Effects of robotic upper limb treatment after stroke on cognitive patterns: A systematic review
Abstract
BACKGROUND:
Robotic therapy (RT) has been internationally recognized for the motor rehabilitation of the upper limb. Although it seems that RT can stimulate and promote neuroplasticity, the effectiveness of robotics in restoring cognitive deficits has been considered only in a few recent studies.
OBJECTIVE:
To verify whether, in the current state of the literature, cognitive measures are used as inclusion or exclusion criteria and/or outcomes measures in robotic upper limb rehabilitation in stroke patients.
METHODS:
The systematic review was conducted according to PRISMA guidelines. Studies eligible were identified through PubMed/MEDLINE and Web of Science from inception to March 2021.
RESULTS:
Eighty-one studies were considered in this systematic review. Seventy-three studies have at least a cognitive inclusion or exclusion criteria, while only seven studies assessed cognitive outcomes.
CONCLUSION:
Despite the high presence of cognitive instruments used for inclusion/exclusion criteria their heterogeneity did not allow the identification of a guideline for the evaluation of patients in different stroke stages. Therefore, although the heterogeneity and the low percentage of studies that included cognitive outcomes, seemed that the latter were positively influenced by RT in post-stroke rehabilitation. Future larger RCTs are needed to outline which cognitive scales are most suitable and their cut-off, as well as what cognitive outcome measures to use in the various stages of post-stroke rehabilitation.
1Introduction
According to the World Health Organization (WHO), cerebral stroke is “rapidly developing clinical signs of focal (or global) disturbance of cerebral function, with symptoms lasting 24 hours or longer or leading to death, with no apparent cause other than of vascular origin” and it is considered the second leading cause of death, the third leading cause of disability worldwide and the first leading cause of disability in the elderly.
Although the prevalence of cerebral stroke almost doubled from 1990 to 2010, from 2.7% to 4.9% for ischemic stroke and from 1.0% to 1.9% for haemorrhagic stroke, overall mortality in the same period decreased by 20% in ischemic stroke and by 25% in haemorrhagic stroke (SPREAD, 2016).
This results in an increase in the population with disability-related strokes in recent years –13.9 million stroke survivors in 1990 vs 25.7 million stroke survivors in 2013 (Feigin et al., 2015), which experiences limitations in ADL and mobility (Kwakkel and Kollen, 2013).
Among the various body districts, upper limb is considered one of the most affected by the cerebrovascular event: at hospital admission after stroke, more than two-thirds of people have arm paresis (and therefore have limited hand-arm function), resulting in reduced upper extremity function, which persists in half of the population affected by this disease to six months after stroke (Mehrholz et al., 2020), due to the complex functions it performs.
In fact, due to the execution of different and fine movement such as reaching, grasping, manipulation, arm transport, but also for its delicate task in perception the recovery of upper limb is complicated but fundamental simultaneously. As a result, the main goal of hand rehabilitation is to optimize dexterity in order to restore a sufficient grade of autonomy in ADL (Houwink et al., 2013).
International guidelines for the rehabilitation of the patient with cerebral stroke (Morone et al., 2021; SPREAD, 2016) reported that robotic therapy (RT) seems to be a safe and tolerable alternative for upper limb rehabilitation in patients with stroke, as it improves muscle strength, motor control and promote functional recovery of the limb. Indeed, RT seems to be a way to increase the amount and the intensity of the therapy, motivating patients to do more repetitions and prolong therapeutic time, as well as can be considered a way to standardize treatment (Gueye et al., 2021).
Besides motor impairment, cognitive decline is often present in stroke survivors: about 30% ischemic stroke survivors show a cognitive impairment which is determined by the MMSE score is lower than 27 (Sun et al., 2014). Several studies confirmed the high prevalence of cognitive impairment after stroke (Lamb et al., 2013; Nys et al., 2005; Pollock et al., 2014) and underlined its significant influence on motor learning strategies (Chen et al., 2013; Thon, 2015), functional recovery, and quality of life (Alt Murphy et al., 2017; Mullick et al., 2015); in fact, the recovery of cognitive impairments have a crucial importance for reintegration into everyday life (Blackburn et al., 2013).
By recent studies, it seems that robotic and technological devices bring stimulation and promoting neuroplasticity (Bressi et al., 2020) through their engaging design (Gueye et al., 2021).
In particular, Xing et al. (2020) highlighted that RT can also play an important role in cognitive recovery: these devices can be useful in encouraging an improvement in neuroplasticity, by stimulating alterations in connectivity in some areas (i.e. premotor cortex, cerebellum, M1 and supplementary motor area).
In fact, with the implementation of new graphical interfaces and more ecological scenarios, as well as more cognitively demanding tasks, robot can allow an active physical and cognitive engagement of patients during robotic therapy by adaptive assistance (Riener et al., 2006), promoting patient’s engagement (Marchal-Crespo et al., 2010), cognitive challenge (Metzger, Lambercy, Califfi, Conti, et al., 2014), automated task difficulty adaptation (Metzger, Lambercy, Califfi, Dinacci, et al., 2014) and visual and auditory feedback (Saposnik and Levin, 2011).
Despite its importance, the efficacy of robotics in restoring cognitive deficits was considered only in few recent studies (Adomavičienė et al., 2019; Aprile et al., 2021; Taravati et al., 2022), that also highlighted the importance of cognitive evaluation as the initial cognitive functions are positively associated with the functional outcome after robot-assisted therapy.
Moreover, cognitive impairment is not often considered as a clinical outcome or as a criterion for inclusion/exclusion of robotic interventions: a recent systematic review on 66 articles and 2214 participants highlighted that most trials that assessed the efficacy of upper limb assisted RT after stroke excluded individuals with cognitive impairments (76% of included studies) and that only a few trials (15% of included studies) measured cognitive outcomes (Everard et al., 2020).
This review confirmed the scarcity of information on the impact of robotic rehabilitation on the cognitive outcome in patients with stroke and the need to deeply analyse the relationship between cognitive recovery and rehabilitation.
Starting from these preliminary data, the aim of this review is to verify whether, in the current state of the literature, cognitive measures are used as inclusion or exclusion criteria and/or outcomes measures in robotic upper limb rehabilitation in stroke patients.
2Materials and methods
2.1Data sources and searches
PubMed and Web of Science were used to perform the literature search. The electronic search was conducted on March 2021. To avoid missing some key studies, cross-referencing was used from each publication obtained through the electronic search. The search strategies, combining relevant search terms with Boolean operators (OR/AND), are listed in Table 1, for the two databases, separately. The protocol was registered on PROSPERO (no CRD42021288946). The Preferred Reporting Items for Systematic Reviews and Meta-Analysis (PRISMA) statement was followed in the reporting.
Table 1
Search strategy
PubMed |
#1 “Stroke”[MeSH Terms] |
#2 “Cerebral hemorrhage”[MeSH Terms] |
#3 (Cerebral hemorrhage[Tiab]) OR (Cerebral hemorrhages[Tiab]) OR (Cerebral haemorrhage[Tiab]) OR (Cerebral haemorrhages[Tiab]) OR (Cerebral hemorrhagic[Tiab]) OR (Cerebrovascular accident[Tiab]) OR CVA OR (Cerebrovascular disease [Tiab]) |
#4 “Brain ischemia”[MeSH Terms] |
#5 (Brain ischemia [Tiab]) OR (Brain ischaemia [Tiab]) |
#6 Paresis [MeSH Terms] |
#7 Plegia [Mesh] |
#8 (hemiplegia [Tiab]) |
#9 #1 OR #2 OR #3 OR #4 OR #5 OR #6 OR #7 OR #8 |
#10 Robotics [Mesh] OR “Exoskeleton Device”[Mesh] |
#11 robot*[tiab] OR “robot assisted”[tiab] OR exoskelet*[tiab] OR “end effector*”[tiab] OR electromechani*[tiab] OR electro-mechani*[tiab] |
#12 #10 OR #11 |
#13 “Upper Extremity”[Mesh] OR “Shoulder”[Mesh] OR “Arm”[Mesh] OR “Elbow”[Mesh] OR “Forearm”[Mesh] OR “Wrist”[Mesh] OR “Hand”[Mesh] |
#14 “upper extremity”[tiab] OR “upper limb”[tiab] OR shoulder[tiab] OR arm[tiab] OR elbow[tiab] OR forearm[tiab] OR wrist[tiab] OR hand[tiab] OR finger*[tiab] |
#15 #13 OR #14 |
#16 “randomized controlled trial”[pt] |
#17 “controlled clinical trial”[pt] |
#18 randomized[tiab] |
#19 placebo[tiab] |
#20 “clinical trials as topic”[mesh: noexp] |
#21 “randomly”[tiab] |
#22 “trial”[ti] |
#23 #16 OR #17 OR #18 OR #19 OR #20 OR #21 OR #22 |
#24 animals [mh] NOT humans [mh] |
#25 #23 NOT #24 |
#26 #9 AND #12 AND #15 AND #25 |
Web of Science |
#1 TS=(stroke OR CVA OR “cerebrovascular disease” OR “cerebrovascular accident” OR hemiparesis OR hemiplegia OR paresis) OR TI=(stroke OR CVA OR “cerebrovascular disease” OR “cerebrovascular accident” OR hemiparesis OR hemiplegia OR paresis) OR AB=(stroke OR CVA OR “cerebrovascular disease” OR “cerebrovascular accident” OR hemiparesis OR hemiplegia OR paresis) |
#2 TS=(“upper limb” OR “upper extremity” OR arm OR forearm OR wrist OR finger OR hand) OR TI=(“upper limb” OR “upper extremity” OR arm OR forearm OR wrist OR finger OR hand) OR AB=(“upper limb” OR “upper extremity” OR arm OR forearm OR wrist OR finger OR hand) |
#3 TS=(random* OR randomized OR “randomized controlled trial” OR RCT) OR TI=(random* OR randomized OR “randomized controlled trial” OR RCT) OR AB=(random* OR randomized OR “randomized controlled trial” OR RCT) |
#4 TS=(robot* OR orthos* OR orthotic* OR automat* OR “computer aided” OR “Computer assisted” OR device* OR electromechanical OR electromechanical OR mechanical OR mechanised OR mechanized OR driven) OR TI=(robot* OR orthos* OR orthotic* OR automat* OR “computer aided” OR “Computer assisted” OR device* OR electromechanical OR electromechanical OR mechanical OR mechanised OR mechanized OR driven) OR AB=(robot* OR orthos* OR orthotic* OR automat* OR “computer aided” OR “Computer assisted” OR device* OR electromechanical OR electromechanical OR mechanical OR mechanised OR mechanized OR driven) |
#5 TS = Rehab* OR AB = rehab* OR TI = rehab* |
#6 #1 AND #2 AND #3 AND #4 AND #5 |
2.2Inclusion criteria
In agreement with the PRISMA guidelines (Page et al., 2021), the inclusion criteria were set following the PICOTS-SD (Brown, 2020) (participants, intervention, comparisons, outcomes, timing of outcome measurement, settings, study design) strategy, as reported below:
• Participants (P)=persons with stroke;
• Intervention (I)=upper limb robotic rehabilitation focused on the recovery of upper limb motor function;
• Comparisons (C)=conventional treatment, no treatment, or treatment with other robotic or technological devices or healthy participants;
• Outcome (O)=upper limb motor function measured by clinical scales or instrumental parameters;
• Timing of outcome measurement (T)=before and after the intervention;
• Settings (S)=inpatient or outpatient settings;
• Study design (SD)=randomized controlled trial (RCT).
We therefore included RCT an patients with stroke comparing an upper limb robotic rehabilitation with other interventions (such as conventional treatment, or other devices) focused on the recovery of upper limb motor function measured by clinical scales or instrumental parameters. RCTs with two or more arms were considered. If studies did not use robotic devices or were not focused on stroke patients, they were excluded from this review.
