Dynamic cognitive remediation for a Traumatic Brain Injury (TBI) significantly improves attention, working memory, processing speed, and reading fluency
Abstract
Background:
In the U.S. 3.8 million people have a Traumatic Brain Injury (TBI) each year. Rapid brain training exercises to improve cognitive function after a mild TBI are needed.
Objective:
This study determines whether cognitive remediation by discriminating the direction a test pattern moves relative to a stationary background (movement figure-ground discrimination) improves the vision and cognitive deficits that result from a TBI, providing a paradigm shift in treatment methods.
Methods:
Movement-discrimination neurotraining was used to remediate low-level visual timing deficits in the dorsal stream to determine whether it improved high-level cognitive functions, such as processing speed, reading fluency, and the executive control functions of attention and working memory in four men with a TBI between the ages of 15–68. Standardized tests, as well as Magnetoencephalography (MEG) brain imaging, were administered at the beginning and end of 8–16 weeks of intervention training to evaluate improvements in cognitive skills.
Results:
Movement-discrimination cognitive neurotraining remediated both low-level visual timing deficits and high-level cognitive functioning, including selective and sustained attention, reading fluency, processing speed, and working memory for all TBI patients we studied. MEG brain imaging, using the Fast-VESTAL procedure, showed that this movement-discrimination training improved time-locked activity in the dorsal stream, attention, and executive control networks.
Conclusions:
Remediating visual timing deficits in the dorsal stream revealed the causal role of visual movement discrimination training in improving high-level cognitive functions such as focusing and switching attention, working memory, processing speed, and reading. This study found that movement-discrimination training was very rapid and effective in remediating cognitive deficits, providing a new approach that is very beneficial for treating a mild TBI.
1Introduction
Concussions causing a Traumatic Brain Injury (TBI) are prevalent in the U.S., since 3.8 million concussions occur each year, with only 1 in 6 of these concussions being diagnosed (concussionfoundation.org). A TBI is a contributing factor in about a third (30.5%) of all injury-related deaths (Faul et al., 2010). The estimated economic cost of a TBI in 2010, including direct and indirect medical costs, is estimated to be approximately $76.5 billion (cdc.gov/traumaticbraininjury/serve.html). Currently, there are few neurotraining programs to improve cognitive skills in those with a TBI.
1.1What is the underlying problem in a TBI?
Research has found that the effects of a TBI reflect disruptions of the neural networks for attention and working memory (Knight, 2007). After suffering a TBI, patients have longer reaction times, are more distractible, and experience difficulty in sustaining attention (Huisman et al., 2004). Attention is manifest as expectancies of the future, providing predictions to be compared with sensory feedback, essential for ensuring the fluid operations of cognitive functions, allowing them to be anticipatory rather than reactive (Ghajar & Ivry, 2008). Compensation for variable predictive timing by increased prefrontal cortical recruitment would be manifest as increased distractibility, working memory deficits, and problems with balance and coordination, and the expended effort may underlie fatigue, headache, irritability, anxiety, and when prolonged, depression in a TBI (Ghajar & Ivry, 2008). The attention network involves portions of the Dorso-Lateral Pre-Frontal Cortex (DLPFC), the Inferior Parietal Lobule (IPL), and the Posterior Parietal Cortex (PPC) (Posner, Walker, Friedrich & Rafal, 1984; Naghavi & Nyberg, 2005; Menon & Uddin, 2010), all components of or receiving input from the visual cortical dorsal stream. Disruption of connectivity of cortical areas in the dorsal stream and prefrontal cortex affect the brain’s predictive capability, and results in deficits in goal-oriented behavior arising from problems with attention and working memory (WM) (Ghajar & Ivry, 2008). Until recently there was no causative explanation for the attention deficits and persistence of symptoms in TBI patients (Huisman et al., 2004). However, new research proposes that cognitive deficits in those with a TBI result from timing deficits (Ghajar & Ivry, 2008), especially in the motion pathways that prevent the motion and pattern pathways of the brain from working together, preventing the attention and executive control networks from working properly, as is shown by this study.
1.2The role of neural response timing in TBI cognitive impairment
The visual system has been hypothesized to exploit the dichotomy of a fast magnocellular channel and a slower parvocellular channel for the purpose of selective attention (Vidysagar, 1999, 2013). Receiving predominantly magnocellular input (Livingstone & Hubel, 1988; Maunsell, Nealey & DePriest 1990; Merigan & Maunsell, 1993), the dorsal stream, specialized for processing the movement and location of objects in space (Ungerleider & Mishkin, 1982; Livingstone & Hubel, 1988; Felleman & Van Essen, 1991), projects from the primary visual cortex, V1, through visual area MT (middle temporal cortex) to the medial superior temporal area (MST) (Kolster, Peeters & Orban, 2010), as well as projecting to V3, V3A and V6 (Pitzalis et al., 2010) into the intraparietal sulcus regions of the PPC, a selective spatial attention area (Posner et al., 1984) that is also used to analyze event timing (Battelli, Pascual-Leone & Cavanagh, 2007). This is in contrast to the ventral stream which receives both magnocellular and parvocellular inputs as it projects from V1 through area V4 and on to the infero-temporal (IT) cortex, an area specialized in extracting details relating to an object’s shape and color (Ungerleider & Mishkin, 1982; Livingstone & Hubel, 1988; Felleman & Van Essen, 1991). Moreover, magnocellular deficits, slowing down neural timing, are persistent in individuals with a history of a mild TBI (Poltavski, Lederer & Cox, 2017). In addition, increased prefrontal cortical recruitment to compensate for the variable predictive timing from these timing deficits may explain many of the “secondary” post-concussive symptoms of a TBI (Ghajar & Ivry, 2008).
When reading, it has been proposed that the PPC uses the spatial information of the location and overall shape and form of a word it receives through the rapid magnocellular pathway to gate the information going into the temporal stream (Vidyasagar, 1999). The information is gated via attentional feedback to the striate cortex and to other regions in the occipito-temporal cortex (Vidyasagar, 1999, 2013; Watanabe et al., 1998; Martinez et al., 1999; Somers, Dale, Seiffert & Tootell, 1999), most likely by top-down feedback which uses synchronized neuronal oscillations at the lower end of the gamma frequency range (Vidyasagar, 2013). This feedback can then be used by parvocellular neurons in the ventral stream, using coupled alpha/gamma oscillations regulated by the pulvinar for sequential processing (Saalman, Pinsk, Wang, Li & Kastner, 2012), as a starting point for deciphering the individual letters (Vidyasagar, 1999, 2013). Each cycle of gamma oscillation focuses an attentional spotlight on the primary visual cortical representation of just one or two letters before sequential recognition of these letters and their concatenation into word strings (Vidyasagar, 2013). The timing, period, envelope, amplitude, and phase of the synchronized oscillations modulating the incoming signals in the striate cortex have a profound influence on the accuracy and speed of reading (Vidyasagar, 2013). The speed determined by the gamma frequency oscillation is the essential rate-limiting step in dyslexia (Vidyasagar, 2013). Movement figure-ground discrimination training is likely to strengthen coupled: 1) theta/gamma activity for the test patterns moving at 6.7 and 8 Hz, and 2) alpha/gamma activity for the test patterns moving at 10 and 13.3 Hz, the patterns following training that improve in sensitivity the most, in this study and previously. Therefore, it is likely that the visual movement-discrimination training paradigm used in this study improves not only magnocellular function and attention, but also magno-parvo integration, movement figure-ground discrimination, and coupled alpha/gamma frequency oscillations.
The movement-discrimination intervention used in this study for cognitive remediation trains the dorsal stream using patterns optimized for low- and high-level dorsal stream processing (Lawton, 2016). In dyslexics, having sluggish magnocellular neurons (Livingstone, Rosen, Drislane & Galaburda, 1991), not only was visual movement discrimination improved following this neurotraining, but also high-level cognitive functions, including reading fluency, directing attention, auditory and visual working memory and multitasking improved significantly (Lawton, 2016; Lawton & Shelley-Tremblay, 2017). This dynamic cognitive remediation is believed to change the timing of neural responses to be faster via intensive training of the dorsal stream, improving magnocellular relative to parvocellular activity (Lawton, 2011, 2016), thereby improving excitatory and inhibitory circuits, based on the data on neural plasticity. This theory is based on the idea that the synchronous firing of neurons controls communication in the brain between different areas (Buzsaki, 2006). If neurons in one area are “sluggish” with respect to neurons in another area, then they will be unable to synchronize, processing speed will be slowed down, and communication, and hence learning, will be compromised. By extensive training on movement-discrimination, we hypothesize that we are improving the attention, executive control, and reading networks. This study investigates whether damage to higher cognitive functions resulting from a TBI can be ameliorated quickly by retraining dorsal visual (motion) pathway neurons to function optimally when dynamic movement-discrimination neurotraining is used for cognitive remediation, providing a paradigm shift in treatment methods.
