Robotic gaming prototype for upper limb exercise: Effects of age and embodiment on user preferences and movement
Abstract
Background:
Effective human-robot interactions in rehabilitation necessitates an understanding of how these should be tailored to the needs of the human. We report on a robotic system developed as a partner on a 3-D everyday task, using a gamified approach.
Objectives:
To: (1) design and test a prototype system, to be ultimately used for upper-limb rehabilitation; (2) evaluate how age affects the response to such a robotic system; and (3) identify whether the robot’s physical embodiment is an important aspect in motivating users to complete a set of repetitive tasks.
Methods:
62 healthy participants, young (<30 yo) and old (>60 yo), played a 3D tic-tac-toe game against an embodied (a robotic arm) and a non-embodied (a computer-controlled lighting system) partner. To win, participants had to place three cups in sequence on a physical 3D grid. Cup picking-and-placing was chosen as a functional task that is often practiced in post-stroke rehabilitation. Movement of the participants was recorded using a Kinect camera.
Results:
The timing of the participants’ movement was primed by the response time of the system: participants moved slower when playing with the slower embodied system (p = 0.006). The majority of participants preferred the robot over the computer-controlled system. Slower response time of the robot compared to the computer-controlled one only affected the young group’s motivation to continue playing.
Conclusion:
We demonstrated the feasibility of the system to encourage the performance of repetitive 3D functional movements, and track these movements. Young and old participants preferred to interact with the robot, compared with the non-embodied system. We contribute to the growing knowledge concerning personalized human-robot interactions by (1) demonstrating the priming of the human movement by the robotic movement – an important design feature, and (2) identifying response-speed as a design variable, the importance of which depends on the age of the user.
1Introduction
Intensity of training and transfer to activities of daily living are key to successful rehabilitation (Gerling et al., 2012; Veerbeek et al., 2017). Intense repetitions lead to an improvement in the ability to perform certain motor tasks, accompanied by a modification of brain activation patterns in some cases (Luft et al., 2004). Several recent studies showed evidence that movement frequency and patient engagement are the most important factors that influence the rehabilitation process (Blank et al., 2014; Kahn et al., 2006). This has led to growing interest in developing devices that support the rehabilitation process and increase patients’ motivation (Bertani et al., 2017; Fasola & Mataric, 2012; Maciejasz et al., 2014; Schoone et al., 2007). How to integrate productive rehabilitation tasks with motivational features is an especially challenging open question (Duret et al., 2015; Nordin et al., 2014).
Early research on systems for upper-limb rehabilitation used robotic manipulators to guide the patient’s hand and arm to the desired positions, typically immobilizing the patient’s wrist to ensure that the desired motions were produced (Krebs et al., 1998). Early clinical trials indicated the clinical benefits of using robotic manipulators in the rehabilitation process, and ensured safety for the participants (Aisen et al., 1997; Krebs et al., 1998; Lum et al., 1999). Later devices expanded the capabilities of robotic manipulators in rehabilitation by adding wrist and hand modules attached to previously developed arm devices (Blank et al., 2014) including exoskeletons, which isolated the motion of specific joints (Lo & Xie, 2012). Those devices eliminate compensatory behaviors by targeting specific joints. However, the trade-off is that they reduce the motion ranges when compared to one-on-one rehabilitation with a therapist (Lo & Xie, 2012).
Alongside the mechanical design of the rehabilitation system, it is important to design the interaction with the user, so as to increase users’ motivation to practice their rehabilitation exercises with the system (Matarić et al., 2007; Tapus et al., 2008). One method to increase patient motivation to exercise is through virtual reality (VR) gaming environments. There has been a wide variety of research on using VR games for upper limb training and rehabilitation aiming for high patient engagement and motivation (Harley et al., 2011) leading to numerous repetitions of a prescribed task (Fasola & Mataric, 2012; Levin et al., 2015). Compared with one-on-one rehabilitation with a therapist, rehabilitation with VR is more readily available and requires fewer resources. When combined with one-on-one rehabilitation with a therapist, VR rehabilitation improves treatment time and cost efficiency (Levin et al., 2015). In addition, VR games enable free motions resulting in a larger variety of motion training and at a low cost (Levin et al., 2015; Rand et al., 2009).
Although some rehabilitation VR environments reflect real-life contexts, such as simulating shopping in a supermarket (Rand et al., 2009), they do not include all of the sensory-motor interactions that people experience in a real setting. 3D functional Activities of Daily Living (ADL) (Gerling et al., 2012) are activities that people do routinely to accomplish everyday goals, and are a key objective for many rehabilitation settings (Kwakkel & Kollen, 2013). Examples of ADL are pick-and-place movements: e.g., picking up a cup from a counter and placing it on a shelf, as opposed to simply lifting one’s arm upwards, which does not directly serve a particular purpose. Functional 3D rehabilitation can also include handling real-life objects, which give veridical haptic feedback that is not available when acting within a fully simulated context. Training which is not functional limits how well patients can transfer the benefits of rehabilitation exercises to real world scenarios (Bertani et al., 2017). For example, studies that investigated the outcomes of using virtual environments for upper limb rehabilitation found evidence for increased arm strength, but the effects on arm use in daily activities were not conclusive (Feys et al., 2015; Holden et al., 1999).
In parallel to the rapid expansion in the field of VR, several studies analyzed the importance of physical presence or embodiment of robotic systems for motivating completion of various tasks. Matarić and colleagues designed a socially assistive robot (SAR) for monitoring and encouraging rehabilitation exercises (Matarić et al., 2007; Tapus et al., 2008). They showed that the physical presence of the robot motivated participants in the exercises despite the lack of physical contact with the participants (i.e., they used a vocal hands-off interaction, where the robot wandered around the participant). Studies by Scassellati and colleagues showed that physical presence leads to increased learning performance and motivation (Leyzberg et al., 2014), and that physical presence of a robotic system (compared to a live video of the same robot) leads to a more positive interaction (Bainbridge et al., 2011). These studies highlighted the importance of the social aspect of a robotic entity in motivating people to perform certain tasks. They further reveal the complexity and multidimensionality of patient motivation in robot-mediated rehabilitation contexts.
There are many open questions concerning the effect on motivation of specific robotic parameters, such as physical embodiment, physical contact with the patient, speed of response, and the social role of the robot. This study aims to advance our understanding of the effect of some of these parameters on patient motivation, as well as of how to effectively integrate motivational parameters with rehabilitation goals.
In the current study, a prototype system that integrates a 3D functional task with a robotic system, using a gamification approach was developed and tested on young and old healthy individuals. The ultimate goal is to use this system in a rehabilitation scheme that requires repetition of functional daily movements, and to turn an otherwise rather boring task (practicing a pick-and-place movement over and over) into an engaging one, by harnessing the benefits of gamification and robotics.
Specifically, the three main goals of the current study were to: (1) develop and test a prototype system for the improvement of the upper limb rehabilitation process; Specifically, design an interactive system capable of motivating the patient for repetitive practicing of 3D functional ADL tasks, and monitoring performance; (2) evaluate whether people of different ages respond to and interact with such a robotic gaming system differently, and (3) identify whether a robot’s physical entity, or embodied presence, is an important aspect in motivating users to complete a set of repetitive tasks.
To achieve these goals, we used a functional pick-and place task of picking up a cup and placing it on a shelf. We gamified this task, by designing a 3-D game of Tic-Tac-Toe, where physical cups of different colors were placed on a shelf grid, at different heights, with each partner attempting to win by placing a series of three cups of the same color in sequence.
Two different 3D Tic-Tac-Toe interactive systems were developed: one using a robotic arm and the other using a computer-controlled lighting system. Two different age groups (young and older) were recruited to evaluate if they interact differently with the system, since aging affects movement parameters (Levy-Tzedek et al., 2010; Levy-Tzedek et al., 2016; Levy-Tzedek, 2017a,b), and may also affect user preferences when interacting with a robotic system (De Graaf & Allouch, 2013). The outcomes were evaluated using the Godspeed questionnaire (Bartneck et al., 2009) and a custom-made questionnaire, together with motion analysis, which was performed on data captured from a Kinect 2.0 camera.
2Methods
2.1Participants
Sixty-two healthy right-handed participants from two different age groups were tested: (1) 40 young students aged 25.6±1.7 years, 23 females and 17 males from whom 23 had previous robotics experience and 17 did not; and (2) 22 older adults aged 73.3±6.2 years, 10 females and 12 males from whom nine had previous robotic experience and 13 did not. Experience was determined based on a preliminary questionnaire. All participants gave their written informed consent to participate.
2.2The game
In a traditional Tic-Tac-Toe game, two opponents play against each other, using pen and paper. On a 3×3 drawn grid, they each, in turn, draw either a circle or an ‘x’, and aim to win by reaching a sequence of three circles or three ‘x’s in a row (horizontal, vertical or diagonal). Here, we modified the game, and used a physical 3D grid with shelves, on which the participants placed cups of one color (green), instead of drawing circles or ‘x’s. The system against which they played either placed blue cups on the grid (in the case of the robotic system), or turned on blue lights at the chosen location on the grid (in the case of the computer-controlled lighting system). The goal here, as in the original game, was to reach a sequence of three cups (or lights) of the same color in a row (horizontal, vertical or diagonal). The participants always placed actual cups on the grid, regardless of the configuration they played against (robot/computer).
