Acupuncture Therapy on Dementia: Explained with an Integrated Analysis on Therapeutic Targets and Associated Mechanisms
Abstract
Background:
Dementia, mainly Alzheimer’s disease (AD) and vascular dementia (VaD), remains a global health challenge. Previous studies have demonstrated the benefits of acupuncture therapy (AT) in improving dementia. Nevertheless, the therapeutic targets and integrated biological mechanisms involved remain ambiguous.
Objective:
To identify therapeutic targets and biological mechanisms of AT in treating dementia by integrated analysis strategy.
Methods:
By the identification of differentially expressed genes (DEGs) of AD, VaD, and molecular targets of AT active components, the acupuncture therapeutic targets associated with the biological response to AD and VaD were extracted. Therapeutic targets-based functional enrichment analysis was conducted, and multiple networks were constructed. AT-therapeutic crucial targets were captured by weighted gene co-expression network analysis (WGCNA). The interactions between crucial targets with AT active components were verified by molecular docking.
Results:
Our results demonstrated that 132 and 76 acupuncture therapeutic targets were associated with AD and VaD. AT-therapeutic crucial targets including 58 for AD and 24 for VaD were captured by WGCNA, with 11 in shared, including NMU, GRP, TAC1, ADRA1D, and SST. In addition, 35 and 14 signaling pathways were significantly enriched by functional enrichment analysis, with 6 mutual pathways including neuroactive ligand-receptor interaction, GABAergic synapse, calcium signaling pathway, cAMP signaling pathway, chemokine signaling pathway, and inflammatory mediator regulation of TRP channels.
Conclusion:
The improvement of AD and VaD by AT was associated with modulation of synaptic function, immunity, inflammation, and apoptosis. Our study clarified the therapeutic targets of AT on dementia, providing valuable clues for complementing and combining pharmacotherapy.
INTRODUCTION
Dementia is a neurocognitive disorder with difficulties in memory, language, and behavior resulting in impairment of daily activities [1]. As the population growth and aging trend, the global dementia population is estimated to surge from 57.4 million cases in 2019 to 152.8 million by 2050, posing a staggering public health challenge [2]. Alzheimer’s disease (AD) and vascular dementia (VaD) are the predominant subtypes of dementia, which can co-occur as mixed dementia with overlapping risk factors and pathological basis [1]. Despite the indistinction between AD and VaD over clinical symptoms, the pathological characteristics of AD are commonly accompanied by neuritic plaques containing amyloid-β (Aβ) and neurofibrillary tangles containing phosphorylated tau, while VaD is marked by chronic infarcts, multiple microinfarcts, or large infarcts [1, 3]. Extracellular deposition of Aβ and intracellular accumulation of hyperphosphorylated tau protein are common triggers of subsequent neurodegeneration in AD including synaptic dysfunction, increased neuronal vulnerability, and progressive neuronal loss that accelerate neuroinflammation leading to the production and release of immune mediators, resulting in disruption of synaptic plasticity [1, 4]. The vascular alterations induced by hypoperfusion, oxidative stress, and inflammation are precursors to the onset of VaD, and synaptic plasticity would be diminished in VaD patients by underlying vascular adverse events arising from a pathological basis that includes endothelial damage, blood-brain barrier breakdown, innate immune activation, and disruption of the trophic coupling between blood vessels and brain cells [1, 4, 5]. Additionally, vascular dysfunction, which is intimately associated with VaD, is also potentially involved in the onset of early AD and exacerbates neurodegeneration [5]. Thus, the complex and persistent pathological process of dementia generates a vicious cycle that gradually exacerbates tissue damage and consequently triggers a series of cognitive dysfunctions.
Although mainstream pharmacological interventions including acetylcholinesterase inhibitors and N-Methyl-D-Aspartic acid (NMDA) receptor antagonist have been administered for the management of dementia symptoms, and anti-inflammatory treatments for neuroinflammation have shown potential benefit [4, 6, 7], considering the moderate efficacy, the range of potentially long-term adverse effects, and specific therapeutic medications that remain to be investigated, non-pharmaceutical interventions (NPIs) are also introduced into the palliative and preventive management of dementia, not only to assist pharmacotherapy, but also to reduce the underlying side effects of pharmacological strategy, thus promoting the health of dementia patients in a comprehensive manner [3, 8].
Acupuncture therapy (AT) is one of the widely used traditional medicine therapies in East Asia for more than 2,000 years [9], and the 2019 World Health Organization Global Report indicated that AT is currently the most common form of practice among diverse traditional and complementary medicines, as reported by 113 Member States [10, 11]. Manual acupuncture (MA) and electroacupuncture (EA) are the main types of AT. In MA, with needles inserted directly into acupoints through the skin, intense mechanical stimulation is triggered by manipulation; in EA, needles are electrified to generate dual mechanical and electrical stimulation to acupoints, and the therapeutic effects of acupuncture are exerted with adjustments of parameters including acupoint selection, stimulation method, and stimulation intensity [12, 13]. From 2010 to 2020, 133 guidelines worldwide included 433 AT recommendations, and statistics demonstrated that neurological disorders were among the most frequently recommended areas for AT application [14, 15]. Evidence maps and overview of systematic reviews indicated that the therapeutic scope of AT covers 77 diseases and conditions under 12 areas, and in particular, AT has moderate efficacy and certainty evidence for the improvement on the severity of dementia symptoms by VaD [11, 16]. Three retrospective cohort studies suggested that integrated measures coupled with AT were beneficial in reducing long-term dementia risk in patients, whether after traumatic brain injury or stroke, in comparison to conventional treatment [17–19]. Assessment of randomized controlled trials (RCTs) concerning efficacy and safety indicated that AT positively improves the cognitive function of dementia patients and demonstrated considerable therapeutic effects in combination with conventional medications compared to pharmacological interventions alone, which not only possessed substantial safety but also contributed additionally to overall health [20–22].
Current research evidence demonstrated that AT has diverse functions in modulation of synaptic plasticity, immunomodulation, anti-inflammation, and anti-apoptosis in response to a series of associated active components including acetylcholine (ACh), noradrenaline (NA), dopamine (DA), serotonin (5-hydroxytryptamine, 5-HT), gamma-aminobutyric acid (GABA), brain-derived neurotrophic factor (BDNF), and glial cell-derived neurotrophic factor (GDNF), thus exerting neuroprotective and cognitive regulatory effects [23–33]. However, the shared and mutually independent therapeutic molecular targets and the integrated biological mechanisms of AT on AD and VaD remain ambiguous. Therefore, by conducting an integrated analysis, we aimed to 1) systematically identify the shared and mutually independent therapeutic targets and crucial targets of AT in treating AD and VaD, 2) investigate the shared and mutually independent integrated biological mechanisms of AT on AD and VaD, and 3) explore target interactions and construct networks of AT on AD and VaD, in an effort to contribute to subsequent ongoing studies and to provide informative support for the future inclusion of AT into NPI management strategies for dementia (Fig. 1).
Fig. 1
Workflow of this study. This figure demonstrates the process of identification of acupuncture therapeutic targets and multiple molecular mechanisms in treating dementia.
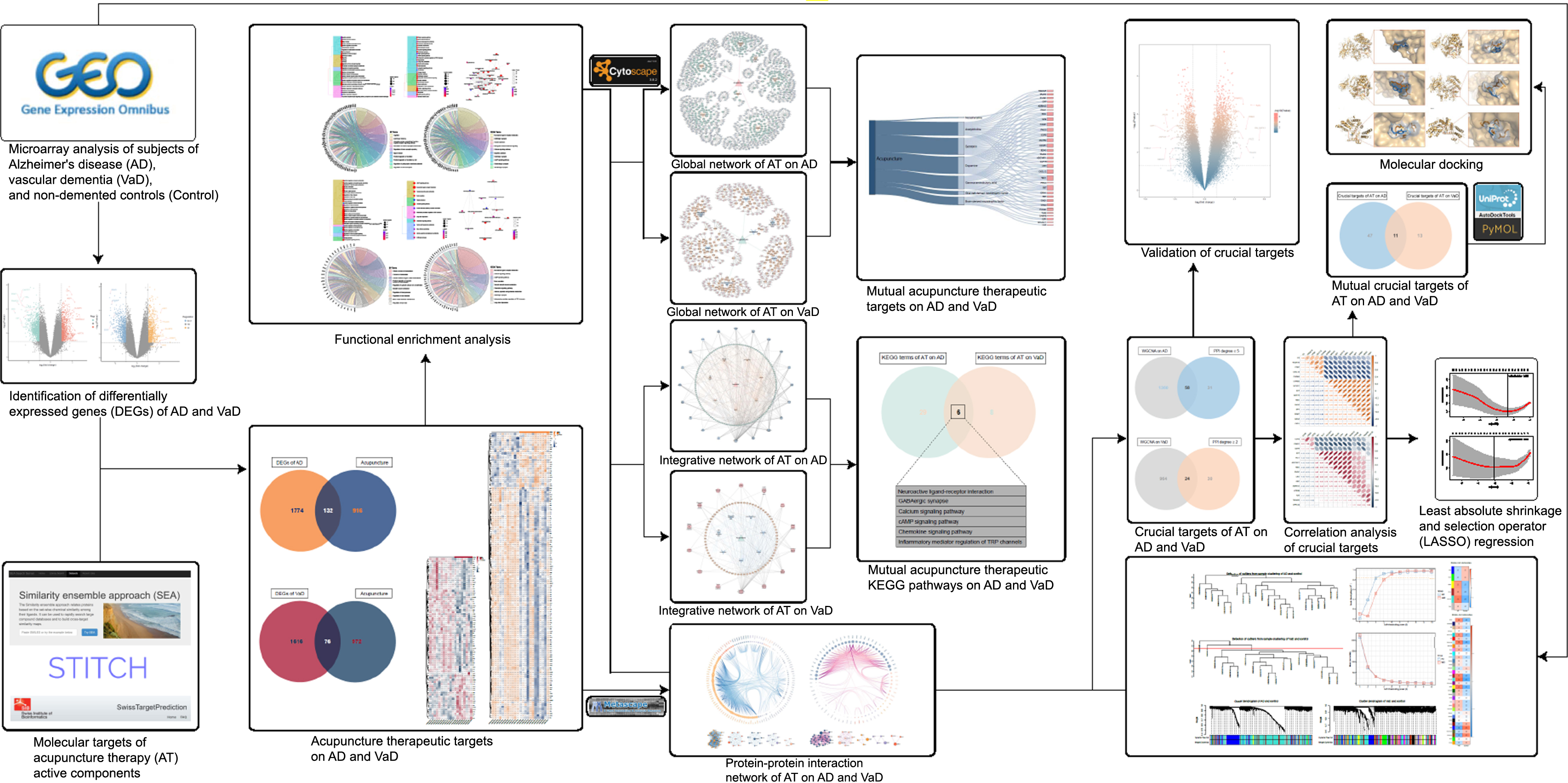
MATERIALS AND METHODS
Datasets search strategy
Datasets relevant to AD and VaD were retrieved from Gene Expression Omnibus (GEO) database (https://www.ncbi.nlm.nih.gov/geo/). The inclusion criteria were defined as 1) the dataset needed to contain both AD, VaD, and matched controls to prevent batch differences, 2) the total sample size of controls combined with either AD or VaD was not less than 15, and 3) the organism of the dataset was Homo sapiens. The exclusion criteria were defined as 1) the dataset contained only matched controls with either AD or VaD, 2) the total sample size of controls combined with AD or VaD was less than 15, 3) the data were derived from non-Homo sapiens, 4) the information was incomplete, and 5) the subject had underlying health conditions or was intervened by any measures.
