Are there any key factors that encourage food delivery applications use during the COVID-19 pandemic in Thailand and the role of HRM?
Abstract
BACKGROUND:
Recently, because of the COVID-19 pandemic, customer sentiment has evolved as the new “normal” for food consumption—as it affects customer behavioral change, including the way customers eat. Therefore, understanding customer demands and needs will help food delivery aggregators and retailers produce sustainable, competitive advantage and drive profitability.
OBJECTIVE:
This paper aims to investigate the determinant factors of Thai consumers’ intention to use on-demand food delivery applications.
METHODS:
Convenient random sampling was used to gather data from 250 participants who have used food delivery applications in Thailand and Amos version 23 to test model validity and the hypotheses.
RESULTS:
The results show that satisfaction is critical for the intention to use food delivery applications. Social influence, trust, convenience, and application quality were found to play an essential role in meeting the needs of satisfaction toward the intention to use, and application quality is the most important. However, habit showed a negative relationship, and price value was found to have a nonsignificant impact on satisfaction.
CONCLUSIONS:
Food delivery aggregators should ensure the quality of application updates and efficiency and that users have the best quality experience to maintain customer satisfaction. However, customers are also concerned about their health and safety, which has altered their purchasing behavior. Therefore, restaurant players should add a third delivery system that optimize online ordering and delivery.
Jankit Chotigo is a Ph.D. student at the Faculty of Technology Management, Ritsumeikan University, Japan. His research interests are e-commerce and technology management.
Yasuo Kadono is a professor and dean at the Graduate School of Technology Management, Ritsumeikan University, and a visiting professor at EMUNI University, Slovenia. He received his Ph.D. in business administration (University of Tsukuba) and his master’s and bachelor’s degrees in applied mathematics and physics (Kyoto University). He has extensive practical experience with McKinsey& Company (Japan, USA, Korea), Accenture, Sumitomo Metal Industries, and Management Science Institute. He has completed important research projects (e.g., METI) in academic, business, and government circles. His teaching and research interests include technology management, competitive strategy, business model creation, innovation management, and information technology.
1Introduction
As shopping restrictions such as social distancing and quarantine are now in place because of COVID-19, online shopping offers the benefit of convenience. As customers have embraced social distancing as a way to slow the spread of the pandemic, there has naturally been a drop-off in in-store shopping, and customers have opted to window-shop online instead. As a result, the e-commerce business sector is likely increasingly growing and will continue post-pandemic. It is predicted that the value of the e-commerce market in 2020 could be as high as $70 billion, or 35% growth from the previous year [1]. While e-commerce sales have soared, orders for delivery service have also increased, especially food delivery. Several aggregators play a role in food delivery service. However, the customers mostly use more massive, better-known aggregators, such as Grab, LINE MAN, GET, and Foodpanda, because of a stronger relationship and credibility. With the ease and simplicity of food delivery applications, customers can order food from a wide variety of establishments with a single tap on mobile devices. Simultaneously, diners have discovered a new opportunity and desire to avoid inconvenience (e.g., traffic jams, scorching weather, and a high PM 2.5 level). Moreover, with the provision of delivery services, such as time saved and convenience to minimize the demand of busy lives, the number of customers adopting delivery services has increased rapidly [2].
At present, customers, particularly busy people, love the food delivery options because they can enjoy fresh and healthy food, even though they are still in the workplace, at home, or under restrictions due to the pandemic (e.g., COVID-19). Previously, food delivery was expected to grow around 10% –20% per year. However, in March and April 2020, during the COVID-19 pandemic, it grew to more than 100%, with orders increasing two to three times [3]. Since food delivery service and application usage behavior are emerging in Thailand, it could affect customer behavioral changes in food consumption. For example, the growth of technology innovation (e.g., application) affected the Japanese information service industry [4].
As a result, this study aims to investigate the determinant factors influencing customer intention to use food delivery applications. This requires establishing an integrated model that investigates the relationship of several antecedents: the unified theory of acceptance and use of technology 2 (UTAUT2—price value, social influence, habit), the information systems (IS) success model (e.g., information quality, system quality, service quality), and the technology acceptance model (TAM) (e.g., attitude toward using). In addition, this paper includes trust and convenience toward satisfaction, providing an understanding of customer intention to use food delivery applications among Thai customers.
In terms of the food delivery context, most studies have focused on the examined factors influencing customer intention regarding new services. Examples include Online to Offline (O2O) service [5], trust transferred [6], usage transfer behavior [7], and moral obligation [8]. The most recent study on food delivery extended the UTAUT2 model with mobile application features to examine continuous use intention [9]. Therefore, this research will go further by considering and examining the impact and importance of satisfaction aspects on customers’ intention to adopt food delivery applications. Besides, satisfaction aspects have still not been fully studied and properly tested against Thailand’s food delivery context. Therefore, considering the satisfaction aspect is worth researching and might contribute to the successful implementation of food delivery applications. Although Thailand does not have the largest food delivery market share in Association of Southeast Asian Nations (ASEAN), it is still developing faster than other countries in the region. There are many reasons why Thailand is an attractive destination for e-commerce for food delivery service: the attractive incentive offered by Thailand for businesses, relatively advanced infrastructure, rising disposable income levels, and highly active online users, which are suitable for food delivery service businesses [10, 11]. However, notably, in food delivery, few studies have provided insight into the suitability of the model that has been used in this field, and the model has not been substantively researched. By addressing these gaps, this study provides a more precise understanding of customer intention to use food delivery applications through evaluating food delivery services to comprehend the importance of consumer psychology. Therefore, this study seeks to understand customers’ attitudes toward intention to use online food delivery applications, and experienced users were selected to examine the empirical findings expected to contribute managerial implications for marketers and academia.
In summary, the research questions of interest to this exploratory paper are as follows:
1. What factors influence customers’ attitudes in terms of satisfaction regarding Thai customers’ intention to use food delivery applications during the COVID-19 pandemic?
2. How do these perceptions affect Thai customers’ intention to use food delivery applications?
3. What strategies should be implemented to im-prove Thai customers’ adoption intentions and restaurant operators’ solutions?
The rest of this paper is organized as follows: the literature review in section 2, which provides a summary of food delivery service, UTAUT2, the IS success model, and vital relational factors; the methodology and research model in section 3; the most relevant finding and result in section 4; the discussion, which provides the theoretical and practical implications, and managerial contributions in section 5; and finally, the conclusion, which provides the research limitations and future research directions in section 6.
2Literature review
2.1Food delivery service
Until recently, people were ordering the food needed for collection in person, even in major cities. Now, new technology has made delivery more convenient for consumers and broadened the range of food available for delivery. At present, the service and industry sectors in Thailand must adjust to support the changing of technology, or what is known as “digital disruption,” which not only causes a change in business methods and production processes but also affects the economy and society of the country through consumer behavioral changes. An example of technology that plays a role in the restaurant business is a food delivery application.
The survey of Kasikorn Research Center [12], found that 63% of the respondents thought that having a food delivery application makes it easier to switch to ordering food and that 88% of the respondents ordered food through the application because of promotions and discounts. The expansion of food delivery and food delivery applications creates the opportunity to generate revenue for those who work in a restaurant chain, which is expected to produce revenue sharing of around $0.8 billion [12]. Food delivery applications had a revenue share of roughly $106 million from the total value of the food delivery business in 2019 (between $1 billion and $1.1 billion), growing by 14% from the previous year and accounting for 8% of the total value of the food business in Thailand in 2019 [12].
The Electronic Transactions Development Agency conducted a food delivery survey and found that 85% of respondents ordered food online, with 51.09% of them being Gen Y. Also, 89% of respondents chose to order the food through the food delivery application, and 61.06% ordered fast food. Gen X spent the most, around $15–$32. Furthermore, the Electronic Transactions Development Agency reported that concern over the situation of the COVID-19 pandemic is one of the critical factors that affect the behavior of consumers in deciding to order food online. The most concerned about the spread of COVID-19 is Gen Y at 40.2% —followed by Gen Z at 30.0%, baby boomers at 28.6%, and Gen X at 26.9%, with a total average of 33.96% [13]. The baby boomer segment was born between 1944 and 1964, Gen X was born between 1965 and 1980, Gen Y was born between 1981 and 1996, and Gen Z was born between 1995 and 2015.
While most restaurants have been turned upside down by the disruptions caused by the Thai government’s reaction to the COVID-19 pandemic, all restaurants have discontinued sit-in dining and now offer takeout through a delivery service. Therefore, the delivery services provide a valuable service by getting food to customers stranded at home while applying social distancing for the safety of their drivers and hungry customers. Food delivery services are among the few local businesses that will survive and thrive during Thailand’s outbreak. The restaurant business has experienced a particularly strong impact of the COVID-19 pandemic. Many restaurants have adopted food delivery to continue doing business. After relying on food delivery and home-cooked meals during the lockdown, diners are now gradually adapting to the “new normal.” At the same time, they remain alert and care for their activities (e.g., eating). The new normal has created a great opportunity for food delivery aggregators because customers are concerned about their health and avoid crowded areas such as shopping malls, restaurants, and cafés. Although these places prepare protection, customers still cannot enjoy the experience as before, so they use a delivery service as their solution. The major food delivery aggregators (such as Grab, LINE MAN, GET, and Foodpanda) served customers with rapid response, offering maximum convenience and transparency with orders (see Table 1). These food delivery aggregators not only enable users to quickly browse menus, prices, and reviews but also allow them to track the progress of their food deliveries in real time. Moreover, online delivery platforms benefit users by enabling them to conveniently explore many local restaurants’ food choices they may not have known existed.
Table 1
Third-party aggregators and their highlights
Aggregators | Highlight |
Grab | Direct contact with the driver |
Calculations estimating waiting time, exact food price, and delivery charge before ordering | |
The delivery driver calls every step of the way | |
LINE MAN | The service always offers promos for discounts |
More choices at night compared to other competitors | |
Foodpanda | Step-by-step notification and it is easy to use. |
Food can be preordered and delivered for free to nearby restaurants. | |
GET | Free delivery vouchers for new users. |
The service can make more than one order and send them to different addresses. |
Besides offering local cuisines and street food, restaurants have an excellent opportunity and advantage afforded by aggregators to attract customers through technology. Restaurants can avoid paying a rental fee because they do not need to provide ample space for customers, so this is a competitive point that may save cost and provide a cheaper food cost for the customer. Still, they must face the service and commission fee from food delivery aggregators [8], so restaurant players should focus on balancing costs and customer needs.
2.2Role of human resource management in food delivery service
Through examining restaurants or cafés and food-related businesses, it might seem like the place for human resource management (HRM) is gradually dwindling. On the contrary, online food businesses need more HR roles than ever. As the online food service and applications continue to boom and expand, particularly during the COVID-19 pandemic, HRM’s role also increases. Although the online food service might be functioning mainly online, employees are still the backbone of the businesses; thus, HR tools are needed. One of the common traits of a successful food delivery service is courteous and reliable staff that work tirelessly to deliver top-notch service to customers. However, with severe competition in the food delivery service sector, hiring the right team and creating the correct HR structure remains one of the biggest challenges. HRM is an integral part of business administration and encompasses important personnel activities to ensure the effective contribution of human capital to the creation and achievement of business strategies [14]. Furthermore, effective HRM is also considered a crucial element of service quality and customer satisfaction [15]. HRM is particularly critical for service businesses that result in a relationship between employee experiences of the organization and customer service experiences [16]. Organizations need to find ways to manage the employment relationship to motivate their employees to provide the support and interaction customers expect. There is a need to align their business strategies and their HRM strategies to pursue long-term competitive advantage [17]. Parry and Tyson [18] revealed that HRM could improve efficiency, service delivery, and market visibility; create a wider customer base; empower managers; and transform HR into a more strategic function. Hence, if companies do not give due emphasis to HR strategies, investment in technologies such as applications or online services may not provide the sought competitive advantage. For service industries whose products involve personal interaction, strategies to achieve competitive advantage inevitably involve employee management.
