateg Prop Manag (2019) ;23: :96–107. DOI: 10.3846/ijspm.2019.7435. |
[26] | Akhavan P , Barakb S , Maghsoudlouc H , Antuchevied J . FQSPM-SWOT for strategic alliance planning and partner selection; case study in a holding car manufacturer company, Technol Econ Dev Econ (2015) ;21: (2):165–85. DOI: 10.3846/20294913.2014.965240. |
[27] | Saaty T . The analytic hierarchy process-what and how it is used, Math Model (1987) ;9: (3-5):161–76. DOI: 10.1016/0270-0255(87)90473-8. |
[28] | Kaboli A , Aryanezhad MB , Shahanaghi K , Niroomand I , A new method for plant location selection problem: A fuzzy-AHP approach. IEEE International Conference on Systems, Man and Cybernetics. 2007;582-6. DOI: 10.1109/ICSMC.2007.4413890. |
[29] | Keshavarz GM , Amiri M , Zavadskas EK , Hooshmand R , Antuchevie J , Fuzzy extension of the codas method for multi-criteria market segment evaluation., J Bus Econ Manag (2017) ;18: (1):1–19. DOI: 10.3846/16111699.2016.1278559. |
[30] | Jakovljevic V , Zizovic M , Pamucar D , Stevic Ž , Albijanic M , Evaluation of human resources in transportation companies using multi-criteria model for ranking alternatives by defining relations between ideal and anti-ideal alternative, Mathematics. (2021) ;9: (9):976. DOI: 10.3390/math9090976. |
[31] | Xin L , An-Quan Z , Man-Hong K , Zhong-Rong T , On the application of fuzzy comprehensive appraisal in human capital evaluation of logistics enterprises. 16th International Conference on Industrial Engineering and Engineering Management. 2009;2012-5. DOI: 10.1109/ICIEEM.2009.5344263. |
[32] | Pan NF , Fuzzy AHP approach for selecting the suitable bridgeconstruction method, AIC (2008) ;17: :958–65. DOI: 10.1016/j.autcon.2008.03.005. |
[33] | Kabo-Bah KJ , Guoan T , Yang X , Na J , Xiong L , Erosion potential mapping using analytical hierarchy process (AHP) and fractal dimension, Heliyon (2022) ;7: :e07125. DOI: 10.1016/j.heliyon.2021.e07125. |
[34] | http://www.eurostat, [Accesed March 1, 2022]. |
[35] | Tzeng G , Huang J Multiple Attribute Decision Making Methods and Applications. CRC Press, Taylor and Francis Group, A Chapman & Hall Book, Boca Raton; (2011) . |
[36] | Gurbuz T , Albayrak YE , An engineering approach to human resources performance evaluation: Hybrid MCDM application with interactions, Appl Soft Comput (2014) ;21: :365–75. DOI: 10.1016/j.asoc.2014.03.025. |
[37] | Mamirkulova G , Mi J , Abbas J , Economic Corridor and tourism sustainability amid unpredictable COVID-19 challenges: Assessing community well-being in the World Heritage Sites, Front Psychol (2022) ;12: :687975. DOI: 10.3389/fpsyg.2022.797568. |
[38] | Kaivo-Oja J , Knudsen MS , Lauraéus T , Reimagining finland as a manufacturing base: The nearshoring potential of finland in an industry 4.0 perspective., Bus Manag Edu (2018) ;16: , 65–80. DOI: 10.3846/bme.2018.2480. |
[39] | Lorincz N , Being an investment target in CEE, Country attractiveness and near-shoring. Budapest Manag Rev (2018) ;49: (5):47–54. DOI: 10.14267/VEZTUD.2018.05.05. |
[40] | Ancarani A , Di Mauro C , Mascali F , Backshoring strategy and the adoption of Industry 4Evidence from Europe. , J World Bus (2019) ;54: (4):360–71. DOI: 10.1016/j.jwb.2019.04.003. |
[41] | Barbieri P , Boffelli A , Elia S , Fratocchi L , Kalchschmidt M , How does Industry 4.0 affect international exposure? , The interplay between firm innovation and home-country policies in post-offshoring relocation decisions. Int Bus Rev (2022) ;31: (4): DOI: 10.1016/j.ibusrev.2022.101992. |
[42] | Kamp B , Gibaja JJ , Adoption of digital technologies and backshoring decisions: Is there a link? Op Manag Res (2021) ;14: :380–402. DOI: 10.1007/s12063-021-00202-2. |