Data preprocessing
Background correction and normalization of the raw data files from the dataset were conducted with methods of normal– exponential convolution and quantile by “limma” R-package. Data quality evaluation was implemented using “arrayQualityMetrics” package, and probe sequence re-annotation was further conducted. After constructing matching index according to the obtained transcripts file Gencode v34, probe sequence file of the dataset was matched to the transcripts for binary alignment (BAM) file with “Rsubread” package, thus extracting information from the BAM file and obtaining matching data with “Rsamtools” package. Finally, normalized and re-annotated gene expression matrix was generated from the included dataset.
Identification of acupuncture therapeutic targets associated with AD and VaD
Dataset GSE122063 (https://www.ncbi.nlm.nih.gov/geo/query/acc.cgi?acc = GSE122063) contained gene expression profiles by microarray of frontal cortex obtained from individuals who died with AD (n = 12), VaD (n = 8), and age-matched controls (n = 11) with intact cognitive function was included. No infarcts in the AD samples and no or very minimal evidence of any typical pathology of AD in VaD cases, thus excluding mixed dementia among the included samples [34]. Analysis of differentially expressed genes (DEGs) was performed on the preprocessed gene expression matrix with empirical Bayes moderated t-tests by “limma” package. The DEGs of AD and VaD were filtered by p-value < 0.05 and | log2 (fold change) |> 1. The “ggplot2” package was applied to reveal either upregulated or downregulated DEGs of AD and VaD by volcano plots.
Previous evidence supported the significance of the active components, mainly ACh, NA, DA, 5-HT, GABA, BDNF, and GDNF, in the pathology of dementia, being able to effectively respond to AT stimulation that could generate underlying biological effects through their downstream networks of molecular targets [23–33]. Therefore, the molecular targets of AT active components were extracted with Canonical SMILES (Simplified Molecular Input Line Entry System) from PubChem database (https://pubchem.ncbi.nlm.nih.gov/) through Similarity Ensemble Approach (SEA) [35] (https://sea.bkslab.org), STITCH5.0 [36] (http://stitch.embl.de), and SwissTargetPrediction [37] (http://www.swisstargetprediction.ch) databases. Finally, acupuncture therapeutic targets associated with the biological response to AD and VaD, referred to as ACAgenes and ACVgenes, were identified by extracting the overlap of molecular targets of AT active components and the DEGs of AD or VaD.
Functional enrichment analysis
Functional enrichment analysis of the ACAgenes or ACVgenes was conducted by “clusterProfiler” [38] and “org.Hs.eg.db” packages. The biological process (BP), cellular component (CC), and molecular function (MF) terms of Gene Ontology (GO) and Kyoto Encyclopedia of Genes and Genomes (KEGG) were obtained and ranked under a p-adjust (Benjamini-Hochberg) value < 0.05. The visualization of the functional enrichment analysis results was performed with “clusterProfiler”, “enrichplot”, and “GOplot” [39] packages. Top 30 BP and KEGG terms of AT on AD and VaD were systematically exhibited as cluster tree plots, and the p-adjust values of the corresponding terms and the quantity of contained genes were marked with color gradient and size scale of nodes. The interaction among KEGG terms was presented as enrichment map plots. The interaction between the more concerning top 10 enriched terms of BP and KEGG with the corresponding targets were revealed by chord plots.
Construction of visual networks
The Metascape [40] (https://metascape.org/) database was applied to evaluate the interaction and clustering of the ACAgenes or ACVgenes. After the classification and integration of the results, the protein-protein interaction (PPI) network was constructed with “ggraph” and “igraph” packages in accordance with the degree value, which defines the number of connections between a single node to other nodes, representing the interactive intensity among the nodes in the PPI network. The MCODE module of Cytoscape 3.8.2 (https://cytoscape.org) was utilized to perform the analysis and construct clustering sub-networks. Further, the global network and acupuncture-component-target-pathway integrative network were constructed by the categorization and integration of associated information. Finally, the mutual targets from ACAgenes and ACVgenes were displayed in Sankey diagram with “networkD3” package, and the mutual KEGG terms were presented in Venn diagram.
Weighted gene co-expression network analysis
Weighted gene co-expression network analysis of microarray data of AD, VaD, and non-demented subjects was performed with “WGCNA” [41] package. The genes with a top 10,000 median absolute deviation were preliminarily filtered, and outlier samples were removed.
The co-expression similarity sij was defined by calculating the Pearson’s correlation coefficient between the expression vector xi and xj from gene i and gene j:
(1)
Further, the adjacency matrix aij was calculated in the following method:
(2)
The value of the adjacency matrix aij representing the strength of network connectivity of gene i and gene j was derived by selecting a reasonable soft-thresholding power (β) to ensure a scale-free topology.
Subsequently, the modules were identified by hierarchical clustering of the weighting coefficient matrix to convert the adjacency matrix into a topological overlap matrix (TOM), which represents the overlap in shared adjacent genes:
(3)
The average linkage hierarchical clustering was conducted according to the TOM-based dissimilarity measure with a minimum gene dendrogram size of 30, and genes with similar expression profiles were grouped into identical gene modules using DynamicTreeCut algorithm. To further diminish the complexity of the network, modules with similarity above 0.75 were merged.
Finally, based on the association of each module with traits (AD or VaD), modules of significant correlations (p < 0.05) were extracted, genes in the modules were filtered (q-weighted < 0.05). The overlap of targets from the PPI network (degree value more or equal to the first quartile) and filtered genes from weighted gene co-expression network analysis (WGCNA) were captured, defined as crucial targets of AT on AD or VaD. Spearman’s correlation analyses with 95% confidence interval of gene expression among crucial targets of AT on AD or VaD with the top 15 values of degree were conducted.
Validation of crucial targets
External datasets from the GEO database were applied as validation datasets, thus checking the robustness of the screened crucial targets. Limited by the current lack of external VaD-related datasets in the GEO database, we evaluated only the crucial targets of AT on AD. In the validation dataset GSE150696 (https://www.ncbi.nlm.nih.gov/geo/query/acc.cgi?acc = GSE150696), the genes involved in AD were identified from gene expression profiles by microarray obtained from individuals who died with AD (n = 9) and elderly controls (n = 9) without neurological or psychiatric diseases [42]. The validation dataset was processed by the “limma” package, the significant DEGs of AD compared to controls at a p-value < 0.05 was identified. The DEGs that were either upregulated or downregulated in the validation dataset were revealed as volcano plot by the “ggplot2” package. The crucial targets that were also notably regulated in the validation dataset were examined by controlling for | log2 (fold change) | > 1.
Molecular docking
Molecular docking was conducted to examine the interaction between AT active components and the corresponding mutual crucial targets of AT on AD and VaD. The crucial targets were screened with UniProt [43] database (https://www.uniprot.org) to enroll targets that have original ligand with Homo sapiens for the organism and with resolution≤2.80Å by X-ray method, excluding ineligible targets. Molecular docking was conducted by AutoDockTools 1.5.6 (https://ccsb.scripps.edu/mgltools/), the support tool for AutoDock Vina (https://vina.scripps.edu/) [44]. The active cavity box parameter was identified by redocking the eligible crucial targets with their original ligands, followed by calculating the root mean square deviation (RMSD) of the original ligands before and after redocking with PyMOL 2.4.0 (https://github.com/schrodinger/pymol-open-source). Targets with RMSD≤2.000 were included to evaluate the reliability of the docking sites. Then targets with RMSD > 2.000 or affinity≥0 after redocking were further excluded. Subsequently, the 3D structures of AT active components accessed from PubChem database were converted into mol2 format files for molecular docking with the corresponding mutual crucial targets of AT on AD and VaD. Following hydrogenation and gasteiger charge process, the identified active cavity box parameter was configured, modes were assigned to 10 and exhaustiveness was set to 8 to obtain the molecular docking results. Finally, PyMOL 2.4.0 was applied to calculate the RMSD of the active components before and after docking with the crucial targets, RMSD≤2.000 was the standard to evaluate the reliability of the docking results, and the visualization was performed.
Least absolute shrinkage and selection operator (LASSO) regression
LASSO regression was performed with “glmnet” [45] package to generate a series of penalty parameters lambda for the logistic model fitted by the crucial targets based on the binomial distribution traits. By conducting ten-fold cross-validation, the optimal lambda corresponding to the minimum mean of binomial deviance values was detected, thus identifying the core targets among the crucial targets that were more strongly associated with AD or VaD and of greater interest, whose regression coefficients that were not penalized to zero under the assurance of the optimal lambda.
RESULTS
Identification of acupuncture therapeutic targets associated with AD and VaD
By differential gene expression analysis, we identified 1,906 and 1,692 DEGs for AD and VaD, respectively. In comparison to controls, 805 of the DEGs for AD were upregulated and 1,101 were downregulated (Fig. 2A, Supplementary Table 1), while 844 of the DEGs for VaD were upregulated and 848 were downregulated (Fig. 2B, Supplementary Table 1).