2.3Equipment
Each of the gaming configurations described below included a Logitech C270 webcam and 10 cups in two different colors (five in each color, for each player, see Fig. 1). Motion quality was quantified using position data collected from a Kinect 2.0 tracking system. The Kinect system outputs a reconstruction of the participant’s skeleton at a rate of 10 Hz.
Fig.1
The experimental system. Left – a participant playing against the robotic system. Right - The central 60×60 cm zone on the larger 1×1m physical grid was used for this experiment. The participant stood on one side of the grid, and the robotic arm was placed on the other side of it. Each had a set of five cups from one color (green for the human, blue for the robot), and placed one cup during each turn. When using the computer-controlled configuration, the robotic arm was not active, and, rather than placing physical cups, the system indicated its choice by turning on blue-colored LEDs in the chosen cell. The LED lights were enclosed in white ping-pong balls affixed to the top of each cell.
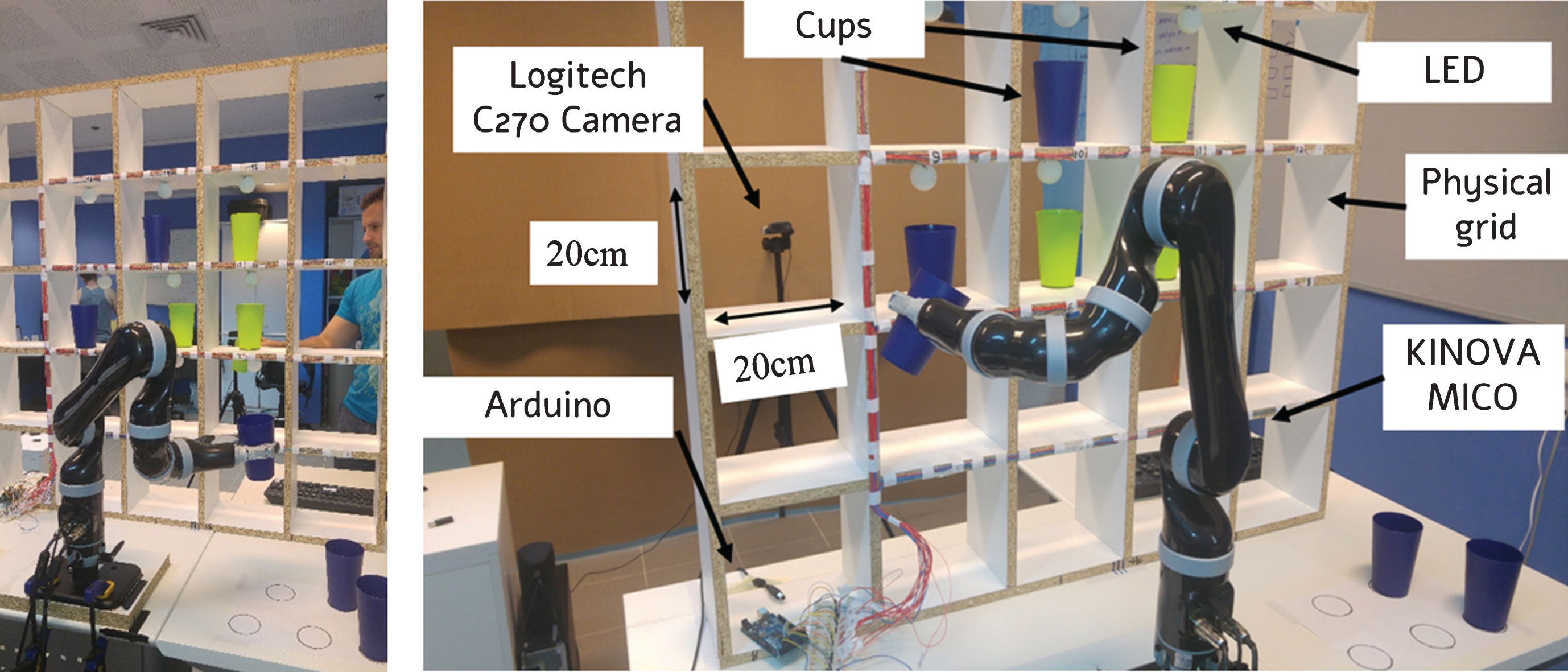
2.3.1Robotic manipulator and motion planning
A KINOVA MICO six-degrees-of-freedom arm was used to manipulate the physical cups. All possible motions were pre-programmed by dividing the task into separate “pick” and “place” actions. The “pick” actions included five possible positions for each cup on the table next to the robotic arm (corresponding to the locations of the five blue cups, placed next to the robotic arm at the start of each game), and the “place” actions included nine possible positions on the physical grid (corresponding to the nine possible locations for placing a cup on the 3×3 grid). The physical grid was a 5×5 grid, and within it was the “active play zone” (3×3), where cups could be placed by the participants (and by the robot, in the embodied configuration). The grid was designed with 25 cells with an intention to escalate the difficulty level of the game in future settings by requiring an extended reach of the patient. The active play zone on the grid was 60×60 cm in size, while the KINOVA arm reach is 55 cm. Thus, the KINOVA manipulator was positioned in the near vicinity of the grid to enable a large variety of pick and place options on the physical grid.
The robotic motion is inherently limited in speed due to safety concerns. In order to minimize the time it took the robotic arm to perform the pick-and-place action, the robot was programmed to pick the first cup before the game started, and each following cup at the end of its turn to play. That is, the robotic arm waited in front of the grid while holding the next cup during the participant’s turn, instead of waiting for the participant to complete his or her turn first before picking up the next cup.
2.3.2Non-embodied system
The non-robotic interactive system was implemented with an Arduino Mega 2560 programmed to control 16 light emitting diodes (LEDs) (Fig. 1). The Arduino was chosen due to its classical fit to a gaming system that requires generating a dynamic response to the user in a short time. Each LED is placed on the grid and connected to the Arduino Mega board. In the nine locations corresponding to the active play zone, the LEDs were covered with ping-pong balls to intensify the visual effect of a LED turning on as a mark of the system’s location selection. The other seven LEDs, placed outside the 3×3 active play zone, were programmed to flash after the user made a choice when playing against the computer-controlled lighting system, to simulate a “thinking” process of the system.
2.4Procedure
Each participant had two sessions consisting of five Tic-Tac-Toe games each: one session played against the robotic system and one session against the Arduino system. In order to avoid bias towards one of the systems, half of the participants started the games against the Arduino system and the other half started against the robotic system. In each game, participants performed between three and five pick-and-place movements, using their right arm, before the game ended (a game ended when one of the opponents won, or tie was reached); The purpose of each player in the Tic-Tac-Toe game was to create a sequence of three cups (or lights, in the case of the computer-controlled system) in three consecutive cells – aligned horizontally, vertically, or along a diagonal. Once the game began, the interactive system was fully autonomous, and not directly controlled by the experimenter. In order to mimic human-like, error-prone behavior, it was designed to choose non-optimal solutions. Specifically, at the start of each session (with the robot or with the computer), the system selected non-optimal initial positions that lowered its chances of winning the game. Once the user won one game, the system gradually improved its selections in order to challenge the user and to avoid boredom. At the end of each game auditory feedback was provided. When the person won, a cheerful sound was played, when the person lost, the sound played was somber, and when a tie was reached, it was neutral.
Ethical approval for this study was obtained from the Ethics Committee (institutional review board) of Ben-Gurion University of the Negev. All experimental procedures were performed in accordance with this ethical approval.
2.5Performance measures
2.5.1Objective measures
The following motion analyses were performed on data from the reconstructed skeleton of the participants derived from the Kinect 2.0 camera: movement time, path length, average hand speed, mean acceleration, mean jerk, and the maximal angle between the upper right arm and the torso. These outcome measures were chosen, as these are important factors to track during neurological rehabilitation (Chen et al., 2017; Laczko et al., 2017; Levy-Tzedek et al., 2007).
Path length was calculated as the integral of the entire hand path from initiation to termination of each trial (Levy-Tzedek et al., 2007; Levy-Tzedek et al., 2012). The vectors that represent the upper right arm and the torso were defined using three points obtained from the Kinect exoskeleton (P1, P2, P3), by using equations 1 and 2 (see Fig. 2):
(1)
(2)
Fig.2
Arm-torso angle calculation. This is an illustration of the angle (θ) calculated between the upper right arm and the torso, using the Kinect 2.0 output skeleton.
