Technological interventions in Functional Capacity Evaluations: An insight into current applications
Abstract
BACKGROUND:
Functional Capacity Evaluation (FCE) is a crucial component within return-to-work decision making. However, clinician-based physical FCE interpretation may introduce variability and biases. The rise of technological applications such as machine learning and artificial intelligence, could ensure consistent and precise results.
OBJECTIVE:
This review investigates the application of information and communication technologies (ICT) in physical FCEs specific for return-to-work assessments.
METHODS:
Adhering to the PRISMA guidelines, a search was conducted across five databases, extracting study specifics, populations, and technological tools employed, through dual independent reviews.
RESULTS:
Nine studies were identified that used ICT in FCEs. These technologies included electromyography, heart rate monitors, cameras, motion detectors, and specific software. Notably, although some devices are commercially available, these technologies were at a technology readiness level of 5–6 within the field of FCE. A prevailing trend was the combined use of diverse technologies rather than a single, unified solution. Moreover, the primary emphasis was on the application of technology within study protocols, rather than a direct evaluation of the technology usability and feasibility.
CONCLUSION:
The literature underscores limited ICT integration in FCEs. The current landscape of FCEs, marked by a high dependence on clinician observations, presents challenges regarding consistency and cost-effectiveness. There is an evident need for a standardized technological approach that introduces objective metrics to streamline the FCE process and potentially enhance its outcomes.
1Introduction
Functional capacity evaluations (FCE) are important return-to-work assessment tools used by rehabilitation professionals to evaluate an individual’s functional performance using similar-condition tasks after an injury or illness [1]. Clinicians use FCEs to compare an individual’s current level of functioning against the demands of their occupation and outline any limitations in their ability to return to work [1]. One method of assessment involves progressive lifting tasks, which consists of observing progressive lifts under increasing load, to determine safe maximal lifts [2]. This involves the clinician observing whether the participant can complete the lift without biomechanical compensations that may cause additional injury and observing subjective cues such as perceived exertion. Rehabilitation professionals, such as occupational therapists and physical therapists, are well-suited to determine whether the client reaches their safe maximal lift for specific movements. Although FCEs are widely used for return-to-work assessment, the validity and reliability of the assessment results can be widely variable depending on clinician experience [3]. For example, in determining the interrater reliability of an FCE, Mitchell et al. [3] describe how an evaluator’s training, knowledge, and experience can influence their insight, confidence, and therefore scoring of an FCE. In their study, all three of the evaluators reported full agreement about the safe maximum for only 20.83% of the 72 lifts assessed [3]. Therefore, it is necessary to outline ways by which the assessment can be better standardized, objectively measured, and conducted in a timelier manner.
There are several FCEs that include different variations of biomechanical elements, such as the WorkWell System, Blankenship System, Matheson FCE, ErgoScience FCE, and the Physical Work Performance Evaluation [2]. Typically, these evaluations consist of testing strength, range of motion, biomechanics, endurance, and dexterity through progressive lifting tasks in various positions [2]. The clinician also reviews past medical history, conducts an interview, and completes a musculoskeletal screen. FCEs may also include cognitive and emotional components to assess abilities such as attention, memory, problem-solving, stress tolerance, and coping strategies [4]. For the purposes of this review, only the physical domain of FCEs will be discussed.
While FCEs are commonly used in clinical settings, questions about their validity, reliability, and criteria for decision-making persist [3, 5]. During the FCE, while the individual performs progressive tasks, the clinician is required to make qualitative and quantitative observations. For instance, they must note perceived effort through the time taken to complete tasks, number of breaks taken, and the use of counterbalance required throughout the tasks [1]. Comparisons of consistency in the control of movement, muscle recruitment, and endurance are also recorded. The potential for clinician subjectivity and experience can significantly impact the validity of the FCE results, making it challenging to derive consistent, objective conclusions about an individual’s work capacity [6]. Additionally, a systematic review by Gouttebarge et al. [7] highlighted that the reliability and validity of FCEs remain inconsistent. In contrast, test-retest reliability has been demonstrated to be good using the Isernhagen Work Systems’ protocol; however, fluctuations in participant performance were found to be a significant source of measurement variability in FCEs [8]. Moreover, an individual’s physical performance during an FCE may be impacted by environmental and physical factors [9]. The potential for variability across clinical, personal, and environmental contexts underscores the need for more rigorous and individualized protocols in FCE administration.
Another key limitation of FCEs is the amount of time that is required to conduct all the components within the battery. This creates a barrier for insurance companies and employers who aim to reduce associated costs from worker’s compensation claims [10]. The components of the FCE required for the individual are dependent on the complexity of the injury and the nature of their work. This could include multiple batteries of assessments that can take hours to complete. As the comprehensiveness of an FCE increases, so do the costs incurred by insurance companies and employers [10]. This escalation also strains clinical resources, demanding more of the assessor’s time, imposing physical and cognitive burdens on both the assessor and the individual being evaluated, and requiring greater utilization of clinical space. [10] In recent years, advancements in information and communication technologies (ICT) have presented potential for novel integration into return-to-work assessments. Exploring how these technologies could be applied in return-to-work FCEs may enhance the analysis of their potential role in supporting clinical decision-making, Additionally, this understanding can help identify key gaps in the current systems, pointing towards areas for ongoing research and development in an effort to improve these tools. Consequently, this study seeks to explore the extent to which ICT could be employed in physical return-to-work FCEs. To investigate such further, three research questions were developed:
1. What are the extent and types of ICT used in return-to-work FCE?
2. What is the level of technology readiness of these technologies?
3. What are the reported facilitators and/or barriers of the use of ICT in FCE protocols?
2Methods
To answer the proposed research questions, a scoping review was conducted in line with the methodological framework outlined by Arksey & O’Malley [11] and in accordance with the Preferred Reporting Items for Systematic Reviews and Meta-Analysis Statement (PRISMA; [12]). After formulating the research questions, a research librarian was consulted to help develop the search strategy. The search terms were related to the concepts of return-to-work FCE, ICT, physical health, musculoskeletal injuries, and disorders (refer to the supplementary materials). Medline, EMBASE, Scopus, CINAHL, and Web of Science – Core Collection were searched using Boolean operators on December 22, 2022, by a Postdoctoral Fellow (MF), and compiled into Covidence (Veritas Health Innovation, Melbourne, Australia). Duplicates were removed, and the articles were assessed for eligibility across each stage of the scoping review process by two independent reviewers who applied the inclusion and exclusion criteria, with a third independent reviewer resolving any conflicts.
2.1Information sources and search strategy
The databases were searched using three search threads related to key concepts of physical conditions, information and communication technologies, and return-to-work evaluations. Keywords for each concept were combined using the Boolean operator “OR” as well as “AND”. Search strings included: (1) (Injur* OR impair* OR disab* OR pain* OR musculoskeletal OR MSK OR back ache* OR backache*); (2) (“Sensor*” OR “artificial intelligence” OR “AI” OR “tracking” OR “software” OR “application*” OR “camera*” OR “machine learning” OR simulation* OR “information technolog*” OR “communication technolog*” OR “ICT” OR “computer monitor*” OR “deep learn*” OR robot* OR “automat*” OR smart OR app* OR VR OR “virtual realit*”); (3) (“Work eval*” OR “occupation* assess*” OR “occupation* eval*” OR “capacity eval*” OR “work* fit*” OR “functional abilit*” OR “capacity assess*” OR “capacity eval*” OR “return to work” OR “perform* eval*” OR “function* assess*” OR “function* eval*” OR “job re-entry” OR “job entry” OR “work* re-entry” OR, OR “work capacit*” OR “work* read*”). Refer to the supplementary materials for additional details.
2.2Inclusion & exclusion criteria
Studies were eligible for inclusion if they utilized any type of ICT in physical return-to-work evaluation, recruited adults aged 18 years and older, available as full-text in peer-reviewed journals or as conference manuscripts, and published in English. No restrictions were placed on article publication dates. Studies that did not provide enough information for categorizing the manuscript, utilized technology outside return-to-work evaluation, study protocols, studies using FCE for cognitive, mental health or behavioural impairments, conference abstracts, review articles, and studies beyond the scope of the review were excluded. A calibration phase was performed amongst the reviewers prior to commencing the abstract and full-text phases with strong interrater reliabilities (Krippendorff’s α= 0.869 and α= 0.829, respectively).
2.3Data extraction & analysis
After completing the title/abstract and full-text screens, eligible articles underwent data extraction. Two independent reviewers extracted data on bibliometric information, study design, study purpose, study length, population features, hardware type, software type, technology readiness level, return-to-work evaluation, and reported data on strengths and limitations. Consensus was achieved by the authors to categorize the technology of each publication by the category of technology. Each technology category was analyzed by determining the number of publications in which it was used, the participants that used it, and its purpose in return-to-work evaluation. The level of readiness of each technology category was also identified using the Technology Readiness Level (TRL) scale [13]. The scale, which ranges from 1– 9, categorizes technology based on its maturity level, defined by how advanced a technology is in its development, and how close it is to being available on the market for purchase. The higher the TRL level, the more accessible and supported the technology is for its intended use. Level 9 technologies are deployed on the market and proven to work effectively for their intended applications over a significant period.
2.4Bias control
Several strategies were implemented to minimize biases in this review. Multiple databases were searched to reduce source bias. All included publication years and countries were used to identify relevant articles. Peer-reviewed papers, or conference manuscripts, available in full-text from electronic abstract systems were included to avoid studies with low methodological rigour. To reduce the effect of publication bias, papers reporting both positive and negative results were included. Further, two independent reviewers conducted the scoping review, with a third reviewer to resolve any disagreements during the selection process when needed to minimize rater bias. Quality appraisals of individual studies were not completed because of the nature of this review. The focus of this review surrounds the use of technology in the methods of capacity evaluation and the outcome of appraisal was insignificant. Thus, the use of appraisal tools was deemed unnecessary.
3Results
The database search (Fig. 1) identified 5534 studies; 4159 studies remained after removing duplicates, 48 of which were eligible for full-text review. Thirty-nine studies were excluded during the full-text review for the different reasons: not a physical return to work assessment (n = 17), using technology outside of the return-to-work evaluation (n = 12), review articles (n = 4), lacking an information/communication technology system (n = 4), and study protocols (n = 2), resulting in a total of nine studies being included in this review. See Fig. 1 for additional details.
Fig. 1
PRISMA Flow Diagram.