Fig. 2
Identification of acupuncture therapeutic targets of Alzheimer’s disease (AD) and vascular dementia (VaD). A) Volcano plot that shows the differentially expressed genes (DEGs) between non-demented control group and AD group. B) Volcano plot that shows the DEGs between non-demented control group and VaD group. C) Venn diagram depicts the 132 overlapped acupuncture therapeutic targets on AD (ACAgenes) between the 1,906 DEGs and the 1,048 molecular targets of acupuncture therapy (AT) active components. D) Venn diagram depicts the 76 overlapped acupuncture therapeutic targets on VaD (ACVgenes) between the 1,692 DEGs and the 1,048 molecular targets of AT active components. E) Heatmap of the 76 ACVgenes in 19 samples. “VaD” group stands for vascular dementia, “Control” group stands for non-demented controls. F) Heatmap of the 132 ACAgenes in 23 samples. “AD” group stands for Alzheimer’s disease, “Control” group stands for non-demented controls.
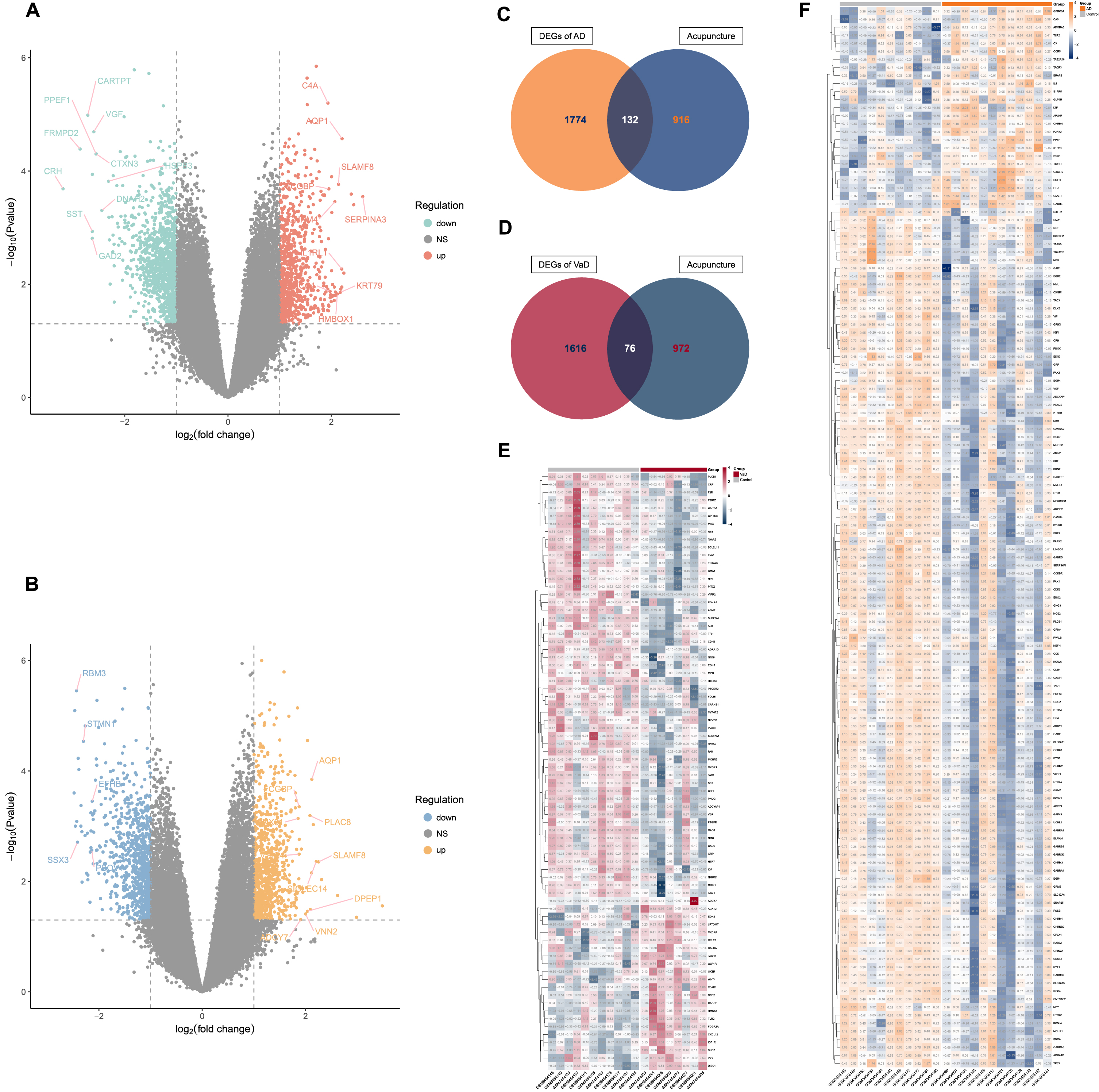
Further, a total of 1,048 molecular targets of AT active components were collected from databases of SEA, STITCH5.0, and SwissTargetPrediction (Supplementary Table 2).
When the DEGs of AD and VaD were compared against the molecular targets of AT active components, 132 ACAgenes and 76 ACVgenes were retained respectively, which were acupuncture therapeutic targets associated with the biological response to AD and VaD (Fig. 2C-F, Supplementary Table 3).
Functional enrichment analysis of acupuncture therapeutic targets associated with AD and VaD
A total of 905 GO terms (Supplementary Table 4) including 749 BPs, 79 CCs, and 77 MFs were enriched and 35 KEGG terms (Supplementary Table 4) were identified from the 132 ACAgenes, and a total of 585 GO terms (Supplementary Table 4) including 530 BPs, 8 CCs, and 47 MFs were enriched and 14 KEGG terms (Supplementary Table 4) were identified from the 76 ACVgenes. The cluster tree plots systematically demonstrated the top 30 BP and KEGG terms (total 14 KEGG terms for VaD) of AT on AD and VaD (Fig. 3A, B, F, G). The complex interactions among KEGG terms were shown as enrichment map plots (Fig. 3C, H).
Fig. 3
Functional enrichment analysis of acupuncture therapeutic targets associated with Alzheimer’s disease (AD) and vascular dementia (VaD). A) Tree plot of top 30 biological process (BP) terms of acupuncture therapy (AT) on AD (p-adjust< 0.05). B) Tree plot of top 30 Kyoto Encyclopedia of Genes and Genomes (KEGG) terms of AT on AD (p-adjust< 0.05). C) Enrichment map plot of top 30 KEGG terms of AT on AD (p-adjust< 0.05). D) Chord plot of top 10 BP terms of AT on AD (p-adjust< 0.05). E) Chord plot of top 10 KEGG terms of AT on AD (p-adjust < 0.05). F) Tree plot of top 30 BP terms of AT on VaD (p-adjust < 0.05). G) Tree plot of all KEGG terms of AT on VaD (p-adjust < 0.05). H) Enrichment map plot of all KEGG terms of AT on VaD (p-adjust < 0.05). I) Chord plot of top 10 BP terms of AT on VaD (p-adjust < 0.05). J) Chord plot of top 10 KEGG terms of AT on VaD (p-adjust < 0.05).
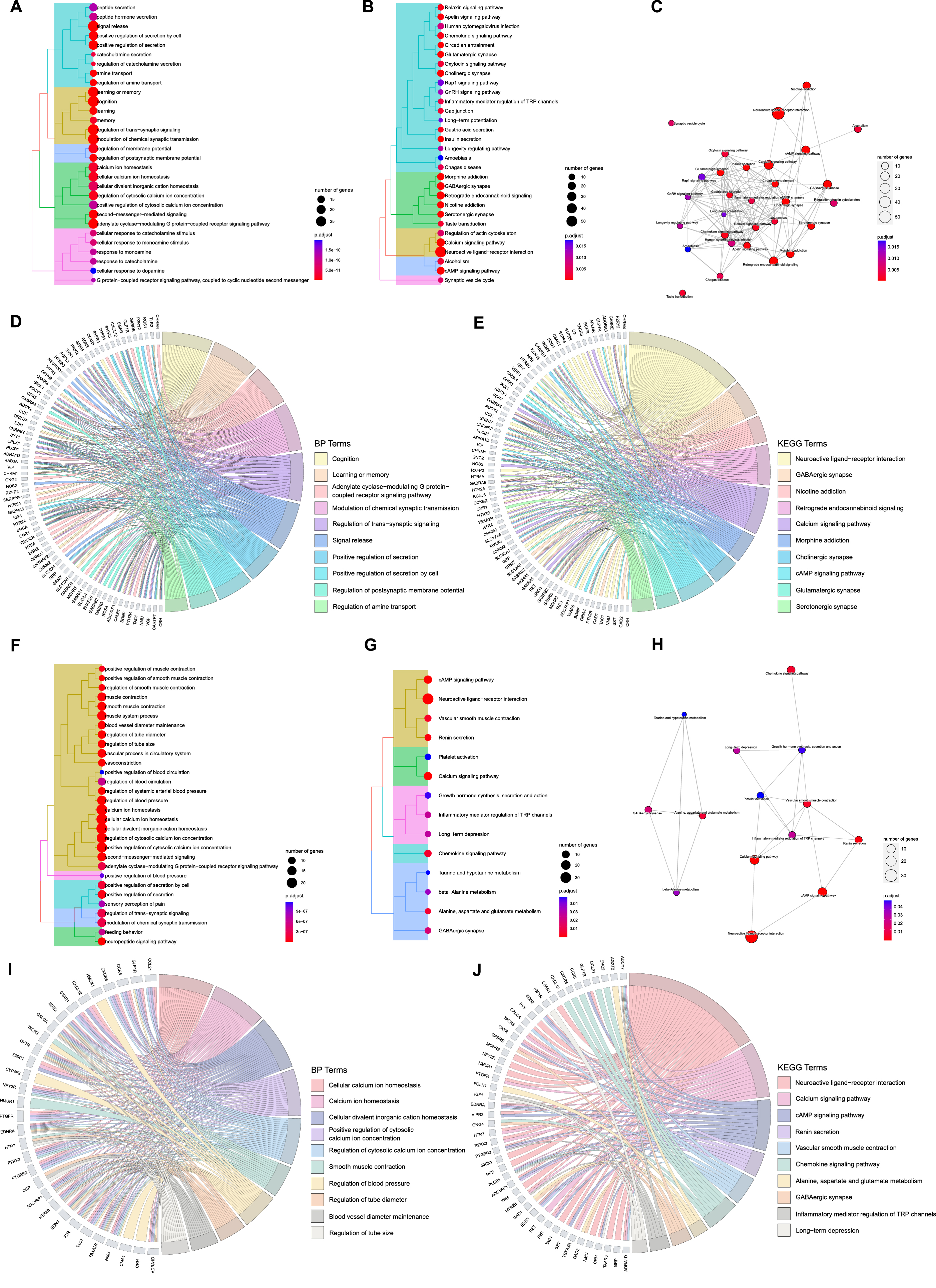
Then, we focused on the more concerning top 10 terms of BP and KEGG with their corresponding targets, as revealed in chord plots (Fig. 3D, E, I, J). For AT treating AD, ACAgenes including NMU, TAC1, CRH, ADCYAP1, and EGFR were involved in many crucial biological processes simultaneously, such as cognition, learning or memory, adenylate cyclase-modulating G protein-coupled receptor signaling pathway, modulation of chemical synaptic transmission, and regulation of trans-synaptic signaling (Fig. 3D); for AT treating VaD, cellular calcium ion homeostasis, calcium ion homeostasis, cellular divalent inorganic cation homeostasis, positive regulation of cytosolic calcium ion concentration, and regulation of cytosolic calcium ion concentration covered most targets, such as ACVgenes of TBXA2R, OXTR, F2R, ADRA1D, and ADCYAP1(Fig. 3I).