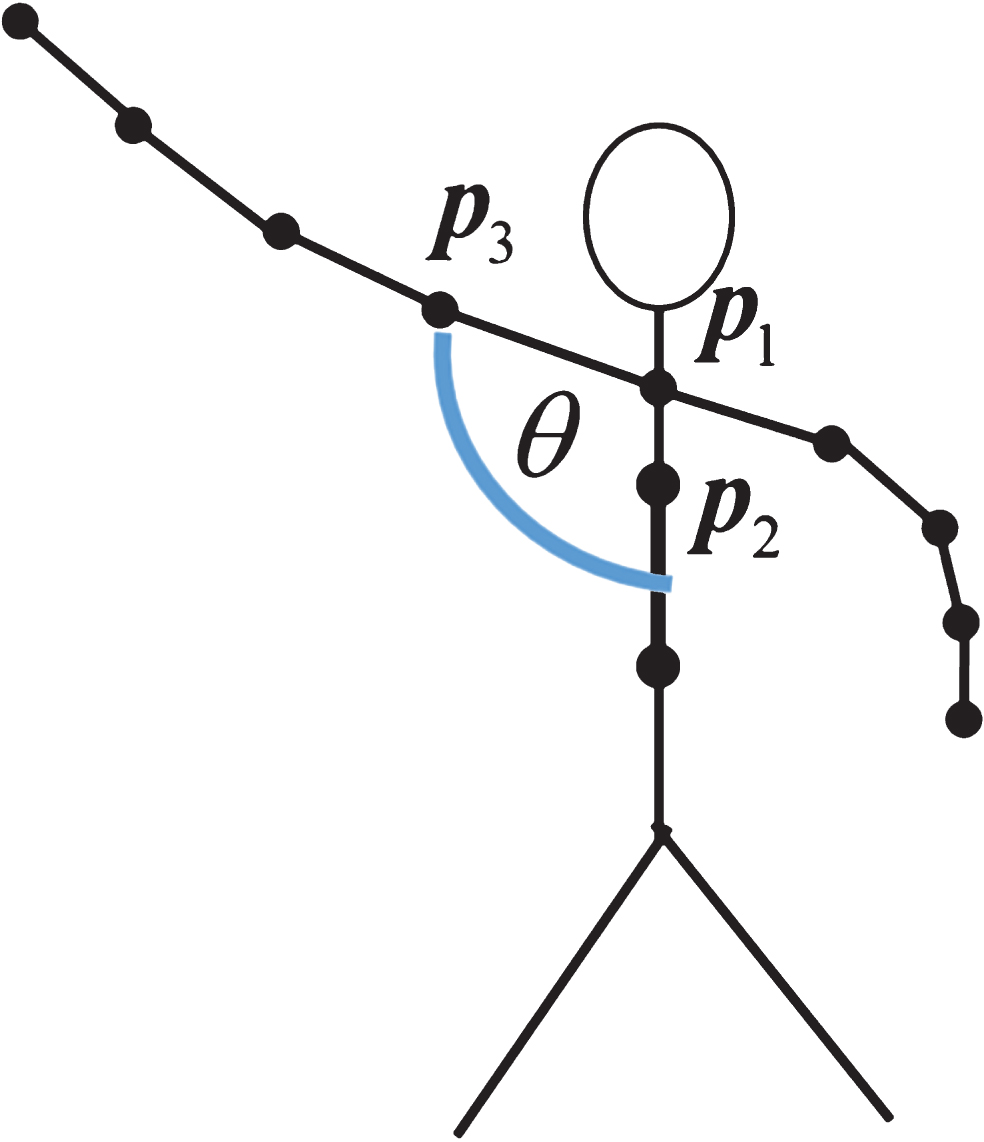
These locations on the skeleton were chosen since P1 and P2 define the vector along the central axis of the body, and P1 and P3 define the vector of the right arm. The angle between these two vectors is the angle of the right arm with respect to the torso. The size of this angle during movement is one of the parameters of interest during movement rehabilitation (Willmann et al., 2007).
2.5.2Subjective measures
The effects of the robotic arm’s physical entity on the participants’ feelings and motivation to continue playing was evaluated by administering: (1) the Godspeed questionnaire (Bartneck et al., 2009) after each of the two game sessions concluded, as well as (2) a custom-made questionnaire at the conclusion of the experiment (Table 1). Analysis of the Godspeed questionnaire was conducted according to the five basic categories defined in the Godspeed original questionnaire.
Table 1
Questionnaire aimed at determining participants’ motivation to continue interacting with each of the systems
Questions | Possible answers |
1.You are required to play two more games. Select your preferred opponent | □ Robot □ Arduino □ No preference |
2.You are required to play 10 more games. Select your preferred opponent | □ Robot □ Arduino □ No preference |
3.The two systems have different execution times. If the execution times were equal, would you change your prior selection? | □ Yes □ No □ I didn’t feel a difference |
4.Which system did you like more? | □ Robot □ Arduino □ I liked both the same |
5.If you could take one of the systems to your home, which one would you take? | □ Robot □ Arduino □ None |
2.6Statistical analysis
The effects of embodiment of the system (Arduino vs. Robot) and of age (young vs. older) on the objective outcome measures was tested using the two-way ANOVA statistical test. The analysis was performed on the log-transformation of the data, which was verified to be normally distributed using a Lilliefors test (Conover, 1999) and a Q-Q plot (Ghasemi & Zahediasl, 2012; Thode, 2002) using the MATLAB statistical toolbox (Mathworks, Natick, MA, v.8.5).
2.7System design
The system included three parallel processes denoted as the Intelligent Process, the Interactive Process, and the Recording Process (Fig. 3). The Intelligent Process is responsible for game management. It schedules the turns between the players (human or system) and performs the decision making for the Interactive Process. The Interactive Process serves as an I/O (input/output) module which responds to commands given by the Intelligent Process and outputs a corresponding result, e.g. moving a cup by the robotic arm, or turning on an Arduino-controlled light at the selected position on the grid. The Recording Process controls the Kinect 2.0 camera and runs in parallel during the entire game.
Fig.3
System-design flow chart.
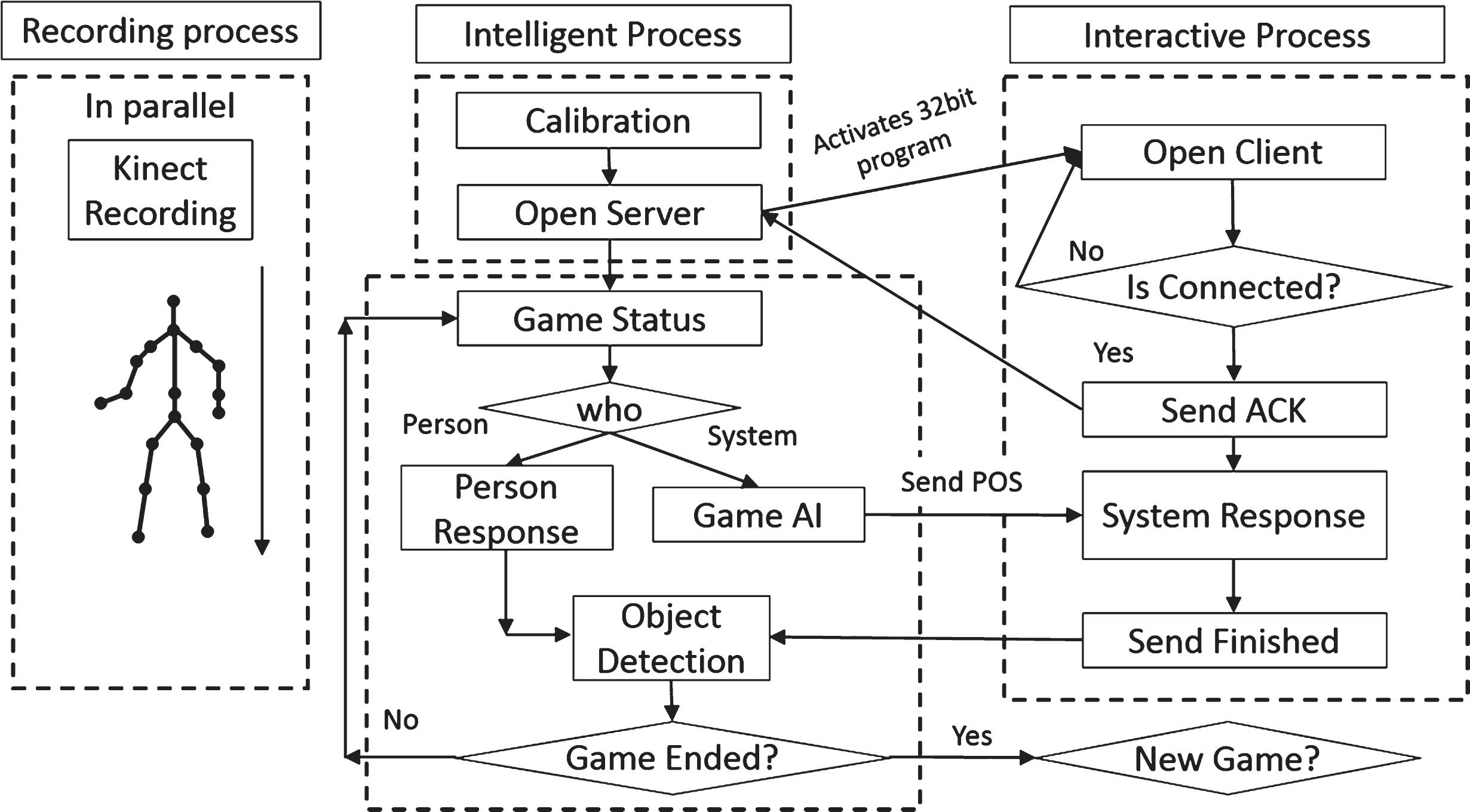
The Intelligent Process flow is as follows: first, the process is semi-manually calibrated (the camera is positioned in its home position) to ensure successful detection of the environment. It then opens a TCP Client-Server communication channel with the Interactive Process. The Intelligent Process then initializes the game parameters (i.e., the current game score, whose turn it is to play). When the person is playing, the system waits for a cue that the person completed his or her turn (by pressing the ‘enter’ key on a computer keyboard) and then activates the object detection scheme. When it is the system’s turn to make a move, the system chooses the response position for the Interactive Process and sends it for execution. Once the Interactive Process completes the task of responding (picking and placing a cup or turning on a light) on the grid, it sends an acknowledgment to the Intelligent Process, to activate the object detection scheme.