2Methods
2.1Participants
Four male subjects who had been diagnosed with a TBI by their doctor, aged 15, 50, 62, and 68 years old, participated in this study. All subjects had tried many different methods to remediate their cognitive deficits, all of these methods being unsuccessful. They were eager to find an effective method to improve the attention and working memory deficits incurred from the TBI that was derailing their lives. All methods used in this study were carried out in accordance with the University of California at San Diego (UCSD) guidelines and regulations for scientific studies on human subjects, and approved by the UCSD IRB. All subjects gave written informed consent prior to study procedures. Subjects were trained how to do the visual timing intervention (movement-discrimination training) at UCSD in the Radiology Imaging Lab. They then completed the visual timing intervention at home, coming into UCSD or one of our staff going to their home once a week, so that their data could be uploaded and we could ensure the data collection was being completed as prescribed. All pre- and post- tests were conducted at the UCSD Radiology Imaging Lab by trained staff.
2.2Intervention: Visual timing training task (Left-right movement discrimination)
The visual timing intervention is called PATH (Perception Attention THerapy) to Insight or PATH at pathtoinsight.com, since following PATH neurotraining high level cognitive functions like attention and executive control, providing insights not previously possible ensue. PATH neurotraining improves the brain’s timing by training movement-discrimination, improving the function of magnocellular neurons in the dorsal stream (‘where’ pathway), relative to parvocellular neurons (Lawton, 2000, 2007, 2015, 2016), resulting in higher level cognitive functions improving as well. A subject was instructed how to do the movement-discrimination training by watching a four-minute movie. The TBI patient sat 57 cm, measured using a marked measuring stick, in front of a computer monitor, with a display similar to the ones in Fig. 1. During the presentation, the bars in the “fish-shaped” window in the center of the screen formed by a sinusoidal grating, moved left or right very briefly (< = 450 ms). The window itself did not move, only the bars within it. The subject reported which way the center pattern moved by pushing the left or right arrow key. A brief tone was presented after incorrect responses. The program adaptively changed the contrast of the test pattern in order to keep the subject at 79% correct (Lawton, 1984). There were also levels of difficulty introduced by making the background pattern more similar to that in the fish, by increasing the pattern’s complexity level, and by increasing the number of directions of movement from one to two directions of motion. Subjects completed between 48 to 77 sessions of movement-discrimination training over 2–4 months.
Fig.1
Sample patterns at Complexity Level 1 for a background two octaves lower in spatial frequency than the test frequency, one octave lower in spatial frequency than the test frequency, equal in spatial frequency to the test frequency, one octave higher in spatial frequency than the test frequency, and two octaves higher in spatial frequency than the 0.5 cyc/deg “fish shaped” test pattern. This same set of backgrounds was presented in this order for each of the 4 test spatial frequencies.

At the start of a session, both the test and background gratings were set to 5% contrast to ensure that the contrast of the test pattern was in the middle of the magnocellular contrast range (Kaplan & Shapley, 1986). The mean luminance was set to 120 cd/m, measured using a Pritchard 1980A Spectra photometer. Each time the subject correctly identified the direction the fish stripes moved, the contrast of the test grating was lowered one step until the subject answered incorrectly. Following the first incorrect response, a double-staircase procedure (Lawton, 1984) was used to estimate the movement-discrimination contrast threshold, which allowed measuring the contrast sensitivity, defined as the reciprocal of the contrast threshold times 100. This staircase procedure estimates the contrast needed for 79% correct responses, providing the most sensitive, repeatable measurements of contrast sensitivity (Higgins, Jaffe, Coletta, Caruso & de Monasterio, 1984). A full training cycle of the movement-discrimination task required 20 threshold determinations: for each of the four test spatial frequencies (0.25, 0.5, 1, and 2 cyc/deg) paired with each of the five background spatial frequencies (equal to the test frequency or±1 or ±2 octaves from the test frequency).
The complexity level increased: 1) the number of sinewave components in the background from one (Fig. 1) to three harmonically related frequencies having a difference frequency equal to the test frequency, shown previously to facilitate movement discrimination (Lawton, 1985, 1989), 2) the background contrast from 5% to 10% to 20%, to increase the amount of parvocellular activity, since magno cells saturate at 10% contrast (Kaplan & Shapley, 1986), and 3) the pattern’s speed of movement after every 4 complexity levels, increasing from 6.7 Hz to 8 Hz (in theta range) to 10 Hz to 13.3 Hz (in alpha range), so that the subject was challenged as the training progressed. Table 1 shows the stimulus conditions for each complexity level. The 20% contrast background required the subject to analyze information from magnocellular activity relative to increased parvocellular activity, making the task more challenging. The order of presentation for each complexity level was chosen to gradually increase the difficulty of the task. Therefore, as the level of complexity increased, the contrast threshold should be higher initially, keeping the task challenging.
Table 1
Stimulus Characteristics at Each Complexity Level
Complexity Level | Pattern Speed | Background Frequencies | Background Contrast |
1 | 6.7 Hz | Single Frequency | 5% |
2 | 6.7 Hz | Multifrequency | 5% |
3 | 6.7 Hz | Multifrequency | 10% |
4 | 6.7 Hz | Multifrequency | 20% |
5 | 8 Hz | Single Frequency | 5% |
6 | 8 Hz | Multifrequency | 5% |
7 | 8 Hz | Multifrequency | 10% |
8 | 8 Hz | Multifrequency | 20% |
9 | 10 Hz | Single Frequency | 5% |
10 | 10 Hz | Multifrequency | 5% |
11 | 10 Hz | Multifrequency | 10% |
12 | 10 Hz | Multifrequency | 20% |
13 | 13.3 Hz | Single Frequency | 5% |
14 | 13.3 Hz | Multifrequency | 5% |
15 | 13.3 Hz | Multifrequency | 10% |
16 | 13.3 Hz | Multifrequency | 20% |
Once all 16 complexity levels of the Motion program were completed, the TBI patient progressed onto the next program, the MotionMemory program. Instead of indicating the direction the center pattern moved by pushing the left or right arrow key as in the Motion program, MotionMemory requires signaling the direction that two separate patterns moved, one after the other, by pushing one of four arrow keys: the up arrow key if the pattern only moved right, the down arrow key if it only moved left, the left arrow key if it moved left and then right, and the right arrow key if the pattern moved right and then left. The two patterns in the MotionMemory task had the same contrast and spatial and temporal frequencies, having different directions of motion or the same direction of motion half the time, the direction of motion of each pattern being determined by a random number generator.
Each threshold in both the Motion and MotionMemory programs required 20–40 trials to complete at each complexity level. A score was given to make the training more game-like. The lower the contrast threshold, the higher was the score. After learning how to do this task, the subject typically took about 10–15 minutes to complete one training cycle, consisting of 20 contrast thresholds. Movement-discrimination was trained for between 10–15 minutes, 2-3 times each week for 8 to 16 weeks, depending on the subject, some preferring to complete direction discrimination training more rapidly than others, the 68 year old completing 77 sessions in two months, the 62 year old completing 60 sessions over 4 months, the 50 year old completing 48 sessions over 2 months, and the 15 year old completing 66 sessions over 4 months. For the subject to complete all 16 complexity levels for both Motion and MotionMemory required at least 64 sessions.
2.2.1Fidelity of implementation
All contrast threshold data with date and time stamps was stored in individual and summary files, and collected automatically by the computer. Therefore, there was no means for tampering with the data collection. Data in summary files showed each subject’s contrast thresholds, how long it took to complete each threshold. At the end of each training cycle, the subject earned a star for each level of complexity completed to let them follow their training progress more easily.
2.3Behavioral measures used before and after intervention
The following standardized tests of reading, attention, and working memory skills were administered to every subject by trained staff at the beginning and end of the intervention training to provide a standardized measure of the improvements in high-level cognitive functions after intervention training. These tests were chosen because they are the “Gold Standard” tests for fast and accurate measurements of fluency-based reading, attention, and working memory (both visual and auditory) skills.
The tests of visual and cognitive skills that each subject completed were:
1. Movement Discrimination Diagnosis program consisting of first session of PATH neurotraining to determine whether the subject had difficulty doing left-right movement discrimination, being characterized by 6 levels of movement-discrimination: 1-above normal, 2-normal, 3-borderline movement discrimination deficits, 4-mild movement discrimination deficits, 5-moderate movement discrimination deficits, and 6-markedly below normal movement discrimination deficits.
2. Computer-Based Reading Speed test, not limited by the person’s rate of speaking, was completed by determining the speed needed to read 6 words of text on the screen from an interesting story (Cheaper by the Dozen) at increasing speeds to measure 2 reading rate thresholds (79% correct) using a double-staircase procedure, after being trained by watching a 4-minute movie.
3. Reading Rate using Nelson Denny Reading Test (NDRT), having a pre- and post- version.
4. Attention using Delis-Kaplan Executive Function System (DKEFS) Color-Word Interference test, where subject had to say the printed color of words that denoted a different color (Stroop test), and Attention Switching, switching between the color of the word and what the word says when surrounded by a rectangular box.
5. Attention Network Test (ANT) (Fan, McCandliss, Fossella, Flombaum & Posner, 2005) was downloaded from https://www.sacklerinstitute.org/cornell/assays_and_tools/ant/jin.fan/. The ANT measures three different attention networks, the alerting, orienting, and executive control attention networks. The subject’s task was to identify the direction of the center arrow of 5 horizontal black arrows (one central target plus four flankers, two on each side), pointing leftward or rightward, against a gray background, by pressing a key with the index finger of the left hand when the center target is pointing left and a key with the index finger of the right hand when the center target is pointing right, while ignoring the spatial location (left or right) of the target relative to the fixation crosshair. If the flanking arrows point in the same direction as the central target arrow, then this was used to measure the Reaction Time (RT) for the orienting attention network. If the flankers pointed the opposite way to the center arrow, then this was used to measure the RT for the Executive Control Network. Subjects were instructed to make their response to the direction of the center target as quickly and accurately as possible.
