Predictors of fatigue self-management behaviors in adults with multiple sclerosis
Abstract
BACKGROUND:
Fatigue is one of the most common and disabling symptoms in people with multiple sclerosis (MS). Fatigue self-management behaviors may be effective in reducing the impact of fatigue in people with MS. However, few studies have examined the factors that influence engagement in fatigue self-management behaviors.
OBJECTIVE:
Identify factors that directly and indirectly influence fatigue self-management behaviors.
METHODS:
Participants with MS (n = 287) completed online questionnaires at baseline and 6-weeks. Guided by the Self- and Family Management Framework, we examined the influence of health status, resources and environment, healthcare utilization, and self-management processes on fatigue self-management behaviors at 6-weeks. Multiple regression and path analyses were conducted.
RESULTS:
The final regression model variables accounted for 41.58% of the variance in fatigue self-management behaviors, which included outcome expectations (β= 0.287), disability (β= 0.265), environmental barriers (β= 0.188), self-efficacy (β= 0.153), symptom severity (β= 0.113), living in an urban community (β= –0.108), and living alone (β= 0.103). Path analysis indicated that outcome expectations may mediate the relationship between disability levels and fatigue self-management behavior.
CONCLUSIONS:
Health status (i.e., disability and symptom severity), environmental factors (e.g., living situation), and self-management processes (i.e., self-efficacy and outcome expectations) may play an important role in influencing engagement in fatigue self-management behaviors.
1Introduction
Multiple sclerosis (MS) is an immune-mediated neurologic disorder affecting the brain and spinal cord (Thompson et al., 2018). MS is one of the leading causes of non-traumatic disability in young adults (Kobelt et al., 2017). Fatigue is a common disabling symptom of MS (Vucic et al., 2010). MS fatigue is defined as a persistent lack of physical and/or mental energy that interferes with usual and desired activities (Penner & Paul, 2017). The consequences of MS fatigue can create widespread lifestyle challenges, including decreased ability to maintain employment, restricted participation in leisure activities, and disruption of interpersonal relationships (Fisk et al., 1994; Skerrett & Moss-Morris, 2006; Smith & Arnett, 2005).
MS fatigue’s multifactorial etiology remains elusive. A complex array of biological, behavioral, and environmental factors is believed to cause fatigue (Krupp, 2006; Vucic et al., 2010). Fatigue self-management interventions may be effective for some people with MS because of the learning of compensatory behaviors that are relevant regardless of the underlying causes of fatigue (Asano & Finlayson, 2014; Plow et al., 2011). Self-management refers to a set of daily behaviors individuals perform to manage a chronic illness and associated symptoms (Lorig & Holman, 2003). Fatigue self-management behaviors can include pacing activities, taking periodic rest breaks, engaging in physical activity, making environmental modifications, and delegating tasks to one’s social support network. Research shows that the effectiveness of fatigue self-management interventions may be influenced by how frequently participants engage in fatigue self-management behaviors (Mathiowetz et al., 2001; Vanage et al., 2003). Thus, it is important to understand factors that influence engagement in fatigue self-management behaviors. Few studies to date have attempted to identify factors that influence fatigue self-management behaviors in people with MS.
The Self- and Family Management Framework may be useful in identifying factors that influence engagement in fatigue self-management behaviors. This model conceptualizes self-management as a process influenced by factors impacting an individual’s motivation and ability to self-manage symptoms (Grey et al., 2006). These factors can function as facilitators and barriers, which are delineated into five categories: (1) Personal/Lifestyle Characteristics, (2) Health Status, (3) Resources, (4) Environment, and (5) Healthcare System (Grey et al., 2015). These facilitators and barriers influence self-management processes, which include knowledge, beliefs, and skills that are used to engage in self-management behaviors (Schulman-Green et al., 2012). Outcome expectations and self-efficacy are posited to be important self-management processes that exert influence on motivation and ability to engage in self-management behaviors (Ryan & Sawin, 2009). According to the Self- and Family Management Framework, facilitators and barriers can indirectly influence fatigue self-management behaviors through self-management processes, such as self-efficacy and outcome expectations.
The purpose of this study was to identify factors that directly and indirectly influence fatigue self-management behaviors. The Self- and Family Management Framework was used to guide the selection and temporal ordering of variables included in the analyses. Specifically, we examined the following: 1) bivariate correlations between facilitators (e.g., social support and wellness services), barriers (e.g. depression and cognitive function), self-management processes (i.e., outcome expectations and self-efficacy), and fatigue self-management behaviors, 2) the relative importance of variables in directly predicting fatigue self-management behaviors, and 3) the mediating effects of self-management processes between facilitators or barriers and fatigue self-management behaviors.