The object detection module (Section 2.2.1) detects the position of the cups placed by the user and determines the game status. The system repeats those actions until one of the players wins the game or there is a tie.
2.8Object detection algorithm
An online image processing algorithm was developed to detect the cup location based on the 3D natural index difference (NDI). An NDI algorithm was chosen because it helps to eliminate illuminations, reflections and shadows (Perez et al., 2000; Vitzrabin & Edan, 2016). The algorithm was implemented using OpenCV 3.0, a Logitech C270 RGB camera and on Intel I7, 2.0 GHz laptop computer with 8GB memory. The algorithm transfers the RGB image captured by the camera to the NDI space (Fig. 4B) using eq. (3). where NDI1,2,3 refers to the NDI generated channels and R, G, and B are the red, green and blue channels of the original image.
Fig.4
Image processing flow. Shown here is an example of the algorithm processing a scenario of three green cups while neglecting blue cups. A – RGB image. B – Image in NDI space. C – Image after morphological operations.
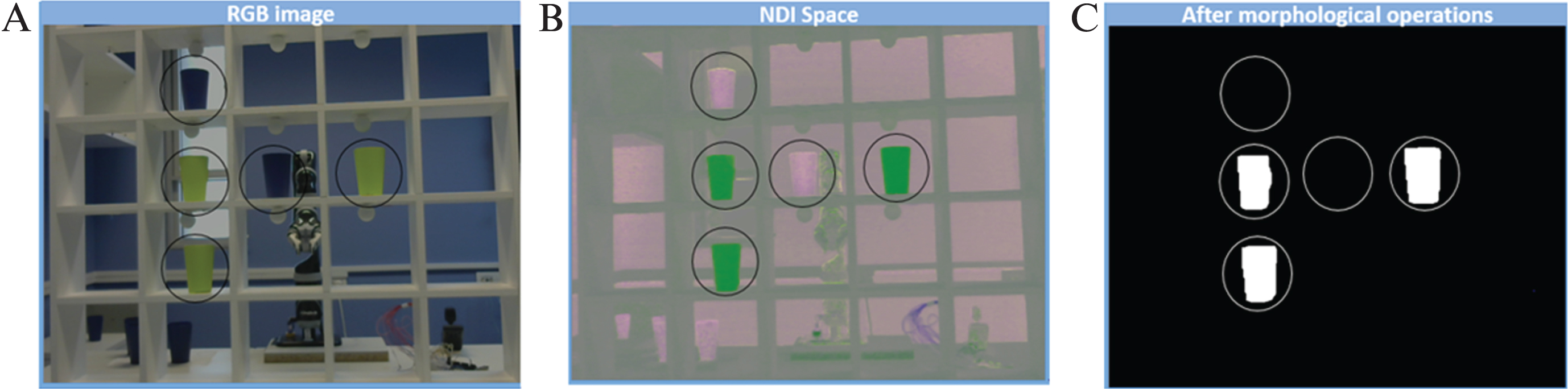
(3)
The objects are segmented from the background using a threshold determined by the user and followed by morphological operations to eliminate noise (see Fig. 4B). The entire process and detection algorithm is detailed in the Appendix.
3Results
Participants won in 38% of all games while playing against the robot and in 35% while playing against the non-embodied Arduino system. The rest of the games were split between a tie (37% and 39% respectively) and a system win (25% and 26% respectively). These results suggest that both systems presented a similar challenge for the participants.
3.1Motion analysis
Data collected from the 62 participants included 2395 pick-and-place trials for playing against the Arduino system and 2383 trials against the robotic system. The number of trials differs between the systems because each game required between five and nine turns until one of the opponents (system or participant) won, or until a tie was reached. Each trial was analyzed separately. 121 (5%) trials of playing against the Arduino and 136 (6%) trials of playing against the robot were not recorded due to connectivity failures with the Kinect and therefore these were not included in the analysis. These connectivity failures occurred during games played by four of the participants. The recorded data were analyzed, and the following outcome measures were calculated: the total path length traversed by the participant’s hand in each trial, the time it took to complete each trial, the maximal angle reached during the trial between the torso and the right arm, the average speed of the participant’s movement, the average acceleration and average jerk of the movement. Table 2 shows the mean and the standard deviation for each outcome measure, as well as the p-values for the comparisons between the robot and the Arduino, the comparison between the two age groups, and the interaction between these factors.
Table 2
Results of the statistical analysis for: path length, maximal arm-torso angle, average velocity, average acceleration and average jerk during each trial. Significant differences for these metrics between the Arduino and the Robot systems or between the young and the older age groups are marked in bold
Path length (m) | Time (min) | Max angle (degree) | Mean Speed [m/sec] | Mean Acceleration [m/sec2] | Mean Jerk [m/sec3] | |
Arduino vs. Robot p-value | 0.603 | 0.006 | 0.878 | 0.001 | 0.881 | 0.089 |
Young vs. Older p-value | 0.24 | 0.76 | 0.21 | 0.001 | 0.28 | 0.97 |
Interaction p-value | 0.60 | 0.12 | 0.79 | 0.16 | 0.69 | 0.98 |
Lilliefors test p-value | 0.127 | 0.269 | 0.329 | 0.493 | 0.407 | 0.105 |
Mean | 1.73 | 4.3 | 77.2 | 0.25 | 1.15 | 10.9 |
Standard deviation | 0.35 | 1.4 | 9.88 | 0.04 | 0.29 | 4.70 |
Results from the Q-Q plots (Fig. 5) showed that the values of all the outcome measures were drawn from a normal distribution. Results from the Lilliefors test for normality support the normality assumption for each of the outcome measures (see the 4th row in Table 2 – a p-value greater than 0.05 supports the normality assumption, needed in order to use the ANOVA test).
Fig.5
Normality testing. Shown here is a QQ-Plot for normality testing of the path length (left) and speed (right) metrics.
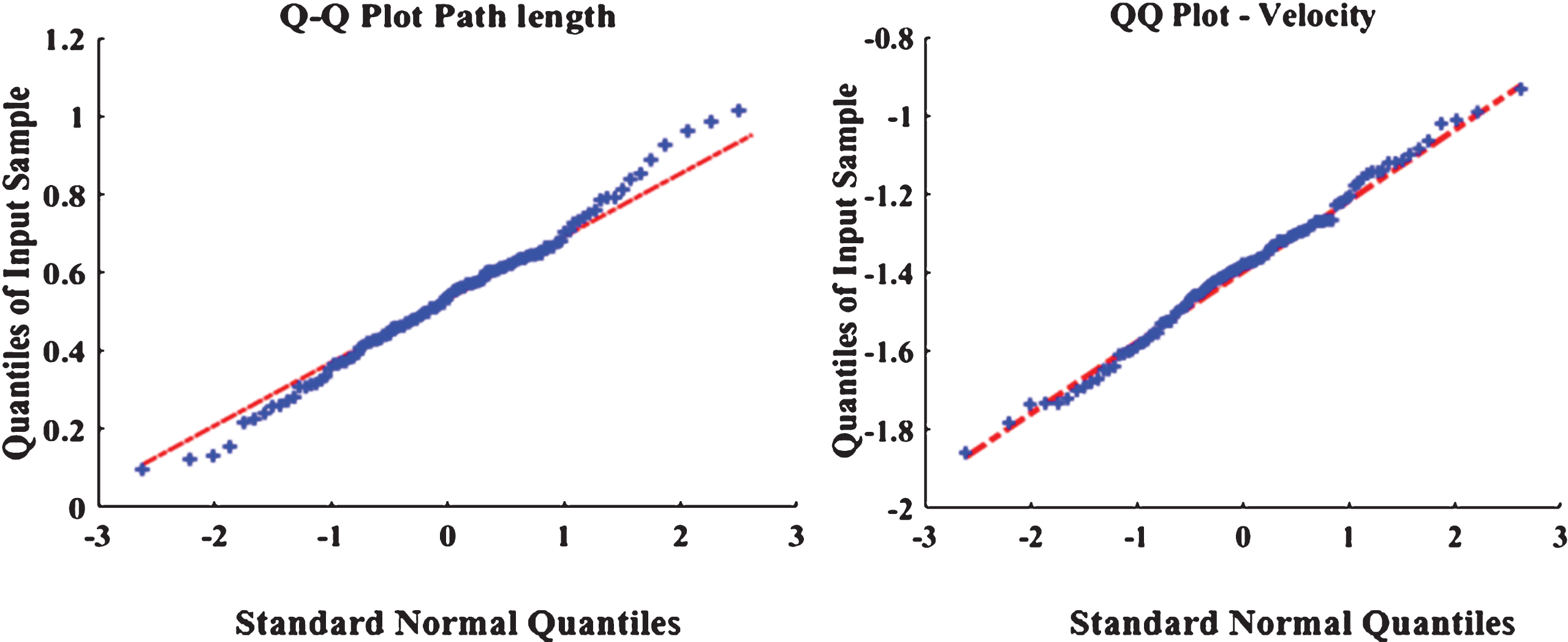
The results from the two-way ANOVA (Table 2) show a significant difference between the non-embodied Arduino-controlled system and the robotic system for the time it took to complete each game (F1,116 = 7.87, p = 0.006) and the average hand speed during the reaching movement (F1,116 = 24.49, p = 0.001). Interestingly, these results suggest that the speed of the participants’ movements was primed by the system they played with (robotic or non-robotic). That is, they moved at a speed that was faster or slower, depending on the response speed of the robot or the non-embodied system. The only statistical difference between the young and the older groups was found in the average speed of their hand movement (F1,116 = 10.54, p = 0.001) (Table 2), where the young participants were 15% faster than the older participants, see Fig. 6.