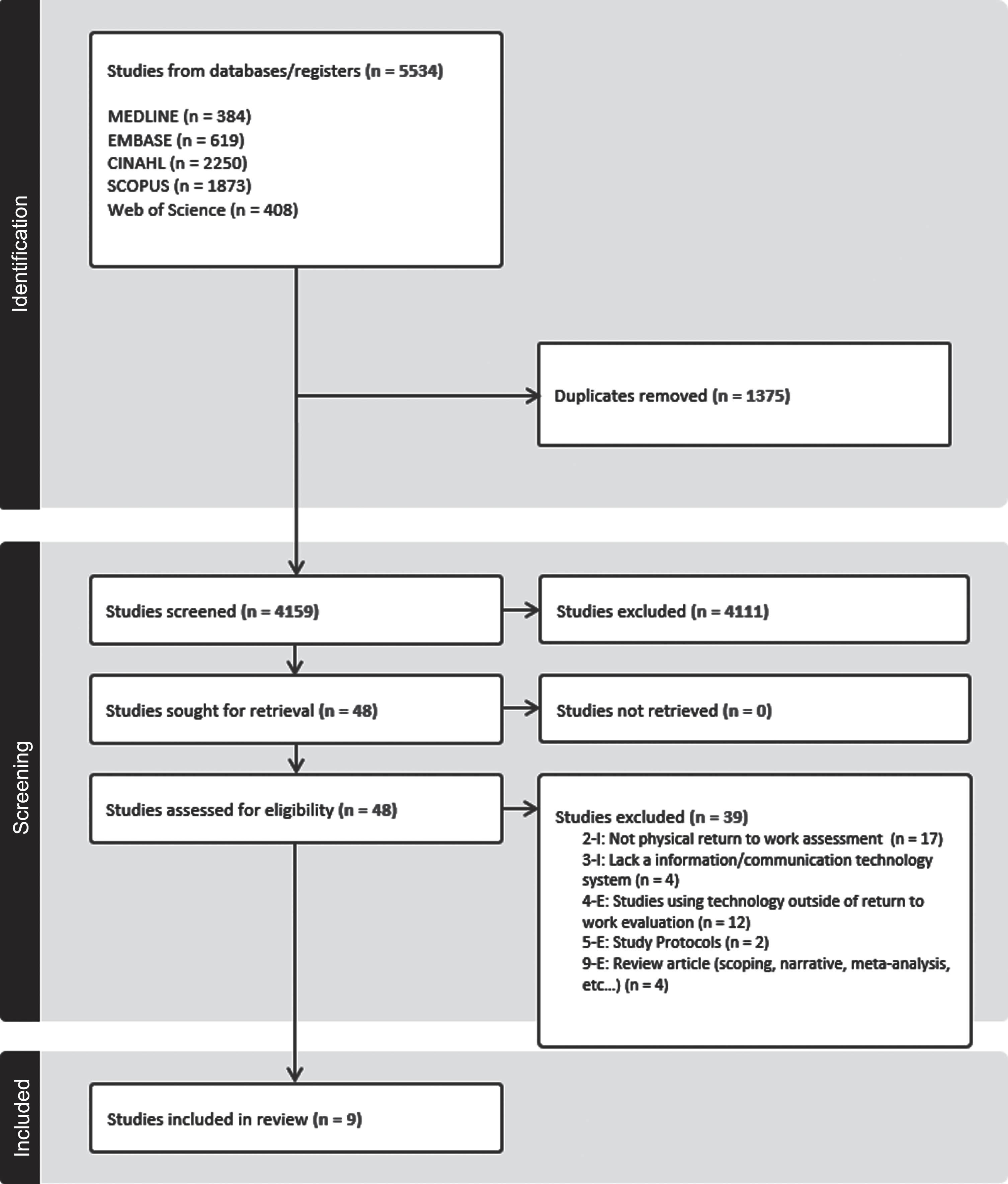
A summary of the included articles can be found in Table 1. All nine studies were published in lower impact factor academic journals (Mean SNIP = 1.26±0.55) with most published in journals with a Q2 (33.33%) and Q3 (44.44%) quartile ranking, with one journal ranked Q1 (11.1%; SCImago, 2007). The majority were published after 2012 (88.89%). There was a total of 313 participants included across the nine studies. The authors in more than half of the studies were affiliated with Australia (55.56%). Other affiliations include Canada (22.22%), Italy (11.11%), and Taiwan (11.11%).
Table 1
Bibliometric, participant characteristics, and ICT used in included studies (N = 9)
First Author (year) | Country of Primary Author | Journal | Purpose | Sample Size (n) | Health Status (n) | Mean Age (SD) | Biological Sex | Hardware | Software | TRL | Summary of Findings |
Allen et al. (2012) [2] | Australia | Work | To confirm the therapist’s clinical judgment in determining a safe maximum lifting capacity and explore the biomechanics of overhead lifting | 30 | No musculoskeletal injuries (30) | 20.9 (0.97) | Male (n = 10) Female (n = 20) | Camcorder | DartFish | 6 | Biomechanical differences were observed in the wrist, elbow, shoulder, and sagittal spine during safe maximal overhead lifting, as determined by the WorkHab FCE, when comparing light loads to maximum loads |
Cole et al. (2004) [15] | Australia | Work | To replicate the lifting aspect of a return-to-work capacity assessment and investigate the compression forces exerted on the lumbar spine | 6 | Healthy (6) | Male: 36.7 (5.8) Female: 38.7 (0.6) | Male (n = 3) Female (n = 3) | Camcorder, Markers, EMG | Peak Motus 2000, Amlab II, 4D WATBAK | 5 | Spinal compression forces during lifting tasks in return-to-work capacity assessments may exceed recommended safety limits. Standardization and regulation of FCE procedures are needed |
Hubley-Kozey et al. (2014) [16] | Canada | Work | To develop metrics for rehabilitation guidance and re-injury prediction in return-to-work decisions by analyzing trunk muscle temporal patterns | 70 | Lower back injury > 12 weeks (35) No back injury within past year (35) | Low back injury: 39.6 (12.0) Control: 35.5 (10.0) | Male (n = 34) Female (n = 36) | EMG | LabviewTM | 6 | Neuromuscular patterns stay altered in LBI individuals, even with apparent recovery in pain and function. These differences in amplitude, response to flexion, and muscle activation offer insights for return-to-work guidelines and re injury mechanisms |
Hung et al. (2014) [14] | Taiwan | Annual International Conference IEEE Eng Med Biol Soc | To employ SEMG to identify and quantify lower back pain | 52 | Chronic lower back pain (26) Healthy (26) | Low Back Pain: 33.27 (NR) Healthy Control: 32.65 (NR) | NR | EMG, Camcorder | NR | SEMG data from lifting capacity assessments can serve as valuable quantitative measures for identifying and quantifying workplace-related low back pain | |
Iosa et al. (2021) [20] | Italy | Frontiers in Neurology | To assess the return-to-work readiness of stroke patients, artificial neural networks (ANNs) were employed to analyze gait assessment data collected through a wireless inertial system | 33 | Stroke in chronic phase (16) Healthy (17) | Stroke: 54.6 (13.7) Healthy Control: 45.7 (13.4) | NR | Inertial Measurement Unit (IMU) | IMU Software, SPSS | 6 | The Feedforward Neural Network outperformed the Forward Stepwise Logistic Regression method in distinguishing patients from healthy subjects and identifying those unable to return to work. It linked the inability to return to work with the first double support phase and trunk rotation range of motion |