6. Wechsler Adult Intelligence Scale (WAIS)-4 Processing Speed required two subtests: the WAIS Symbol Search subtest which required subjects to scan a target group and search a group of symbols, indicating whether one of the target symbols appeared in the search group, and the WAIS Digit Symbol Coding subtest, where the subject filled in boxes below digits with symbols that were paired with them in a key at the top of the page. Both of these subtests were timed for two minutes each. The scaled scores from each subtest were combined to create an overall Processing Speed Index score.
7. WAIS-4 Working Memory Index is based on the Digit Span subtest, where the subject had to repeat a list of spoken numbers, requiring the subject to remember subsequently more numbers: in the correct order, backwards, and in numerical sequence on three different subtests, and the Letter-Number Sequencing subtest which required sequencing subsequently more numbers and letters in the correct numerical and alphabetic sequence. Only presentation of the numbers and letters were timed for one second each for these working memory tests.
8. Visual Working Memory (VWM) and Auditory Working Memory (AWM) using the Test of Information Processing Skills (TIPS). The subject recalled a sequence of letters presented one at a time, either visually or verbally: right after seeing or hearing the entire sequence of letters, after counting 10 numbers in sequence, and after repeating a short sentence with animal names for the VWM task, and fruit names for the AWM, being asked to remember these animal and fruit names 3 minutes after completing the VWM and AWM task to measure Delayed Recall. Only presentation of the letters were timed for two seconds each for these working memory tests.
All of these cognitive assessments, which were age-appropriate, took about 2 hours to complete. If two forms of the standardized test were available, as is the case for the NDRT, then we used the alternate form on the post-test to prevent practice effects. The other tests cannot be improved by practice alone, since processing speed, attention, and working memory limited the subject’s performance. Measuring significant improvements in these measures at the end of intervention training was used to determine whether this training was effective in improving different cognitive skills.
2.4Behavioral statistical analyses
At the end of this study all data for the 4 subjects was averaged together and analyzed using either a two-factor ANOVA without replication for the Contrast Sensitivity (CS) data, or paired-sample t-tests to compare initial to final scores on standardized tests of cognitive skills. All tests were one-sided since TBI patients were expected to increase in visual and cognitive skills if the intervention was effective, with significance level α= 0.05 for all testing. The effect size and power were calculated post-hoc from group parameters assuming no correlation between pre and post tests using G*Power (Faul, Erdfelder, Lang & Buchner, 2007). The Benjamini-Hochberg (B-H) procedure (Benjamini & Hochberg, 1995) was used to control the false discover rate in multiple comparison to avoid Type I errors (false positives). The formula for computing the B-H correction is (i/m) *Q, where i is the Rank, as listed in the Table 2, m is the total number of tests (14), and Q is the false discovery rate, here set to 5%.
Table 2
Improvement in Visual and Cognitive Skills Following PATH neurotraining
Test | Initial Mean±SE | Final Mean±SE | df | t-value | Effect Size | Power | P value | Rank | (i/m)Q |
AWM + WMI | 44% ±9% | 54% ±9% | 7 | –3.91 | 0.2 | 0.13 | 0.001 | 1 | 0.004 |
Both Reading Tests | 243wpm±59 | 360±68 | 7 | –3.21 | 0.32 | 0.21 | 0.004 | 2 | 0.007 |
Movement Deficits | 4.5±0.4 | 1.5±0.1 | 3 | 5.2 | 1.67 | 0.81 | 0.007 | 3 | 0.011 |
Attention Switching | 51% ±3% | 65% ±3% | 3 | –4.54 | 0.82 | 0.36 | 0.01 | 4 | 0.014 |
Nelson-Denny | 128wpm±8 | 186±16 | 3 | –3.61 | 0.82 | 0.35 | 0.02 | 5 | 0.018 |
Processing Speed | 29% ±5 | 56% ±0.4 | 3 | –2.79 | 1.4 | 0.7 | 0.03 | 6 | 0.021 |
Reading Speed | 359wpm±76 | 534±75 | 3 | –2.91 | 0.41 | 0.03 | 0.03 | 7 | 0.025 |
ANT Exec Control | 220msec±47 | 149msec±30 | 3 | 3.03 | 1.27 | 0.62 | 0.03 | 8 | 0.029 |
WAIS WMI | 41% ±9% | 53% ±9% | 3 | –3.08 | 0.24 | 0.1 | 0.03 | 9 | 0.032 |
ANT Orient | 70msec±24 | 37msec±13 | 3 | 2.68 | 1.21 | 0.58 | 0.04 | 10 | 0.036 |
Attention | 32% ±5% | 50% ±1% | 3 | –2.56 | 0.95 | 0.43 | 0.04 | 11 | 0.039 |
Delayed Recall | 25% ±5% | 76% ±5% | 3 | –2.62 | 1.85 | 0.87 | 0.04 | 12 | 0.043 |
Visual WM | 60% ±8% | 76% ±7% | 3 | –2.38 | 0.38 | 0.15 | 0.05 | 13 | 0.046 |
Auditory WM | 48% ±10% | 55% ±11% | 3 | –2.32 | 0.13 | 0.08 | 0.05 | 14 | 0.050 |
2.5MEG imaging
MEG responses to the N-back (N = 2) working memory (WM) task were collected using the VectorViewä whole-head MEG system (Elekta-Neuromag, Helsinki, Finland) with 306 MEG channels. The N-back task is one of the most frequently used WM paradigms (Gevins & Cutillo, 1993) to investigate the neural basis of WM processes. Meta-analyses indicate that the WM network consists of six reliably activated cortical regions (Owen, McMillan, Laird & Bullmore, 2005; Phillips, Parry, Mandalis & Lah, 2017): (1) bilateral rostral prefrontal cortex (rPFC) including frontal pole (FP, BA 10), ventromedial prefrontal cortex vmPFC, and orbitofrontal cortex (OFC, BA 11); (2) bilateral dlPFC (BA 9, 46); and (3) bilateral ventrolateral prefrontal cortex (vlPFC) or frontal operculum (BA 45,47); (4) bilateral medial posterior parietal cortex (PPC), including the precuneus, and the inferior parietal lobules (approximate BA7,40); (5) bilateral premotor cortex (BA 6, 8); and (6) dorsal cingulate/medial premotor cortex, including supplementary motor area (SMA; BA 32, 6). The cerebellum is also consistently activated during WM.
Subjects underwent MEG recordings while performing an N-back WM task. The task entails on-line monitoring, updating, and manipulation of remembered information. During the task, the subject was required to monitor a series of letters (both upper and lower case) presented for 500 ms in the middle of the screen. A fixation cross was presented during the 3000 ms interstimulus interval. The subject was instructed to respond only when a letter was presented that matched (i.e. target) the one presented n trials previously, while not to respond to the unmatched stimuli (non-target). Two load conditions were used (1-back and 2-back), which placed increasing demands on WM processes. About 50 trials per load condition were collected for each subject. Performance was recorded using an MEG-compatible response pad, in which index finger blocks-and-unblocks a laser-beam.
Subjects were seated in an upright position inside a multi-layer magnetically-shielded room (IMEDCO-AG) at the UCSD MEG Center. Two MEG exams were performed for each subject who did not have metals objects in their brains, one before and another after the movement-discrimination intervention. MEG data were sampled at 1000 Hz and were run through a high-pass filter with a 0.1 Hz cut-off, and a low-pass filter with a 330 Hz cut-off. Eye blinks and eye movements were monitored using two pairs of bipolar electrodes with one pair placed above and below the left eye, and the other pair placed on the two temples. Heart signals were monitored with another pair of bipolar electrodes. Precautions were taken to ensure head stability; foam wedges were inserted between the subject’s head and the inside of the unit, and a Velcro strap was placed under the subject’s chin and anchored in superior and posterior axes. Head movement across different sessions was about 2-3 mm on average.
MEG sensor waveforms in raw (un-averaged) format were first run through MaxFilter, also known as signal space separation (Taulu, Kajola, & Simola, 2004; Taulu, Simola & Kajola, 2004; Song et al., 2008), to remove external interferences (e.g., magnetic artifacts due to metal objects, strong cardiac signals, environment noises, etc.). Next, residual artifacts near the sensor array due to eye movements and residual cardiac signals were removed via Independent Component Analysis using Fast-ICA (http://research.ics.aalto.fi/ica/fastica/) (Hyvarinen, 1999, Hyvarinen & Oja, 2000). The waveforms associated with top independent components (ICs) were examined by an experienced MEG data analyst, along with ECG and EOG signals. ICs associated with eye blinks, eye movements, heartbeats, and other artifacts were removed.
Following the pre-processing step, N-back MEG sensor-waveform datasets were run through band-pass filters for beta band (15–30 Hz). Each data set was then divided into trials, each with 2.5-second duration (–500 ms to 1500 ms with respect to the stimulus onset). In the present study, we focused on the trials associated with target stimuli.