Fig.6
Average hand speed. Shown here per age group (left) and per system (right). Error bars denote standard error. An asterisk denotes a significant difference between the two groups or systems (p = 0.001).
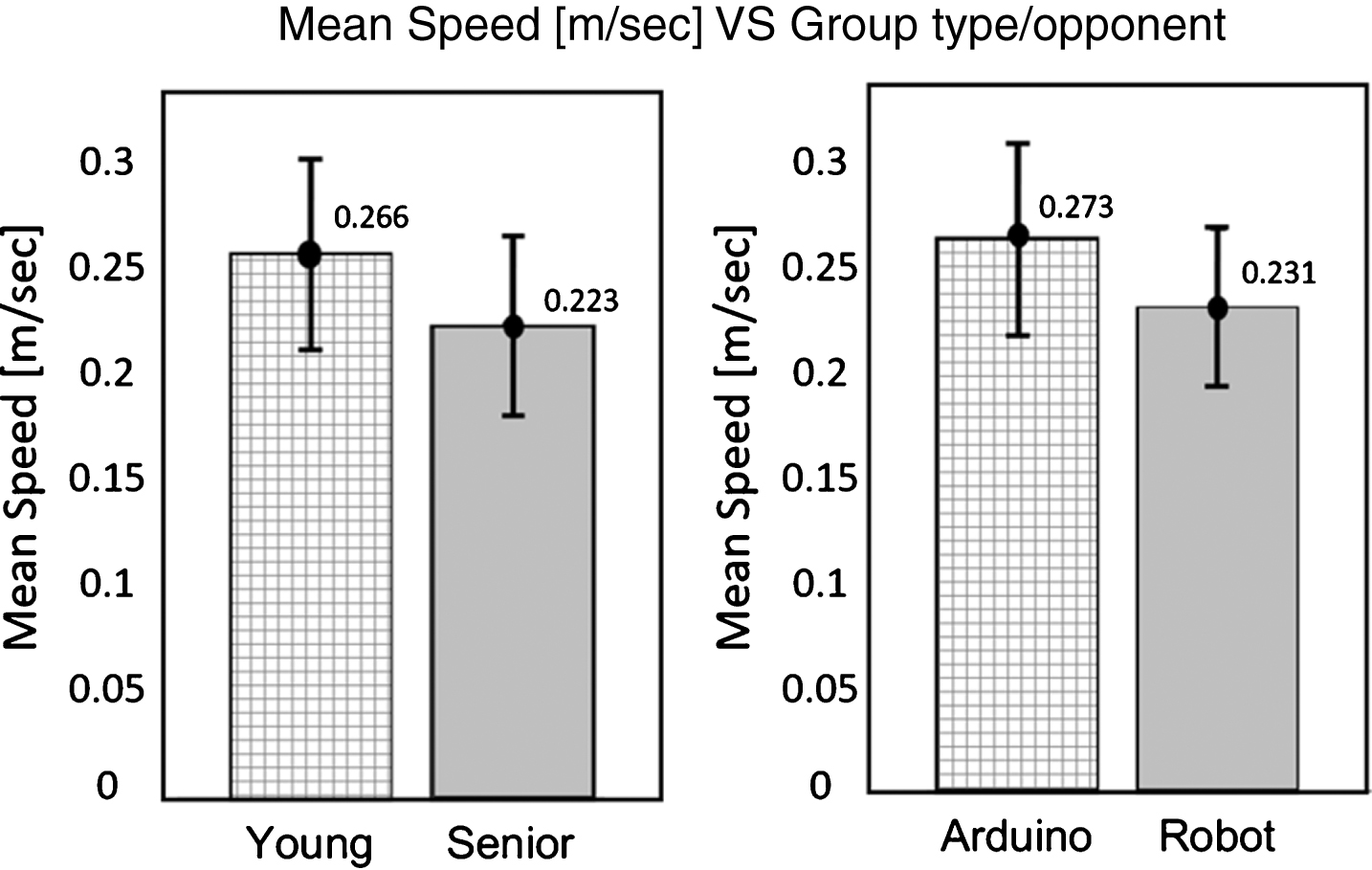
3.2Gaming strategies
Analysis of the frequency of approaching each cell (Fig. 7) revealed that the participants tended to select cells based on a combination of two factors: the cell’s physical proximity to the location where they stood, and its strategic value in winning the game. Specifically, the number of repetitions of cup placing per cell on the physical grid shows that cell number five was infrequently approached (Fig. 7). This cell was at the farthest column on the grid from the location where the participants were instructed to stand (to the left of the grid), and did not have a strategic value for winning the game. A potential explanation for this finding is that the distance and associated energy costs of reaching for a cell that does not offer strategic benefit, caused participants to choose closer cells or ones that have more strategic value (i.e., corner cells). This result is interesting as it implies that game design should include consideration of the natural tendencies of participants. This could be used to direct the participants to specific otherwise less-reached cells, which would result in more extended arm movements.
Fig.7
Number of cell selections by participants.
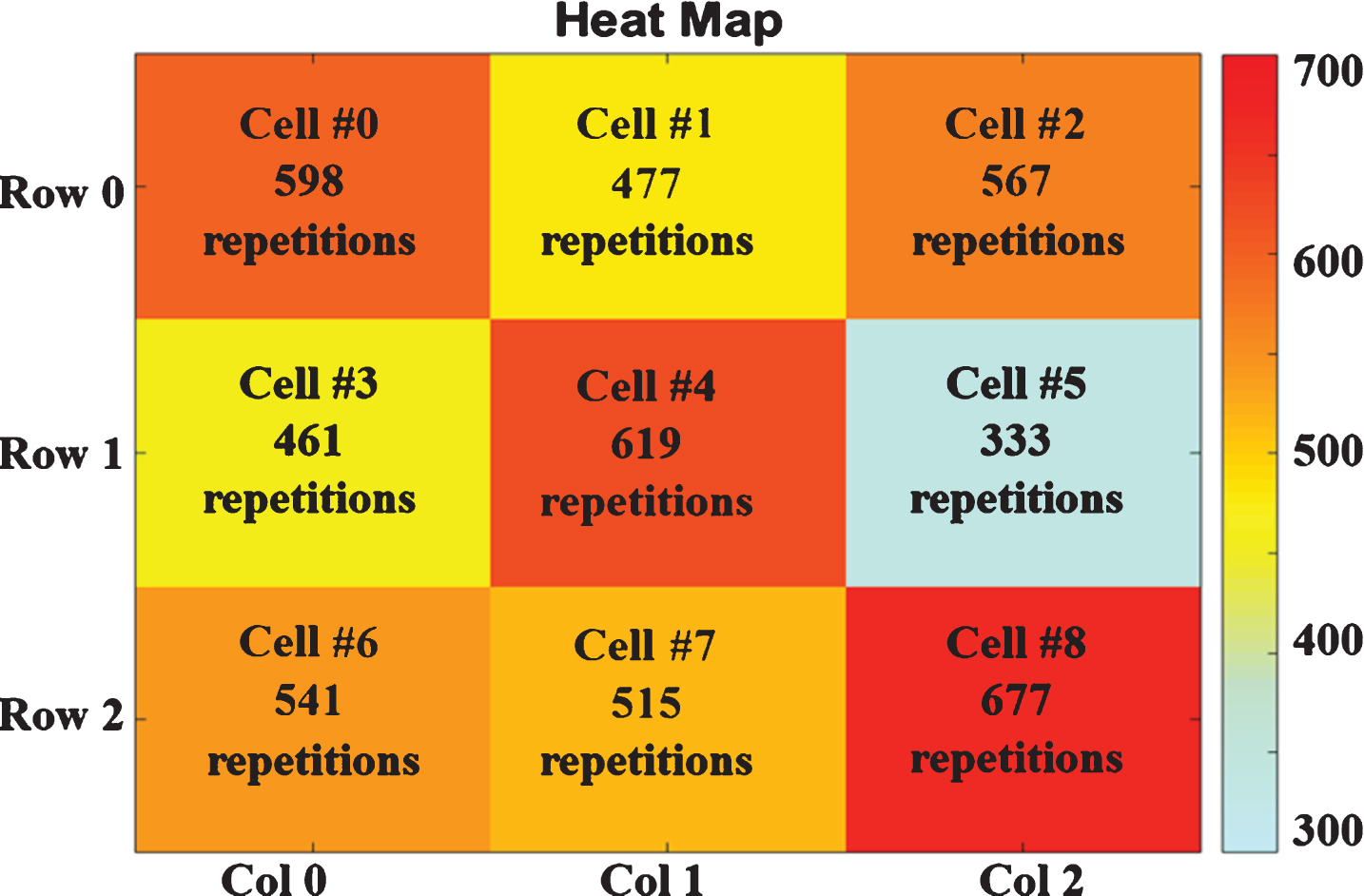
3.3Subjective measures
Both age groups demonstrated a preference for playing two more games with the robotic arm, compared with the non-embodied system. In the young group, 60% indicated a clear preference for playing with the robotic system, compared to 41% in the older group (59% of this group did not have a clear preference, see Fig. 8). When participants (both young and older) were asked to play 10 more games, their preference towards the robotic system decreased. 55% of the young participants and 13% of the older preferred the Arduino system (question 2, Fig. 8) as opposed to 33% and 0% that preferred the Arduino in the case of playing only two more games (question 2, Fig. 8). This is most likely due to the fact that the robotic system was slower in its reaction time as the robotic arm had to actually complete a movement compared to the Arduino system, which acted faster (all that was required to mark a choice by the system was to turn on an LED, which is instantaneous). Similar results were obtained for the rest of the questions, where, in general, the robotic system was preferable for both the young and senior age groups (questions 3,4 and 5, Fig. 8).
