Smooth leader or sharp follower? Playing the mirror game with a robot
Abstract
Background:
The increasing number of opportunities for human-robot interactions in various settings, from industry through home use to rehabilitation, creates a need to understand how to best personalize human-robot interactions to fit both the user and the task at hand. In the current experiment, we explored a human-robot collaborative task of joint movement, in the context of an interactive game.
Objective:
We set out to test people’s preferences when interacting with a robotic arm, playing a leader-follower imitation game (the mirror game).
Methods:
Twenty two young participants played the mirror game with the robotic arm, where one player (person or robot) followed the movements of the other. Each partner (person and robot) was leading part of the time, and following part of the time. When the robotic arm was leading the joint movement, it performed movements that were either sharp or smooth, which participants were later asked to rate.
Results:
The greatest preference was given to smooth movements. Half of the participants preferred to lead, and half preferred to follow. Importantly, we found that the movements of the robotic arm primed the subsequent movements performed by the participants.
Conclusion:
The priming effect by the robot on the movements of the human should be considered when designing interactions with robots. Our results demonstrate individual differences in preferences regarding the role of the human and the joint motion path of the robot and the human when performing the mirror game collaborative task, and highlight the importance of personalized human-robot interactions.
1Introduction
Human-robot interactions are becoming more prevalent in a variety of contexts (Stein, 2012). One domain which has seen an increase in development of dedicated robotics is physical rehabilitation (Stein, 2012). They have the potential to reduce the reliance on one-on-one therapy time, and improve the rehabilitation process (Munih & Bajd, 2011). Hillman defined rehabilitation robotics as the application of robotic technology to the rehabilitation needs of people with disabilities as well as those of the growing elderly population (Hillman, 1998). This suggests that the need for rehabilitation robotics will increase in the near future, since, according to the World Health Organization (WHO), senior citizens at least 65 years of age will increase in number by 88% in the coming years (Krebs et al., 2008).
Robots today are able to assist the patients in various aspects of the rehabilitation process, including active and passive guidance, coaching and tracking the performance of the patient (Maciejasz et al., 2014). However, clinical evidence so far indicates their major contribution is in the number of repetitions given to the recovering patient (Brochard et al., 2010), rather than an improvement in the ability to perform movement, compared to human-mediated therapy (Lo et al., 2010). The success of rehabilitation robotics thus hinges on the ability to understand what elements of the robotic therapy scheme can be improved in order to achieve clinical progress that augments human-mediated therapy in a clinically meaningful manner. One area that has been identified as a potential key to increased benefit from therapy is patient motivation (Colombo et al., 2007). Since rehabilitation is a long-term process, it is important to understand how to keep patients motivated to interact with the robot over an extended period of time.
The robot’s physical embodiment, its physical presence, appearance, and its shared context with the user, are fundamental for creating a long term engaging relationship with the user (Tapus et al., 2007). Eriksson et al. (2005) found that some post-stroke patients immediately started to attribute human intentions and emotions to the rehabilitation robot, such as talking to it as if to a child. People naturally engage with physically embodied creatures, which is critical for time-extended interaction as required in post-stroke rehabilitation (Eriksson et al., 2005). Potkonjak et al. (2001), suggest that human-like movements of the robot can help induce feelings of comfort in the interacting human.
There is evidence suggesting that combining robotics with a gamification approach significantly improves the functional ability of the patients by engaging and motivating them (Li et al., 2014). After discharge from rehabilitation facilities, when returning home, the patients train less than in the physical therapy clinic and often regress and fail to fulfill their potential and to sustain skill levels they had formerly achieved during their rehabilitation (Jacobs et al., 2013). One of the main causes for this reduced level of training is ‘diminished motivation’ (Jacobs et al., 2013). Since training is tedious and patients lose confidence regarding their potential to improve, Jacobs et al. (2013) suggests the gamification approach for making the exercise itself less dull. da Silva Cameirão et al. (2011) show that rehabilitation with a rehabilitation gaming system facilitates the functional recovery of the upper limb in the acute phase of stroke, particularly in activities of daily living.
A relatively new category of rehabilitation robots, the socially assistive robots (SAR) (Eriksson et al., 2005) is very well suited for the incorporation of a gamification approach into robotics. The goal of SAR is to provide assistance to human users through social interaction, usually when performing an exercise program. SARs create close and effective interaction with a human user for the purpose of giving assistance and achieving measurable progress in convalescence, rehabilitation, learning, etc. (Feil-Seifer et al., 2005). Fasola and Mataric (2013) presented the SAR exercise system: a social agent that serves as a coach and as an active participant in the exercise program. According to Brooks et al. (2014) as with any interaction, it is logical to design a robot-human game interactions in terms of social human-human models. This social interaction between the robot and the user, besides maintaining user engagement and influencing intrinsic motivation, is useful in order to achieve the physical exercise task. Similarly, other robotic systems have also been designed to serve as coaches in an exercise program (Martin et al., 2015). For example, the NAO robot (Martin et al., 2015) has been used to ask patients to assume a given pose, and to monitor and encourage the patient when difficulties arise. Kang et al. (2005) demonstrated another therapist robot which monitors and encourages cardiac patients during breathing exercises.
However, a partnership approach in human-human interaction is preferable to a coaching approach in order to achieve collaboration according to Knight (2011). Here, we applied this approach to human-robot interaction. Our ultimate goal is to develop an interactive movement protocol to be used as part of a rehabilitation session, with the robotic arm leading the users through a series of movements prescribed by a therapist. As a first step towards this goal, we performed this preliminary study, with young healthy individuals, to test user preferences in this paradigm.
One of the reasons we chose the mirror game as the experimental task (please see the Methods section for details) was to exploit the positive outcome of the chameleon effect. Chartrand et al. (1999) show this effect in the following experiment: they had people mimic the posture and movements of participants and showed that mimicry facilitates the smoothness of interactions and increases liking between interaction partners. In addition, Shimada et al. (2008) have shown that the chameleon effect, which increases the likeability of a person who mimics another during a conversation, appears not only with humans but also with computer-generated agents. They used an android with a very humanlike appearance and studied the principle of human-human communication. They compared mimic and non-mimic conditions when an android conversed with participants face-to-face. Likeability toward an android increased when the android mimicked its partner. Moreover, research has shown that people relate more to other people or representations of people that resemble themselves. For example, Hasler et al. (2017) found that people tended to mimic the motions of a virtual person that looked similar to their own avatar. We anticipated that including bi-directional mimicking in the interaction paradigm will help to create a closer connection between the robot and theperson.
We hypothesized that the movement of the robotic arm can be used to prime the movements of the participants. Priming can be described as a behavior change generated by a preceding stimulus (Stoykov et al., 2017). Priming can take several forms, including cognitive, visual, and motor (Bargh et al., 1992; Fazioet et al., 1986; Gillmeister et al., 2008; Hermans et al., 1994, 2001; Stoykov et al., 2017; Westlund et al., 2017). Although various types of priming have been long studied in the field of psychology, movement priming is a relatively new topic of research in the fields of motor control and rehabilitation (Stoykov et al., 2017).
Recent studies have looked into movement priming between humans and robots (Eizicovits et al., unpublished data, Oberman et al., 2007; Pierno et al., 2008; Press et al., 2005). For example, we showed in a recent experiment a demonstration of movement priming by a robotic arm: participants moved significantly slower when playing with a slow robotic arm, compared to when playing with a fast, non-embodied, system (Eizicovits et al., unpublished data). These findings contrast with recent reports, which suggest that observation of human movement, but not of robotic movement, leads to visuomotor priming (Press et al., 2005; Tai et al., 2004).
Movement priming can be beneficial, for example, in the context of rehabilitation, if the robot can induce the user to perform more desirable movements. However, it can potentially also have a negative effect: Carpintero et al. (2010) describe how a robotic scrub nurse has been developed to assist a human surgeon during surgical interventions, handing surgical tools to the surgeon. We suggest that if the surgeon will be primed by the robotic nurse’s movements, he or she may adjust the movement speed of their own hand to the speed of the robotic nurse’s movement, and a pace that is too slow or too fast, or a movement pattern that is too sharp (robotic), may interfere with the surgery. Rather than using his or her normal pace and movement pattern, the surgeon may be primed by the robotic movement, which is likely not as refined as the surgeon’s skilled movement pattern, thus potentially causing harm to thepatient.
Therefore, it is important to note when a priming effect is present, and treat this effect as a design feature. Based on our previous experience (Eizicovits et al., unpublished data), we expect to see movement priming by the robotic arm when playing the mirror game.
In a previous study (Levy-Tzedek et al., 2017), we investigated the preferences and motivation of 23 participants to continue a movement-based interaction with an embodied robotic arm, compared to interacting with its non-embodied screen projection (see Fig. 1). We found that the movement pattern of the participants did not differ in the embodied vs. the non-embodied condition.
Fig.1
The experimental setup in (Levy-Tzedek et al., 2017). Top: a participant playing with the robotic arm. Bottom: a participant playing with the projection of the robotic arm on a screen.