Different from the conventional MEG approach in which sensor waveforms are averaged with respect to the onset of the stimuli, the sensor covariance matrices for individual trials were calculated. Then a total sensor-waveform covariance matrix of the target condition was calculated by averaging across the covariance matrices from individual trials for the target stimuli. Then the covariance matrices across trials were averaged. Using the total covariance matrix, voxel-wise MEG source magnitude images that cover the whole brain were obtained for each subject, and each frequency band, following the Fast-VESTAL procedure (Huang et al., 2014; Huang et al., 2016), measuring time-locked signals during a working memory N-back task to evaluate improvements in brain function. An objective pre-whitening method was applied to remove correlated environmental noise and objectively select the dominant eigen-modes of sensor-waveform covariance matrix (Huang et al., 2014).
Voxel-wise whole brain MEG source magnitude images obtained from Fast-VESTAL were first spatially co-registered to the MNI-152 (Grabner et al., 2006) brain-atlas template using a linear affine transformation program, FLIRT, in the FSL software package (Smith et al., 2004; Woolrich et al., 2009). Then in MNI-152 space, the MEG source magnitude images were spatially smoothed using a Gaussian kernel with 5 mm full width half maximum (FWHM), followed by a logarithmic transformation using FSL. Next, in MNI-152 coordinates, voxel-wise paired-sample t-test statistical analysis was performed to detect differences in brain activation during the MEG N-back task before and after the PATH neurotraining intervention. Cluster analysis with threshold of corrected p = 0.01was used to correct the family-wise error across voxels.
3Results
3.1Visual skills improved significantly following training to improve visual timing
This study, examining the efficacy of improving the brain’s visual timing, found that movement-discrimination training significantly improved both the visual and cognitive skills of all four TBI subjects, see Table 2. Initially all subjects had difficulty discriminating the direction of movement, as can be seen by the low contrast sensitivity for the first level of complexity in Fig. 2 and the initial movement–discrimination (MD) evaluation in Fig. 3a, showing all had magnocellular deficits. None of the subjects could discriminate left-right movement at the faster speeds used at higher complexity levels until they were trained to discriminate left-right movement at the slower speeds used at lower levels of complexity. As the pattern’s speed of movement increased after each 4 complexity levels, see Table 1, patterns were displayed on the screen for shorter durations. Since the staircase procedure took approximately the same number of trials to complete as found at lower levels of complexity, the movement-discrimination task was completed more quickly at higher complexity levels than at lower complexity levels.
Fig.2
Improvements in contrast sensitivity (CS) as complexity level increased for one direction of movement, averaged over 4 subjects, plotting mean and SE CS for each test spatial frequency.
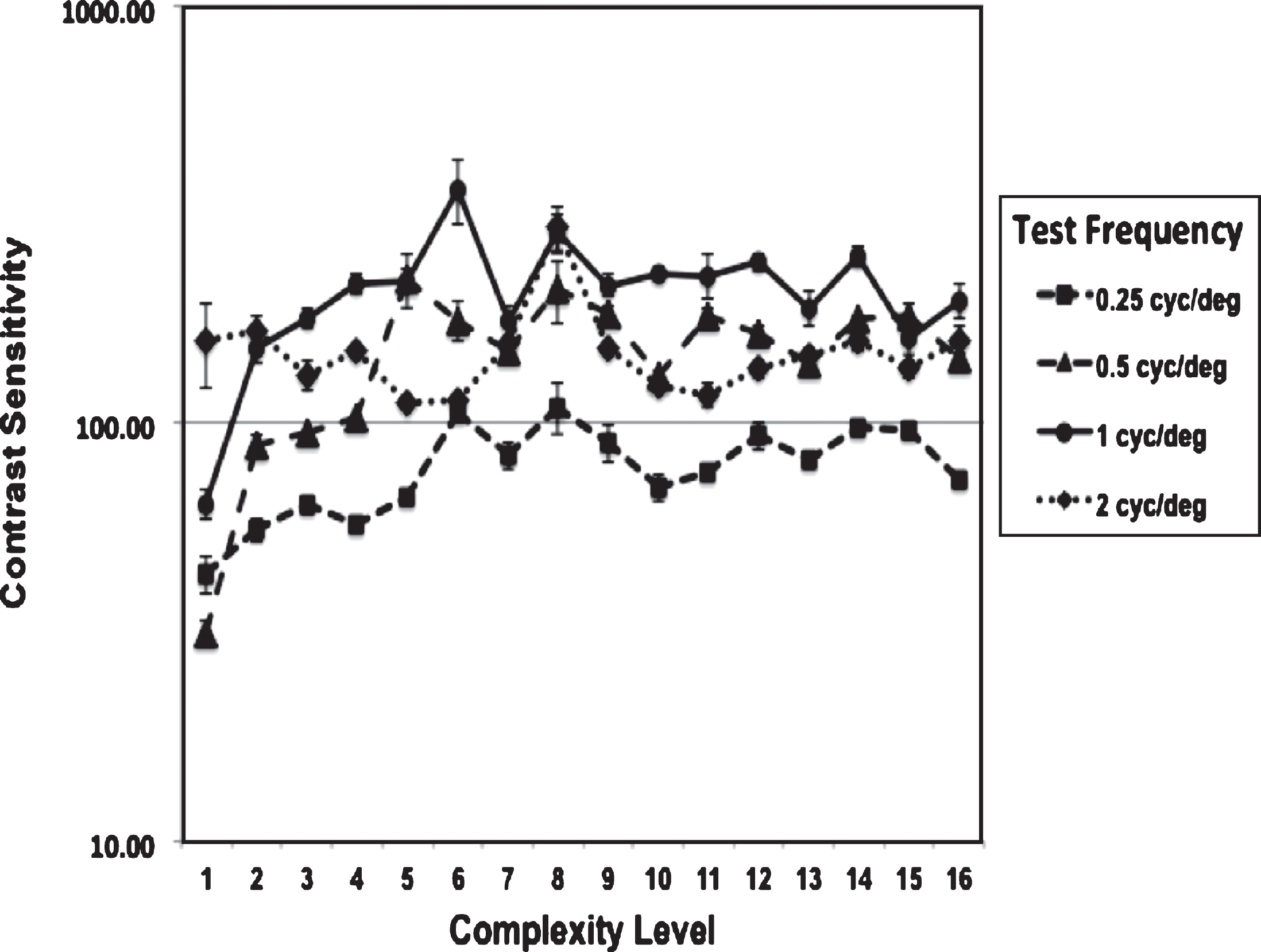
Fig.3
Mean and Standard Error of Improvements in Cognitive Skills following PATH neurotraining, all improvements being significant, as shown in Table 2. A. Improvements in movement discrimination deficits, where the degree of movement discrimination (MD) deficit corresponds to 1: Above Normal, 2: Normal, 3: Borderline, 4: Mild, 5: Moderate, 6: Markedly Below Normal; B. Standardized percentile rank of Processing Speed Index measured using WAIS-4 coding and symbol search subtests; C. Improvements in Reading Speed measured using the Computer-Based Reading Speed test, and the Nelson-Denny Reading Rate Test; D. Improvements in the Attention Network Test (ANT) for both Orienting and Executive Control Network; E. Improvements in Attention measured using the DKEFS color word interference test; F. Improvements in Working Memory (WM) measured using the Test of Information Processing Skills for Visual (VWM), Auditory (AWM), and Delayed Recall, and the WAIS-4 Working Memory Index (WMI).