Fig.8
Participant preferences. These were measured at the end of the experiment.
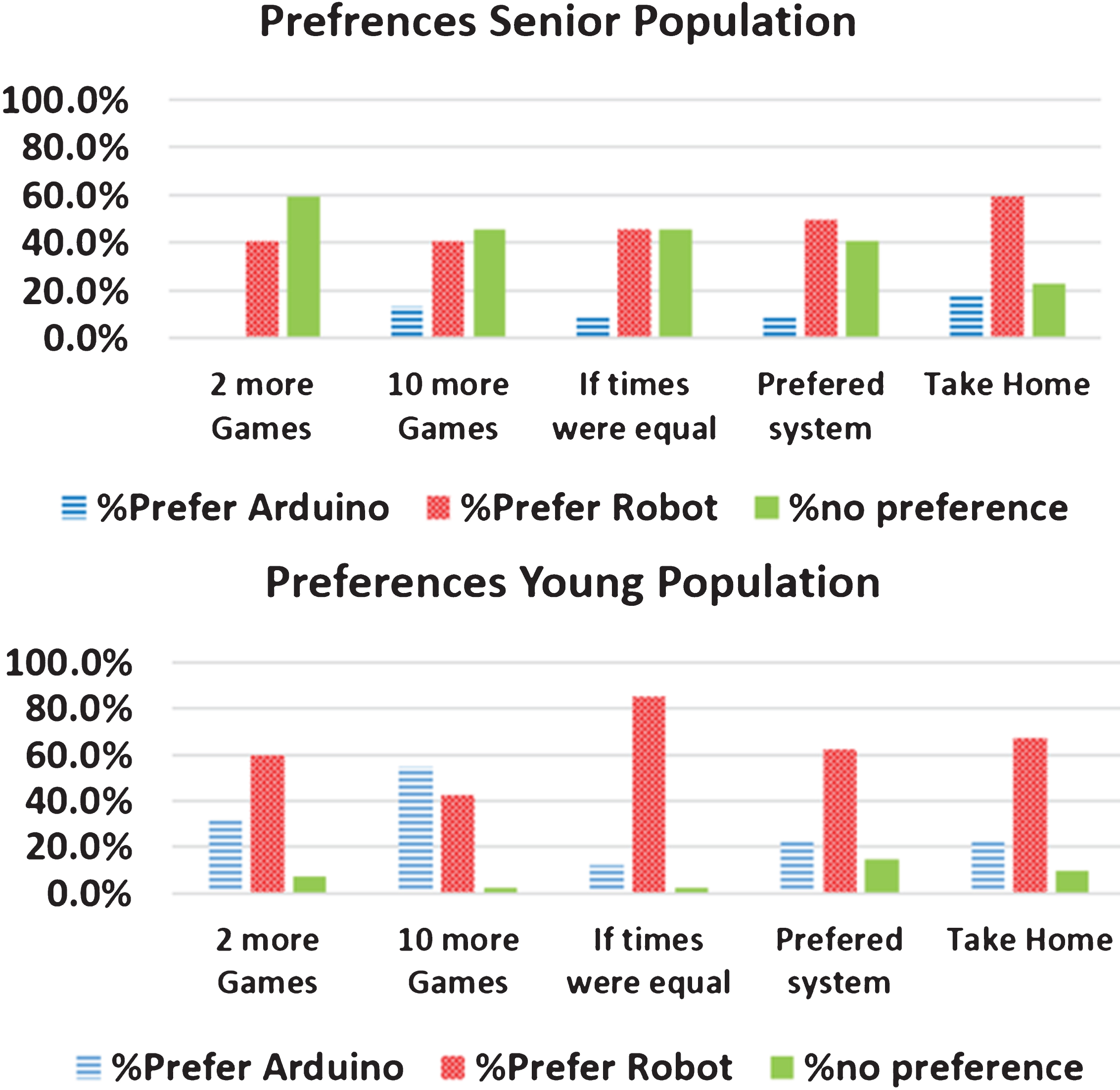
When asked to explain their preferences, the majority (10 out of 11) of the senior participants stated that they did not perceive a difference between the systems. Senior participants who preferred the robotic system claimed that it felt more human-like (in 10 out of 11 cases). The young participants that preferred the robotic system explained that it was more interesting, fun and appealing (17 out of 25), the rest reported it was more human-like (8 out of 25). Young participants that preferred the Arduino stated that it was due to timing issues in 7 out of 13 cases, three of them felt it was more sophisticated and the remaining three did not specify a reason. When the young participants were asked, “would they change their preference if there were no timing differences between the systems,” eight of the 13 who preferred the Arduino said they would prefer the robot. Thirty four out of 40 young participants (85%) noted they would prefer the robotic system if there were no timing differences.
The results of the Godspeed questionnaire (Table 3) are consistent with the outcomes from the custom-built questionnaire, and were collected as subjective scalar grades from one to five. On average, participants gave a higher grade to the robotic system compared with the Arduino system in terms of anthropomorphism (3.4 vs. 3.0, respectively), likeability (3.61 vs. 3.31) and animacy (3.14 vs. 2.71) (Table 3). The average score in all three categories being close to 3 (out of 5), suggests that there is potential for developing more engaging robotic interactions (e.g., by personalization of the system, or by adding human-like features to the robotic entity).
Table 3
Results for the Godspeed questionnaire, summarized by categories. Scale was from one to five, where five was the highest
Arduino | Robot | |||
Average | Standard deviation | Average | Standard deviation | |
Anthropomorphism | 3.02 | 2.04 | 3.40 | 1.14 |
Animacy | 2.79 | 1.39 | 3.14 | 1.27 |
Likeability | 3.31 | 1.22 | 3.61 | 1.09 |
Perceived intelligence | 3.74 | 1.09 | 3.81 | 1.05 |
Perceived safety | 3.79 | 1.31 | 3.83 | 1.31 |
Perceived safety without Quiescent – Surprised question | 4.29 | 0.86 | 4.35 | 0.82 |
A stronger preference for the robotic system was revealed in their responses to the Godspeed questionnaire. For example, when participants were asked, “Is the system more stagnant or lively”, the participants gave a 3.4 average score (std = 1.0) for the robot vs. a 2.6 average score (std = 1.3) for the Arduino. Another important outcome of the Godspeed questionnaire is that the overall perceived safety of the systems was rather high (3.8 for both systems). It is worth noting that a large number of participants did not understand the question of “Quiescent – Surprised” (which is part of the questions in the perceived safety). When omitting this question, the average grade for perceived safety increases to 4.3 for the robot and 4.4 for the Arduino system.
4Discussion and conclusions
The three main goals of the current study were (1) to design and test a prototype system, to be ultimately used for the improvement of upper limb rehabilitation processes; (2) to evaluate whether people of different ages respond to and interact with such a robotic gaming system differently; and (3) to identify whether the robot’s physical entity is an important aspect in motivating users to complete a set of repetitive tasks.