An emerging sustainable HRM approach might be effective for a firm that adopts a long-term perspective to improve employees’ quality of life to achieve a social sustainability outcome [19]. Moreover, the firm should be aware of creating conditions that facilitate the balance of sustainability behaviors between work and life at home [20]. The sustainable HR approach to performance management as part of the bundle of HRM practices will balance organizational financial goals and provide positive human and social outcomes to gain a competitive advantage. This suggests that sustainable HRM must be carefully tailored to each firm’s situation. Therefore, to obtain greater strength, the sustainable HRM system should enhance the clarity of interpretation of HR practices among employees. Also, business leaders must recognize HR’s strategic role in delivering value to the organization [21].
For companies to survive and be successful in the rapidly changing business world, it is necessary to understand and accept its challenges and adapt business activities to the requirements raised by its characteristics and trends. Identifying risks connected to HR is the first step of such a strategy [22]. One of the biggest challenges for HR managers is how to engage employees effectively during the COVID-19 pandemic. Ahmed et al. [23] found that knowledge sharing could be a possible way to engage employees during the pandemic. Therefore, to maintain the organization’s high performance, employee engagement and knowledge sharing could be the best solution to meet the current HR challenges.
2.3UTAUT2 (price value, habit, and social influence)
The UTAUT model was developed by Venkatesh et al. (2003) and is widely used by many researchers in many fields. It has been useful to explain about 70% of the variance in behavioral intention and 50% of the variance in technology use [24]. The UTAUT model has four fundamental constructs—performance expectancy, effort expec-tancy, social influence, and facilitating conditions—that influence behavioral intention to use technology. The UTAUT model was initially designed to explain employee technology acceptance and use but not provide context to the consumer. Therefore, Venkatesh et al. [25] proposed a new comprehensive UTAUT model called UTAUT2 to improve analytical ability by paying particular attention to customer use through incorporating the original model with three key constructs: hedonic motivation, price value, and habit (see Fig. 1). Performance expectancy is a concept in line with perceived usefulness. Effort expectancy is also in line with perceived ease of use in TAM, but both expectancies are replaced by convenience to provide a more comprehensive model. Some studies have found that effort expectancy is not significant with regard to the intention to use technology because of the ubiquity of smartphones and the similarity of common features that put the customer at ease in using new applications [26–28]. Lee et al. [26] found that facilitating conditions and hedonic motivation are not key factors that encourage customers to use delivery applications. This may be explained by time usage, which is quite short when customers use delivery applications to order food, so customers still do not enjoy fun and enjoyment from using delivery applications.
Fig. 1
The unified theory of acceptance and use of technology 2 model.
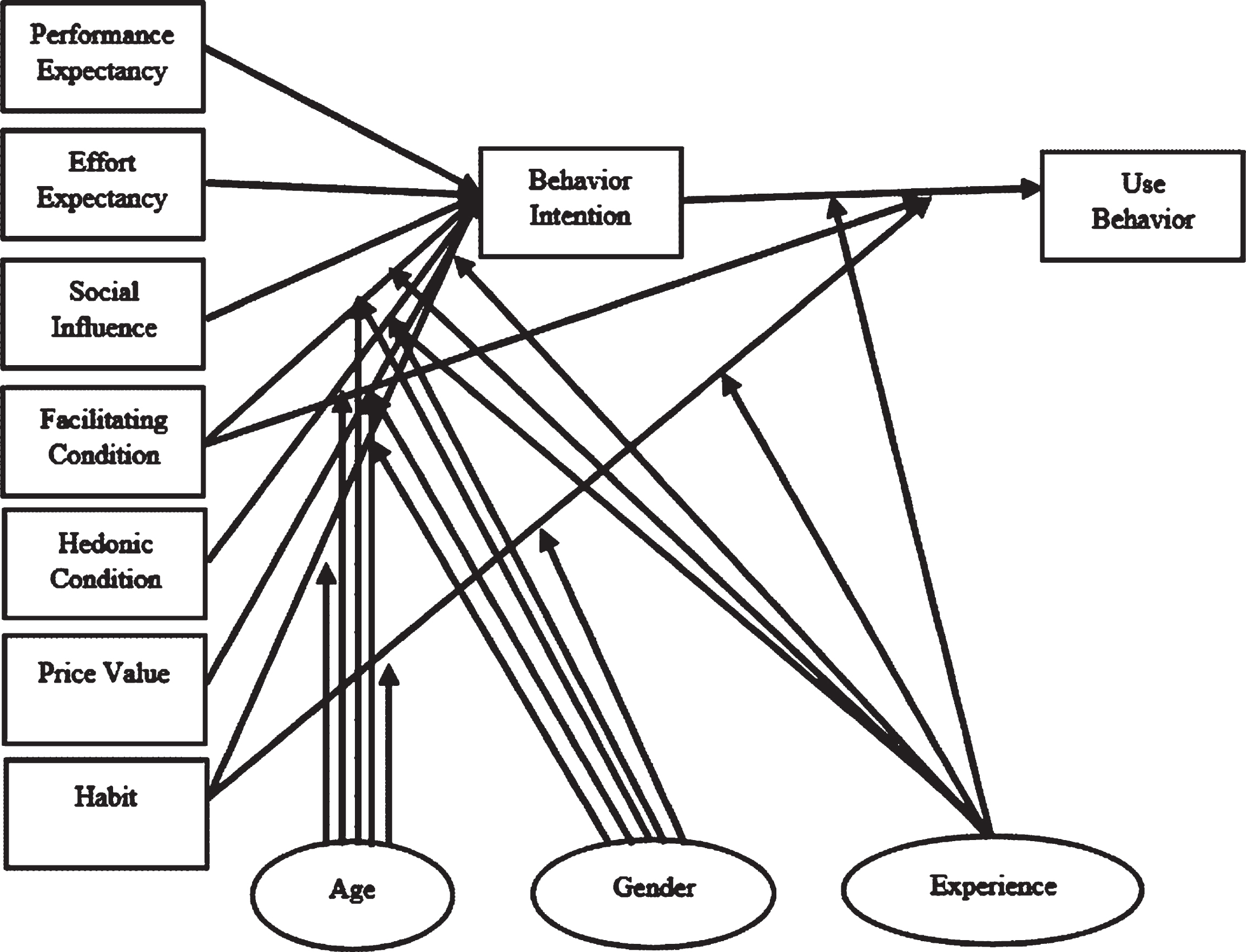
Price is important for the adoption of new technology. Online shopping can offer a lower price to customers because of the lower operating cost of an online business. The monetary benefit from online shopping can be derived from a lower price, promotions and discounts, and a loyalty point system. We followed the idea of Dodds et al. [29] and define price value as a customer’s cognitive trade-off between the perceived benefit of a food delivery application and the monetary cost of using it. The price value is positive when the customer is satisfied that the delivery application’s use is greater than the monetary cost.
Following the idea of Venkatesh et al. [24], we define social influence as the degree to which individuals perceive that important others believe they should use a food delivery application. Social influence as a direct determinant of behavioral intention is represented as the subjective norm in Theory of Reasoned Action (TRA), Technology Acceptance Model 2 (TAM2), Theory of Planned Behavior/Decomposed Theory of Planned Behavior (TPB/DTPB), Combined TAM-TPB (C-TAM-TPB), social factors in Model of PC Utilization (MPCU), and image in Innovation Diffusion Theory (IDT) [24]. The new user who never uses the food delivery application will show the intention to check and gather information on a service or product before deciding by asking, questioning, or searching to increase their perception and understanding. As a person in society, a user is very likely surrounded by friends, family, and colleagues and susceptible to their suggestions. When friends, family, and colleagues of a user start to use a particular food delivery application, the user will be influenced, and the user will adopt it more quickly. As food delivery applications are widely used in society, users strongly consider the opinion of the people they trust since users regard a significant person’s attitude as one of the decision criteria. Likewise, personal satisfaction is given the most priority. Customer satisfaction is often influenced by the quality of the interpersonal interaction between the user and the contact person [27]. In the early stage of adopting the new technology (i.e., a new application), if customers are satisfied with using it for the first time, they will show the intention to use it more often.
Habit is defined as an individual who automatically performs behaviors because of experience using a food delivery application [25]. The empirical findings of Venkatesh et al. [25] about the role of habit in technology use delineate different underlying processes by which habit influences technology use. Habit represents the involuntary behavior to use technology for any activity. Habitual users of food delivery will tend to browse online for food and order it as part of their natural response. As Khalifa and Liu [30] suggested, if customers have the same level of satisfaction with an online store, a customer with high levels of habit will be more likely to repurchase from the same online store than those without such a habit. Such automaticity allows habitual behaviors to be performed easily and quickly [31]. Prior research has reported that habit is a major driver of affect [32]. Affect is defined as “feelings of joy, pleasure, depression, displeasure, or hate associated by an individual with a particular act” [33], an emotional response to the thought of the behavior [32]. Satisfaction is also an effect [30]. HT directly affects satisfaction [32]. Therefore, a customer is likely to be more satisfied with food delivery via an application if they have acquired the habit of application use. We, therefore, offer the following hypotheses:
H1: Price value (PV) positively affects customer satisfaction toward the use of food delivery applications.
H2: Social influence (SI) positively affects customer satisfaction toward the use of food delivery applications.
H3: Habit (HT) positively affects customer satisfaction toward the use of food delivery applications.
2.3.1Convenience
As the definition of convenience is not easy to explain, many prior studies have focused on convenience in the time element. Brown and McEnally [34] suggested that it is important to consider convenience at all stages in the process of food consumption and determine the proportionate importance that consumers attach to the time and energy used in the acquisition, consumption, and disposal. Darian and Cohen [35] added the new elements of consumer decision-making, preparation, and clearing up to the three scales mentioned above. Therefore, this study conceptualizes convenience as the degree to which a consumer is inclined to save time—considering place, acquisition, use, and execution—during the use of a food delivery application [7]. The importance of convenience in online marketing in the food industry is increasing, especially in an emergency crisis (i.e., COVID-19) in which the influence of convenience should be emphasized. The study of Jiang et al. [36] stated that convenience is one of the principal motivations for users to adopt electronic technology because customers must be convinced of their value before they are willing to use new technology. The convenience of the internet has an impact on customers who are willing to purchase a product. It is possible to place an order anytime and anywhere, and this channel meets the customer’s desire to save time and effort [36]. Moreover, online shopping convenience is one of the essential keys to creating a pleasant shopping experience. Many prior studies have reported that a greater convenience orientation positively affects the usage of convenience food [37], ready meals, and takeout [38]. In the online shopping experience, we found that convenience constructs directly affect satisfaction. Prior studies involving a convenience context have reported that the overall measure of convenience influences satisfaction [39, 40]. We, therefore, offer the following hypothesis:
H4: Convenience (C) positively affects customer satisfaction toward the use of food delivery applications.
2.3.2Application quality
The IS success model was developed by Delone and McLean in 1992 [41]. Many prior studies have attempted to redefine or extend the original IS success model from a different perspective to provide a more comprehensive model. Some researchers found the weakness of the original IS success model [42]. Therefore, Delone and McLean responded and redeveloped the IS success model in 2003 [43]. The updated model consists of six factors: system quality, information quality, service quality, intention to use, user satisfaction, and net benefit, as shown in Fig. 2. The results demonstrated that three characteristics of quality used to evaluate the system affected the intention to use and satisfaction negatively or positively, depending on the net benefit provided. The original IS success model [41] was used by many researchers to evaluate the success of various types of IS—for example, e-commerce [44], the web portal [45], O2O service [7], and mobile catering applications [46]. This study proposes application quality, which combines three main factors of the IS success model: system quality, information quality, and service quality. We define application quality as a multidimensional interface stimulating positive or negative user responses resulting from the interaction between users and an application [47]. Pantano and Priporas’s [48] study found that to encourage customers to adopt mobile commerce, the following features are required: reasonable price, shopping efficiency, transaction security, and service quality. Furthermore, Chi [47] reported that the perceived quality measure is consistent with information quality, service quality, and system quality and that perceived quality positively affects customer satisfaction. The quality of a food delivery application—which is related to visual merchandising, information, the transaction process, and service response—influences customer pleasure and arousal to support quality customer experiences and purchasing decisions. We, therefore, offer the following hypothesis:
Fig. 2
The information systems success model.