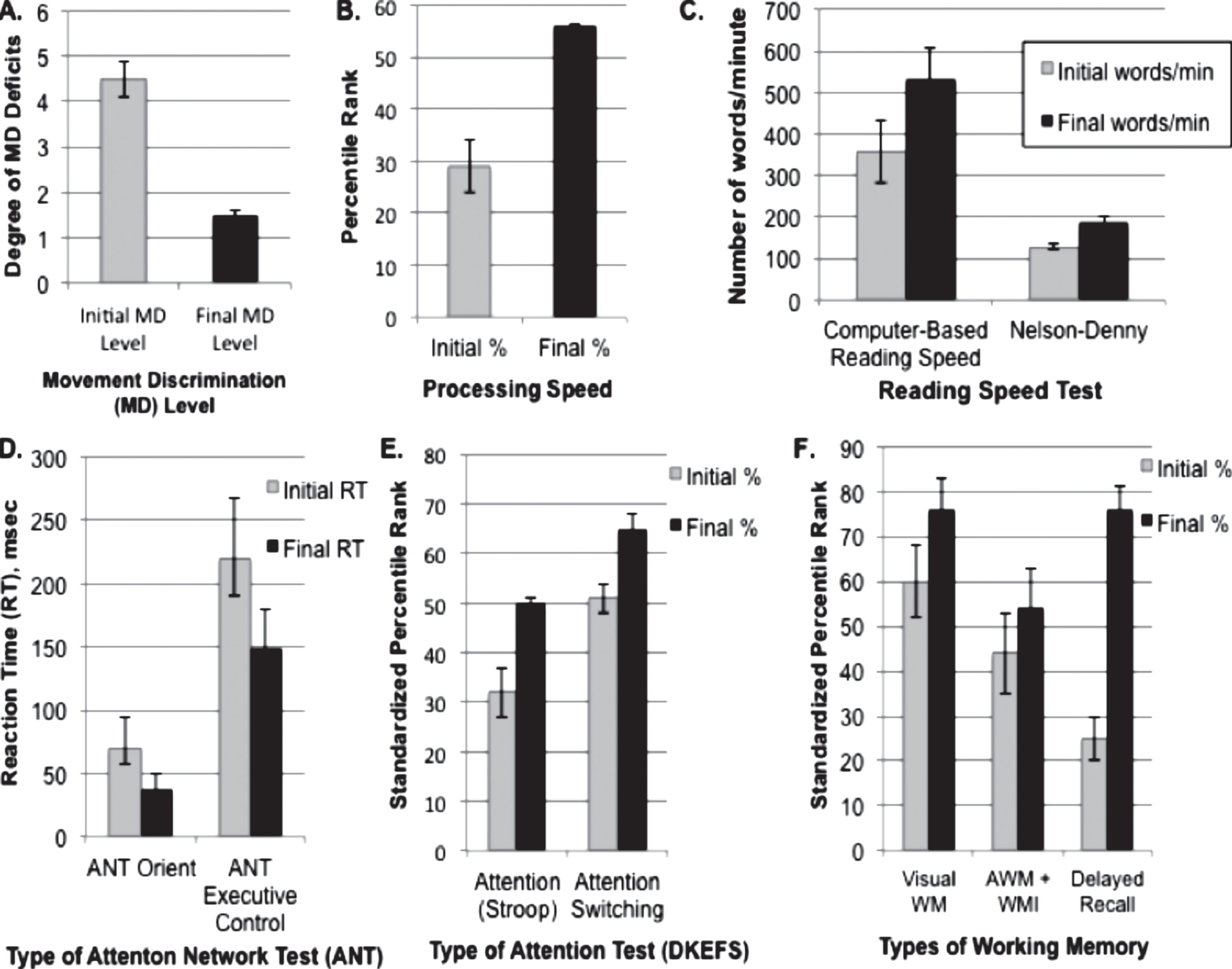
A two-factor ANOVA without replication was used to analyze the Contrast Sensitivity (CS) values. The average CS needed to discriminate left-right movement increased significantly, [F = 3.03, df = 15, p = 0.002], for the four TBI subjects as the complexity level was increased from 1 to 16, shown in Fig. 2. There was also a significant difference, [F = 31.6, df = 3, p < 0.0001], in the CS measured for different test spatial frequencies. The highest CS was measured for discriminating the direction the 1 cyc/deg test pattern moved (solid black line), 1 cyc/deg corresponding to the lowest spatial frequency channel, based on physiological data (Blakemore & Campbell, 1969).
These results found that direction discrimination sensitivity improved: 1) the most for the lowest spatial frequency channel, 1 cyc/deg, which moves twice as far in the same amount of time as the higher 2 cyc/deg test pattern, 2) the least for the 0.25 cyc/deg test pattern which requires pooling across spatial frequency channels to complete the task, and 3) more when a wider background frame of reference consisting of multiple spatial frequencies that are a harmonic (multiple) of the test frequency was presented, as found in typically developing observers (Lawton, 1985, 1989, Lawton & Shelley-Tremblay, 2017), and older adults (Lawton & Stephey, 2009). These results show that movement-discrimination training significantly improved visual skills for a wide range of ages, for those from 15 to 68 years old as shown in Table 2, p = 0.011, when p values were corrected using the B-H procedure to avoid false positives, having a large effect size of 1.67. These improvements are also shown in Fig. 3A, depicting the change in visual processing level measured by the PATH neurotraining diagnosis module before (mildly to moderately below normal) and after (above normal) movement-discrimination training. The pre/post comparisons in Fig. 3 provide an unbiased assessment of the resulting improvement in visual skills shown in Fig. 2.
3.2Cognitive skills improved significantly following training to improve visual timing
Pre/post comparisons using standardized tests found that training on direction discrimination significantly improved each TBI patient’s cognitive skills, see Table 2 and Figs. 3B-3E. All p values reported below correspond to those corrected to avoid false positives using the B-H procedure described in the Methods. Processing Speed, Fig. 3B, improved significantly, p = 0.021, having a relatively large effect size, 1.4, for such a small sample size. Reading speed measures, see Fig. 3C, using either the computer-based reading speed test that was not limited by the person’s rate of speaking, and the Nelson-Denny reading rate test that measured how many words were spoken in one minute improved significantly, p = 0.025, p = 0.018, respectively. When the reading speed scores were combined and the Bonferoni correction applied for two tests of reading speed, see Table 2, then reading speed improved significantly more, p = 0.007.
Attention also improved significantly as shown in Table 2 and Figs. 3D-3E. When attention was measured using the ANT, Fig. 3D, then both orienting and executive control attention measures improved significantly, p = 0.036, p = 0.029, with large effect sizes, 1.21 and 1.27 respectively. When attention was measured using the DKEFS color-word interference test, Fig. 3E, then both inhibition, i.e. attention, and inhibition switching, cognitive flexibility, improved significantly, p = 0.039, p = 0.014, respectively, having large effect sizes, 0.95 and 0.82 respectively, for such a small sample size.
Working memory also improved significantly, shown in Table 2 and Fig. 3E, with visual working memory (VWM) p = 0.046, auditory working memory (AWM) p = 0.05, the WAIS working memory index (WMI) p = 0.032, and Delayed Recall p = 0.043 having a large effect size equal to 1.85. Since both the AWM and WAIS were administered by having the subject repeat spoken letters and numbers and the initial and final mean scores were approximately the same values, the AVM and WMI scores were combined and plotted together in Fig. 3E, being significant at p = 0.004 when the Bonferoni correction was applied for these two tests of working memory. Since this was a small sample size, much larger significance levels and effect sizes are expected in future studies with larger sample sizes.
All subjects reported noticeable improvements in sequential processing, multitasking, movement figure-ground discrimination, and widening their useful field of view. The 15 year-old’s mom reported that she has seen a significant difference in her son’s behavior, reducing emotional and aggressive outbursts, improving reading, since words don’t seem jumbled anymore, improving his memory and focus of attention, both being a stumbling block to learning after his TBI, since completing the PATH neurotraining that has impacted his recovery significantly. He noticed that when he listens to people talk, the sound from their voice now matches the movement of their mouth, and that the tunnel effect experienced when his teachers spoke has lessened significantly, i.e. when his teachers’ spoke they seemed to be in a tunnel and by the time the words got back to him they were muffled and often illegible, being able to see their lips move, but not being able to understand what it was they were saying. The 50 year-old subject, who had incurred a severe TBI when a 20 pound boulder crashed through his car while driving home on the I-80 freeway seven years earlier, noticed that when riding his bicycle, he could now hear the sounds of the ocean and birds that were 3 blocks away, when before that was not possible. The 62 year-old was recovering from a stroke and found that only after PATH neurotraining was he able to recover cognitive speed, attentiveness, and short-term memory incurred from a massive hemorrhagic stroke and a TBI. The 68 year-old was very grateful for the many improvements in cognitive skills he noticed in his daily life after a short amount of movement-discrimination training, enabling him to read again.
3.3Brain Function measured using MEG imaging improved significantly
MEG scans on TBI patients confirm this improvement in brain function as well. MEG scans, shown in Fig. 4, found significant improvements in the functioning of the Dorsal Stream (V1, V3, MT, and MST), the Attention Networks (Anterior Cingulate Cortex (ACC), and precuneus/Posterior Cingulate Cortex (PCC), and the Executive Control Networks (DLPFC), in support of the behavioral changes in visual and cognitive functioning shown above. When analyzed using a paired-sample t-test (alpha = 0.05, two-tailed), these results were significant at p = 0.01 and the minimum effect size was 0.88. Following PATH neurotraining, over recruitment in brain areas outside the proper WM network was no longer needed.
Fig.4
Significant MEG signal increases (color scale is based on paired-sample t-tests having a t-value between 3 to 3.5 that correspond to significance levels between p = 0.01 to p = 0.001) in post- versus pre-PATH intervention exams. The MNI-152 Z-coordinates for those 4 slides were: 43, 20, 7 and –6 respectively. Green arrows: V1; Blue arrows: MT; Magenta arrows: V3; Cyan arrow: MST; Red arrows: ACC.; Yellow arrow: precuneus/PCC; White arrows: DLPFC.