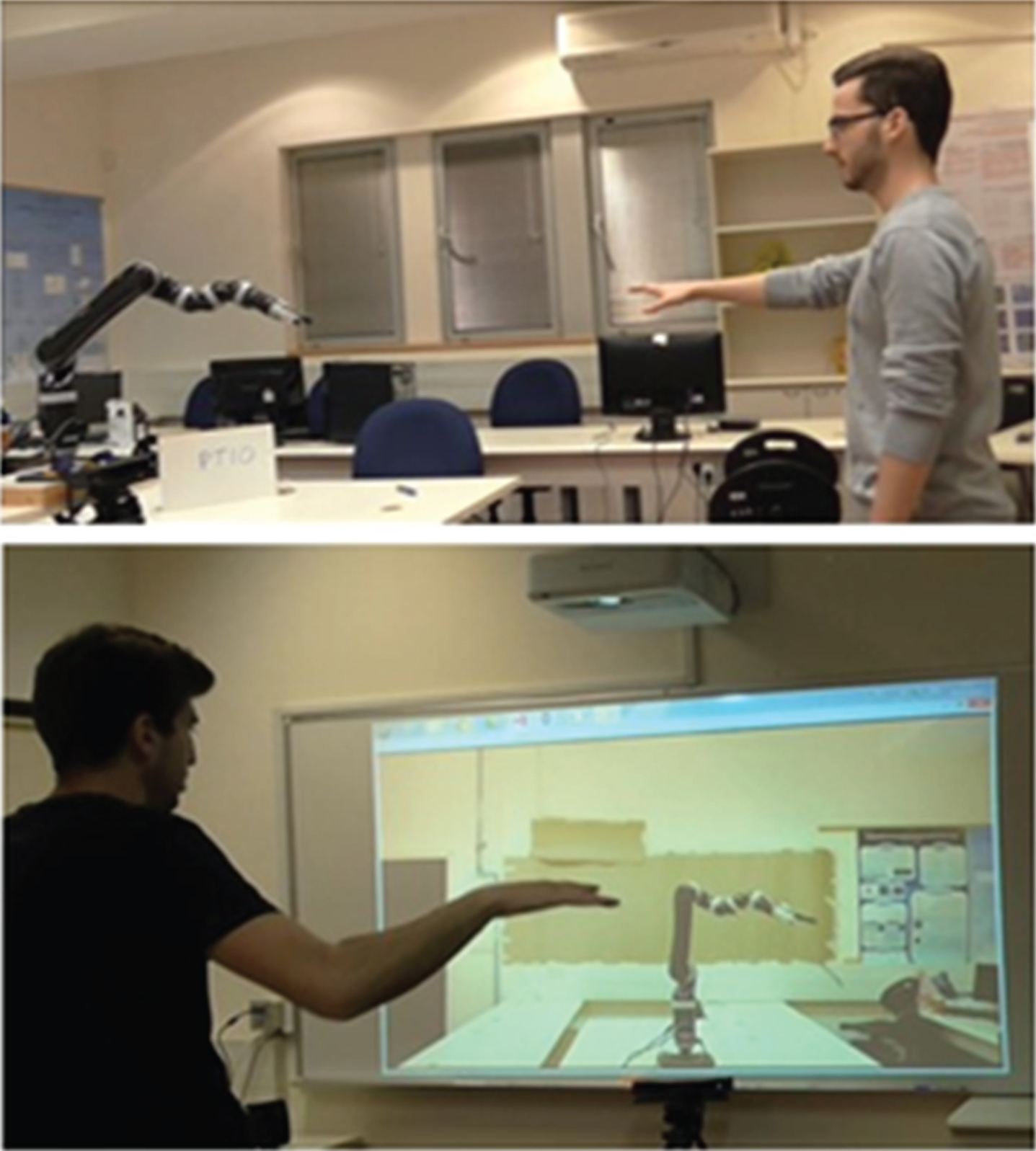
We also found that the participants that played with the embodied robot found it to be significantly more humanlike and likable than its screen projection. Most participants said that they enjoyed the novelty, and found it enjoyably challenging to adjust their movements to the robot’s movements. However, there were several disadvantages to the design of that study. They were: 1) Participants only followed their robotic partner, and did not have an opportunity to lead. 2) In that study, the distance of participants was 1.4 meters from the robot’s hand. Therefore, the participants stood far from their mirror-game partner. This may have caused them to feel alienated from both partners (embodied and non-embodied), and may underlie the non-enthusiastic responses to both embodied and non-embodiedsystems.
In the current experiment, each player – robot or human – took turns following the other in performing movements in 3D space. There were two conditions: (1) the participant led, and the robotic arm mimicked his/her movements, or (2) the robot led and the participant followed with his/her right hand. For the robot-led condition, we designed a set of six pre-defined movements, three of which were smooth and three were sharp (see Fig. 2), to test which category of movements (smooth vs. sharp) participants prefer. In this exploratory study, we were interested in examining which features in this game were more enjoyable for participants. When this interaction will be used in the future for rehabilitation, we will want to make sure that the participants will derive maximum enjoyment and will therefore be motivated to continue the interaction. We asked: 1) whether they preferred to lead or to follow the robot; 2) which motion paths participants preferred from the six robot-generated movements; and 3) whether the human movement will be primed by the robotic movement. We hypothesized that the participants would prefer the smooth movements because they are more reminiscent of human movements.
Fig.2
The shapes outlined by the robotic arm. Left column: The sharp movements Rectangle, Horizontal zigzag and Vertical zigzag. Right column: Circle, Infinity sign and Cloud.
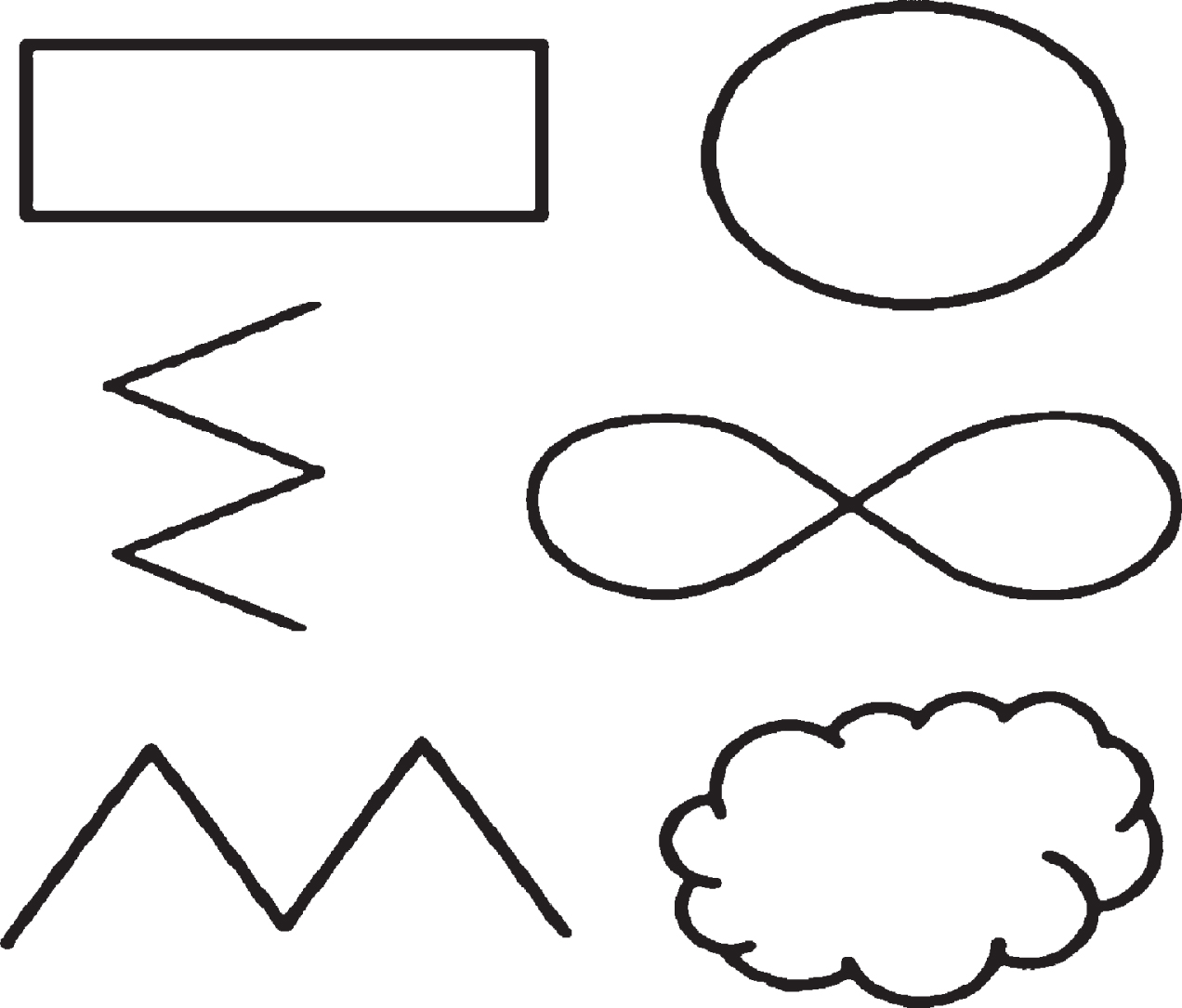
2METHODS
2.1The mirror game
The participants played the mirror game with the robotic arm as a partner. This game is a theatre exercise in which actors put their hands up against each other, in close proximity, though not touching, and move together in space – one leading and the other following (Hart et al., 2014). The mirror game has been described as a powerful tool in creating a sense of togetherness between participants (Hart et al., 2014; Noy et al., 2011). It has been shown to activate the mirror neurons in the ventral pre-motor cortex (Zhai et al., 2014), with the leader showing more prefrontal activation than the follower (Gueugnon et al., 2016), suggesting that improvising and creating new movements from scratch (the leader) is a more demanding task for the prefrontal cortex than simply following. Neurons in this area are activated both when performing a given action and when observing a similar action performed by others (Rizzolatti et al., 1996). Such activation is particularly relevant in motor rehabilitation where patients are often required to replicate movements shown to them by a physical therapist. To the best of our knowledge, this is the first time that the interactive mirror game is studied with a robot as one ofthe partners.
