What Does ChatGPT Know About Dementia? A Comparative Analysis of Information Quality
Abstract
The quality of information about dementia retrieved using ChatGPT is unknown. Content was evaluated for length, readability, and quality using the QUEST, a validated tool, and compared against online material from three North American organizations. Both sources of information avoided conflicts of interest, supported the patient-physician relationship, and used a balanced tone. Official bodies but not ChatGPT referenced identifiable research and pointed to local resources. Users of ChatGPT are likely to encounter accurate but shallow information about dementia. Recommendations are made for information creators and providers who counsel patients around digital health practices.
INTRODUCTION
Information about Alzheimer’s disease (AD) and other dementias is increasingly being accessed online by persons with lived experience, as well as their caregivers, family members, and friends [1–4]. Higher quality sources tend to have balanced information, relevant health information sources, and discuss modifiable risk factors [5, 6]. Lower quality dementia information sources may contain misleading information and fail to adhere to ethical principles, such as informed consent [5, 7, 8]. For example, online tests intended to diagnose AD have low validity and low alignment with ethical standards in medicine [9]. As new online sources of dementia information continue to emerge, it is important to consider whether these sources are truly beneficial for people living with dementia and their families [8].
In November 2022, OpenAI launched “ChatGPT” (Conversational Generative Pre-training Transformer), a large language model, for public use for a period of “research preview” [10]. ChatGPT is an online platform that allows anyone to input text and receive newly generated conversation-like responses. Underlying the platform is an artificial neural network that has been fine-tuned through human feedback [10, 11]. The service has been explosively popular, reaching 100 million unique users within two months [12]. Many potential applications have been identified for this tool in the health domain including streamlining workflows, triaging patients, and reducing research labor [13, 14]. However, there are concerns about information security, harmful applications (e.g., plagiarism, identity theft, misinformation), and inaccurate outputs, colloquially referred to as “hallucinations” [15, 16].
According to one systematic review from March 2023, the quality of medical information in ChatGPT responses is moderate but too unreliable for use in a clinical setting [17]. One early study finds that TikTok users are skeptical of the credibility of health information originating from ChatGPT [18] but, on the whole, the available data tends to prioritize the physician or researcher’s perspective. Research at the intersection of dementia and Large Language Models is evolving rapidly, with promising potential applications. For example, GPT-3 can determine an individual’s dementia diagnosis from a speech sample [19]. However, information on how patients and families may be using chatbot platforms to seek out health information for themselves, or what they may find when they do, is still emerging. Recently, Hristidis and colleagues compared ChatGPT responses to google query results for questions about dementia and cognitive decline [20]. For prompts, these authors’ questions focused on information about AD, derived from the Alzheimer’s Disease Knowledge Scale, and questions focused on obtaining services and support. They found that ChatGPT performed poorly on providing time-stamped and clearly sourced information, while Google results sometimes represented commercial entities. Information retrieved from both sources scored poorly on readability. In other work, clinicians judged ChatGPT responses to AD and dementia queries to be satisfactory with some limitations [21], as did formal and informal caregivers of persons living with dementia [22].
In the present work, we ask: what is the quality of AD and dementia information encountered by users of ChatGPT? In particular, how does this information compare to vetted information from non-commercial entities with expertise in AD and dementia? Our objective is to emulate a naïve user’s search strategy when initially interfacing with ChatGPT, and to compare the quality of material obtained from the Large Language Model to comparable public-facing materials from AD-related organizations in North America. We selected prompts that were intended to simulate queries from a non-expert, based on statements that had been included on these organizations’ “Frequently asked questions” pages. We applied a validated scale for online health information to understand, in a granular way, the strengths and weaknesses of these two information sources. Similar methodologies have recently been used to assess the quality of other types of health information in response to common questions posed to ChatGPT, including total joint arthroplasty, cirrhosis and hepatocellular carcinoma, amyloidosis, and gastrointestinal health [23–26].