James et al. (2013) [1] | Australia | Journal of Occupational Rehabilitation | To investigate the relationship between alterations in SEMG activity during a bench-to-shoulder lifting task and a therapist’s assessment of a safe maximum lift | 20 | Healthy (20) | 39.5 (14.8) | Male (n = 10) Female (n = 10) | Heart rate monitor, Camcorder, SEMG | DartFish, LabChart | 6 | While SEMG-measured muscle activity increased with weight, it did not significantly change before and after the therapist-determined safe maximum lift |
Karpman et al. (2020) [17] | Canada | Work | To assess the effectiveness of two Actigraph devices (waist and wrist) in measuring performance across five FCE tasks: maximum lifting, sustained overhead work, and a 6-minute walk test | 46 | Healthy (46) | 23.7 (3.7) | Male (n = 24) Female (n = 22) | ActiGraph, Heart rate monitor | Actilife 6 | 6 | The Actigraph device showed a moderate, positive correlation with maximum weight lifted at the waist but had poor agreement between waist and wrist placements, favoring waist placement for FCE tests |
Melino et al. (2014) [18] | Australia | Work | To assess kinematic differences between WorkHab FCE’s minimum and safe maximum floor-to-bench lift | 28 | Healthy (28) | 20.8 (1.02) | Male (n = 10) Female (n = 18) | Camcorder | DartFish | 6 | The observed changes in movement during the WorkHab FCE floor-to-bench lift validate the safe maximum lift method and assessors’ clinical judgment, aiding in identifying an individual’s peak capacity during lifting |
West et al. (2018) [19] | Australia | Work | To assess alterations in joint angles during the WorkHab FCE bench-to-shoulder lift, comparing the minimum and safe maximum load conditions | 28 | Healthy (28) | 20.9 (NR) | Male (n = 9) Female (n = 19) | Camcorder | DartFish | 6 | Notable variations in joint angles occurred between the minimum and safe maximum loads in the thoracic spine, elbow, and shoulder during the WorkHab FCE bench-to-shoulder lifts; findings validate the clinical judgments of assessors in setting safe maximum lift limits |
Total | 313 | Clinical Population: 77 Healthy: 236 | 31.61 (7.93) | Male: 100 Female: 128 NR: 85 |
Note: NR – Not Reported; EMG: Electromyography; SEMG: Surface Electromyography; TRL: Technology Readiness Level.
3.1Extent and types of ICT Used in Return-to-Work Evaluation
The ICT used for FCEs as reported in the literature were inclusive of various hardware and software. Technologies were divided into the following categories based on emerging themes from content analyses: electromyography (EMG), heart rate monitors, video camera/camcorders, motion detectors, and software-based approaches. Surface EMG were used to measure patterns of muscle activation and recruitment, camcorders were used to record data to measure joint angles, landmarking, and confirming proper biomechanics, and motion sensors were used to measure joint angles and rotation, gait analysis, and acceleration.
EMG were used in 44.4% of studies [in 1, 14–16], heart rate monitors in 55.6% [in 1, 2, 17–19], camcorders in 66.7% [in 1, 2, 14, 15, 18, 19], and motion detectors in 44.4% [in 15–17, 20]. Several pieces of software were used by each study but typically corresponded to the hardware involved. Table 2 provides details of all software used. Of the four studies using EMG, all used surface electrodes. Motion detectors used across studies included the Ascension Electromagnetic Flock of BirdsTM (FOB, 33% of studies), Motion Capture systems (33.3%), and reflective markers on different body parts (33.3%). Other ICT included wearable inertial measurement units (33.3%), and an Actigraph monitor (33.3%). See Table 2 for a summary of studies organized by technology category.