2Methods
2.1Subjects and study design
A longitudinal study was conducted in 300 participants with MS who completed online questionnaires at baseline, 6 weeks, and 3 months. The analyses for this study included data from 270 participants who completed questionnaires at baseline and 6-weeks (see Fig. 1). Participants were recruited via referrals from physicians’ practices, advertisements in newsletters by the National MS Society, and in-person fundraising events and informational seminars. Individuals interested in participating in the study called or emailed the research office. All screening procedures occurred over the phone. Individuals who completed the consent process over the phone and returned the signed informed consent were enrolled in the study.
Fig. 1
Flowchart of participant recruitment.
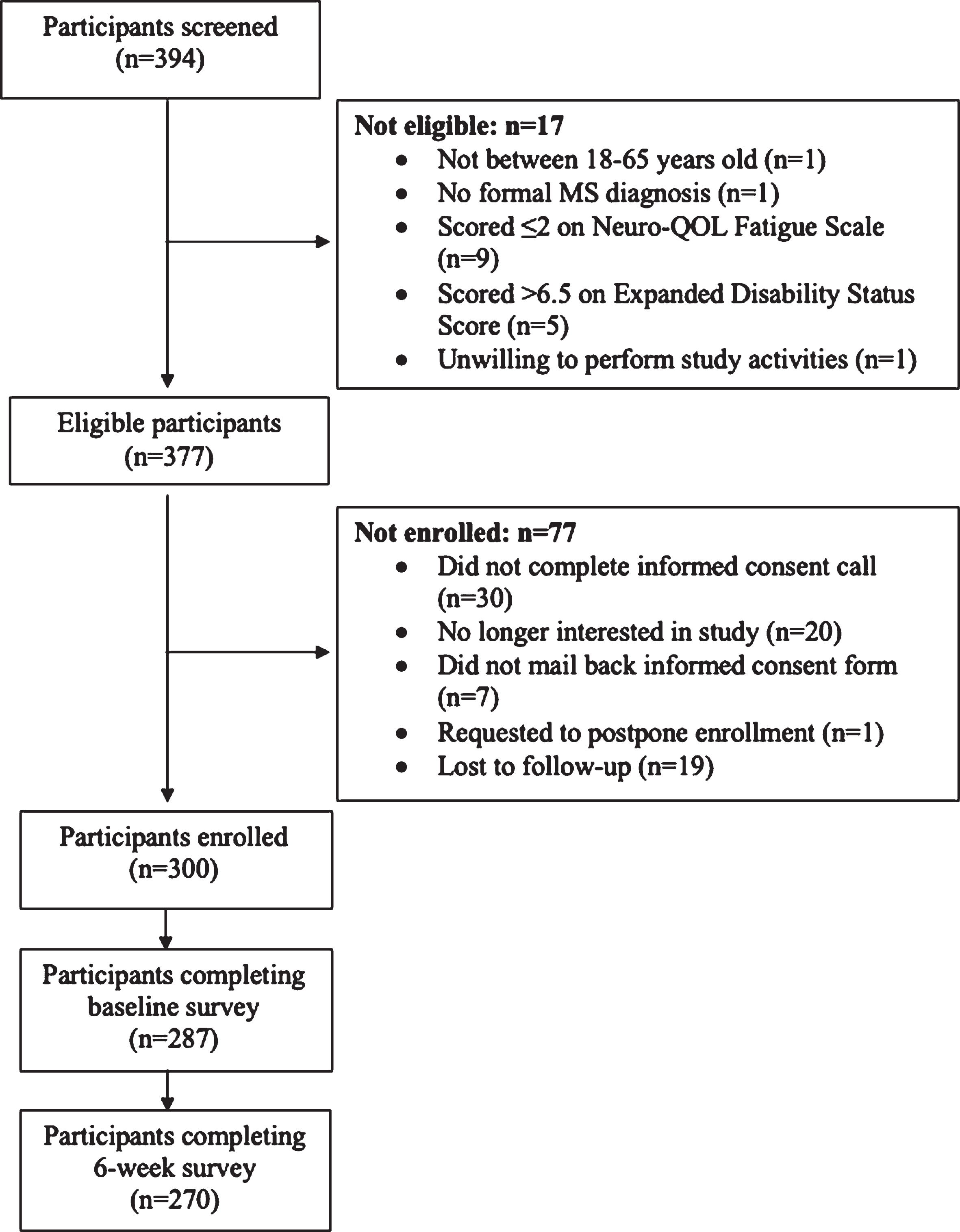
Those eligible for the study included adults between 18 and 65 years of age, a self-report diagnosis of MS, and had at least mild MS fatigue (> 2 on Neuro-QOL Fatigue Scale (Cella et al., 2012)). Individuals who had a self-report Expanded Disability Status Scale (Kurtzke, 1983) score > 6.5 (reflecting the inability to walk more than 5 meters without aid) and severe cognitive deficits (< 12 on the short Orientation-Memory-Concentration Test (Katzman et al., 1983)) were excluded. University Hospitals Cleveland Medical Center’s Institutional Review Board and Department of Defense’s Human Research Protection Office review board approved this study.