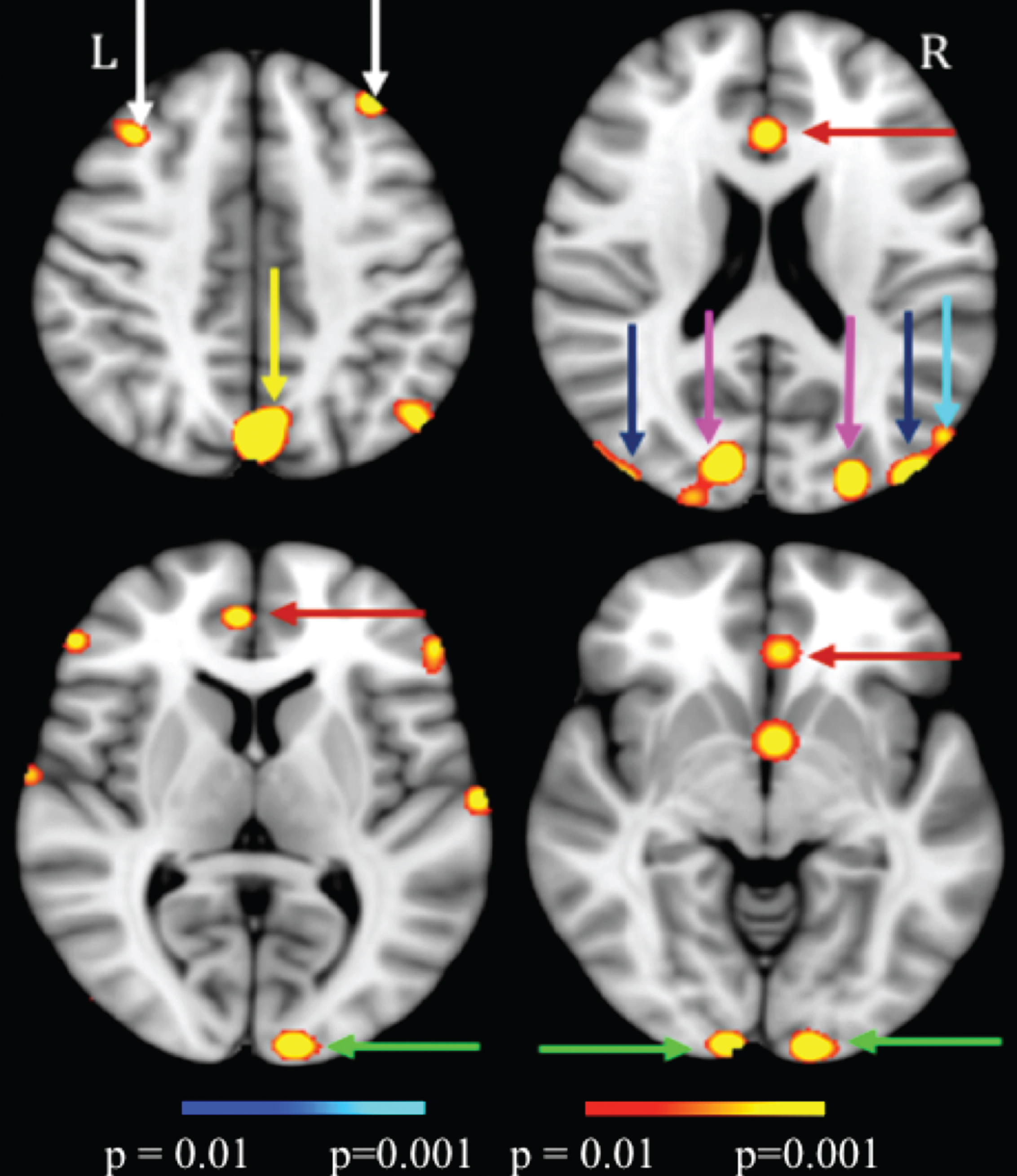
These results show that after a short amount of visual movement-discrimination training not only visual skills, but also many cognitive skills, including focusing and switching attention, processing speed, reading, and working memory improved significantly.
4Discussion
4.1Visual timing deficits can limit attention, reading, and memory acquisition
Our working hypothesis is that a TBI causes magnocellular neurons in the dorsal cortical visual pathway (V1-MT) to become more sluggish, causing visual timing deficits that disrupt processing at higher levels of dorsal stream processing, causing deficits in attention and executive control. These TBI patients thereby lacked the ability to focus attention, process sequential information quickly and accurately, multitask, and remember their train of thoughts. This study found that when movement-discrimination training was done using patterns optimal for activating the V1-MT network (Hupe, Payne, Lomer, Girad & Bullier, 1998; Allman, Miezin & McGuiness, 1985; De Valois, Cottaris, Mahon, Elfar & Wilson, 2000), these visual timing deficits were remediated for those with a TBI, causing attention, reading fluency, processing speed, and working memory, all high-level cognitive functions, to improve significantly. These behavioral improvements were also found by improved functionality of the dorsal, attention, and executive control networks, as shown by MEG brain source imaging. The magnocellular deficits found in those having a mild TBI, as shown previously (Poltavski et al., 2017) and in this study, were remediated quickly by completing a short amount of movement-discrimination training designed to optimally activate magno- relative to parvo- cellular neurons in the dorsal stream.
These improvements in cognitive skills following movement-discrimination training were found previously in typically-developing children (Lawton & Shelley-Tremblay, 2017) and dyslexics (Lawton, 2016) and substantiated by brain imaging studies in an adult dyslexic (Lawton & Huang, 2015). After a short amount of movement-discrimination training in dyslexic fourth graders three times/week for 6 weeks, dorsal stream activity improved as shown in Visual Evoked Potentials (Shelley-Tremblay, Syklawer & Ramkissoon, 2011), consistent with a recent pilot study using magnetoencephalography (MEG) source imaging (Lawton & Huang, 2015) that found improved function in both the dorsal stream (V1, V3, MT, MST areas) and fronto-parietal attention networks following 8 weeks of movement-discrimination training in an adult 29 year-old dyslexic for 10–15 minutes twice a week.
Visual timing deficits resulting from sluggish magnocellular (motion-sensitive) neurons in the dorsal stream are likely to be highly involved in the cognitive deficits of those with dyslexia (Lawton, 2011, 2016; Lawton & Shelley-Tremblay, 2017; Saalman, Pigarev & Vidyasagar, 2007; Vidyasagar, 1999), in older adults (Lawton & Stephey, 2009), and in those with a TBI (Poltavski et al., 2017). Convergent evidence is consistent with the suggestion of a relationship between visual dorsal stream processing and cognitive abilities, such that poor dorsal stream processing relates to slower timing (Lawton, 2011, 2016; Lawton & Shelley-Tremblay, 2017), causing deficits in visual and cognitive skills. Slower processing speeds and more effortful attention have been found to be prevalent in TBI patients (Ghajar & Ivry, 2008; Husiman et al., 2004). Reduced information processing speed can explain problems in memory encoding and retrieval, because this mental slowing can lead to superficial processing and inefficient strategies where over recruitment of cortical areas is required (Jolles, 1986). In this study, improvements in visual and cognitive skills at different levels of processing in the dorsal stream and attention and executive control networks were found following movement-discrimination neurotraining for the TBI subjects both behaviorally and using MEG brain imaging. This study found that this neurotraining opened the attention gateway so less effort was spent decoding incoming information, and more effort was spent on interpreting the information, improving cognitive skills by using the proper WM network.
4.2Movement discrimination relative to stationary background which is mediated by the V1-MT network represents a core deficit in a TBI
This study found that a core deficit in TBI subjects may be difficulty in discriminating the movement of an object relative to its background, this task being analyzed within the dorsal stream (Allman et al., 1985). This hypothesis is consistent with the person having a mild TBI having cognitive deficits caused by the disruption of connectivity of cortical areas in the dorsal stream, found to affect the brain’s predictive capability, resulting in deficits in goal-oriented behavior arising from problems with attention and working memory (Ghajar & Ivry, 2008). Goal-oriented behavior is normally transmitted both by feedforward magnocellular (low-contrast movement) input, and from feedback at the attended location from lateral inferior-parietal (LIP) to middle temporal (MT) cortex (Stein, 2001) and from MT to V1 (primary visual cortex) (Hupe et al., 1998). Moreover, feedback in the dorsal stream from MT to V1 improves movement figure-ground discrimination (Hupe et al., 1998), such as low contrast patterns that maximally activate magnocellular neurons (Kaplan & Shapley, 1986; Sclar, Maunsell & Lennie, 1990), as used in this study. Training with the stationary background frame of reference provided by single and multifrequency backgrounds improved the TBI subject’s ability to discriminate the direction of movement, most likely by taking advantage of MT’s center-surround organization (Allman et al., 1985) to facilitate movement discrimination, enabling the person with a TBI to improve in reading fluency and processing speed, attend to wider regions of space, and remember more easily.
Previous results (Lawton, 1985, 1989, 2011, 2016; Lawton & Shelley-Tremblay, 2017) support the hypothesis that multifrequency backgrounds confer an advantage when discriminating the direction of motion, by providing a wider, more structured frame of reference. Even though the dorsal stream consists of predominantly magnocellular neurons, there is input to the dorsal stream from parvocellular neurons (Nassi, Lyon & Callaway, 2006; Callaway, 1998; Maunsell et al., 1990) from the lateral geniculate nucleus (lgn), V1, and V4, all projecting to MT, enabling parvocellular activity to provide a background frame of reference for discriminating the direction of movement in the dorsal stream. This stationary background frame of reference makes motion discrimination easier (Lawton, 1985, 1989, 2016).
The significant improvements in processing speed, attention and working memory following movement-discrimination training suggests that training early in the visual dorsal stream improved higher levels of processing in the dorsal stream, and in particular the PPC where: 1) spatial attention has been demonstrated to feedback to early visual cortical areas (Watanabe et al., 1998; Martinez et al., 1999; Somers et al., 1999), and 2) selective endogenous attention is encoded (Posner et al, 1984; Menon & Uddin, 2010), the PPC projecting to the DLPFC, where working memory is encoded (Menon & Uddin, 2010). The control of spatial attention in early visual cortex is likely to be directed by regions of the PPC and DLPFC (Silver, Ress & Heeger, 2005).