2.3Study selection and data extraction
Two independent reviewers evaluated the studies retrieved from the electronic search based on the titles and abstracts of the studies. After this preliminary screening process, the full text of all eligible studies was analyzed and independently evaluated to determine whether or not they met the inclusion criteria. A third reviewer was brought in to resolve any disagreements on the study’s eligibility between the two reviewers. The flow diagram of the article selection procedure is reported in Fig. 1. After inclusion, the study characteristics, research goals, and main findings were extracted and summarized. Specifically, the extracted information included: total number of patients randomized, mean time since the acute event of the enrolled patients (classified as lower or higher than 6 months), description and dose of the intervention in the experimental group, description, and dose of the intervention in the control group(s), primary and secondary outcomes. The PEDro scale (Cashin and McAuley, 2020) was used to assess the methodological quality of the studies.
Fig. 1
PRISMA 2020 flow diagram for new systematic reviews which included searches of databases, registers and other sources.
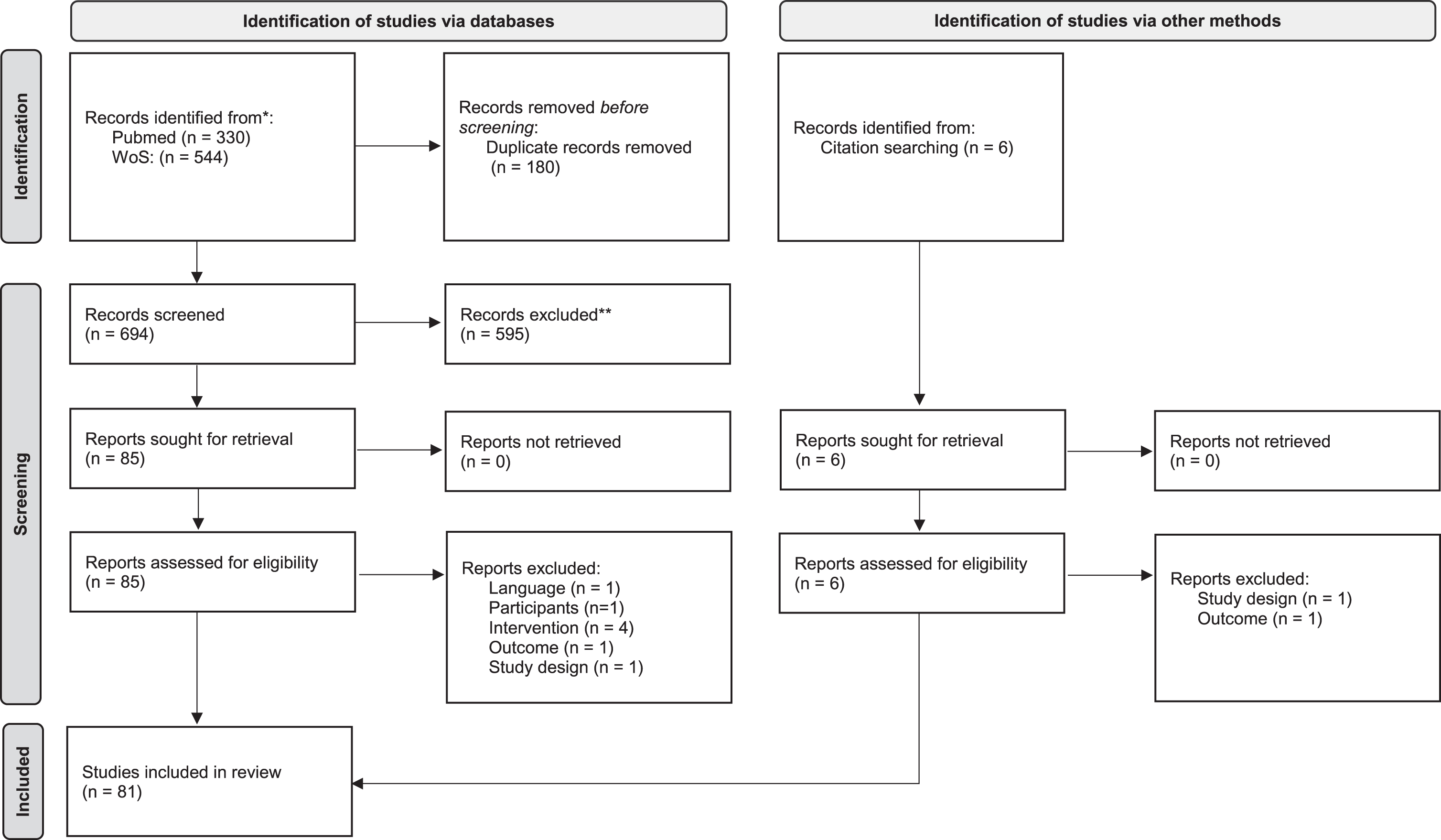
Moreover, in line with the goal of this review, the following data were considered and analyzed: presence of cognitive inclusion criteria (with description, when applicable), cognitive outcome measures (with description, when applicable), and any investigation of the relationship between motor and cognitive outcomes (with description, when applicable).
3Results
3.1Data synthesis
We found 880 records through the research method. After duplicates were removed, articles were screened for title and abstract, and 90 records were assessed for eligibility.
After full-text reading, 81 studies were included in the qualitative analysis of this systematic review. Figure 1 reports the flowchart of the research.
Supplementary Table 1 summarizes the characteristics of the included studies. According to the inclusion criteria, all reports are RCTs published between 2000 (Volpe et al., 2000) and 2021 (Ambrosini et al., 2021; Chinembiri et al., 2021; Doost et al., 2021; Gueye et al., 2021; H. C. Lee et al., 2021; Park, 2021).
3.2Population
The studies included a total population of 3922 stroke patients. The sample size varied from 12 (Brokaw et al., 2014; Daly et al., 2005; Iwamoto et al., 2019) to 770 (Rodgers et al., 2019a).
Time onset varied in according to studies’ inclusion criteria: twenty-nine studies included only patients whose time since the stroke event was under 6 months; thirty-three studies included only chronic stroke patients while the remaining studies included both phases. Time between stroke onset and start of treatment was specified in 67 studies and it ranged between 14 days (Gueye et al., 2021; Volpe et al., 2000) and 9 months (Park, 2021). Supplementary Table 1 reports population characteristics.
3.3Intervention
Robot upper limb trainingwas used in the intervention group of all included studies. Complete treatment characteristics are reported in Supplementary Table 1.
3.4Robotic treatment characteristics
All studies have at least one group that underwent RT. According to the treated district, in 18 studies (Abdullah et al., 2011; Chinembiri et al., 2021; Cho & Song, 2019; Fazekas et al., 2007; Han Yoo et al., 2012; Horsley et al., 2019; Iwamoto et al., 2019; Kahn et al., 2006; Klamroth-Marganska et al., 2014; M. Lee et al., 2018; Park et al., 2020; Perini et al., 2021; Qian et al., 2017; Rodgers et al., 2019b; Serrezuela et al., 2020; Takahashi et al., 2016; Timmermans et al., 2014; Tramontano et al., 2020) the entire upper limb was treated; shoulder was rehabilitated in five studies (Burgar et al., 2011; Cho and Song, 2021; Kim et al., 2019; Lum et al., 2002; Straudi et al., 2020); only Mazzoleni et al. (2019) considered wrist rehabilitation, while hand rehabilitation was considered in 13 studies (Ang et al., 2014; Calabrò et al., 2019; Grigoras et al., 2016; Y. Huang et al., 2020; Hwang et al., 2012; H. C. Lee et al., 2021; Orihuela-Espina et al., 2016; Park, 2021; Ranzani et al., 2020; Sale et al., 2014; Susanto et al., 2015; Vanoglio et al., 2017; Villafañe et al., 2018). None of the studies rehabilitated only elbow or forearm joints.
The remaining studies combined the treatment of two or more different districts: 20 (Ambrosini et al., 2021; Carpinella et al., 2020; Daly et al., 2005, 2019; Dehem et al., 2019; Doost et al., 2021; Gandolfi et al., 2019; K. W. Lee et al., 2016; Lee KW et al., 2017; S. H. Lee et al., 2020; Lo et al., 2010; Lum et al., 2002; Masiero et al., 2007, 2014; McCabe et al., 2015; Rabadi et al., 2008; Rosenthal et al., 2019; Tomić et al., 2017; Volpe et al., 2000; Xu et al., 2020) rehabilitated shoulder and elbow; robotic rehabilitation for forearm and wrist was considered in eleven studies (Hesse et al., 2005, 2014; Housman et al., 2009; Hsieh et al., 2011, 2016; Hsu et al., 2019; C. S. Hung, Hsieh, Wu, Chen, et al., 2019; C. S. Hung, Hsieh, Wu, Lin, et al., 2019; C. Shan Hung et al., 2019; Liao et al., 2012; Wu et al., 2012); wrist and hand was treated in two studies (Kutner et al., 2010; Wolf et al., 2015); Qian et al. (2019) used a robotic device for elbow, wrist and hand rehabilitation, while five studies (Daunoraviciene et al., 2018; Gueye et al., 2021; Jiang et al., 2021; Taveggia G et al., 2016; Zengin-Metli et al., 2018) underwent RT for shoulder, elbow and hand.
Conroy et al. (2019) combined two robots, one for shoulder and elbow and the other for wrist, as well as Edwards et al. ((2019); Aprile et al. (2020) used different robotic devices for the treatment of the upper limb, treating shoulder, elbow, wrist and hand in different phases; Conroy et al. (2011) rehabilitated, with robotic devices, shoulder and elbow in one group and forearm and wrist in another, while Brokaw et al. (2014) combined a robot for shoulder rehabilitation with one for hand rehabilitation.
Robotic characteristics (i.e., name, industry and country of production were specified in all studies except seven (Abdullah et al., 2011; Grigoras et al., 2016; Y. Huang et al., 2020; Qian et al., 2017, 2019; Serrezuela et al., 2020; Susanto et al., 2015) that used their own robotic prototypes to treat patients enrolled. Bi-Manu Track (Reha-Stim Co., Berlin, Germany) (Hesse et al., 2003) was the most used robotic device, followed by Armeo Spring (Hocoma AG, Volketswil, Switzerland) (Armeo®Spring –Hocoma, n.d.), Amadeo (Tyromotion, Graz) (Balasubramanian et al., 2010), Mit Manus (Interactive Motion Technologies, Cambridge, MA) (Aisen et al., 1997) –also described as InMotion2 (Interactive Motion Technologies, Inc., Cambridge, MA, USA) (Krebs et al., 1998).
