Neural correlates of memory recovery: Preliminary findings in children and adolescents with acquired brain injury
Abstract
Background:
After acquired brain injury (ABI), patients show various neurological impairments and outcome is difficult to predict. Identifying biomarkers of recovery could provide prognostic information about a patient’s neural potential for recovery and improve our understanding of neural reorganization. In healthy subjects, sleep slow wave activity (SWA, EEG spectral power 1–4.5 Hz) has been linked to neuroplastic processes such as learning and brain maturation. Therefore, we suggest that SWA might be a suitable measure to investigate neural reorganization underlying memory recovery.
Objectives:
In the present study, we used SWA to investigate neural correlates of recovery of function in ten paediatric patients with ABI (age range 7–15 years).
Methods:
We recorded high-density EEG (128 electrodes) during sleep at the beginning and end of rehabilitation. We used sleep EEG data of 52 typically developing children to calculate age-normalized values for individual patients. In patients, we also assessed every-day life memory impairment at the beginning and end of rehabilitation.
Results:
In the course of rehabilitation, memory recovery was paralleled by longitudinal changes in SWA over posterior parietal brain areas. SWA over left prefrontal and occipital brain areas at the beginning of rehabilitation predicted memory recovery.
Conclusions:
We show that longitudinal sleep-EEG measurements are feasible in the clinical setting. While posterior parietal and prefrontal brain areas are known to belong to the memory “core network”, occipital brain areas have never been related to memory. While we have to remain cautious in interpreting preliminary findings, we suggest that SWA is a promising measure to investigate neural reorganization.
1Introduction
After acquired brain injury (ABI), patients show various neurological impairments (Amlie-Lefond, Sébire, & Fullerton, 2008; Beauchamp & Anderson, 2013; Kuhtz-Buschbeck et al., 2003). The prediction of outcome is difficult (i.e., some patients recover faster or better than others). Identifying biomarkers of recovery could provide prognostic information about a patient’s neural potential for recovery and improve our understanding of neural reorganization (Boyd et al., 2017). Investigating neural reorganization in children and adolescents is particularly challenging, since brain maturation is ongoing. So far, only a few longitudinal studies have been performed (for reviews see Dennis, Babikian, Giza, Thompson, & Asarnow, 2017; King, Ellis, Seri, & Wood, 2019), and none have investigated the link between neural reorganization and recovery of function.
In healthy subjects, many studies provide evidence that neural plasticity is related to slow wave activity (SWA) in the sleep-EEG (for a review see Tononi & Cirelli, 2014). For instance, in adults and children, SWA is locally increased after a learning experiences involving specific brain areas (e.g., Huber, Ghilardi, Massimini, & Tononi, 2004; Wilhelm et al., 2014). Also, the pattern of SWA changes in the course of development reflects ongoing brain maturation (Buchmann et al., 2011; Kurth et al., 2010). In the context of neural plasticity after brain injury (i.e., neural reorganization), one study investigated adult patients with chronic stroke and found local changes in SWA after speech therapy (Sarasso et al., 2014). We suggest that SWA might be a promising measure to investigate neural reorganization in children and adolescents with ABI.
In a previous paper, we reported cross-sectional alterations in SWA when comparing children and adolescents with ABI to typically developing children and adolescents. In the present paper, we present a subgroup of patients in which we investigated longitudinal changes in SWA and changes in every-day life memory impairment. We aimed to evaluate the feasibility of longitudinal sleep-EEG measurements in the clinical setting and to report preliminary findings of our approach using SWA to investigate neural correlates of recovery of function.
2Methods
2.1Participants
For the present study, we used a subgroup of a larger study population for which cross-sectional results were published in a previous paper (Mouthon et al., 2017). Twenty-two paediatric patients with ABI were recruited from the Swiss Children’s Rehab in Affoltern am Albis (Switzerland) between January 2013 and November 2014. One additional patient was recruited in August 2016. All parents gave written informed consent. Patients gave verbal consent. The study was approved by the local ethics committee. For the present study, we selected patients showing at least mild every-day life memory impairments at the beginning of rehabilitation. We excluded patients with disorders of consciousness (n = 5), patients showing no memory impairments (n = 1), and patients younger than 7 years of age (n = 2), be-cause memory function is scored differently in these patients. For five patients, memory function assessment was missing at the time point of the first EEG recording. In the end, ten patients were included. Demographic and clinical characteristics of the patients are shown in Table 1. All patients showed at least mild every-day life memory impairments at the beginning of rehabilitation. High-density EEG recordings and memory function scores were obtained at two time-points: at the beginning (T1), and end (T2) of rehabilitation. The first assessment (T1) took place early after admission (time since injury: 1.9±0.9 months). At this time all patients were in a subacute state after ABI. For two patients, in which hospitalization time exceeded 6 months (patients 3 and 4), we used an intermediate measurement instead of the measurement at the end of rehabilitation to assure comparable time intervals between the measurements (time interval for all patients: 3.5±1.5 months).
Table 1
Demographic and clinical characteristics of patients with acquired brain injury
Patient | Age, sex | Injury etiology | Affected subcortical structures | Affected cortical structures |
Pat 1 | 14 y, F | Right frontoparietal intraparenchymal and intraventricular hemorrhage with stroke (right capsula interna), cerebral edema | Right cortico-spinal tract, right capsula interna | Right g. frontalis medius and inferior; right g. precentralis; right g. postcentralis; right g. parietalis superior; right precuneus |
Pat 2 | 14 y, M | Stroke (right a. cerebri media and anterior) | Right g. frontalis, superior, medius and inferior; right g. precentralis; right g. postcentralis; right g. parietalis superior | |
Pat 3 | 13 y, M | TBI, intraventricular hemorrhage; subdural hematoma, cerebral edema, shearing injuries, DAI | Brainstem, midbrain, cerebellum; bilateral basal ganglia; corpus callosum | |
Pat 4 | 15 y, M | TBI, subarachnoid hemorrhage; subdural hematoma, shearing injuries, DAI | Brainstem, corpus callosum | |
Pat 5 | 7 y, F | TBI, contusion, cerebral edema, shearing injuries, DAI | Bilateral cerebellum, corpus callosum | |
Pat 6 | 9 y, F | TBI, contusion | Right g. frontalis superior and medius | |
Pat 7 | 10 y, M | Cardiac arrest, hypoxic-ischemic encephalopathy | Bilateral basal ganglia | |
Pat 8 | 14 y, F | Subarachnoid, intraparenchymal and intraventricular hemorrhagic stroke (cerebral arteriovenous malformation) | Left forceps major | |
Pat 9 | 13 y, F | Intraventricular and intraparenchymal hemorrhagic stroke | Left forceps major, left hippocampus, left ventromedial thalamus | |
Pat 10 | 13 y, M | Neuroborreliosis, meningoencephalitis | Brainstem, bilateral basal ganglia |
F = female; M = male; y = years; TBI = traumatic brain injury; DAI = diffuse axonal injury; g. = gyrus; ncl. = nucleus; a. = arteria.
We used high-density sleep EEG data from 52 typically developing children for the calculation of age norms. The data were provided by earlier studies (Kurth et al., 2010; Pugin et al., 2015).