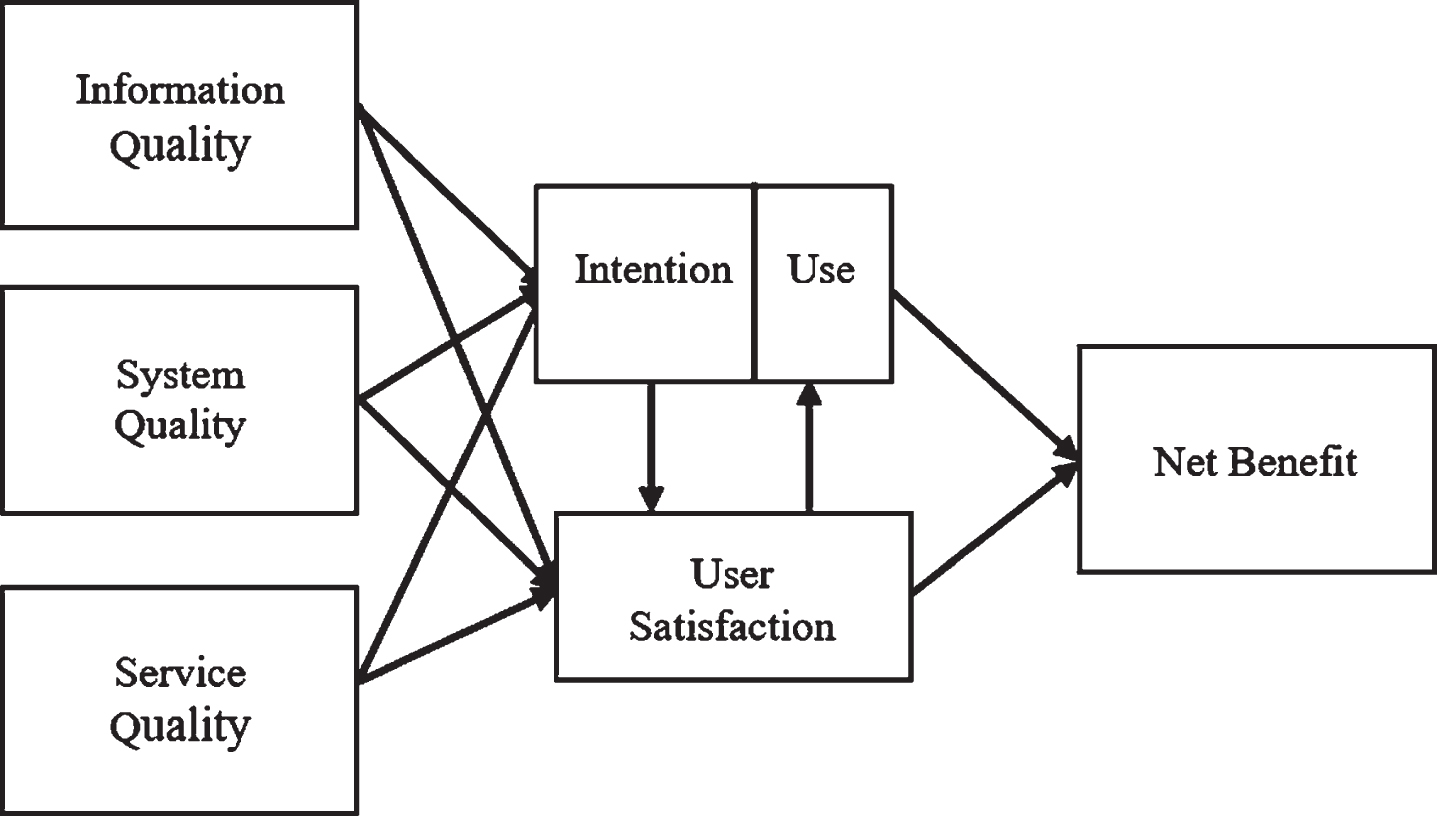
H5: Application quality (AQ) positively affects customer satisfaction toward the use of food deli-very applications.
2.3.3Trust
Another critical point in getting consumers to use new technology (e.g., an application) is to build trust. Trust is widely used and has been described in various fields, such as management [49], marketing [50], IS [51, 52], social media [53], and e-commerce [54]. We describe trust as a belief that a food delivery application from which a customer obtains service performs its business with a customer in a responsible, dependable, and competent manner, as well as behaves in a way that is not harmful to its customer and considers customers’ best interests [55]. In the e-commerce context, trust is seen as being of considerable importance in the process of building and maintaining customer relationships. In offline commerce, face-to-face interaction or interpersonal communication can create trust. However, mainly as a new online business, it is challenging to build trust with consumers. Therefore, a new business player should provide adequate information—such as company info, policies, and customer service and support, which are necessary to establish customer trust—to customers. Also, the information must be accessible, and effort should be made to avoid confusing customers. Conversely, a well-known business can quickly create consumer confidence, which is particularly useful when companies launch new services because consumers already have confidence in the efficiency and reputation of these companies. A successful relationship with customers, particularly in the online context, requires businesses to describe their information collection process, dissemination practices, and policies. In turn, customers must trust the company’s policies before providing personal information in order to enable businesses to advance customer relationships [56]. Therefore, trust is considered to be an essential factor in developing online relationships with customers; the online seller should establish a high level of trust to encourage customer confidence and reliability to engage their intention to use [57]. The benefit of an offline business is that it is more reliable than an online business because of face-to-face interaction. Therefore, if online businesses that provide services using an application that uses intermediaries to deliver goods and services through individuals (such as food delivery) allow consumers to choose the person who provides the service, it will help the business create more credibility and satisfaction. Trust and satisfaction are two key ingredients for long-term business relationships with customers, and trust directly influences satisfaction [58]. The relationship between trust and satisfaction is supported by prior studies—which have confirmed that trust and satisfaction have positive relations [59], with trust being the most significant determinant of customer satisfaction [60]. We, therefore, offer the following hypothesis:
H6: Trust (T) positively affects customer satisfaction toward the use of food delivery applications.
2.3.4Satisfaction
TAM was proposed by Davis [61]. TAM explains technology adoption with only two factors—per-ceived usefulness and perceived ease of use—and incorporates them with attitude toward technology adoption (see Fig. 3), but in the end, the factors omit the attitude because of a low mediating effect on technology adoption. The attitude expressed by an individual’s positive or negative feelings evaluates technology use [62]. The attitude has both positive and negative expressions, but if consumers have a negative mind-set to use from the beginning, it may cause them to avoid using such technology. Therefore, we propose satisfaction, which shows a positive expression. As an effect of intrinsic motivation, a service provider’s user-friendliness may increase satisfaction with the use of its food delivery application. Customers tend to be satisfied with the use of technology if they perceive it meets their expectations. We define satisfaction as customer judgment that the food delivery application’s product or service provides a pleasurable level of consumption-related fulfillment [63]. The satisfied customers can create a viral effect using technology, especially new technology, if they recommend or share their impressions with others [64]. High customer satisfaction should reduce the operating cost of a company in the future because the company does not need to spend more on acquiring customers [65]. Satisfaction plays a significant role in building and maintaining customer relationships and other vital assets [66]. Tsai et al. [67] demonstrated that satisfied customers are more likely to continue with their intention with a particular organization than dissatisfied customers. Previous empirical studies have supported satisfaction as a positive influence on future intention [68], a key influence on forming a customer’s future intention [69]. To achieve a company’s ultimate goal, the company should devote sincere attention to satisfied customers to increase its competitive advantage. This point is even more critical in e-commerce because the customer does not directly deal with the seller and cannot judge the seller trustworthy [70]. Anderson and Srinivasan [71] reported that customers willing to repurchase from e-retailers believe in assuring an ongoing satisfaction level. We, therefore, offer the following hypothesis:
Fig. 3
The technology acceptance model.
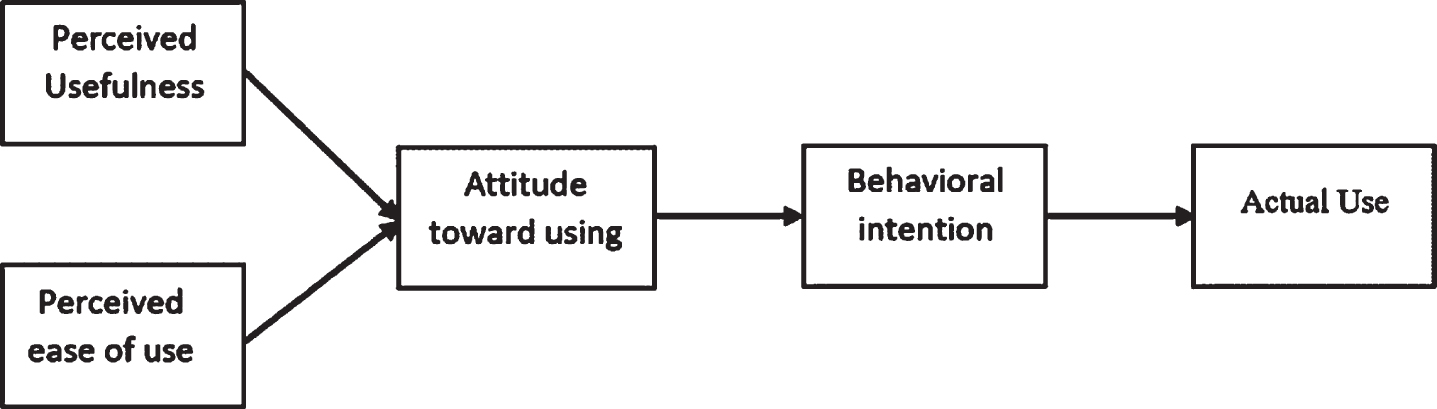
H7: Satisfaction (SAT) positively affects customer intention to use food delivery applications.
3Methodology
Figure 4 illustrates an overview of the research methodology. For the theoretical assessment, the first step was to conceptualize the constructs of interest, including defining each construct and identifying its constituent domains. Next, items or indicators for each construct were selected based on the literature reviews of these constructs. Each of the selected items was re-examined by content and for theory-based validity. If an adequate set of items was not achieved at this stage, new items had to be created based on the intended construct’s conceptual definition. For the empirical assessment, a research instrument was created, comprising all the delicate construct items, and administered to representative respondents from the target population. Next, the steps mentioned in section 3.2 to section 4.2 were carried out to assess validity and reliability and assure that our operationalized measures were adequate and accurate.
3.1Structural model
This study aims to examine the key factors that facilitate customers’ experiences in terms of satisfaction with the intention to use food delivery applications. The UTAUT2 model was considered theoretically and practically useful to utilize as the theoretical basis in this research. To make it fit food delivery applications, some modifications were necessary. Therefore, this study is designed based on the main indicators of UTAUT2 (social influence, price value, and habit), combined with quality constructs from the IS success model and three variables (convenience, trust, and satisfaction) explicitly proposed to be important in the food delivery application context. The modifications of the model are presented below.
First, performance expectancy, effort expectancy, hedonic motivation, and facilitating conditions were excluded from the model, although they have been proven in prior studies to be significant [25]. Considering an example of dining activity, performance expectancy is not required. Likewise, given the overlap between performance expectancy and perceived usefulness, it has been found that the impact of performance expectancy toward intention might be uncertain, as findings for the impact of performance expectancy on adoption have been mixed [9, 73, 74]. Since mobile applications are omnipresent nowadays and due to the intuitive use of food delivery applications, effort expectancy does not exist. Previous studies have also proved the nonsignificance of effort expectancy [9, 27]. As consumers use food delivery applications as a beneficial service rather than to have fun or entertain, hedonic motivation was also not considered in the model. Also, prior studies have failed to prove the role of hedonic motivation [75, 76]. Experienced users are not concerned about facilitating conditions because they have gained experience and know how to use food delivery applications. Also, no huge effort is needed to use these applications as a navigator to guide the user. Moreover, these applications are designed to fully support users. Recent studies also support the nonsignificant impact of facilitating conditions [27, 73].