2.2Participants
Twenty-two healthy, right-handed participants (13 female, 9 male), aged 20–30 (25.2±2.0), signed informed consent and were assigned semi-randomly to a different order of the experimental conditions (leader/follower, presentation order of the different shapes). Two participants reported being diagnosed with ADHD. The experimental protocol was approved by the Ben Gurion University Ethics Committee.
2.3Experimental setup
We used a Kinova MICO robotic arm and a Microsoft Kinect camera v.2.0, a customized version of Microsoft’s skeleton tracker, to capture the participants’ movements. The Microsoft Kinect depth camera captures the location of the participant’s skeleton, which we used to continuously acquire the location of the participants’ right hand during the experiment (see Fig. 3). In each of 22 trials, each lasting 15–20 secs, participants were instructed to either follow or lead the robotic arm. When they were instructed to lead, the robotic arm followed the participant’s hand (see Fig. 4). There was a questionnaire before and after the experiment based on the Godspeed questionnaire (Bartneck et al., 2009). Participants stood 45 cm away from the base of the robot, such that their hands were approximately 15 cm from the robotic hand. The experiment progressed through three phases: in Phase 1, the participant led the joint movement with the robot following. The instruction was to perform any movement that comes to mind. There were two such trials (termed trials 1A and 1B), each lasting 20 secs. In Phase 2, the robotic arm led the movement, and the participant followed. The robotic arm outlined three smooth movements (a circle, an infinity sign and a cloud) and three sharp movement (a vertical zigzag, a horizontal zigzag and a rectangle); each shape was repeated 3 times in a semi-random order. Each participant was presented with one of four pre-determined semi-randomized sequences of the 18 robotic-led movements. The participants were not shown the drawn shapes as shown here in Fig. 2, but rather saw them traced by the robotic arm, and were asked to try recognize the shapes they were following. In Phase 3, the participant once again led the interaction with the robotic arm for two subsequent trials (termed trials 3A and 3B, respectively) each lasting 20 secs.
Fig.3
Experimental equipment. Top: The Kinova MICO robotic arm, which served as a partner in the mirror game. Bottom: a demonstration of the real-time location of the participants’ hand captured and displayed via a Kinect 2.0 camera (Left), as the participant interacted with the robotic arm (Right).
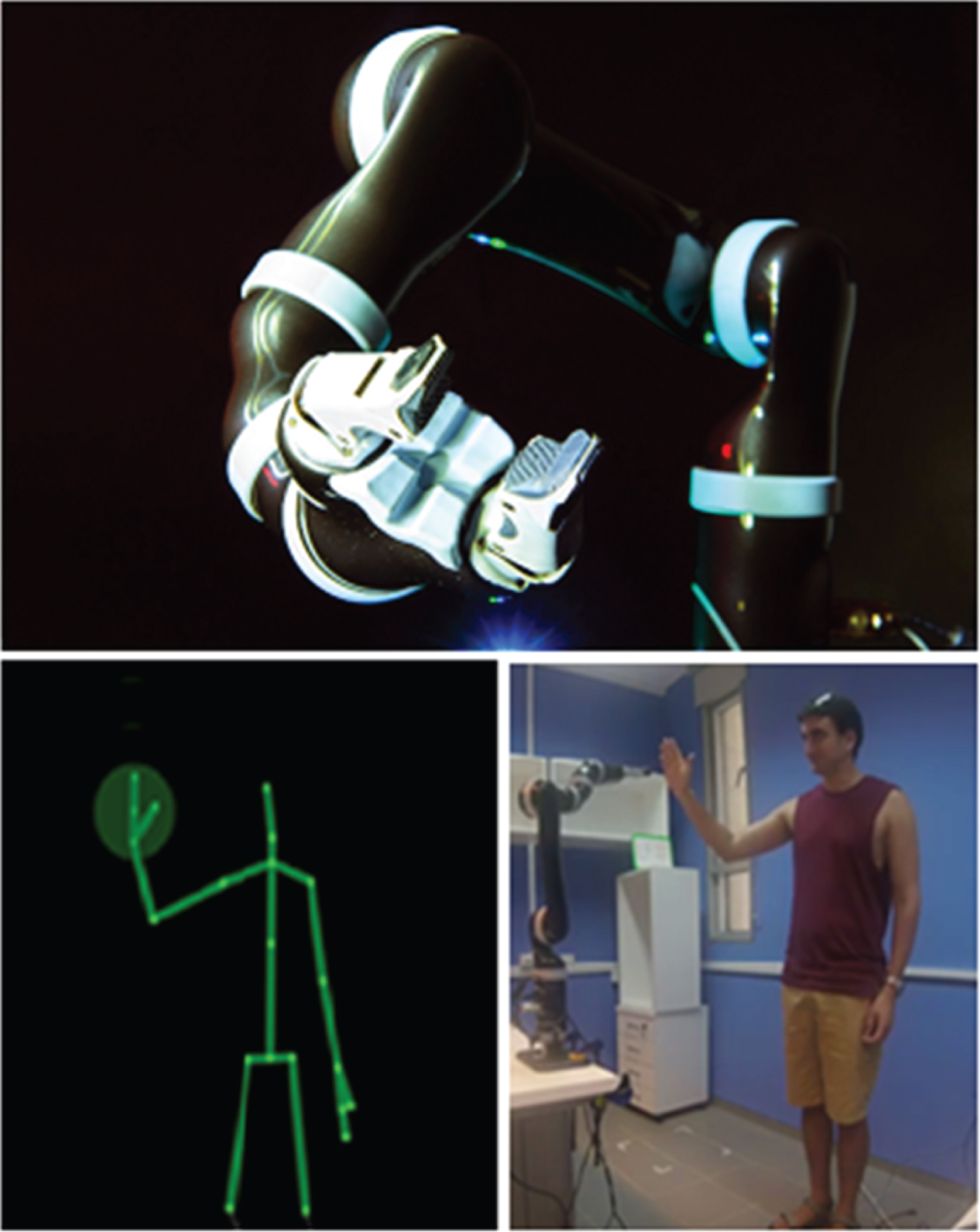
Fig.4
The experimental setup. Shown here is a participant playing the mirror game with the robotic partner.
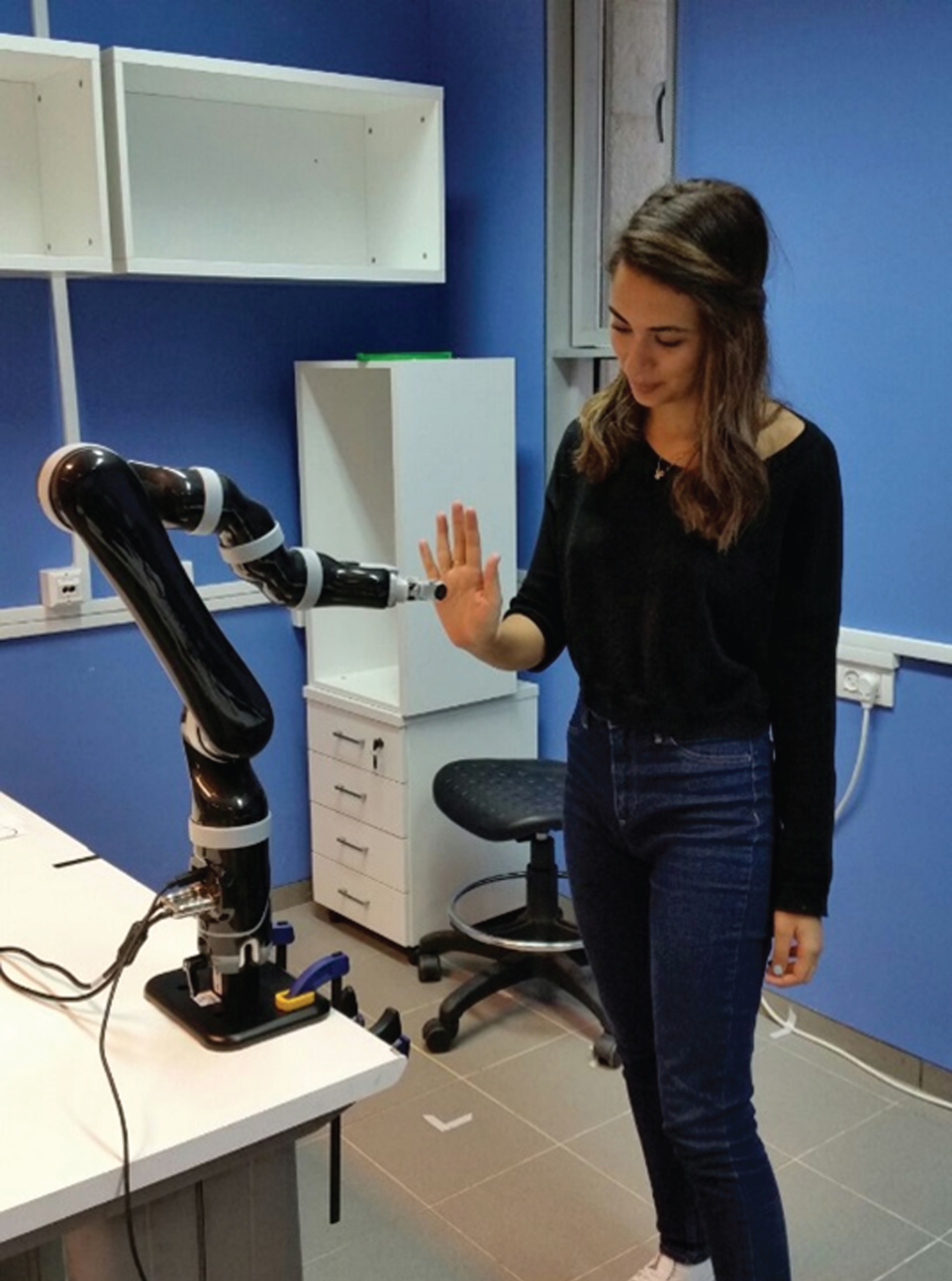
During Phases 1 and 3, the participant’s hand location, captured by the Kinect camera, was sent to the robotic arm as a position command, so that it moved in synchronization with the participant. A questionnaire, made up of mostly open questions, was administered at the end of the experiment to test the participants’ reactions and motivation to continue interaction with the robot. Participants were asked to note up to three movements of the robot which they liked most (see Table 1).