4.3Improving visual timing improves feedback from high cortical areas to lower levels
The sluggish magnocellular neurons appear to not only result in attention deficits, an impairment in the low gamma frequencies reducing feedback in visual cortical areas (Vidyasagar, 2013), but also disrupted processing in lateral intraparietal cortex (LIP) and Frontal Eye Fields (FEF), either within a fixation, between fixation sequences, or both (Vidyasagar, 1999; Fischer, 2012). Moreover, finding that movement-discrimination training improved not only reading fluency, but also attention and working memory in those with a TBI indicates that movement-discrimination training helps develop the attention and executive control networks, also shown by MEG imaging. This study provides more evidence that abnormal visual motion processing is a fundamental cause of reading, attention, and memory problems in those with a TBI. By improving the attention network’s functioning, movement-discrimination training provides a wider usable field of view so that more objects are perceived in their correct location in a single glance (Lawton & Stephey, 2009). Movement-discrimination training improves a person’s ability to detect the synchronicity of multiple objects in space and their trajectories over time, most likely by increasing the ease of magno-parvo integration, thereby facilitating movement discrimination relative to a stationary background within a wider window of focused attention (Lawton & Shelley-Tremblay, 2017). Moreover, there is evidence that improvements in cognitive skills after this movement-discrimination training are sustained over time (Lawton, 2011).
5Conclusions
PATH neurotraining is designed to activate the dorsal stream at both: 1) low levels (V1-MT network), improving visual processing, and 2) at high levels, improving attention (PPC), and working memory (DLPFC), improving high-level cognitive skills (Lawton & Huang, 2015; Lawton, 2016; Lawton & Shelley-Tremblay, 2017). Improving cognitive function by training on left-right movement discrimination is a novel method (Lawton, 2000, 2015) that this study found to be both rapid and effective in improving cognitive skills in TBI patients. Only when low-level visual timing deficits are remediated in those with a TBI are the improvements in high-level cognitive functions, such as reading fluency, attention, and memory, commonly found after a mild TBI, improved quickly, these improvements being sustained over time. This is the first time that a study has found that improving low-level movement discrimination in the dorsal stream improves high-level cognitive functioning, both behaviorally and using MEG brain imaging, improving the attention and executive control networks in those with a TBI. Additional studies with a larger sample size are needed to verify that these improvements are sustained over time. Since movement-discrimination (PATH) neurotraining is so rapid and effective in remediating cognitive deficits, it offers a new approach that is very beneficial for treating a mild TBI.
Acknowledgments
This work was supported in part by Merit Review Grants from the Department of Veterans Affairs to M.X. Huang (I01-CX000499, I01-RX001988, MHBA-010-14F, NURC-022-10F, NEUC-044-06S). We thank our research associates, Ashley Robb Swan and Annemarie Angeles Quinto, at the VA San Diego Healthcare System and the University of California, San Diego. We also thank Dr. John Shelley-Tremblay for doing the behavioral statistical analysis.
The first author TL has a potential conflict of interest, since she is the developer of PATH to Insight (PATH), and was employed by Perception Dynamics Institute. The role of this author was to design the behavioral study, recruit and train staff, run daily operations and help write the article. She had no part in collecting or analyzing the data, thereby having no influence over the results we obtained. The other author MXH declares that the research was conducted in the absence of any commercial or financial relationships that could be construed as a potential conflict of interest.
References
1 | Allman, J. , Miezin, F. , McGuiness, E. . ((1985) ). Stimulus specific responses from beyond the classical receptive field: Neurophysiological mechanisms for local-global comparisons in visual neurons. Annual Review of Neuroscience, 8: , 407–430. |
2 | Battelli, L. , Pascual-Leone, A. , Cavanagh, P. . ((2007) ). The ‘when’ pathway of the right parietal lobe. Trends In Cognitive Science, 11: , 204–210. |
3 | Benjamini, Y. , Hochberg, Y. . ((1995) ). Controlling the false discovery rate: A practical and powerful approach to multiple testing. Journal of the Royal Statistical Society, Series B, 57: , 289–300. |
4 | Blakemore, C. Campbell,F.W.. ((1969) ). On the existence of neurones in the human visual system selectively sensitive to the orientation and size of retinal images. Journal of Physiology, 203: , 237–260. |
5 | Buzsaki, G. . ((2006) ). The Rhythms of the Brain. Oxford University Press. |
6 | Callaway, E.M. . ((1998) ). Local circuits in primary visual cortex of the macaque monkey. Annual Review of Neuroscience, 21: , 47–74. |
7 | De Valois, R.L. , Cottaris, N.P. , Mahon, L.E. , Elfar, S.D. , Wilson, J.A. . ((2000) ). Spatial and temporal receptive fields of geniculate and cortical cells and directional selectivity. Vision Research, 40: , 3685–3702. |
8 | Fan, J. , McCandliss, B.D. , Fossella, J. , Flombaum, J.I. , Posner, M.I. . ((2005) ). The activation of attentional networks. NeuroImage, 26: , 471–479. |
9 | Faul,F. Erdfelder,E. Lang,A.-G. , & Buchner,A. ((2007) ). G*Power 3: A flexible statistical power analysis program for the social, behavioral, and biomedical sciences. Behavior Research Methods, 39: , 175–191. |
10 | Faul, M. , Xu, L. , Wald, M.M. , Coronado, V.G. . ((2010) ). Traumatic Brain Injury in the United States: Emergency Department Visits, Hospitalizations, and Deaths 2002–2006. Atlanta, GA: Centers for Disease Control and Prevention, National Center for Injury Prevention and Control, 1–74. |
11 | Felleman, D.J. , Van Essen, D.C. . ((1991) ). Distributed hierarchical processing in the primate cerebral cortex. Cerebral Cortex, 1: , 1–47. |
12 | Fischer, B. . ((2012) ). Subitizing, dynamic vision, saccade and fixation control in dyslexia, In: Stein,J. . & Kaoula,Z., . editors. Visual Aspects of Dyslexia. Oxford University Press. pp. 15–43. |
13 | Gevins, A.S. , Cutillo, B.C. . ((1993) ). Neuroelectric evidence for distributed processing in human working memory. Electroencephalography and Clinical Neurophysiology, 87: , 128–143. |
14 | Ghajar, J. , Ivry, R.B. . ((2008) ). The predictive brain state: Timing deficiency in traumatic brain injury?. Neurorehabilitation and Neural Repair, 22: , 217–227. |
15 | Grabner, G. , Janke, A.L. , Budge, M.M. , Smith, D. , Pruessner, J. , Collins, D.L. . ((2006) ). Symmetric atlasing and model based segmentation: An application to the hippocampus in older adults. Medical Image Computing and Computer Assisted Intervention, 9: , 58–66. |
16 | Higgins, K.E. , Jaffe, M.J. , Coletta, N.J. , Caruso, R.C. , de Monasterio, F.M. . ((1984) ). Spatial Contrast Sensitivity. Archives of Ophthalmology, 102: , 2067–2074. |
17 | Kolster, H. , Peeters, R. , Orban, G.A. . ((2010) ). The retinotopic organization of the human middle temporal area MT/V5 and its cortical neighbors. The Journal of Neuroscience, 30: (29), 9801–9820. |
18 | Huang, M.X. , Huang, C.W. , Robb, A. , Angeles, A. , Nichols, S.L. , Baker, D.G. , Song, T. , Harrington, D.L. , Theilmann, R.J. , Srinivasan, R. , Heister, D,. , Diwakar,M. Canive, J.M. , Edgar, J.C. , Chen, Y.-H. , Ji, Z. , Shen, M. , El-Gabalawy, F. , Levy, M. , McLay, R. , Webb-Murphy, J. , Liu, T.T. , Drake, A, . . Lee,R.R. ((2014) ). MEG source imaging method using fast L1 minimum-norm and its applications to signals with brain noise and human resting-state source amplitude images. NeuroImage, 84: , 585–604. |
19 | Huang, C.W. , Huang, M.X. , Ji, Z. , Swan, A.R. , Angeles, A.M. , Song, T. , Huang, J.W. , Lee, R.R. . ((2016) ). High-resolution MEG source imaging approach to accurately localize Broca’s area in patients with brain tumor or epilepsy. Clinical Neurophysiology, 127: , 2308–2316. |
20 | Huisman, T.A. , Schwamm, L.H. , Schaefer, P.W. , Koroshetz, W. J, . , Shetty-Alva,N. Ozsunar, Y. , Wu, O. , Sorensen, A.G. . ((2004) ). Diffusion tensor imaging as potential biomarker of white matter injury in diffuse axonal injury. American Journal of Neuroradiology, 25: , 370–376. |
21 | Hupe, J.M. , Payne, A.C. , Lomer, B.R. , Girad, S.G. , Bullier, J. . ((1998) ). Cortical feedback improves discrimination between figure and background by V1, V2, and V3 neurons. Nature, 39: , 784–787. |