2.2Assessment of memory function
Every-day life memory function was assessed using the memory item of the Functional Independence Measure for Children (WeeFIM®). The WeeFIM is a standardized clinical assessment that measures performance in daily life activities, taking into account the need for assistance. It shows evidence of reliability, validity, and responsiveness to change during rehabilitation for children and adolescents with ABI (McCauley et al., 2012). All items are rated on a 7-level ordinal scale. (The higher the score, the better the performance.) Examples for the memory rating are: “recognizing people frequently encountered”, “remembering daily routines”, or “executing requests without being reminded”. The rating was performed by trained nurses.
Memory improvement was quantified by the difference between the WeeFIM memory scores at T1 and T2 (i.e., Δ memory score = memory score T2 –memory score T1).
2.3High-density EEG recordings, data processing and analysis
High-density EEG is a method that is easy to apply in the clinical setting. Recordings during sleep are especially convenient since they can be performed at the bedside and do not additionally burden the patient during the day. Furthermore, the recordings provide a large amount of high-quality data, as in contrast to wake EEG data, there are almost no artefacts due to eye movements or muscle activity.
We used a high-density EEG system (Electrical Geodesics Inc.) to record brain activity during sleep. The 128 electrodes allow for a good spatial resolution. Recordings were sampled at 500 Hz and referenced to the vertex (Cz). The recorded EEG data were then band-pass filtered between 0.5 and 40 Hz and down-sampled to 128 Hz. Epochs containing artefacts were semi-automatically and visually rejected (Huber et al., 2000). Electrodes showing poor EEG signal quality were excluded. Data from electrodes above the ears (= 109 electrodes) were average-referenced. Missing data from excluded electrodes were interpolated using spherical linear interpolation (Wilhelm et al., 2014). Sleep stages were visually determined based on American Academy of Sleep Medicine standard criteria (20 s epochs, (Iber, Ancoli-Israel, Chesson, & Quan, 2007)). EEG spectral power was calculated for 20 s epochs (fast Fourier transform routine, Hanning window, average over five consecutive 4 s epochs). SWA was calculated by summing up spectral power from 1 to 4.5 Hz. We selected 90 epochs (= 30 min) of maximal SWA throughout the entire night and averaged SWA across the selected epochs, as previously done (Mouthon et al., 2017). Since we were interested in local SWA changes, we used relative SWA values for further calculations. Relative SWA values were obtained by dividing SWA values at each electrode by the average across all 109 electrodes (Huber et al., 2004; Kurth et al., 2010). Absolute SWA values are subject to profound day-to-day changes, which makes this measure less sensitive to uncover local changes in SWA. We then mapped the topographical distribution of SWA across the scalp.
Brain areas involved in memory processes under-go maturational changes throughout childhood and adolescence (DeMaster, Pathman, Lee, & Ghetti, 2014; Dumontheil, Burgess, & Blakemore, 2008; Ghetti & Bunge, 2012; Lavenex & Banta Lavenex, 2013; Robey, Buckingham-Howes, Salmeron, Black, & Riggins, 2014). Thus, maturation-dependent differences are likely to mask differences related to injury. To compare injury-related alterations in SWA across patients, we controlled for age-related differences. We used a previously developed approach to calculate age-normalized SWA values for individual patients (Mouthon et al., 2017). First, we determined SWA age norms (average and standard deviation) for different age groups of typically developing children and adolescents: 4–7 years (n = 10), 8–10 years (n = 11), 11–13 years (n = 16), 14–16 years (n = 15). Then, we calculated deviations from the age norm for all patients. For each electrode, we subtracted the group average from the patient’s individual relative SWA value and divided this difference by the group’s standard deviation [i.e., (individual value –group average)/group sd]. We used this “number of sd from the norm” as SWA value for further analysis. Changes in SWA were quantified by the difference between SWA at T1 and T2 (i.e., Δ SWA = SWA T2 –SWA T1).
2.4Electrode localization
We assigned electrode sites to cortical structures using a template that was provided by an earlier study. This template was based on the co-registration of high-density EEG electrode locations and individual MRI scans in a population of healthy children and adolescents (see Kurth et al., 2012).
2.5Statistical analyses
Since memory function was rated on an ordinal scale, all correlation analyses were performed using two-tailed Spearman correlation. The statistical significance level, α, was set at 0.05.
In a first step, we performed electrode-wise correlations between SWA (sd from norm) and memory improvement (i.e., “Δ SWA×Δ memory score”, “SWA T1×Δ memory score”) to visualize regions of interest (clusters of at least two neighbouring electrodes showing significant correlations). For the exploratory purpose of our analysis, we did not correct for multiple comparisons.
In a next step, we calculated average SWA for all regions of interest and performed correlation analyses with memory function. We used the bootstrap method to estimate the robustness of significant correlations (Efron, 1988; Efron & Tibshirani, 1986). We set the confidence level at 95%. For the correlation “SWA T1×Δ memory score”, we additionally performed a partial correlation controlling for the effects of initial memory score (memory score T1).
3Results
3.1Sleep structure
All patients tolerated the high-density EEG net well, and we could record good quality data throughout the entire night. At both time points (T1 and T2), the patient’s sleep structure differed from the sleep structure of typically developing children and adolescents (see Supplementary Table 1 for sleep variables and statistics). We found no statistically significant differences in sleep structure between the patients’ measurements at T1 and T2 (two-tailed paired t-tests).
3.2Age-normalized SWA topography
As previously shown, the patient’s individual SWA topographies (i.e., SWA distribution across the scalp) showed both age-specific and lesion-related aspects (see Fig. 1 for two examples, see (Mouthon et al., 2017) for more details). For further analysis, we calculated individual age-normalized SWA topographies based on age norms (see Fig. 1, see methods for details).
Fig. 1
Examples of SWA age norm, individual SWA and age-normalized SWA (at T1). (Left column) SWA average topographies for two age groups. (Middle column) Individual SWA topographies for two patients. (Right column) Age-normalized individual SWA topographies (sd from norm). Maximal values are indicated in red, minimal values in blue.