MATERIALS AND METHODS
Prompt selection
We collected a list of questions about AD and dementia from three organizations based in three North American countries: Canada, Mexico, and the USA. One organization per country was selected according to the following criteria: 1) operates at a national level; 2) focused on AD and dementia, rather than broader topics such as aging or health; 3) hosts a website accessible through search engines with freely available content in a question-and-answer format. Where more than one organization existed in a single country, the largest (in terms of annual revenue) was selected. Google Translate in Google Chrome was used to translate the FEDMA webpage into English, and information was then saved in its English format for further analysis.
Generation of ChatGPT responses
Questions were collected from all three organizations (Alzheimer Society of Canada n = 8, FEDMA n = 10, Alzheimer’s Association n = 2). Two duplicates were removed, leading to a final pool of 18 questions. These questions were pooled together in a randomized order (see Supplementary Methods) and presented as originally written in English or in their post-translation English format in one batch to the free version of ChatGPT (ChatGPT-3.5) available on April 11, 2023. We used an account linked to a newly-created email account, a newly-created phone number with a Canadian area code, and a Google Chrome Incognito browser window with two tracker-blocking extensions enabled: Privacy Badger and uBlock Origin [27, 28].
Analysis
Eight sets of responses were prepared for coding. For each of the three organizations, responses to the questions were extracted from the body text of their websites, excluding any graphics or offset boxes of additional information (n = 3 sets: Org_CA, Org_MX, Org_US). A pooled set of responses from all organizations was prepared (n = 1 set: Org_Pooled). From the set of ChatGPT responses, the questions and answers matched to each organization’s website were extracted, forming three sets of responses (n = 3 sets: Chat_CA, Chat_MX, Chat_US). The pooled batch of ChatGPT responses was also analysed as a whole (n = 1 set: Chat_Pooled).
Each set of responses was coded by two independent coders using the QUality Evaluation Scoring Tool (QUEST). The QUEST is a validated scale to evaluate the quality of online health information [29]. It has seven criteria: authorship, attribution, type of study, conflict of interest, currency, complementarity, and tone. Total QUEST scores can range from zero to 28, and higher scores represent higher quality of information. Each point on the QUEST is meaningful, with no standard cut-off score. For example, an overall score of 21 indicates higher quality than an overall score of 20. The complementarity score can range from zero to one. Scores on the authorship, currency, and type of study criteria can range from zero to two. Scores on the attribution, conflict of interest, and tone criteria can range from zero to six. The ‘type of study’ criteria is only assessed if articles score 2 or 3 on attribution. The two coders reached an initial inter-rater agreement of 83%, and differences in scores were resolved through discussion between coders and consultation with a third member of the research team if necessary. After discussion coders were in perfect agreement about the final scores. Word counts and Flesch-Kincaid readability of responses were calculated using analytic tools available through Microsoft Word. The Flesch-Kincaid Grade Level test yields the U.S. school grade level at which a text can be understood. Lower scores indicate a better readability [30].
RESULTS
The text from organizations varied in length and may have been written with different audiences in mind; responses from FEDMA (Mexico) were shorter on average than responses from the Alzheimer Society (Canada), and both organizations had responses that were much shorter than the responses from the Alzheimer’s Association (USA).
In the QUEST instrument, scores are typically assigned to the entire body of a text rather than to single questions, sentences, or paragraphs. Scores for each organization’s responses as well as ChatGPT responses to these same questions can be found in Table 1. When analyzed as a single pool, the set of responses written by the three AD organizations (Org_Pooled) received a total QUEST score of 21 out of a possible 28 points. ChatGPT responses to the same questions (Chat_Pooled) received a score of 16. Scores for individual criteria in the QUEST are depicted in Fig. 1. All organization and ChatGPT sets of responses received full points for Conflict of Interest (Org_CA, Org_MX, Org_US, Chat_CA, Chat_MX, Chat_US; “unbiased information,” absence of product endorsements). Most organization and ChatGPT responses received the one available point for Complementarity (Org_CA, Org_MX, Org_US, Chat_CA, Chat_MX, but not Chat_US; “support of the patient-physician relationship”). All organizations and 1/3 ChatGPT responses received full points for tone (Org_CA, Org_MX, Org_US, and Chat_MX; “Balanced/cautious support” of claims). Two ChatGPT responses (Chat_CA, Chat_US) received a score of three (no discussion of limitations of claims). Finally, one organization received a score of zero for Attribution (Org_MX; no sources), one received a score of three (Org_CA; scientific research was available through links), and one received a score of six (Org_US; the text referred to identifiable scientific studies). This score also triggered the coding of Type of Study, on which the organization (Alzheimer’s Association, USA) received an additional score of two (studies were clinical in nature). All organization and ChatGPT text responses (Org_CA, Org_MX, Org_US, Chat_CA, Chat_MX, Chat_US) received scores of zero for Authorship (“author’s name and qualifications clearly stated” scores full points) and Currency (“article is dated within the last 5 years” scores full points).