Table 2
PEDro scale
Author, year of publication | Eligibility criteria* | Random allocation | Concealed allocation | Baseline comparability | Blind subjects | Blind therapists | Blind assessors | Adequate follow-up | Intention-to-treat analysis | Between-group comparisons | Point estimates and variability | Tot |
Abdullah HA, 2011 | 1 | 1 | 1 | 1 | 0 | 0 | 1 | 1 | 0 | 1 | 0 | 6/10 |
Ambrosini E, 2021 | 0 | 1 | 0 | 1 | 0 | 0 | 1 | 1 | 0 | 1 | 1 | 6/10 |
Ang KK, 2014 | 1 | 1 | 0 | 1 | 0 | 0 | 1 | 1 | 0 | 1 | 1 | 6/10 |
Aprile I, 2020 | 1 | 1 | 0 | 1 | 0 | 0 | 1 | 0 | 1 | 1 | 1 | 6/10 |
Brokaw EB, 2014 | 1 | 1 | 0 | 0 | 0 | 0 | 1 | 0 | 0 | 1 | 1 | 4/10 |
Burgar CG, 2011 | 1 | 1 | 0 | 1 | 0 | 0 | 1 | 1 | 0 | 1 | 1 | 6/10 |
Calabrò RS, 2019 | 1 | 1 | 1 | 1 | 0 | 0 | 1 | 1 | 1 | 1 | 1 | 8/10 |
Carpinella I, 2020 | 1 | 1 | 1 | 1 | 0 | 0 | 1 | 1 | 1 | 1 | 1 | 8/10 |
Chinambiri B, 2021 | 1 | 1 | 0 | 0 | 0 | 0 | 1 | 1 | 0 | 1 | 1 | 5/10 |
Cho KH, 2019 | 0 | 1 | 1 | 1 | 0 | 0 | 1 | 1 | 1 | 1 | 1 | 8/10 |
Cho KH, 2021 | 1 | 1 | 1 | 1 | 0 | 0 | 1 | 1 | 1 | 1 | 1 | 8/10 |
Conroy SS, 2011 | 1 | 1 | 0 | 1 | 0 | 0 | 1 | 1 | 0 | 1 | 1 | 6/10 |
Conroy SS, 2019 | 1 | 1 | 0 | 1 | 0 | 0 | 1 | 1 | 0 | 1 | 1 | 6/10 |
Daly JJ, 2005 | 1 | 1 | 0 | 1 | 0 | 0 | 1 | 1 | 0 | 0 | 0 | 5/10 |
Daly JJ, 2019 | 1 | 1 | 1 | 1 | 0 | 0 | 0 | 1 | 0 | 1 | 1 | 6/10 |
Daunoraviciene K, 2018 | 0 | 1 | 0 | 1 | 0 | 0 | 0 | 1 | 0 | 1 | 1 | 5/10 |
Dehem S, 2019 | 1 | 1 | 1 | 1 | 0 | 0 | 1 | 0 | 1 | 1 | 1 | 7/10 |
Doost MY, 2021 | 1 | 1 | 0 | 0 | 1 | 0 | 0 | 0 | 0 | 0 | 1 | 3/10 |
Edwards DJ, 2019 | 1 | 1 | 0 | 1 | 1 | 1 | 1 | 0 | 0 | 1 | 1 | 7/10 |
Fazekas G, 2007 | 0 | 1 | 0 | 0 | 0 | 0 | 1 | 0 | 0 | 0 | 0 | 2/10 |
Gandolfi M, 2019 | 1 | 1 | 1 | 1 | 0 | 0 | 1 | 1 | 1 | 1 | 1 | 8/10 |
Grigoras AV, 2021 | 1 | 1 | 0 | 1 | 0 | 0 | 0 | 1 | 0 | 1 | 1 | 5/10 |
Gueye T, 2021 | 1 | 1 | 0 | 1 | 0 | 0 | 0 | 1 | 0 | 1 | 1 | 6/10 |
Han Yoo D, 2013 | 0 | 1 | 0 | 1 | 0 | 0 | 1 | 0 | 0 | 1 | 1 | 5/10 |
Hesse S, 2005 | 1 | 1 | 1 | 0 | 0 | 0 | 1 | 1 | 1 | 1 | 1 | 7/10 |
Hesse S, 2014 | 1 | 1 | 0 | 1 | 0 | 0 | 1 | 1 | 1 | 1 | 1 | 7/10 |
Horsley S, 2019 | 1 | 1 | 1 | 1 | 0 | 0 | 1 | 1 | 1 | 1 | 1 | 8/10 |
Housman SJ, 2009 | 1 | 1 | 0 | 1 | 0 | 0 | 1 | 0 | 0 | 1 | 1 | 5/10 |
Hsieh YW, 2011 | 1 | 1 | 1 | 1 | 0 | 0 | 1 | 1 | 1 | 1 | 1 | 8/10 |
Hsieh YW, 2016 | 0 | 1 | 1 | 1 | 0 | 0 | 1 | 1 | 0 | 1 | 1 | 7/10 |
Hsu Hy, 2019 | 0 | 1 | 1 | 1 | 0 | 0 | 1 | 1 | 1 | 1 | 1 | 8/10 |
Huang Y, 2020 | 1 | 1 | 1 | 1 | 0 | 0 | 1 | 1 | 1 | 1 | 1 | 8/10 |
Hung CS, 2019 | 0 | 1 | 1 | 1 | 0 | 0 | 1 | 1 | 1 | 1 | 1 | 8/10 |
Hung CS Hsieh YW, 2019 | 1 | 1 | 1 | 1 | 0 | 0 | 1 | 1 | 0 | 1 | 1 | 7/10 |
Hung CS, Lin KC, 2019 | 0 | 1 | 1 | 1 | 0 | 0 | 1 | 1 | 1 | 1 | 1 | 8/10 |
Hwang Ch, 2012 | 1 | 1 | 0 | 1 | 0 | 0 | 1 | 1 | 0 | 1 | 1 | 6/10 |
Iwamoto Y, 2019 | 1 | 1 | 0 | 1 | 0 | 0 | 0 | 1 | 1 | 1 | 1 | 6/10 |
Jiang S, 2021 | 1 | 1 | 0 | 1 | 0 | 0 | 0 | 1 | 1 | 1 | 1 | 6/10 |
Kahn LE, 2006 | 1 | 1 | 0 | 0 | 0 | 0 | 1 | 0 | 0 | 1 | 1 | 4/10 |
Kim MS, 2019 | 1 | 1 | 0 | 1 | 0 | 0 | 1 | 1 | 0 | 1 | 0 | 5/10 |
Klamroth-Marganska V, 2014 | 1 | 1 | 1 | 1 | 0 | 0 | 1 | 1 | 1 | 1 | 1 | 8/10 |
Kutner NG, 2010 | 0 | 1 | 1 | 1 | 0 | 0 | 1 | 0 | 0 | 1 | 1 | 6/10 |
Lee HC, 2021 | 1 | 1 | 0 | 1 | 0 | 0 | 1 | 1 | 1 | 1 | 1 | 7/10 |
Lee KW, 2016 | 1 | 1 | 0 | 1 | 0 | 0 | 0 | 0 | 0 | 1 | 1 | 4/10 |
Lee KW, 2017 | 1 | 1 | 0 | 1 | 0 | 0 | 0 | 1 | 0 | 1 | 1 | 5/10 |
Lee MJ, 2018 | 1 | 1 | 0 | 1 | 0 | 0 | 1 | 1 | 1 | 1 | 1 | 7/10 |
Lee SH, 2020 | 1 | 1 | 1 | 1 | 0 | 0 | 1 | 1 | 0 | 1 | 1 | 7/10 |
Liao WW, 2012 | 1 | 1 | 1 | 1 | 0 | 0 | 1 | 1 | 0 | 1 | 1 | 7/10 |
Lo AC, 2010 | 1 | 1 | 0 | 1 | 0 | 0 | 1 | 1 | 1 | 1 | 1 | 7/10 |
Lum PS, 2002 | 0 | 1 | 0 | 1 | 0 | 0 | 1 | 1 | 0 | 1 | 1 | 6/10 |
Lum PS, 2006 | 0 | 1 | 0 | 0 | 0 | 0 | 1 | 0 | 0 | 1 | 1 | 4/10 |
Masiero S, 2007 | 1 | 1 | 0 | 1 | 0 | 0 | 1 | 1 | 0 | 1 | 1 | 6/10 |
Masiero S, 2014 | 1 | 1 | 0 | 1 | 0 | 0 | 1 | 0 | 1 | 1 | 1 | 6/10 |
Mazzoleni S, 2019 | 1 | 1 | 0 | 1 | 1 | 0 | 0 | 1 | 0 | 1 | 1 | 6/10 |
McCabe J, 2015 | 0 | 1 | 0 | 1 | 0 | 0 | 1 | 1 | 1 | 1 | 1 | 7/10 |
Orihuela-Espina F, 2016 | 1 | 1 | 0 | 1 | 0 | 0 | 0 | 1 | 1 | 1 | 1 | 6/10 |
Park JH, 2020 | 1 | 1 | 1 | 1 | 1 | 0 | 0 | 1 | 0 | 1 | 1 | 7/10 |
Park JH, 2021 | 1 | 1 | 1 | 1 | 0 | 0 | 1 | 1 | 1 | 1 | 1 | 8/10 |
Perini G, 2020 | 1 | 1 | 0 | 1 | 1 | 0 | 1 | 1 | 1 | 1 | 1 | 8/10 |
Qian Q, 2017 | 1 | 1 | 0 | 1 | 0 | 0 | 1 | 1 | 0 | 1 | 1 | 6/10 |
Qian Q, 2019 | 1 | 1 | 0 | 1 | 0 | 0 | 0 | 1 | 1 | 1 | 1 | 6/10 |
Rabadi M, 2008 | 1 | 1 | 1 | 0 | 0 | 0 | 1 | 1 | 0 | 1 | 1 | 6/10 |
Ranzani R, 2020 | 1 | 1 | 0 | 1 | 0 | 0 | 0 | 1 | 1 | 1 | 1 | 6/10 |
Rodgers H, 2019 | 1 | 1 | 1 | 1 | 0 | 0 | 1 | 1 | 1 | 1 | 1 | 8/10 |
Rosenthal O, 2019 | 1 | 1 | 0 | 1 | 0 | 0 | 1 | 0 | 0 | 1 | 1 | 5/10 |
Sale P, 2014 | 0 | 1 | 0 | 0 | 0 | 0 | 1 | 1 | 1 | 1 | 1 | 6/10 |
Serrezuela RR, 2020 | 0 | 1 | 0 | 1 | 0 | 0 | 1 | 1 | 0 | 1 | 0 | 5/10 |
Straudi S, 2019 | 1 | 1 | 0 | 1 | 0 | 0 | 1 | 0 | 1 | 1 | 1 | 6/10 |
Susanto EA, 2015 | 0 | 1 | 0 | 1 | 0 | 0 | 1 | 1 | 1 | 1 | 1 | 7/10 |
Takahashi K, 2016 | 1 | 1 | 0 | 0 | 0 | 0 | 1 | 1 | 0 | 1 | 1 | 5/10 |
Taveggia G, 2016 | 0 | 1 | 0 | 1 | 0 | 0 | 1 | 1 | 1 | 1 | 1 | 7/10 |
Timmermans AA, 2014 | 1 | 1 | 1 | 1 | 0 | 0 | 1 | 1 | 1 | 1 | 1 | 8/10 |
Tomić TJ, 2017 | 1 | 1 | 0 | 1 | 0 | 0 | 1 | 1 | 1 | 1 | 1 | 7/10 |
Tramontano M, 2020 | 1 | 1 | 1 | 1 | 0 | 0 | 1 | 1 | 1 | 1 | 1 | 8/10 |
Vanoglio F, 2017 | 1 | 1 | 1 | 1 | 0 | 0 | 1 | 1 | 0 | 1 | 1 | 7/10 |
Villafañe JH, 2018 | 0 | 1 | 0 | 1 | 0 | 0 | 1 | 1 | 1 | 1 | 1 | 7/10 |
Volpe BT, 2000 | 1 | 1 | 0 | 1 | 0 | 0 | 1 | 1 | 0 | 1 | 1 | 6/10 |
Wolf SL, 2015 | 1 | 1 | 0 | 1 | 0 | 0 | 1 | 1 | 1 | 1 | 1 | 7/10 |
Wu CY, 2012 | 0 | 1 | 1 | 1 | 1 | 0 | 1 | 1 | 0 | 1 | 1 | 8/10 |
Xu Q, 2020 | 1 | 1 | 0 | 1 | 0 | 1 | 1 | 1 | 0 | 1 | 1 | 7/10 |
Zengin-Metli D, 2018 | 1 | 1 | 0 | 1 | 0 | 0 | 0 | 1 | 1 | 1 | 1 | 6/10 |
*Eligibility criteria was not used to calculate the PEDro score.
Except for Brokaw et al. (2014) and Kutner et al. (2010) – who reported the total time of RT – and Rosenthal et al. (2019) –who didn’t specify session duration – all studies specified RT duration characteristics (i.e., total of sessions, sessions per week, duration of each session). RT ranged from 2 to 64 sessions, with an average of 4 sessions per week. Duration of each RT varied from 20 to 120 min.
Therefore, patients performed conventional therapy, propaedeutic therapy, or additional therapy (i.e., functional electrical stimulation –FES) in more than half of the studies. Characteristics of RT and additional therapy are reported in Supplementary Table 1.
3.5Comparison
All studies have at least one control group. All the studies control groups are composed of patients who have the same baseline characteristics as the robotic group, except for Doost et al. (2021), who used a group of healthy subjects to normalize the obtained data.