2.2Measures
Participants completed online recall-based questionnaires on a computer, tablet, or smartphone, taking about 30 to 45 minutes to complete. Participants were emailed a unique Qualtrics survey link at baseline and were asked to complete the same questionnaires at 6 weeks. The survey collected data on demographics, self-reported health status, lifestyle and environmental factors, and utilization of healthcare services. The Self- and Family Management Framework was used to guide the selection of variables included in the analyses. This model was selected because is it supported by empirical data, and it depicts multi-level factors that can influence self-management behaviors (Schulman-Green et al., 2016). The descriptions of the questionnaires below and variables used in the analyses are organized into the five facilitators and barriers categories depicted in the Self- and Family Management Framework (see Fig. 2): (1) Personal/Lifestyle Characteristics, (2) Health Status, (3-4) Resources and Environment, and (5) Healthcare System. Cronbach’s alpha (α) and test-retest reliability (intraclass correlation coefficient, ICC) statistics reported below are derived from the baseline and 6 week data in this study.
Fig. 2
Model and variable selection based on the Self- and Family Self-Management Model (Grey et al., 2006). Note. PDDS: Patient Disease Determinant Steps; BMI: Body mass index; MSNQ: MS Neuropsychological Screening Questionnaire; CHIEF-SF: Craig Hospital Inventory of Environmental Factors–Short Form.
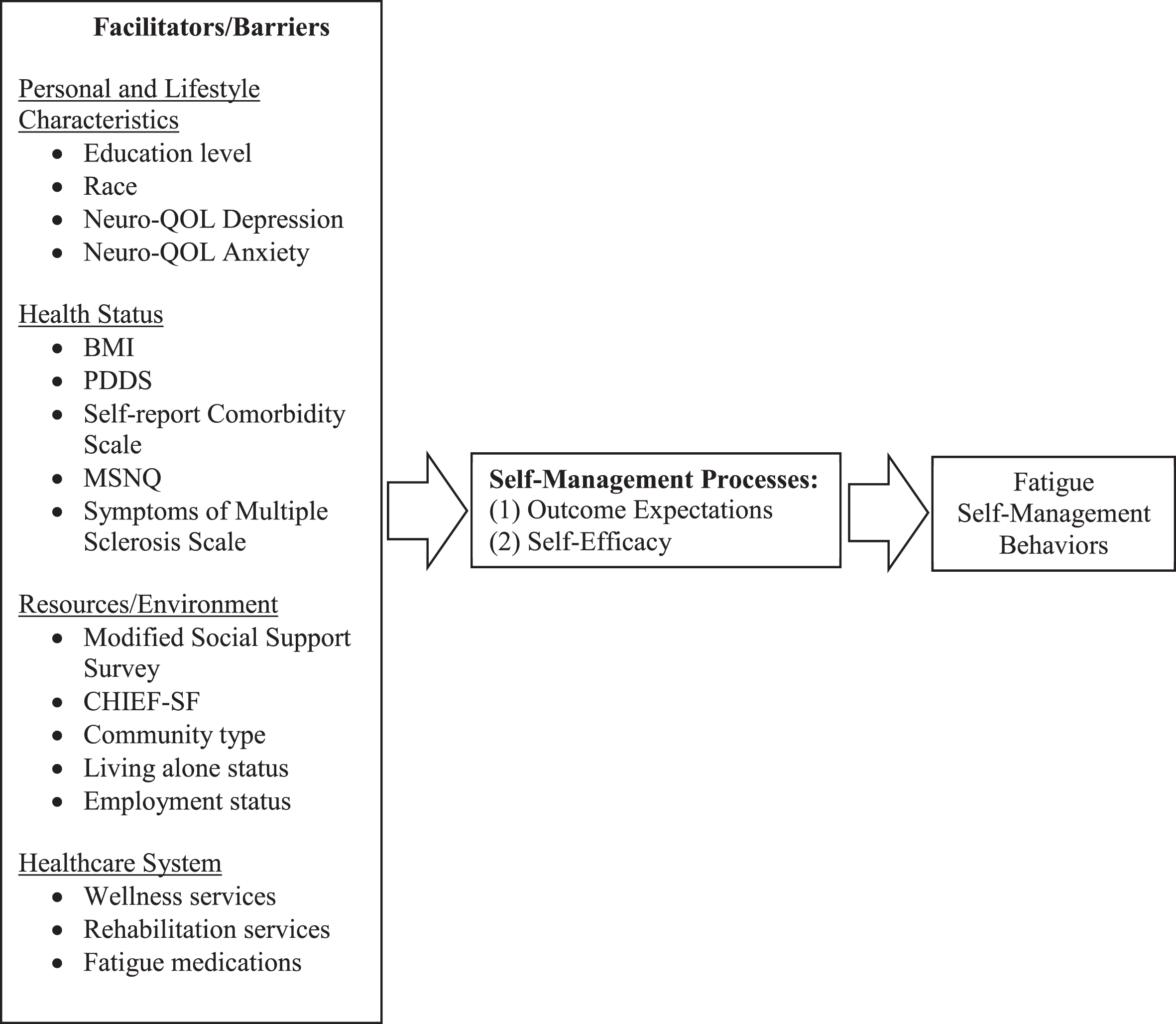
2.2.1Fatigue self-management behavior
The Energy Conservation Strategy Survey (Mallik et al., 2005) was the dependent variable used in the analyses. It measures the frequency of engaging in 14 different fatigue self-management behaviors on a three-point scale. The fatigue self-management behaviors included taking rest breaks, using adaptive equipment, positioning the body while doing an activity, asking for help, and eliminating part or all of an activity. Response options were “never tried this strategy,” “tried it, but I don’t use it regularly,” and “currently using the strategy on a regular basis” (α= 0.92; ICC = 0.86).
2.2.2Personal and lifestyle characteristics