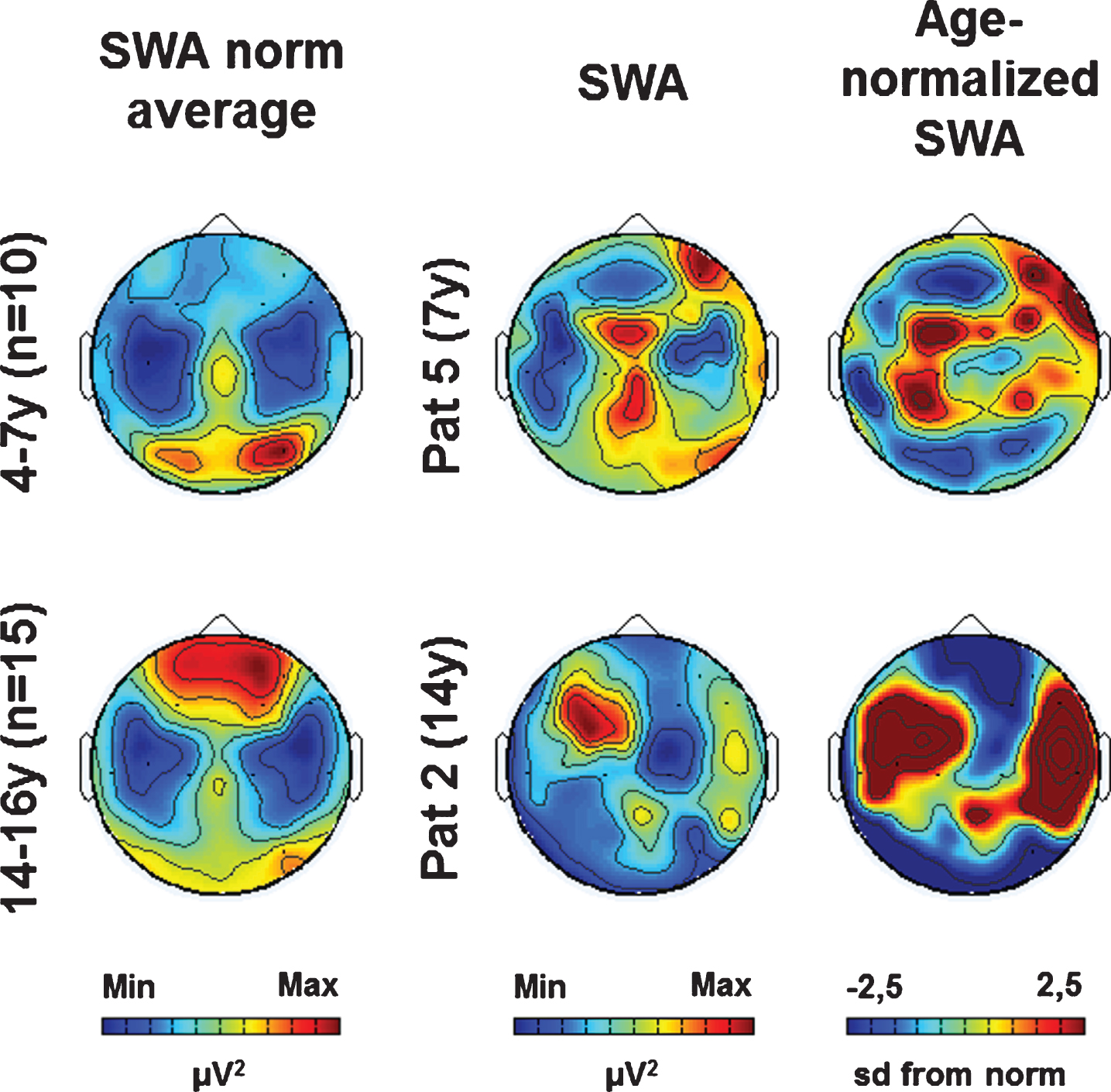
3.3Longitudinal changes in SWA and memory improvement
We performed electrode-wise correlations be-tween the individual age-normalized SWA topo-graphies (sd from the norm, see methods for details) and memory improvement. We used the measurements at the beginning and end of rehabilitation (SWA T1, memory score T1; SWA T2, memory score T2) to determine individual longitudinal changes in SWA (Δ SWA) and memory improvement (Δ memory score). The electrode-wise correlation analysis (Δ SWA×Δ memory score) showed a posterior parietal region of interest (BA 7, Fig. 2A). Cluster SWA change was positively correlated with memory improvement (r = 0.89 p < 0.001, bootstrapped 95% CI of the correlation coefficient = [0.27, 0.98], Fig. 2B). To examine the specificity of the correlation between cluster SWA change and memory improvement, we performed correlations between cluster SWA change and improvements in the two motor subscales of the WeeFIM (i.e., the subscale self-care including eating, grooming, bathing, dressing and toileting, and the subscale mobility including transfers and locomotion). Neither was related to changes in posterior parietal SWA (self-care: r = –0.10 p = 0.79; mobility: r = –0.11 p = 0.77).
Fig. 2
Correlation analyses of longitudinal changes in SWA and memory improvement. (A) Topographic distribution of correlation coefficients. Black dots indicate significant p-values (p < 0.05). (B) SWA increase in the parietal cluster was positively associated with memory improvement.
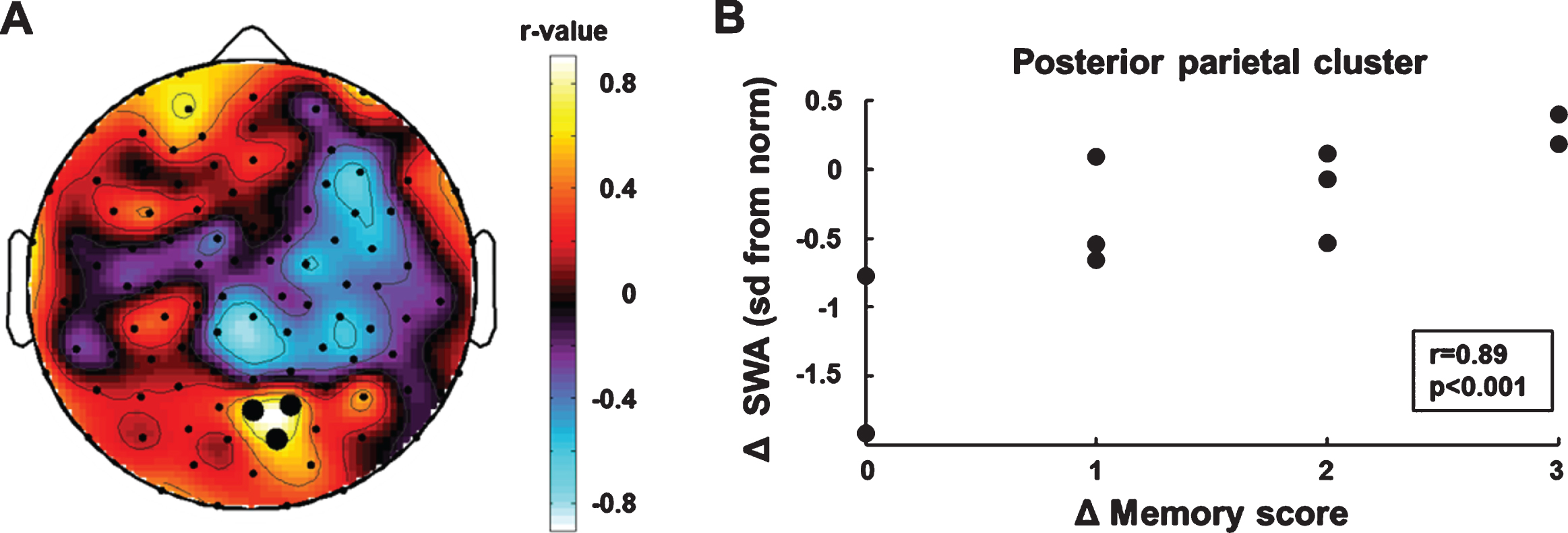
3.4Initial SWA predicting memory improvement
We performed an electrode-wise correlation analysis of initial SWA and memory improvement (SWA T1×Δ memory score) to identify brain areas in which SWA at T1 predicted memory improvement in the course of rehabilitation. This analysis yielded two regions of interest (Fig. 3A). One electrode cluster was located over left prefrontal brain areas (BA 10). SWA in this prefrontal cluster was positively correlated with memory improvement (r =0.81, p = 0.004, bootstrapped 95% CI of the correlation coefficient = [0.32, 0.95], Fig. 3B). Cluster SWA was not related to the motor subscales of the WeeFIM (self-care: r = –0.01 p = 0.81; mobility: r = –0.07 p = 0.85). The second electrode cluster was located over occipital brain areas. Cluster SWA was negatively correlated with memory improvement (r = –0.74, p = 0.014, bootstrapped 95% CI of the correlation coefficient = [–0.97, –0.12], Fig. 3C). Cluster SWA was not related to the motor subscales of the WeeFIM (self-care: r = –0.17 p = 0.65; mobility: r = –0.23 p = 0.52). The correlations between initial SWA and memory improvement remained similar in magnitude when performing partial correlations controlling for the effect of the initial memory score (left prefrontal cluster: r = 0.78, p = 0.013, bootstrapped 95% CI of the correlation coefficient = [0.21, 0.98]; occipital cluster: r = –0.67, p = 0.049, bootstrapped 95% CI of the correlation coefficient = [–0.98, 0.17]).
