Combining neurofeedback with source estimation: Evaluation of an sLORETA neurofeedback protocol for chronic tinnitus treatment
Abstract
Background:
Alpha/delta neurofeedback has been shown to be a potential treatment option for chronic subjective tinnitus. Traditional neurofeedback approaches working with a handful of surface electrodes have been criticized, however, due to their low spatial specificity.
Objective:
The purpose of this study was to evaluate an innovative tomographic neurofeedback protocol that combines neural activity measured across the whole scalp with sLORETA source estimation.
Methods:
Forty-eight tinnitus patients participated in 15 neurofeedback training sessions as well as extensive pre, post, and follow-up testing. Patients were randomly assigned to either a tomographic (TONF) or a traditional electrode-based neurofeedback (NTNF) group. Main outcome measures of this study were defined as tinnitus-related distress measured with the Tinnitus Handicap Inventory (THI) and Tinnitus Questionnaire (TQ), tinnitus loudness, and resting-state EEG activity in trained frequency bands.
Results:
For both groups a significant reduction of tinnitus-related distress and tinnitus loudness was found. While distress changes remained persistent irrespective of group, loudness levels returned to baseline in the follow-up period. No significant between-group differences between the 2 neurofeedback applications (TONF vs. NTNF) were found, which suggests a similar contribution to symptom improvement. The trained alpha/delta ratio increased significantly over the course of the training and remained stable in the follow-up period. This effect was found irrespective of group on both surface and source levels with no meaningful differences between the 2 groups.
Conclusions:
Our study shows that a tomographic alpha/delta protocol should be considered a promising addition to tinnitus treatment but that more individually specific neurofeedback protocols should be developed.
1Introduction
The condition of perceiving a permanent ringing or hissing sound is commonly referred to as chronic subjective tinnitus. Although it affects roughly 15% of people in Western societies, this condition still remains largely untreated (Henry, Dennis, & Schechter, 2005). For many affected people the penetrating nature of the percept and the lack of treatment options for it lead to severe impairments in quality of life, and sometimes even the development of other health issues and comorbidities such as depression or anxiety (Dobie, 2003; Heller, 2003; Holmes & Padgham, 2008; Langguth, Landgrebe, Kleinjung, Sand, & Hajak, 2011).
Electrophysiological recordings with electroencephalography (EEG) or magnetoencephalography (MEG) have recently led to a better understanding of the origin of the chronic phantom sound. Comparing spontaneous neuronal activity of human tinnitus patients with healthy controls, many previous studies reported enhanced delta (0.5–4 Hz) and decreased alpha (8.5–12 Hz) oscillations for the tinnitus group (Adjamian, Sereda, & Hall, 2009; Ashton et al., 2007; Kahlbrock & Weisz, 2008; Lorenz, Müller, Schlee, Hartmann, & Weisz, 2009; Schlee et al., 2008; Weisz, Dohrmann, & Elbert, 2007; Weisz, Moratti, Meinzer, Dohrmann, & Elbert, 2005; Weisz, Müller, et al., 2007). It is hypothesized that this pattern is indicative of increased spontaneous firing of thalamic fibers due to deprived auditory input combined with decreased lateral inhibition processes at the cortical level (De Ridder, Vanneste, Langguth, & Llinás, 2015; Llinás, Ribary, Jeanmonod, Kronberg, & Mitra, 1999; Weisz, Dohrmann, & Elbert, 2007).
Using neuromodulation methods such as neurofeedback, other scholars have already tried to modulate the abnormalities found in these frequency bands and to test its effects on tinnitus perception (for a review, see Güntensperger, Thüring, Meyer, Neff, & Kleinjung, 2017). Neurofeedback is a non-invasive approach that combines electrophysiological recording with direct sensory—mostly visual—feedback of brain activity that would otherwise not be directly perceived. This real-time perception of neuronal activity, and the learning initiated through the rewarding of positive and inhibiting of negative changes, leads to the permanent establishment of the targeted patterns after a sufficient number of training sessions. Currently, neurofeedback is receiving increasing attention as a promising treatment of a multitude of psychological disorders, and has already been prominently applied in the therapy of attention deficit hyperactivity disorder (ADHD) (Arns, De Ridder, Strehl, Breteler, & Coenen, 2009; Gevensleben et al., 2009; Lévesque, Beauregard, & Mensour, 2006; Lubar, Swartwood, Swartwood, & O’Donnell, 1995; Strehl et al., 2017; van Doren et al., 2019). For tinnitus treatment, alpha/delta protocols aiming at increasing alpha and decreasing delta oscillations over auditory areas have been the most intensely studied (Crocetti, Forti, & Del Bo, 2011; Dohrmann, Elbert, Schlee, & Weisz, 2007). In a previous report by our group, we found that this neurofeedback protocol leads to meaningful and also partly stable reductions of self-reported tinnitus distress and loudness (Güntensperger, Thüring, Kleinjung, Neff, & Meyer, 2019). Furthermore, these behavioral changes were found to be linked to training-induced changes of resting-state brain activity, suggesting a specific effect of this protocol.
Despite recent success, traditional neurofeedback protocols using EEG to measure feedback-relevant brain activity have been criticized due to their low spatial specificity (e.g., White, Congedo, & Ciorciari, 2014). Even though electrodes on the scalp convey electrical brain activity in real-time, the exact sources or neural generators of the measured signal are usually unknown. This issue is commonly referred to as the inverse problem and dates back to Helmholtz (1853). Since the signal acquired at a given electrode thus always reflects activity originating from multiple brain regions, neurofeedback protocols that use this signal for feedback are unable to constrain the training effects to specific brain areas. Rather, it is highly likely that the effects occur on a more global level or that the procedure affects multiple, spatially adjacent but functionally different brain regions simultaneously.
Recently, inverse solution algorithms have been developed with the aim of identifying neural generators of EEG signals. Since the inverse problem is unsolvable by definition, certain a priori constraints related to the physiological properties of EEG signal generation are necessary (Michel et al., 2004). Neural sources of recorded EEG on the scalp can thus only be estimated and every solution entails uncertainty. Examples for frequently used source estimation techniques are Low Resolution Electromagnetic Tomography (LORETA) (Pascual-Marqui, Michel, & Lehmann, 1994), its improved version, Standardized Low Resolution Electromagnetic Tomography (sLORETA) (Pascual-Marqui, 2002), and Beamformer algorithms (van Veen, van Drongelen, Yuchtman, & Suzuki, 1997).
To increase spatial resolution, tomographic neurofeedback (TONF) protocols have been developed that combine classical sparse-electrode (NTNF) approaches with EEG source estimation. Congedo, Lubar, and Joffe (2004) designed a tomographic neurofeedback protocol by implementing the LORETA algorithm in feedback calculation with 19 active electrodes. Six healthy subjects were able to alter the intended EEG pattern (i.e., an increase of their beta/alpha ratio) specifically in the training region (here defined as the anterior cingulate cortex, ACC). Follow-up studies using tomographic neurofeedback with the same algorithm confirmed the clinical relevance of this approach by showing improvements in working memory, attention, and self-regulation processes of patients with addiction disorders or ADHD (Cannon et al., 2007; Cannon et al., 2006; Cannon, Lubar, Sokhadze, & Baldwin, 2008). Two studies investigated the effectiveness of tomographic neurofeedback in the treatment of chronic tinnitus.
Hartmann, Lorenz, Müller, Langguth, and Weisz (2013) worked with eight subjects who trained an increase in their alpha frequency band. They used dipole-source space projection to estimate the signal recorded with 32 EEG electrodes in two regional dipole-sources situated bilaterally in the temporal cortex. The results of this study showed tinnitus-related distress to be significantly lowered after the training period and alpha activity over the right primary auditory cortex (PAC) to be significantly increased. The group of Vanneste, Joos, Ost, and De Ridder (2016) chose LORETA source estimation for their tomographic tinnitus protocol. In this study, 58 tinnitus patients received alpha-up, beta- and gamma-down neurofeedback over the posterior cingulate cortex (PCC) as the neural target area of the tinnitus protocol or over the lingual gyrus as the neural control region. A decrease of tinnitus-related distress was reported for the PCC-group which was attributed to neurofeedback-induced changes in functional and effective connectivity between PCC and different areas of their proposed tinnitus distress network (Vanneste et al., 2010).
Despite the fact that tomographic EEG neurofeedback has thus already been shown to hold great potential, as yet only two studies have attempted to implement it in tinnitus treatment. This project aims to expand this research by comparing a tomographic neurofeedback protocol with an already established traditional neurofeedback option and to evaluate its potential benefit in the treatment of chronic tinnitus. In our recent work, we showed that alpha-up/delta-down neurofeedback with an individually adjusted alpha reward band resulted in improvements of tinnitus symptoms and intended alterations in brain activity (Güntensperger et al., 2019). For the tinnitus group reported in this recent publication, feedback-relevant EEG was measured with only four fronto-central electrodes on the scalp (FC1, FC2, F3, F4). These were chosen based on previous successful studies with the same protocol in which they were hypothesized to represent activity in the auditory cortex (Crocetti et al., 2011; Dohrmann et al., 2007). However, as already mentioned, it remains unclear whether this sparse-electrode approach is able to alter cortical activity patterns in this brain region specifically. Indeed, we confirmed this reservation as the increase of the trained alpha/delta ratio was found across the whole scalp and was not specific to the trained electrode sites. A second group of tinnitus patients was included in this clinical project, the results of which will be reported here. The neurofeedback training protocol of this group was identical (alpha-up/delta-down) but for one difference: tomographic (TONF) instead of the classical sparse-electrode (NTNF) neurofeedback was used (see section 2.2 for details). Feedback-relevant activity was thus measured with 31 (instead of four) active EEG electrodes on the scalp and an sLORETA source estimation algorithm was implemented in feedback generation aiming at limiting training effects to the auditory brain regions.
The main outcome measures of this study were tinnitus-related distress, subjective tinnitus loudness, and resting-state alpha/delta ratio on surface and source levels. As already reported for the non-tomographic (NTNF) group (Güntensperger et al., 2019), we expected meaningful and stable symptom improvements, in particular for tinnitus distress. Considering our data, we hypothesized significant decreases of THI, TQ, as well as loudness scores between pre- and post-tests. Furthermore, differences between the two follow-up time points compared to the baseline (pre-test) were expected to be significantly lower. Lastly, we hypothesized that neurofeedback of both groups would lead to the proposed changes in EEG patterns. The trained alpha/delta ratio was thus expected to increase between pre- and post-tests, and also to remain on this significantly higher level in the follow-up period. In this context, we expected tomographic neurofeedback to result in more specific effects over auditory areas and hence that the EEG patterns of this patient group would reflect spatially restricted alterations over auditory areas.