Personal and lifestyle characteristics emphasize the importance of knowledge, culture, and psychological and emotional factors on influencing self-management behaviors. If there is a lack of congruence between self-management behaviors and cultural practices, cultural backgrounds and beliefs may serve as a barrier to self-management. Emotions may promote or discourage self-management behaviors. Depression may decrease motivation to engage in self-management behaviors, whereas anxiety may facilitate self-management due to increased symptom monitoring (Schulman-Green et al., 2016).
Information on education as well as race and ethnicity were collected. Participants were asked to indicate their highest level of education completed. Responses ranged from “Less than 7th grade” to “PhD or Equivalent”. Response options for race and ethnicity were Hispanic or Non-Hispanic and “white”, “African-American or Black”, “American Indian or Alaskan Native”, “Native Hawaiian or Pacific Islander”, “Asian or Asian Indian”, “Arab”, or “Multiracial”.
Depression and anxiety were evaluated using Neuro-QOL (Miller et al., 2016). The 8-item depression short form Neuro-QOL Depression assesses for feelings of hopelessness, negative affect (e.g., sadness, guilt), decrease in positive affect (e.g., loss of interest), information-processing deficits (e.g., problems in decision-making), and negative views of self (e.g., self-critic) on a four-point scale ranging from ‘never” to “always” over a period of one week (α= 0.94; ICC = 0.90). Neuro-QOL Anxiety is an 8-item form assessing for unpleasant thoughts or feelings related to fear, helplessness, worries, and hyperarousal on a four-point scale, ranging from “never” to “always” over a period of one week (α= 0.93; ICC = 0.84).
2.2.3Health status
Body Mass Index (BMI), disability, cognitive function, and symptom severity were selected as indicators of health status. The health status of an individual can interfere with self-management efforts while increasing the need to self-manage symptoms. For instance, obesity may increase the risk of developing further medical problems that involve more complex treatment plans that are more difficult to follow (Khaodhiar et al., 1999). Cognitive function may also impede self-management behavior due to decreased ability to recognize signs and symptoms, impaired problem-solving skills, or forgetting to carry out self-management tasks (Schulman-Green et al., 2016).