Fig. 3
Correlation analyses of initial SWA and memory improvement. (A) Topographic distribution of correlation coefficients. Black dots indicate significant p-values (p < 0.05). (B) Higher SWA in the left frontal cluster was positively associated with memory improvement. (C) Higher SWA in the occipital cluster was negatively associated with memory improvement.
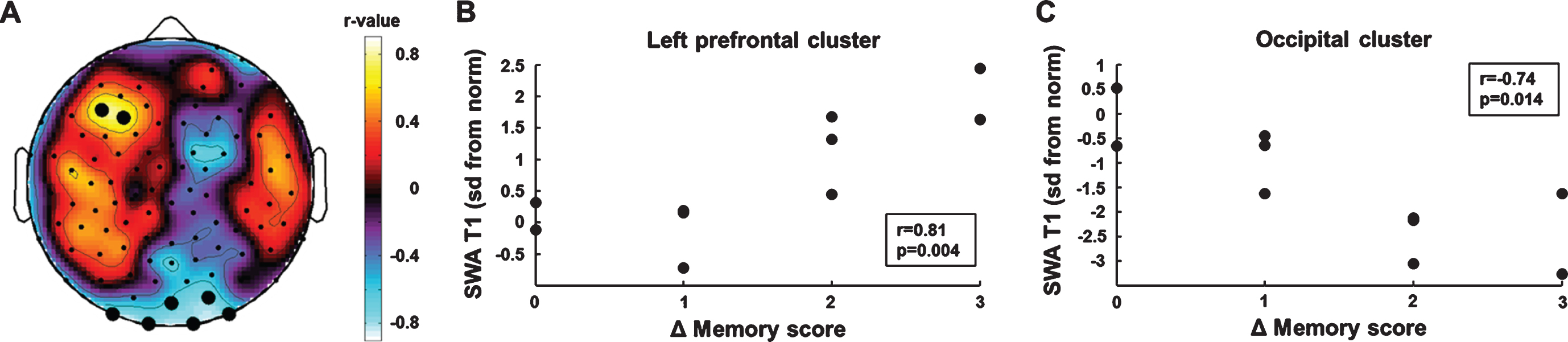
4Discussion
In the present study, we performed longitudinal measurements of every-day life memory function and sleep EEG SWA in children and adolescents with ABI. Our aim was to use SWA to investigate neural correlates of recovery of function.
4.1Age-normalization of SWA topography
To compare injury-related alterations in SWA across patients, we controlled for age-related differences. We determined individual age-normalized SWA topographies based on norm values for different age groups. We chose this approach because it is known that, like cortical grey matter thickness, SWA shows region-specific non-linear changes in the course of development (Buchmann et al., 2011; Campbell & Feinberg, 2009; Feinberg, Davis, de Bie, Grimm, & Campbell, 2011; Feinberg, Thode, Chugani, & March, 1990; Giedd et al., 1999; Gogtay et al., 2004; Huttenlocher & Dabholkar, 1997; Jenni & Carskadon, 2004; Kurth et al., 2010; Kurth et al., 2012; Lustenberger et al., 2017; Ringli & Huber, 2011; Shaw et al., 2008). By calculating age-normalized SWA values at each electrode location, we can account for both non-linearity and region-specificity.
4.2Neural changes paralleling memory improvement
The results of our exploratory approach showed that memory improvement from the first to the second measurement was related to longitudinal changes in SWA over posterior parietal brain areas (BA 7). The posterior parietal cortex (PPC) is part of a memory “core network” known to be involved in different memory functions. This “core network” includes the mentioned PPC, the medial temporal lobe (MTL), and the prefrontal cortex (PFC) (e.g., Benoit & Schacter, 2015; Burgess, Gonen-Yaacovi, & Volle, 2011; Cona, Scarpazza, Sartori, Moscovitch, & Bisiacchi, 2015; Martin et al., 2007; Poppenk, Moscovitch, McIntosh, Ozcelik, & Craik, 2010; Ranganath & Ritchey, 2012). Previous studies investigating neural correlates of different impaired memory functions after ABI reported alterations within the memory “core network” (DeMaster, Johnson, Juranek, & Ewing-Cobbs, 2017; Di Paola et al., 2015; Di Stefano et al., 2000; McCauley et al., 2010; Palacios et al., 2013; Schaapsmeerders et al., 2015; Snaphaan, Rijpkema, van Uden, Fernández, & de Leeuw, 2009) and connecting white matter tracts (Kraus et al., 2007; McCauley et al., 2011; Niogi et al., 2008; Palacios et al., 2013; Roberts, Mathias, & Rose, 2016). The PPC is an intriguing part of the memory “core network”. While its activation during different memory tasks is a consistent finding (Benoit & Schacter, 2015; Burgess et al., 2011; Cona et al., 2015), patients with PPC lesions do not show systematic memory deficits (Berryhill, 2012; Schoo et al., 2011; Sestieri, Shulman, & Corbetta, 2017). This phenomenon has also been described as the “posterior parietal paradox” (Schoo et al., 2011). Interestingly, two studies applying high-frequency repetitive transcranial magnetic stimulation (rTMS) to the PPC in healthy subjects could show that episodic memory improved after stimulation (Nilakantan, Bridge, Gagnon, VanHaerents, & Voss, 2017; Wang & Voss, 2015). This memory-enhancing effect of parietal rTMS was also demonstrated in Alzheimer’s patients (Koch et al., 2018). In our study, memory improvement was associated with longitudinal changes in posterior parietal SWA. This indicates that local changes in SWA might be used to detect brain areas involved in memory network restoration.
Since we did not assess any other cognitive function, we cannot rule out that the longitudinal changes in the PPC reflect a network reorganization related to a more general cognitive recovery. It has been shown that for instance attention and working memory also involve the PPC (e.g., Behrmann, Geng, & Shomstein, 2004; Eriksson, Vogel, Lansner, Bergström, & Nyberg, 2015). A study investigating attention and memory in Alzheimer’s patients suggested that memory impairment is caused by attention network dysfunction in the PPC (Veldsman, Zamboni, Butler, & Ahmed, 2019).