Among the top 10 terms in the results of KEGG analysis, we found that ACAgenes such as GABRB3, ADCY2, GNG2, GABRD, and KCNJ6 participated in multiple pathways including retrograde endocannabinoid signaling, cholinergic synapse, and glutamatergic synapse (Fig. 3E), while ACVgenes such as ADCY7, PLCB1, ENDRA, PTGER2, and EDN2 were involved in diverse pathways including renin secretion, vascular smooth muscle contraction, and chemokine signaling pathway (Fig. 3J). A total of 4 significant terms, neuroactive ligand-receptor interaction, GABAergic synapse, calcium signaling pathway, and cAMP signaling pathway, are shared mainly.
Construction of visual networks based on acupuncture therapeutic targets associated with AD and VaD
The interactions among the differentially expressed 132 ACAgenes and 76 ACVgenes were revealed in the PPI network (Fig. 4A, B). With the successive increment of degree values, both the size of nodes enlarges and the density of edges enhances correspondingly, which leads to gradual enhancement of the interaction intensity on targets. As shown by the degree value ranking of 118 out of 132 ACAgenes, GNG2 (57.00) possessed the maximal degree value, followed by GNG3 (54.00), NMU (46.00), MCHR2 (44.00), and MCHR1 (44.00), respectively (Supplementary Table 5). Moreover, clustering was examined and 5 clustering sub-networks dominated by hub genes in the PPI network were identified (Fig. 4A), including APLNR, CRH, GABRA1, GAD1, and PAK1. Among the 66 out of 76 ACVgenes, GNG4 (38.00) possessed the maximal degree value, followed by MCHR2 (30.00), NMU (30.00), NMUR1 (30.00), and ADCY7 (24.00), respectively (Supplementary Table 5), and 5 hub genes were also further identified (Fig. 4B), including ADRA1D, GLP1R, IGF1, CRP, and GAD1. Subsequently, the global networks of AT on AD and VaD were constructed to systematically display the associations of the AT active components, ACAgenes or ACVgenes, and KEGG pathways related to each target (Fig. 4C, D). And the acupuncture-component-target-pathway integrative networks were constructed to integrate and summarize the information from the global networks (Supplementary Figure 1A, B). Finally, the 32 mutual targets from ACAgenes and ACVgenes were displayed in the Sankey diagram (Fig. 4E), such as TBXA2R, TACR3, PLCB1, GRP, and ADRA1D, and the 6 KEGG terms sharing of AT on AD and VaD were presented in Venn diagram (Fig. 4F), including neuroactive ligand-receptor interaction, GABAergic synapse, calcium signaling pathway, cAMP signaling pathway, chemokine signaling pathway, and inflammatory mediator regulation of TRP channels.
Fig. 4
Construction of visual networks based on acupuncture therapeutic targets associated with Alzheimer’s disease (AD) and vascular dementia (VaD). A) PPI network and clustering sub-networks of acupuncture therapy (AT) on AD. In this network, the interaction of 118 targets in PPI network out of 132 acupuncture therapeutic targets on AD (ACAgenes) is displayed. According to the increasing degree value from 1.00 to 57.00, the size of nodes and the density of edges correspondingly increase, leading to gradual enhancement of the interaction intensity on targets, and 5 clustering networks driven by 5 hub genes are further identified. B) PPI network and clustering sub-networks of AT on VaD. In this network, the interaction of 66 targets in PPI network out of 76 acupuncture therapeutic targets on VaD (ACVgenes) is displayed. The degree values of targets increase from 1.00 to 38.00, and 5 clustering networks driven by 5 hub genes are further identified. C) Global network of AT on AD. In this network, the associations of the 7 acupuncture active components, 132 ACAgenes, and the Kyoto Encyclopedia of Genes and Genomes (KEGG) pathways related to each target are systematically displayed. D) Global network of AT on VaD. In this network, the associations of the 7 acupuncture active components, 76 ACVgenes, and the KEGG pathways related to each target are systematically displayed. E) Sankey diagram of the 32 mutual targets from ACAgenes and ACVgenes. F) Venn diagram depicts KEGG terms sharing of AT on AD and VaD.
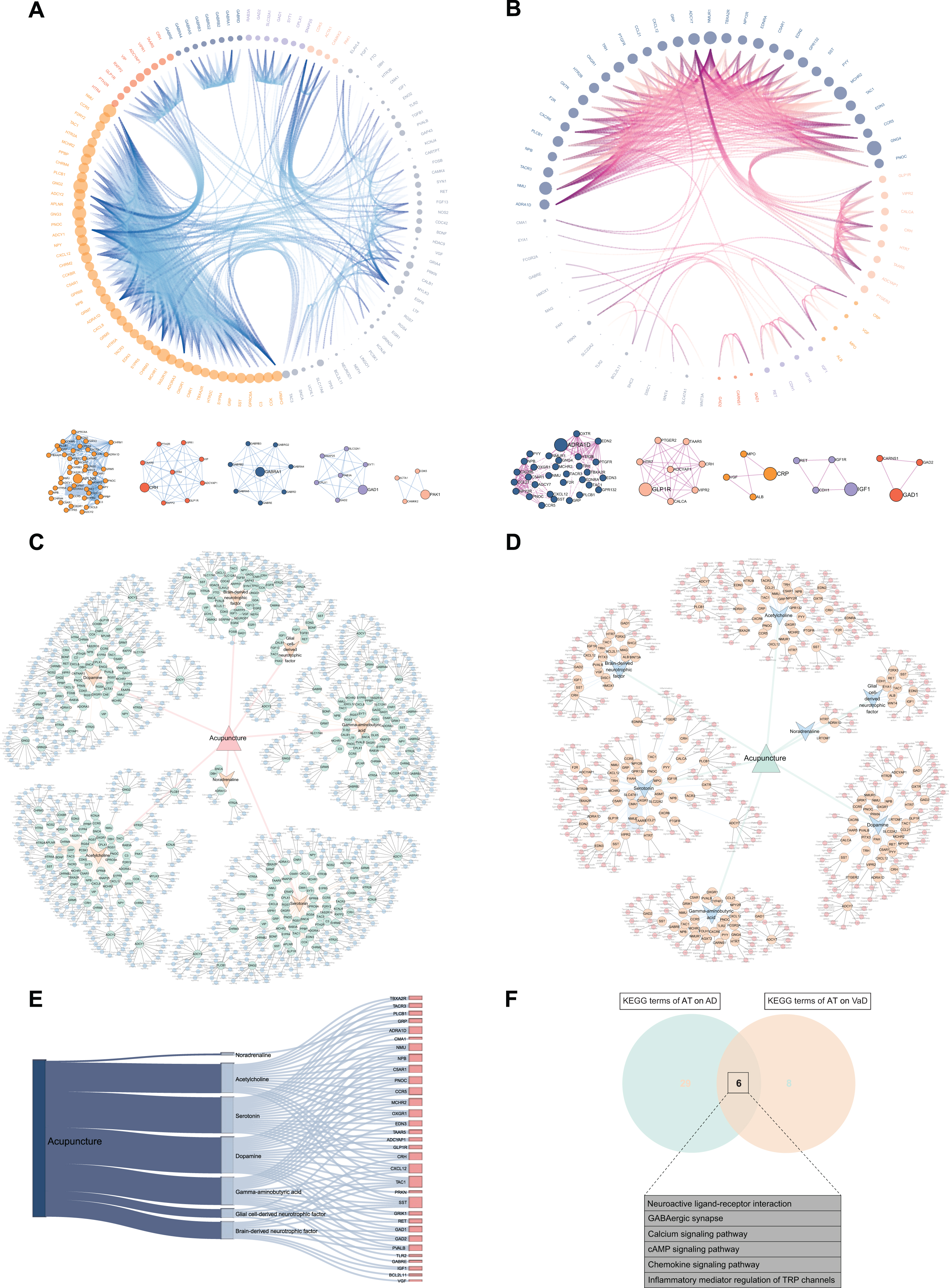
Screening of crucial targets by performance of weighted gene co-expression network analysis
WGCNA was conducted to further screen out crucial targets for AT on AD and VaD. The genes with the top 10,000 median absolute deviations were filtered, followed by the removal of 0 and 2 outlier samples for the analysis of AD or VaD with controls respectively. Finally, 23 and 17 clustered samples were extracted to construct the respective matrices of AD or VaD with controls (Fig. 5A, B).
Fig. 5
Screening of crucial targets by performance of weighted gene co-expression network analysis (WGCNA). A) Cluster analysis of samples from Alzheimer’s disease (AD) and controls to detect outliers. B) Cluster analysis of samples from vascular dementia (VaD) and controls to detect outliers. C) Determination of soft-thresholding power β. D) Evaluation of mean connectivity according to soft-thresholding power β. E) Identification of modules of AD by DynamicTreeCut algorithm. F) Identification of modules of VaD by DynamicTreeCut algorithm. G) Heatmaps of the correlation between modules and clinical traits of VaD. Each row corresponds to a module, and column to a trait. Each cell contains the corresponding correlation and p-value. Modules notably associated with VaD are marked with red boxes. H) Heatmaps of the correlation between modules and clinical traits of AD. Each cell contains the corresponding correlation and p-value. Modules notably associated with AD are marked with red boxes. I) Spearman’s correlation analysis with 95% confidence interval for the crucial targets of acupuncture therapy (AT) on AD with the top 15 values of degree. Significant correlations are designated with asterisk (*p < 0.05; **p < 0.01; ***p < 0.001). J) Spearman’s correlation analysis with 95% confidence interval for the crucial targets of AT on VaD with the top 15 values of degree. Significant correlations are designated with asterisk (*p < 0.05; **p < 0.01;***p < 0.001). K) Venn diagram depicts 58 overlapped crucial targets of AT on AD. L) Venn diagram depicts 24 overlapped crucial targets of AT on VaD.