In sixty studies patients in the control group underwent conventional therapy, of these in three studies (Burgar et al., 2011; Masiero et al., 2007; Volpe et al., 2000) patients were exposure to the robot in addition to the traditional treatment.
Regarding the remaining studies, in fifteen studies, comparison underwent two different types of RT: Iwamoto et al. (2019) and Conroy et al. (2019) combined RT and conventional therapy.
Therefore, in four studies (Daly et al., 2005, 2019; Hesse et al., 2005; McCabe et al., 2015) functional neuromuscular electrical stimulation (FNS) or FES was used in control group. Characteristics of comparison population and their rehabilitation programme are reported in Supplementary Table 1.
3.6Cognitive inclusion/exclusion criteria
A total of seventy-three article included cognitive inclusion or exclusion criteria.
Nineteen studies looked at both cognitive inclusion and exclusion criteria, twenty-nine looked at only inclusion criteria, and twenty-five looked at cognitive exclusion criteria.
A total of forty-nine studies considered cognitive inclusion criteria and thirty-seven used a single or more scales to evaluate cognitive inclusion performances.
The Mini Mental State Examination (MMSE) (Folstein et al., 1975) was the most used cognitive screening (twenty-nine studies out of forty-six studies), however the cut off varied based on the studies: 24 points was the cutoff longer used (Calabrò et al., 2019; Gandolfi et al., 2019; Han Yoo et al., 2012; Hsieh et al., 2011; Hsu et al., 2019; Hung CS et al., 2019; Kutner et al., 2010; Perini et al., 2021; Tramontano et al., 2020), followed by 22 (Hsieh et al., 2016; C. S. Hung, Hsieh, Wu, Chen, et al., 2019; C. Shan Hung et al., 2019; Liao et al., 2012; Wu et al., 2012), 21 (Daunoraviciene et al., 2018; Y. Huang et al., 2020; Qian et al., 2017, 2019; Susanto et al., 2015) (50,68,73,104,106), 20 (Ambrosini et al., 2021; Sale et al., 2014), 18 (Jiang et al., 2021; Masiero et al., 2007). Only Dehem et al. (2019) used a cut-off of 15 points, but it was also required that patients had the ability to “understand instructions”. Therefore, Rosenthal et al. (2019) did not specify the cutoff utilized.
Five studies specified the use of Korean version of MMSE (Park and Kwon, 1989) with different cut offs according to each study: three studies (Cho and Song, 2019, 2021; Park, 2021) settled it at 24 points; Lee et al. (2018) considered 21 points while Lee et al. (2017) considered patients with a MMSE ≥ 10 points.
Other scales used were: Lowenstein Occupational Therapy Cognitive Assessment (LOTCA) (Hsu et al., 2019), Line Bisection Test and the Korean version of the Motor-free Visual Perception Test—Third Edition (MVPT-3) (Park, 2021), Catherine Bergego Scale (Gueye et al., 2021), Cognistat instrument (Lum et al., 2002) and Short Portable Mental Status Questionnaire (Wolf et al., 2015).
Regarding the exclusion criteria, forty-four studies considered at least one cognitive evaluation. Similar to cognitive inclusion criteria, MMSE (Folstein et al., 1975) is the most used assessment scale, although the cutoff is not standardized: it varied from 20 (Carpinella et al., 2020) to 27 (Orihuela-Espina et al., 2016), however the most commonly used value is 21 (Daunoraviciene et al., 2018; Lum et al., 2006; Straudi et al., 2020; Taveggia G et al., 2016). Therefore, Kim et al. (2019) evaluated their patients using Korean version of the MMSE (Park and Kwon, 1989), with a 15 points cut-off. Another evaluation scale used to evaluate severe cognitive deficit was the Levels of Cognitive Functioning-Revised (Ranzani et al., 2020).
Other assessment scales used could be classified according to the neuropsychologic impairments: the Alexander Scale (Hwang et al., 2012) was the only assessment scale for apraxia; the Bell Test (Masiero et al., 2014) was used to assess attention level, while the Barrage Test (Masiero et al., 2014) and the Star Cancellation Test (Wolf et al., 2015) were used to assess neglect. Regarding aphasia, four assessments were used: the NIH Stroke Scale (question IX) (Hwang et al., 2012), the Neuropsychological Aphasia Test (Masiero et al., 2014), the Gellanza-Coen Test (Masiero et al., 2014) and the Goodglass and Kaplan Test (Ranzani et al., 2020). Supplementary Table 1 reports data on cognitive inclusion and exclusion criteria.
3.7Cognitive outcome
Seven studies (Daunoraviciene et al., 2018; Gueye et al., 2021; Iwamoto et al., 2019; Park, 2021; Ranzani et al., 2020; Volpe et al., 2000; Zengin-Metli et al., 2018) analyzed cognitive outcomes.
The most used was the Functional Indipendence Measure (FIM) cognitive subscore that was analyzed in three studies (Iwamoto et al., 2019; Volpe et al., 2000; Zengin-Metli et al., 2018); the MMSE was used in two studies (Ranzani et al., 2020; Zengin-Metli et al., 2018). Other measures of cognitive outcomes are: the Addenbrooke’s Cognitive Examination-Revised (ACE-R) (Daunoraviciene et al., 2018), the Frontal Assessment Battery (FAB) (Ranzani et al., 2020) and the Montreal Cognitive Assessment (MoCA) (Gueye et al., 2021).
Unilateral spatial neglet was investigated in two different studies with three different scales: the Albert Test (AT) (Park, 2021; Ranzani et al., 2020), the Line Bisection Test (LBT) (Park, 2021) and the Catherine Bergego Scale (CBS) (Park, 2021).
In all the studies, a clinical cognitive evaluation was performed before and after the treatment. One study (Iwamoto et al., 2019) carried out mid-term pre-treatment and post-treatment evaluations every 5 days, while Ranzani et al. (2020) assessed follow-up evaluations at 8 weeks and 6 months after the end of treatment. Results are shown in Supplementary Table 1.
Significant improvement in between group analysis is shown in ACE-R (Daunoraviciene et al., 2018). FIM cognitive subscore showed significant improvement in both experimental and control groups in two out of three studies (Volpe et al., 2000; Zengin-Metli et al., 2018).
Ranzani et al. (2020)s observed minor improvements –not statistical significant –in both groups over time in FAB score.
Regarding MMSE, Zengin-Metli et al. (2018) found a significant improvement in the control group, maybe because a significant difference was observed between the robot and the control group in terms of pre-treatment MMSE levels (pretraining: 17 normal robotic group vs 8 normal and 6 mild in control group), while no significant improvement was found in Ranzani et al. (2020). Therefore, no significant improvement was found in MoCA results (Gueye et al., 2021).
Regarding unilateral spatial neglet, Park et al. (2021) found that RT reduces hemispatial neglect symptoms in the participants’ activities of daily living: AT and LBT improved significally in experimental group, while CBS showed significant improvement in both groups, but a statistically significant difference in changes in RT group. Instead, Ranzani et al. (2020) observed only minor improvements in both groups over time.
3.8Methodological quality
Methodological quality was assessed with PEDro scale (Cashin and McAuley, 2020): according to the compilation guidelines of the assessment scale, “eligibility criteria” was not used to calculate the PEDro score. According to the literature, in order to simplify the interpretation of results, articles were classified in four categories: a total PEDro score of 0-3 are considered ‘poor’, 4-5 ‘fair’, 6-8 ‘good’, and 9-10 ‘excellent’. Therefore, for trials evaluating complex interventions (e.g., exercise) a total PEDro score of 8/10 is optimal (Summary of Measurement Properties of the PEDro Scale –PEDro, n.d.).
Details of the PEDro score are reported in Table 2: the lower evaluation was 2/10 (Fazekas et al., 2007) and the higher was 8/10 (Calabrò et al., 2019; Carpinella et al., 2020; Cho and Song, 2019, 2021; Gandolfi et al., 2019; Horsley et al., 2019; Hsieh et al., 2016; Hsu et al., 2019; Y. Huang et al., 2020; C. S. Hung, Hsieh, Wu, Lin, et al., 2019; C. Shan Hung et al., 2019; Klamroth-Marganska et al., 2014; Park, 2021; Perini et al., 2021; Rodgers et al., 2019b; Timmermans et al., 2014; Tramontano et al., 2020; Wu et al., 2012).
Most of the studies (sixty-three over eighty-one) were considered “good”, while there were not “excellent” evaluation.
“Random allocation” was undertaken by all studies while “concealed allocation” was considered only in 28 studies and “baseline comparability” in 71 studies. Most of the studies did not consider the blind of patient and therapists, of all the articles included, six articles (Doost et al., 2021; Edwards et al., 2019; Mazzoleni et al., 2019; Park, 2021; Perini et al., 2021; Wu et al., 2012) and two (Edwards et al., 2019; Wu et al., 2012) of the therapists. Assessors were blind in 66 over 81 studies.
Regarding the statistical analysis, 66 studies had “adequate follow-up”, thirty-nine studies reported “intention-to-treat analysis”, while “between-group comparisons” were reported in 78 studies and “point estimates and variability” were 76 studies.
4Discussion
Stroke survivors experience motor dysfunction and impaired memory and cognition. These symptoms are associated with disruption of normal neuronal function, interhemispherical connections and synaptic activity, and thus disruption of the normal neural circuit. Physical exercise is considered an effective and feasible rehabilitation strategy to improve cognitive and motor recovery after ischemic stroke through the facilitation of neuroplasticity.
Cognitive disorders are frequently in stroke patients: a pooled data analysis conducted in 2009 (V. S. Huang and Krakauer, 2009) showed a prevalence of 38 % (95% confidence intervals, 32 % to 43%) of post-stroke cognitive impairment in the first year after stroke. This confirms the needing of an early and routine assessment of the cognitive disorder. In order to structure an efficient individual rehabilitative protocol, predict future outcomes, such as cognitive impairment, and evaluate the effectiveness of intervention are fundamental in stroke rehabilitation. In fact, cognitive impairment can influence the recovery of motor and activity daily living: functions like attention and memory allow people to stay focused and improve the ability to cope with the proposed tasks, moreover these cognitive functions form the foundation of all cognitive performances. So if a patient is exercised in attention and memory he could improve easily in cognitive functioning (Aprile et al., 2021).
In addition, the hand and the upper limb are a powerful organizer of human experience and play a central role in cognitive processes: motor recovery is not only related to the motor processes but also to the development of cognitive and sensory strategies (Sallés et al., 2017). Without hand functions (i.e. grasping or manipulation), important information, connected with tactile, somatosensory and proprioceptive system, cannot be developed resulting in a limited reworking at the central level.
Consequently, it becomes fundamental to analyze both motor and cognitive outcomes when talking about upper limb rehabilitation: to obtain a recovery that focuses on the quality of functions and abilities, it is important to promote an adequate reorganization of neural patterns and an adequate activation of the existing patterns prior to the injury.(Arya et al., 2011)
The aim of this review is to verify whether, in the current state of the literature, cognitive measures are used as inclusion or exclusion criteria and/or outcome measures in robotic upper limb rehabilitation in stroke patients.
The results of this review show that 90% (seventythree out of eighty-one) of the included studies had at least one cognitive inclusion or exclusion criteria, while cognitive outcomes were only assessed in seven studies (9%). The high percentage of criteria for cognitive inclusion and exclusion shows that stroke rehabilitation focuses mainly on motor recovery(Everard et al., 2020) robotic and/or electromechanical devices in combination with traditional neuromotor approaches promote motor control and functional recovery of the limb in patients with stroke (SPREAD, 2016).
Research on stroke rehabilitation is focusing on demonstrating the effectiveness of new rehabilitative approaches based on current knowledge of neuroplasticity mechanisms. Among these, robotic rehabilitation is a useful therapy because it seems to have a positive effect on neuronal plasticity(SPREAD, 2016), due to its characteristics of intensity, repeatability, significance and multisensoriality.
Modern models of cognitive rehabilitation embrace the plastic processes of the brain involved in relearning or recovery of cognitive function following brain injury and with that follows that cognitive rehabilitation training needs to be delivered frequently, intensively and with appropriate level of difficulty to have an effect (Wilms, 2020).