Table 2
Summary of included studies organized by technology category
ICT category | Hardware models | Software Used with Hardware | Number and Types of Studies using ICT | Sample Size (n) | Weighted Mean Age (Weighted SD) | Purpose/Use of ICT in RTW | References |
EMG | Biocapture 150 &electrodes) (n = 1)Amlab II EMG system with 2 Ag/AgCl electrodes &lifier (n = 1)Surface electrodes (ADInstruments MLA 1010), ADI Powerlab 8SP (ML 785) &dual bioamplifiers (ML135) (n = 1)EMG (Ag/AgCl, 10 mm circular electrodes &AMT-8 EMG systems amplifier) (n = 1) | Unknown EMG softwareAmlab II EMG System &Amlab II Acquisition SoftwareLabChart software (v7.1.2)Labview Software (version 7) | Non-randomized experimental studies (k = 4) | 148 | 36.21 (9.43) | To measure the activity of participant’s muscle activation during evaluation | [1, 14–16] |
HR Monitor | Unspecified polar HR monitor (n = 1)Unspecified HR monitor (n = 3)WorkHab included HR monitor (n = 1) | Non-randomized experimental studies (n = 1)Observational (n = 3)Cross-Sectional (n = 1) | 152 | 24.18 (5.73) | To measure heart rate as a metric for safe assessment | [1, 2, 17–19] | |
Video Camera | Panasonic SVHS NV-MS5 Video Camera (n = 1)Sony HRD-HC9E Camcorder (n = 2)Sony Handycam Camcorders Unknown Model (n = 2)Unspecified camcorder (n = 1) | Dartfish ProSuite (unknown version)Dartfish ProSuite (unknown version) | Non-randomised experimental study (n = 3)Observational study (n = 3) | 164 | 27.59 (5.24) | To measure changes in joint angles and lifts during an FCE | [1, 2, 14, 15, 18, 19] |
Motion Detectors | Electromagnetic Flock of BirdsTM (FOB) MotionCapture system (n = 1)Actigraph wG3TX-BT (n = 1)10 reflective markers on different body joints – illuminated by HE-888 Universal 500 W spotlights (n = 1)Wearable inertial measurement unit (n = 1) | Actigraph 6 softwarePeak Motus 2000 Artificial Neural Network (ANN) | Non-randomised experimental study (n = 2) | 155 | 36.10 (9.96) | To obtain kinematic data including joint angles and angular motion | [15–17, 20] |
*Note: Studies typically used multiple technologies, and may have been classified under multiple components.
Surface EMG (SEMG) throughout the technology and FCE literature were used to measure participants’ muscle recruitment and activity in relation to the identification of biomechanical determination of a safe maximal lift. Results from Hung et al. [14] found that changes in SEMG data obtained during a lift are a useful quantitative measure to aid in the identification and quantification of low back pain (LBP) in the workplace, but this was found to be most observable during lifts conducted at 30% of the individual’s maximum. Similarly, Hubley-Kozey et al. [16] noted observable alterations in SEMG activity during a functional lift when comparing subjects with LBP to healthy individuals. However, in a separate study, no observable differences were found in SEMG activity when participants were lifting weights lighter than weights that were heavier than their therapist-determined safe maximal lift [1]. In addition, heart rate monitors were used in most studies to monitor participants’ heart rates during an FCE. Heart rate monitors are commonly used in FCEs as a protocol for determining threshold heart rate levels [17].
Camcorders were reportedly used for various purposes, which included measuring changes in joint angles, detecting anatomical landmarks, and confirming proper lifting mechanics during FCE tasks. West et al. [19] and Melino et al. [18] used camcorders on the right sagittal plane to measure changes in joint angles between minimum and safe maximum lifts. They reported that this made it difficult to identify if any trunk rotation occurred during the lift and suggested that adding another camera on the left sagittal plane may have resulted in a more accurate measurement and analysis of the joints. Similarly, Allen [2] found that due to the placement of the camcorder, they were unable to measure joint angles when participants moved in the oblique plane.
Motion detectors were used for measuring joint angles and angular motion, analyzing gait, and measuring acceleration. Cole et al. [15] used reflective markers used in conjunction with a camcorder for the purpose of obtaining a set of 2-dimensional (2D) kinematic data. Similarly, Hubley-Kozey et al. [16] used an electromagnetic motion capture system to record the 3D angular motion of the pelvis when assessing activation patterns in those with LBP. Various software were used extensively with motion capture systems to process and analyze the temporal and motion data.
3.2Technology Readiness Levels of ICTs in Return-to-Work Evaluation
All studies in the review employed technologies classified as TRL levels 5 or 6, suggesting that these new or integrated technologies underwent testing in simulated or real-world FCE environments. Contrary to using a singular technology that amalgamates multiple inputs, the studies used a blend of distinct technologies to gather data from various origins. Although some of the devices reported are commercially available, with varying prices and features, the frequent employment of multiple devices in an evaluation system within the laboratory testing environment decreased the TRL score across studies.
3.3Reported Facilitators and/or Barriers of Technologies
In the studies reviewed, there was no mention or report of barriers or facilitators regarding the technologies employed for analyzing physical FCE tasks. This could be attributed to the fact that each study had distinct objectives focused on utilizing the technology within their methods, rather than specifically examining the feasibility, usability, and implementation of the technology.
4Discussion
This scoping review aimed to explore the use of ICT applications in return-to-work FCE evaluations. The types of technology, applications, technology readiness levels, strengths, and limitations were extracted from each study. While there is an emerging interest in the use of ICT in physical return-to-work FCEs, research remains sparse. Many technologies are focused on measuring kinesiophysical variations during various FCE components. Although these technologies are market-ready, their combined use in evaluations is not widespread. ICT could potentially assess various aspects of FCEs, and previous studies suggest opportunities for these technologies to be used in a complementary and integrated manner. The predominant reliance on clinician observations in many FCEs can raise concerns about reliability. Introducing standardized technology with quantitative metrics can lead to more consistent evaluations, yet interpretation and extrapolation to specific demands within the work environment depend on the assessor’s clinical judgment and experience. Although, the traditional FCE approach remains time consuming and costly, technological integration, while promising in streamlining evaluations, also presents challenges, especially in terms of result interpretation.