BMI was calculated from participants’ self-reported height and weight (ICC = 0.96). Patient-Determined Disease Steps (PDDS) (Hohol et al., 1999) is a valid and reliable measure of disability in people with MS (Hohol et al., 1999). The scoring of this survey is determined by an individual’s self-reported ability to walk, use of mobility aids, and the impact of symptoms on daily activities. It is scored on a scale from 1 to 8 ranging from minimal MS impact and normal ambulatory function with no activity limitation to a bedridden status (ICC = 0.96).
Number of co-morbid conditions was measured using the Self-Report Comorbidity Questionnaire for Multiple Sclerosis (Horton et al., 2010). This questionnaire includes 36 comorbidities that are frequently reported in general and the population with MS (e.g. diabetes, hypertension, rheumatoid arthritis, depression). Participants were asked “has a doctor ever told you that you have. . . ?”. For each comorbidity, the participant indicated the presence or absence of the condition (ICC = 0.93).
Cognitive function was measured with the MS Neuropsychological Screening Questionnaire (MSNQ) (Benedict et al., 2004). This 15-item self-report questionnaire reflects neuropsychological competence with activities of daily living (i.e. “Do you lose your thoughts while listening to someone speak?” “Are you slow when trying to solve problems?”) and is strongly correlated with low cognitive performance. MSNQ is scored on a four-point scale indicating frequency and degree of disruption, from “never, does not occur” to “very often, very disruptive” (α= 0.95; ICC = 0.95).
The Symptoms of Multiple Sclerosis Scale (McMillan & Moore, 2006) measured the degree to which individuals experience common MS symptoms such as fatigue, pain, visual impairments, paralysis, bladder difficulties, lack of concentration, inability to communicate, bowel difficulties, numbness, tremors, loss of balance, and spasticity on a 5-point scale, ranging from “never” to “always” (α= 0.78; ICC = 0.93).
2.2.4Resources and environment
The Self- and Family Management Framework designates resources and environment as two independent categories of facilitators and barriers (Grey et al., 2015). For our purposes, we have combined resources and environment into single category as they are derived from home, work, and community factors. Living with other members at home can support or deter self-management efforts, either by enhancing self-management tasks or creating competing demands posed by health problems of other family members. The work environment may pose schedule and time constraints interfering with an individual’s ability to take medication or follow prescribed dietary and exercise regimens. Limited accessibility adaptations, social stigma, and lack of public awareness of chronic illnesses can function as barriers to self-management (Schulman-Green et al., 2016). An individual’s residing community can exert influence on one’s ability to self-manage their condition. For instance, rural communities may face barriers such as limited transportation access and increased physical or emotional isolation (Sav et al., 2015), which may discourage performance of self-management tasks.
Social support, environmental barriers, community type, living alone status, and employment status were selected as indicators of resources and the environment. The Modified Social Support Survey (Sherbourne & Stewart, 1991) is a 5-item, abbreviated version of the Social Support Survey, developed as part of the Medical Outcomes Study to assess different domains of perceived social support. Participants responded to the frequency at which another person was available to support them in a particular social situation. The social support domains included tangible support (“how often is someone available to take you to the doctor if you need to go?”), emotional support (“. . . to understand your problems?”), affectionate support (“. . . to hug you?), and positive social interaction (“. . . to have a good time with?”) (α= 0.88; ICC = 0.87).
The Craig Hospital Inventory of Environmental Factors–Short Form (CHIEF-SF) (Whiteneck et al., 2004) is used to measure perceived barriers in the physical and social environment. Participants rate how often they experience the environmental barrier in the past 12 months and whether it has been a big or small problem. Questions include: “how often has the ability of transportation been a problem. . . ”, “how often has the natural environment, temperature, terrain, climate made it difficult to do what you want. . . ”, and “how often have other people’s attitudes toward you been a problem at home. . . school or work?” (ICC = 0.85).
Community type, living alone status, and employment status were evaluated in the demographics survey. Participants indicated if their living environment was “rural”, “suburban”, or “urban”. Participants indicated their living situation at home by answering “do you live alone?” with “yes” or “no”. Participants answered if they were currently retired, unemployment, working part-time (< 40 hours per week) or working full-time (≥40 hours per week).