Moreover, some recent studies have proven that RT could influence cognitive abilities as well as motor functions, for example, RT seems to reduce neglect compared to visual cueing in the neglect sides (Park, 2021; Reinhart et al., 2012) and could cause cortical activity changes (shown by functional MRI) (Calabrò et al., 2016). This underlines the need of a cognitive evaluation at the rehabilitation beginning, during and at the end of the rehabilitation treatment.
Therefore, the exclusion of people with cognitive problems makes it impossible to generate clinical considerations for the whole population affected by stroke, because the results are limited to a low percentage of stroke population (Everard et al., 2020). In line with Everard et al. (2020) and Stinear et al. (2020), in this systematic review emerges the need to implement trials that include this type of patients to verify the positive cognitive effects of RT on stroke patients affected by cognitive decline post-stroke. The cognitive evaluation also allows not to exclude any patient a priori but rather to evaluate the rehabilitation margins including the use of robotics / technology to implement recovery.
Another result that emerges from this review is the lack of a common line for both inclusion and exclusion criteria, with consequent limitation of the evaluation of the effects of robotic rehabilitation therapies and increased risks of overestimation and/or underestimation.
A recent systematic scoping review (Saa et al., 2019) highlighted that the MMSE is the most used scale, although a heterogeneity of the cognitive instruments used is still present. This result is in line with ours, moreover in this systematic review also emerges the lack of a common cut-off between the studies: a recent study (Bour et al., 2010) highlighted how cut-off score in the screening for at least 4 impaired domains and dementia were 26/27 and 23/24 with a sensitivity of 0.82 and 0.96, respectively (Bour et al., 2010).
The MMSE has modest qualities in screening for mild cognitive disturbances and is adequate in screening for moderate cognitive deficits or dementia in stroke patients 1 month after stroke, however Huang et al. (2009) reported that the MoCA have a higher sensitivity and specificity for initial cognitive functional screening after stroke. It would be useful to develop a guideline that identifies which scale and cutoff should be used in the various stages of poststroke rehabilitation.
The heterogeneity in the inclusion and exclusion criteria is also present in the studies that investigated the effects of robotic rehabilitation on cognitive performance: in four out of the seven included studies (Daunoraviciene et al., 2018; Park, 2021; Ranzani et al., 2020; Volpe et al., 2000), there was an improvement in some memory-attentive performances, with consequent effect on daily autonomy (Daunoraviciene et al., 2018; Park, 2021). It is important to emphasize that in the study of Zengin-Metli (Zengin-Metli et al., 2018) the MMT increased only in the control group because a significant difference was observed between the robot and the control group in terms of pre-treatment MMT levels: 17 of 20 patients in experimental group and 8 in the control group were in the normal cognitive level while 6 were mild cognitive impairment in the control group. Instead, Ranzani et al. (2020) pointed out that small changes in control and study groups could be linked to the saturation of the scales used in their study in a mildly/moderately impaired population.
The heterogeneity of the rehabilitative protocols, the lack of a comparability with the baseline of the patients included and the use of no standard assessment protocols do not allow the generalization of the results, both in a positive and negative sense.
Further randomized and controlled trials with an adequate number of patients are therefore needed, with a battery of similar cognitive tests in order to compare the different studies and generalize the results obtained according to the various stroke phases.
This systematic review has some limitations: heterogeneous evaluations and treatments, all stroke patients were included, without rigid stratification for clinical and radiological characteristics. These could lead to a failure to generalize our results. Therefore, studies until 2021 were included, the lack of 2022 may have excluded studies that could have led to different results in this review.
5Conclusion
This systematic review highlights that more than 90% of the studies included considered a cognitive inclusion and exclusion criteria, while only 9% considered cognitive outcomes.
Future larger RCTs are needed in order to outline which clinical scales are most suitable and with which cut-off, as well as what cognitive outcome measures to use in the various stages of post-stroke rehabilitation. Therefore, future studies are needed to test the use of robotics in patients with cognitive impairment in order to generalize the results obtained with RT in stroke patients.
Finally, we must not forget that the same cognitive difficulties affect motor recovery and residual disabilities in a decisive way. Containing and improving these difficulties would also have a significant impact on social and health expenditure for greater preservation of patients’ autonomy.
Acknowledgments
The present study has been carried out within the framework of the “Italian Consensus Conference on Rehabilitation Assisted by Robotic and Electromechanical Devices for Persons with Disability of Neurological Origin” (CICERONE), promoted by the Italian Society of Physical and Rehabilitation Medicine (SIMFER, Società Italiana di Medicina Fisica e Riabilitativa) and Italian Society of Neurological Rehabilitation (SIRN, Società Italiana di Riabilitazione Neurologica).
Group name: Upper limbworking group within the “Italian Consensus Conference on Rehabilitation Assisted by Robotic and Electromechanical Devices for Persons with Disability of Neurological Origin” (CICERONE): Michela Agostini; Irene Aprile; Chiara Arienti; Ludovica Baluardo; Alberto Battistini; Paolo Benanti; Valentina Boetto; Paolo Boldrini; Donatella Bonaiuti; Marco Bravi; Federica Bressi; Benedetta Campagnola; Emanuela Casanova; Enrico Castelli; Laura Cricenti; Manuela Desilvestri; Francesco Draicchio; Vincenzo Falabella; Serena Filoni; Silvia Galeri; Marialuisa Gandolfi; Stefano Gargano; Daniele Giansanti; Francesca Gimigliano; Mauro Grigioni; Giuseppe La Rosa; Lorenzo Lippi; Dario Marino; Alex Martino Cinnera; Stefano Mazzoleni; Stefano Mazzon; Sandra Miccinilli; Franco Molteni; Giovanni Morone; Matteo Paci; Angela Palomba; Cristiano Pecchioli; Luca Perrero; Maurizio Petrarca; Salvatore Petrozzino; Alessandro Picelli; Federico Posteraro; Emanuele Francesco Russo; Fabio Santacaterina; Michele Senatore; Monica Sicari; Claudio Marcello Solaro; Silvia Sterzi; Sofia Straudi; Giuseppe Turchetti.
Conflict of interest
None of the authors disclose any financial or personal relationships with other people or organizations that could inappropriately influence (bias) this work. The authors have no affiliation with any organization with a financial interest, direct or indirect, in the subject matter or materials discussed in the manuscript (such as consultancies, employment, paid expert testimony, honoraria, speakers bureaus, retainers, stock options or ownership, patents or patent applications or travel grants) that may affect the conduct or reporting of the work submitted.
Funding
The authors report no funding.
Supplementary materials
[1] Supplementary Table 1 is available from https://dx.doi.org/10.3233/NRE-220149.
References
1 | Abdullah H.A. , Tarry C. , Lambert C. , Barreca S. , & Allen B.O. ((2011) ). Results of clinicians using a therapeutic robotic system inan inpatient stroke rehabilitation unit. Journal of NeuroEngineering and Rehabilitation, 8: (1). |
2 | Adomavicienė A. , Daunoravicienė K. , Kubilius R. , Varzaitytė L. , & Raistenskis J. ((2019) ). Influence of New Technologies on Post-Stroke Rehabilitation: A Comparison of Armeo Spring to the Kinect System. Medicina (Kaunas) [Internet]. [cited 2022 Mar 17];55: (4). Available from: https://pubmed.ncbi.nlm.nih.gov/30970655/ |
3 | Aisen M.L. , Krebs H.I. , Hogan N. , McDowell F. , & Volpe B.T. ((1997) ). The effect of robot-assisted therapy and rehabilitative training on motor recovery following stroke. Arch Neurol [Internet]. [cited 2022 May 26];54: (4), 443–6. Available from: https://pubmed.ncbi.nlm.nih.gov/9109746/ |
4 | Alt Murphy M. , Baniña M.C. , & Levin M.F. ((2017) ). Perceptuo-motor planning during functional reaching after stroke. Exp Brain Res [Internet]. [cited 2022 Mar 17];235: (11), 3295–306. Available from: https://pubmed.ncbi.nlm.nih.gov/28803362/ |
5 | Ambrosini E. , Gasperini G. , Zajc J. , Immick N. , Augsten A. , Rossini M. , et al. ((2021) ). A Robotic System with EMG-Triggered Functional Eletrical Stimulation for Restoring Arm Functions inStroke Survivors. Neurorehabil Neural Repair [Internet]. [cited 2022 May 31];35: (4), 334–45. Available from: https://pubmed.ncbi.nlm.nih.gov/33655789/ |
6 | Ang K.K. , Guan C. , Phua K.S. , Wang C. , Zhou L. , Tang K.Y. , et al. ((2014) ). Brain-computer interface-based robotic end effector system for wrist and hand rehabilitation: Results of a three-armed randomized controlled trial for chronic stroke. Frontiers in Neuroengineering, 7: (JUL). |
7 | Aprile I. , Germanotta M. , Cruciani A. , Loreti S. , Pecchioli C. , Cecchi F. , et al. ((2020) ). Upper Limb Robotic Rehabilitation AfterStroke: A Multicenter, Randomized Clinical Trial. J Neurol Phys Ther[Internet]. [cited 2022 Jun 22];44: (1), 3–14. Available from: https://pubmed.ncbi.nlm.nih.gov/31834217/ |
8 | Aprile I. , Guardati G. , Cipollini V. , Papadopoulou D. , Monteleone S. , Redolfi . , et al. ((2021) ). Influence of Cognitive Impairment on the Recovery of Subjects with Subacute Stroke Undergoing Upper Limb Robotic Rehabilitation. Brain Sci [Internet]. [cited 2022 Mar 17];11: (5). Available from: https://pubmed.ncbi.nlm.nih.gov/33946452/ |
9 | Armeo®Spring –Hocoma [Internet]. [cited 2022 May 26]. Available from: https://www.hocoma.com/solutions/armeo–spring/ |
10 | Balasubramanian S. , Klein J. , & Burdet E. ((2010) ). Robot-assistedrehabilitation of hand function. Curr Opin Neurol [Internet][cited2022 May 26];23: (6), 661–70. Available from: https://pubmed.ncbi.nlm.nih.gov/20852421/ |
11 | Blackburn D.J. , Bafadhel L. , Randall M. , & Harkness K.A. ((2013) ). Cognitive screening in the acute stroke setting. Age Ageing [Internet]. [cited 2022 Mar 17];42: (1), 113–6. Available from: https://pubmed.ncbi.nlm.nih.gov/22923608/ |