2Methods
This study was part of a larger clinical project, the data of which has already been published in Güntensperger et al. (2019). The methods described in this report therefore partly reproduce the wording of this recent publication.
2.1Participants
Fifty-three chronic tinnitus patients were recruited at the Department of Otorhinolaryngology (University Hospital Zurich). Participants had to be diagnosed with chronic subjective tinnitus (>0.5 years), be between 18 and 75 years old, have adequate knowledge of the German language, wear no cochlear implants, suffer from no psychiatric or neurological disorder, have no acute suicidal tendency, show no signs of drug or alcohol addiction, and have no current prescriptions for tranquilizers, neuroleptics, or anti-epileptics. Three participants decided to leave the trial before finishing the full study procedure (described in section 2.2) and were thus excluded from data analysis. Furthermore, two patients who completed the full study procedure had to be excluded because their depression scores at all four time points suggested clinically relevant depressive symptoms (i.e., BDI sum-scores of more than 18 points). Unfortunately, one patient died due to an unrelated health issue before completion of the last follow-up measurement. The obtained data for this patient was however included in the analysis as they were successfully gathered for the neurofeedback training, as well as the post-testing and the first follow-up measurement.
Table 1 shows the demographic and clinical details of the 48 participants included in the final analysis. Participants of the final sample were between 24 and 75 years old with a mean age of 46.83 (SD = 12.8). The sample consisted of 38 males and 10 females.
Table 1
Demographic, Health, and Tinnitus Characteristics of Study Sample
Mean | SDa | Median | Min | Max | |
Age (years) | |||||
NTNF | 46.29 | 12.22 | 44 | 24 | 71 |
TONF | 47.38 | 13.61 | 50.5 | 25 | 75 |
Tinnitus Duration (months) | |||||
NTNF | 78.92 | 74.63 | 40 | 18 | 312 |
TONF | 148.04 | 159.36 | 114 | v8 | 720 |
Age of Onset (years) | |||||
NTNF | 39.75 | 14.66 | 39 | 14 | 67 |
TONF | 35.17 | 13.65 | 36 | 7 | 55 |
Mean Hearing Loss (dB) | |||||
NTNF | 7.54 | 8.25 | 4.4 | 0 | 22.8 |
TONF | 7.32 | 8.80 | 4.05 | 0 | 34.4 |
Tinnitus Loudness (0–100)b | |||||
NTNF | 53.25 | 19.57 | 50 | 20 | 95 |
TONF | 54.83 | 30.16 | 57.5 | 8 | 100 |
Tinnitus Distress (THI)c | |||||
NTNF | 29.33 | 14.70 | 27 | 4 | 56 |
TONF | 35.83 | 18.79 | 31 | 14 | 84 |
Tinnitus Distress (TQ)c | |||||
NTNF | 23.75 | 11.63 | 23 | 6 | 45 |
TONF | 28.92 | 15.97 | 31 | 7 | 74 |
Depression (BDI)d | |||||
NTNF | 6.29 | 4.34 | 7 | 0 | 13 |
TONF | 5.38 | 4.17 | 4 | 0 | 15 |
Anxiety (BAI)d | |||||
NTNF | 7.12 | 5.77 | 6.5 | 0 | 21 |
TONF | 5.25 | 3.35 | 4 | 1 | 14 |
Note. aSD = Standard Deviation. bSubjective tinnitus loudness rated on a scale from 0 to 100. cSum-scales indicating tinnitus-related distress (THI: 0–100, TQ: 0–84). dSum-scales indicating severity of depressive/anxiety symptoms (0–63).
Thirty-five participants described their tinnitus percept as tonal, three as noise-like, and ten indicated an undefinable type. Most participants (n = 39) perceived tinnitus in both ears, however 16 subjects of this group indicated a left- while 11 specified a right-sided tendency. Of the remaining subjects, four indicated a clear left lateralization, three a right lateralized tinnitus, and two experienced it diffusely inside their heads.
Mean tinnitus loudness was rated as 54.04 (SD = 25.17), while mean distress measured with the THI consisted of 32.58 points (SD = 17.01), and of 26.33 points (SD = 14.06) points measured with the TQ. These values suggested “mild tinnitus” according to the THI and “slight tinnitus” according to the TQ for the overall group on average.
Participants were randomly assigned to one of two study groups that followed identical study procedures (described in detail in section 2.2) with the only difference being the tomographic (TONF) or non-tomographic (NTNF) neurofeedback application (details in section 2.4). The groups were matched according to age, gender, and hearing loss, and were of equal size (n = 24). Participants were not informed of their group assignation, and this project is thus considered a single-blind randomized controlled trial. The study was approved by the appropriate Ethics Committee (Kantonale Ethikkommission Project KEK-ZH-Nr. 2014-0594) and was registered online at ClinicalTrials.gov (NCT02383147) and kofam.ch (SNCTP000001313).
2.2Procedure
Prior to the start of the neurofeedback training period, participants gave their informed consent, underwent audiometric screening, and participated in the baseline measurements (t1) of the study. This screening assessment consisted of resting-state EEG measurements (details in section 2.3) and the completion of a set of questionnaires covering demographics, tinnitus-related symptoms, as well as other psychological and health-related questions. The forms for this subjective measurement were chosen according to the guidelines of the Tinnitus Research Initiative (TRI) (Landgrebe et al., 2012; Langguth et al., 2007). In particular, an adjusted version of the Tinnitus Sample Case History Questionnaire (TSCHQ) was used to obtain information about demographics, tinnitus properties, prior treatment attempts, and other tinnitus-related issues. Participants evaluated their tinnitus distress using the German version of the Tinnitus Handicap Inventory (THI) (German version by Kleinjung, Fischer, et al., 2007) and the Tinnitus Questionnaire (TQ) (German version by Goebel & Hiller, 1994). For these two forms, sum-scores range between 0–100 in the former and 0–84 in the latter case. Additionally, participants completed German versions of Beck’s Depression Inventory (BDI) (Hautzinger, Bailer, Worall, & Keller, 1995), Beck’s Anxiety Inventory (BAI) (Prinz & Petermann, 2015), the short form of the WHO Quality of Life scale (WHOQOL-BREF) (Angermeyer, Kilian, & Matschinger, 2000), Symptom Check List (SCL-K-9) (Klaghofer & Brähler, 2001), and Short Form Health Questionnaire (SF-36) (Bullinger, Kirchberger, & Ware, 1995). The main behavioral outcome measures of this study were defined as self-reported tinnitus loudness (rated from 1 “very low” to 100 “very high”) and sum-scores of THI and TQ.
After the screening, participants were randomly assigned to one of two study groups (TONF vs. NTNF) and participated in 15 weekly neurofeedback training sessions (details in section 2.4). Post-measurements (t2) were performed immediately after the completion of the training period by repeating resting-state EEG measurements as well as questionnaire surveys. Two follow-up measurements were conducted 3 and 6 months after the training period. While questionnaires were completed at both follow-up time points, resting-state EEG was only recorded at the first (t3). Participants completed the final survey for the last follow-up measurement (t4) at home after all preceding measurements had been conducted at the Department of Otorhinolaryngology and the University of Zurich.
2.3EEG recording
For recording of resting-state EEG at t1, t2, and t3, we used a BrainAmp DC amplifier system in combination with active channel actiCap electrode caps (Brain Products, Munich, Germany). The 64 silver/silver chloride electrodes were arranged according to the 5/10 electrode position system (Oostenveld & Praamstra, 2001). FCz electrode served as reference for the recording while the ground electrode was placed at AFz.
Data was sampled with a sampling rate of 1000 Hz. Furthermore, direct current (DC) mode with a high-cutoff filter of 1000 Hz and a slope of 12 dB/octave was used for recording. Electrodes were prepared with conductive paste prior to recording in order to keep impedance below 10 kΩ. The measurement was performed in a sound-proof and electromagnetically shielded room, and patients were given minimal instructions: to sit upright and to avoid excessive movements in order to minimize potential artifacts.
Resting-state EEG was then recorded over a time span of 8 minutes during which subjects were asked to open (EO) and close (EC) their eyes in regular intervals. For playback of these instructions, Presentation software (Neurobehavioral Systems, Inc., 2010) was used, and a fixation cross was presented during eyes-open segments. After the first measurement, patients were given some time to relax and were subsequently informed about the second part of EEG recordings. This time, participants were explicitly instructed to allow their tinnitus to come naturally during the 8 minutes of measurement and to try not to suppress it. This clear instruction given before the second resting-state measurement (as opposed to the minimal before the first) has been shown to make the measurement situation more comparable with the neurofeedback situation because a patient’s tinnitus is usually more salient during neurofeedback treatment (Güntensperger et al., 2019). Therefore the resting-state activity of eyes-open segments of this second recording was chosen as main electrophysiological outcome measure.
2.4Neurofeedback training
Thirty-one silver/silver chloride electrodes according to the 10/20 system and a NeuroAmp amplifier (BEE Medic GmbH, Singen, Germany) were used for acquiring neurofeedback EEG. The signal was referenced to electrodes placed on the earlobes of subjects, and ground electrode was placed at AFz. The sampling rate of the recording was set at 500 Hz, and impedance was kept below 20 kΩ. The EEG signal was processed in real-time using the software Cygnet 2.0.3.34 (BEE Medic GmbH, Singen, Germany). While only activity measured at four fronto-central electrodes (FC1, FC2, F3, and F4) was used for feedback generation of the NTNF group, the signal of all 31 electrodes was considered for the TONF group. In the latter case, the implemented sLORETA algorithm estimated the signal at four sources over the primary auditory cortex (55/–25/10 and 55/–30/10 for right Heschl’s gyri, –55/–25/10 and –55/–30/10 for left Heschl’s gyri). Only activity over these sources was set as relevant for the neurofeedback of the TONF group. It is important to note that, even though only four electrodes were feedback-relevant for participants of the NTNF group, all 31 electrodes were equally placed and prepared for these participants in order to disguise group affiliation. Visual feedback was implemented in the computer simulation Inner Tube (Somatic Vision, Encinitas, CA, USA). Patients observed a space ship, the speed, navigation accuracy and visibility of which changed according to their brain activity.