Fig. 1
QUEST scores for each coded text. QUEST Items “Authorship” and “Currency” were excluded because all texts received scores of zero. “Type of Study” is only coded if “Attribution” score is six or more. Higher scores on each item indicate higher quality.
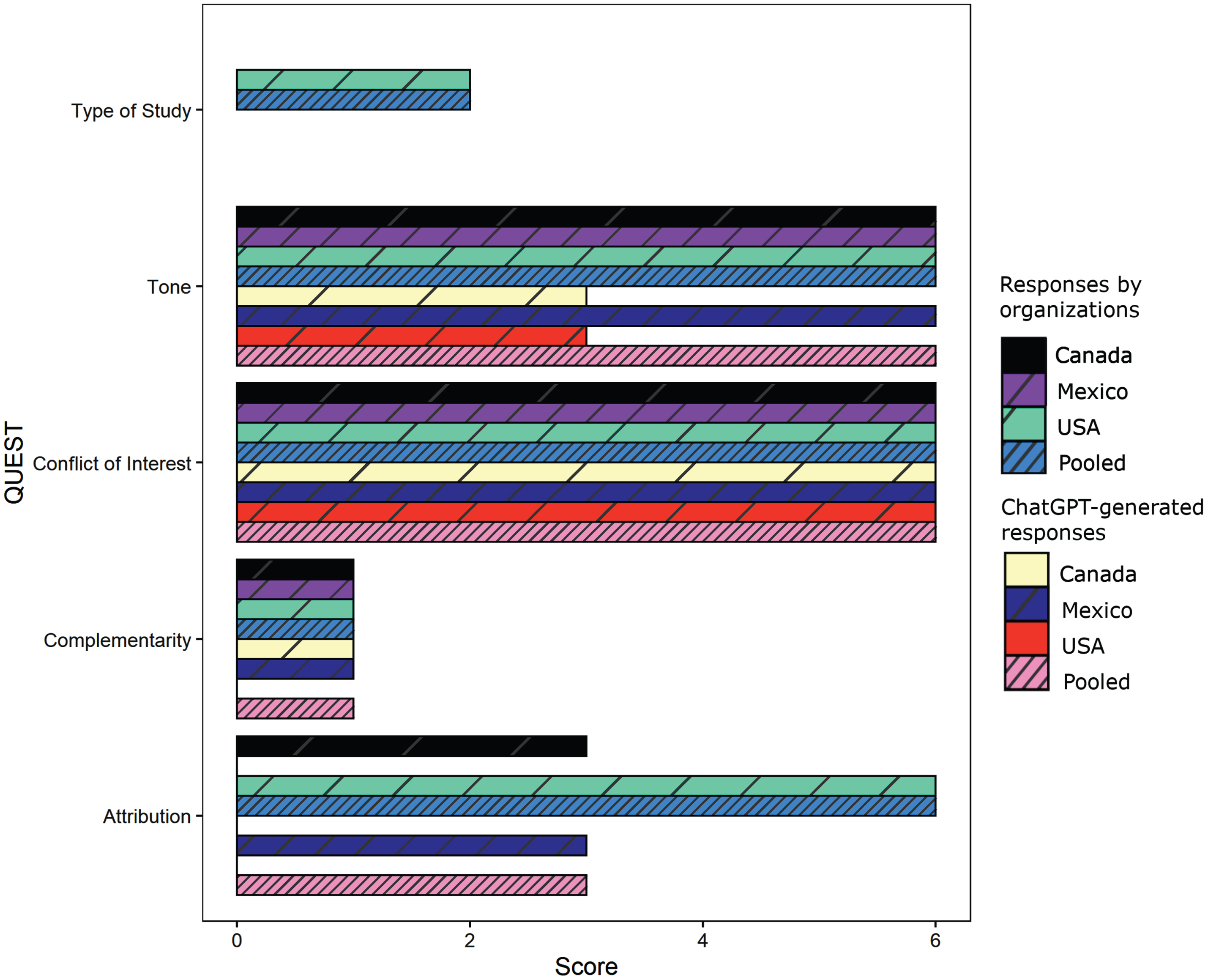
Table 1
Characteristics of text responses from across sources
Source of questions | Source of | QUEST | Flesch-Kincaid | Average words |
responses | Total1 | Grade Level2 | per response | |
Alzheimer Society (CA) | Organization | 16 | 9.0 | 112 |
Federacion Mexicana de Alzheimer (MX) | Organization | 13 | 12.33 | 904 |
Alzheimer’s Association (US) | Organization | 21 | 11.2 | 905 |
Pooled | Organization | 21 | 10.9 | 185 |
Alzheimer Society (CA) | ChatGPT | 10 | 12.9 | 169 |
Federacion Mexicana de Alzheimer (MX) | ChatGPT | 16 | 14.3 | 190 |
Alzheimer’s Association (US) | ChatGPT | 9 | 13.7 | 193 |
Pooled | ChatGPT | 16 | 13.7 | 181 |
FEDMA (MX) text was auto-translated from Spanish to English before coding. 1Higher scores indicate higher quality of information. 2Lower scores indicate better readability. 3Score of 17.9 in the original Spanish. 4An average of 96 words per response in the original Spanish.
Text responses from organizations had a lower Flesch-Kincaid Grade Level, or better readability, than text responses from ChatGPT for the whole pooled texts (i.e., Org_Pooled versus Chat_Pooled) as well as each of the three sets of text for each country (i.e., Org_CA versus Chat_CA, Org_MX versus Chat_MX, Org_US versus Chat_US). In other words, Canadian answers to Canadian questions received a lower Flesch-Kincaid Grade Level, or higher readability, than ChatGPT answers to Canadian questions, and so on. This pattern is not driven by the multi-syllabic words “Alzheimer” or “dementia”; it holds true if these are removed. ChatGPT responses consistently averaged around 170–190 words per answer, while responses from AD organizations were more varied (90–905 words).
DISCUSSION