12 | Bour A. , Rasquin S. , Boreas A. , Limburg M. , & Verhey F. ((2010) ). How predictive is the MMSE for cognitive performance afterstroke? Journal of Neurology [Internet]. [cited 2022 May31];257: (4), 630. Available from: /pmc/articles/PMC2848722/ |
13 | Brokaw E.B. , Nichols D. , Holley R.J. , & Lum P.S. ((2014) ). Robotic therapy provides a stimulus for upper limb motor recoveryafter stroke that is complementary to and distinct from conventional therapy. Neurorehabilitation and Neural Repair, 28: (4), 367–76. |
14 | Brown D. ((2020) ). A Review of the PubMed PICO Tool: Using Evidence-Based Practice in Health Education. Health Promot Pract [Internet]. [cited 2022 May 31];21: (4), 496–8. Available from: https://pubmed.ncbi.nlm.nih.gov/31874567/ |
15 | Bressi F. , Bravi M. , Campagnola B. , Bruno D. , Mazzolla A. , Santacaterina F. , et al. ((2020) ). Robotic treatment of the upperlimb in chronic stroke and cerebral neuroplasticity: a systematic review –Pub Med. J Biol Regul Homeost Agents [Internet]. [cited 2022 Mar 17];34: (5 Suppl.3), 11–44. Available from: https://pubmed.ncbi.nlm.nih.gov/33386032/ |
16 | Burgar C.G. , Lum P.S. , Erika Scremin A.M. , Garber S.L. , Machielvan der Loos H.F. , Kenney . , et al. ((2011) ). Robot-assistedupper-limb therapy in acute rehabilitation setting following stroke:Department of veterans affairs multisite clinical trial. Journal of Rehabilitation Research and Development, 48: (4), 445–58. |
17 | Calabrò R.S. , Russo M. , Naro A. , Milardi D. , Balletta T. , Leo . , et al. ((2016) ). Who May Benefit From Armeo Power Treatment? A Neurophysiological Approach to Predict Neurorehabilitation Outcomes. PM R [Internet]. [cited 2022 May 31];8: (10), 971–8. Available from: https://pubmed.ncbi.nlm.nih.gov/26902866/ |
18 | Calabrò R.S. , Accorinti M. , Porcari B. , Carioti L. , Ciatto L. , Billeri L. , et al. ((2019) ). Does hand robotic rehabilitationimprove motor function by rebalancing interhemispheric connectivityafter chronic stroke? Encouraging data from arandomised-clinical-trial. Clinical Neurophysiology 130: (5), 767–80. |
19 | Cashin A.G. , & McAuley J.H. ((2020) ). Clinimetrics: Physiotherapy Evidence Database (PEDro) Scale. J Physiother [Internet]. [cited 2022 May 26];66: (1), 59. Available from: https://pubmed.ncbi.nlm.nih.gov/31521549/ |
20 | Carpinella I. , Lencioni T. , Bowman T. , Bertoni R. , Turolla A. , Ferrarin M. , et al. ((2020) ). Effects of robot therapy on upper body kinematics and arm function in persons post stroke: a pilot randomized controlled trial. Journal of NeuroEngineering and Rehabilitation 17: (1). |
21 | Chinembiri B. , Ming Z. , Kai S. , Xiu Fang Z. , & Wei C. ((2021) ). The fourier M2 robotic machine combined with occupational therapy onpost-stroke upper limb function and independence-related quality oflife: A randomized clinical trial. Topics in Stroke Rehabilitation 28: (1), 1–18. |
22 | Cho K.H. , & Song W.K. ((2021) ). Effects of two different robot-assisted arm training on upper limb motor function andkinematics in chronic stroke survivors: A randomized controlledtrial. Topics in Stroke Rehabilitation 28: (4), 241–50. |
23 | Chen C. , Leys D. , & Esquenazi A. ((2013) ). The interaction betweenneuropsychological and motor deficits in patients after stroke. Neurology [Internet]. [cited 2022 Mar 17];80: (3 Suppl 2). Available from: https://pubmed.ncbi.nlm.nih.gov/23319483/ |
24 | Cho K.H. , & Song W.K. ((2019) ). Robot-Assisted Reach Training Withan Active Assistant Protocol for Long-Term Upper Extremity Impairment Poststroke: A Randomized Controlled Trial. Archives of Physical Medicine and Rehabilitation 100: (2), 213–9. |
25 | Conroy S.S. , Wittenberg G.F. , Krebs H.I. , Zhan M. , Bever C.T. , & Whitall J. ((2019) ). Robot-Assisted Arm Training in ChronicStroke: Addition of Transition-to-Task Practice. Neurorehabilitation and Neural Repair 33: (9), 751–61. |
26 | Conroy S.S. , Whitall J. , Dipietro L. , Jones-Lush L.M. , Zhan M. , Finley M.A. , et al. ((2011) ). Effect of gravity on robot-assistedmotor training after chronic stroke: A randomized trial. Archives of Physical Medicine and Rehabilitation, 92: (11), 1754–61. |
27 | Daly J.J. , Hogan N. , Perepezko E.M. , Krebs H.I. , Rogers J.M. , Goyal K.S. , et al. ((2005) ). Response to upper-limb robotics and functional neuromuscular stimulation following stroke. Journal of Rehabilitation Research and Development 42: (6), 723–36. |
28 | Daly J.J. , McCabe J.P. , Holcomb J. , Monkiewicz M. , Gansen J. , & Pundik S. ((2019) ). Long-Dose Intensive Therapy Is Necessary for Strong, Clinically Significant, Upper Limb Functional Gains and Retained Gains in Severe/Moderate Chronic Stroke. Neurorehabilitation and Neural Repair 33: (7), 523–37. |
29 | Daunoraviciene K. , Adomaviciene A. , Grigonyte A. , Griskevicius J. , & Juocevicius A. ((2018) ). Effects of robotassisted training on upper limb functional recovery during the rehabilitation of poststroke patients. In: Technology and Health Care. IOS Press; S533–42. |
30 | Dehem S. , Gilliaux M. , Stoquart G. , Detrembleur C. , Jacquemin G. , Palumbo S. , et al. ((2019) ). Effectiveness of upper-limbrobotic-assisted therapy in the early rehabilitation phase afterstroke: A single-blind, randomised, controlled tria. Annals of Physical and Rehabilitation Medicine 62: (5), 313–20. |
31 | Doost M.Y. , Benoît Herman Denis A. , Sapin J. , Galinski D. , Riga . , et al. ((2021) ). Bimanual motor skill learning and roboticassistance for chronic hemiparetic stroke: A randomized controlledtrial. Neural Regeneration Research 16: (8), 1566–73. |
32 | Edwards D.J. , Cortes M. , Rykman-Peltz A. , Chang J. , Elder J. , Thickbroom . , et al. ((2019) ). Clinical improvement with intensiverobot-assisted arm training in chronic stroke is unchanged bysupplementary tDCS. Restorative Neurology and Neuroscience 37: (2), 167–80. |
33 | Everard G.J. , Ajana K. , Dehem S.B. , Stoquart G.G. , Edwards M.G. , & Lejeune T.M. ((2020) ). Is cognition considered inpost-stroke upper limb robot-assisted therapy trials? A briefsystematic review. International Journal of RehabilitationResearch Internationale Zeitschrift fur Rehabilitations forschung Revue internationale de recherches de readaptation [Internet].[cited 2022 Mar 17];43: (3), 195–8. Available from: https://pubmed.ncbi.nlm.nih.gov/32769583/ |
34 | Fazekas G. , Horvath M. , Troznai T. , & Toth A. ((2007) ). Robot-mediated upper limb physiotherapy for patients with spastichemiparesis: A preliminary study. Journal of Rehabilitation Medicine 39: (7), 580–2. |
35 | Feigin V.L. , Krishnamurthi R.V. , Parmar P. , Norrving B. , Mensah G.A. , Bennett D.A. , et al. ((2015) ). Update on the Global Burden ofIschemic and Hemorrhagic Stroke in 1990-2013: The GBD 2013 Study. Neuroepidemiology [Internet]. [cited 2022 Mar 21];45: (3), 161–76. .Available from: https://pubmed.ncbi.nlm.nih.gov/26505981/ |
36 | Folstein M.F. , Folstein S.E. , & McHugh P.R. ((1975) ). “Mini-mental state”. A practical method for grading the cognitivestate of patients for the clinician. J Psychiatr Res [Internet]. [cited 2022 May 26];12: (3), 189–98. Available from: https://pubmed.ncbi.nlm.nih.gov/1202204/ |
37 | Gandolfi M. , Valè N. , Dimitrova E.K. , Mazzoleni S. , Battini E. , Filippetti M. , et al. ((2019) ). Effectiveness of robot-assisted upper limb training on spasticity, function and muscle activity in chronic stroke patients treated with botulinum toxin: A randomized single-blinded controlled trial. Frontiers in Neurology 10: (JAN). |
38 | Gueye T. , Dedkova M. , Rogalewicz V. , Grunerova-Lippertova M. , & Angerova Y. ((2021) ). Early post-stroke rehabilitation for upper limbmotor function using virtual reality and exoskeleton: equallyefficient in older patients. Neurol Neurochir Pol [Internet][cited 2022 Mar 17];55: (1), 91–6. Available from: https://pubmed.ncbi.nlm.nih.gov/33314016/ |
39 | Grigoras A.V. , Irimia D.C. , Poboroniuc M.S. , & Popescu C.D. ((2016) ). Testing of a hybrid FES-robot assisted hand motor trainingprogram in sub-acute stroke survivors. Advances in Electricaland Computer Engineering 16: (4), 89–94. |
40 | Han Yoo D. , Jun Cha Y. , Kyoung Kim S. , & Shin Lee J. (2012). Effect of Three-Dimensional Robot-Assisted Therapy on Upper Limb Function of Patients with Stroke. |
41 | Hesse S. , Schulte-Tigges G. , Konrad M. , Bardeleben A. , & Werner C. ((2003) ). Robot-assisted arm trainer for the passive andactive practice of bilateral forearm and wrist movements inhemiparetic subjects. Archives of Physical Medicine and Rehabilitation 84: (6), 915–20. |
42 | Hesse S. , Werner C. , Pohl M. , Rueckriem S. , Mehrholz J. , & Lingnau M.L. ((2005) ). Computerized arm training improves the motorcontrol of the severely affected arm after stroke: A single-blinded randomized trial in two centers. Stroke 36: (9), 1960–6. |
43 | Hesse S. , Heß A. , Werner C.C. , Kabbert N. , & Buschfort R. ((2014) ). Effect on arm function and cost of robot-assisted grouptherapy in subacute patients with stroke and a moderately toseverely affected arm: A randomized controlled trial. Clinical Rehabilitation, 28: (7) 637–47. |
44 | Housman S.J. , Scott K.M. , & Reinkensmeyer D.J. ((2009) ). Arandomized controlled trial of gravity-supported, computer-enhancedarm exercise for individuals with severe hemiparesis. Neurorehabilitation and Neural Repair 23: (5), 505–14. |
45 | Houwink A. , Nijland R.H. , Geurts A.C. , & Kwakkel G. ((2013) ). Functional recovery of the paretic upper limb after stroke: whoregains hand capacity? Arch Phys Med Rehabil [Internet]. [cited 2022 Mar 17]; 94: (5), 839–44. Available from: https://pubmed.ncbi.nlm.nih.gov/23201317/ |
46 | Horsley S. , Lannin N.A. , Hayward K.S. , & Herbert R.D. ((2019) ). Additional early active repetitive motor training did not prevent contracture in adults receiving task-specific upper limb training after stroke: a randomised trial. Journal of Physiotherapy 65: (2), 88–94. |
47 | Hsieh Y.W. , Liing R.J. , Lin K.C. , Wu C.Y. , Liou T.H. , Lin J.C. , et al. ((2016) ). Sequencing bilateral robot-assisted arm therapy and constraint-induced therapy improves reach to press and trunkkinematics in patients with stroke. Journal of Neuro Engineering and Rehabilitation 13: (1). |
48 | Hsieh Y.W. , Wu C.Y. , Liao W.W. , Lin K.C. , Wu K.Y. , & Lee C.Y. ((2011) ). Effects of treatment intensity in upper limb robot-assisted therapy for chronic stroke: A pilot randomized controlled trial. Neurorehabilitation and Neural Repair 25: (6), 503–11. |