4.3Predictors of memory improvement
Our exploratory approach also showed that initial SWA over left prefrontal and occipital brain areas predicted memory recovery (i.e., improvement of memory performance from the first to the second measurement). PFC activation during different memory tasks is a consistent finding (Benoit & Schacter, 2015; Burgess et al., 2011; Cona et al., 2015). In contrast to PPC lesions, which do not systematically result in impaired memory functions, PFC lesions are known to cause deficits in episodic and prospective memory (Bertossi, Tesini, Cappelli, & Ciaramelli, 2016; Di Stefano et al., 2000; Umeda, Kurosaki, Terasawa, Kato, & Miyahara, 2011). It has been hypothesized that the PFC has a higher-level role and mediates MTL and PPC activity (Burgess et al., 2011; Sestieri et al., 2017). This hypothesis is further supported by findings from studies that investigated changes in memory function, in the course of normal development and aging (for reviews seeGhetti & Bunge, 2012; Tromp, Dufour, Lithfous, Pebayle, & Després, 2015)). Such changes (i.e., improvement in the course of development and decline in older age) potentially rely on structural and functional changes in the PFC. Cortical maturation or atrophy might, respectively, increase or decrease the capacity of the PFC to orchestrate memory network function. Furthermore, a study using transcranial direct current stimulation (tDCS) could show that stimulating the left PFC improved memory function in healthy adults (Medvedeva et al., 2018). Our finding that prefrontal SWA predicts memory improvement indicates that local alterations in SWA might be a useful measure to detect brain areas involved in memory network restoration.
Similar to the PPC, the PFC has also been related to attention and working memory (Bahmani et al., 2019; Eriksson et al., 2015). Further, the PFC is known to be a key brain area involved in executive functions (for a review see Koechlin, 2016). It has also been hypothesized that the PFC might be a cognitive control unit, monitoring many cognitive processes (Miller & Cohen, 2001). Thus, the predictive value of SWA in the PFC might not be specific for memory recovery. It is possible that the PFC also plays a role in the recovery of other cognitive functions.
The finding that lower initial occipital SWA predicts memory recovery is difficult to interpret. Unlike the PFC, occipital brain areas are not part of the memory “core network”, and to our knowledge, also attention, working memory, and executive functions have never been related to occipital brain areas.
4.4Limitations
A major limitation of our study is the small number of patients and the heterogeneity of the sample. While our approach specifically aims at minimizing age-related differences, it is not possible to control for lesion-related factors (e.g., size and site of the lesion). These factors may have different effects on the recovery process and increase variability in our data. Future studies could focus on specific etiology groups (e.g., traumatic brain injury or stroke). The findings we report, are preliminary and have to be interpreted with caution. The memory subscale of the WeeFIM is a clinical rating scale and not as precise as neuropsychological assessments. Additionally, the WeeFIM shows a ceiling effect since it measures the presence or absence of deficits and not maximal memory capacity. However, in pediatric patients showing memory deficits, WeeFIM memory scores and memory scores assessed using neuropsychological tests correspond well (McBride, 2015). We used the WeeFIM because it was the only available longitudinal assessment. In clinical routine, neuropsychological assessments were not standardized across patients and in most of the cases only performed at the beginning of rehabilitation. While neuropsychological tests provide information about maximal memory capacity, the WeeFIM provides information about a child’s real every-day life memory performance, which makes it relevant for patients and their social environment. We suggest that future studies should include both neuropsychological tests and observational rating scales since they provide complementary information. Another limitation is the specificity of the relationship between SWA and memory recovery with respect to other cognitive domains as for example attention. Future studies should include assessments of working memory, processing speed, attention, and executive functions. Such studies could differentiate between SWA that is related to general cognitive recovery (i.e., brain areas in which SWA is similarly related to improvements in different cognitive functions) and SWA that is related to the recovery of specific cognitive functions. However, we can rule out that SWA is merely related to overall recovery from ABI since the findings for memory recovery are not related to motor recovery. Another limitation of our study is that we cannot determine whether our findings are related to spontaneous or therapy-induced neural reorganization. During the subacute state, spontaneous recovery processes are still ongoing but in parallel, intensive rehabilitation therapy can enhance recovery (Wieloch & Nikolich, 2006). Specific therapy effects could be investigated in the chronic state, when the time window of spontaneous recovery is closed.
5Conclusions
In the present study, we performed longitudinal high-density EEG measurements during sleep in children and adolescents with every-day life memory impairment after ABI. We show that such measurements are feasible in the clinical setting. To our knowledge, this is the first longitudinal study investigating brain activity and functional outcome in children and adolescents with ABI. We report preliminary results linking memory recovery to parietal, prefrontal, and occipital brain areas. While the PPC and the PFC are known to belong to the memory “core network”, occipital brain areas have never been related to memory. This indicates that we have to remain cautious when interpreting preliminary findings. However, we suggest that the approach to use SWA as a measure to investigate neural reorganization is promising. Future studies, including a larger number of patients and assessing functions more specifically, could investigate whether SWA has the potential to serve as a biomarker for recovery of function.
Conflict of interest
The authors declare that the research was conducted in the absence of any commercial or financial relationships that could be construed as a potential conflict of interest.
Funding
This work was supported by the Anna-Müller Grocholski Foundation, the Mäxi Foundation, the Swiss National Science Foundation (grant P00P3-135438) and the Clinical Research Priority Program “Sleep and Health” of the University of Zurich.
Acknowledgments
The authors thank the nurses and the physicians of the Rehabilitation Center for Children and Adolescents in Affoltern am Albis for their support and all the patients and families for their participation. They also thank the sleep research lab of the University Children’s Hospital Zurich for the provided data from typically developing children.
Supplementary material
[1] The supplementary material is available in the electronic version of this article: https://dx.doi.org/10.3233/RNN-201140.