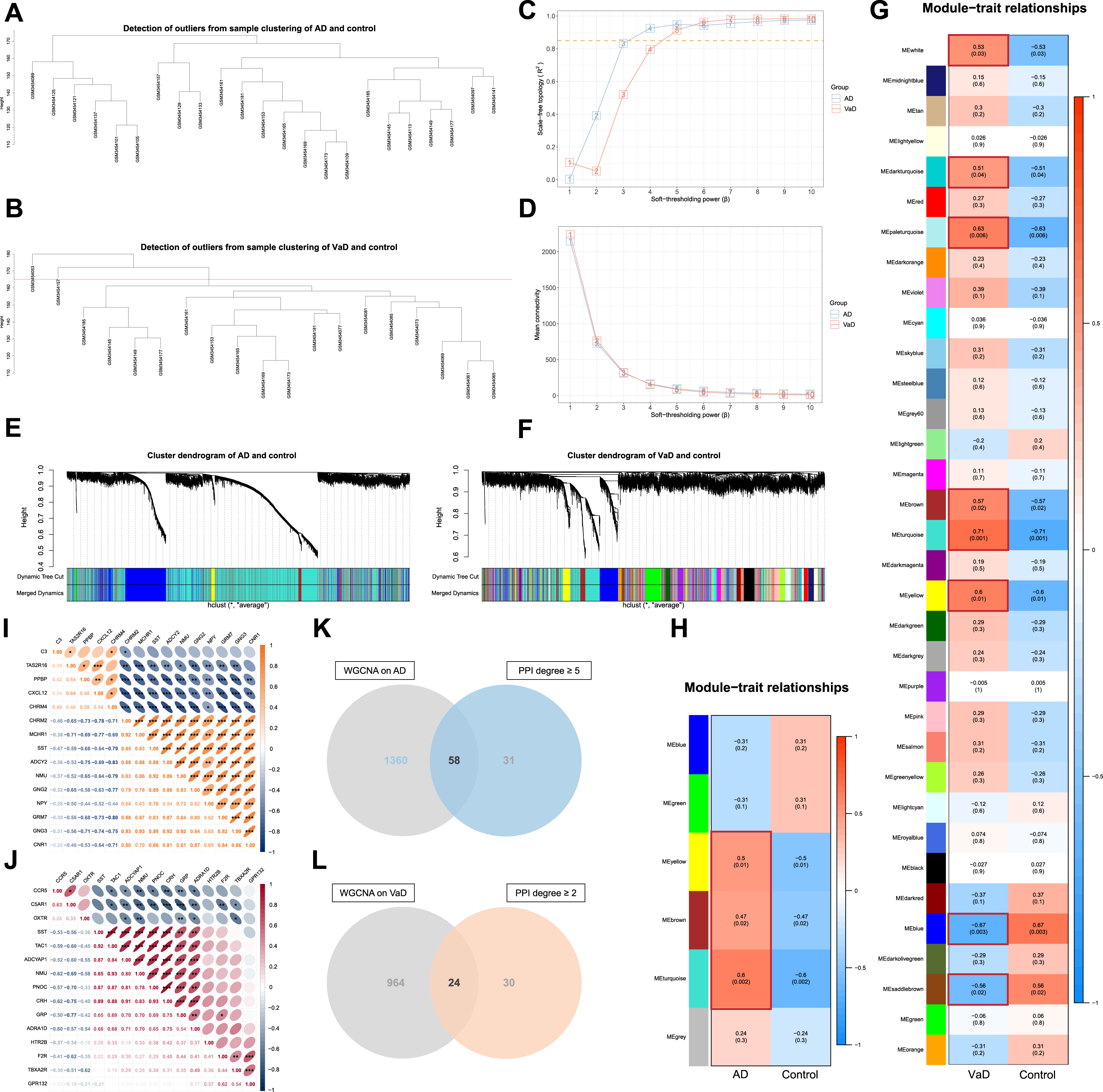
Subsequently, each adjacency matrix aij was calculated from the soft-thresholding power (β) (for AD, β = 4, scale free R2 = 0.925, slope = –1.610; for VaD, β = 5, scale free R2 = 0.914, slope = -2.500) followed by the scale-free topology (Fig. 5C, D). And the co-expression similarity sij was derived from Pearson’s correlation coefficient of the expression vector between each gene.
Hierarchical clustering of average linkage was performed according to the dissimilarity that was measured based on the TOM with a minimum size of 30 for the gene dendrogram, and unclustered genes were grouped into grey modules (MEgrey). A total of 6 and 34 modules of AD and VaD were identified by DynamicTreeCut algorithm, and the modules required no merging due to the similarity less than 0.75 (Fig. 5E, F, Supplementary Figure 2A, B). The TOM network heatmaps were displayed by randomly selected genes (Supplementary Figure 2C, D).
Finally, modules of significant correlations (p < 0.05) with AD and VaD were extracted (Fig. 5G, H), genes in the modules were filtered (q-weighted < 0.05). A total of 58 and 24 targets that overlap with the PPI network of degree value more or equal to the first quartile (5.000 and 2.000) were extracted as crucial targets of AT on AD and VaD (Fig. 5K, L, Supplementary Table 3). The Spearman’s correlation analyses indicated that the expression of most crucial targets were significantly correlated with one another (*p < 0.05; **p < 0.01; ***p < 0.001) (Fig. 5I, J).
Validation of screened crucial targets
The analysis of differential gene expression for the validation dataset indicated a total of 13,536 significant DEGs in AD compared to controls (p < 0.05) (Supplementary Figure 3, Supplementary Table 6). A total of 41 (70.69%) of the 58 crucial targets of AT on AD that we screened retained differential expression in the validation dataset, when controlling for | log2 (fold change) |> 1, a total of 31 (75.61%) out of these 41 crucial targets remained notably regulated, indicating the relative robustness of the screened crucial targets of AT on AD.
Molecular docking of AT active components with crucial targets
A total of 11 mutual crucial targets of AT on AD and VaD were extracted (Fig. 6A). including NMU, GRP, TAC1, ADRA1D, SST, PNOC, C5AR1, CRH, ADCYAP1, TAAR5, and GAD2. Molecular docking was conducted for the eligible mutual crucial targets TAC1 and GAD2 of AD and VaD. By redocking TAC1 and GAD2 with their original ligands, the active cavity box parameter setting centers were identified, RMSD and affinity values indicated the reliability of the docking sites. Furthermore, in accordance with the matching of TAC1 and GAD2 with the AT active components, molecular docking was conducted with ACh, DA, 5-HT, and GABA. PyMOL 2.4.0 (https://github.com/schrodinger/pymol-open-source) visualization revealed that ACh formed 2 hydrogen bonds with amino acid residue ASN-569 of TAC1 (Fig. 6B); DA formed 4 hydrogen bonds with ASN-569, SER-568, and GLU-567 of TAC1 (Fig. 6C), 4 hydrogen bonds with ARG-558, GLN-181, and SER-546 of GAD2 (Fig. 6F); 5-HT formed 4 hydrogen bonds with GLU-567, SER-566, SER-568, and ASN-569 of TAC1 (Fig. 6D); GABA formed 4 hydrogen bonds with ASN-569, GLU-567, SER-568, and ASP-565 of TAC1 (Fig. 6E), 5 hydrogen bonds with GLN-548, ARG-558, GLN-181, and SER-546 of GAD2 (Fig. 6G). The RMSDs of ACh, DA, 5-HT, and GABA before and after docking with TAC1 were 1.065, 0.876, 1.233, and 1.261, respectively. The RMSDs of DA and GABA before and after docking with GAD2 were 1.297 and 0.919. All RMSDs≤2.000, indicating that the AT-associated active components with TAC1 and GAD2 are stable in binding.
Fig. 6
Molecular docking of acupuncture therapy (AT) active components with crucial targets. A) Venn diagram depicts the 11 mutual crucial targets of AT on Alzheimer’s disease (AD) and vascular dementia (VaD). B) Acetylcholine (ACh) formed 2 hydrogen bonds with amino acid residue ASN-569 of TAC1. C) Dopamine (DA) formed 4 hydrogen bonds with amino acid residues ASN-569, SER-568, and GLU-567 of TAC1. D) Serotonin (5-hydroxytryptamine, 5-HT) formed 4 hydrogen bonds with amino acid residues GLU-567, SER-566, SER-568, and ASN-569 of TAC1. E) Gamma-aminobutyric acid (GABA) formed 4 hydrogen bonds with amino acid residues ASN-569, GLU-567, SER-568, and ASP-565 of TAC1. F) DA formed 4 hydrogen bonds with amino acid residues ARG-558, GLN-181, and SER-546 of GAD2. G) GABA formed 5 hydrogen bonds with amino acid residues GLN-548, ARG-558, GLN-181, and SER-546 of GAD2.