At present, when a non-expert user accesses information about AD and other dementias using the ChatGPT chatbot, they are likely to find information that is similar in quality to content originating from North American AD organizations, but with critical differences. ChatGPT, like official channels, avoids endorsing specific products and instructs the reader to speak with a physician about questions and concerns. However, AD organizations were more likely than ChatGPT to explicitly state the limits of current scientific knowledge, and to use more accessible language. They also referred or linked to specific, identifiable scientific literature, while ChatGPT made no such statements, and other work finds it to be unreliable when it does refer to specific research findings [15, 16, 31]. Although organization responses had a better readability than ChatGPT responses, none of the sets of responses achieved the desired readability level for public-facing patient health information (< grade 6) [32]. We suggest that efforts be made to improve the readability of health information sources online so that patients can derive maximum benefit from these resources.
This paper focused on the experience of users who may access ChatGPT to answer their questions about AD and other dementias. However, this research has further implications for clinical practice and research. Some individuals view the internet as a highly accessible source of dementia information, especially when a healthcare professional is unavailable [1], and ChatGPT is another popular resource that individuals may use to get answers to their questions [12]. ChatGPT’s accessibility may contribute to improved health literacy, but may also facilitate the spread of misinformation among users, influence decision-making in healthcare, and expose users to biases in the information provided [14]. Evidence-based guidelines are urgently needed to ensure that ChatGPT is used in a way that benefits patients, healthcare delivery and research progress.