Table 1
The end-of-experiment questionnaire
Questions | Possible answers |
1. Rank the level at which you found the robot likeable on a scale of 1–5 (‘1’–‘I do not like it’, ‘5’–‘I like it’) | □ 1 □ 2 □ 3 □ 4 □ 5 |
2. What were the things that challenged you during the interaction with the robot? | ———————— |
3. Was the experience more enjoyable when you led, or when you were following the robot’s movements? | □ Preferred to lead □ Preferred to follow |
4. Would you prefer another interaction with the robot instead of the one in the current experiment? | □ Yes □ No |
5. If you were offered to continue the interaction with the robot leading, would you be interested? | □ Yes □ No |
6. If you were offered to continue the interaction with you leading would you be interested? | □ Yes □ No |
7. Rank up to three of the robot’s movements (out of the six), which you most liked, and explain why? (*here, participants were shown a diagram with all six movements, as shown in Fig. 2 above) | □ 1□ 2□ 3 □ 4 □ 5 □ ———————— |
2.4Data analysis
In order to quantify the changes between the two human-led phases (phase 1 and phase 3), we calculated the volume in 3D space taken up by the shapes the participants performed in 3D, and the number of movement reversals during each phase (i.e., how many times the participants changed the direction of their movement in each phase). A detailed explanation on how each measure was calculated is provided below. For each measure, the values obtained for trials 1A and 1B were averaged to give a single value for phase 1, and similarly, the values of trials 3A and 3B were averaged to give a single value for phase 3.
2.4.1Volume
The volume of each shape was calculated by calculating the maximal extent of the movement along each of the three axes (x, y and z), and multiplying all three by one another.
2.4.2Movement reversals
The number of movement reversals was calculated by finding the points along the velocity traces in each of the three movement axes, where a change in the sign occurred (from positive to negative velocity, or vice versa), indicating the movement direction changed.
2.5Statistical anaysis
We used a paired t-test in order to test whether the differences between the volumes and the number of movement reversals between phase 1 and phase 3 were statistically significant. We performed the statistical analysis using the SPSS Statistics toolbox (Version 22.0.0.0).
3Results
3.1Preferred movements
Figure 5 shows the robot-generated movements on the x axis, ordered according to the participants’ preferences, from left (highest preference) to right (lowest preference). The three most preferred robot movements were, in order of preference: the infinity sign (18 votes), the vertical zigzag (11 votes) and the circle (9 votes).
Fig.5
Participants’ ratings of the robot-led movements. The robot-generated movements on the x axis, ordered according to the participants’ preferences.
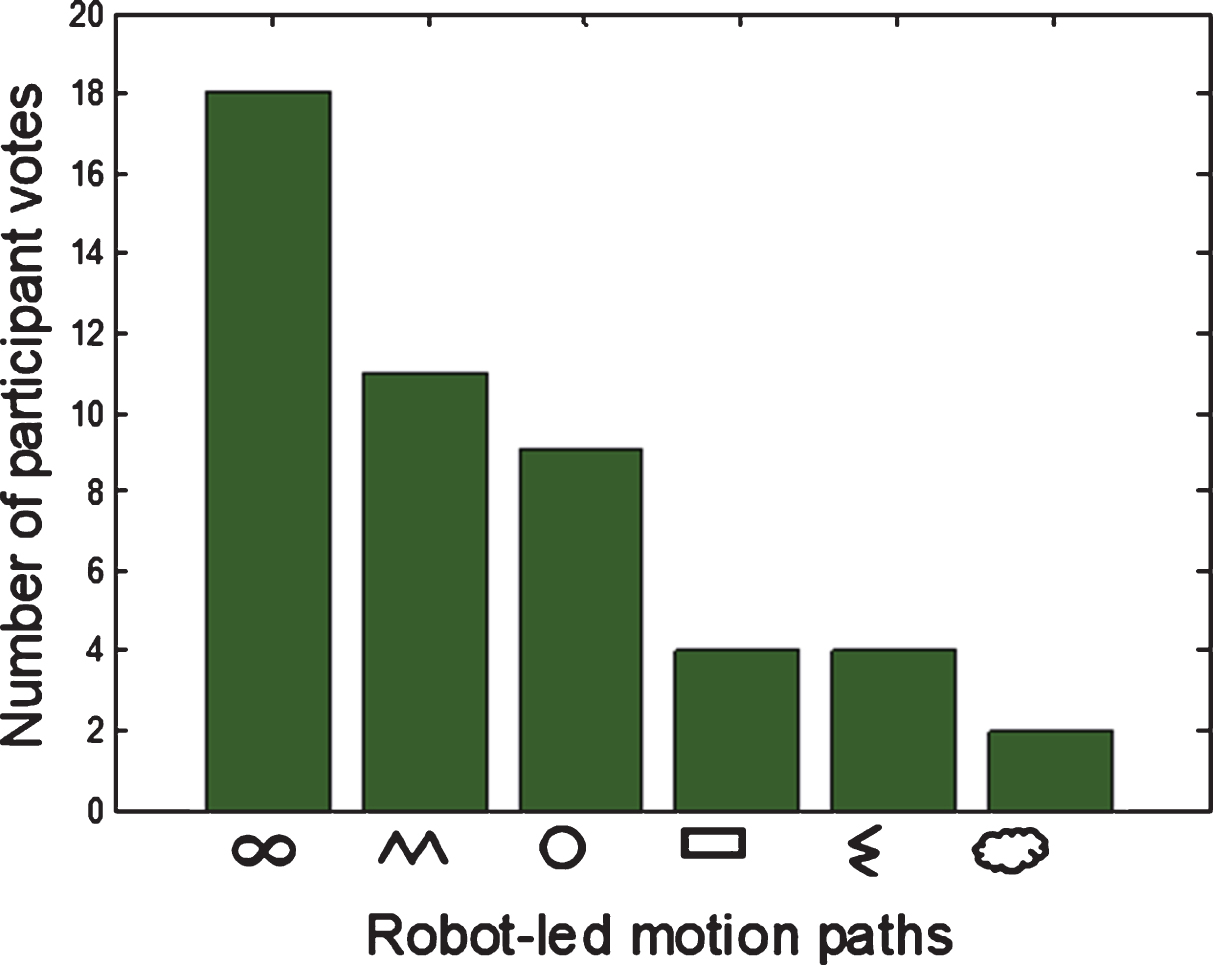
The reasons participants gave for their preference of the infinity shape were that it was a smooth, flowing and interesting motion. In addition, they noted that the infinity sign is simpler than the cloud shape and more challenging than the circle. Participants who liked the vertical zigzag said it reminds them of the motions people perform in real life (like dribbling a ball). The participants noted that the motion of tracing the circle felt familiar, simple andhumanlike.
The least preferred motions were the horizontal zigzag and the cloud. Many participants said the cloud was a long, incomprehensible and complex movement. They were asked whether they would describe the interaction with the robot as a game, and 18 out of 22 answered that they would.
3.2The interaction with the robotic arm
Participants were asked if they would prefer another interaction with the robot instead of the one in the current experiment. While the majority say they would not, (14 out of 22), there were eight participants that said they would be glad if it involved a voice interaction and physical contact. Participants were asked which part of the interaction with the robot they most liked. Exactly half of the participants (11 out of 22) preferred to lead, and the other half preferred to follow the robot. The half that preferred to lead said that making the robot imitate them was interesting and challenging, and that they enjoyed its human-like movements. The other half that preferred to follow found it fun and interesting to mimic specific structured movements. The participants were asked whether they would be interested in performing additional movements with the robot leading. Nineteen out of 22 said yes, and three out of 22 said they would not. When asked whether they would like perform additional movements as leaders, 18 out of 22 said yes, and four out of 22 said theywould not.