While an increase in alpha power was rewarded with acceleration of the ship, an increase in delta deteriorated autopilot accuracy and visibility of the simulation. The rewarded alpha frequency band was set in the range of±2 Hz around an individual alpha peak determined for each participant, and the inhibited delta band was set in the range of 3–4 Hz. During the feedback, the Cygnet software allowed for automatic filtering and excluded movement artifacts and system voltage (45–55 Hz) from feedback. Each session lasted for 15 minutes and was repeated once a week.
2.5EEG data analysis
Brain Vision Analyzer 2 (Brain Products, Munich, Germany) was used for preprocessing the EEG data. Band-pass filters with Butterworth zero-phase filters between 0.1 Hz and 80 Hz with slopes of 24 dB/octave at the low, and 48 dB/octave at the high cutoffs were first applied to the data. A band-rejection filter with a central frequency of 50 Hz, a bandwidth of 1 Hz, and a slope of 24 dB/octave was used in order to eliminate possible line noise. Next, very noisy or dead channels were identified by visual inspection and temporarily excluded from the data. An independent component analysis (ICA) with a restricted Infomax algorithm was performed and, in doing so, the EEG signal decomposed into its independent components. The resulting components were inspected to identify if they were indicative of eye-blinks, pulse, or other regular artifacts. Obvious cases were subsequently removed from the data with the inverse ICA procedure. After this step, previously excluded channels and electrodes with high amounts of remaining noise were replaced by using spline-type topographical interpolations. As a last step, data were carefully visually inspected and any remaining irregular artifacts (e.g., muscle movements) removed. Before segmenting data into eyes-closed and eyes-open conditions, an average reference over all channels was applied. The implicit reference of data recording (FCz) was re-included into the data and used for subsequent analysis.
In the next step, data were imported into MATLAB Statistics Toolbox 2017a (The MathWorks Inc., Natick, Massachusetts, United States) and EEGLAB 14.1.1b (Delorme & Makeig, 2004) for surface-based analysis. A hamming window with 2 s window length and 1s overlap was first applied on the data of eyes-open segments. Next, Fast Fourier Transforms (FFT) for each 2 s-segment were computed, logarithmized and averaged over all segments for each patient resulting in power values in decibels (dB) with a frequency resolution of 0.5 Hz. Subsequently, power values in the rewarded (individual) alpha range were divided by those in the inhibited (3–4 Hz) delta range. The resulting alpha/delta ratio was then averaged over the four electrodes (FC1, FC2, F3, F4) used for training of the NTNF group.
Analysis on source level was performed using the LORETA toolbox (version 20150810). Technical details of implemented power analysis and source estimation are provided in Pascual-Marqui (2002). The lead field behind the sLORETA algorithm is described in Fuchs, Kastner, Wagner, Hawes, and Ebersole (2002) and the integrated electrode position system in Jurcak, Tsuzuki, and Dan (2007). Standard electrode positions were registered with the integrated tool and the transformation matrix was regularized according to an estimated signal to noise ratio of 100. The analysis of log-transformed sLORETA data in the frequency domain resulted in mean current density values (mA/mm2) that were exported and averaged over the four voxels used for neurofeedback training of the TONF group (see section 2.4).
2.6Statistics
Data analysis was performed using the statistical environment R (R Core Team, 2017) including packages “ggplot2” (Wickham, 2009), “ggsignif” (Ahlmann-Eltze, 2017), “Hmisc” (Harrell Jr, 2017), “jtools” (Long, 2017), “multcomp” (Hothorn, Bretz, & Westfall, 2008), “nlme” (Pinheiro, Bates, DebRoy, Sarkar, & R Core Team, 2017), and “xtable” (Dahl, 2016). Time effects for behavioral (THI and TQ sum-scores, tinnitus loudness) and EEG-related data were estimated by means of repeated-measures mixed model analyses of variance (ANOVA). Using the “nlme” package in R, the model was specified as lme(X∼time, random=∼1|subject, data, method=“ML”, ”na.action=na.exclude) and compared to a baseline model using only the intercept as predictor lme(X∼1, random=∼1|subject,data,method=“ML”,na.action=na.exclude).
Additionally, a model with group-term (group + time) was calculated to test for group effects within time points: lme(X∼time + group, random = ∼1|subject, data, method=“ML”, na.action=na.exclude). An additional model with group*time interaction was fitted on the data in order to reveal potential differences between the TONF and NTNF group between time points. This model was specified as: lme(X ∼time*group, random =∼1|subject, data, method=“ML”, na.action=na.exclude). In order to evaluate training success and stability of potential changes, a priori defined contrasts comparing t1 with all other time points (t2, t3 and t4 for behavioral, t2 and t3 for EEG data) were analyzed for all these models using Bonferroni corrected one-tailed t-tests. Furthermore, effect sizes r for these analyses were calculated. According to Field, Miles, and Field (2012), we converted them directly from respective t-values and labeled r = 0.1 a small, r = 0.3 a medium, and r = 0.5 a large effect (Cohen, 1988). Finally, in order to reveal any other potential differences between time points, post-hoc Tukey tests were performed comparing each of the four time points with each other. The alpha threshold was set at p = .05 for all statistical tests.
3Results
3.1Behavioral results
Results concerning changes in tinnitus-related symptoms of both neurofeedback groups (TONF: group with tomographic neurofeedback, NTNF: group with surface-based neurofeedback) are summarized in Fig. 1 and in Table 2. As described in section 2.6, three repeated-measures ANOVA mixed models with the factor time were calculated and compared to the baseline model (model without factor time): (a) a model without group-term (data is not dependent on neurofeedback group); (b) a model with group-term but no interaction (data within but not between time points is dependent on neurofeedback group); and (c) a model with group-term and group*time interaction (data within and between time points is dependent on neurofeedback group).
Fig. 1
Plots showing tinnitus-related symptoms for the two neurofeedback groups (dark: tomographic neurofeedback, gray: surface-based neurofeedback) before (t1), 1 week after (t2), 3 months after (t3), and 6 months after (t4) training. Tinnitus Handicap Inventory (THI) and Tinnitus Questionnaire (TQ) were analyzed as measures for tinnitus-related distress, and complemented with subjective tinnitus loudness (0–100). Error bars represent±1 standard error for within-subjects designs according to Morey (2008).
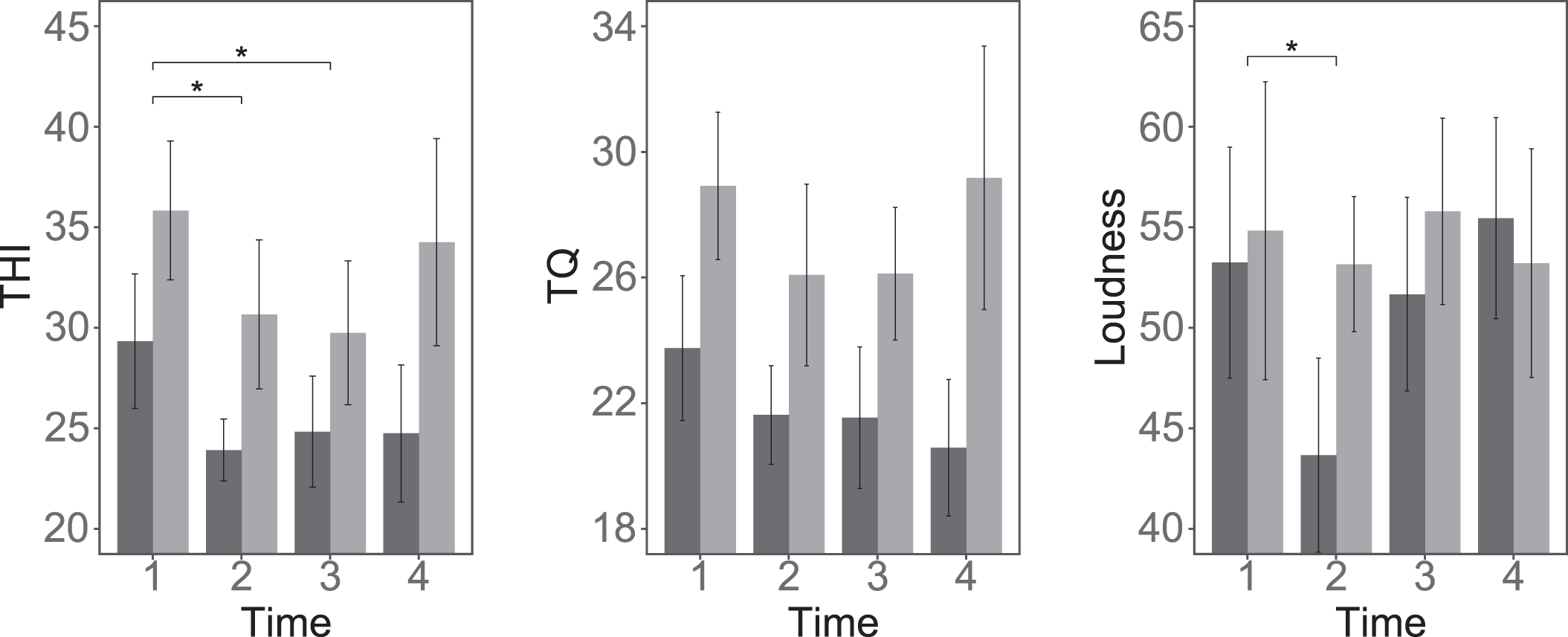
Table 2
Primary Outcome Variables
T1 | T2 | T3 | T4 | |
THI | ||||
NTNF | 29.33 (14.70) | 23.92 (12.71) | 24.83 (12.48) | 24.75 (16.48) |
TONF | 35.83 (18.79) | 30.67 (17.37) | 29.75 (22.78) | 34.26 (23.87) |
TQ | ||||
NTNF | 23.75 (11.63) | 21.62 (12.03) | 21.54 (11.18) | 20.58 (12.81) |
TONF | 28.92 (15.97) | 26.08 (15.98) | 26.12 (18.10) | 29.17 (19.91) |
Loudness | ||||
NTNF | 53.25 (19.57) | 43.67 (22.42) | 51.67 (22.00) | 55.46 (17.28) |
TONF | 54.83 (30.16) | 53.17 (27.95) | 55.79 (28.43) | 53.22 (31.53) |
EEG Source Ratio | ||||
NTNF | 1.262 (0.216) | 1.346 (0.223) | 1.377 (0.270) | |
TONF | 1.303 (0.183) | 1.370 (0.158) | 1.374 (0.193) | |
EEG Surface Ratio | ||||
NTNF | 0.961 (0.042) | 0.978 (0.044) | 0.968 (0.041) | |
TONF | 0.950 (0.051) | 0.963 (0.055) | 0.966 (0.060) |
Note. Values are M (SD). T1 = baseline. T2 = after neurofeedback. T3 = 3-month follow-up. T4 = 6-month follow-up. THI = Tinnitus Handicap Inventory. TQ = Tinnitus Questionnaire.