References
1 | Amlie-Lefond, C. , Sébire, G. , & Fullerton, H.J. ((2008) ). Recent developments in childhood arterial ischaemic stroke. The Lancet Neurology, 7: (5), 425–435. doi: 10.1016/S1474-4422(08)70086-3 |
2 | Bahmani, Z. , Clark, K. , Merrikhi, Y. , Mueller, A. , Pettine, W. , Isabel Vanegas, M. ,... Noudoost, B. ((2019) ). Prefrontal Contributions to Attention and Working Memory. Curr Top Behav Neurosci, 41: , 129–153. doi: 10.1007/7854_2018_74 |
3 | Beauchamp, M.H. , & Anderson, V. ((2013) ). Cognitive and psychopathological sequelae of pediatric traumatic brain injury. Handbook of Clinical Neurology, 112: , 913–920. doi: 10.1016/B978-0-444-52910-7.00013-1 |
4 | Behrmann, M. , Geng, J.J. , & Shomstein, S. ((2004) ). Parietal cortex and attention. Current Opinion in Neurobiology, 14: (2), 212–217. doi: 10.1016/j.conb.2004.03.012 |
5 | Benoit, R.G. , & Schacter, D.L. ((2015) ). Specifying the core network supporting episodic simulation and episodic memory by activation likelihood estimation. Neuropsychologia, 75: , 450–457. doi: 10.1016/j.neuropsychologia.2015.06.034 |
6 | Berryhill, M.E. ((2012) ). Insights from neuropsychology: pinpointing the role of the posterior parietal cortex in episodic and working memory. Frontiers in Integrative Neuroscienceront, 6: , 31. doi: 10.3389/fnint.2012.00031 |
7 | Bertossi, E. , Tesini, C. , Cappelli, A. , & Ciaramelli, E. ((2016) ). Ventromedial prefrontal damage causes a pervasive impairment of episodic memory and future thinking. Neuropsychologia, 90: , 12–24. doi: 10.1016/j.neuropsychologia.2016.01.034 |
8 | Boyd, L.A. , Hayward, K.S. , Ward, N.S. , Stinear, C.M. , Rosso, C. , Fisher, R.J. ,... Cramer, S.C. ((2017) ). Biomarkers of Stroke Recovery: Consensus-Based Core Recommendations from the Stroke Recovery and Rehabilitation Roundtable. Neurorehabilitation and Neural Repair, 31: (10-11), 864–876. doi: 10.1177/1545968317732680 |
9 | Buchmann, A. , Ringli, M. , Kurth, S. , Schaerer, M. , Geiger, A. , Jenni, O.G. , & Huber, R. ((2011) ). EEG sleep slow-wave activity as a mirror of cortical maturation. Cerebral Cortex, 21: (3), 607–615. doi: 10.1093/cercor/bhq129 |
10 | Burgess, P.W. , Gonen-Yaacovi, G. , & Volle, E. ((2011) ). Functional neuroimaging studies of prospective memory: what have we learnt so far? Neuropsychologia, 49: (8), 2246–2257. doi: 10.1016/j.neuropsychologia.2011.02.014 |
11 | Campbell, I.G. , & Feinberg, I. ((2009) ). Longitudinal trajectories of non-rapid eye movement delta and theta EEG as indicators of adolescent brain maturation. Proceedings of the National Academy of Sciences of the United States of America, 106: , 5177–5180. doi: 0812947106 [pii] 10.1073/pnas.0812947106. Epub 2009 Mar 23 |
12 | Cona, G. , Scarpazza, C. , Sartori, G. , Moscovitch, M. , & Bisiacchi, P.S. ((2015) ). Neural bases of prospective memory: a meta-analysis and the “Attention to Delayed Intention” (AtoDI) model. Neuroscience and Biobehavioral Reviews, 52: , 21–37. doi: 10.1016/j.neubiorev.2015.02.007 |
13 | DeMaster, D. , Johnson, C. , Juranek, J. , & Ewing-Cobbs, L. ((2017) ). Memory and the hippocampal formation following pediatric traumatic brain injury. Brain and Behavior, 7: (12), e00832. doi: 10.1002/brb3.832 |
14 | DeMaster, D. , Pathman, T. , Lee, J.K. , & Ghetti, S. ((2014) ). Structural development of the hippocampus and episodic memory: developmental differences along the anterior/posterior axis. Cerebral Cortex, 24: (11), 3036–3045. doi: 10.1093/cercor/bht160 |
15 | Dennis, E.L. , Babikian, T. , Giza, C.C. , Thompson, P.M. , & Asarnow, R.F. ((2017) ). Diffusion MRI in pediatric brain injury. Child’s Nervous System, 33: (10), 1683–1692. doi: 10.1007/s00381-017-3522-y |
16 | Di Paola, M. , Phillips, O. , Costa, A. , Ciurli, P. , Bivona, U. , Catani, S. ,... Carlesimo, G. A. ((2015) ). Selective Cognitive Dysfunction Is Related to a Specific Pattern of Cerebral Damage in Persons With Severe Traumatic Brain Injury. The Journal of Head Trauma Rehabilitation, 30: (6), 402–410. doi: 10.1097/HTR.0000000000000063 |
17 | Di Stefano, G. , Bachevalier, J. , Levin, H.S. , Song, J.X. , Scheibel, R.S. , & Fletcher, J.M. ((2000) ). Volume of focal brain lesions and hippocampal formation in relation to memory function after closed head injury in children. Journal of Neurology, Neurosurgery, and Psychiatry, 69: (2), 210–216. |
18 | Dumontheil, I. , Burgess, P.W. , & Blakemore, S.J. ((2008) ). Development of rostral prefrontal cortex and cognitive and behavioural disorders. Developmental Medicine and Child Neurology, 50: (3), 168–181. doi: 10.1111/j.1469-8749.2008.02026.x |
19 | Efron, B. ((1988) ). Bootstrap confidence intervals: Good or bad? Psychological Bulletin, 104: , 293–296. |
20 | Efron, B. , & Tibshirani, R. ((1986) ). Bootstrap methods for standard errors, confidence intervals, and other measures of statistical accuracy. Statistical Science, 1: , 54–77. |
21 | Eriksson, J. , Vogel, E.K. , Lansner, A. , Bergström, F. , & Nyberg, L. ((2015) ). Neurocognitive Architecture of Working Memory. Neuron, 88: (1), 33–46. doi: 10.1016/j.neuron.2015.09.020 |
22 | Feinberg, I. , Davis, N.M. , de Bie, E. , Grimm, K.J. , & Campbell, I.G. ((2011) ). The maturational trajectories of NREM and REM sleep durations differ across adolescence on both school-night and extended sleep. American Journal of Physiology-Regulatory, Integrative and Comparative Physiology, 302: , R533–540. doi: ajpregu.00532.2011 [pii] 10.1152/ajpregu.00532.2011. Epub 2011 Nov 23 |
23 | Feinberg, I. , Thode, H.C. , Chugani, H.T. , & March, J.D. ((1990) ). Gamma distribution model describes maturational curves for delta wave amplitude, cortical metabolic rate and synaptic density. Journal of Theoretical Biology, 142: (2), 149–161. |
24 | Ghetti, S. , & Bunge, S.A. ((2012) ). Neural changes underlying the development of episodic memory during middle childhood. Developmental Cognitive Neuroscience, 2: (4), 381–395. doi: 10.1016/j.dcn.2012.05.002 |
25 | Giedd, J.N. , Blumenthal, J. , Jeffries, N.O. , Castellanos, F.X. , Liu, H. , Zijdenbos, A. ,... Rapoport, J.L. ((1999) ). Brain development during childhood and adolescence: a longitudinal MRI study. Nature Neuroscience, 2: (10), 861–863. doi: 10.1038/13158 |