The results of this scoping review suggest that the accuracy of data from the various ICT employed to record individuals’ performance outcomes during an FCE is contingent upon the manner of their use, placement, and administration. Calibration, a process tailored to individual factors such as body composition, device positioning, perspiration, skin temperature, skin colour, and resistance, is essential for certain technologies like landmarking for video marker placement, EMG, and heart rate monitors; however, it can introduce inconsistencies and be time-consuming [21–24]. All the technologies reportedly used in FCEs necessitate training and expertise, with many requiring the use of additional software, with various data metric outputs. Interpreting these results into actionable clinical recommendations can be challenging without a thorough understanding of the data. Therefore, while these technologies can enhance FCEs, it is essential to consider the potential limitations and potential barriers to their use by assessors.
Motion detectors were used in video analysis and incorporated into software programs in several studies to analyze and capture movement data during biomechanical tasks performed as part of an FCE. These are beneficial as they provide an objective measure of an individual’s movement patterns and can be complimented with other technologies such as an accelerometer. Drawbacks to these devices include cost, technical expertise, and familiarity with system set-up requirements including data interpretation [25]. Motion detectors and associated motion analysis software can be costly to obtain and require significant computing resources to use, making overall cost a barrier to researchers and clinical assessors. Many of these systems are highly complex and software translation often requires specialized training, time, and expertise to optimize data quality and reduce error [25]. Consequently, there’s a pressing need for a platform with a user-friendly interface that enables accurate and precise recordings, thereby boosting the practical applicability of these systems in clinical settings.
Various brands of video cameras/camcorders were used in the studies for video capture. Despite the potential for high-quality video capture, the accuracy of motion analysis can be challenging to translate into biomechanical findings. Camcorders can record joint angles and body positioning during common FCE tasks, which could offer valuable insights to clinicians. However, these devices might not capture less apparent factors, such as muscle fatigue or weakness alone[25]. Moreover, video capture is prone to subjective error as manually processing video footage can be time-consuming and laborious, unless there is robust software to record and interpret associated algorithms [26]. Despite these limitations, using a camcorder in conjunction with measurement software (e.g., DartFish; Fribourg, Switzerland) to record and measure changes in joint angles could potentially yield a more accurate and objective measure than a clinician’s visual observation.
Surface EMG (SEMG) measurement is useful in FCEs as it provides a non-invasive objective measure of muscle activation and function with real-time feedback [27]. Although useful, SEMG devices are susceptible to sensitivity interference. External influences such as skin temperature, perspiration, and resistance can have a significant impact on the accuracy of readings, potentially leading to skewed or erroneous results [24, 28]. In addition to these physiological factors, environmental disturbances, such as electromagnetic fields from nearby electronic devices, can introduce unwanted noise; these potentially interfere with muscle contractions, further compromising the precision of measurements [29]. An important drawback of SEMG is that it is primarily designed to measure the activity of superficial muscles, thereby less suitable of detecting and quantifying the activity of deeper muscle recruitment and introduces signal crosstalk from superficial layers [30]. This limitation means that a comprehensive analysis of muscle function, especially in activities that heavily involve deep muscles such as the quadratus lumborum, might not be fully achievable with SEMG alone; therefore, it is crucial to consider supplemental or alternative methods for a more thorough evaluation.
Heart rate monitors offer objective exertional measures during FCEs, providing real-time data to inform decisions around tasks such as lifting. These devices can enhance individualized testing by helping clinicians adjust the level of exertion according to an individual’s capabilities. Despite these advantages, heart rate monitors have limitations. They do not capture variables such as blood pressure, perceived exertion, or oxygen consumption [31]. Wrist-worn monitors can be less accurate and introduce errors compared to other avenues of heart rate monitoring [21, 32]. Additionally, factors like poor sensor placement, device movement during exercise, and electrical interference can create artifact [33]. These challenges suggest the need for careful consideration when using this technology in FCEs.
4.1Future implications
Ongoing multidisciplinary research is needed to investigate how to effectively incorporate technologies into FCEs as there are currently no established guidelines and no standardized norms. Additionally, incorporating technology into FCEs would require initial hands-on training for clinicians regarding device use and device placement, along with specialized training related to corresponding software use and data interpretation. Although technology is being used to measure certain components of an FCE in the studies reported such as lifting or bending tasks, there does not seem to be a technology available on the market that can support a clinician in carrying out a comprehensive FCE evaluation from initiation to completion.
With further research and development, the technologies discussed in this review have the potential to be combined in a way that can improve the standardization and efficiency of FCEs. Currently, technologies being used in FCEs have varying measurement techniques and assessment components which makes it difficult to establish standardized and comparable results across studies. This could be improved with a single system that monitors real-time information from a variety of sensors, cameras, and other technologies, along with software to integrate the data. A single system that can encompass these variables can help to automate the process of FCEs and support more comparable results across assessments.
Although FCEs can be extensive due to detailed assessments, clinicians often face the challenge of tight time constraints in a demanding clinical environment. The clinician must incorporate and observe various levels of progressive lifts to determine a safe maximum. By using a combined technology, sensors and software can potentially determine a safe maximum before an expert clinician is able to. Moreover, the use of technologies can provide a more standardized and precise quantification of measurements. For example, if multiple clinicians were to implement an FCE for a given client, the outcomes reached by each assessment could vary due to variations in clinician observation and measurement. While a system-driven approach allows for precise measurement of joint angles using sensors, cameras, and software, reducing human error, it is important to note that such systems can also introduce potential errors in data collection due to various factors, as previously highlighted.
Although we included artificial intelligence (AI) in our search strategy, there was no research to indicate this is being used with FCE at present. AI can produce meaningful results of the assessment in several ways. For example, AI could increase the usability of the technology by reducing the hands-on training required for each individual technology by promoting the automatization of screening and result interpretation. It can also provide the clinician with instructions and cues for the assessment, making the process more streamlined. Moreover, it can provide personalized instructions and feedback to participants.