For the tinnitus questionnaire THI and for tinnitus loudness, the model without group-term showed the best fit on the data. This suggests a significant effect of the factor time on tinnitus distress and tinnitus loudness but no effect of group affiliation.
In the following paragraphs, results of a priori defined contrasts of these significant models only will thus be reported. For TQ, none of the models showed an improved fit compared to the baseline model suggesting no significant time effects in this case.
3.1.1THI
The results of the repeated-measures mixed model ANOVA are summarized in Table 3. The analysis suggested a significant effect of the factor time on tinnitus distress, χ2(3) = 13.11, p = .004. The model with the factor group+time, as well as the model with the factor group*time showed no improved fit on the data. A priori defined contrasts of the best fitting model showed a significant decrease between t1 (M = 32.58, SD = 17.01) and t2 (M = 27.29, SD = 15.44), t(140) = –3.17, p = .003 (one-tailed). This decrease was persistent up to 3 months after completion of training since a significant difference between t1 and t3 (M = 27.29, SD = 18.34) was found, t(140) = –3.17, p = .003 (one-tailed). However, the difference between t1 and t4, 6 months after the training, was not significant. Post-hoc Tukey tests corroborated the significant results and revealed no further significant differences. Effect sizes were r = 0.26 for t1–t2, r = 0.26 for t1–t3, and r = 0.17 for t1–t4.
Table 3
Results of the Repeated-Measures Mixed Model ANOVA and A Priori Defined Contrasts
χ2 | t | df | p | |
THI | ||||
ANOVA | ||||
time | 13.11** | 3 | 0.004 | |
time + group | 2.11 | 1 | 0.146 | |
time*group | 1.54 | 3 | 0.672 | |
Contrasts | ||||
t1–t2 | –3.17** | 140 | 0.003 | |
t1–t3 | –3.17** | 140 | 0.003 | |
t1–t4 | –2.01 | 140 | 0.070 | |
TQ | ||||
ANOVA | ||||
time | 5.42 | 3 | 0.143 | |
time + group | 2.03 | 1 | 0.154 | |
time*group | 3.92 | 3 | 0.270 | |
Contrasts | ||||
t1–t2 | –2.00 | 140 | 0.071 | |
t1–t3 | –2.02 | 140 | 0.069 | |
t1–t4 | –1.17 | 140 | 0.367 | |
Loudness | ||||
ANOVA | ||||
time | 7.92* | 3 | 0.048 | |
time + group | 0.30 | 1 | 0.583 | |
time*group | 4.74 | 3 | 0.192 | |
Contrasts | ||||
t1–t2 | –2.19* | 140 | 0.045 | |
t1–t3 | –0.12 | 140 | 1.355 | |
t1–t4 | 0.37 | 140 | 1.070 | |
EEG Source Ratio | ||||
ANOVA | ||||
time | 10.88** | 2 | 0.004 | |
time + group | 0.17 | 1 | 0.677 | |
time*group | 0.58 | 2 | 0.747 | |
Contrasts | ||||
t1–t2 | 2.58* | 94 | 0.012 | |
t1–t3 | 3.15** | 94 | 0.002 | |
EEG Surface Ratio | ||||
ANOVA | ||||
time | 14.36** | 2 | 0.001 | |
time + group | 0.52 | 1 | 0.469 | |
time*group | 2.62 | 2 | 0.269 | |
Contrasts | ||||
t1–t2 | 3.73*** | 94 | 0.000 | |
t1–t3 | 2.85** | 94 | 0.005 |
Note. A priori defined contrast are indicated for the ANOVA model with the factor time. P-values of contrast analysis are Bonferroni corrected and one-tailed. ***p < .001; **p < .01; *p < .05.
3.1.2TQ
The results of the repeated-measures mixed model ANOVA suggested no significant effects of for the factors time nor group on tinnitus distress measured with TQ (see Table 3). As expected based on these results, a priori defined contrasts of the time model suggested no significant changes of TQ sum-scores (p > .05). For the sake of completeness, however, differences between t1 (M = 26.33, SD = 14.06) and t2 (M = 23.85, SD = 14.17), t(140) = –2, p = .071 (one-tailed), as well as between t1 and t3 (M = 23.83, SD = 15.06), t(140) = –2.02, p = .069 (one-tailed) and the respective effect sizes of r = 0.17 for t1–t2 and r = 0.17 for t1–t3 are reported here. According to Cohen (1988), these values suggest a small effect. Post-hoc Tukey tests revealed no further significant differences.
3.1.3Loudness
In the case of tinnitus loudness, the repeated-measures mixed model ANOVA with the factor time and without the factor group represented the data best (see Table 3). A priori defined contrasts of this model showed a significant decrease between t1 (M = 54.04, SD = 25.17) and t2 (M = 48.42, SD = 25.52), t(140) = –2.19, p = .045 (one-tailed). The effect size of this contrast was r = 0.18. This decrease, however, was not persistent since no significant difference between t1 and t3 (M = 53.73, SD = 25.23), t(140) = –0.12, p = 1.355 (one-tailed) nor between t1 and t4 (M = 54.36, SD = 25.02), t(140) = 0.37, p = 1.070 (one-tailed) was found. Furthermore, the Tukey post-hoc test revealed a significant increase between t2 and t4 (p = .049) suggesting a return of the rated tinnitus loudness to baseline values 6 months after the training.
As can be seen in Fig. 1, there was a visible difference between the two groups regarding the training effect from t1 to t2. This prompted further explorative analysis. The results of these analyses showed that, while the difference between t1 and t2 was significant for the NTNF group: t1 (M = 53.25, SD = 19.57), t2 (M = 43.67, SD = 22.42), t(69) = –2.74, p = .012 (one-tailed), for the TONF group it was not: t1 (M = 54.83, SD = 30.16), t2 (M = 53.17, SD = 27.95), t(68) = –0.45, p = .984 (one-tailed).
This difference between groups should not be labelled statistically meaningful, however, as contrasts of the group*time interaction model indicate non-significance for the interaction, t(137) = 1.55, p = .123.
3.2EEG results
Data of resting-state EEG measurements for the two neurofeedback groups are summarized in Fig. 2 and Table 2. Repeated-measures mixed model ANOVA were performed for the trained alpha/delta ratio on both surface and source level data.
Fig. 2
Plots showing alpha-delta ratio for the two neurofeedback groups (dark: tomo- graphic neurofeedback, gray: surface-based neurofeedback) before (t1), 1 week after (t2), and 3 months after (t3) training. EEG data was analyzed on source level (over the four voxels used for neurofeedback of the ToNF group) and on surface level (over the four electrodes used for neurofeedback of the NTNF group). Error bars represent±1 standard error for within-subjects designs according to Morey (2008).
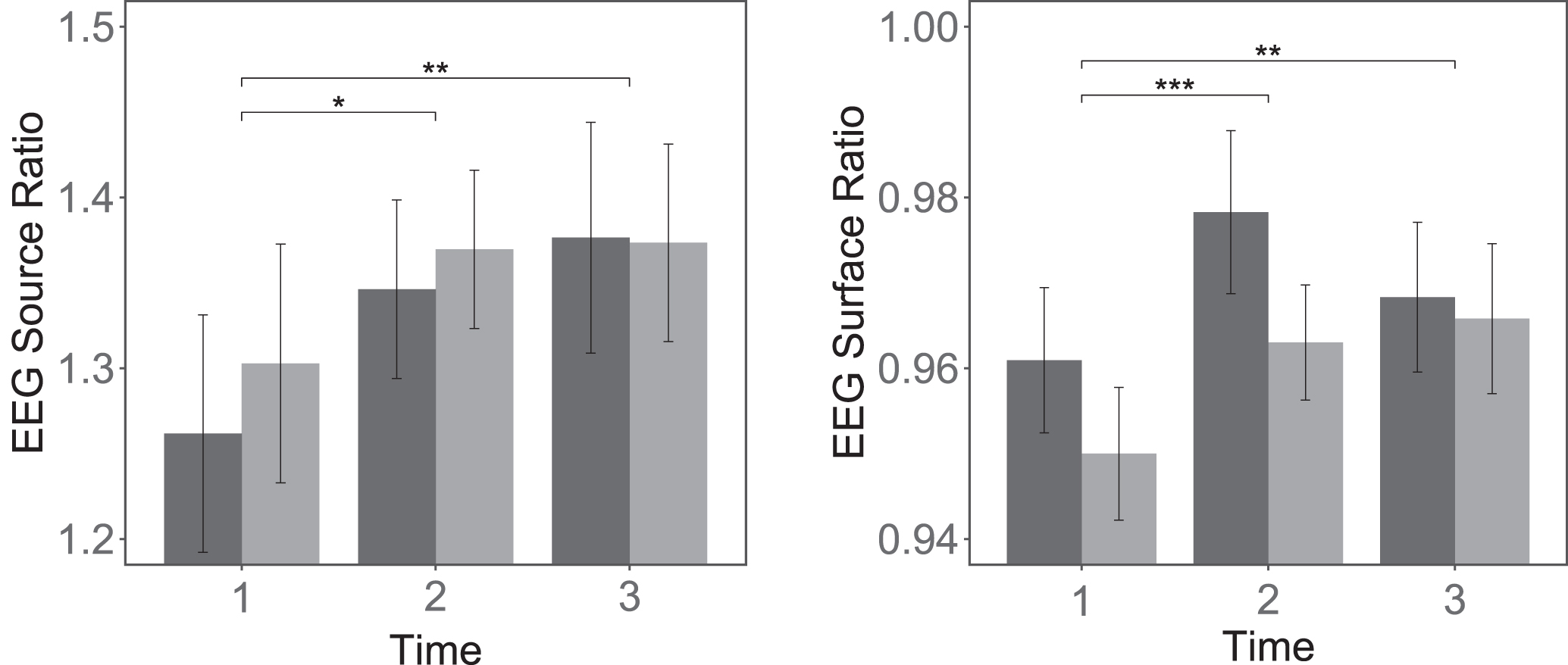
Results of the analyses indicate the best fit on the data for the model without group-term, suggesting significant effects of the factor time but no effect of group affiliation. In the following paragraphs, results of a priori defined contrasts will thus be reported for the model without group-term.