Second, application quality and consumer satisfaction levels were included in the research model because perceived quality and satisfaction are at the core of motivating consumers to adopt food delivery applications. Previous research suggests that consumer perceptions of quality determine technology acceptance [46, 47]. The importance of customer satisfaction does not discriminate, as it can make or break a business’s success. The more satisfied users are, the more likely they are to spend more and recommend a food delivery application to friends and family members. The studies of Alalwan [9] and Wang et al. [46] prove that satisfaction facilitates and increases customer intention.
Third, with the advent of food delivery services, the standards of convenience have risen even further. To succeed, restaurant operators will have to continuously up the standard and make it more convenient for consumers to get fresh food delivered to their doorsteps. More importantly, there is a consensus in the literature on food delivery services that convenience plays an important role [8]. Trust is another critical factor in consumers’ online purchasing decisions. The lack of trust is a fundamental reason why consumers do not purchase a product from a site. Many prior studies on mobile commerce have noted that the greater consumers’ trust, the more likely they are to engage their intention [74, 77]. The conceptual model, including the proposed path hypothesis, is shown in Fig. 5.
Fig. 5
The research model.
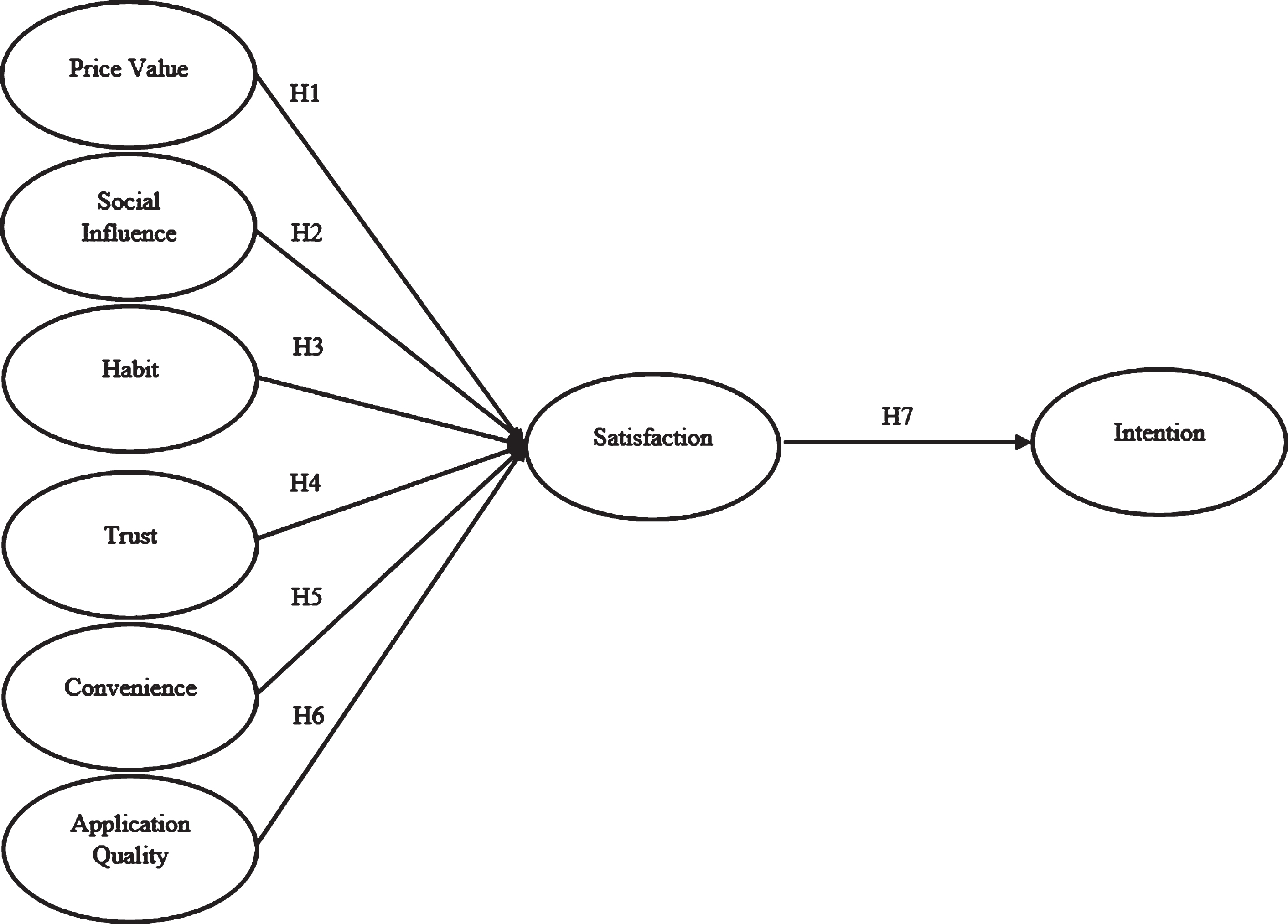
3.2Measurement model
This empirical research is based on a nonprobabilistic sampling method. The scale items used to measure the construct were selected after an intensive and careful review of relevant literature. We chose questionnaires that were developed based on an energetic body, a well-documented scale, and a match with the current research context. The items of price value were adapted from Venkatesh et al. [25], convenience was borrowed from Liu et al. [78], application quality was proposed by Mutaz et al. [45], and subsequent conceptualization of information quality by Lee et al. [26]. Trust was developed from Xiao et al. [6]; social influence, habit, and intention to use were derived by Lee et al. [26]; and satisfaction was based on the instruments proposed by Ashfaq et al. [79]. Specifically, the data set was collected in Thailand. The questionnaire was first translated into Thai by the authors, and it was tested as a pilot study among 20 respondents who had experienced using food delivery. Based on their feedback, we revised some items to improve clarity and comprehensibility. Then the authors translated them back into English to ensure consistency. Moreover, Cronbach’s alpha was used to check the reliability, and a pilot study revealed that all the constructs captured more than 0.7, which proves that all the constructs are reliable [80]. The final scales and items are presented in Appendix 1.
3.3Sampling and data collection
Recently, there was a boom in the delivery service business. This was due to various factors that allowed for rapid expansion, whether it is the rapid growth of the e-commerce market, B2C online customer behavior, or technology trends that makes applications smarter and better respond to user needs. The food industry was also affected by these reasons. The delivery service business started to provide more food deliveries to match consumer demands. The food delivery service is growing faster than expected. In addition to delivery, the platform can do much more, creating a situation that benefits all parties. The delivery service providers’ new strategy has offered aggressive service tightly related to the customer’s lifestyle to engage users continuously. At present, many factors (e.g., COVID-19) may have caused the “new normal” in customer consumption and customer behavior. Among these changes, this study focuses on how food delivery leads to satisfaction toward the intention to use food delivery applications. The target respondents are smartphone users who have used a food delivery application. Hence, this study seeks to understand customer attitude and examines the extent to which satisfaction with food delivery applications affects customer intention to use these applications. We developed exploratory and confirmatory research with a quantitative approach. The survey instrument to test the hypothesis and propose the research model was developed from prior studies. The relevant measures and scales were adapted and slightly modified from studies to prove the research questions’ consistency and validity. The modification was made to fit the specific context of food delivery applications. Each item was evaluated using a five-point Likert scale, from strongly disagree = 1 to strongly agree = 5. This research model contains eight constructs; each construct was measured with multiple items.
Data collection took place from April 1–30, 2020, via an online survey administered by Google Forms. Invitation links for the survey were sent to 304 prospective respondents who wanted to participate. Fifty-four respondents were deleted because they returned incomplete surveys. Therefore, surveys from 250 eligible respondents were collected. Tables 2 and 3 summarize the demographic and behavioral characteristics of the participants. It was found that the sample had an almost equal number of males and females. Most of the participants (32.0%) were 31–35 years old. Regarding education level, the majority (60.0%) had bachelor’s degrees; personal monthly income data showed 48.8% were earning between $500 and $1,000; and 43.2% were office workers. In terms of their usage behavior, most of the respondents (54.8%) use Grab delivery service; 43.6% use it two to three times per week, and 39.6% reported an average spending of $3.05–$3.10 per time.
Table 2
Descriptive statistic of samples
Demographic Variable | Item | Frequency | Percentage (%) |
Gender | Male | 127 | 50.8 |
Female | 123 | 49.2 | |
Age | Below 25 years | 33 | 13.2 |
25–30 years | 56 | 22.4 | |
31–35 years | 80 | 32.0 | |
36–40 years | 58 | 23.2 | |
Above 40 years | 23 | 09.2 | |
Education | High School | 23 | 09.2 |
Vocational | 35 | 14.0 | |
Bachelor’s degree | 150 | 60.0 | |
Master’s degree | 42 | 16.8 | |
Income | Below $500 | 50 | 20.0 |
$500–1,000 | 122 | 48.8 | |
$1,001–1,460 | 68 | 27.2 | |
$1,461–2,435 | 9 | 03.6 | |
Above $2,435 | 1 | 00.4 | |
Occupation | Unemployed | 7 | 02.8 |
Self-employed | 55 | 22.0 | |
Office worker | 108 | 43.2 | |
Government officer | 45 | 18.0 | |
Student | 31 | 12.4 | |
Retired | 4 | 01.6 |
Table 3
Characteristic statistic of samples
Characteristic variable | Item | Frequency | Percentage (%) |
The food delivery service providers | Grab | 137 | 54.8 |
GET | 22 | 08.8 | |
Line Man | 32 | 12.8 | |
Lalamove | 25 | 10.0 | |
FoodPanda | 14 | 05.6 | |
Others | 20 | 08.0 | |
Usage frequency for 1 week | 1 time | 71 | 28.4 |
2–3 times | 109 | 43.6 | |
4–6 times | 57 | 22.8 | |
7 times | 10 | 04.0 | |
Above 7 times | 3 | 01.2 | |
Average spending per times | Below $3.5 | 39 | 15.6 |
$3.5–10 | 99 | 39.6 | |
$10.1–16 | 77 | 30.8 | |
Above $16 | 35 | 14.0 |
4Data analysis and results
This study used confirmatory factor analysis (CFA) based on Amos version 23 software to access the factor structure and validate the scale of the model. The congeneric model was examined for model fit, reliability, convergent validity, and discriminant validity. Following Hair et al.’s (2010) recommendation, certain indices should be considered: the goodness-of-fit index (GFI), normed fit index (NFI), root mean square error of approximation (RMSEA), root mean square residuals, standardized root mean residual, comparative fit index (CFI), and Tucker–Lewis index. The primary model consisted of 8 constructs with 27 indicators: price value (PV) = 3 indicators, social influence (S) = 3 indicators, habit (H) = 3 indicators, convenience (C) = 3 indicators, application quality (AQ) = 6 indicators, satisfaction (Sa) = 3 indicators, and intention (I) = 3 indicators. The results of the initial CFA revealed a lack of fit (x2/df = 1.797; GFI = 0.854; RMSEA = 0.057; root mean square residuals = 0.019; standardized root mean residual = 0.037; NFI = 0.917; CFI = 0.961; Tucker–Lewis index = 0.954). To improve the model fit, some items were removed on the basis of modification indices, factor loading, and standardized residuals [81].
4.1Measurement model results: Confirmatory factor analysis model fitness was tested initially in the CFA, followed by evaluating the reliability and validity constructs
To achieve an acceptable model fit and confirm that this model is appropriate to predict adoption of the food delivery application, it was necessary to eliminate 3 of the original 27 indicators. The indices’ results, along with their recommended values of model fit, are shown in Table 4. We conclude that the goodness-of-fit indices met the recommended levels, suggesting that the research model provides a good fit for the data [81].