Currently ICT is not being used to inform clinical decision-making in FCEs; however, machine learning and decision-making based approaches have the potential to provide the clinician and participant with amalgamated data in a meaningful way. For example, estimates on maximum carrying loads based on data of joint angles obtained from cameras and muscle activity from SEMG could be combined in a way that would be beneficial for clinicians. Although this review reflects a variety of technologies that are being used for this purpose, additional research is needed to advance technological development in thisfield.
4.2Limitations
This review presents several limitations. The primary objective of the review was to outline the range of technologies available for return-to-work, without an evaluation of their efficacy. Potential bias exists due to the exclusion of papers not written in the English language. Although a comprehensive search of peer-reviewed literature was conducted, the review was based on a relatively small sample of studies (N = 9). Of these, two studies may have drawn on similar or overlapping participant samples and technology methodologies [18, 19]; this might have limited the diversity of insights. Additionally, there is potential that certain publications, especially within the grey literature, were overlooked. This review focused solely on technologies in physical FCEs for the return-to-work context, not considering their application in other contexts, such as sports performance. Yet, reflecting on the use of external systems in elite sports, which track biomechanical and physiological data without impairing performance, could provide valuable insights into their potential effects on functional task performance during FCEs. Furthermore, while the potential of AI in FCEs was underscored, the authors of this scoping review acknowledge that other untested ICT might be available for return-to-work evaluations.
5Conclusion
At present, the application of ICT in return-to-work physical FCE assessments is still at an early stage of applied research, and these technologies have not been widely adopted in clinical practice. While multiple studies reviewed here explored technologies targeting specific FCE tasks, the literature lacks mention of an all-encompassing technology or system to address all components and tasks within an FCE. Present-day FCE practices are often characterized by time-consuming processes with associated costs, and the potential reliance on subjective judgments by clinicians. This highlights the pressing need for a standardized technological solution offering objective measurable data on FCE performance capacity, with ongoing research, development, and innovation required. Envisioning a unified technological approach could promote more accurate, precise, and time-efficient evaluations, while enhancing FCE evaluation and patient outcomes. This innovation could stand to benefit every stakeholder involved in treatment planning through to return-to-work decision making.
Ethical approval
Not applicable.
Informed consent
Not applicable.
Conflict of interest
The authors declare that they have no conflict of interest.
Acknowledgments
The authors would like to thank the research librarian team at the University of Alberta for their assistance and guidance during the database search.
Funding
The authors report no funding.
Supplementary material
[1] The supplementary material is available in the electronic version of this article: https://dx.doi.org/10.3233/WOR-230560.
References
[1] | James C , MacKenzie L , Capra M Quantification of the safe maximal lift in functional capacity evaluations: comparison of muscle recruitment using SEMG and therapist observation, J Occup Rehabil. (2013) ;23: :419–27. https://doi.org/10.1007/S10926-012-9407-8. |
[2] | Allen JL , James C , Snodgrass SJ The effect of load on biomechanics during an overhead lift in the WorkHab Functional Capacity Evaluation. Work. (2012) ;43: :487–96. https://doi.org/10.3233/WOR-2012-1386. |
[3] | Mitchell D , Hancock E , Alexander L An investigation of the inter-rater reliability of the Valpar Joule functional capacity evaluation in healthy adults. Work. (2015) ;53: :337–45. https://doi.org/10.3233/WOR-152154. |
[4] | Zilbershlag Y , Josman N The functional cognitive evaluation and model to assess older adults’ ability to function in their homes in the community. Phys Occup Ther Geriatr. (2019) ;37: :151–70. https://doi.org/10.1080/02703181.2019.1622620. |
[5] | Chen JJ Functional Capacity Evaluation & Disability. Iowa Orthop J. (2007) ;27: :121. |
[6] | Innes E , Straker L Validity of work-related assessments. Work. (1999) ;13: (2):125–142. |
[7] | Gouttebarge V , Wind H , Kuijer PPFM , Frings-Dresen MHW Reliability and validity of Functional Capacity Evaluation methods: A systematic review with reference to Blankenship system, Ergos work simulator, Ergo-Kit and Isernhagen work system. Int Arch Occup Environ Health. (2004) ;77: :527–37. https://doi.org/10.1007/S00420-004-0549-7. |
[8] | Gross DP , Battié MC Reliability of safe maximum lifting determinations of a functional capacity evaluation, Phys Ther. ((2002) ;82: :364–71. https://doi.org/10.1093/PTJ/82.4.364. |
[9] | Gross DP Measurement properties of performance-based assessment of functional capacity. J Occup Rehabil. (2004) ;14: :165–74. https://doi.org/10.1023/B:JOOR.0000022759.30446.4F. |