3.2.1Source level
The results of the repeated-measures mixed model ANOVA suggested a significant effect of the factor time on EEG source data, χ2(2) = 10.88, p = .004. Both other models did not show an improved fit on the data suggesting no influence of group affiliation (see Table 3). A priori defined contrasts of the model with the best fit showed a significant increase of the trained alpha/delta ratio between t1 (M = 1.2824, SD = 0.1988) and t2 (M = 1.358, SD = 0.1916), t(94) = 2.58, p = .012 (one-tailed). Moreover, alpha/delta ratio was found to be increased on a stable basis as a significant difference between t1 and t3 (M = 1.375, SD = 0.2319), t(94) = 3.15, p = .002 (one-tailed) was found. Effect sizes were r = 0.26 for t1–t2, and r = 0.31 for t1–t3, and effects are thus considered small to medium. Post-hoc Tukey tests supported the significant results and revealed no further significant differences.
3.2.2Surface level
Training effects of alpha/delta ratio over the four EEG electrodes used for the neurofeedback of the NTNF group showed a significant effect of the factor time, χ2(2) = 14.36, p < .001. Also in the case of surface data, the two models taking group affiliation into account did not show an improved fit on the data (see Table 3). A priori defined contrasts showed a significant ratio-increase between t1 (M = 0.9555, SD = 0.0466) and t2 (M = 0.9707, SD = 0.0499), t(94) = 3.73, p < .001 (one-tailed). Effect size of this finding was indicated at r = 0.36 suggesting a medium effect. Also in this case, the effect was not transient but persistent as the significant contrast between t1 and t3 (M = 0.9671, SD = 0.051) suggests, t(94) = 2.85, p = .005 (one-tailed). Effect size in this case was r = 0.28. Post-hoc Tukey tests corroborated these results and revealed no further significant differences.
4Discussion
In this project a tomographic alpha/delta neurofeedback protocol based on sLORETA source estimation was used in the treatment of chronic tinnitus for the first time. The two groups of tinnitus patients included in this study followed the training and pre-post test routine in exactly the same way with the single difference being the calculation of their feedback-relevant EEG activity. The neurofeedback protocol was identical for both study groups and aimed at alpha-up, delta-down training with a reward frequency adjusted to each individual’s alpha peak. However, for the NTNF group, the feedback was calculated on the basis of four active electrodes on the scalp, thus following previous neurofeedback treatment attempts of chronic tinnitus (Crocetti et al., 2011; Dohrmann et al., 2007; Güntensperger et al., 2019). In contrast, the signal of 31 EEG electrodes was considered for feedback generation of the TONF group. An implemented sLORETA algorithm estimated brain activity in four voxels over the auditory cortex and filtered EEG data such that only activity generated in these sources was used for feedback. In the following, the results of data analysis concerning the primary outcome measures are reviewed and critically discussed.
4.1Tinnitus-related distress
Results of the trial suggest that tinnitus patients of both groups benefited from the neurofeedback intervention (see Table 3 and Fig. 1). Tinnitus-related distress measured with the THI decreased over the course of the training and remained stable on a lower level up to 3 months after training completion (see section 3.1.1). Even though 6 months after the training no significant difference was found compared to baseline measures, Tukey post-hoc tests suggested no statistically meaningful increase in the follow-up period. Furthermore, tinnitus-related distress measured with TQ was on a lower level after the training compared to baseline. In this case, neither the results of the main ANOVA model nor contrast analysis of the four time points revealed significant differences, but data did suggest a statistical trend (see section 3.1.2).
On average, the study group was able to reduce THI scores by –5.29 and TQ scores by –2.48 points. But are these differences, statistically significant or not, also clinically relevant? As simple as this question may sound, there is still no clear consensus in the tinnitus literature about which size of difference should be termed clinically meaningful for patients. Regarding THI, recommended changes range from 6 points (Zeman et al., 2011) up to 20 points (Newman, Sandridge, & Jacobson, 1998). For TQ, the work by Kleinjung, Steffens, et al. (2007) indicated a minimal difference of 5 points to be clinically relevant but Hall, Mehta, and Argstatter (2018) recently suggested a higher cutoff of 12 points. Whichever of these we adopt, it seems that tinnitus-related distress of patients in our study on average did not change in a clinically meaningful way. It should be mentioned, however, that neither of these questionnaires were initially developed to account for measuring longitudinal treatment-related changes in distress. While the THI more generally measures impact of tinnitus on everyday function, TQ was designed to assess various psychological aspects of tinnitus (e.g., including impact on auditory perception), and both thus only partly cover tinnitus-related distress.
Categorizing the questions of these two questionnaires, Kennedy, Wilson, and Stephens (2004) showed that only 68% of questions concern psychological or emotional tinnitus distress. In the case of TQ, only 47% of questions serve this purpose which might explain the higher amount of inter-individual variance (see Fig. 1) and, thus, the non-significant results. The Tinnitus Functional Index (TFI), which has been specifically developed as a treatment-responsive measure (Meikle et al., 2012) and recently validated (Peter et al., 2017), might have been more suitable to cover treatment-related changes in this context.
Figure 1 illustrates the time course of tinnitus symptoms for the two neurofeedback groups. Even though results of the two groups seem to differ within and across time, repeated-measures mixed models considering group affiliation of the patients as a factor in the model did not show an improved fit on the data. This suggests that the neurofeedback treatment had roughly the same effect on changes in tinnitus-related distress regardless of whether the feedback was applied on the basis of only four active electrodes (NTNF) or was acquired with 31 electrodes and source estimation (TONF). The question that arises in this context is why the neurofeedback protocol designed to evoke spatially specific effects over auditory brain areas—that is, regions were the maladaptive processes leading to tinnitus have been proposed to originate from (De Ridder, Vanneste, Langguth, & Llinás, 2015; Llinás et al., 1999; Weisz, Dohrmann, & Elbert, 2007)—does not show different effects when compared to the unspecific NTNF protocol. Possible reasons for the absence of this between-group difference might be found in the high inter-individual variability of tinnitus manifestation. Recent research in the tinnitus field suggests that tinnitus should not be considered a homogeneous phenomenon that sounds and feels the same for all sufferers (e.g., Landgrebe et al., 2010; Langguth, Kreuzer, Kleinjung, & De Ridder, 2013; van den Berge et al., 2017). Rather, it has consistently been shown that many aspects of the tinnitus percept vary greatly across individuals according to their specific tinnitus history. Current brain models regarding tinnitus emergence and manifestation take this view into account (e.g., De Ridder et al., 2014; Sedley, Friston, Gander, Kumar, & Griffiths, 2016). Most of these models are distanced from the idea that tinnitus emerges exclusively in auditory areas but rather consider it to be coded in various sub-networks distributed across the whole brain. This holds in particular for individuals with a long history of tinnitus symptoms. In this context, a distress network has been proposed that includes structures of the limbic system (e.g., anterior cingulate cortex and amygdala), prefrontal areas (e.g., dorsolateral prefrontal cortex), and also the insula (De Ridder et al., 2014). Furthermore, Rauschecker, Leaver, and Mühlau (2010) found that the limbic system, in particular amygdala, nucleus accumbens, and ventromedial prefrontal cortex (vmPFC), are part of an inhibitory frontostriatal gating mechanism. They found that these structures via the thalamic reticular nuclei, are part of a noise-cancelling feedback loop, which is able to protect the auditory central system from any unpleasant sounds under normal conditions (Rauschecker, May, Maudoux, & Ploner, 2015). Husain (2015) were able to confirm the involvement of limbic structures and found that the lack of habituation to the constant tinnitus tone might be explained by a suppressed response from amygdala or insula when conveying emotions. An even more comprehensive framework has recently been proposed by Sedley et al. (2016) on the basis of predictive coding. According to this group higher brain areas are responsible for decreasing top-down prediction errors and for resetting the default prediction of the auditory system to expect tinnitus.
Following these considerations, it is not surprising that neurofeedback training aimed at influencing activity in auditory areas exclusively does not always lead to additional benefits regarding tinnitus-related distress. Based on this indication, future tomographic neurofeedback treatments should target the large scale, whole-brain networks involved in tinnitus generation and maintenance instead of confining the training to auditory areas. For instance, previous EEG studies have shown that low theta and a high amount of beta oscillations in insula and anterior cingulate cortex might be linked to tinnitus (e.g., De Ridder, Congedo, & Vanneste, 2015; Meyer et al., 2017). Based on these findings, it may be beneficial to extend the auditory alpha/delta protocol with theta-up/beta-down training in insula and ACC.
4.2Tinnitus loudness
Data analysis revealed that, across the whole study group, neurofeedback led to a significant decrease in tinnitus loudness. However, this decrease was followed by an obvious (see Fig. 1) and, according to the Tukey post-hoc test, statistically meaningful return to baseline levels in the follow-up period. A possible explanation for this issue might be that the number of 15 training sessions was not high enough to affect the loudness of the percept in a sustainable way. However, two studies working with the same protocol (Crocetti et al., 2011; Dohrmann et al., 2007) both reported a stable decrease of tinnitus intensity with only 10 and 12 sessions, respectively. Another reason for this inconsistency might be the length of individual neurofeedback sessions which was 15 minutes in our study and 30 or 20 minutes in the aforementioned studies. Furthermore, patients in our project trained only once a week while the frequency was higher (2–3 times per week) in the previous studies. Therefore, higher intensity (in frequency or length) of neurofeedback training might be considered a crucial factor for longer-lasting effects regarding tinnitus loudness.