26 | Gogtay, N. , Giedd, J.N. , Lusk, L. , Hayashi, K.M. , Greenstein, D. , Vaituzis, A.C. ,... Thompson, P.M. ((2004) ). Dynamic mapping of human cortical development during childhood through early adulthood. Proceedings of the National Academy of Sciences of the United States of America, 101: , 8174–8179. doi: 10.1073/pnas.0402680101 0402680101 [pii] |
27 | Huber, R. , Ghilardi, M.F. , Massimini, M. , & Tononi, G. ((2004) ). Local sleep and learning. Nature, 430: , 78–81. doi: 10.1038/nature02663 nature02663 [pii] |
28 | Huber, R. , Graf, T. , Cote, K.A. , Wittmann, L. , Gallmann, E. , Matter, D. ,... Achermann, P. ((2000) ). Exposure to pulsed high-frequency electromagnetic field during waking affects human sleep EEG. Neuroreport, 11: , 3321–3325. |
29 | Huttenlocher, P.R. , & Dabholkar, A.S. ((1997) ). Regional differences in synaptogenesis in human cerebral cortex. Journal of Comparative Neurology, 387: , 167–178. doi: 10.1002/(SICI)1096-9861(19971020)387:2<167::AID-CNE1>3.0.CO;2-Z [pii] |
30 | Iber, C. , Ancoli-Israel, S. , Chesson, A.L. , & Quan, S.F. ((2007) ). The AASM manual for the scoring of sleep and associated events: rules, terminology and technical specifications (1 st ed.). Westchester, Illinois: American Academy of Sleep Medicine. |
31 | Jenni, O.G. , & Carskadon, M.A. ((2004) ). Spectral analysis of the sleep electroencephalogram during adolescence. Sleep, 27: , 774–783. |
32 | King, D.J. , Ellis, K.R. , Seri, S. , & Wood, A.G. ((2019) ). A systematic review of cross-sectional differences and longitudinal changes to the morphometry of the brain following paediatric traumatic brain injury. Neuroimage Clinical, 23: , 101844. doi: 10.1016/j.nicl.2019.101844 |
33 | Koch, G. , Bonnì, S. , Pellicciari, M.C. , Casula, E.P. , Mancini, M. , Esposito, R. ,... Bozzali, M. ((2018) ). Transcranial magnetic stimulation of the precuneus enhances memory and neural activity in prodromal Alzheimer’s disease. Neuroimage, 169: , 302–311. doi: 10.1016/j.neuroimage.2017.12.048 |
34 | Koechlin, E. ((2016) ). Prefrontal executive function and adaptive behavior in complex environments. Current Opinion in Neurobiology, 37: , 1–6. doi: 10.1016/j.conb.2015.11.004 |
35 | Kraus, M.F. , Susmaras, T. , Caughlin, B.P. , Walker, C.J. , Sweeney, J.A. , & Little, D.M. ((2007) ). White matter integrity and cognition in chronic traumatic brain injury: a diffusion tensor imaging study. Brain, 130: (Pt 10), 2508–2519. doi: 10.1093/brain/awm216 |
36 | Kuhtz-Buschbeck, J.P. , Hoppe, B. , Gölge, M. , Dreesmann, M. , Damm-Stünitz, U. , & Ritz, A. ((2003) ). Sensorimotor recovery in children after traumatic brain injury: analyses of gait, gross motor, and fine motor skills. Developmental Medicine and Child Neurology, 45: (12), 821–828. |
37 | Kurth, S. , Ringli, M. , Geiger, A. , LeBourgeois, M. , Jenni, O.G. , & Huber, R. ((2010) ). Mapping of cortical activity in the first two decades of life: a high-density sleep electroencephalogram study. Journal of Neuroscience, 30: , 13211–13219. doi: 30/40/13211 [pii] 10.1523/JNEUROSCI.2532-10.2010 |
38 | Kurth, S. , Ringli, M. , Lebourgeois, M.K. , Geiger, A. , Buchmann, A. , Jenni, O.G. , & Huber, R. ((2012) ). Mapping the electrophysiological marker of sleep depth reveals skill maturation in children and adolescents. Neuroimage, 63: , 959–965. doi: S1053-8119(12)00334-5 [pii] 10.1016/j.neuroimage.2012.03.053. Epub 2012 Mar 28 |
39 | Lavenex, P. , & Banta Lavenex, P. ((2013) ). Building hippocampal circuits to learn and remember: insights into the development of human memory. Behavioural Brain Research, 254: , 8–21. doi: 10.1016/j.bbr.2013.02.007 |
40 | Lustenberger, C. , Mouthon, A.L. , Tesler, N. , Kurth, S. , Ringli, M. , Buchmann, A. ,... Huber, R. ((2017) ). Developmental trajectories of EEG sleep slow wave activity as a marker for motor skill development during adolescence: a pilot study. Developmental Psychobiology, 59: (1), 5–14. doi: 10.1002/dev.21446 |
41 | Martin, T. , McDaniel, M.A. , Guynn, M.J. , Houck, J.M. , Woodruff, C.C. , Bish, J.P. ,... Tesche, C.D. ((2007) ). Brain regions and their dynamics in prospective memory retrieval: a MEG study. International Journal of Psychophysiology, 64: (3), 247–258. doi: 10.1016/j.ijpsycho.2006.09.010 |
42 | McBride, T. ((2015) ). Neuropsychological scores and WeeFIM cognitive ratings of children with traumatic brain injury: A brief report. Brain Injury, 29: (7-8), 951–954. doi: 10.3109/02699052.2015.1022881 |
43 | McCauley, S.R. , Wilde, E.A. , Anderson, V.A. , Bedell, G. , Beers, S.R. , Campbell, T.F. ,... Workgroup, Pediatric TBI Outcomes. ((2012) ). Recommendations for the use of common outcome measures in pediatric traumatic brain injury research. Journal of Neurotrauma Neurotrauma, 29: (4), 678–705. doi: 10.1089/neu.2011.1838 |
44 | McCauley, S.R. , Wilde, E.A. , Bigler, E.D. , Chu, Z. , Yallampalli, R. , Oni, M.B. ,... Levin, H.S. ((2011) ). Diffusion tensor imaging of incentive effects in prospective memory after pediatric traumatic brain injury. Journal of Neurotrauma, 28: (4), 503–516. doi: 10.1089/neu.2010.1555 |
45 | McCauley, S.R. , Wilde, E.A. , Merkley, T.L. , Schnelle, K.P. , Bigler, E.D. , Hunter, J.V. ,... Levin, H.S. ((2010) ). Patterns of cortical thinning in relation to event-based prospective memory performance three months after moderate to severe traumatic brain injury in children. Developmental Neuropsychology, 35: (3), 318–332. doi: 10.1080/87565641003696866 |
46 | Medvedeva, A. , Materassi, M. , Neacsu, V. , Beresford-Webb, J. , Hussin, A. , Khan, N. ,... Galli, G. ((2018) ). Effects of Anodal Transcranial Direct Current Stimulation Over the Ventrolateral Prefrontal Cortex on Episodic Memory Formation and Retrieval. Cerebral Cortex. doi:10.1093/cercor/bhx347 |
47 | Miller, E.K. , & Cohen, J.D. ((2001) ). An integrative theory of prefrontal cortex function. Annual Review of Neuroscience, 24: , 167–202. doi: 10.1146/annurev.neuro.24.1.167 |