Table 4
Result of measurement model
Fit indices | Cut-off point | Initial measurement model | Modified measurement model |
x2/df | < 3 | 1.797 | 1.377 |
GFI | > 0.90 | 0.854 | 0.908 |
RMSEA | < 0.08 | 0.057 | 0.039 |
RMSR | < 0.05 | 0.019 | 0.015 |
SRMR | < 0.08 | 0.033 | 0.027 |
NFI | > 0.80 | 0.917 | 0.943 |
CFI | > 0.90 | 0.961 | 0.984 |
TLI | > 0.90 | 0.954 | 0.980 |
To validate the survey instrument, we examined the convergent and discriminant validity. Convergent validity is evaluated by measuring the standardized path loadings, composite reliability (CR), and average variance extracted to prove reliability and validity. Table 5 indicates all reflective item constructs. The item loadings reported ranged from 0.760 to 0.912, and according to Hair et al. (2010), factor loading estimates should be higher than 0.5 and ideally 0.7 or higher. In the present study, the value of CR reported ranged from 0.835 to 0.928; CR is usually satisfied by a recommended value of 0.7 (for an exploratory study, 0.6 could be accepted [81]. The value of average variance extracted reported ranged from 0.684 to 0.812; the recommended value of AVE should exceed 0.5 [82]. This finding indicates that the items meet the requirement of convergent validity, and all indicators are closely related to the given constructs. Discriminant validity is valid when the square root of each construct’s AVE is larger than its correlations with other constructs [83], as shown in Table 6. The results show that discriminant validity is satisfactory for all constructs, implying that the model meets the criteria to support discriminant validity.
Table 5
Construct reliability and validity
Construct | Standardized Loading | Composite Reliability (CR) | Average Variance Extracted (AVE) | R2 | |
Price Value (PV) | PV1 | 0.839 | 0.890 | 0.730 | |
PV2 | 0.852 | ||||
PV3 | 0.872 | ||||
Social Influence (SI) | S1 | 0.891 | 0.890 | 0.730 | |
S2 | 0.905 | ||||
S3 | 0.760 | ||||
Habit (HT) | H1 | 0.828 | 0.867 | 0.684 | |
H2 | 0.823 | ||||
H3 | 0.831 | ||||
Trust (T) | T1 | 0.818 | 0.835 | 0.629 | |
T2 | 0.763 | ||||
T3 | 0.797 | ||||
Convenience (C) | C1 | 0.831 | 0.885 | 0.720 | |
C2 | 0.871 | ||||
C3 | 0.843 | ||||
Application Quality (AQ) | AQ1 | 0.844 | 0.910 | 0.772 | |
AQ5 | 0.906 | ||||
AQ6 | 0.885 | ||||
Satisfaction (SAT) | Sa1 | 0.912 | 0.928 | 0.812 | 0.770 |
Sa2 | 0.902 | ||||
Sa3 | 0.889 | ||||
Intention (I) | I1 | 0.846 | 0.916 | 0.785 | 0.710 |
I2 | 0.902 | ||||
I3 | 0.908 |
Table 6
The discriminant validity of the measurement model
Variable | Price value | Social influence | Habit | Trust | Convenience | Application quality | Satisfaction | Intention |
Price value | 0.854 | |||||||
Social influence | 0.836 | 0.854 | ||||||
Habit | 0.754 | 0.764 | 0.827 | |||||
Trust | 0.643 | 0.707 | 0.771 | 0.793 | ||||
Convenience | 0.639 | 0.675 | 0.756 | 0.728 | 0.848 | |||
Application quality | 0.719 | 0.765 | 0.779 | 0.750 | 0.707 | 0.879 | ||
Satisfaction | 0.653 | 0.714 | 0.670 | 0.715 | 0.677 | 0.823 | 0.901 | |
Intention | 0.718 | 0.769 | 0.692 | 0.767 | 0.728 | 0.791 | 0.798 | 0.886 |
The bold number in the diagonal row represents the value of the square root of the average variance extracted (AVE) value for each latent construct, while the value below represents squared inter-construct correlations.
4.2Structural model results: Path coefficient analysis
The relationships between and among factors in terms of the combination of direct and indirect effects are referred to as the total effects. Table 7 shows the overall total effect of each prediction factor on each endogenous factor. For example, the standardized total effect of application quality on intention is 0.473, which means that when application quality goes up by 1 standard deviation, the intention will go up by 0.473 standard deviations. Conversely, the total effect on intention from habit is –0.146, which means that an increase of habit of 1 standard deviation would predict a change in intention by –0.146 standard deviation.
Table 7
Standardized total effects on endogenous factors
Variable | Price value | Social influence | Habit | Trust | Convenience | Application quality | Satisfaction | Intention |
Price value 1 | 0.839 | 0.000 | 0.000 | 0.000 | 0.000 | 0.000 | 0.000 | 0.000 |
Price value 2 | 0.851 | 0.000 | 0.000 | 0.000 | 0.000 | 0.000 | 0.000 | 0.000 |
Price value 3 | 0.873 | 0.000 | 0.000 | 0.000 | 0.000 | 0.000 | 0.000 | 0.000 |
Social influence 1 | 0.000 | 0.890 | 0.000 | 0.000 | 0.000 | 0.000 | 0.000 | 0.000 |
Social influence 2 | 0.000 | 0.908 | 0.000 | 0.000 | 0.000 | 0.000 | 0.000 | 0.000 |
Social influence 3 | 0.000 | 0.757 | 0.000 | 0.000 | 0.000 | 0.000 | 0.000 | 0.000 |
Habit 1 | 0.000 | 0.000 | 0.828 | 0.000 | 0.000 | 0.000 | 0.000 | 0.000 |
Habit 2 | 0.000 | 0.000 | 0.823 | 0.000 | 0.000 | 0.000 | 0.000 | 0.000 |
Habit 3 | 0.000 | 0.000 | 0.831 | 0.000 | 0.000 | 0.000 | 0.000 | 0.000 |
Trust 1 | 0.000 | 0.000 | 0.000 | 0.825 | 0.000 | 0.000 | 0.000 | 0.000 |
Trust 2 | 0.000 | 0.000 | 0.000 | 0.762 | 0.000 | 0.840 | 0.000 | 0.000 |
Trust 3 | 0.000 | 0.000 | 0.000 | 0.790 | 0.000 | 0.849 | 0.000 | 0.000 |
Convenience 1 | 0.000 | 0.000 | 0.000 | 0.000 | 0.834 | 0.891 | 0.000 | 0.000 |
Convenience 2 | 0.000 | 0.000 | 0.000 | 0.000 | 0.870 | 0.636 | 0.000 | 0.000 |
Convenience 3 | 0.000 | 0.000 | 0.000 | 0.000 | 0.841 | 0.540 | 0.000 | 0.000 |
Application quality 1 | 0.000 | 0.000 | 0.000 | 0.000 | 0.000 | 0.844 | 0.000 | 0.000 |
Application quality 5 | 0.000 | 0.000 | 0.000 | 0.000 | 0.000 | 0.906 | 0.000 | 0.000 |
Application quality 6 | 0.000 | 0.000 | 0.000 | 0.000 | 0.000 | 0.885 | 0.000 | 0.000 |
Satisfaction | 0.044 | 0.167 | –0.174 | 0.200 | 0.159 | 0.561 | 0.000 | 0.000 |
Satisfaction 1 | 0.039 | 0.150 | –0.156 | 0.179 | 0.143 | 0.504 | 0.898 | 0.000 |
Satisfaction 2 | 0.039 | 0.148 | –0.154 | 0.177 | 0.141 | 0.498 | 0.887 | 0.000 |
Satisfaction 3 | 0.039 | 0.149 | –0.155 | 0.178 | 0.142 | 0.501 | 0.892 | 0.000 |
Intention | 0.037 | 0.141 | –0.146 | 0.168 | 0.134 | 0.473 | 0.843 | 0.000 |
Intention 1 | 0.032 | 0.120 | –0.125 | 0.144 | 0.114 | 0.404 | 0.719 | 0.853 |
Intention 2 | 0.033 | 0.126 | –0.131 | 0.151 | 0.120 | 0.424 | 0.755 | 0.897 |
Intention 3 | 0.034 | 0.128 | –0.133 | 0.153 | 0.122 | 0.429 | 0.765 | 0.908 |
To analyze the structural model, as shown in Fig. 5, the model, path coefficients, and R2 for the endogenous construct were tested. The x2/df for the structural model was 1.613 and by chi-square was 371.20 with Degree of Freedom (DF) being 230 (p = 0.000). The GFI = 0.892, adjusted GFI = 0.859, NFI = 0.931, CFI = 0.972, and RMSEA = 0.050. These fit measures are comparable to those obtained with the CFA and show a good fit measure. The path coefficients are found at both significant and nonsignificant paths, the significant paths found at the p = 0.001, p = 0.05, and p = 0.1 levels, with price value to satisfaction path at p = 0.638, the social influence to satisfaction path at p = 0.096, the habit to satisfaction path at p = 0.092, the trust to satisfaction path at p = 0.024, the convenience to satisfaction path at p = 0.036, the application quality to satisfaction path at p = 0.000, and the satisfaction to intention path at p = 0.000. The price value to satisfaction path coefficient at β= 0.04, the social influence to satisfaction of β= 0.17, the habit to satisfaction of β= –0.17, the trust to satisfaction of β= 0.20, the convenience to satisfaction of β= 0.16, the application quality to satisfaction of β= 0.56 (relatively stronger than the other paths to satisfaction), and the satisfaction to intention of β= 0.84.
The R2 measure is used to explain the proportion of the dependent variable by influencing variables. For the overall R2 for satisfaction and intention, the dependent variables are 0.77 and 0.71, respectively, which is considered strong (see Table 5). The model testing in Fig. 5. shows that one of the seven hypotheses, price value (H1), is nonsignificant; two of the seven hypotheses, social influence (H2) and habit (H3), are supported at a significance level of p < 0.10; two of the seven hypotheses, trust (H4) and convenience (H5), are supported at a significance level of p < 0.05; and two of the seven hypotheses, application quality (H6) and satisfaction (H7), are supported at a significance level of p < 0.001. To summarize, the model provided support for six hypotheses (H2–H7) in their predicted directions.
5Discussion
5.1Theoretical implications
The primary purpose of this study was to understand the key drivers of food delivery applications. We propose that food delivery applications are driven by customer satisfaction—which, in turn, is driven by six relational factors: price value, social influence, habit, trust, convenience, and application quality. Empirical support was found for six of the seven research hypotheses (H2–H7), as shown in Fig. 6.
Fig. 6
Results of the structural modeling analysis.