Also in the case of tinnitus loudness, the repeated-measures mixed model designs with group-terms did not show improved fits on the data, suggesting no between-group differences within or across time (see Table 3). This effect could not be shown despite the noticeable difference between groups in Fig. 1 and the exploratory analysis revealing significant effects for NTNF but not for TONF (see section 3.1.3). Therefore, the logical conclusion is that both groups reacted in a similar way regarding their tinnitus loudness (i.e., a decrease after the training, and an increase in the follow-up phase). As in the previous case with tinnitus distress, it should be noted that tinnitus sub-networks also need to be taken into account for tinnitus intensity. Regarding the perception (experience) of the tinnitus sound, De Ridder, Elgoyhen, Romo, and Langguth (2011) have suggested that activation in auditory areas alone is insufficient. For conscious perception to arise, activity in sensory areas must be connected to networks composed of regions in the cingulate, parietal, frontal and most importantly the insular cortex that code the importance and salience of percepts (see also Meyer et al., 2016). Sensory stimuli are only consciously perceived after they have been classified as relevant and important by means of this sub-network. Since the recruitment of additional brain circuits is thus necessary for initial perception, also the intensity of a percept (in this case tinnitus loudness) is dependent on this co-activation. Neurofeedback focused specifically on auditory areas ignores these networks, a fact that should be considered in future studies.
4.3Electrophysiological parameters
An analysis of EEG-related parameters was performed in order to have proof of concept that the neurofeedback intervention presented here indeed leads to the proposed effects in the participants’ brain activity. Source data over the four voxels used for TONF and alpha/delta ratio over the four active electrodes of the NTNF group suggested that learning of the proposed EEG rhythm occurred in both groups. Repeated-measures mixed model ANOVA performed over both groups combined revealed significant effects of the factor time and, furthermore, significant ratio increases between pre and post measurements. In addition, these effects were confirmed 3 months after completion of the training period, suggesting a persistent change in resting-state brain activity.
When a group-term was included in the multilevel model, the fit was not improved (see Table 3). This suggests no meaningful differences between electrophysiological changes of the TONF and the NTNF group on both, surface and source levels. This contradicted our hypotheses as we expected surface-related changes to be more distinct for the NTNF group and changes on source level to be predominant in the TONF group. It is important to keep in mind, however, that both neurofeedback protocols were developed in order to target activity over auditory areas. The four fronto-central electrodes (FC1, FC2, F3, F4) of the NTNF group were chosen because of their high probability to reflect activity in auditory brain regions according to Pantev et al. (1995, 1) (as cited in Dohrmann et al., 2007). Hence it is reasonable to assume that the high validity of these electrodes for representing the auditory cortex might explain the unexpected training-induced alterations on source level for members of the NTNF group. This might serve as a possible explanation as to why electrophysiological parameters changed equally on surface and source levels for participants of both tomographic and non-tomographic neurofeedback groups.
4.4Limitations
One major limitation of the study design is the lack of a sham control group due to restrictions of time, funding, ethical reasons and other arguments discussed previously (Güntensperger et al., 2017). The risk of unspecific effects is particularly high in the context of chronic tinnitus where many participants hopefully turn to any new treatment approach after unsuccessfully trying a wide variety of therapies on their own. Expectations of researcher and participant and the effect of simply participating in a study may thus serve as alternate explanations for the reductions in THI and TQ scores. Kreuzer et al. (2012) for instance found around a 5 point decrease of TQ values and a 6 point THI reduction in a mere waiting-list control group. However, apart from a sole evaluation of subjective tinnitus symptoms, we focused our analysis extensively on not voluntarily modifiable neurophysiological data recorded with resting-state EEG. As has been extensively discussed in our recent publication (Güntensperger et al., 2019), this data at least partially speaks against a purely unspecific explanation. However, without a sham control group it is not possible to properly distinguish specific and unspecific effects of the treatment and, therefore, to draw final conclusions about the effectiveness of this neurofeedback protocol. In this context, a recently published “Trials” article may soon provide novel insights (Jensen et al., 2020). The author outline a randomized controlled trial comparing an alpha/delta neurofeedback group with an active beta/theta training control group and a passive diary control group. The comparison of the results of these three groups may lead to a better understanding of specific and non-specific effects in neurofeedback treatment for tinnitus.
4.5Conclusion
Overall, alpha/delta neurofeedback seems to be a valid treatment addition for chronic tinnitus. Surface-based as well as tomographic neurofeedback both lead to sustainable effects on tinnitus-related distress. Effects on tinnitus loudness are transient but might be prolonged with higher training frequency (2–3 sessions per week) and longer individual training sessions (min. 20 minutes). It should be noted, however, that the higher specificity of tomographic neurofeedback might be disadvantageous in some cases as it exclusively focuses on auditory areas and ignores other tinnitus-relevant areas (i.e., areas of the distress and/or salience network). Future studies should try to include these regions (e.g., anterior cingulate or insular cortex) as targets for tomographic neurofeedback in order to develop more specific neurofeedback protocols.
Acknowledgments
The authors disclose the following financial support for research, authorship, and/or publication of this article: ‘VeluxStiftung’, ‘Zürcher Stiftung für das Hören (ZSFH)’, ‘Fonds zur Förderung des akademischen Nachwuchses (FAN) des Zürcher Universitätsvereins (ZUNIV)’, University Research Priority Program ‘Dynamics of Healthy Aging’ of the University of Zurich.
The authors are further indebted to the TINNET - COST Action BM1306 ‘Better Understanding the Heterogeneity of Tinnitus to Improve and Develop New Treatments’ for providing a network, which allows exchange of knowledge among tinnitus researchers in Europe. During the work on his dissertation, DG was a pre-doctoral fellow of LIFE (International Max Planck Research School on the Life Course; participating institutions: MPI for Human Development, Humboldt- Universität zu Berlin, Freie Universität Berlin, University of Michigan, University of Virginia, University of Zurich).
References
1 | Adjamian, P. , Sereda, M. , & Hall, D.A. ((2009) ). The mechanisms of tinnitus: Perspectives from human functional neuroimaging. Hearing Research, 253: , 15–31. doi:10.1016/j.heares.2009.04.001 |
2 | Ahlmann-Eltze, C. ((2017) ). Ggsignif: Significance brackets for ’ggplot2’. R package version 0.4.0. Retrieved from https://CRAN.R-project.org/package=ggsignif |
3 | Angermeyer, M.C. , Kilian, R. , & Matschinger, H. ((2000) ). WHOQOL-100 und WHOQOL-BREF: Handbuch für die deutschsprachige Version der WHO Instrumente zur Erfassung von Lebensqualität. Göttingen: Hogrefe. |
4 | Arns, M. , De Ridder, S. , Strehl, U. , Breteler, M. , & Coenen, A. ((2009) ). Efficacy of neurofeedback treatment in ADHD: The effects on inattention, impulsivity and hyperactivity: A meta-analysis. Clinical EEG and Neuroscience, 40: , 180–189. |
5 | Ashton, H. , Reid, K. , Marsh, R. , Johnson, I. , Alter, K. , & Griffiths, T.D. ((2007) ). High frequency localised “hot spots” in temporal lobes of patients with intractable tinnitus: A quantitative electroencephalographic (QEEG) study. Neuroscience Letters, 426: , 23–28. doi:10.1016/j.neulet.2007.08.034 |
6 | Bullinger, M. , Kirchberger, I. , & Ware, J. ((1995) ). Der deutsche SF-36 Health Survey Übersetzung und psychometrische Testung eines krankheitsübergreifenden Instruments zur Erfassung der gesundheitsbezogenen Lebensqualität. Zeitschrift für Gesundheitswissenschaften, 3: , 21. |
7 | Cannon, R.L. , Lubar, J.F. , Congedo, M. , Thornton, K. , Towler, K. , & Hutchens, T. ((2007) ). The effects of neurofeedback training in the cognitive division of the anterior cingulate gyrus. International Journal of Neuroscience, 117: , 337–357. doi:10.1080/00207450500514003. eprint: 17365119 |
8 | Cannon, R.L. , Lubar, J.F. , Gerke, A. , Thornton, K. , Hutchens, T. , & McCammon, V. ((2006) ). EEG spectral-power and coherence: LORETA neurofeedback training in the anterior cingulate gyrus. Journal of Neurotherapy, 10: , 5–31. |
9 | Cannon, R.L. , Lubar, J.F. , Sokhadze, E. , & Baldwin, D.R. ((2008) ). LORETA neurofeedback for addiction and the possible neurophysiology of psychological processes influenced: A case study and region of interest analysis of LORETA neurofeedback in right anterior cingulate cortex. Journal of Neurotherapy, 12: , 227–241. doi:10.1080/10874200802501948 |