Participants were asked to rank the level at which they found the robot likeable on a scale of 1–5, with ‘1’ being ‘I do not like it’, and ‘5’ being ‘I like it’. The average score was 4.04±0.93 (see Fig. 6).
Fig.6
Robot likeability. The distribution of participant responses to the question of how likeable they found the robot (‘1’- ‘I do not like it’, ‘5’ - ‘I like it’).
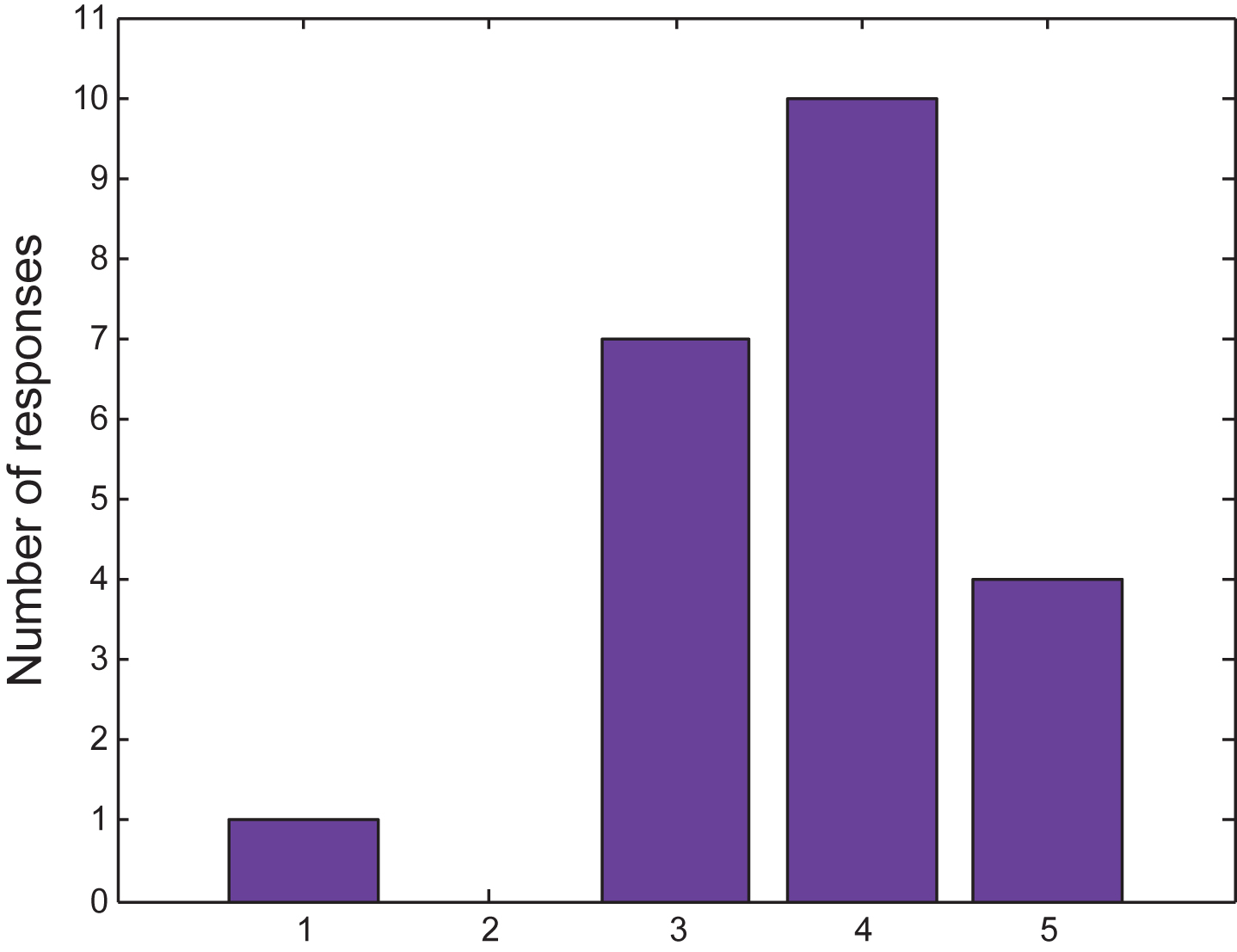
3.3Priming
Figure 7 shows movement traces from four participants. As is apparent in the figure, in trials 3A and 3B, which take place after phase 2, in which the robot leads through prescribed movements, the participants begin to create movements that are more closed and recognizable than the movements they produced in trials 1A and 1B. It appears that the movement of the robotic arm is priming the movement of the participants.
Fig.7
Sample movement traces from the participant-led phases 1 and 3. Each row shows the traces from the movements of a single participant (P1, P2, P3, P4). In each row, the images from left to right show the movements the participant performed while the robot followed him or her (Phases 1A, 1B, 3A, and 3B, respectively).
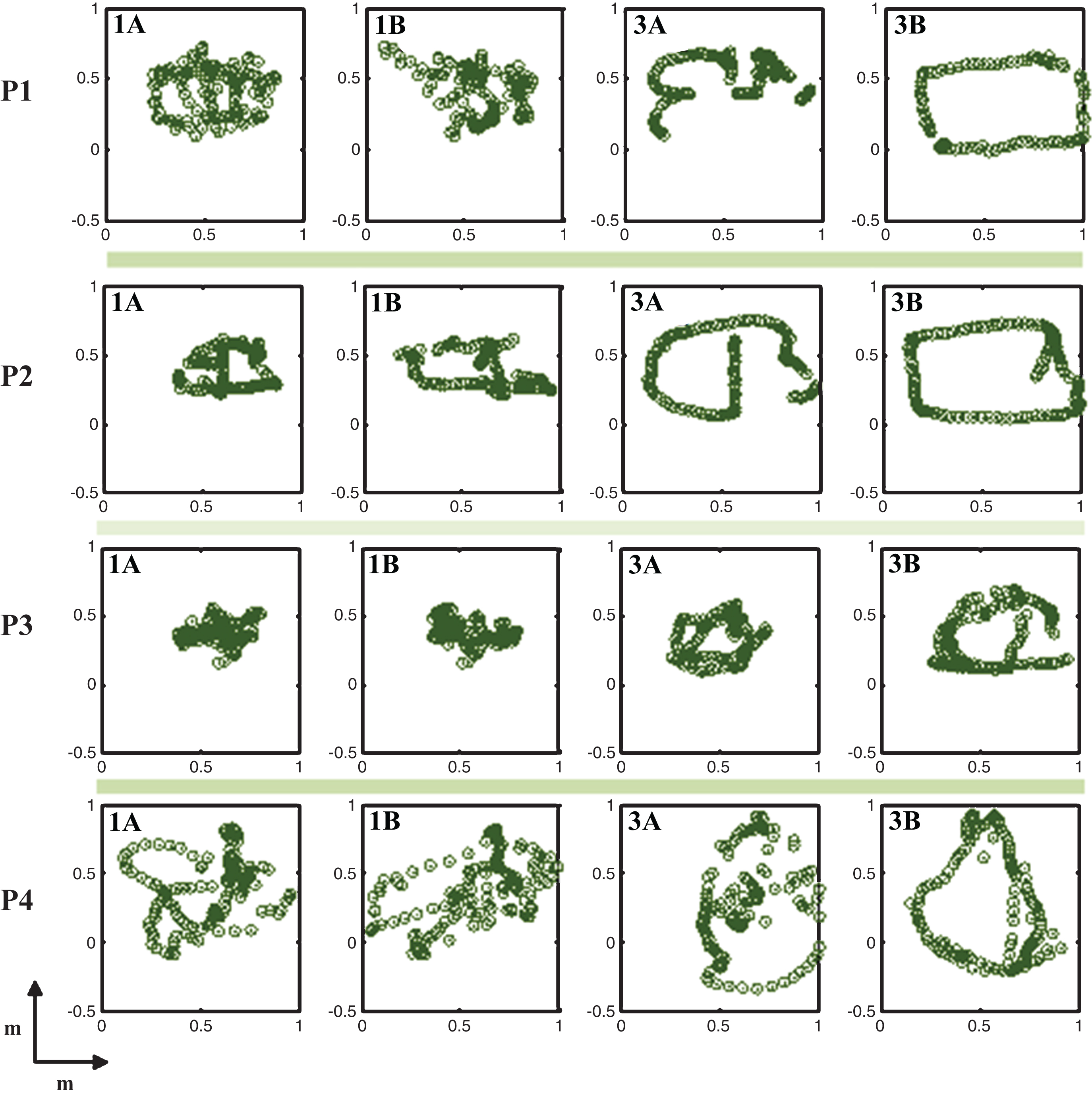
3.4Volume
The volume of the movements participants made during phase 3 (0.33±0.19 m3) was significantly larger than the volume of the movements they performed during phase 1 (0.26±0.16 m3), (t21 = –3.201, p < 0.05). On average, the volume in phase 3 was 0.074 m3 larger than the volume in phase 1 (95% CI [–0.122, –0.026]). In phase 2, the volume of movements was, on average 1.16±0.26 m3. From Fig. 8, showing the volume of each individual participant in trials 1A, 1B, 2, 3A and 3B, normalized to each person’s 1A value, it is apparent that there was a continuous trend of increase in average movement volume. The volume of the movements in phase 2 was, on average, 0.9 m3 larger than the volume in phase 1 and 0.83 m3 larger than the volume inphase 3.