49 | Hsu H.Y. , Chiu H.Y. , Kuan T.S. , Tsai C.L. , Su F.C. , & Kuo L.C. ((2019) ). Robotic-assisted therapy with bilateral practice improves task and motor performance in the upper extremities of chronic stroke patients: A randomised controlled trial. Australian Occupational Therapy Journal 66: (5), 637–47. |
50 | Huang Y. , Nam C. , Li W. , Rong W. , Xie Y. , Liu Y. , et al. ((2020) ) A comparison of the rehabilitation effectiveness ofneuromuscular electrical stimulation robotic hand training and purerobotic hand training after stroke: A randomized controlled trial. Biomedical Signal Processing and Control 1: , 56. |
51 | Huang V.S. , & Krakauer J.W. ((2009) ). Robotic neurorehabilitation:a computational motor learning perspective. J Neuroeng Rehabil [Internet]. [cited 2022 May 30];6: (1). Available from: https://pubmed.ncbi.nlm.nih.gov/19243614/ |
52 | Hung C.S. , Hsieh Y.W. , Wu C.Y. , Lin K.C. , Lin J.C. , & Yeh L.M. , et al. ((2019) ). Comparative Assessment of Two Robot-AssistedTherapies for the Upper Extremity in PeopleWith Chronic Stroke. American Journal of Occupational Therapy 73: (1). |
53 | Hung Shan C , Lin Chung K , Chang Ying W , Huang Chih W , Chang Y.J. , Chen Ling C , et al. ((2019) ). Unilateral vs Bilateral Hybrid Approaches for Upper Limb Rehabilitation in Chronic Stroke: A Randomized Controlled Trial. Archives of Physical Medicine and Rehabilitation 100: (12), 2225–32. |
54 | Hung C.S. , Hsieh Y.W. , Wu C.Y. , Chen Y.J. , Lin K.C. , Chen C.L. , et al. ((2019) ). Hybrid Rehabilitation Therapies on Upper-Limb Function and Goal Attainment in Chronic Stroke. OTJROccupation, Participation and Health 39: (2), 116–23. |
55 | Hwang C.H. , Seong J.W. , & Son D.S. ((2012) ). Individual finger synchronized robot-assisted hand rehabilitation in subacute tochronic stroke: A prospective randomized clinical trial of efficacy. Clinical Rehabilitation 26: (8), 696–704. |
56 | Iwamoto Y. , Imura T. , Suzukawa T. , Fukuyama H. , Ishii T. , Taki . , et al. ((2019) ). Combination of Exoskeletal Upper Limb Robot and Occupational Therapy Improve Activities of Daily Living Function inAcute Stroke Patients. Journal of Stroke and Cerebrovascular Diseases 28: (7), 2018–25. |
57 | Jiang S. , You H. , Zhao W. , & Zhang M. ((2021) ). Effects of short-term upper limb robot-assisted therapy on the rehabilitationof sub-acute stroke patients. Technology and Health Care 29: (2), 295–303. |
58 | Kahn L.E. , Zygman M.L. , Rymer W.Z. , & Reinkensmeyer D.J. ((2006) ). Robot-assisted reaching exercise promotes arm movementrecovery in chronic hemiparetic stroke: A randomized controlled pilot study. Journal of NeuroEngineering and Rehabilitation 21: , 3. |
59 | Kim M.S. , Kim S.H. , Noh S.E. , Bang H.J. , & Lee KM. ((2019) ). Robotic-Assisted Shoulder Rehabilitation Therapy Effectively Improved Poststroke Hemiplegic Shoulder Pain: A Randomized Controlled Trial. Archives of Physical Medicine and Rehabilitation 100: (6), 1015–22. |
60 | Klamroth-Marganska V. , Blanco J. , Campen K. , Curt A. , Dietz V. , Ettlin . , et al. ((2014) ). Three-dimensional, task-specific robottherapy of the arm after stroke: A multicentre, parallel-group randomised trial. The Lancet Neurology 13: (2), 159–66. |
61 | Krebs H.I. , Hogan N. , Aisen M.L. , & Volpe B.T. ((1998) ). Robot-aided neurorehabilitation. IEEE Trans Rehabil Eng [Internet]. [cited 2022 May 26];6: (1), 75–87. Available from: https://pubmed.ncbi.nlm.nih.gov/9535526/ |
62 | Kutner N.G. , Zhang R. , Butler A.J. , Wolf S.L. , & Alberts J.L. ((2010) ). Quality-of-life change associated with robotic-assisted therapy to improve hand motor function in patients with subacutestroke: A randomized clinical trial. Physical Therapy 90: (4), 493–504. |
63 | Kwakkel G. , & Kollen B.J. ((2013) ). Predicting activities afterstroke: what is clinically relevant? Int J Stroke [Internet]. [cited2022 Mar 17];8: (1), 25–32. Available from: https://pubmed.ncbi.nlm.nih.gov/23280266/ |
64 | Lamb F. , Anderson J. , Saling M. , & Dewey H. ((2013) ). Predictors of subjective cognitive complaint in postacute older adult stroke patients. Arch Phys Med Rehabil [Internet]. [cited 2022 Mar17];94: (9), 1747–52. Available from: https://pubmed.ncbi.nlm.nih.gov/23529143/ |
65 | Lee K.W. , Kim S.B. , Lee J.H. , Lee S.J. , & Yoo S.W. ((2016) ). Effect of upper extremity robot-assisted exercise on spasticity instroke patients. Annals of Rehabilitation Medicine 40: (6), 961–71. |
66 | Lee K.W. , Kim S.B. , Lee J.W. , Lee S.J. , & Kim I.W. ((2017) ). Effect of robot-assisted game training on upper extremity functionin stroke patients. Annals of Rehabilitation Medicine 41: (4), 539–46. |
67 | Lee M.J. , Lee J.K. , & Lee S.M. ((2018) ). Effects of robot-Assisted therapy on upper extremity function andactivities of daily living in hemiplegic patients: A single-blinded, randomized, controlled trial. Technology and Health Care 26: (4), 659–66. |
68 | Lee S.H. , Park G. , Cho D.Y. , Kim H.Y. , Lee J.Y. , Kim S. , et al. ((2020) ). Comparisons between end-effector and exoskeleton rehabilitation robots regarding upper extremity function among chronic stroke patients with moderate-to-severe upper limb impairment. Scientific Reports 10: (1). |
69 | Lee H.C. , Kuo F.L. , Lin Y.N. , Liou T.H. , Lin J.C. , & Huang S.W. ((2021) ). Effects of Robot-Assisted Rehabilitation on Hand Function of People With Stroke: A Randomized, Crossover-Controlled, Assessor-Blinded Study. Am J Occup Ther. [Internet]. [cited 2022 May 31];75: (1). Available from: https://pubmed.ncbi.nlm.nih.gov/33399050/ |
70 | Liao W.W. , Wu C.Y. , Hsieh Y.W. , Lin K.C. , & Chang W.Y. ((2012) ). Effects of robot-assisted upper limb rehabilitation on daily function and real-world arm activity in patients with chronicstroke: A randomized controlled trial. Clinical Rehabilitation 26: (2), 111–20. |
71 | Lo A.C. , Guarino P.D. , Richards L.G. , Haselkorn J.K. , Wittenberg G.F. , Federman D.G. , et al. ((2010) ). Robot-Assisted Therapy for Long-Term Upper-Limb Impairment after Stroke. New England Journal of Medicine 362: (19), 1772–83. |
72 | Lum P.S. , Burgar C.G. , van der Loos M. , Shor P.C. , Majmundar M. , & Yap R. ((2006) ). MIME robotic device for upper-limb neurorehabilitation in subacute stroke subjects: A follow-up study. Journal of Rehabilitation Research and Development 43: (5), 631–42. |
73 | Lum P.S. , Burgar C.G. , Shor P.C. , Majmundar M. , & van der Loos M. ((2002) ). Robot-assisted movement training compared with conventional therapy techniques for the rehabilitation of upper-limbmotor function after stroke. Archives of Physical Medicine and Rehabilitation 83: (7), 952–9. |
74 | Masiero S. , Celia A. , Rosati G. , & Armani M. ((2007) ). Robotic-Assisted Rehabilitation of the Upper Limb After Acute Stroke. Archives of Physical Medicine and Rehabilitation 88: (2), 142–9. |
75 | Masiero S. , Armani M. , Ferlini G. , Rosati G. , & Rossi A. ((2014) ). Randomized trial of a robotic assistive device for the upperextremity during early inpatient stroke rehabilitation. Neurorehabilitation and Neural Repair 28: (4), 377–86. |
76 | Marchal-Crespo L. , McHughen S. , Cramer S.C. , & Reinkensmeyer D.J. ((2010) ). The effect of haptic guidance, aging, and initial skilllevel on motor learning of a steering task. Exp Brain Res [Internet]. [cited 2022 Mar 17];201: (2), 209–20. Available from: https://pubmed.ncbi.nlm.nih.gov/19820920/ |
77 | Mazzoleni S. , Tran V.do. , Dario P. , & Posteraro F. ((2019) ). Effects of Transcranial Direct Current Stimulation (tDCS) CombinedWith Wrist Robot-Assisted Rehabilitation on Motor Recovery in Subacute Stroke Patients: A Randomized Controlled Trial. IEEETransactions on Neural Systems and Rehabilitation Engineering 27: (7), 1458–66. |
78 | McCabe J. , Monkiewicz M. , Holcomb J. , Pundik S. , & Daly J.J. ((2015) ). Comparison of robotics, functional electrical stimulation,and motor learning methods for treatment of persistent upper extremity dysfunction after stroke: A randomized controlled trial. Archives of Physical Medicine and Rehabilitation 96: (6), 981–90. |
79 | Metzger J.C. , Lambercy O. , Califfi A. , Conti F.M. , & Gassert R. ((2014) ). Neurocognitive robot-assisted therapy of hand function. IEEE Trans Haptics [Internet]. [cited 2022 Mar17];7: (2), 140–9. Available from: https://pubmed.ncbi.nlm.nih.gov/24968378/ |
80 | Metzger J.C. , Lambercy O. , Califfi A. , Dinacci D. , Petrillo C. , Rossi P. , et al. ((2014) ). Assessment-driven selection and adaptationof exercise difficulty in robot-assisted therapy: a pilot study witha hand rehabilitation robot. J Neuroeng Rehabil [Internet].[cited 2022 Mar 17];11: (1). Available from: https://pubmed.ncbi.nlm.nih.gov/25399249/ |
81 | Mehrholz J. , Pollock A. , Pohl M. , Kugler J. , & Elsner B. ((2020) ). Systematic review with network meta-analysis of randomizedcontrolled trials of robotic-assisted arm training for improving activities of daily living and upper limb function after stroke. J Neuroeng Rehabil [Internet]. [cited 2022 Mar 17];17: (1) .Available from: https://pubmed.ncbi.nlm.nih.gov/32605587/ |
82 | Morone G. , Palomba A. , Martino Cinnera A. , Agostini M. , Aprile I. , Arienti C. , et al. ((2021) ). Systematic review of guidelines toidentify recommendations for upper limb robotic rehabilitation afterstroke. Eur J Phys Rehabil Med [Internet]. [cited 2022 Mar17];57: (2), 238–45. Available from: https://pubmed.ncbi.nlm.nih.gov/33491943/ |
83 | Mullick A.A. , Subramanian S.K. , & Levin M.F. ((2015) ). Emerging evidence of the association between cognitive deficits and arm motor recovery after stroke: A meta-analysis. Restor Neurol Neurosci . [Internet]. [cited 2022 Mar 17];33: (3), 389–403. Available from: https://pubmed.ncbi.nlm.nih.gov/26410581/ |
84 | Nys G.M.S. , van Zandvoort M.J.E. , de Kort P.L.M. , van der Worp H.B. , Jansen B.P.W. , Algra A. , et al. ((2005) ). The prognostic valueof domain-specific cognitive abilities in acute first-ever stroke. Neurology [Internet]. [cited 2022 Mar 17];64: (5),821–7. Available from: https://pubmed.ncbi.nlm.nih.gov/15753416/ |
85 | Orihuela-Espina F. , Roldán G.F. , Sánchez-Villavicencio I. , Palafox L. , Leder R. , Sucar L.E. , et al. ((2016) ). Robottraining for hand motor recovery in subacute stroke patients: Arandomized controlled trial. Journal of Hand Therapy 29: (1), 51–7. |
86 | Page M.J. , McKenzie J.E. , Bossuyt P.M. , Boutron I. , Hoffmann T.C. , Mulrow C.. , et al. ((2021) ). The PRISMA 2020 statement: An updated guideline for reporting systematic reviews. PLOS Medicine [Internet]. [cited 2022 May 31];18: (3), e1003583. Available from: https://journals.plos.org/plosmedicine/article?id=10.1371/journal.pmed.1003583 |