10 | Cohen, J. ((1988) ). Statistical power analysis for the behavioral sciences (2nd edition). Hillsdale: Erlbaum. |
11 | Congedo, M. , Lubar, J.F. , & Joffe, D. ((2004) ). Low-resolution electromagnetic tomography neurofeedback. IEEE Transactions on Neural Systems and Rehabilitation Engineering, 12: , 387–397. doi:10.1109/TNSRE.2004.840492 |
12 | Crocetti, A. , Forti, S. , & Del Bo, L. ((2011) ). Neurofeedback for subjective tinnitus patients. Auris, nasus, larynx, 38: , 735–738. doi:10.1016/j.anl.2011.02.003. eprint: 21592701 |
13 | Dahl, D.B. ((2016) ). Xtable: Export tables to latex or html. R package version 1.8-2. Retrieved from https://CRAN.R-project.org/package=xtable |
14 | De Ridder, D. , Congedo, M. , & Vanneste, S. ((2015) ). The neural correlates of subjectively perceived and passively matched loudness perception in auditory phantom perception. Brain and behavior, 5: , e00331. Journal Article Research Support, Non-U.S. Gov’t. doi:10.1002/brb3.331. eprint: 25874164 |
15 | De Ridder, D. , Elgoyhen, A.B. , Romo, R. , & Langguth, B. ((2011) ). Phantom percepts: Tinnitus and pain as persisting aversive memory networks. Proceedings of the National Academy of Sciences of the United States of America, 108: , 8075–8080. doi:10.1073/pnas.1018466108 |
16 | De Ridder, D. , Vanneste, S. , Langguth, B. , & Llinás, R.R. ((2015) ). Thalamocortical dysrhythmia: A theoretical update in tinnitus. Frontiers in Neurology, 6: . doi:10.3389/fneur.2015.00124 |
17 | De Ridder, D. , Vanneste, S. , Weisz, N. , Londero, A. , Schlee, W. , Elgoyhen, A.B. , & Langguth, B. ((2014) ). An integrative model of auditory phantom perception: tinnitus as a unified percept of interacting separable subnetworks. Neuroscience & Biobehavioral Reviews, 44: , 16–32. Journal Article Research Support, Non-U.S. Gov’t. doi:10.1016/j.neubiorev.2013.03.021. eprint: 23597755 |
18 | Delorme, A. , & Makeig, S. ((2004) ). EEGLAB: an open source toolbox for analysis of single-trial EEG dynamics including independent component analysis. Journal of Neuroscience Methods, 134: , 9–21. |
19 | Dobie, R.A. ((2003) ). Depression and tinnitus.. eprint. Otolaryngologic clinics of North America, 36: , 383–388. eprint: 12856305 |
20 | Dohrmann, K. , Elbert, T. , Schlee, W. , & Weisz, N. ((2007) ). Tuning the tinnitus percept by modification of synchronous brain activity. Restorative Neurology and Neuroscience, 25: , 371–378. eprint: 17943012 |
21 | Field, A.P. , Miles, J. , & Field, Z. ((2012) ). Discovering statistics using R. London: Sage. |
22 | Fuchs,M., Kastner,J., Wagner,M., Hawes,S., & Ebersole,J.S. ((2002) ). A standardized boundary element method volume conductor model. Clinical neurophysiology, 113: , 702–712. Journal Article. eprint: 11976050 |
23 | Gevensleben, H. , Holl, B. , Albrecht, B. , Vogel, C. , Schlamp, D. , Kratz, O. ,. . . Heinrich, H. ((2009) ). Is neurofeedback an efficacious treatment for ADHD? A randomised controlled clinical trial. Journal of Child Psychology and Psychiatry, and Allied Disciplines, 50: , 780–789. Journal Article Randomized Controlled Trial Research Support,Non-U.S.Gov’t. doi:10.1111/j.1469-7610.2008.02033.x. eprint: 19207632 |
24 | Goebel, G. , & Hiller, W. ((1994) ). Tinnitus-Fragebogen (TF). Standardinstrument zur Graduierung des Tinnitusschweregrades. Ergebnisse einer Multicenterstudie mit dem Tinnitus-Fragebogen (TF). HNO, 42: , 166–177. English Abstract Journal Article Multicenter Study. eprint: 8175381 |
25 | Güntensperger, D. , Thüring, C. , Kleinjung, T. , Neff, P. , & Meyer, M. ((2019) ). Investigating the Efficacy of an Individualized Alpha/Delta Neurofeedback Protocol in the Treatment of Chronic Tinnitus. Neural Plasticity, 2019: , 3540898. Journal Article Research Support, Non-U.S. Gov’t. doi:10.1155/2019/3540898. eprint: 31049052 |
26 | Güntensperger, D. , Thüring, C. , Meyer, M. , Neff, P. , & Kleinjung, T. ((2017) ). Neurofeedback for Tinnitus Treatment - Review and Current Concepts. Frontiers in Aging Neuroscience, 9: , 386. PMC5717031 Journal Article Review. doi:10.3389/fnagi.2017.00386. eprint: 29249959 |
27 | Hall, D.A. , Mehta, R.L. , & Argstatter, H. ((2018) ). Interpreting the Tinnitus Questionnaire (German version): What individual differences are clinically important? International Journal of Audiology, 1-5. Journal Article. doi:10.1080/14992027.2018.1442591. eprint: 29490515 |
28 | Harrell F. E. Jr ((2017) ). Hmisc: Harrell miscellaneous. R package version 4.0-3. Retrieved from https://CRAN.R-project.org/package=Hmisc |
29 | Hartmann, T. , Lorenz, I. , Müller, N. , Langguth, B. , & Weisz, N. ((2013) ). The effects of neurofeedback on oscillatory processes related to tinnitus. Brain Topography. doi:10.1007/s10548-013-0295-9. eprint: 23700271 |
30 | Hautzinger, M. , Bailer, M. , Worall, H. , & Keller, F. ((1995) ). BDI Beck-Depressions-Inventar Testhandbuch. Bern: Hans Huber. |
31 | Heller, A.J. ((2003) ). Classification and epidemiology of tinnitus. Otolaryngologic clinics of North America, 36: , 239–248. doi:10.1016/S0030-6665(02)00160-3 |
32 | Helmholtz, H. ((1853) ). Ueber einige Gesetze der Vertheilung elektrischer Ströme in körperlichen Leitern, mit Anwendung auf die thierisch-elektrischen Versuche (Schluss.). Annalen der Physik und Chemie, 165: , 353–377. doi:10.1002/andp.18531650702 |
33 | Henry, J.A. , Dennis, K.C. , & Schechter, M.A. ((2005) ). General review of tinnitus: Prevalence, mechanisms, effects, and management. Journal of Speech, Language, and Hearing Research, 48: , 1204–1235. |
34 | Holmes, S. , & Padgham, N.D. ((2008) ). The incidence, management and consequence of tinnitus in older adults. Reviews in Clinical Gerontology, 18: , 269. doi:10.1017/S0959259809002883 |
35 | Hothorn, T. , Bretz, F. , & Westfall, P. ((2008) ). Simultaneous inference in general parametric models. Biometrical Journal, 50: (3), 346–363. |
36 | Husain, F.T. ((2015) ). Neural networks of tinnitus in humans: Elucidating severity and habituation. Hearing Research. JOURNAL ARTICLE. doi:10.1016/j.heares.2015.09.010. eprint: 26415997 |
37 | Jensen, M. , Hüttenrauch, E. , Schmidt, J. , Andersson, G. , Chavanon, M.-L. , & Weise, C. ((2020) ). Neurofeedback for tinnitus: Study protocol for a randomised controlled trial assessing the specificity of an alpha/delta neurofeedback training protocol in alleviating both sound perception and psychological distress in a cohort of chronic tinnitus sufferers. Trials, 21: , 382. Journal Article. doi:10.1186/s13063-020-04309-y. eprint: 32370767 |
38 | Jurcak, V. , Tsuzuki, D. , & Dan, I. ((2007) ). 10/20, 10/10, and 10/5 systems revisited: Their validity as relative head-surface-based positioning systems. NeuroImage, 34: , 1600–1611. Journal Article Research Support, Non-U.S. Gov’t. doi:10.1016/j.neuroimage.2006.09.024. eprint: 17207640 |
39 | Kahlbrock, N. , & Weisz, N. ((2008) ). Transient reduction of tinnitus intensity is marked by concomitant reductions of delta band power. BMC Biology, 6: , 4. Journal Article Research Support, Non-U.S. Gov’t. doi:10.1186/1741-7007-6-4. eprint: 18199318 |
40 | Kennedy, V. , Wilson, C. , & Stephens, D. ((2004) ). Quality of life and tinnitus. Audiological Medicine, 2: , 29–40. doi:10.1080/16513860410027349 |
41 | Klaghofer, R. , & Brähler, E. ((2001) ). Konstruktion und Teststatistische Prüfung einer Kurzform der SCL-90-R. Zeitschrift für Klinische Psychologie, Psychiatrie und Psychotherapie. |
42 | Kleinjung, T. , Fischer, B. , Langguth, B. , Sand, P. , Hajak, G. , Dvorakova, J. , & Eichhammer, P. ((2007) ). Validierung einer deutschsprachigen Version des „Tinnitus Handicap Inventory”. Psychiatrische Praxis, 34: , 140–142. doi:10.1055/s-2006-940218 |
43 | Kleinjung, T. , Steffens, T. , Sand, P. , Murthum, T. , Hajak, G. , Strutz, J. ,. . . Eichhammer, P. ((2007) ). Which tinnitus patients benefit from transcranial magnetic stimulation? Otolaryngology - Head and Neck Surgery, 137: , 589–595. Journal Article Research Support, Non-U.S. Gov’t. doi:10.1016/j.otohns.2006.12.007. eprint: 17903575 |
44 | Kreuzer, P.M. , Goetz, M. , Holl, M. , Schecklmann, M. , Landgrebe, M. , Staudinger, S. , & Langguth, B. ((2012) ). Mindfulness-and body-psychotherapy-based group treatment of chronic tinnitus: a randomized controlled pilot study. BMC Complementary and Alternative Medicine, 12: , 235. |
45 | Landgrebe, M. , Azevedo, A. , Baguley, D.M. , Bauer, C.A. , Cacace, A.T. , Coelho, C. ,... Langguth, B. ((2012) ). Methodological aspects of clinical trials in tinnitus: A proposal for an international standard. Journal of Psychosomatic Research, 73: , 112–121. doi:10.1016/j.jpsychores.2012.05.002 |
46 | Landgrebe, M. , Zeman, F. , Koller, M. , Eberl, Y. , Mohr, M. , Reiter, J. ,... Langguth, B. ((2010) ). The Tinnitus Research Initiative (TRI) database: A new approach for delineation of tinnitus subtypes and generation of predictors for treatment outcome. BMC Medical Informatics, 10: , 1–7. |
47 | Langguth, B. , Goodey, R. , Azevedo, A. , Bjorne, A. , Cacace, A.T. , Crocetti, A. ,. . . Vergara, R. ((2007) ). Consensus for tinnitus patient assessment and treatment outcome measurement: Tinnitus Research Initiative meeting, Regensburg, July 2006. Progress in Brain Research, 166: , 525–536. doi:10.1016/S0079-6123(07)66050-6 |
48 | Langguth, B. , Kreuzer, P.M. , Kleinjung, T. , & De Ridder, D. ((2013) ). Tinnitus: Causes and clinical management. The Lancet Neurology, 12: , 920–930. doi:10.1016/S1474-4422(13)70160-1 |