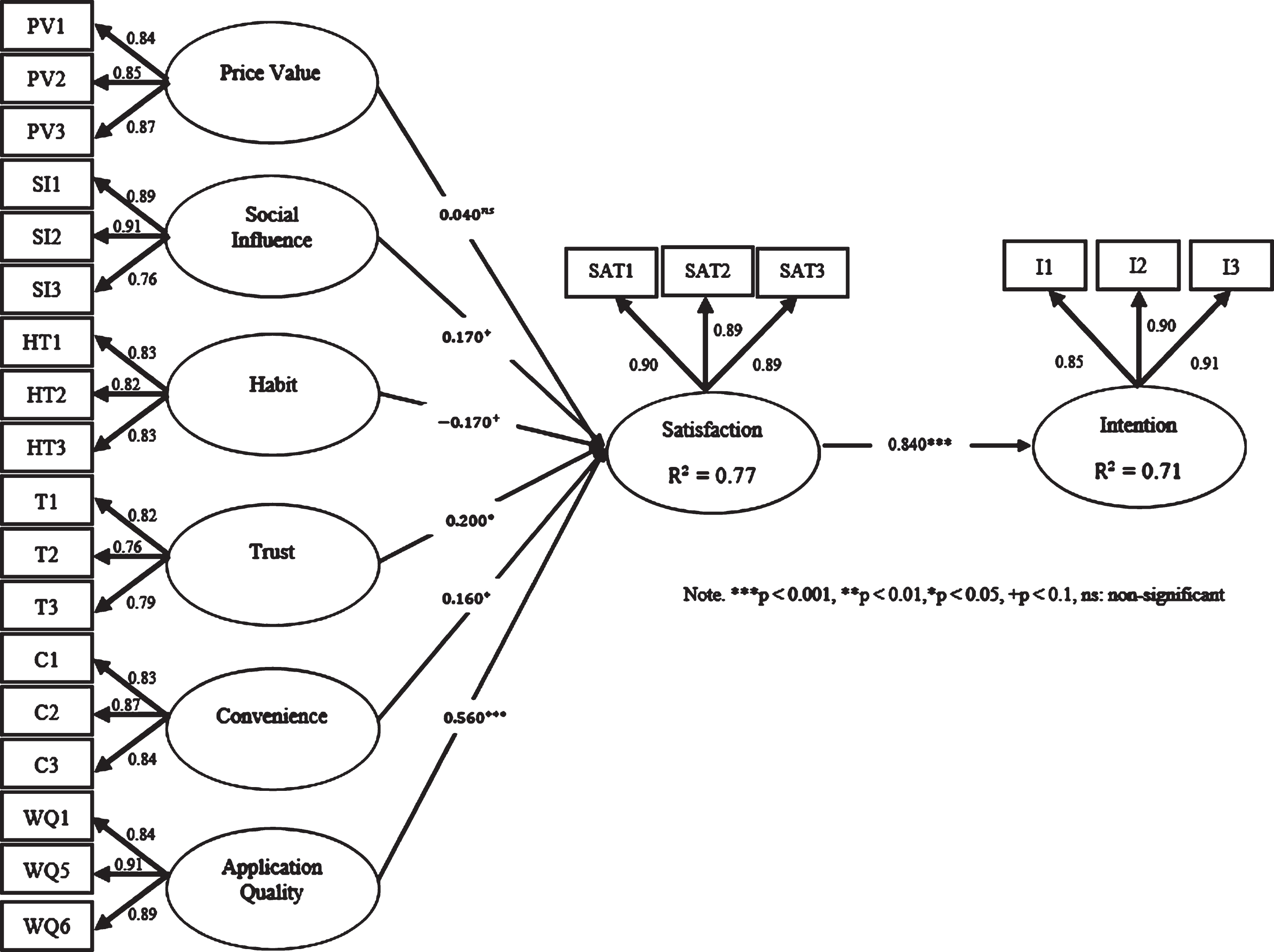
Somewhat surprisingly, of the main predictors of satisfaction toward adoption—namely, price value (H1), which is one of the main predictions of a fundamental theory (UTAUT2)—price value was not validated as a significant predictor of satisfaction toward adoption, which shows the opposite result from the previous study [25]. Notably, this empirical finding supports the notion that food delivery application users have discovered there are no additional price benefits between placing a food order with/without a delivery application [26], and financial concern seems to play an insignificant role because of decreasing cost (e.g., delivery fee, commission fee) [84]. The invalidated relationship between price value and satisfaction toward adoption exhibits that, given today’s food delivery application toward pleasant feelings, the user’s perceptions regarding commission fees, discounts, or coupons using food delivery applications do not represent a strong indicator anymore. This may imply that the competition among food delivery aggregators has intensified, so they already provide a reasonable price and always launch their promotions or special events to encourage users to use food delivery services. Therefore, prices do not help users decide whether to opt-in or not [76].
Also, the results provide empirical clarification that the paths from the two factors social influence (H2) and trust (H4) are mediating effects of satisfaction toward adoption. The strength of social influence is consistent with the original studies of UTAUT on information technology [24], late IS theory [25], and other recent conceptualizations of IS adoption [26, 74, 85]: users will be more willing to use technology if they gain a favorable opinion from friends, family, or referents. However, some studies in mobile commerce [27] and airline e-commerce [75] have found a nonsignificant influence of social influence on intention to use technology. Moreover, the findings on trust agree with prior studies in e-commerce [60, 77, 86] that trust has a long-term impact on future relationships and intention through satisfaction, and it can be concluded that a greater trust of the user results in greater intention to use any service.
The empirically proven factor habit (H3), as a significant factor negatively determining the satisfaction to adopt food delivery applications (β= –0.17; p < 0.10), implies that it is an important aspect considered by users in formulating their satisfaction to use or reject food delivery applications. In addition, the negative sign indicates that users are less likely to be satisfied and discouraged to use food delivery applications, with a higher degree of expectation of recovery from COVID-19. The spread of COVID-19 has forced consumers to cope with changing their eating habits. Being stuck at home, consumers tend to eat more to cope with stress or boredom, and right now, people find an abundance of both [87], which may be the cause of a negative relationship. Moreover, current eating habits require social distancing, which may not form actual customer intention, so eating habits will be in constant evolution throughout the upcoming year as consumers decide which habits to keep and which to return to the “old normal.” As McKinsey’s [49] survey found, consumers plan to resume some of their out-of-home activities soon, and dining at a restaurant or bar is on the list. This proves that if safety measures are in place and the development of a COVID-19 vaccine is confirmed, consumers will start the shift to their “normal” out-of-home activities, which directly affects the intention to use food delivery.
Convenience (H5) is a factor that plays a crucial role as a direct driver of satisfaction, provided the most convenient access to save time and effort in using applications lead to a stronger feeling of satisfaction. This critical role of convenience is supported by studies on customer experience [40] and usage transfer behavior [7]. This result reflects the convenience-oriented user’s decisive perception of a food delivery application matching their pleasant feeling.
Our results show that satisfaction is usually derived from application quality (H6). This result is consistent with studies that have demonstrated the important role of application quality in the context of m-commerce [47] and e-service [88]. Online customer satisfaction forms during the interaction between customers and components of the application and service [88]. Continued updates in application quality and service provision that meet customer needs and expectations will improve customer shopping experiences toward intention to use [47].
This study supports the five factors that perform the role of mediator satisfaction and exhibit a high explanatory power, which explains 77% of the variance. Finally, the results reveal that satisfaction (H7) is the critical dominant factor toward intention to use food delivery applications, with a high explanatory power of 71% of the variance. This result coincides with IS studies that have confirmed that satisfaction determines the user’s continuance intention to use [9, 46, 89] and that satisfaction has a positive effect on the intention to recommend [88]. Our result implies that customers who adopt food delivery applications are more likely to evaluate such a claim as satisfied and raise their intention to use.
5.2Practical implications
In addition to the theoretical implications, the results of this study also provide implications for practice. First is the validation of application quality as the strongest predictor of satisfaction toward the intention to use. As a result, food delivery aggregators or retailers should develop high-quality applications that provide a seamless experience between customers and the applications, which would provide future benefits [45]. Moreover, such an application should have interactive features that respond in a way that feels natural to the user, the presentation of products should be improved practically, and the application should be implemented to attract customer interest to stimulate and place an order [36, 47, 89]. Besides, extra efforts should be made to improve the quality of the system (e.g., features, the payment system, real-time tracking), information (e.g., delivery area, event, certification), and service (e.g., delivery, in-app chat) for the fulfillment of the customer aspect and their shopping experience.
Second, both social influence and trust show a mediating effect on satisfaction toward intention to use food delivery applications. Therefore, food delivery aggregators should deploy advertising via social media and focus on extroverted users—who are likely to share an experience from their previous use, which will encourage potential users to use the applications. These methods will help general users become aware of the benefits of using food delivery applications [85]. Moreover, the marketing strategy should focus on reputation building to gain positive feedback from users and should promote and encourage user intentions and trust [27, 77]. The information quality and security assurance provided by these applications will help to improve trustworthiness. Proper security and privacy protection should be implemented to enhance and maintain perceived trust from users [77]. Offering useful and complete advice will eventually result in a higher intention to adopt food delivery applications.
Third, this study shows that habit is a significant factor hindering satisfaction toward the intention to use. Therefore, food delivery providers or restaurant players should wait to observe customer behavior after COVID-19 recovery to propose the proper consumer retention strategy and maintain customer loyalty. Moreover, according to a research study from University College London, forming a habit takes an average of 66 days [90], and Thai customers have already surpassed that mark. This means that new habits and practices have formed already, and consumers will soon begin to blend their new practices with old routines [91].
Fourth, the role of convenience encourages customer satisfaction and intention. Hsu et al. [39] and Jiang et al. [36] suggested that convenience provides additional improvement and minimize service time, search efforts, conditions, and energy spent to meet customer expectations. Therefore, business operators should provide the easiest and fastest service and effort to aid customers’ perception of convenience and enhance satisfaction. Food delivery aggregators offer greater convenience than restaurants; hence, they would benefit more and earn a competitive advantage. As food delivery often involves a payment method and a service person during food shopping and delivery, offering users opportunities to choose the form of payment and the service person may help them derive more satisfaction.
Last, it is important to address satisfaction because it plays a vital role in increasing customer intention and competitive advantage. Customer satisfaction is paramount in sustaining a business in a highly competitive market [40]. Also, food delivery aggregators and retailers should maximize customer expectations and needs during customers’ use of food delivery applications. This will strengthen the relationship with customers [79, 92]. Therefore, food delivery aggregators and retailers should fulfill customers’ needs and understand the ingredients to achieve customer satisfaction.
5.3Managerial contributions
This study’s findings can be interpreted to provide specific practical/industry solutions in order for the food industry, consumers, and academia to appropriate value from their experiences. The food industry can utilize the findings in their food delivery services.
In this regard, when setting a service fee or commission fee, a business must make sure that the fees set are reasonable and appropriate with customers’ living expenses while bearing in mind the fees its competitors charge. Special treatments are also necessary to keep customers loyal to a business and attract other customers.
Marketers should also provide more value to their customers, such as helpful tips that benefit them, and then their followers will engage and share with others in return. Building an engaged excited following requires tapping into emotions and paying attention to customer needs. Followers who are engaged in a business will more likely share its content with others, thereby expanding its influence. Creating high-quality content and a good social media campaign can help to drive brand awareness, increasing customer retention and/or boosting sales and reputation.
Food delivery practitioners must be aware and make sure their customers are safe when they shop with them. Clearly showing contact information and being always available through responding quickly puts customers at ease, knowing that when they encounter problems, they will be able to get in touch with a professional customer service.
Customers who wish to engage in food delivery services want to experience convenience. Food delivery practitioners should ensure that they optimize the customer menu for delivery and that customers enjoy a fast delivery time. The food delivery applications should be easy to access to help customers find popular restaurants to order some delicious food and easy to use to make an order. Multiple options for payment, packaging, and so forth should be provided, making customers feel more comfortable purchasing from the service. Offering customers opportunities to choose ingredients simply and effectively also provides an advantage. The key to delivery is that it should be fast, smooth, and satisfactory for every customer.
Developing and improving food delivery applications could enhance the customer’s experience of ordering food by focusing more on the quality of food delivery applications. Food delivery applications should be designed in such a way that customers are afforded interactivities and responsiveness with the application. Therefore, a minimalistic design approach should be adopted to create a simple and highly usable application. With the massive number of food delivery users, the existing technical support must have the ability to support concurrent users and do so very quickly without crashing. The easy navigation design, advanced search, consistency, and information provided will all improve the application’s usability to a great extent. It would be best to offer live chat assistance within the application through which customers can live chat with the support team whenever they need to. Displaying too much content should be avoided; in contrast, more and more images should be provided to make the application more interactive. Moreover, providing the ability to sign up via social media accounts and enabling customers to share content from the application is great for extending services to other customers.
Besides, as mentioned above, in terms of improving service effectiveness, customer satisfaction, and higher business performance, food delivery providers and restaurant operators should understand and cultivate good HRM practices—as food businesses are associated with human capital and need to carefully manage, particularly during the COVID-19 pandemic. Tensay and Singh’s [93] study revealed that HRM systems, employee engagement, and organizational performance have positive and significant relationships with each other. Thus, implementing proper HR practices helps to enhance the effect of performance on both the individual and the organization. Therefore, attention should be paid to HRM practices—adapting and implementing them through investing a significant amount of time, thereby improving business performance and enhancing employee productivity. As a result, HRM will help reduce business costs, achieve business goals, and build an engaged workforce that is satisfied, motivated, and committed to providing the expected service. Moreover, providing a mutual benefit perspective between the organization and individual employees must involve being resilient to cope with work challenges imposed by the organizational-level bundles of sustainable HRM practices.