2.2.5Healthcare system
The healthcare system functions as an important component to support self-management behavior by reflecting access to care, one’s ability to navigate the healthcare system, and gain knowledge on effective self-management strategies (Schulman-Green et al., 2016). Indicators of the healthcare system were utilization of wellness services, rehabilitation services, and prescribed fatigue medications. Participants indicated any active engagement in community-based programs that focus on prompting healthy behaviors (e.g. nutrition counseling, educational programs, physical activity classes or recreational and leisure programs), responded if they were currently receiving any rehabilitation services (e.g. physical or occupational therapy), and were asked whether they were taking any prescribed medications for treating fatigue.
2.2.6Self-management processes
Outcome expectations and self-efficacy were selected from the Social Cognitive Theory, which function as one of the behavioral models underlying the Self-and Family Management Framework. Outcome expectations represent a person’s prediction of how likely a particular behavior or action will help attain a desired outcome (Constantino, 2012). When individuals perceive positive consequences of self-management, they expend more effort on self-management (Schulman-Green et al., 2016). The Energy Conservation Strategy Survey (Mallik et al., 2005) was used to measure outcome expectations. After each question on the frequency of engaging in a fatigue self-management behavior, participants rated how likely each fatigue self-management behavior would be in managing their fatigue on a 10-point scale ranging from “not effective” to “very effective” (α= 0.83; ICC = 0.90).
Self-efficacy refers to one’s personal judgment of their ability to successfully execute a behavior (Bandura, 1977; Lorig & Holman, 2003; Plow et al., 2015). The Self-Efficacy for Performing Energy Conservation Strategies (Liepold & Mathiowetz, 2005) is a 14-item survey assessing one’s confidence in the ability to engage in fatigue self-management behaviors. It is measured on a 10-point scale ranging from “not at all confident” to “highly confident.” For example, participants answered “how confident are you that you can delegate part or all of an activity to manage your fatigue?” (α= 0.91; ICC = 0.83).
2.3Analyses
Statistical analyses were conducted with SPSS version 26. Assumptions for the statistical analyses were examined and met, including normality, linearity, and non-multicollinearity. The influence of potential outliers and missing data were also examined and deemed not to have a substantial influence on results. Participants with missing data were excluded from the analyses (approximately 20%). Bivariate Pearson’s and Spearman’s rho correlations were used to examine the associations among facilitators, barriers, self-management processes, and fatigue self-management behaviors. A multiple linear regression model was used to examine the relative importance of variables in predicting fatigue self-management behaviors. Variable selection was performed by using only significant bivariate correlates in a backwards elimination method. The criteria to include and remove variables was set at a p-value of 0.05 and 0.10, respectively. The PROCESS Macro (Hayes, 2018) was used to conduct a multiple mediator path analysis. The variables tested for mediation were self-efficacy and outcome expectations. The dependent variable was fatigue self-management behaviors. Independent variables were those included in the final multiple linear regression model. A separate multiple mediator model that simultaneously included both self-efficacy and outcome expectations was developed for each of the independent variables. The mediation analyses consisted of calculating beta coefficients for the effect of facilitators and barriers at baseline on the self-management processes at 6 weeks (Pathway A), the association between the self-management processes and fatigue self-management behaviors at 6 weeks (Pathway B), and effects of the total (Pathway C) and indirect (Pathway AB) pathways (see Fig. 3). Mediation was identified with bias-corrected bootstrapped 95% asymmetrical confidence intervals (CI) for the indirect effect when the CIs did not include zero (Hayes, 2018). The sample size was sufficient to detect small-to-medium effects of independent variables on fatigue self-management behaviors and medium-to-large mediation effects with a bias-corrected bootstrap (Faul et al., 2009; Fritz & Mackinnon, 2007; Schoemann et al., 2017).
Fig. 3
Hypothesized model of independent variables (facilitators and barriers) and fatigue self-management behaviors, mediated by self-management factors of outcome expectations and self-efficacy.