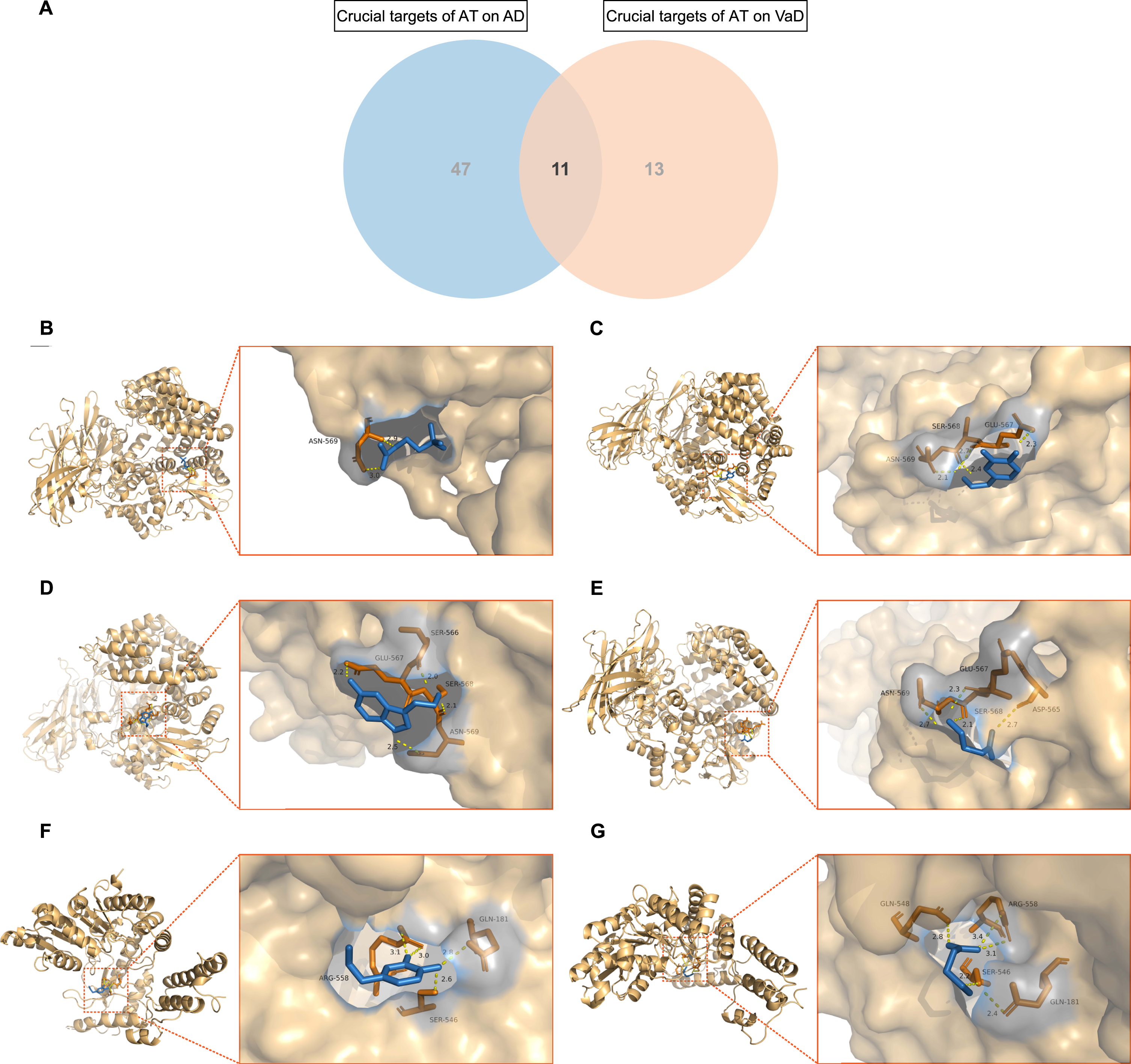
Identification of core targets by LASSO
Machine learning algorithm of LASSO regression was used to identify the core targets for AT on AD and VaD from the series of crucial targets. The optimal penalty parameter lambda corresponding to the minimum mean of binomial deviance values by LASSO regression in AD or VaD was detected to retain groups of core targets, as 6 core targets including GNG2, CHRM4, HTR2C, TAAR5, VIPR1, and GABRG2 under the lambda of 0.094 for AD (Supplementary Figure 4A), and 9 including NMU, GPR132, TBXA2R, HTR2B, SST, C5AR1, RET, CARNS1, and BCL2L11 under 0.048 for VaD (Supplementary Figure 4B).
DISCUSSION
Previous studies on AT for dementia have tended to be either based on the evaluation of efficacy in clinical patients or in-depth exploration of a single mechanism based on animal models, but systematic multi-target and multi-mechanism studies of AT on dementia patients remain limited. Here, for the translation significance of our findings, we started from the microarray of dementia patients via data mining, combined with the AT-associated active components identified in animal models, to reveal the therapeutic targets and biological integrated mechanisms of AT by bioinformatics and network topology approaches. Our results indicated that multiple genes and signaling pathways associated with the regulation of synaptic function, immunity, and inflammation are simultaneously and potentially involved in the shared or mutually independent biological processes of AT on AD and VaD, which depend on the initial participation of a series of active components.
In previous RCTs, AT has demonstrated benefits in enhancing cognition of patients with cognitive impairment, potentially contributing to preventing or delaying the onset of AD and VaD [46, 47], and assisting pharmacotherapy to jointly improve dementia symptoms and overall clinical status, serving as a safe and effective NPI [20–22]. The active components underlying the therapeutic mechanism of AT were revealed in a series of animal models, mainly involving neurotransmitters including ACh, NA, DA, 5-HT, GABA, and neurotrophic factors including BDNF and GDNF [23–33]. In our analysis, we first collected and integrated the downstream molecular targets of these AT active components through multiple databases and compared them with the DEGs of AD and VaD to screen for therapeutic targets on which AT has potential impact, the 132 ACAgenes and 76 ACVgenes.
Then a range of therapeutic mechanisms of AT were revealed via functional enrichment analysis on ACAgenes and ACVgenes, in which the KEGG terms shared including neuroactive ligand-receptor interaction, calcium (Ca2 +) signaling pathway, cAMP signaling pathway, chemokine signaling pathway, GABAergic synapse, and inflammatory mediator regulation of transient receptor potential (TRP) channels. Ca2 + signaling pathway is intimately involved in neurotransmitter release, synaptic plasticity, and other crucial neuronal functions through binding to calmodulin (CaM), triggering the activation and conformational changes of Ca2 + /calmodulin dependent protein kinase II (CaMKII) and CaMKIV, thus serving in synaptic strengthening and regulating the transcription of cAMP response element-binding protein, participating in memory formation [48]. In previous studies, physical stimulation generated by MA can be perceived by TRP vanilloid receptors 1 (TRPV1) to modulate Ca2 + permeability and transmit signals to nerve terminals via calcium wave propagation [49], while EA stimulation can be beneficial to considerably upregulating CaM1, CaMKII, and CaMKIV in the hippocampus and modulate cognitive functions [50]. In AD with excessive Aβ production and the hyperphosphorylated tau or VaD triggered by adverse vascular events, inflammation locally recruits activated microglia and astrocytes to over-secrete multiple pro-inflammatory cytokines and chemokines, while chemokine signaling further enables the recruitment of more microglia, thereby repetitively exacerbating neuroinflammation and mediating the emergence and deterioration of cognitive dysfunction [51, 52]. The levels of pro-inflammatory cytokines and chemokines can be effectively suppressed by MA intervention, which has a positive influence on the regulation of peripheral immune function and inflammatory response and contributes to the improvement of cognition [52]. Substantial evidence also suggested that AT-induced neuroprotective responses were associated with GABAergic inhibitory neurotransmission, and that AT could raise serum GABA levels while reducing serum glutamate levels and the glutamate/GABA ratio [53]. In addition, we identified the therapeutic mechanisms of AT for AD and VaD that were mutually independent of each other, of which, the KEGG terms associated with AD included retrograde endocannabinoid signaling, cholinergic, glutamatergic, and serotonergic synapse, and the KEGG terms associated with VaD included renin secretion and vascular smooth muscle contraction. Cannabinoid (CB) receptors are crucial components of the endocannabinoid system [53], with AT intervention, the two major types of receptors, CB1 and CB2 receptors, could be activated to reduce the expression levels of glutamate and its receptors and elevate GABA and its receptors, restoring the excitatory/inhibitory balances through glutamatergic and GABAergic signaling to exert excitotoxic preventive, neuroinflammatory suppressive, and neuroprotective effects on damaged neurons, thus benefiting the symptomatic relief of AD [25, 32, 53, 54]. In VaD, Renin and angiotensin-converting enzyme (ACE) initiate an enzymatic cascade that promotes the production of angiotensin (Ang) II, which in turn mediates the activation of Ang II type 1 receptor (AT1R), leading to disruption of neuronal homeostasis, vascular remodeling and smooth muscle contraction, endothelial dysfunction from increased inflammation and oxidative stress, and impaired cognitive function in VaD patient [55]. EA can effectively downregulate the hippocampal ACE/Ang II/AT1R axis and upregulate the antagonistic ACE2/Ang-(1–7)/Mas receptor axis, improving the aforementioned adverse vascular events and exerting neuroprotective benefits [55, 56]. Overall, with therapeutic targets, AT can improve the cognitive function of AD and VaD independently or jointly via a range of mechanisms.
By further constructing PPI networks of ACAgenes and ACVgenes and performing WGCNA, the crucial targets of AT on AD or VaD in the global targets were captured. In particular, 11 shared crucial targets were identified, including NMU (neuromedin U), GRP (gastrin releasing peptide), TAC1 (tachykinin precursor 1), ADRA1D (adrenoceptor alpha 1D), SST (somatostatin), PNOC (prepronociceptin), C5AR1 (complement C5a receptor 1), CRH (corticotropin releasing hormone), ADCYAP1 (adenylate cyclase activating polypeptide 1), TAAR5 (trace amine associated receptor 5), and GAD2 (glutamate decarboxylase 2). By molecular docking, we found that the AT active components can effectively bind to the specific sites of TAC1 and GAB2 stably. In addition, we used LASSO regression to identify core targets of AT that were closely associated with disease traits from 58 and 24 crucial targets of AT on AD and VaD, including GNG2 (G protein subunit gamma 2), CHRM4 (cholinergic receptor muscarinic 4), HTR2C (5-hydroxytryptamine receptor 2C), TAAR5 (trace amine associated receptor 5), VIPR1 (vasoactive intestinal peptide receptor 1), and GABRG2 (gamma-aminobutyric acid type A receptor subunit gamma 2) associated with AD, and NMU, GPR132 (G protein-coupled receptor 132), TBXA2R (thromboxane A2 receptor), HTR2B (5-hydroxytryptamine receptor 2B), SST, C5AR1, RET (Ret proto-oncogene), CARNS1 (carnosine synthase 1), and BCL2L11 (BCL2 like 11) associated with VaD. NMU is a member of the neuromedin superfamily and functions as an immunomodulator by binding to its two G protein-coupled receptors (GPCR), including NMU receptor 1 expressed mainly in peripheral tissues and NMUR2 in central nervous system [57]. It was shown that NMU does not interfere with mRNA levels of anti-inflammatory cytokines in lipopolysaccharide-induced memory impairment and neuronal death mice but protects memory function and neuronal cell survival by upregulating BDNF in hippocampus-derived microglia and astrocytes [57, 58]. TAC1 encodes substance P (SP) and neurokinin A that bind to the corresponding GPCRs NK1 receptor and NK2 receptor, respectively, where SP mediates memory facilitation and reinforcement and induces increased blood-brain barrier permeability and activation of microglia and astrocytes, while hippocampal cholinergic neurotransmission modulates amygdala function through NK2 receptors in the medial septum [59]. The tachykinin receptors as mentioned above can be activated by excess Aβ and glutamate to counteract neuronal transmission and interact with the cholinergic system to induce ACh release in the neostriatum [60, 61]. GAD2, also referred to as the 65 kDa isoform (GAD65), is one of the isoforms of glutamic acid decarboxylase (GAD) that, together with GAD67, mediates the metabolism of glutamate and facilitates the synthesis of GABA from glutamate, and this GABAergic and glutamatergic synapses balance is principally moderated by astrocytes through the glutamate/GABA-glutamine cycle, thus avoiding the excitotoxicity of glutamate involved in the pathological process of dementia [25]. Collectively, AT can mediate potential therapeutic effects for AD and VaD jointly or independently via a complex network of molecular targets.