We simulated the experience of a naïve, English-speaking North American user accessing ChatGPT, rather than using a variety of prompts to probe all possible answers from the chatbot. This is a limitation of the study, as the program can give different responses to the same question at different timepoints and is sensitive to prompt wording; it may even reverse yes-and-no answers about health material [33]. However, based on preliminary tests conducted by the authors to inform the final methodology, answers to simple questions tended to be somewhat shallow and repetitive, as others have reported [34]. The text we sourced from the three organizations was not perfectly matched; for example, the answers from the Alzheimer’s Association were in the format of longer narratives, while responses on the FEMDA site were in the format of a brief FAQ page, and these may be intended for slightly different audiences. For these reasons, the three sources should not be directly compared to one another but rather taken as examples of the types of communications national AD organizations may offer. Furthermore, we did not capture online information from provincial- or state-level organizations, nor from national health websites that are broader in scope (e.g., National Institute on Aging). Our data represents one snapshot of a user’s potential search behavior when seeking AD and dementia online but does not comprehensively cover all possible results in this scenario.
Another limitation of this work was the use of an auto-translated version of the FEDMA (Mexico) page, which may have introduced slight changes in meaning that could have affected certain scoring criteria, despite Google Translate software’s high accuracy for Spanish-to-English translations [35]. Average words per response was not meaningfully altered in the translation process (English: mean of 90 words per response; Spanish: 96 words). Readability in the original Spanish was lower (i.e., higher grade level) than the English translation. Translation differences are unlikely to affect the QUEST elements that are operationalized with concrete features that are either present or absent, such as the inclusion of sources (Attribution) or statements of support for the physician-patient relationship (Complementarity). However, the Tone score should be interpreted with some caution. We believe that, on balance, the inclusion of the FEDMA data is still valuable and aligns with the results from the Canadian and US sources.
Importantly, official AD sites contained high-quality information that was not captured by the QUEST scale, including links to resources about stigma, recommendations about person-first language, resources for care partners, and contact information for users to connect with local and in-person resources. ChatGPT responses did not include these types of information.
Finally, as part of our analysis, we evaluated all organization responses as a pooled text. Although analyzing pooled responses serves as a method to compare the overall quality of organization and ChatGPT-generated information, we cannot exclude the possibility that the quality of ChatGPT answers might have differed when presented with questions from one source at a time.
Our results align with prior work comparing dementia information from ChatGPT to general Google search queries which found that ChatGPT information had a low readability and a lack of sources or timestamps [20]. The unique contributions of the present work are the comparison specifically to non-partisan, non-profit websites as high-quality sources of online information and the use of a validated scale for a multi-dimensional evaluation of content quality. Specifically, our findings that ChatGPT did provide statements of support for the patient-physician relationship, did not endorse specific products, and failed to discuss limitations of claims are all novel.
The QUEST instrument places value on the presence of authorship and currency information and references to identifiable high-quality scientific literature of particular types. We found that both ChatGPT and the organization websites did not provide author/source identities or credentials, nor did they provide timestamped information. ChatGPT in the form we examined here does not prioritize these things and seldom includes them unless explicitly instructed by prompting. This reflects the methods used to construct Large Language Models and their priorities; outputs represent learned patterns from the training data, rather than traceable, clearly sourced statements with a human author that is accountable for making them. This is different from the potential goals of an organization, and non-profit health bodies may want to take advantage of their ability to increase the transparency of their information along these dimensions as a feature that sets them apart from Large Language Models.
Healthcare providers should keep the strengths and limitations of ChatGPT in mind as they counsel patients on whether to use this new resource and how to do so appropriately. Based on this early evidence, persons living with AD and other dementias will find mostly correct but shallow information and will not be connected to the local and specific resources they may need. The tool is likely to rapidly evolve, and its future impact on patients and families will require ongoing exploration. The types of information accessed by patients, the impact that this has on real health decision-making behaviors, and the future of Large Language Models are all outstanding and urgent questions.
ACKNOWLEDGMENTS
The authors have no acknowledgments to report.