22 | Hyvarinen, A. . ((1999) ). Fast and robust fixed-point algorithms for independent component analysis. IEEE Transactions on Neural Networks, 10: , 626–634. |
23 | Hyvarinen, A. , Oja, E. . ((2000) ). Independent component analysis: Algorithms and applications. Neural Networks, 13: , 411–430. |
24 | Jolles, J. . ((1986) ). Cognitive, emotional and behavioral dysfunctions in aging and dementia. Progress in Brain Research, 70: , 15–39. |
25 | Kaplan, E. , Shapley, R.M. . ((1986) ). The primate retina contains two types of ganglion cells, with high- and low-contrast sensitivity. Proceedings of the National Academy of Sciences, 83: , 2755–2757. |
26 | Knight, R.T. . ((2007) ). Neuroscience. Neural networks debunk phrenology. Science, 316: , 1578–1579. |
27 | Lawton, T. . ((1984) ). The effect of phase structures on spatial phase discrimination. Vision Research, 24: , 139–148. |
28 | Lawton, T. . ((1985) ). Spatial Frequency Spectrum of Patterns Changes The Visibility Of Spatial-Phase Differences. Journal of the Optical Society of America A, 2: , 1140–1152. |
29 | Lawton, T. . ((1989) ). Outputs of paired Gabor filters summed across background frame of reference predicts direction of movement. IEEE Transactions on Biomedical Engineering, 36: , 130–139. |
30 | Lawton,T. ((2000) ). Methods and Apparatus For Diagnosing and Remediating Reading Disorders. United States Patent No 6,045,515, Washington, DC: U.S. Patent and Trademark Office. |
31 | Lawton,T. ((2007) ). Training direction-discrimination sensitivity remediates a wide spectrum of reading skills. Optometry & Vision Development, 38: , 37–51. |
32 | Lawton, T. . ((2011) ). Improving magnocellular function in the dorsal stream remediates reading deficits. Optometry and Visual Development, 42: , 142–154. |
33 | Lawton, T. . ((2015) ). Diagnosing and Remediating Cognitive Deficits Involving Attention, Sequential Processing, Reading, Speed of Processing, and Navigation. United States Patent No. 8,979,263 B2, Washington, DC: U.S. Patent and Trademark Office. |
34 | Lawton, T. . ((2016) ). Improving Dorsal Stream Function in Dyslexics by Training Figure/Ground Motion Discrimination Improves Attention, Reading Fluency, and Working Memory. Frontiers in Human Neuroscience, 397: . 10.3389/fnhum.2016.00397. |
35 | Lawton, T. , Huang, M.X. . ((2015) ). Improving Visual Timing Enhances Cognitive Functioning by Altering Dorsal Stream and Attention Networks, 12th Annual World Congress of the Society for Brain Mapping and Therapeutics, Los Angeles, CA. March 7.. |
36 | Lawton, T. , Shelley-Tremblay, J. . ((2017) ). Training on movement figure-ground discrimination remediates low-level visual timing deficits in the dorsal stream, improving high-level cognitive functioning, including attention, reading fluency, and memory. Frontiers in Human Neuroscience, https://www.frontiersin.org/articles/10.3389/fnhum.2017.00236/full. |
37 | Lawton, T. , Stephey, D. . ((2009) ). Training direction discrimination improves usable field of view, short term memory, and navigation in older adults. Optometry and Visual Development, 40: , 82–93. |
38 | Livingstone, M.S. , Hubel, D.H. . ((1988) ). Segregation of color, form, movement and depth: Anatomy, physiology, and perception. Science, 240: , 740–749. |
39 | Livingstone, M.S. , Rosen, G.D. , Drislane, F.W. , Galaburda, A.M. . ((1991) ). Physiological and anatomical evidence for a magnocellular defect in developmental dyslexia. Proceedings of the National Academy of Sciences, 88: , 7943–7947. |
40 | Martinez, A. , Anllo-Vento, L. , Sereno, M.I. , Frank, L.R. , Buxton, R.B. , Dubowitz, D.J. , Wong, E.C. , Hinrichs, H.J. , Hillard, S.A. . ((1999) ). Involvement of striate and extrastriate cortical areas in spatial attention. Nature Neuroscience, 2: , 364–369. |
41 | Maunsell, J.H. , Nealey, T.A. , DePriest, D.D. . ((1990) ). Magnocellular and parvocellular contributions to responses in the middle temporal visual area (MT) of the macaque monkey. The Journal of Neuroscience, 10: , 3323–3334. |
42 | Menon, V. , Uddin L. Q. . ((2010) ). Saliency, switching, attention and control: A network model of insula function. Brain Structure and Function, 214: , 655–667. |
43 | Naghavi, H.R. , Nyberg, L. . ((2005) ). Common fronto-parietal activity in attention, memory, and consciousness: Shared demands on integration? Consciousness and Cognition, 14: , 390–425. |
44 | Nassi, J.J. , Lyon, D.C. , Callaway, E.M. . ((2006) ). The parvocellular LGN provides a robust disynaptic input to the visual motion area MT. Neuron, 50: , 319–327. |
45 | Owen, A.M. , McMillan, K.M. , Laird, A.R. , Bullmore, E. . ((2005) ). N-back working memory paradigm: A meta-analysis of normative functional neuroimaging studies. Human Brain Mapping, 25: , 46–59. |
46 | Phillips, N.L. , Parry, L. , Mandalis, A. , Lah, S. . ((2017) ). Working memory outcomes following traumatic brain injury in children: A systematic review with meta-analysis. Child Neuropsychology, 23: , 26–66. |
47 | Pitzalis, S. , Sereno, M.I. , Committeri, G. , Fattori, P. , Galati, G. , Patria, F. , Galletti, C. . ((2010) ). Human V6: The medial motion area. Cerebral Cortex, 20: (2), 411–424. |
48 | Poltavski, D. , Lederer, P. , Cox, L.K. . ((2017) ). Visually evoked potential markers of concussion history in patients with convergence insufficiency. Optometry and Vision Sciences, 94: , 742–750. |
49 | Posner, M.I. , Walker, J.A. , Friedrich, F.J. , Rafal, R.D. . ((1984) ). Effects of parietal injury on covert orienting of attention. The Journal of Neuroscience, 4: , 1863–1874. |
50 | Saalman, Y.B. , Pigarev, I.N. , Vidyasagar, T.R. . ((2007) ). Neural mechanism of visual attention: How top-down feedback highlights relevant locations. Science, 316: , 1612–1615. |
51 | Saalman, Y.B. , Pinsk, M.A. , Wang, L. , Li, X. , Kastner, S. . ((2012) ). The pulvinar regulates information transmission between cortical areas based on attention demands. Science, 337: , 753–756. |
52 | Sclar, G. , Maunsell, J. H. R. , Lennie, P. . ((1990) ). Coding of image contrast in central visual pathways of the macaque monkey. Vision Research, 30: , 1–10. |
53 | Shelley-Tremblay, J.F. , Syklawer, S. , Ramkissoon, I. . ((2011) ). The effect of magno-parvocellular integration training on fluency and visual evoked potentials in poor readers. Journal of Behavioral Optometry, 22: , 31–37. |
54 | Silver, M.A. , Ress, D. , Heeger, D.J. . ((2005) ). Topographic maps of visual spatial attention in human parietal cortex. Journal of Neurophysiology, 94: , 58–71. |
55 | Smith, S.M. , Jenkinson, M. , Woolrich, M.W. , Beckmann, C.F. , Behrens, T.E. , Johansen-Berg, H. , Bannister, P.R. , De, L.M. , Drobnjak, I. , Flitney, D.E. , Niazy, R.K. , Saunders, J. , Vickers, J. , Zhang, Y. , De, S.N. , Brady, J.M. , Matthews, P.M. . ((2004) ). Advances in functional and structural MR image analysis and implementation as FSL. NeuroImage, 23: (Suppl 1), S208–S219. |
56 | Somers, D.C. . ((2014) ). Attentional “Spotlight” in Early Visual Cortex. New Visual Neuorsciences, Werner,J.S., & Chalupa,L.M., editors, MIT Press, pp. 1097–1106. |
57 | Somers, D.C. , Dale, A.M. , Seiffert, A.E. , Tootell, R.B. . ((1999) ). Functional MRI reveals spatial specific attentional modulations in human primary visual cortex. Proceedings of the National Academy of Sciences, 96: , 1665–1668. |
58 | Song, T. , Gaa, K. , Cui, L. , Feffer, L. , Lee, R.R. , Huang, M.X. . ((2008) ). Evaluation of signal space separation via simulation. Medical & Biological Engineering & Computing, 46: , 923–932. |
59 | Stein, J. . ((2001) ). The magnocellular theory of developmental dyslexia. Dyslexia, 7: , 12–36. |
60 | Taulu, S. , Kajola, M. , Simola, J. . ((2004) ). Suppression of interference and artifacts by the Signal Space Separation Method. Brain Topography, 16: , 269–275. |
61 | Taulu, S. , Simola, J. , Kajola, M. . ((2004) ). MEG recordings of DC fields using the signal space separation method (SSS). Neurology & Clinical Neurophysiology, 35: , 1–4. |
62 | Vidyasagar, T.R. . ((1999) ). A neuronal model of attentional spotlight: Parietal guiding the temporal. Brain Research Reviews, 30: , 66–76. |
63 | Vidyasagar, T.R. . ((2013) ). Reading into neuronal oscillations in the visual system: Implications for developmental dyslexia. Frontiers in Human Neuroscience, 7: , 1–10. |
64 | Watanabe, T. , Sosaki, Y. , Miyauchi, S. , Putz, B. , Fujimaki, N. , Nielsen, M. Takino, R. , Miyakawa, S. . ((1998) ). Attention-regulated activity in human primary visual cortex. Journal of Neurophysiology, 79: , 2218–2221. |
65 | Woolrich, M.W. , Jbabdi, S. , Patenaude, B. , Chappell, M. , Makni, S. , Behrens, T. , Beckmann, C. , Jenkinson, M. , Smith, S.M. . ((2009) ). Bayesian analysis of neuroimaging data in FSL. NeuroImage, 45: , S173–S186. |