[10] | Branton EN , Arnold KM , Appelt SR , Hodges MM , Battié MC , Gross DP A short-form functional capacity evaluation predicts time to recovery but not sustained return-to-work. J Occup Rehabil. (2010) ;20: :387–93. https://doi.org/10.1007/S10926-010-9233-9/METRICS. |
[11] | Arksey H , O’Malley L Scoping studies: Towards a methodological framework. Int J Soc Res Methodol. (2005) ;8: :19–32. |
[12] | Moher D , Liberati A , Tetzlaff J , Altman DG , Altman D , Antes G , et al. Preferred reporting items for systematic reviews and meta-analyses: The PRISMA statement. PLoS Med. (1000) ;6: :e1000097. https://doi.org/10.1371/journal.pmed.1000097. |
[13] | Sadin SR , Povinelli FP , Rosen R The NASA technology push towards future space mission systems. Acta Astronaut. (1989) ;20: :73–7. https://doi.org/10.1016/0094-5765(89)90054-4. |
[14] | Hung CC , Shen TW , Liang CC , Wu WT Using surface electromyography (SEMG) to classify low back pain based on lifting capacity evaluation with principal component analysis neural network method. Annu Int Conf IEEE Eng Med Biol Soc. (2014) ;2014: :18–21. https://doi.org/10.1109/EMBC.2014.6943518. |
[15] | Cole MH , Grimshaw PN , Burden AM Loads on the lumbar spine during a work capacity assessment test. Work. (2004) ;23: :169–78. |
[16] | Hubley-Kozey C , Moreside JM , Quirk DA Trunk neuromuscular pattern alterations during a controlled functional task in a low back injured group deemed ready to resume regular activities. Work. (2014) ;47: :87–100. https://doi.org/10.3233/WOR-131689. |
[17] | Karpman J , Gross DP , Manns P , Tomkins-Lane C Do wearable fitness devices correlate with performance-based tests of work-related functional capacity? Work. (2020) ;66: :201–11. https://doi.org/10.3233/WOR-203164.. |
[18] | Melino NL , James C , Snodgrass SJ The effect of load in a floor-to-bench lift during the WorkHab functional capacity evaluation, Work. (2014) ;49: :585–96. https://doi.org/10.3233/WOR-131698.. |
[19] | West N , Snodgrass SJ , James C The effect of load on biomechanics of the back and upper limb in a bench to shoulder lift during the WorkHab Functional Capacity Evaluation. Work. (2018) ;59: :201–10. https://doi.org/10.3233/WOR-172677. |
[20] | Iosa M , Capodaglio E , Pelà S , Persechino B , Morone G , Antonucci G , et al. Artificial neural network analyzing wearable device gait data for identifying patients with stroke unable to return to work. Front Neurol. (2021) ;12: :650542. https://doi.org/10.3389/FNEUR.2021.650542. |
[21] | Kondama Reddy R , Pooni R , Zaharieva DP , Senf B , El Youssef J , Dassau E , et al. Accuracy of wrist-worn activity monitors during common daily physical activities and types of structured exercise: Evaluation study. JMIR mHealth uHealth. (2018) ;6: (12):e10338. https://doi.org/10.2196/10338. |
[22] | Parks MT , Wang Z , Siu KC Current low-cost video-based motion analysis options for clinical rehabilitation: A systematic seview. Phys Ther. (2019) ;99: :1405–25. https://doi.org/10.1093/PTJ/PZZ097. |
[23] | Khan MH , Zöller M , Farid MS , Grzegorzek M Marker-based movement analysis of human body parts in therapeutic procedure. Sensors (Basel). (2020) ;20: :1–19. https://doi.org/10.3390/S20113312. |
[24] | Merletti R , Campanini I , Rymer WZ , Disselhorst-Klug C Editorial: Surface electromyography: Barriers limiting widespread use of sEMG in clinical assessment and neurorehabilitation. Front Neurol. (2021) ;12: :642257. https://doi.org/10.3389/FNEUR.2021.642257/PDF. |
[25] | Menolotto M , Komaris DS , Tedesco S , O’flynn B , Walsh M Motion capture technology in industrial applications: A systematic review, Sensors (Basel). (2020) ;20: :1–25. https://doi.org/10.3390/S20195687. |
[26] | Colyer SL , Evans M , Cosker DP , Salo AIT A review of the evolution of vision-based motion analysis and the integration of advanced computer vision methods towards developing a markerless system. Sports Med Open. (2018) ;4: :1–15. https://doi.org/10.1186/S40798-018-0139-Y/FIGURES/7. |
[27] | Merletti R , Botter A , Troiano A , Merlo E , Minetto MA Technology and instrumentation for detection and conditioning of the surface electromyographic signal: State of the art. Clin Biomech (Bristol, Avon). (2009) ;24: :122–34. https://doi.org/10.1016/J.CLINBIOMECH.2008.08.006. |
[28] | McManus L , De Vito G , Lowery MM Analysis and biophysics of surface EMG for physiotherapists and kinesiologists: Toward a common language with rehabilitation engineers. Front Neurol. (2020) ;11: :576729. https://doi.org/10.3389/FNEUR.2020.576729/BIBTEX. |
[29] | Chowdhury RH , Reaz MBI , Bin Mohd Ali MA , Bakar AAA , Chellappan K , Chang TG Surface electromyography signal processing and classification techniques. Sensors (Basel). (2013) ;13: :12431. https://doi.org/10.3390/S130912431. |
[30] | Criswell E Cram’s introduction to surface electromyography. 2nd ed. Sudbury, Massachusetts: Jones & Bartlett Publishers; 20110. |
[31] | American College of Sports Medicine ACSM’s guidelines for exercise testing and prescription. 11th ed. Philadelphia, Pennsylvania: Wolters Kluwer; 2022. |
[32] | Düking P , Giessing L , Frenkel MO , Koehler K , Holmberg HC , Sperlich B Wrist-worn wearables for monitoring heart rate and energy expenditure while sitting or performing light-to-vigorous physical activity: Validation study, JMIR mHealth uHealthe (2020) ;8: (5):e16716. https://doi.org/10.2196/16716. |
[33] | Imtiaz SA , Mardell J , Saremi-Yarahmadi S , Rodriguez-Villegas E ECG artefact identification and removal in mHealth systems for continuous patient monitoring, Healthc Technol Lett. (2016) ;3: :171. https://doi.org/10.1049/HTL.2016.0020. |