This study has several strengths. We conducted an integrated analysis of AT in treating AD and VaD to identify shared and mutually independent therapeutic targets and biological mechanisms to provide a comprehensive perspective on the potential therapeutic effects of AT. Moreover, we conducted a robust screening of therapeutic targets by constructing PPI networks in combination with WGCNA and LASSO regression, thus identifying a series of crucial and core targets of AT in treating AD and VaD, and performing targets validation to provide informative support for further studies. Nevertheless, this study has some limitations. First, we only investigated several crucial active components associated with the therapeutic effects of acupuncture, but other active components involved in acupuncture treatment remain to be explored by more high-quality evidence. Then, the databases that were used in this study are subject to continuous updates, so more functional or expression-related hits or targets remain to be supported by further studies. Moreover, relevant datasets with larger sample sizes are limited, and due to the majority of studies focusing on AD and the remaining limited datasets of VaD, we validated only the crucial targets of AT in treating AD. Additionally, the therapeutic effects of acupuncture are complicated by multidimensional parameters including acupoint selection, manipulation method, stimulation intensity, and session, and the heterogeneity in acupuncture prescriptions also induces variation. Thus a detailed classified analysis according to the characteristics of concrete acupuncture prescriptions is desirable.
In conclusion, AT, in response of diverse crucial active components (ACh, NA, DA, 5-HT, GABA, BDNF, GDNF) with multiple pathways, effectively modulates the downstream molecular targets network, exerts synaptic plasticity regulatory, immunomodulatory, anti-inflammatory, and anti-apoptotic functions, and alleviates the symptoms of dementia. As an effective and safe NPI, AT holds additional and considerable benefits in avoiding or mitigating the possible long-term side effects of pharmacological therapy, assisting the current management strategy, as well as improving the patients’ quality of life and relieving the pressure on the health systems.
DATA AVAILABILITY
The gene expression profiles by microarray that support the findings of this study are available in the datasets (https://www.ncbi.nlm.nih.gov/geo/query/acc.cgi?acc = GSE122063) and (https://www.ncbi.nlm.nih.gov/geo/query/acc.cgi?acc = GSE150696) from the Gene Expression Omnibus (GEO) database(https://www.ncbi.nlm.nih.gov/geo/). The molecular targets of acupuncture active components are available in the Similarity Ensemble Approach (https://sea.bkslab.org), STITCH 5.0 (http://stitch.embl.de),and SwissTargetPrediction (http://www.swisstargetprediction.ch). The information on protein-protein interaction is available from the Metascape (https://metascape.org/). The structures of targets can be freely downloaded from the UniProt database (https://www.uniprot.org). The Cytoscape 3.8.2 (https://cytoscape.org), AutoDockTools 1.5.6 (https://ccsb.scripps.edu/mgltools/), AutoDock Vina (https://vina.scripps.edu/), and PyMOL 2.4.0(https://github.com/schrodinger/pymol-open-source) are all open-source software that can be freely downloaded for academic use to construct and to visualize networks and molecular docking respectively. All other data are available within the article, Supporting Information is available from authors upon request.
ACKNOWLEDGMENTS
The authors gratefully acknowledge the developers involved and the researchers sharing the data.
FUNDING
The authors have no funding to report.
CONFLICT OF INTEREST
The authors have no conflict of interest to report.
SUPPLEMENTARY MATERIAL
[1] The supplementary material is available in the electronic version of this article: https://dx.doi.org/10.3233/JAD-221018.
REFERENCES
[1] | Robinson L , Tang E , Taylor JP ((2015) ) Dementia: Timely diagnosis and early intervention. BMJ 350: , h3029. |
[2] | Collaborators GBDDF ((2022) ) Estimation of the global prevalence of dementia in 2019 and forecasted prevalence in 2050: An analysis for the Global Burden of Disease Study 2019. Lancet Public Health 7: , e105–e125. |
[3] | Arvanitakis Z , Shah RC , Bennett DA ((2019) ) Diagnosis and management of dementia: Review. JAMA 322: , 1589–1599. |
[4] | Grande G , Qiu C , Fratiglioni L ((2020) ) Prevention of dementia in an ageing world: Evidence and biological rationale. Ageing Res Rev 64: , 101045. |
[5] | Iadecola C ((2013) ) The pathobiology of vascular dementia. Neuron 80: , 844–866. |
[6] | Ardura-Fabregat A , Boddeke E , Boza-Serrano A , Brioschi S , Castro-Gomez S , Ceyzeriat K , Dansokho C , Dierkes T , Gelders G , Heneka MT , Hoeijmakers L , Hoffmann A , Iaccarino L , Jahnert S , Kuhbandner K , Landreth G , Lonnemann N , Loschmann PA , McManus RM , Paulus A , Reemst K , Sanchez-Caro JM , Tiberi A , Van der Perren A , Vautheny A , Venegas C , Webers A , Weydt P , Wijasa TS , Xiang X , Yang Y ((2017) ) Targeting neuroinflammation to treat Alzheimer’s disease. CNS Drugs 31: , 1057–1082. |
[7] | O’Brien JT , Thomas A ((2015) ) Vascular dementia. Lancet 386: , 1698–1706. |
[8] | Livingston G , Huntley J , Sommerlad A , Ames D , Ballard C , Banerjee S , Brayne C , Burns A , Cohen-Mansfield J , Cooper C , Costafreda SG , Dias A , Fox N , Gitlin LN , Howard R , Kales HC , Kivimaki M , Larson EB , Ogunniyi A , Orgeta V , Ritchie K , Rockwood K , Sampson EL , Samus Q , Schneider LS , Selbaek G , Teri L , Mukadam N ((2020) ) Dementia prevention, intervention, and care: 2020 report of the Lancet Commission. Lancet 396: , 413–446. |
[9] | Guzman-Martinez L , Calfio C , Farias GA , Vilches C , Prieto R , Maccioni RB ((2021) ) New frontiers in the prevention, diagnosis, and treatment of Alzheimer’s disease. J Alzheimers Dis 82: , S51–S63. |
[10] | WHO ((2019) ) WHO global report on traditional and complementary medicine 2019. World Health Organization, Geneva. |
[11] | Lu L , Zhang Y , Tang X , Ge S , Wen H , Zeng J , Wang L , Zeng Z , Rada G , Avila C , Vergara C , Tang Y , Zhang P , Chen R , Dong Y , Wei X , Luo W , Wang L , Guyatt G , Tang C , Xu N ((2022) ) Evidence on acupuncture therapies is underused in clinical practice and health policy. BMJ 376: , e067475. |
[12] | Chen T , Zhang WW , Chu YX , Wang YQ ((2020) ) Acupuncture for pain management: Molecular mechanisms of action. Am J Chin Med 48: , 793–811. |
[13] | Fei YT , Cao HJ , Xia RY , Chai QY , Liang CH , Feng YT , Du YR , Yu MK , Guyatt G , Thabane L , Lao LX , Liu JP , Zhang YQ ((2022) ) Methodological challenges in design and conduct of randomised controlled trials in acupuncture. BMJ 376: , e064345. |
[14] | Tang X , Shi X , Zhao H , Lu L , Chen Z , Feng Y , Liu L , Duan R , Zhang P , Xu Y , Cui S , Gong F , Fei J , Xu NG , Jing X , Guyatt G , Zhang YQ ((2022) ) Characteristics and quality of clinical practice guidelines addressing acupuncture interventions: A systematic survey of 133 guidelines and 433 acupuncture recommendations. BMJ Open 12: , e058834. |
[15] | Zhang YQ , Lu L , Xu N , Tang X , Shi X , Carrasco-Labra A , Schunemann H , Chen Y , Xia J , Chen G , Liu J , Liu B , Wang J , Qaseem A , Jing X , Guyatt G , Zhao H ((2022) ) Increasing the usefulness of acupuncture guideline recommendations. BMJ 376: , e070533. |
[16] | Lu L , Zhang Y , Ge S , Wen H , Tang X , Zeng JC , Wang L , Zeng Z , Rada G , Avila C , Vergara C , Chen R , Dong Y , Wei X , Luo W , Wang L , Guyatt G , Tang CZ , Xu NG ((2022) ) Evidence mapping and overview of systematic reviews of the effects of acupuncture therapies. BMJ Open 12: , e056803. |
[17] | Juan YH , Livneh H , Huang HJ , Lu MC , Yeh CC , Tsai TY ((2019) ) Decreased risk of dementia among patients with traumatic brain injury receiving acupuncture treatment: A population-based retrospective cohort study. J Head Trauma Rehabil 34: , E17–E23. |
[18] | Chu SA , Chen TY , Chen PY , Tzeng WJ , Liang CL , Lu K , Chen HJ , Wu CC , Chen JH , Tsai CC , Wang HK ((2021) ) Acupuncture may decrease the incidence of post-stroke dementia: A Taiwan nationwide retrospective cohort study. Front Neurol 12: , 657048. |
[19] | Shih CC , Yeh CC , Hu CJ , Lane HL , Tsai CC , Chen TL , Liao CC ((2017) ) Risk of dementia in patients with non-haemorrhagic stroke receiving acupuncture treatment: A nationwide matched cohort study from Taiwan’s National Health Insurance Research Database. BMJ Open 7: , e013638. |
[20] | Kwon CY , Lee B ((2021) ) Acupuncture for behavioral and psychological symptoms of dementia: A systematic review and meta-analysis. J Clin Med 10: , 3087. |