An interactive physical gaming prototype system for the improvement of upper limb rehabilitation that encourages repetitive practice of 3D functional tasks was designed and the feasibility of its implementation was tested with young and older healthy adults. The findings suggest that the system could motivate participants to complete repetitive exercises that simulate the motion required in daily tasks (such as placing a cup on a shelf). Further studies with stroke patients should assert whether this gamified approach to encourage the performance of a rote repetitive task indeed enhances therapeutic value during the rehabilitation period. The system also provided important outcome measures regarding the motion quality, specifically the reaching distance, arm extent and the arm-torso angle with this setup. Older participants were found to move at a significantly lower movement speed compared with the younger adults. Both groups preferred to interact with the embodied robotic system in the short term, but their preferences were modified depending on the extent of time they were asked to continue playing with the system. The clear preference for the robotic system in the short term (when asked to play two more games), demonstrated by the young group, dropped, and the older group chose the non-embodied system more frequently when asked to play 10 more games.
Another key finding revealed differences between how the senior population and the young population related to the response time of the system. Some of the participants in the young group expressed impatience with the time it took the robot to make its moves, while the participants in the senior group, who themselves often perform slower movements (Levy-Tzedek et al., 2016), did not express dissatisfaction with the slower reaction time of the robotic system, compared to the computer-controlled one. This finding suggests that system personalization should also take into account the user’s movement response time. For example, patients who suffered an injury, which might limit their response time, may prefer a robotic system with slow response time, while patients who are able to respond faster may prefer a faster-moving robotic system.
It cannot be ruled out that the preferences of the two age groups were affected by their internal representations evoked by the terms “robot” and “Arduino” – the former is a familiar concept to most people, whereas the latter is a platform that is likely more familiar to the younger group.
This system is the first, to the best of our knowledge, which incorporates a robotic device as a partner in a game designed for rehabilitation, with the goal of performing a real-life 3D functional task. Now that we have demonstrated the feasibility of the system, and its acceptance by a group of older people, a future study should directly examine the effects of this proposed intervention on stroke patients.
An important feature of the proposed system is the ability to track the performance of the patients, in terms of success rates, as well as in terms of exact movement patterns. In future elaborations of the setup, this information can be used to monitor patients’ performance in real time, and adjust the game parameters (e.g., timing, or locations selected by the robot) or the feedback that the users receive on the quality of their movements. Their performance over multiple sessions can be recorded and compared to detect changes over time, and adjust the exercise program accordingly.
The findings suggest that the embodiment of the system improves the participants’ motivation to continue the interaction. Both age groups preferred the robotic system over a computer-controlled lighting system despite its slower speed, in the short term. These results are in line with previous research that showed positive effects of using robotic embodiment to encourage repetitive task completion and learning (Leyzberg et al., 2014; Matarić et al., 2007; Tapus et al., 2008). By its very nature, the non-embodied system cannot physically move cups, and therefore it must indicate its choice of a cell on the grid in some other fashion (here we designed it to be a system of computer-controlled lights). This difference between the action of the participant (picking and placing a cup) and that of the system (turning on a light) may have affected the preference of the participants when asked to choose between the embodied and the non-embodied systems.
Having established a preference for the robotic entity, the robot’s appeal could likely be strengthened, from the mid-range scores the robotic system received in this study to higher scores in the future by adding more engaging features to the design. Bretan et al. (2015), found that user engagement can be increased using a robotic system that focuses on facial expressions and gaze. Thus, it should be examined in future studies whether a different robotic design (e.g., a more human-like interface) might improve the perception of the system by the users, and increase their motivation to practice repetitive tasks. Previous research showed that robots with display screens are perceived as smarter and with a better personality compared to robots without one (Broadbent et al., 2013).
Finally, we found that the speed of the system (embodied and non-embodied) primed the speed of the participants’ movements. This is in line with our previous findings of movement priming by a robotic arm (Kashi & Levy-Tzedek, 2018). It is an important design feature that should be taken into account when designing a human-robot interaction: the movement of the robot is likely to affect the movement of the user. This finding is likely related to the fact that humans tend to adapt to their partners’ movement pattern (Roy & Edan, 2017; Stoykov et al., 2017), and can be used to influence the timing of the human movement if and when needed (for example, to speed up the movements to increase the challenge or slow down movements to reduce user fatigue).
5Summary
In summary, we demonstrated the feasibility of the proposed system to be used as a tool to both encourage the performance of a repetitive 3D functional movement, and track users’ movement profile during the task. We found that the movement speed of the participants was primed by the speed of the system with which they interacted (robot vs. non-robot), which is important to consider when designing human-robot interaction systems. We also found differences between the age groups in the importance they ascribed to the movement timing of the robotic system. Both groups indicated a preference for the embodied system over a non-embodied one. The results of this study indicate the importance of tailoring human-robot interactions to the specific characteristics of the users, with age being an important factor.
Appendices
Appendix: Object detection (NDI)
The algorithm includes two stages, a six-step offline stage followed by a seven-step online stage, Fig. S1. The RGB image is first divided into its three channels. Each of the channels is then transformed to the NDI space using eq. 4. Prior to the conversion, each channel is converted to a representation of float 32bit to avoid overflow in the conversion to the NDI space. The transformation results in a number between –1 and 1, thus to complete this stage another transformation to the classical representation of 0–255 is conducted, eq. 5. Where Ch1,2,3 refers to the NDI generated channels and R, G, B are the red, green and blue channels of the original image.
(A1)
(A2)
The third sub-stage is where both the offline and online stages begin to differ. In the offline stage, a combination of three thresholds (one for each of the NDI channels) is searched. The best combination that will yield the best detection for a specific object must be selected, and thus for this matter a simple user interface was developed, Fig. S2. The user interface allows the user to manually adjust the selected thresholds while viewing in parallel their influence on the image. Once the thresholds are selected, morphological operations are performed to fill and combine close detected pixels, and to reduce noise in the detection. The noise reduction is conducted using erode and dilatation. The effects of the entire process are displayed to the user, which can store the selected thresholds.
The third sub-stage of the online detection uses the pre-calculated thresholds to segment the object from the rest of the image. It then continues with morphological operations, followed by detecting the object positions based on their contours. The filling operation prevents situations where the object might be sliced in the middle and thus will result in finding two contours for one object. Finally, the detected objects are converted from image pixels to physical grid positions using eq. 6. Where xGridPos and yGridPos are the physical cell positions on the grid, objX and objY are the centers of the detected contour and the normalization to frame width and height keeps the detection inside the physical limitations. The multiplication with 5 and 4, is done to fit the unsymmetrical image to the physical grid which includes a 5×5 cell grid. An illustration of the detection process and the results are shown in Fig. 4.
(A3)
Acknowledgments
The authors thank Yoav Raanan, Yedidya Silverman and Tamir Duvdevani for their assistance in running the experiments. The research was partially supported by the Ben-Gurion University of the Negev through the Helmsley Charitable Trust, the Agricultural, Biological and Cognitive Robotics Initiative, the Marcus Endowment Fund, and the Rabbi W. Gunther Plaut Chair in Manufacturing Engineering. The research was further supported by the Brandeis Leir Foundation, the Brandeis Bronfman Foundation, the Promobilia Foundation, the Borten Family Foundation grant, the Planning and Budgeting Committee I-CORE Program and The Israel Science Foundation (ISF) (1716/12) through the Learning in a Networked Society (LINKS) center and grants number (535/16 and 2166/16).
Supplementary material
[1] The supplementary material is available in the electronic version of this article: http://dx.doi.org/10.3233/RNN-170802.