FUNDING
This work was supported by the Alzheimer’s Association, AGE-WELL NCE., The Canadian Consortium on Neurodegeneration in Aging, and the BC Children’s Hospital Foundation.
CONFLICT OF INTEREST
JMR received grant funding from the Alzheimer’s Association, one of the three national organizations studied in this manuscript, for a project on the topic of social media and dementia research.
DATA AVAILABILITY
Data from ChatGPT is publicly accessible, though note that the program may not yield the same answer to prompts. The specific data supporting the findings of this study are available on request from the corresponding author.
SUPPLEMENTARY MATERIAL
[1] The supplementary material is available in the electronic version of this article: https://dx.doi.org/10.3233/JAD-230573.
REFERENCES
[1] | Allen F , Cain R , Meyer C ((2020) ) Seeking relational information sources in the digital age: A study into information source preferences amongst family and friends of those with dementia, Dementia 19: , 766–785. |
[2] | Dixon E , Anderson J , Blackwelder D , Radnofsky M , Lazar A ((2022) ) Barriers to online dementia information and mitigation. Proc SIGCHI Conf Hum Factors Comput Syst CHI Conf 2022: , 513. |
[3] | Kernisan LP , Sudore RL , Knight SJ ((2010) ) Information-seeking at a caregiving website: A qualitative analysis, J Med Internet Res 12: , e1548. |
[4] | Soong A , Au ST , Kyaw BM , Theng YL , Tudor Car L ((2020) ) Information needs and information seeking behaviour of people with dementia and their non-professional caregivers: A scoping review. BMC Geriatr 20: , 61. |
[5] | Robillard JM , Feng TL ((2016) ) Health advice in a digital world: Quality and content of online information about the prevention of Alzheimer’s disease. J Alzheimers Dis 55: , 219–229. |
[6] | Robillard JM , Johnson TW , Hennessey C , Beattie BL , Illes J ((2013) ) Aging 2.0: Health information about dementia on Twitter, PLoS One 8: , e69861. |
[7] | Robillard JM ((2016) ) The online environment: A key variable in the ethical response to complementary and alternative medicine for Alzheimer’s disease. J Alzheimers Dis 51: , 11–13. |
[8] | Robillard JM , Cleland I , Hoey J , Nugent C ((2018) ) Ethical adoption: A new imperative in the development of technology for dementia. Alzheimers Dement 14: , 1104–1113. |
[9] | Robillard JM , Illes J , Arcand M , Beattie BL , Hayden S , Lawrence P , McGrenere J , Reiner PB , Wittenberg D , Jacova C ((2015) ) Scientific and ethical features of English-language online tests for Alzheimer’s disease. Alzheimers Dement (Amst) 1: , 281–288. |
[10] | Introducing ChatGPT, https://openai.com/blog/chatgpt. |
[11] | Forbes, The Next Generation of Large Language Models, https://www.forbes.com/sites/robtoews/2023/02/07/thenext-generation-of-large-language-models/. |
[12] | Milmo D (2023) ChatGPT reaches 100 million users two months after launch. The Guardian, https://www.theguardian.com/technology/2023/feb/02/chatgpt-100-million-users-open-ai-fastest-growing-app. |
[13] | Forbes, Revolutionizing Healthcare: The Top 14 Uses of ChatGPT in Medicine And Wellness, https://www.forbes.com/sites/bernardmarr/2023/03/02/revolutionizinghealthcare-the-top-14-uses-of-chatgpt-in-medicine-andwellness/. |
[14] | Sallam M ((2023) ) ChatGPT utility in health care education, research, and practice: Systematic review on the promising perspectives and valid concerns. Healthcare (Basel) 11: , 887. |
[15] | De Angelis L , Baglivo F , Arzilli G , Privitera GP , Ferragina P , Tozzi AE , Rizzo C ((2023) ) ChatGPT and the rise of large language models: The new AI-driven infodemic threat in public health., Front Public Health 11: , 1166120. |