Fig.8
Normalized movement volume. Data from the four human-led trials of all participants are shown here, along with the data from the robot-led trials (phase 2). For each participant, the values were normalized by the first trial, 1A, in order to track the relative increase across trials.
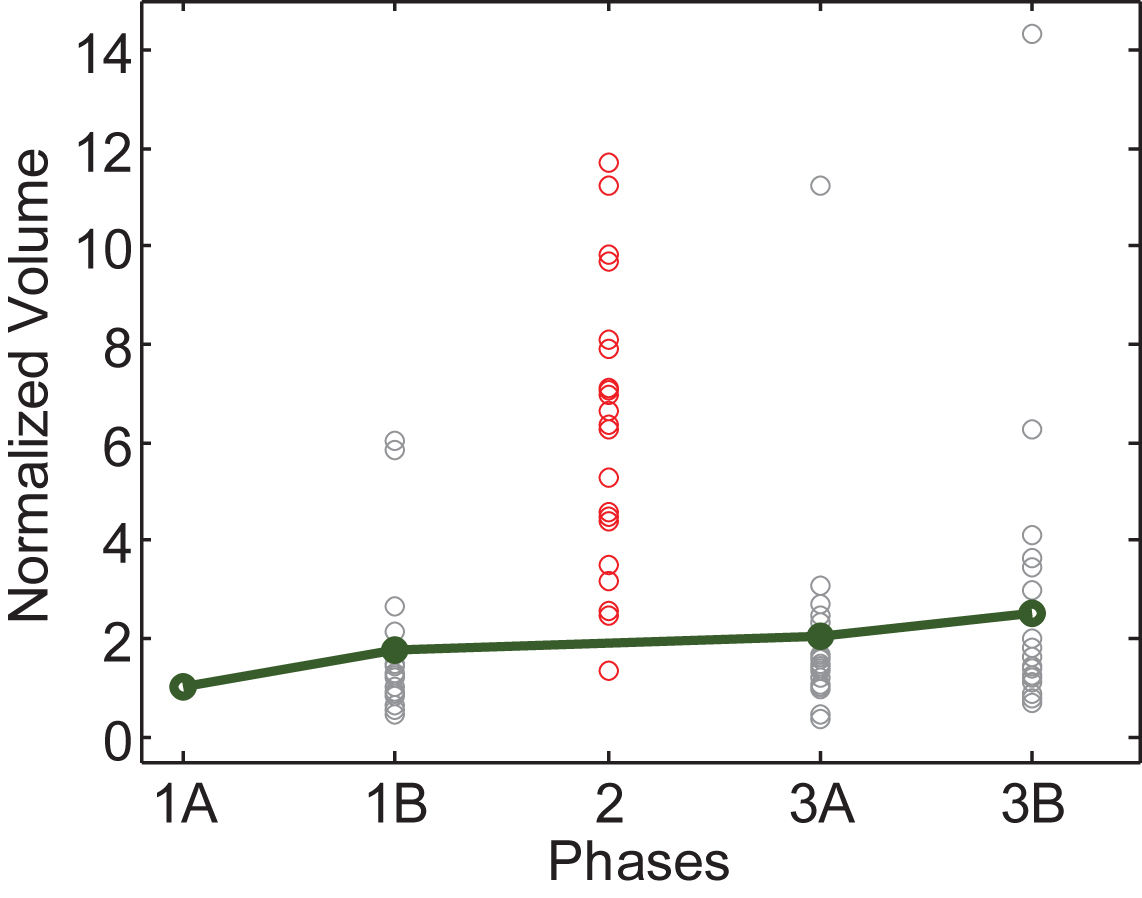
3.5Movement reversals
The number of movement reversals made during phase 1 was significantly larger than those made during phase 3 (t21 = 2.219, p < 0.05). On average, the number of movement reversals in phase 1 (9.9±2.6) was 1.6 greater than the number of reversals in phase 3 (8.3±2.2, 95% CI [0.100, 3.104]). In phase 2, the number of movement reversals was, on average 4.6±2.4. Figure 9 shows the average number of movement reversals made by participants in phase 1, in phase 2, and in phase 3. In phase 2 there were, on average, 5.3 less movement reversals than in phase 1, and 3.7 less movement reversals than in phase 3.
Fig.9
Number of movement reversals. The number of movement reversals per participant in the first human-led phase (Phase1), in the robot-led phase (phase 2), and in the second human-led phase (phase 3).
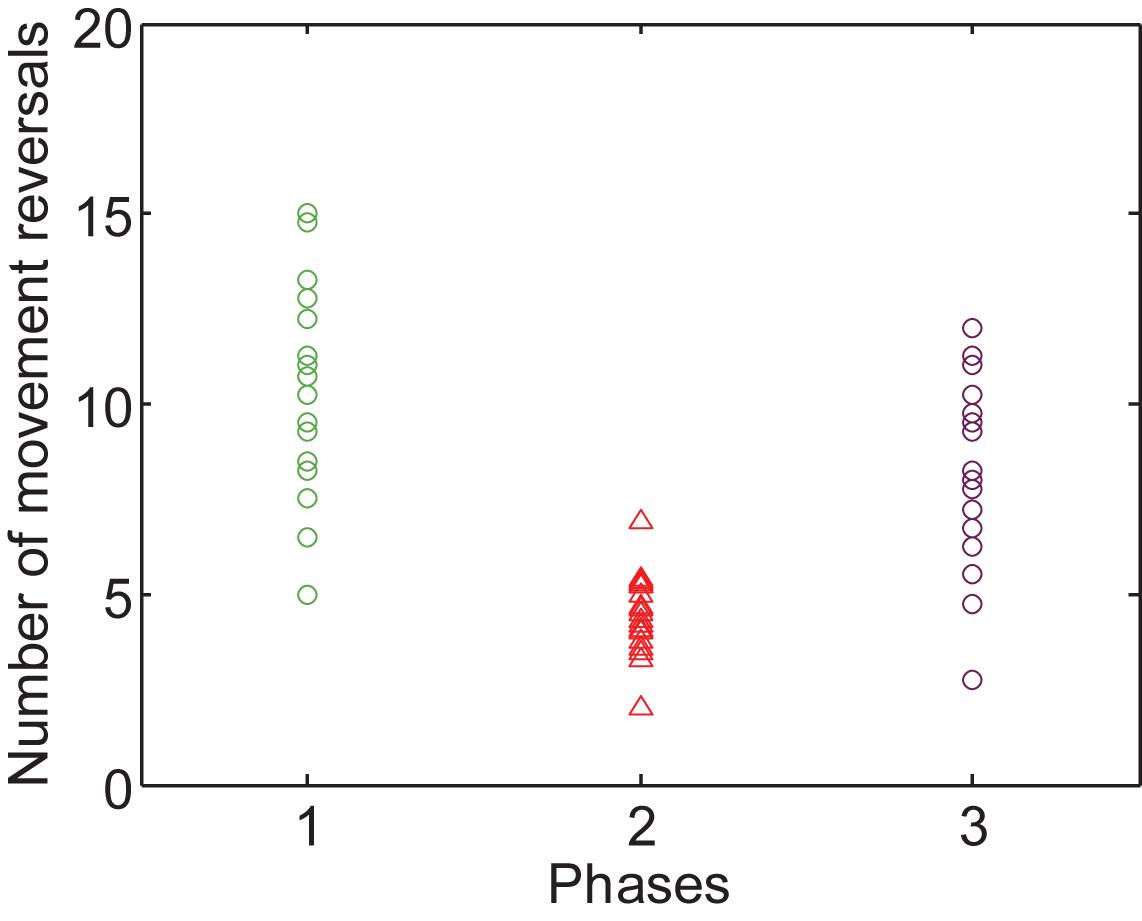
4Discussion
Our main goal in this study was to test user preferences when interacting with a robot on a joint-movement task, as a first step towards developing an interactive movement protocol to be used as part of a rehabilitation session.
4.1Robots in rehabilitation
Munih and Bajd (2011) suggested that motor rehabilitation was better after robotic assistance therapy than following conventional treatment. The effectiveness of robots in rehabilitation has also been demonstrated on hundreds of people with disabilities (Tejima, 2001), including following neurological damage such as stroke (Krebs et al., 2008) or spinal-cord injury (Crespo et al., 2009). Takahashi et al. (2008) highlights the importance of sensorimotor integration to motor learning after stroke and indeed their fMRI findings suggests that robotic therapy changed sensorimotor cortex function in a task-specific manner. As robots in the realm of rehabilitation become more prevalent, it is imperative to understand how to best motivate users to continue interacting with them over long periodsof time.
4.2Preferences
4.2.1Preferred movements
We hypothesized that the participants would prefer the smooth movements, i.e., the infinity sign, the circle and the cloud, since those movements are reminiscent of familiar humanlike movements. The results show this was the case for the first two shapes, but not for the latter. Of course, not all human movements are smooth, though there is an overall tendency to minimize jerk when performing movements of the upper arm (Flash & Hogan, 1985). We asked participants what were their reasons for preferring certain motions. Their verbal accounts revealed that recognizing the shape of the motion had much influence on selecting it as a favorite motion path. It appears many participants did not like the cloud shape because most of them did not recognize what this shape represented. Support for this finding can be found in Sciutti et al. (2015). They found that the ability to interact with other people hinges crucially on the possibility to anticipate how their actions would unfold. Human observers can detect different intentions and use them to deduct the intention which motivates the action (Sciutti et al., 2015) and from it, predict the motion path. Thus, it is likely that familiarity with the tracked shape in the current experiment correlated with increased preference due to their ability to predict the ensuing motion path. Other potential explanations for the motion preferences, which were not explored in the current experiment, include potentially different levels of energy expenditure in the different motion paths, or anatomical difficulties in performing some motions comparedto others.