References
1 | Aisen M.L. , Krebs H.I. , Hogan N. , McDowell F. , & Volpe B.T. ((1997) ) The effect of robot-assisted therapy and rehabilitative training on motor recovery following stroke. Archives of Neurology, 54: (4), 443–446. |
2 | Bainbridge W.A. , Hart J.W. , Kim E.S. , & Scassellati B. ((2011) ) The benefits of interactions with physically present robots over video-displayed agents. International Journal of Social Robotics, 3: (1), 41–52. |
3 | Bartneck C. , Kulić D. , Croft E. , & Zoghbi S. ((2009) ) Measurement instruments for the anthropomorphism, animacy, likeability, perceived intelligence, and perceived safety of robots. International Journal of Social Robotics, 1: (1), 71–81. |
4 | Bertani R. , Melegari C. , Maria C. , Bramanti A. , Bramanti P. , & Calabrò R.S. ((2017) ) Effects of robot-assisted upper limb rehabilitation in stroke patients: A systematic review with meta-analysis. Neurological Sciences, 1–9. |
5 | Blank A.A. , French J.A. , Pehlivan A.U. , & O’Malley M.K. ((2014) ) Current trends in robot-assisted upper-limb stroke rehabilitation: Promoting patient engagement in therapy. Current Physical Medicine and Rehabilitation Reports, 2: (3), 184–195. |
6 | Bretan M. , Hoffman G. , & Weinberg G. ((2015) ) Emotionally expressive dynamic physical behaviors in robots. International Journal of Human-Computer Studies, 78: , 1–16. |
7 | Broadbent E. , Kumar V. , Li X. , Sollers 3rd J. , Stafford R.Q. , & MacDonald B.A. et al. ((2013) ) Robots with display screens: A robot with a more humanlike face display is perceived to have more mind and a better personality. PLoS One, 8: (8), e72589. |
8 | Chen X. , Siebourg-Polster J. , Wolf D. , Czech C. , Bonati U. , & Fischer D. et al. ((2017) ) Feasibility of using microsoft kinect to assess upper limb movement in type III spinal muscular atrophy patients. PLoS One, 12: (1), e0170472. |
9 | Conover W. ((1999) ), Practical nonparametric statistics, 3rd edn wiley, New York, 250–257. |
10 | De Graaf M.M. , & Allouch S.B. ((2013) ) Exploring influencing variables for the acceptance of social robots. Robotics and Autonomous Systems, 61: (12), 1476–1486. |
11 | Duret C. , Hutin E. , Lehenaff L. , & Gracies J. ((2015) ) Do all sub acute stroke patients benefit from robot-assisted therapy? A retrospective study. Restorative Neurology and Neuroscience, 33: (1), 57–65. |
12 | Fasola J. , & Mataric M.J. ((2012) ) Using socially assistive human–robot interaction to motivate physical exercise for older adults. Proceedings of the IEEE, 100: (8), 2512–2526. |
13 | Feys P. , Coninx K. , Kerkhofs L. , De Weyer T. , Truyens V. , & Maris A. et al. ((2015) ) Robot-supported upper limb training in a virtual learning environment: A pilot randomized controlled trial in persons with MS. Journal of Neuroengineering and Rehabilitation, 12: (1), 1. |
14 | Gerling K. , Livingston I. , Nacke L. , & Mandryk R. ((2012) ) Full-body motion-based game interaction for older adults. Paper presented at the Proceedings of the SIGCHI Conference on Human Factors in Computing Systems, pp. 1873–1882. |
15 | Ghasemi A. , & Zahediasl S. ((2012) ) Normality tests for statistical analysis: A guide for non-statisticians. International Journal of Endocrinology and Metabolism, 10: (2), 486–489. |
16 | Harley L. , Robertson S. , Gandy M. , Harbert S. , & Britton D. ((2011) ) The design of an interactive stroke rehabilitation gaming system. Paper presented at the International Conference on Human-Computer Interaction, pp. 167–173. |
17 | Holden M. , Todorov E. , Callahan J. , & Bizzi E. ((1999) ) Virtual environment training improves motor performance in two patients with stroke: Case report. Journal of Neurologic Physical Therapy, 23: (2), 57–67. |
18 | Kahn L.E. , Lum P.S. , Rymer W.Z. , & Reinkensmeyer D.J. ((2006) ) Robot-assisted movement training for the stroke-impaired arm: Does it matter what the robot does? Journal of Rehabilitation Research and Development, 43: (5), 619. |
19 | Kashi S. , & Levy-Tzedek S. ((2018) ) Smooth leader or sharp follower? Playing the Mirror Game with a Robot. Restorative Neurology and Neuroscience, 36: , 147–159. |
20 | Krebs H.I. , Hogan N. , Aisen M.L. , & Volpe B.T. ((1998) ) Robot-aided neurorehabilitation. IEEE Transactions on Rehabilitation Engineering, 6: (1), 75–87. |
21 | Kwakkel G. , & Kollen B. ((2013) ) Predicting activities after stroke: What is clinically relevant? International Journal of Stroke, 8: (1), 25–32. |
22 | Laczko J. , Scheidt R.A. , Simo L.S. , & Piovesan D. ((2017) ) Inter-joint coordination deficits revealed in the decomposition of endpoint jerk during goal-directed arm movement after stroke. IEEE Transactions on Neural Systems and Rehabilitation Engineering, 25: (7), 798–810. |
23 | Levin M.F. , Weiss P.L. , & Keshner E.A. ((2015) ) Emergence of virtual reality as a tool for upper limb rehabilitation: Incorporation of motor control and motor learning principles. Physical Therapy, 95: (3), 415–425. doi: 10.2522/ptj.20130579 [doi] |
24 | Levy-Tzedek S. , Krebs H.I. , Shils J. , Apetauerova D. , & Arle J. ((2007) ) Parkinson’s disease: A motor control study using a wrist robot. Advanced Robotics, 21: (10), 1201–1213. |
25 | Levy-Tzedek S. , Krebs H.I. , Song D. , Hogan N. , & Poizner H. ((2010) ) Non-monotonicity on a spatio-temporally defined cyclic task: Evidence of two movement types? Experimental Brain Research, 202: (4), 733–746. |
26 | Levy-Tzedek S. , Maidenbaum S. , Amedi A. , & Lackner J. ((2016) ) Aging and sensory substitution in a virtual navigation task. PLoS One, 11: (3), e0151593. |
27 | Levy-Tzedek S. ((2017) a). Motor errors lead to enhanced performance in older adults. Scientific Reports, 7. |
28 | Levy-Tzedek S. ((2017) b). Changes in Predictive Task Switching with Age and with Cognitive Load. Frontiers in Aging Neuroscience, 9: , 375. |
29 | Levy-Tzedek S. , Hanassy S. , Abboud S. , Maidenbaum S. , & Amedi A. ((2012) ) Fast, accurate reaching movements with a visual-to-auditory sensory substitution device. Restorative Neurology and Neuroscience, 30: (4), 313–323. |
30 | Leyzberg D. , Spaulding S. , & Scassellati B. ((2014) ) Personalizing robot tutors to individuals’ learning differences. Paper presented at the Proceedings of the 2014 ACM/IEEE International Conference on Human-Robot Interaction, pp. 423–430. |
31 | Lo H.S. , & Xie S.Q. ((2012) ) Exoskeleton robots for upper-limb rehabilitation: State of the art and future prospects. Medical Engineering & Physics, 34: (3), 261–268. |
32 | Luft A.R. , McCombe-Waller S. , Whitall J. , Forrester L.W. , Macko R. , & Sorkin J.D. et al. ((2004) ) Repetitive bilateral arm training and motor cortex activation in chronic stroke: A randomized controlled trial. Jama, 292: (15), 1853–1861. |
33 | Lum P.S. , Burgar C.G. , Kenney D.E. , Van der Loos H.F. , Machiel ((1999) ) Quantification of force abnormalities during passive and active-assisted upper-limb reaching movements in post-stroke hemiparesis. IEEE Transactions on Biomedical Engineering, 46: (6), 652–662. |
34 | Maciejasz P. , Eschweiler J. , Gerlach-Hahn K. , Jansen-Troy A. , & Leonhardt S. ((2014) ) A survey on robotic devices for upper limb rehabilitation. Journal of Neuroengineering and Rehabilitation, 11: (1), 1. |
35 | Matarić M.J. , Eriksson J. , Feil-Seifer D.J. , & Winstein C.J. ((2007) ) Socially assistive robotics for post-stroke rehabilitation. Journal of NeuroEngineering and Rehabilitation, 4: (1), 1. |
36 | Nordin N. , Xie S.Q. , & Wünsche B. ((2014) ) Assessment of movement quality in robot-assisted upper limb rehabilitation after stroke: A review. Journal of Neuroengineering and Rehabilitation, 11: (1), 137. |
37 | Perez A. , Lopez F. , Benlloch J. , & Christensen S. ((2000) ) Colour and shape analysis techniques for weed detection in cereal fields. Computers and Electronics in Agriculture, 25: (3), 197–212. |
38 | Rand D. , Weiss P.L.T. , & Katz N. ((2009) ) Training multitasking in a virtual supermarket: A novel intervention after stroke. American Journal of Occupational Therapy, 63: (5), 535–542. |
39 | Roy S. , & Edan Y. ((2017) ) Givers & receivers perceive hantasks differently: Implications for human-robot collaborative system design. ArXiv Preprint arXiv:1708.06207. |
40 | Schoone M. , van Os P. , & Campagne A. ((2007) ) Robot-mediated active rehabilitation (ACRE) A user trial. Paper presented at the 2007 IEEE 10th International Conference on Rehabilitation Robotics, pp. 477–481. |
41 | Stoykov M.E. , Corcos D.M. , & Madhavan S. ((2017) ) Movement-based priming: Clinical applications and neural mechanisms. Journal of Motor Behavior, 49: (1), 88–97. |
42 | Tapus A. , Ţăpuş C. , & Matarić M.J. ((2008) ) User—robot personality matching and assistive robot behavior adaptation for post-stroke rehabilitation therapy. Intelligent Service Robotics, 1: (2), 169–183. |
43 | Thode H.C. ((2002) ) Testing for normality CRC press. |
44 | Veerbeek J.M. , Langbroek-Amersfoort A.C. , van Wegen E.E. , Meskers C.G. , & Kwakkel G. ((2017) ) Effects of robot-assisted therapy for the upper limb after stroke: A systematic review and meta-analysis. Neurorehabilitation and Neural Repair, 31: (2), 107–121. |
45 | Vitzrabin E. , & Edan Y. ((2016) ) Adaptive thresholding with fusion using a RGBD sensor for red sweet-pepper detection. Biosystems Engineering, 146: , 45–56. |
46 | Willmann R.D. , Lanfermann G. , Saini P. , Timmermans A. , te Vrugt J. , & Winter S. ((2007) ) Home stroke rehabilitation for the upper limbs. Paper presented at the Engineering in Medicine and Biology Society, 2007. EMBS 2007. 29th Annual International Conference of the IEEE. |