[21] | Jia Y , Zhang X , Yu J , Han J , Yu T , Shi J , Zhao L , Nie K ((2017) ) Acupuncture for patients with mild to moderate Alzheimer’s disease: A randomized controlled trial. BMC Complement Altern Med 17: , 556. |
[22] | Wang S , Yang H , Zhang J , Zhang B , Liu T , Gan L , Zheng J ((2016) ) Efficacy and safety assessment of acupuncture and nimodipine to treat mild cognitive impairment after cerebral infarction: A randomized controlled trial. BMC Complement Altern Med 16: , 361. |
[23] | Cai M , Lee JH , Yang EJ ((2019) ) Electroacupuncture attenuates cognition impairment via anti-neuroinflammation in an Alzheimer’s disease animal model. J Neuroinflammation 16: , 264. |
[24] | Ferreira-Vieira TH , Guimaraes IM , Silva FR , Ribeiro FM ((2016) ) Alzheimer’s disease: Targeting the cholinergic system. Curr Neuropharmacol 14: , 101–115. |
[25] | Govindpani K , Calvo-Flores Guzman B , Vinnakota C , Waldvogel HJ , Faull RL , Kwakowsky A ((2017) ) Towards a better understanding of GABAergic remodeling in Alzheimer’s disease. Int J Mol Sci 18: , 1813. |
[26] | Jin W , Kim MS , Jang EY , Lee JY , Lee JG , Kim HY , Yoon SS , Lee BH , Chang S , Kim JH , Choi KH , Koo H , Gwak YS , Steffensen SC , Ryu YH , Kim HY , Yang CH ((2018) ) Acupuncture reduces relapse to cocaine-seeking behavior via activation of GABA neurons in the ventral tegmental area. Addict Biol 23: , 165–181. |
[27] | Kim JH , Choi KH , Jang YJ , Bae SS , Shin BC , Choi BT , Shin HK ((2013) ) Electroacupuncture acutely improves cerebral blood flow and attenuates moderate ischemic injury via an endothelial mechanism in mice. PLoS One 8: , e56736. |
[28] | Liu S , Wang Z , Su Y , Qi L , Yang W , Fu M , Jing X , Wang Y , Ma Q ((2021) ) A neuroanatomical basis for electroacupuncture to drive the vagal-adrenal axis. Nature 598: , 641–645. |
[29] | Pchitskaya E , Popugaeva E , Bezprozvanny I ((2018) ) Calcium signaling and molecular mechanisms underlying neurodegenerative diseases. Cell Calcium 70: , 87–94. |
[30] | Simic G , Babic Leko M , Wray S , Harrington CR , Delalle I , Jovanov-Milosevic N , Bazadona D , Buee L , de Silva R , Di Giovanni G , Wischik CM , Hof PR ((2017) ) Monoaminergic neuropathology in Alzheimer’s disease. Prog Neurobiol 151: , 101–138. |
[31] | Yang J , Litscher G , Li H , Guo W , Liang Z , Zhang T , Wang W , Li X , Zhou Y , Zhao B , Rong Q , Sheng Z , Gaischek I , Litscher D , Wang L ((2014) ) The effect of scalp point cluster-needling on learning and memory function and neurotransmitter levels in rats with vascular dementia. Evid Based Complement Alternat Med 2014: , 294103. |
[32] | Yu CC , Du YJ , Wang SQ , Liu LB , Shen F , Wang L , Lin YF , Kong LH ((2020) ) Experimental evidence of the benefits of acupuncture for Alzheimer’s disease: An updated review. Front Neurosci 14: , 549772. |
[33] | Zhang Y , Lan R , Wang J , Li XY , Zhu DN , Ma YZ , Wu JT , Liu ZH ((2015) ) Acupuncture reduced apoptosis and up-regulated BDNF and GDNF expression in hippocampus following hypoxia-ischemia in neonatal rats. J Ethnopharmacol 172: , 124–132. |
[34] | McKay EC , Beck JS , Khoo SK , Dykema KJ , Cottingham SL , Winn ME , Paulson HL , Lieberman AP , Counts SE ((2019) ) Peri-infarct upregulation of the oxytocin receptor in vascular dementia. J Neuropathol Exp Neurol 78: , 436–452. |
[35] | Keiser MJ , Roth BL , Armbruster BN , Ernsberger P , Irwin JJ , Shoichet BK ((2007) ) Relating protein pharmacology by ligand chemistry. Nat Biotechnol 25: , 197–206. |
[36] | Szklarczyk D , Santos A , von Mering C , Jensen LJ , Bork P , Kuhn M ((2016) ) STITCH 5: Augmenting protein-chemical interaction networks with tissue and affinity data. Nucleic Acids Res 44: , D380–D384. |
[37] | Daina A , Michielin O , Zoete V ((2019) ) SwissTargetPrediction: Updated data and new features for efficient prediction of protein targets of small molecules. Nucleic Acids Res 47: , W357–W364. |
[38] | Yu G , Wang LG , Han Y , He QY ((2012) ) clusterProfiler: An R package for comparing biological themes among gene clusters. OMICS 16: , 284–287. |
[39] | Walter W , Sanchez-Cabo F , Ricote M ((2015) ) GOplot: An R package for visually combining expression data with functional analysis. Bioinformatics 31: , 2912–2914. |
[40] | Zhou Y , Zhou B , Pache L , Chang M , Khodabakhshi AH , Tanaseichuk O , Benner C , Chanda SK ((2019) ) Metascape provides a biologist-oriented resource for the analysis of systems-level datasets. Nat Commun 10: , 1523. |
[41] | Langfelder P , Horvath S ((2008) ) WGCNA: An R package for weighted correlation network analysis. BMC Bioinformatics 9: , 559. |
[42] | Low CYB , Lee JH , Lim FTW , Lee C , Ballard C , Francis PT , Lai MKP , Tan MGK ((2021) ) Isoform-specific upregulation of FynT kinase expression is associated with tauopathy and glial activation in Alzheimer’s disease and Lewy body dementias. Brain Pathol 31: , 253–266. |
[43] | UniProt C ((2021) ) UniProt: The universal protein knowledgebase in 2021. Nucleic Acids Res 49: , D480–D489. |
[44] | Trott O , Olson AJ ((2010) ) AutoDock Vina: Improving the speed and accuracy of docking with a new scoring function, efficient optimization, and multithreading. J Comput Chem 31: , 455–461. |
[45] | Friedman J , Hastie T , Tibshirani R ((2010) ) Regularization paths for generalized linear models via coordinate descent. J Stat Softw 33: , 1–22. |
[46] | Zhang J , Hu S , Liu Y , Lyu H , Huang X , Li X , Chen J , Hu Q , Xu J , Yu H ((2022) ) Acupuncture treatment modulate regional homogeneity of dorsal lateral prefrontal cortex in patients with amnesic mild cognitive impairment. J Alzheimers Dis 90: , 173–184. |
[47] | Huang L , Yin X , Li W , Cao Y , Chen Y , Lao L , Zhang Z , Mi Y , Xu S ((2021) ) Effects of acupuncture on vascular cognitive impairment with no dementia: A randomized controlled trial. J Alzheimers Dis 81: , 1391–1401. |
[48] | Cascella R , Cecchi C ((2021) ) Calcium dyshomeostasis in Alzheimer’s disease pathogenesis. Int J Mol Sci 22: , 4914. |
[49] | Wu SY , Chen WH , Hsieh CL , Lin YW ((2014) ) Abundant expression andfunctional participation of TRPV1 at Zusanli acupoint (ST36) inmice: Mechanosensitive TRPV1 as an “acupuncture-respondingchannel”. BMC Complement Altern Med 14: , 96. |
[50] | Bai L , Zhang D , Cui TT , Li JF , Gao YY , Wang NY , Jia PL , Zhang HY , Sun ZR , Zou W , Wang L ((2020) ) Mechanisms underlying the antidepressant effect of acupuncture via the CaMK signaling pathway. Front Behav Neurosci 14: , 563698. |
[51] | Minter MR , Taylor JM , Crack PJ ((2016) ) The contribution of neuroinflammation to amyloid toxicity in Alzheimer’s disease. J Neurochem 136: , 457–474. |
[52] | Pan P , Ma Z , Zhang Z , Ling Z , Wang Y , Liu Q , Lin X , Xu P , Yang D , Zhi H , Wang R , Zhang X ((2021) ) Acupuncture can regulate the peripheral immune cell spectrum and inflammatory environment of the vascular dementia rat, and improve the cognitive dysfunction of the rats. Front Aging Neurosci 13: , 706–834. |
[53] | Hu B , Bai F , Xiong L , Wang Q ((2017) ) The endocannabinoid system, a novel and key participant in acupuncture’s multiple beneficial effects. Neurosci Biobehav Rev 77: , 340–357. |
[54] | Liu Z , Chen X , Gao Y , Sun S , Yang L , Yang Q , Bai F , Xiong L , Wang Q ((2015) ) Involvement of GluR2 up-regulation in neuroprotection by electroacupuncture pretreatment via cannabinoid CB1 receptor in mice. Sci Rep 5: , 9490. |
[55] | Noureddine FY , Altara R , Fan F , Yabluchanskiy A , Booz GW , Zouein FA ((2020) ) Impact of the renin-angiotensin system on the endothelium in vascular dementia: Unresolved issues and future perspectives. Int J Mol Sci 21: , 4268. |
[56] | Feng P , Wu Z , Liu H , Shen Y , Yao X , Li X , Shen Z ((2020) ) Electroacupuncture improved chronic cerebral hypoperfusion-induced anxiety-like behavior and memory impairments in spontaneously hypertensive rats by downregulating the ACE/Ang II/AT1R axis and upregulating the ACE2/Ang-(1-7)/MasR axis. Neural Plast 2020: , 9076042. |
[57] | Ye Y , Liang Z , Xue L ((2021) ) Neuromedin U: Potential roles in immunity and inflammation. Immunology 162: , 17–29. |
[58] | Iwai T , Iinuma Y , Kodani R , Oka J ((2008) ) Neuromedin U inhibits inflammation-mediated memory impairment and neuronal cell-death in rodents. Neurosci Res 61: , 113–119. |
[59] | Borbely E , Scheich B , Helyes Z ((2013) ) Neuropeptides in learning and memory. Neuropeptides 47: , 439–450. |
[60] | Kimura H , Schubert D ((1993) ) Amyloid beta-protein activates tachykinin receptors and inositol trisphosphate accumulation by synergy with glutamate. Proc Natl Acad Sci U S A 90: , 7508–7512. |
[61] | Arenas E , Alberch J , Perez-Navarro E , Solsona C , Marsal J ((1991) ) Neurokinin receptors differentially mediate endogenous acetylcholine release evoked by tachykinins in the neostriatum. J Neurosci 11: , 2332–2338. |