87 | Park J.H. , & Kwon Y.C. ((1989) ). Standardization of Korean versionof the Mini-Mental State Examination (MMSE-K) for use in theelderly. Part II. Diagnostic validity, Journal of Korean Neuropsychiatric Association [Internet]. [cited 2022 May31];28: (3), 508–13. Available from: https://ci.nii.ac.jp/naid/10030766381/ |
88 | Park J.H. ((2021) ). The effects of robot-assisted left-hand trainingon hemispatial neglect in older patients with chronic stroke: Apilot and randomized controlled trial. Medicine 100: (9), e24781. |
89 | Park J.H. , Park G. , Kim H.Y. , Lee J.Y. , Ham Y. , Hwang . , et al. ((2020) ). A comparison of the effects and usability of twoexoskeletal robots with and without robotic actuation for upperextremity rehabilitation among patients with stroke: asingle-blinded randomised controlled pilot study. Journal of NeuroEngineering and Rehabilitation 17: (1). |
90 | Perini G. , Bertoni R. , Thorsen R. , Carpinella I. , Lencioni T. , Ferrarin M. , et al. ((2021) ). Sequentially applied myoelectrically controlled FES in a task-oriented approach and robotic therapy forthe recovery of upper limb in post-stroke patients: A randomized controlled pilot study. Technology and Health Care 29: (3), 419–29. |
91 | Pollock A. , Farmer S.E. , Brady M.C. , Langhorne P. , Mead G.E. , Mehrholz J. , et al. ((2014) ). Interventions for improving upper limb function after stroke. Cochrane Database Syst Rev [Internet].[cited 2022 Mar 17];2014: (11). Available from: https://pubmed.ncbi.nlm.nih.gov/25387001/ |
92 | Qian Q. , Hu X. , Lai Q. , Ng S.C. , Zheng Y. , Poon W. ((2017) ). Early stroke rehabilitation of the upper limb assisted with anelectromyography-driven neuromuscular electrical stimulation-roboticarm. Frontiers in Neurology 8: (SEP). |
93 | Qian Q. , Nam C. , Guo Z. , Huang Y. , Hu X. , Ng S. , et al. ((2019) ). Distal versus proximal –An investigation on different supportive strategies by robots for upper limb rehabilitation afterstroke: A randomized controlled trial. Journal of NeuroEngineering and Rehabilitation 16: (1). |
94 | Rabadi M.H. , Galgano M. , Lynch D. , Akerman M. , Lesser M. , & Volpe B.T. ((2008) ). A pilot study of activity-based therapy in thearm motor recovery post stroke: A randomized controlled trial. Clinical Rehabilitation 22: (12), 1071–82. |
95 | Ranzani R. , Lambercy O. , Metzger J.C. , Califfi A. , Regazzi S. , Dinacci D. , et al. ((2020) ). Neurocognitive robot-assisted rehabilitation of hand function: A randomized control trial on motor recovery in subacute stroke. Journal of NeuroEngineering and Rehabilitation 17: (1). |
96 | Reinhart S. , Schmidt L. , Kuhn C. , Rosenthal A. , Schenk T. , Keller . , et al. ((2012) ). Limb activation ameliorates body-relateddeficits in spatial neglect. Frontiers in Human Neuroscience 0: (JUNE 2012), 188. |
97 | Riener R. , Lünenburger L. , & Colombo G. ((2006) ). Human-centered robotics applied to gait training and assessment. J Rehabil Res Dev [Internet]. [cited 2022 Mar17];43: (5), 679–93. Available from: https://pubmed.ncbi.nlm.nih.gov/17123208/ |
98 | Rodgers H. , Bosomworth H. , Krebs H.I. , van Wijck F. , Howel D. , Wilson . , et al. ((2019) ). Robot assisted training for the upper limbafter stroke (RATULS): a multicentre randomised controlled trial. Lancet [Internet]. [cited 2022 Mar 17];394: (10192),51–62. Available from: https://pubmed.ncbi.nlm.nih.gov/31128926/ |
99 | Rodgers H. , Bosomworth H. , Krebs H.I. , van Wijck F. , Howel D. , Wilson . , et al. ((2019) ). Robot assisted training for the upper limbafter stroke (RATULS): a multicentre randomised controlled trial. The Lancet 394: (10192), 51–62. |
100 | Rosenthal O. , Wing A.M. , Wyatt J.L. , Punt D. , Brownless B. , Ko-Ko . , et al. ((2019) ). Boosting robot-assisted rehabilitation ofstroke hemiparesis by individualized selection of upper limb movements –A pilot study. Journal of NeuroEngineering and Rehabilitation 16: (1). |
101 | Saa J.P. , Tse T. , Baum C. , Cumming T. , Josman N. , Rose . , et al. ((2019) ). Longitudinal evaluation of cognition after stroke–A systematic scoping review. PLoS ONE [Internet].[cited 2022 May 30];14: (8). Available from: /pmc/articles/PMC6715188/ |
102 | Saposnik G. , & Levin M. ((2011) ). Virtual reality in stroke rehabilitation: a meta-analysis and implications for clinicians. Stroke [Internet]. [cited 2022 Mar 17];42: (5), 1380–6. Available from: https://pubmed.ncbi.nlm.nih.gov/21474804/ |
103 | Sale P. , Mazzoleni S. , Lombardi V. , Galafate D. , Massimiani M.P. , Posteraro F. , et al. ((2014) ). Recovery of hand function withrobot-assisted therapy in acute stroke patients: Arandomized-controlled trial. International Journal of Rehabilitation Research 37: (3), 236–42. |
104 | Serrezuela R.R. , Quezada M.T. , Zayas M.H. , Pedrón A.M. , Hermosilla D.M. , & Zamora R.S. ((2020) ). Robotic therapy for the hemiplegic shoulder pain:Apilot study. Journal of NeuroEngineering and Rehabilitation 17: (1). |
105 | SPREAD. (2016). Ictus cerebrale: linee guida italiane di prevenzione e trattamento Raccomandazioni e Sintesi VIII edizione. |
106 | Sun J.H. , Tan L. , Yu J.T. ((2014) ). Post-stroke cognitive impairment: epidemiology, mechanisms and management. Ann Transl Med [Internet]. [cited 2022 Mar 17];2: (8). Available from: https://pubmed.ncbi.nlm.nih.gov/25333055/ |
107 | Straudi S. , Baroni A. , Mele S. , Craighero L. , Manfredini F. , Lamberti N. , et al. ((2020) ). Effects of a Robot-Assisted ArmTraining Plus Hand Functional Electrical Stimulation on Recovery After Stroke: A Randomized Clinical Trial. Archives of Physical Medicine and Rehabilitation 101: (2), 309–16. |
108 | Stinear C.M. , Lang C.E. , Zeiler S. , & Byblow W.D. ((2020) ). Advances and challenges in stroke rehabilitation. The Lancet Neurology 19: (4), 348–60. |
109 | Susant E.A.. , Ton R.K.Y. , Ockenfel C. , & Ho N.S.K. ((2015) ). Efficacy of robot-assisted fingers training in chronic strokesurvivors: A pilot randomized-controlled trial. Journal of NeuroEngineering and Rehabilitation 12: (1). |
110 | Summary of measurement properties of the PEDro scale –PEDro [Internet]. [cited 2022 May 31]. Available from: https://pedro.org.au/english/summary-of-measurement-properties-of-the-pedro-scale/ |
111 | Taveggia G. , Borboni A. , Salvi L. , Mulé C. , Fogliaresi S. , Villafañe J.H. , et al. ((2016) ). Efficacy of robot-assisted rehabilitation for the functional recovery of the upper limb inpost-stroke patients: a randomized controlled study. Eur J Phys Rehabil Med 52: (6), 767–73. |
112 | Taravati S. , Capaci K. , Uzumcugil H. , & Tanigor G. ((2022) ). Evaluation of an upper limb robotic rehabilitation program on motorfunctions, quality of life, cognition, and emotional status inpatients with stroke: a randomized controlled study. Neurol Sci [Internet]. [cited 2022 Mar 17];43: (2), 1177–88. Available from: https://pubmed.ncbi.nlm.nih.gov/34247295/ |
113 | Takahashi K. , Domen K. , Sakamoto T. , Toshima M. , Otaka Y. , Seto M. , et al. ((2016) ). Efficacy of Upper Extremity Robotic Therapyin Subacute Poststroke Hemiplegia: An Exploratory Randomized Trial. Stroke 47: (5), 1385–8. |
114 | Thon B. ((2015) ). Cognition and motor skill learning. Annals of Physical and Rehabilitation Medicine 58: , e25. |
115 | Timmermans A.A.A. , Lemmens R.J.M. , Monfrance M. , Geers R.P.J. , Bakx W. , Smeets R.J.E.. , et al. ((2014) ). Effects of task-oriented robot training on arm function, activity, and quality of life inchronic stroke patients: A randomized controlled trial. Journalof NeuroEngineering and Rehabilitation 11: (1). |
116 | Tomić T.J.D. , Savić A.M. , Vidaković A.S. , Rodić S.Z. , Isaković M.S. , Rodríguez-De-Pablo . , et al. ((2017) ). Arm Assist robotic system versus matched conventional therapy for poststroke upper limb rehabilitation: Arandomized clinical trial. BioMed Research International, 2017: . |
117 | Tramontano M. , Morone G. , Palomba A. , de Angelis S. , Mercuro A. , Caltagirone C. , et al. ((2020) ). Effectiveness of a sensor-based technology in upper limb motor recovery in post-acute stroke neurorehabilitation: a randomized controlled trial. J Biol Regul Homeost Agents 34: (5 Suppl. 3)(Technology in Medicine.), 165–74. |
118 | Vanoglio F. , Bernocchi P. , Mulè C. , Garofali F. , Mora C. , Taveggia G , et al. ((2017) ). Feasibility and efficacy of a robotic device for hand rehabilitation in hemiplegic stroke patients: Arandomized pilot controlled study. Clinical Rehabilitation 31: (3), 351–60. |
119 | Villafañe J.H. , Taveggia G. , Galeri S. , Bissolotti L. , Mullè C. , Imperio . , et al. ((2018) ). Efficacy of Short-Term Robot-Assisted Rehabilitation in Patients With Hand Paralysis AfterStroke: A Randomized Clinical Trial. Hand 13: (1), 95–102. |
120 | Volpe B. , Krebs H. , Hogan N. , Edelstein L. , Diels C. , & Aisen M. (2000). A novel approach to stroke rehabilitation Robotaided sensorimotor stimulation. |
121 | Wilm I.L. ((2020) ). The computerized cognitive training alliance –A proposal for a therapeutic alliance model for home-based computerized cognitive training. Heliyon [Internet]. [cited 2022 Jun 13];6: (1). Available from: https://pubmed.ncbi.nlm.nih.gov/32042977/ |
122 | Wolf S.L. , Sahu K. , Bay R.C. , Buchanan S. , Reiss A. , Linder S. , et al. ((2015) ). The HAAPI (Home Arm Assistance Progression Initiative) Trial: A Novel Robotics Delivery Approach in Stroke Rehabilitation. Neurorehabilitation and Neural Repair 29: (10), 958–68. |
123 | Wu C.Y. , Yang C.L. , Chuang L.L. , Lin K.C. , Chen H.C. , Chen M.D. , et al. (2012). Effect of Therapist-Based Versus Robot-Assisted Bilateral Arm Training on Motor Control, Functional Performance, and Quality of Life After Chronic Stroke: A Clinical Trial [Internet]. Available from: https://oup.com/ptj/article/92/8/1006/2735271 |
124 | Xing Y. , & Bai Y. ((2020) ). A Review of Exercise-Induced Neuroplasticity in Ischemic Stroke: Pathology and Mechanisms. Mol Neurobiol [Internet]. [cited 2022 Mar 17];57: (10),4218–31. Available from: https://pubmed.ncbi.nlm.nih.gov/32691303/ |
125 | Xu Q. , Li C. , Pan Y. , Li W. , Jia T. , Li . , et al. ((2020) ). Impact of smart force feedback rehabilitation robot training onupper limb motor function in the subacute stage of stroke. NeuroRehabilitation 47: (2), 209–15. |
126 | Zengin-Metli . , Ozbudak-Demir . , Eraktas . , Binay-Safer . , & Ekiz T. ((2018) ) Effects of robot assistive upper extremity rehabilitation on motor and cognitive recovery, the quality of life, and activities of daily living in stroke patients. Journal of Back and Musculoskeletal Rehabilitation 31: (6), 1059–64. |