6Conclusion, limitations, and future research directions
Along with technology innovation, the COVID-19 pandemic has taken food delivery service, which had been accelerating and boosting food delivery growth due to increased consumer demand for delivery, to another level. In particular, online food delivery has grown by 150% compared to the same period last year [94]. Food delivery service as a business has surged in popularity and customer behavioral change, especially as customers try to adapt to the “new normal.” This shows that consumers are adapting to conform to the delivery service, particularly food delivery. Moreover, COVID-19 pandemic has been instrumental in changing how the food industry works and does business. Therefore, understanding the determinant factors that influence the customers’ intention to use food delivery applications and knowing the best customers should bring added value and convenience to encourage more consumers—potentially resulting in an increase in sales and revenue, which is significant for today’s market.
This research model was empirically tested among Thai consumers who already use a food delivery application. The results reveal that application quality has the most definite impact on customer satisfaction. Application quality should balance three core qualities: system quality, information quality, and service quality. As a result, food delivery aggregators should ensure the quality of application updates and efficiency. To increase satisfaction in application quality, food delivery aggregators should provide a user interface that is extremely interactive and accurate and provides up-to-date information (e.g., fees, secured transactions, delivery conditions, customer support, etc.). Furthermore, the food delivery service should ensure that users have the best quality experience to maintain customer satisfaction because it is essential to develop and maintain willingness, positive opinions, and a long-term customer relationship. Therefore, maintaining and enhancing customer satisfaction will be the key to restaurant players‘ success and challenging task. Our findings show that satisfaction is a strong factor that shapes customers’ attitudes toward accepting food delivery applications. This finding is consistent with the results of studies on mobile food-ordering apps in Jordan [9] and Taiwan [46], which indicate that new adopters of technology are more likely to evaluate the actual outcome with their expectations, and if the business can treat the customer well enough, they are likely to reuse such service. Besides, restaurant players should not overlook the “new normal.” Still, they should adapt as quickly as possible because customers are already aware of the impact of a pandemic and public health crises (e.g., COVID-19). Furthermore, customers are also concerned about their health and safety, which has altered their purchasing behavior and led to customer behavioral change to demand food in different ways. Therefore, restaurant players should add a third delivery system and adapt the menu to optimize online ordering and delivery. However, restaurant players do not need to build their platform; they can choose to use the benefit of a food delivery aggregator’s service instead and pay commissions and service fees in exchange.
Although this study provides meaningful results, there are some limitations. First, the sample used was recruited only in Thailand; therefore, the study only included Thai customers’ perspectives, which may not be generalizable to other countries’ settings. Furthermore, the respondents’ recruitment may not represent general populations because some respondents may have completed the questionnaire with little thought or reflected a self-selection bias, and this research paid no attention to customers who have rejected using food delivery applications, in which the generalization of the results and model may be questioned. Therefore, it would be interesting to replicate our model, with appropriate adjustments, in additional studies in other countries and the highlighted kind of customer, which will provide greater empirical support for this study’s findings.
Second, we mainly conducted a cross-sectional study; it may not reflect comprehensive enough insight, as the number of food delivery users is increasing over time, particularly during the COVID-19 pandemic. Also, the food delivery service and applications are still in their initial stages but are fast-changing and developing over time. Moreover, this study gathered data during a particular time; thus, longitudinal research may be necessary to gather more insight and deepen the understanding of the interrelationship among factors relevant to food delivery applications.
Third, although this research model explained 71% of the variance in intention to use food delivery applications, the variance remains unexplained, so future research should add some new constructs (e.g., the unique feature of food delivery applications). Innovation features were found to play an important role in the adoption of mobile food delivery applications [9]. Thus, future research might integrate the unique features in order to broaden the range of context and enhance explanatory power on the intention to use food delivery applications.
Further research should be conducted in different cultural aspects, mainly in countries where food delivery applications are more adopted by the population, for comparative analysis to generalize these findings. Additionally, more empirical and theoretical evidence is needed regarding the role of unique features, HRM practices, or other constructs in order to analyze which attributes increase the explanatory power of intention to use food delivery applications. Another interesting route to extend insight might involve comparing users’ behavioral intentions before/after and during the COVID-19 pandemic to identify the differences and investigate the change of importance in the constructs. Exploration of these issues can be worth pursuing.
Author contributions
CONCEPTION: Jankit Chotigo
METHODOLOGY: Jankit Chotigo
DATA COLLECTION: Jankit Chotigo
INTERPRETATION OR ANALYSIS OF DATA: Jankit Chotigo and Yasuo Kadono
PREPARATION OF THE MENUSCRIPT: Jankit Chotigo
REVISION FOR IMPORATNT INTELLECTUAL CONTENT: Jankit Chotigo and Yasuo Kadono
SUPERVISION: Yasuo Kadono
Supplementary material
[1] The appendix table is available in the electronic version of this article: https://dx.doi.org/10.3233/HSM-201140.
References
[1] | Tesgsauce Team. Thailand E-commerce Landscape combines businesses in the Thai e-commerce market in depth in 2020; 2020. [updated 2020 May 18; cited 2020 June 29]. Available from: https://techsauce.co/tech-and-biz/thailand-e-commerce-landscape-covid-19. |
[2] | Iran H , Markm M . Ridesharing hits hyper-growth; 2017. [updated 2017 Jun 01; cited 2020 Jun 15]. Available from: https://www.brookings.edu/blog/the-avenue/2017/06/01/ridesharing-hits-hyper-growth/. |
[3] | MGR ONLINE. Food delivery” in COVID lock-down doubling the amount of plastic waste in urban areas; 2020. [updated 2020 Mar 23; cited 2020 Jun 15]. Available from: https://www.marketingoops.com/exclusive/how-to/how-to-register-food-delivery/. |
[4] | Kadono Y . Management of Software Engineering Innovation in Japan. 1st ed. Tokyo: Springer; (2015) . |
[5] | Hwang S , Kim S . Does my experience affect satisfaction with and loyalty toward O2O services? Computers in Human Behavior. (2018) ;82: :70–80. |
[6] | Xiao L , Mi C , Zhang Y , Ma J . Examining Consumers’ Behavioral Intention in O2O Commerce from a Relational Perspective: an Exploratory Study. Information Systems Frontiers. Springer. (2017) ;21: (5):1045–68. |
[7] | Chen CC , Hsiao KL , Hsieh CH . Understanding usage transfer behavior of two way O2O services. Computers in Human Behavior. (2019) ;(100):184–91. |
[8] | Roh M , Park K . Adoption of O2O food delivery services in South Korea: The moderating role of moral obligation in meal preparation. International Journal of Information Management. (2019) ;47: (August):262–73. |
[9] | Alalwan AA . Mobile food ordering apps: An empirical study of the factors affecting customer e-satisfaction and continued intention to reuse. International Journal of Information Management. (2020) ;(50):28–44. |
[10] | Fitch S . Thai food delivery market taking off. Bangkok Post; 2020. [updated 2020 Nov 13; cited 2020 Dec 17]. Available from: https://www.bangkokpost.com/business/2019015/thai-food-delivery-market-taking-off. |
[11] | Repro A . Food Delivery: Trend & Market Report 2020. Repro; 2020. [updated 2020 May 07; cited 2020 Dec 17]. Available from: https://en.repro.io/blog/food-delivery-trend-market-report. |
[12] | Kresearch. Food Delivery Application pushes continuous growth in the Food Delivery business; 2019. [updated 2019 May 22; cited 2020 Jun 15]. Available from: https://www.kasikornresearch.com/th/analysis/k-econ/business/Pages/z2995.aspx?fbclid=IwAR2KHbKGS0Fi4Bc6uIEMyeTrLOjg2d9p80YDA7c1KzNKUImsB0qM24Wf3M. |
[13] | ETDA. ETDA reveals that Gen-Y people order food online the most, and over 40% order because of the Covid-19; 2020 [updated 2020 Mar 24; cited 2020 Apr 12]. Available from: https://www.etda.or.th/content/online-food-delivery-survey-during-the-prevention-of-covid-19.html. |
[14] | JacksonSE, ShulerRS. Managing Human Resources through Strategic Partnership, 11th ed., Thomson, (2011) . |
[15] | Li JM , Yang JS , Wu HH . Improving service quality and organization performance through human resource practices. A case study. Total Quality Management & Business Excellence. (2008) ;19: (9):969–85. |
[16] | Ehrhart KH , Chung-Herrera BG . HRM at your service: Developing effective HRM systems in the context of E-service. Organizational Dynamics. (2008) ;37: (1):75–85. |
[17] | Blount Y , Castleman T , Swatman PMC . E-Commerce, Human Resource Strategies, and Competitive Advantage: Two Australian Banking Case Studies. International Journal of Electronic Commerce. (2005) ;9: (3):74–89. |
[18] | Parry E , Tyson S . Desired goals and actual outcomes of e-HRM. Human Resource Management Journal. (2010) ;21: (3):335–54. |
[19] | Mariappanadar S . Do HRM systems impose restriction on employee quality of life? Evidence from a sustainable HRM perspective. Journal of Business Research. (2020) ;118: :38–48. |
[20] | Shareef MA , Dwivedi YK , Kumar V , Kumar U . Content design of advertisement for consumer exposure: Mobile marketing through short messaging service. International Journal of Information Management. (2017) ;37: (4):257–68. |
[21] | Karmen A . Understanding sustainable human resource management-organizational value linkages: The strength of the SHRM system. Human System Management. (2020) ;39: (1):51–68. |
[22] | Popescu S , Santa R , Teleba F , Ilesan H . A structured framework for identifying risks source related to human resource in a 4. 0 working environment perspective. Human Syatem Management. (2020) ;39: (4):511–27. |
[23] | Ahmed T , Khan M S , Thitivesa D , Siraphatthada Y , Phumdara T . Impact of employee engagement and knowledge sharing on organizational performance: Study of HR challenges in COVID-19 pandemic. Human Systems Management. (2020) ;39: (4):589–601. |
[24] | Venkatesh V , Morris MG , Davis GB , Davis FD . User Acceptance of Information Technology: Toward a Unified View. MIS Quarterly. (2003) ;27: (3):425–78. |
[25] | Venkatesh V , Thong JYL , Xu X . Consumer acceptance and use of information technology: Extending the unified theory of acceptance and use of technology. MIS Quarterly. (2012) ;36: (1):157–78. |
[26] | Lee SW , Sung HJ , Jeon HM . Determinants of Continuous Intention on Food Delivery Apps: Extending UTAUT2 with Information Quality. Sustainability. (2019) ;11: (11), 3141;1–15. |
[27] | Shaw N , Sergueeva K . The non-monetary benefits of mobile commerce: Extending UTAUT2 with perceived value. International Journal of Information Management. (2019) ;45: :44–55. |