48 | Mouthon, A.L. , Meyer-Heim, A. , Kurth, S. , Ringli, M. , Pugin, F. , van Hedel, H.J. , & Huber, R. ((2017) ). High-Density Electroencephalographic Recordings During Sleep in Children and Adolescents With Acquired Brain Injury. Neurorehabilitation and Neural Repair, 1545968316688794. doi:10.1177/1545968316688794 |
49 | Nilakantan, A.S. , Bridge, D.J. , Gagnon, E.P. , VanHaerents, S.A. , & Voss, J.L. ((2017) ). Stimulation of the Posterior Cortical-Hippocampal Network Enhances Precision of Memory Recollection. Current Biology, 27: (3), 465–470. doi: 10.1016/j.cub.2016.12.042 |
50 | Niogi, S.N. , Mukherjee, P. , Ghajar, J. , Johnson, C.E. , Kolster, R. , Lee, H. ,... McCandliss, B.D. ((2008) ). Structural dissociation of attentional control and memory in adults with and without mild traumatic brain injury. Brain, 131: (Pt 12), 3209–3221. doi: 10.1093/brain/awn247 |
51 | Palacios, E.M. , Sala-Llonch, R. , Junque, C. , Fernandez-Espejo, D. , Roig, T. , Tormos, J.M. ,... Vendrell, P. ((2013) ). Long-term declarative memory deficits in diffuse TBI: correlations with cortical thickness, white matter integrity and hippocampal volume. Cortex, 49: (3), 646–657. doi: 10.1016/j.cortex.2012.02.011 |
52 | Poppenk, J. , Moscovitch, M. , McIntosh, A.R. , Ozcelik, E. , & Craik, F.I. ((2010) ). Encoding the future: successful processing of intentions engages predictive brain networks. Neuroimage, 49: (1), 905–913. doi: 10.1016/j.neuroimage.2009.08.049 |
53 | Pugin, F. , Metz, A.J. , Wolf, M. , Achermann, P. , Jenni, O.G. , & Huber, R. ((2015) ). Local increase of sleep slow wave activity after three weeks of working memory training in children and adolescents. Sleep, 38: , 607–614. doi: sp-00230-14 [pii] 10.5665/sleep.4580 10.5665/sleep.4580 |
54 | Ranganath, C. , & Ritchey, M. ((2012) ). Two cortical systems for memory-guided behaviour. Nature Reviews Neuroscience, 13: (10), 713–726. doi: 10.1038/nrn3338 |
55 | Ringli, M. , & Huber, R. ((2011) ). Developmental aspects of sleep slow waves: linking sleep, brain maturation and behavior. Progress in Brain Research, 193: , 63–82. doi: 10.1016/B978-0-444-53839-0.00005-3 |
56 | Roberts, R.M. , Mathias, J.L. , & Rose, S.E. ((2016) ). Relationship Between Diffusion Tensor Imaging (DTI) Findings and Cognition Following Pediatric TBI: A Meta-Analytic Review. Developmental Neuropsychology, 41: (3), 176–200. doi: 10.1080/87565641.2016.1186167 |
57 | Robey, A. , Buckingham-Howes, S. , Salmeron, B.J. , Black, M.M. , & Riggins, T. ((2014) ). Relations among prospective memory, cognitive abilities, and brain structure in adolescents who vary in prenatal drug exposure. Journal of Experimental Child Psychology, 127: , 144–162. doi: 10.1016/j.jecp.2014.01.008 |
58 | Sarasso, S. , Määttä, S. , Ferrarelli, F. , Poryazova, R. , Tononi, G. , & Small, S.L. ((2014) ). Plastic changes following imitation-based speech and language therapy for aphasia: a high-density sleep EEG study. Neurorehabilitation and Neural Repair, 28: (2), 129–138. doi: 10.1177/1545968313498651 |
59 | Schaapsmeerders, P. , van Uden, I.W. , Tuladhar, A.M. , Maaijwee, N.A. , van Dijk, E.J. , Rutten-Jacobs, L.C. ,... Kessels, R.P. ((2015) ). Ipsilateral hippocampal atrophy is associated with long-term memory dysfunction after ischemic stroke in young adults. Human Brain Mapping, 36: (7), 2432–2442. doi: 10.1002/hbm.22782 |
60 | Schoo, L.A. , van Zandvoort, M.J. , Biessels, G.J. , Kappelle, L.J. , Postma, A. , & de Haan, E.H. ((2011) ). The posterior parietal paradox: Why do functional magnetic resonance imaging and lesion studies on episodic memory produce conflicting results? Journal of Neuropsychology, 5: (Pt 1), 15–38. doi: 10.1348/174866410X504059 |
61 | Sestieri, C. , Shulman, G.L. , & Corbetta, M. ((2017) ). The contribution of the human posterior parietal cortex to episodic memory. Nature Reviews Neuroscience, 18: (3), 183–192. doi: 10.1038/nrn.2017.6 |
62 | Shaw, P. , Kabani, N.J. , Lerch, J.P. , Eckstrand, K. , Lenroot, R. , Gogtay, N. ,... Wise, S.P. ((2008) ). Neurodevelopmental trajectories of the human cerebral cortex. Journal of Neuroscience, 28: (14), 3586–3594. doi: 10.1523/JNEUROSCI.5309-07.2008 |
63 | Snaphaan, L. , Rijpkema, M. , van Uden, I. , Fernández, G. , & de Leeuw, F.E. ((2009) ). Reduced medial temporal lobe functionality in stroke patients: a functional magnetic resonance imaging study. Brain, 132: (Pt 7), 1882–1888. doi: 10.1093/brain/awp133 |
64 | Tononi, G. , & Cirelli, C. ((2014) ). Sleep and the price of plasticity: from synaptic and cellular homeostasis to memory consolidation and integration. Neuron, 81: , 12–34. doi: S0896-6273(13)01186-0 [pii] 10.1016/j.neuron.2013.12.025 |
65 | Tromp, D. , Dufour, A. , Lithfous, S. , Pebayle, T. , & Després, O. ((2015) ). Episodic memory in normal aging and Alzheimer disease: Insights from imaging and behavioral studies. Ageing Research Reviews, 24: (Pt B), 232–262. doi: 10.1016/j.arr.2015.08.006 |
66 | Umeda, S. , Kurosaki, Y. , Terasawa, Y. , Kato, M. , & Miyahara, Y. ((2011) ). Deficits in prospective memory following damage to the prefrontal cortex. Neuropsychologia, 49: (8), 2178–2184. doi: 10.1016/j.neuropsychologia.2011.03.036 |
67 | Veldsman, M. , Zamboni, G. , Butler, C. , & Ahmed, S. ((2019) ). Attention network dysfunction underlies memory impairment in posterior cortical atrophy. Neuroimage Clinical, 22: , 101773. doi: 10.1016/j.nicl.2019.101773 |
68 | Wang, J.X. , & Voss, J.L. ((2015) ). Long-lasting enhancements of memory and hippocampal-cortical functional connectivity following multiple-day targeted noninvasive stimulation. Hippocampus, 25: (8), 877–883. doi: 10.1002/hipo.22416 |
69 | Wieloch, T. , & Nikolich, K. ((2006) ). Mechanisms of neural plasticity following brain injury. Current Opinion in Neurobiology, 16: , 258–264. doi: S0959-4388(06)00062-6 [pii] 10.1016/j.conb.2006.05.011 |
70 | Wilhelm, I. , Kurth, S. , Ringli, M. , Mouthon, A.L. , Buchmann, A. , Geiger, A. ,... Huber, R. ((2014) ). Sleep slow-wave activity reveals developmental changes in experience-dependent plasticity. Journal of Neuroscience, 34: , 12568–12575. doi: 34/37/12568 [pii] 10.1523/JNEUROSCI.0962-14.2014 |