We focused on subjective individual preferences, rather than on objective metrics, such as accuracy of following, since the goal of this work was to find the type of movements that participants are most motivated to engage in. Future studies could look at the contribution of biological movement pattern to user preferences (Noceti et al., 2015).
In this study we focused on user enjoyment and motivation when performing simple geometrical shapes. Future studies should look in to movements that carry their therapeutic value in the context of rehabilitation.
4.2.2Leading or following
The results show an even distribution between those participants who preferred to lead and those that preferred to follow. However, 16 out of the 22 participants complained that when they led, the robot did not always correctly perceive the location of their hand so it did not follow them perfectly. Other comments were that the robotic arm was following at too slow a pace. In addition, when they moved towards the robot it moved towards them, while they expected it to move back away from them. This was a design choice we made when programming the mirror game, though in retrospect it may have been better to do the opposite (maintain a set distance between the robotic hand and the participant’s hand). Due to the fact that more than 70% of the participants had technical comments about the leading section, it is possible that a modified version of the leading phase (better timing and constant distance from the user’s hand) may lead to increased preference of participantsto lead.
There were about 35% participants that said they would prefer the interaction with the robot involved voice and physical contact, suggesting people prefer an interaction that is more human-like.
4.3Robot primed human movement
In addition to understanding user preferences, it is important to study whether there is a priming effect by the robotic movement on the human movement, so that this effect can be taken into consideration when designing interactions with robots.
Priming can be manifest in a variety of forms. For example, the encounter between a storytelling robot and a child in which the robot tells stories that include extensive vocabulary and emotional expression, enriches the vocabulary of the children and makes their stories sounds more expressive and complex (Westlund et al., 2017). Other priming studies (Bargh et al., 1992; Fazio et al., 1986; Gillmeister et al., 2008; Hermans et al., 1994, 2001) have shown that the time needed to evaluate a congruent target stimulus (e.g., left arrow priming cue preceding a left button press) is significantly shorter than trials on which the priming cue and the target are incongruent (e.g., right arrow priming cue preceding a left button press) (Hermans et al., 2001). An example for movement priming was found by Gillmeister et al. (2008), who showed that performing a movement with a certain body part was faster after observing that body part move, than after observing a different body part. Priming can originate from various types of cues, including computer-generated human agents, who were found to prime movements of human participants (Kilteni et al., 2013). Participants were embodied in virtual reality (VR) by different avatars who were of varied races, and dressed differently, and were asked to play on a drum. The researchers found that the appearance of the avatar affected the performance on the drumming task (Kilteniet al., 2013).
More recently, movement priming between humans and robots was also investigated (Eizicovits et al. unpublished data, Oberman et al., 2007; Pierno et al., 2008; Press et al., 2005). Oberman et al., showed, using EEG recording, that robotic movements activate the human mirror neuron system (Oberman et al., 2007). Pierno et al., found that interaction with robots had an effect on visuomotor priming processes only for children with autism and not for typically developed children (Pierno et al., 2008). We showed in a recent experiment a demonstration of movement priming by a robotic arm; Participants, both young and old, performed movements that were significantly slower when playing with a slow robotic arm, compared to when playing with a fast, non-embodied, system (Eizicovits et al., unpublished data).
Movement priming in the current experiment manifest itself as an increase in both an increase in the volume of movement and a decrease in the number of movement reversals after the participants followed the robot-led movements.
4.3.1Volume
We found that the volume of the movements participants made in phase 3, after they followed the robotic arm in phase 2, was significantly larger than that in phase 1. As shown in Fig. 8, there was a continuous trend of increase in the average movement volume. It appears that the movement of the robotic arm primed the participants’ movements. Another potential explanation for the continuous trend of movement-volume increase is that participants started feeling more at ease with leading the robot as they gained more experience doing that, which led to them increasing the explored volume with more comfort.
4.3.2Movement reversals
We found that the number of movement reversals made during phase 3 was significantly smaller than that made during phase 1. A smaller number of movement reversals suggests a more continuous, smoother movement, as can be qualitatively seen in Fig. 7.
The evidence we present here, suggesting the robotic arm’s movement primed the movements of the participants, contrasts with recent behavioral and neuroimaging studies, which found that observation of human movement, but not of robotic movement, gives rise to visuomotor priming (Press et al., 2005; Tai et al., 2004). Tai et al., show that PET scanning has detected significant activation in the left premotor cortex, where the mirror neurons are presumed to reside, when human participants observed manual grasping actions performed by a human model, but not when they were performed by a robotic arm (Tai et al., 2004). Other studies found that watching a human perform an action resulted in a shorter reaction time than when seeing a robot perform the same action (Press et al., 2005; Wiese et al., 2012). The seeming contradiction between our findings and those of the previous studies may stem from different reasons in each case. The Press et al. study used still images, whereas we used an embodied, physically present robot. The physical presence may play an important role in movement priming by a robot. Tai et al. (2004) suggest that the mirror neurons of the premotor cortex appear to be biologically tuned. In their robot condition, the participants observed the robot preforming non-biological movements, whereas we included a range of movement shapes that included smooth movements, reminiscent of biological movements. This may have underlied the priming of the participants’ movements following the robot-led condition in the current experiment.
The results we report here are encouraging, as movement priming by the robot may be harnessed for rehabilitation, if the robot can induce the user to perform desirable movements.
4.4Future work
In the future, we expect the socially assistive robotic paradigm to eventually improve motor learning and motor rehabilitation beyond the capabilities of conventional methods, since it will enhance the motivational aspect, which can then lead to physical improvement.
This experiment demonstrates the contribution of robotic motion paths to the user preferences within a collaborative human-robot interaction, and the interpersonal differences (e.g., preference to lead or to follow), which together highlight the importance of personalized human-robot interactions. When designing an exercise program, for example, in which it is important to maintain the user’s motivation to complete the program, it would be beneficial to take into account the individual preferences for motion paths and for leading or following during the long-term interaction.
Acknowledgments
The authors wish to thank Amit Loutati for help with the experimental design, and Yoav Raanan for help with programming the robotic movements. The authors gratefully acknowledge funding by the Leir Foundation; the Bronfman Foundation; the Promobilia Foundation; The Israeli Science Foundation (grants # 535/16 and 2166/16); and by the Helmsley Charitable Trust through the Agricultural, Biological and Cognitive Robotics Initiative and by the Marcus Endowment Fund, both at Ben-Gurion University of the Negev.