[28] | Wu B , Chen X . Continuance intention to use MOOCs: Integrating the technology acceptance model (TAM) and task technology fit (TTF) model. Computers in Human Behavior. (2017) ;67: :221–32. |
[29] | Dodds WB , Monroe KB , Grewal D . Effects of Price, Brand, and Store Information on Buyers’ Product Evaluations. Journal of Marketing Research. (1991) ;28: (3):307–19. |
[30] | Khalifa M , Liu V . Online consumer retention: contingent effects of online shopping habits and online shopping experience. European Journal of Information Systems. (2007) ;16: (6):780–92. |
[31] | Quinn J , Wood W . Everyday habits and self-regulation. Advances in Consumer Research. (2005) ;32: :34–35. |
[32] | Limayem M , Hirt SG . Force of habit and information systems usage: theory and initial validation. Journal of the Association for Information Systems. (2003) ;(4):65–97. |
[33] | Triandis HC . Attitude and Attitude Change. New York: John Wiley & Sons; (1971) . |
[34] | Brown LG , McEnally MR . Convenience: Definition, structure, and application. Journal of Marketing Management. (1993) ;2: (2):47–56. |
[35] | Darian JC , Cohen J . Segmenting by consumer time shortage. Journal of Consumer Marketing. (1995) ;12: (1):32–44. |
[36] | Jiang L , Jiang N , Liu S . Consumer perceptions of e-service convenience: an exploratory study. Procedia Environmental Sciences. (2011) ;11: :406–10. |
[37] | Bava CM , Jaeger SR , Park J . Constraints upon food provisioning practices in ‘busy’ women’s lives: Trade-offs which demand convenience. Appetite. (2008) ;50: (2):486–98. |
[38] | Buckley M , Cowan C , McCarthy M . The convenience food market in Great Britain: Convenience food lifestyle (CFL) segments. Appetite. (2007) ;49: (3):600–17. |
[39] | Hsu CL , Chen MC , Chang KC , Chao CM . Applying loss aversion to investigate service quality on logistics: a moderating effect on service convenience. Int J Oper Prod Manag. (2010) ;30: (5):508–25. |
[40] | Srivastava M , Kaul D . Social interaction, convenience, and customer satisfaction: The mediating effect of customer experience. Journal of Retailing and Consumer Services. (2014) ;21: (6):1028–37. |
[41] | DeLone WH , McLean ER . Information Systems Success: The Quest for the Dependent Variable. Information Systems Research. (1992) ;3: (1):60–95. |
[42] | Pitt LF , Watson RT , Kavan CB . Service Quality: A Measure of Information Systems Effectiveness. MIS Quarterly. (1995) ;19: (2):173–87. |
[43] | DeLone W , McLean E . The DeLone and McLean model of information systems success: a ten-year update. Journal of Management Information Systems. (2003) ;19: (4):9–30. |
[44] | Molla A , Licker PS . E-Commerce Systems Success: An Attempt to Extend and respecify the DeLone and McLean model of IS Success. Journal of Electronic Commerce Research. (2001) ;2: :1–11. |
[45] | Mutaz MA , Dima J , Enas A . Measuring web portals success: a re-specification and validation of the DeLone and McLean information systems success model. Int J Business Information Systems. (2013) ;14: (1):96–133. |
[46] | Wang YS , Tseng TH , Wang WT , Shih YW , Chan PY . Developing and validating a mobile catering app success model. International Journal of Hospitality Management. (2018) ;7: :19–30. |
[47] | Chi T . Mobile Commerce Website Success: Antecedents of Consumer Satisfaction and Purchase Intention. Journal of Internet Commerce. (2018) ;17: (3):189–215. |
[48] | Pantano E , Priporas CV . The effect of mobile retailing on consumers’ purchasing experiences: A dynamic perspective. Computers in Human Behavior. (2016) ;61: :548–55. |
[49] | McKinsey & Company. Consumer Sentiment and Behavior Continue toReflect theUncertainty of theCOVID-19Crisis: McKinsey & Company; [updated 2020 Jul 08; cited 2020 Aug 30]. Available from: www.mckinsey.com/business-functions/marketing-and-sales/our-insights/a-global-view-of-how-consumer-behavior-is-changing-amid-covid-19. |
[50] | Doney PM , Cannon JP . An Examination of the Nature of Trust in Buyer-Seller Relationships. Journal of Marketing. (1997) ;61: :35–51. |
[51] | Chen J , Shen XL . Consumers’ decisions in social commerce context: An empirical investigation. Decision Support Systems. (2015) ;(79):55–64. |
[52] | Kim DJ , Ferrin DL , Rao HR . Trust and Satisfaction, Two Stepping Stones for Successful E-Commerce Relationships: A Longitudinal Exploration. Information Systems Research. (2009) ;20: (2):237–57. |
[53] | Dutot V . Adoption of Social Media Using Technology Acceptance Model. International Journal of Technology and Human Interaction. (2014) ;10: (4):18–35. |
[54] | Chen X , Huang Q , Davison RM , Hua Z . What Drives Trust Transfer? The Moderating Roles of Seller-Specific and General Institutional Mechanisms. International Journal of Electronic Commerce. (2015) ;20: (2):261–89. |
[55] | Baumann C , Burton S , Elliott G and Kehr H M . Prediction of attitude and behavioral intentions in retail banking. The International Journal of Bank Marketing. (2007) ;(25):102–10. |
[56] | Milne MJ , Adler RW . Exploring the Reliability of Social and Environmental Disclosures Content Analysis, Accounting, Auditing & Accountability Journal. (1999) ;12: (2):237–56. |
[57] | Grabosky P . (2001). The nature of trust in the online world; 2001. [updated 2001 Apr 23; cited 2020 June 12]. Available from: https://aic.gov.au/publications/archive/the-nature-of-trust-online. |
[58] | Balasubramanian S , Konana P , Menon N . Customer Satisfaction in Virtual Environments: A Study of Online Investing. Management Science. (2003) ;49: (7):871–89. |
[59] | Ganesan S . Determinants of long-term orientation in a buyer-seller relationship. Journal of Marketing. (1994) ;58: (2):1–19. |
[60] | Kim DJ , Ferrin DL , Rao HR . A trust-based consumer decision-making model in electronic commerce: the role of trust, perceived risk, and their antecedent. Decision Support Systems. (2008) ;44: (2):544–64. |
[61] | Davis FD . Perceived usefulness, perceived ease of use, and user acceptance of information technology. Management Information System Quarterly. (1989) ;13: (3):319–40. |
[62] | Fishbein M and Icek A . Belief, Attitude, Intention, and Behavior: An Introduction to Theory and Research. Reading. MA: Addison-Wesley; (1975) . |
[63] | Oliver RL , Swan JE . Consumer Perceptions of Interpersonal Equity and Satisfaction in Transactions: A Field Survey Approach. Journal of Marketing. (1989) ;53: (April):21–35. |
[64] | Reichheld FF . The One Number You Need to Grow,” Harvard Business Review. (2003) ;81: :47–54. |
[65] | Reichheld FF , Sasser WE . Zero Defections: Quality Comes to Services. Harvard Business Review. (1990) ;68: (September/October):105–11. |
[66] | Keller KL . Conceptualizing, Measuring, and Managing Brand Equity. Journal of Marketing. (1993) ;57: :1–22. |
[67] | Tsai HT , Heng-Chiang H , Yi-Long J , Wen-Kuo C . Why on-line customers remain with a particular e-retailer: An integrative model and empirical evidence. Psychology & Marketing. (2006) ;23: (5):447–64. |
[68] | Tussyadiah IP . Factors of satisfaction and intention to use peer-to-peer accommodation. International Journal of Hospitality Management. (2016) ;55: :70–80. |
[69] | Fornell C , Johnson MD , Anderson EW , Jaesung C , Bryant BE . The American Customer Satisfaction Index: Nature, Purpose, and Findings. Journal of Marketing. (1996) ;60: (4):7–18. |
[70] | Gefen D , Benbasat I , Pavlou PA . A Research Agenda for Trust in Online Environments. Journal of Management Information Systems. (2008) ;24: (4):275–86. |
[71] | Anderson RE , Srinivasan SS . E-Satisfaction and E-Loyalty: A Contingency Framework. Psychology & Marketing. (2003) ;20: (2):123–38. |
[72] | Bhattacherjee A . Social Science Research: Principles, Methods, and Practices. Textbooks Collection: Book 3; 2012. |
[73] | Ramírez-Correa P , Rondán-Cataluña FJ , Arenas-Gaitán J , Martín-Velicia F . Analyzing the acceptation of online games in mobile devices: An application of UTAUT2. Journal of Retailing and Consumer Services. (2019) ;50: :85–93. |
[74] | Verkijika SF . Factors influencing the adoption of mobile commerce applications in Cameroon. Telematics and Informatics. (2018) ;35: (6):1665–74. |
[75] | Escobar-Rodríguez T , Carvajal-Trujillo E . Online drivers of consumer purchase of website airline tickets. Journal of Air Transport Management. (2013) ;32: :58–64. |
[76] | Oliveira T , Thomas M , Baptista G , Campos F . Mobile payment: Understanding the determinants of customer adoption and intention to recommend the technology. Computers in Human Behavior. (2016) ;61: :404–14. |
[77] | Escobar-Rodríguez T , Carvajal-Trujillo E . Online purchasing tickets for low cost carriers: An application of the unified theory of acceptance and use of technology (UTAUT) model. Tourism Management. (2014) ;43: :70–88. |
[78] | Liu F , Zhao X , Chau P , Tang Q . Roles of perceived value and individual differences in the acceptance of mobile coupon applications. Internet Research. (2015) ;25: (3):471–95. |
[79] | Ashfaq M , Yun J , Waheed A , Khan MS , Farrukh M . Customers’ Expectation, Satisfaction, and Repurchase Intention of Used Products Online: Empirical Evidence from China. SAGE Open. (2019) ;9: (2):1–14. |
[80] | Nunnally JC . Psychometric theory. New York: Mcgraw-Hill; (1978) . |
[81] | Hair JF , Black WC , Babin BJ , Anderson RE . Multivariate data analysis. 7th ed. NJ: Prentice-Hall; (2010) . |
[82] | Ryu MH , Kim J , Kim S . Factors affecting application developers’ loyalty to mobile platforms. Computers in Human Behavior. (2014) ;40: :78–85. |
[83] | Bagozzi RP , Yi Y , Phillips LW . Assessing construct validity in organizational research. Administrative Science Quarterly. (1991) ;36: (3):421–58. |
[84] | Macedo IM . Predicting the acceptance and use of information and communication technology by older adults: An empirical examination of the revised UTAUT2. Computers in Human Behavior. (2017) ;75: :935–48. |
[85] | Morosan C , DeFranco A . It’s about time: Revisiting UTAUT2 to examine consumers’ intentions to use NFC mobile payments in hotels. International Journal of Hospitality Management. (2016) ;53: :17–29. |
[86] | Palvia P . The role of trust in e-commerce relational exchange: A unified model. Information & Management. (2009) ;46: (4):213–20. |
[87] | DeBroff S . How COVID-19 Has Impacted Consumer Food Habits. Food Manufacturing; 2020. [updated 2020 May 18; cited 2020 Aug 29]. Available from: www.foodmanufacturing.com/consumer-trends/blog/21133823/how-covid19-has-impacted-consumer-food-habits. |
[88] | Finn A , Wang L , Frank T . Attribute Perceptions, Customer Satisfaction, and Intention to Recommend E-Services. Journal of Interactive Marketing. (2009) ;23: (3):209–20. |
[89] | Blasco Lopez M , Recuero Virto N , San-Martín S . Local Food Shopping: Factors Affecting Users’ Behavioral E-Loyalty. Administrative Sciences. (2018) ;8: (47):1–20. |
[90] | Lally P , Van Jaarsveld CHM , Potts HWW , Wardle J . How are habits formed: Modelling habit formation in the real world. European Journal of Social Psychology. (2009) ;40: (6):998–1009. |
[91] | Bufkin M . COVID-19 Is Spawning New Consumer Food-Buying Habits. AGDAILY; 2020 [updated 2020 May 16; cited 2020 Aug 30]. Available from: www.agdaily.com/news/covid-19-new-consumer-food-buying-habits/. |
[92] | Belanche D , Casaló LV , Guinalíu M . Website usability, consumer satisfaction and the intention to use a website: The moderating effect of perceived risk. Journal of Retailing and Consumer Services. (2012) ;19: (1):124–32. |
[93] | Tensay AT , Singh M . The nexus between HRM, employee engagement and organizational performance of federal fublic service organizations in Ethiopia. Heliyon. (2020) ;6: (6):e04094. |
[94] | Econ. Food delivery business booms amid Covid-19 outbreak. The Nation; 2020. [updated 2020 Aug 10; cited 2020 Dec 24] Available from: https://nationthailand.com/business/30392754?utm_source=homepage&utm_medium=internal_referral. |