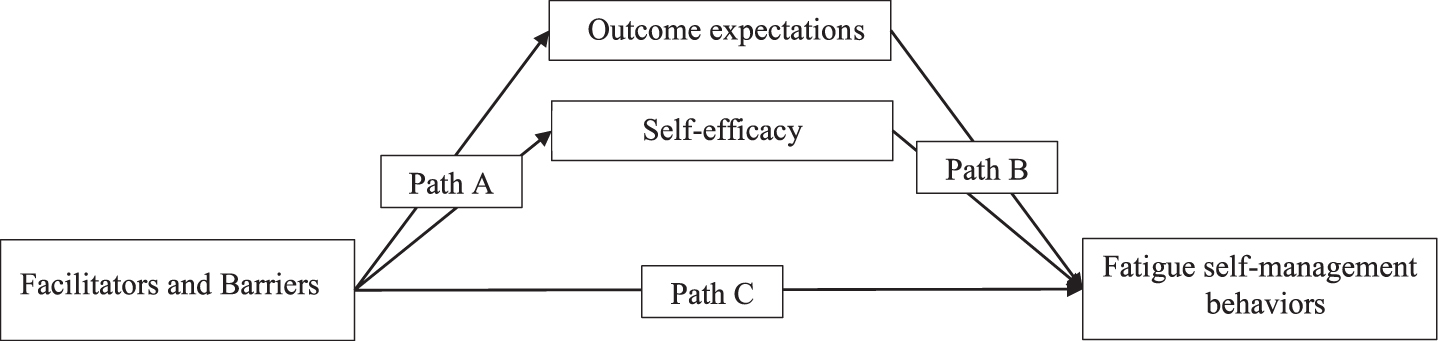
3Results
3.1Significant bivariate correlations
Table 1 shows the characteristics of the research sample as well as the correlations between the independent variables (i.e., facilitators and barriers) and fatigue self-management behaviors. Bivariate correlations indicated that MSNQ, Self-Report Comorbidity Scale, Symptoms of MS Scale, PDDS, living alone, employment status, CHIEF-SF, utilization of rehabilitation services, utilization of wellness services, outcome expectations, and self-efficacy were significantly associated with performing fatigue self-management behaviors.
Table 1
Demographic and disease characteristics
Measure | Value | Correlation with FSMB |
Description of Sample | ||
Female gender, count (%) | 247(85.7) | |
Age, Mean (SD) | 51.7 (11.4) | |
Relapsing Remitting MS, count (%) | 220 (76.2) | |
Disease duration in years, Mean (SD) | 12.8 (9.0) | |
Primary Outcome (dependent variable) | ||
Fatigue self-management behaviors#, Mean (SD) | 1.4625 (0.4) | 1.00 |
Personal and Lifestyle Characteristics | ||
Education level, count (%) | –0.109 | |
Less than high school | 4 (1.4) | |
High school graduate | 21 (7.3) | |
1–3 years of college | 68 (23.3) | |
College university/graduate | 111 (38.0) | |
Master’s degree | 62 (21.2) | |
PhD or equivalent | 22 (7.5) | |
Race (White), count (%) | 247 (85.8) | –0.092 |
Neuro-QOL Depression##, Mean (SD) | 15.3665 (7.3) | 0.014 |
Neuro-QOL Anxiety##, Mean (SD) | 19.2877 (7.3) | 0.053 |
Health Status | ||
BMI, Mean (SD) | 28.5540 (7.0) | –0.031 |
PDDS##, Mean (SD) | 3.6065 (2.1) | 0.441** |
Self-Report Comorbidity Scale##, Mean (SD) | 4.4021 (3.6) | 0.155* |
MSNQ##, Mean (SD) | 24.5737 (12.4) | 0.178** |
Symptoms of Multiple Sclerosis | 34.6899 (7.3) | 0.316** |
Scale##, Mean (SD) | ||
Resources and Environment | ||
Modified Social Support Survey#, Mean (SD) | 68.2420 (26.3) | 0.096 |
CHIEF-SF##, Mean (SD) | 1.0832 (0.9) | 0.247** |
Community living type, count (%) | –0.182** | |
Rural | 52 (18.1) | |
Suburban | 182 (63.4) | |
Urban | 53 (18.5) | |
Living alone, count (%) | 49 (17) | 0.118* |
Employment status, count (%) | –0.288** | |
Unemployed/retired | 164 (57.5) | |
Part-time | 41 (14.4) | |
Full-time | 80 (28.1) | |
Healthcare System | ||
Receiving wellness services, count (%) | 224 (79.7) | 0.125* |
Receiving rehabilitation services, count (%) | 232 (82.6) | 0.119* |
Receiving fatigue medications, count (%) | 178 (63.3) | 0.085 |
Self-Management Processes | ||
Self-efficacy#, Mean (SD) | 6.7307 (1.8) | 0.407** |
Outcome expectations#, Mean (SD) | 6.6514 (1.8) | 0.482** |
Note: # = higher score means better or healthier outcome, ## = lower score means better or healthier outcome, *p < 0.05, **p < 0.01. FSMB: Fatigue self-management behaviors; SD: Standard Deviation PDDS: Patient Disease Determinant Steps; BMI: Body mass index; MSNQ: MS Neuropsychological Screening Questionnaire; CHIEF-SF: Craig Hospital Inventory of Environmental Factors–Short Form.