References
1 | Bargh J.A. ((1992) ). The ecology of automaticity: Toward establishing the conditions needed to produce automatic processing effects, The American Journal of Psychology, 181–199. |
2 | Bartneck C. , Kulić D. , Croft E. , & Zoghbi S. ((2009) ). Measurement instruments for the anthropomorphism, animacy, likeability, perceived intelligence, and perceived safety of robots, International Journal of Social Robotics, 1: (1), 71–81. |
3 | Brochard S. , Robertson J. , Médée B. , & Remy-Neris O. ((2010) ). What’s new in new technologies for upper extremity rehabilitation? Current Opinion in Neurology, 23: (6), 683–687. |
4 | Brooks A.G. , Gray J. , Hoffman G. , Lockerd A. , Lee H. , & Breazeal C. ((2004) ). Robot’s play: Interactive games with sociable machines, Computers in Entertainment (CIE), 2: (3), 10–10. |
5 | Carpintero E. , Perez C. , Morales R. , Garcia N. , Candela A. , & Azorin J.M. ((2010) ). Development of a robotic scrub nurse for the operating theatre. In Biomedical Robotics and Biomechatronics (BioRob), 2010 3rd IEEE RAS and EMBS International Conference on IEEE, 504–509. |
6 | Chartrand T.L. , & Bargh J.A. ((1999) ). The chameleon effect: The perception–behavior link and social interaction, Journal of Personality and Social Psychology, 76: (6), 893. |
7 | Colombo R. , Pisano F. , Mazzone A. , Delconte C. , Micera S. , Carrozza M.C. , ...& Minuco G. ((2007) ). Design strategies toimprove patient motivation during robot-aided rehabilitation, Journal of Neuroengineering and Rehabilitation, 4: (1), 3. |
8 | da Silva Cameirão M. , Bermúdez i Badia S. , Duarte E. , & Verschure P.F. ((2011) ). Virtual reality based rehabilitationspeeds up functional recovery of the upper extremities afterstroke: A randomized controlled pilot study in the acute phase ofstroke using the rehabilitation gaming system, RestorativeNeurology and Neuroscience, 29: (5), 287–298. |
9 | Eizicovits D. , Edan Y. , Tabak I. , & Levy-Tzedek S. “Robotic gaming prototype for upper limb rehabilitation: Effects of age and embodiment on user preferences and movement characteristics” (unpublished data). |
10 | Eriksson J. , Mataric M.J. , & Winstein C.J. ((2005) ). Hands-off assistive robotics for post-stroke arm rehabilitation, In Rehabilitation Robotics, 2005. ICORR 2005. 9th International Conference on IEEE, 21–24. |
11 | Fasola J. , & Mataric M. ((2013) ). A socially assistive robot exercise coach for the elderly, Journal of Human-Robot Interaction, 2: (2), 3–32. |
12 | Fazio R.H. , Sanbonmatsu D.M. , Powell M.C. , & Kardes F.R. ((1986) ). On the automatic activation of attitudes, Journal of Personality and Social Psychology, 50: (2), 229. |
13 | Feil-Seifer D. , & Mataric M.J. ((2005) ). Defining socially assistive robotics, In Rehabilitation Robotics, 2005. ICORR 2005. 9th International Conference on IEEE, 21–24. |
14 | Flash T. , & Hogan N. ((1985) ). The coordination of arm movements: An experimentally confirmed mathematical model, Journal of Neuroscience, 5: (7), 1688–1703. |
15 | Gueugnon M. , Salesse R.N. , Coste A. , Zhao Z. , Bardy B.G. , & Marin L. ((2016) ). The acquisition of socio-motor improvisation in the mirror game, Human Movement Science, 46: , 117–128. |
16 | Gillmeister H. , Catmur C. , Liepelt R. , Brass M. , & Heyes C. ((2008) ). Experience-based priming of body parts: A study of action imitation, Brain Research, 1217: , 157–170. |
17 | Hart Y. , Noy L. , Feniger-Schaal R. , Mayo A.E. , & Alon U. ((2014) ). Individuality and togetherness in joint improvised motion, PLoS One, 9: (2), e87213. |
18 | Hasler B.S. , Spanlang B. , & Slater M. ((2017) ). Virtual racetransformation reverses racial in-group bias, PLoS One, 12: (4), e0174965. |
19 | Hermans D. , & De Houwer J. ((1994) ). Affective and subjective familiarity ratings of 740 Dutch words, Psychologica Belgica, 34: (2-3), 115–139. |
20 | Hermans D. , De Houwer J. , & Eelen P. ((2001) ). A time course analysis of the affective priming effect, Cognition & Emotion, 15: (2), 143–165. |
21 | Hillman M. ((1998) ). Introduction to the special issue on rehabilitation robotics, Robotica, 16: (5), 485–485. |
22 | Jacobs A. , Timmermans A. , Michielsen M. , Vander Plaetse M. , & Markopoulos P. ((2013) ). CONTRAST: Gamification of arm-hand training for stroke survivors, In CHI’13 Extended Abstracts on Human Factors in Computing Systems ACM, 415–420. |
23 | Kang K.I. , Freedman S. , Mataric M.J. , Cunningham M.J. , & Lopez B. ((2005) ). A hands-off physical therapy assistance robot for cardiac patients. In Rehabilitation Robotics, 2005. ICORR 2005. 9th International Conference on IEEE, 337–340. |
24 | Kilteni K. , Bergstrom I. , & Slater M. ((2013) ). Drumming in immersive virtual reality: The body shapes the way we play, IEEE Transactions on Visualization and Computer Graphics, 19: (4), 597–605. |
25 | Knight J. ((2011) ). What good coaches do, Educational Leadership, 69: (2), 18–22. |
26 | Krebs H.I. , Dipietro L. , Levy-Tzedek S. , Fasoli S.E. , Rykman-Berland A. , Zipse J. , ...& Volpe B.T. ((2008) ). A paradigm shift for rehabilitation robotics, IEEE Engineering in Medicine and Biology Magazine, 27: (4), 61–70. |
27 | Levy-Tzedek S. , Berman S. , Stiefel Y. , Sharlin E. , Young J. , & Rea D. ((2017) ). Robotic Mirror Game for movement rehabilitation. In Virtual Rehabilitation (ICVR), 2017 International Conference on IEEE, 1–2. |
28 | Li C. , Rusák Z. , Horváth I. , Ji L. , & Hou Y. ((2014) ). Current status of robotic stroke rehabilitation and opportunities for a cyber-physically assisted upper limb stroke rehabilitation. In Proceedings of the 10th International Symposium on Tools and Methods of Competitive Engineering TMCE 2014, Budapest, Hungary, May 19–23. |
29 | Lo A.C. , Guarino P.D. , Richards L.G. , Haselkorn J.K. , Wittenberg G.F. , Federman D.G. , et al. ((2010) ). Robot-assisted therapy for long-term upper-limb impairment after stroke, New England Journal of Medicine, 362: (19), 1772–1783. |
30 | Maciejasz P. , Eschweiler J. , Gerlach-Hahn K. , Jansen-Troy A. , & Leonhardt S. ((2014) ). A survey on robotic devices for upper limb rehabilitation, Journal of Neuroengineering and Rehabilitation, 11: (1), 3. |
31 | Marchal-Crespo L. , & Reinkensmeyer D.J. ((2009) ). Review of control strategies for robotic movement training after neurologic injury, Journal of Neuroengineering and Rehabilitation, 6: (1), 20. |
32 | Martin A. , González J.C. , Pulido J.C. , García-Olaya Á. , Fernández F. , & Suárez C. ((2015) ). Therapy monitoring and patient evaluation with social robots. In Proceedings of the 3rd 2015 Workshop on ICTs for Improving Patients Rehabilitation Research Techniques ACM, 152–155. |
33 | Munih M. , & Bajd T. ((2011) ). Rehabilitation robotics, Technology and Health Care, 19: (6), 483–495. |
34 | Noceti N. , Sciutti A. , & Sandini G. ((2015) ). Cognition helps vision: Recognizing biological motion using invariant dynamic cues, In International Conference on Image Analysis and Processing, 676–686. |
35 | Noy L. , Dekel E. , & Alon U. ((2011) ). The mirror game as aparadigm for studying the dynamics of two people improvising motiontogether, Proceedings of the National Academy of Sciences, 108: (52), 20947–20952. |
36 | Oberman L.M. , McCleery J.P. , Ramachandran V.S. , & Pineda J.A. ((2007) ). EEG evidence for mirror neuron activity during the observation of human and robot actions: Toward an analysis of the human qualities of interactive robots, Neurocomputing, 70: (13), 2194–2203. |
37 | Pierno A.C. , Mari M. , Lusher D. , & Castiello U. ((2008) ). Robotic movement elicits visuomotor priming in children with autism, Neuropsychologia, 46: (2), 448–454. |
38 | Potkonjak V. , Tzafestas S. , Kostic D. , & Djordjevic G. ((2001) ). Human-like behavior of robot arms: General considerations and the handwriting task— Part I: Mathematical description of human-like motion: Distributed positioning and virtual fatigue, Robotics and Computer-Integrated Manufacturing, 17: (4), 305–315. |
39 | Press C. , Bird G. , Flach R. , & Heyes C. ((2005) ). Robotic movement elicits automatic imitation, Cognitive Brain Research, 25: (3), 632–640. |
40 | Rizzolatti G. , Fadiga L. , Gallese V. , & Fogassi L. ((1996) ). Premotor cortex and the recognition of motor actions, Cognitive Brain Research, 3: (2), 131–141. |
41 | Shimada M. , Yamauchi K. , Minato T. , Ishiguro H. , & Itakura S. ((2008) ). Studying the Influence of the Chameleon Effect on Humans using an Android. In Intelligent Robots and Systems, 2008. IROS 2008. IEEE/RSJ International Conference on IEEE, 767–772. |
42 | Stoykov M.E. , Corcos D.M. , & Madhavan S. ((2017) ). Movement-Based Priming: Clinical Applications and Neural Mechanisms, Journal of Motor Behavior, 49: (1), 88–97. |
43 | Stein J. ((2012) ). Robotics in rehabilitation: Technology as destiny, Am J Phys Med Rehabil, 91: (11), 199–203. |
44 | Sciutti A. , Ansuini C. , Becchio C. , & Sandini G. ((2015) ). Investigating the ability to read others’ intentions using humanoid robots, Frontiers in Psychology, 6: . |
45 | Takahashi C.D. , Der-Yeghiaian L. , Le V. , Motiwala R.R. , & Cramer S.C. ((2007) ). Robot-based hand motor therapy after stroke, Brain, 131: (2), 425–437. |
46 | Tapus A. , Maja M. , & Scassellatti B. ((2007) ). The grand challenges in socially assistive robotics, IEEE Robotics and Automation Magazine, 14: (1), 35. |
47 | Tejima N. ((2001) ). Rehabilitation robotics: A review, Advanced Robotics, 14: (7), 551–564. |
48 | Wiese E. , Wykowska A. , Zwickel J. , & Müller H.J. ((2012) ). I see what you mean: How attentional selection is shaped byascribing intentions to others, PLoS One, 7: (9), e45391. |
49 | Westlund K. , Jacqueline M. , Jeong S. , Park H.W. , Ronfard S. , Adhikari A. , ...& Breazeal C.L. ((2017) ). Flat vs. expressivestorytelling: Young children’s learning and retention of a social robot’s narrative, Frontiers in Human Neuroscience, 11: , 295. |
50 | Zhai C. , Alderisio F. , Tsaneva-Atanasova K. , & Di Bernardo M. ((2014) ). A novel cognitive architecture for a human-like virtual player in the mirror game. In Systems, Man and Cybernetics (SMC), IEEE International Conference on IEEE, 754–759. |