[16] | Deng J , Lin Y ((2022) ) The benefits and challenges of ChatGPT: An overview. Front Comput Intell Syst 2: , 81–83. |
[17] | Li J , Dada A , Kleesiek J , Egger J (2023) ChatGPT in healthcare: A taxonomy and systematic review. medRxiv, https://doi.org/10.1101/2023.03.30.23287899. |
[18] | Sharevski F , Loop JV , Jachim P , Devine A , Pieroni E (2023) Talking abortion (mis) information with ChatGPT on Tik-Tok. ArXiv Prepr, ArXiv230313524. |
[19] | Agbavor F , Liang H ((2022) ) Predicting dementia from spontaneous speech using large language models, PLoS Digit Health 1: , e0000168. |
[20] | Hristidis V , Ruggiano N , Brown EL , Ganta SRR , Stewart S ((2023) ) ChatGPT vs Google for queries related to dementia and other cognitive decline: Comparison of results, J Med Internet Res 25: , e48966. |
[21] | Huang SS , Song Q , Beiting KJ , Duggan MC , Hines K , Murff H , Leung V , Powers J , Harvey TS , Malin B (2023) Fact check: Assessing the response of ChatGPT to Alzheimer’s disease statements with varying degrees of misinformation. Medrxiv, doi: 10.1101/2023.09.04.23294917. |
[22] | Saeidnia HR , Kozak M , Lund BD , Hassanzadeh M (2023) Evaluation of ChatGPT’s responses to information needs and information seeking of dementia patients. Research Square, https://doi.org/10.21203/rs.3.rs-3223915/v1. |
[23] | Dubin JA , Bains SS , Chen Z , Hameed D , Nace J , Mont MA , Delanois RE ((2023) ) Using a Google web search analysis to assess the utility of ChatGPT in total joint arthroplasty. J Arthroplasty 38: , 1195–1202. |
[24] | Yeo YH , Samaan JS , Ng WH , Ting PS , Trivedi H , Vipani A , Ayoub W , Yang JD , Liran O , Spiegel B , Kuo A ((2023) ) Assessing the performance of ChatGPT in answering questions regarding cirrhosis and hepatocellular carcinoma. Clin Mol Hepatol 29: , 721–732. |
[25] | King RC , Samaan JS , Yeo YH , Kunkel DC , Habib AA , Ghashghaei R (2023) A multidisciplinary assessment of ChatGPTs knowledge of amyloidosis. medRxivi, https://doi.org/10.1101/2023.07.17.23292780. |
[26] | Lahat A , Shachar E , Avidan B , Glicksberg B , Klang E ((2023) ) Evaluating the utility of a large language model in answering common patients’ gastrointestinal health-related questions: Are we there yet? , Diagnostics 13: , 1950. |
[27] | Electronic Frontier Foundation (2023) Privacy Badger. privacybadger. org |
[28] | Hill R (2023) uBlock Origin. https://ublockorigin.com/ |
[29] | Robillard JM , Jun JH , Lai J-A , Feng TL ((2018) ) The QUEST for quality online health information: Validation of a short quantitative tool. BMC Med Inform Decis Mak 18: , 87. |
[30] | Friedman DB , Hoffman-Goetz L ((2006) ) A systematic review of readability and comprehension instruments used for print and web-based cancer information. Health Educ Behav 33: , 352–373. |
[31] | Haman M , Skolník M (2023) Using ChatGPT to conduct a literature review. Account Res. doi: 10.1080/08989621.2023.2185514. |
[32] | Eltorai AEM , Ghanian S , Adams CA , Born CT , Daniels AH ((2014) ) Readability of patient education materials on the American Association for Surgery of Trauma Website, Arch Trauma Res 3: , e18161. |
[33] | Zuccon G , Koopman B (2023) Dr ChatGPT, tell me what I want to hear: Howprompt knowledge impacts health answer correctness. ArXiv Prepr ArXiv230213793. |
[34] | Cahan P , Treutlein B ((2023) ) A conversation with ChatGPT on the role of computational systems biology in stem cell research. Stem Cell Rep 18: , 1–2. |
[35] | Taira BR , Kreger V , Orue A , Diamond LC ((2021) ) A pragmatic assessment of Google translate for emergency department instructions. J Gen Intern Med 36: , 3361–3365. |