49 | Langguth, B. , Landgrebe, M. , Kleinjung, T. , Sand, P. , & Hajak, G. ((2011) ). Tinnitus and depression. The World Journal of Biological Psychiatry, 12: , 489–500. Journal Article Research Support, Non-U.S. Gov’t Review. doi:10.3109/15622975.2011.575178. eprint: 21568629 |
50 | Lévesque, J. , Beauregard, M. , & Mensour, B. ((2006) ). Effect of neurofeedback training on the neural substrates of selective attention in children with attention-deficit/hyperactivity disorder: a functional magnetic resonance imaging study. Neuroscience Letters, 394: , 216–221. doi:10.1016/j.neulet.2005.10.100. eprint: 16343769 |
51 | Llinás, R.R. , Ribary, U. , Jeanmonod, D. , Kronberg, E. , & Mitra, P.P. ((1999) ). Thalamocortical dysrhythmia: A neurological and neuropsychiatric syndrome characterized by magnetoencephalography. Proceedings of the National Academy of Sciences of the United States of America, 96: , 15222–15227. eprint: 10611366 |
52 | Long, J.A. ((2017) ). Jtools: Analysis and presentation of social scientific data. R package version 0.5.0. Retrieved from https://cran.r-project.org/package=jtools |
53 | Lorenz, I. , Müller, N. , Schlee, W. , Hartmann, T. , & Weisz, N. ((2009) ). Loss of alpha power is related to increased gamma synchronization—A marker of reduced inhibition in tinnitus? Neuroscience Letters, 453: , 225–228. doi:10.1016/j.neulet.2009.02.028 |
54 | Lubar, J.F. , Swartwood, M.O. , Swartwood, J.N. , & O’Donnell, P.H. ((1995) ). Evaluation of the effectiveness of EEG neurofeedback training for ADHD in a clinical setting as measured by changes in T.O.V.A. scores, behavioral ratings, and WISC-R performance. Biofeedback and Self-Regulation, 20: , 83–99. doi:10.1007/BF01712768 |
55 | Meikle, M.B. , Henry, J.A. , Griest, S.E. , Stewart, B.J. , Abrams, H.B. , McArdle, R. ,... Vernon, J.A. ((2012) ). The Tinnitus Functional Index: Development of a new clinical measure for chronic, intrusive tinnitus. Ear and Hearing, 33: , 153–176. Clinical Trial Journal Article Multicenter Study Research Support, Non-U.S. Gov’t Validation Studies. doi:10.1097/AUD.0b013e31822f67c0. eprint: 22156949 |
56 | Meyer, M. , Neff, P. , Grest, A. , Hemsley, C. , Weidt, S. , & Kleinjung, T. ((2017) ). EEG oscillatory power dissociates between distress- and depression-related psychopathology in subjective tinnitus. Brain Research, 1663: , 194–204. Journal Article. doi:10.1016/j.brainres.2017.03.007. eprint: 28315312 |
57 | Meyer, M. , Neff, P. , Liem, F. , Kleinjung, T. , Weidt, S. , Langguth, B. , & Schecklmann, M. ((2016) ). Differential tinnitus-related neuroplastic alterations of cortical thickness and surface area. Hearing Research, 342: , 1–12. Journal Article. doi:10.1016/j.heares.2016.08.016. eprint: 27671157 |
58 | Michel, C.M. , Murray, M.M. , Lantz, G. , Gonzalez, S. , Spinelli, L. , & Grave de Peralta, R. ((2004) ). EEG source imaging. Clinical Neurophysiology, 115: , 2195–2222. doi:10.1016/j.clinph.2004.06.001 |
59 | Morey, R.D. ((2008) ). Confidence intervals from normalized data: A correction to Cousineau. Tutorial in Quantitative Methods for Psychology, 4: , 61–64. |
60 | Newman, C.W. , Sandridge, S.A. , & Jacobson, G.P. ((1998) ). Psychometric adequacy of the Tinnitus Handicap Inventory (THI) for evaluating treatment outcome. Journal of the American Academy of Audiology, 9: , 153–160. Journal Article. eprint: 9564679 |
61 | Oostenveld, R. , & Praamstra, P. ((2001) ). The five percent electrode system for high-resolution EEG and ERP measurements. Clinical Neurophysiology, 112: , 713–719. |
62 | Pantev, C. , Bertrand, O. , Eulitz, C. , Verkindt, C. , Hampson, S. , Schuierer, G. , & Elbert, T. ((1995) ). Specific tonotopic organizations of different areas of the human auditory cortex revealed by simultaneous magnetic and electric recordings. Electroencephalography and Clinical Neurophysiology, 94: , 26–40. |
63 | Pascual-Marqui, R.D. ((2002) ). Standardized low-resolution brain electromagnetic tomography (sLORETA): technical details. Methods and Findings in Experimental and Clinical Pharmacology, 24: , 5–12. eprint: 12575463 |
64 | Pascual-Marqui, R.D. , Michel, C.M. , & Lehmann, D. ((1994) ). Low resolution electromagnetic tomography: a new method for localizing electrical activity in the brain. International Journal of Psychophysiology, 18: , 49–65. |
65 | Peter, N. , Kleinjung, T. , Jeker, R. , Meyer, M. , Klaghofer, R. , & Weidt, S. ((2017) ). Tinnitus functional index: validation of the German version for Switzerland. Health and Quality of Life Outcomes, 15: , 94. PMC5420117 Journal Article. doi:10.1186/s12955-017-0669-x. eprint: 28476163 |
66 | Pinheiro, J. , Bates, D. , DebRoy, S. , Sarkar, D. , & R Core Team. (2017). nlme: Linear and nonlinear mixed effects models. R package version 3.1-131. Retrieved from https://CRAN.R-project.org/package=nlme |
67 | Prinz, M. , & Petermann, F. ((2015) ). Beck Angst-Inventar (BAI). Zeitschrift für Psychiatrie, Psychologie und Psychotherapie. |
68 | R Core Team. (2017). R: A language and environment for statistical computing. R Foundation for Statistical Computing. Vienna, Austria. Retrieved from https://www.R-project.org/ |
69 | Rauschecker, J.P. , Leaver, A.M. , & Mühlau, M. ((2010) ). Tuning out the noise: limbic-auditory interactions in tinnitus. Neuron, 66: , 819–826. doi:10.1016/j.neuron.2010.04.032. eprint: 20620868 |
70 | Rauschecker, J.P. , May, E.S. , Maudoux, A. , & Ploner, M. ((2015) ). Frontostriatal gating of tinnitus and chronic pain. Trends in Cognitive Sciences, 19: , 567–578. Journal Article Research Support, N.I.H., Extramural Research Support, Non-U.S. Gov’t Review. doi:10.1016/j.tics.2015.08.002. eprint: 26412095 |
71 | Schlee, W. , Dohrmann, K. , Hartmann, T. , Lorenz, I. , Müller, N. , Elbert, T. , & Weisz, N. ((2008) ). Assessment and modification of the tinnitus-related cortical network. Seminars in Hearing, 29: , 270–287. doi:10.1055/s-0028-1082033 |
72 | Sedley, W. , Friston, K.J. , Gander, P.E. , Kumar, S. , & Griffiths, T.D. ((2016) ). An integrative tinnitus model based on sensory precision. Trends in Neurosciences, 39: , 799–812. Review Journal Article. doi:10.1016/j.tins.2016.10.004. eprint: 27871729 |
73 | Strehl, U. , Aggensteiner, P. , Wachtlin, D. , Brandeis, D. , Albrecht, B. , Arana, M. ,. . . Holtmann, M. ((2017) ). Neurofeedback of slow cortical potentials in children with attention-deficit/hyperactivity disorder: A multicenter randomized trial controlling for unspecific effects. Frontiers in Human Neuroscience, 11: , 135. PMC5374218 Journal Article. doi:10.3389/fnhum.2017.00135. eprint: 28408873 |
74 | van den Berge, M. J. , Free, R. H. , Arnold, R. , De Kleine, E. , Hofman, R. , van Dijk, J. Marc C. , & van Dijk, P. ((2017) ). Cluster analysis to identify possible subgroups in tinnitus patients. Frontiers in Neurology, 8: , 115. |
75 | van Doren, J. , Arns, M. , Heinrich, H. , Vollebregt, M.A. , Strehl, U. , & K Loo, S. ((2019) ). Sustained effects of neurofeedback in ADHD: A systematic review and meta-analysis. European Child & Adolescent Psychiatry, 28: , 293–305. Journal Article Meta-Analysis Systematic Review. doi:10.1007/s00787-018-1121-4. eprint: 29445867 |
76 | van Veen, B.D. , van Drongelen, W. , Yuchtman, M. , & Suzuki, A. ((1997) ). Localization of brain electrical activity via linearly constrained minimum variance spatial filtering. IEEE Transactions on Biomedical Engineering, 44: , 867–880. Journal Article. doi:10.1109/10.623056. eprint: 9282479 |
77 | Vanneste, S. , Joos, K. , Ost, J. , & De Ridder, D. ((2016) ). Influencing connectivity and cross-frequency coupling by real-time source localized neurofeedback of the posterior cingulate cortex reduces tinnitus related distress. Neurobiology of Stress, 1-14. doi:10.1016/j.ynstr.2016.11.003 |
78 | Vanneste, S. , Plazier, M. , van der Loo, E. , van de Heyning, P. , Congedo, M. , & De Ridder, D. ((2010) ). The neural correlates of tinnitus-related distress. NeuroImage, 52: , 470–480. |
79 | Weisz, N. , Dohrmann, K. , & Elbert, T. ((2007) ). The relevance of spontaneous activity for the coding of the tinnitus sensation. Progress in Brain Research, 166: , 61–70. doi:10.1016/S0079-6123(07)66006-3 |
80 | Weisz, N. , Moratti, S. , Meinzer, M. , Dohrmann, K. , & Elbert, T. ((2005) ). Tinnitus perception and distress is related to abnormal spontaneous brain activity as measured by magnetoencephalography. PLoS Medicine, 2: , e153. doi:10.1371/journal.pmed.0020153. eprint: 15971936 |
81 | Weisz, N. , Müller, S. , Schlee, W. , Dohrmann, K. , Hartmann, T. , & Elbert, T. ((2007) ). The neural code of auditory phantom perception. Journal of Neuroscience, 27: , 1479–1484. doi:10.1523/JNEUROSCI.3711-06.2007 |
82 | White, D.J. , Congedo, M. , & Ciorciari, J. ((2014) ). Source-based neurofeedback methods using EEG recordings: Training altered brain activity in a functional brain source derived from blind source separation. Frontiers in Behavioral Neuroscience, 8: , 373. Journal Article. doi:10.3389/fnbeh.2014.00373. eprint: 25374520 |
83 | Wickham, H. ((2009) ). Ggplot2: Elegant graphics for data analysis. Springer-Verlag New York. Retrieved from http://ggplot2.org |
84 | Zeman, F. , Koller, M. , Figueiredo, R.R. , Aazevedo, A. , Rates, M. , Coelho, C. ,. . . Landgrebe, M. ((2011) ). Tinnitus handicap inventory for evaluating treatment effects: which changes are clinically relevant? Otolaryngology - Head and Neck Surgery, 145: , 282–287. Comparative Study Journal Article Multicenter Study Research Support, Non-U.S. Gov’t. doi:10.1177/0194599811403882. eprint: 21493265 |