Table 2 reports the backwards selection regression model to determine the relative importance of each variable in predicting engagement in fatigue self-management behaviors. The final model included outcome expectations, self-efficacy, Symptoms of MS Scale, PDDS, living alone, community living type, and CHIEF-SF. Living in an urban environment was associated with decreased engagement in fatigue self-management behaviors, whereas living alone, self-efficacy, outcome expectation, Symptoms of MS Scale, CHIEF-SF, and PDDS were associated significantly with increased engagement in fatigue self-management behaviors. Outcome expectations (β= 0.287) explained the most variance in the fatigue self-management behaviors, followed by PDDS (β= 0.265), CHIEF-SF (β= 0.188), self-efficacy (β= 0.153), Symptoms of MS scale (β= 0.113), living in an urban community (β= –0.108), and living alone (β= 0.103). The final model variables accounted for 41.58% of the variance in fatigue self-management behaviors.
Table 2
Final regression model predicting fatigue self-management behaviors at 6-weeks
Facilitator /Barrier Measure | Standardized B-coefficient | Standard error | P-value |
Living alone status | 0.103 | 0.052 | 0.035 |
Community living type | –0.108 | 0.033 | 0.030 |
Symptoms of Multiple Sclerosis Scale | 0.113 | 0.003 | 0.052 |
Self-efficacy | 0.153 | 0.017 | 0.052 |
CHIEF-SF | 0.188 | 0.026 | < 0.001 |
PDDS | 0.265 | 0.10 | < 0.001 |
Outcome expectations | 0.287 | 0.018 | < 0.001 |
Note. CHIEF-SF: Craig Hospital Inventory of Environmental Factors–Short Form; PDDS: Patient Disability Determinant Steps.
3.2Mediator analysis
Table 3 reports the mediator path results of the significant independent variables included in the final model predicting fatigue self-management behaviors. Only the PDDS had a significant effect on both outcome expectations and self-efficacy for fatigue self-management behaviors at 6 weeks (Path A). Symptoms of MS Scale, community living type, and CHIEF-SF did not have significant effects on outcome expectations or self-efficacy for fatigue self-management behaviors (Path A). Living alone had a significant effect only on outcome expectations at 6 weeks (Path A). Outcome expectations were significantly associated with fatigue self-management behaviors at 6-weeks across all independent variables (Path B). Self-efficacy was inconsistent in significantly being associated with fatigue-self management behaviors at 6-weeks (Path B). Only outcome expectations mediate the effects between PDDS and fatigue self-management behaviors (Path AB). Self-efficacy was not a significant mediator across all independent variables.
Table 3
Results of multiple mediator path analyses
Facilitator and Barrier | Mediator | Path AB (SE) [95% CI] | Path A (SE) [95% CI] | Path B (SE) [95% CI] | Path C |
PDDS | Outcome Expectations | 0.0134 (0.0055) [0.0044, 0.0260] | 0.1779 (0.0499) [0.0787, 0.2743] | 0.0759 (0.0189) [0.0388, 0.1131] | |
Self-Efficacy | 0.0035 (0.0038) [–0.0027, 0.0123] | 0.1711 (0.0529) [0.0670, 0.2752] | 0.0205 (0.0178) [–0.0145, 0.0556] | ||
0.0693 (0.01) [0.0496, 0.0890] | |||||
Symptoms of MS Scale | Outcome Expectations | –0.0004 (0.0015) [–0.0033, 0.0026] | –0.0039 (0.0145) [–0.0325, 0.0247] | 0.0954 (0.0187) [0.0585, 0.1323] | |
Self-Efficacy | –0.0002 (0.0005) [–0.0013, 0.0008] | –0.0079 (0.0151) [–0.0377, 0.0219] | 0.0230 (0.0180) [–0.0125, 0.0584] | ||
0.0184 (0.0028) [0.0129, 0.0240] | |||||
CHIEF-SF | Outcome Expectations | –0.0153 (0.0120) [–0.0402, 0.0082] | –0.1892 (0.1226) [–0.4305, 0.0522] | 0.0811 (0.0187) [0.0444, 0.1179] | |
Self-Efficacy | –0.0206 (0.0115) [–0.0460, –0.0014] | –0.4642 (0.1245) [–0.7093, 0.2190] | 0.0444 (0.0184) [0.0082 0.0806] | ||
0.1519 (0.0247) [0.1033, 0.2006] | |||||
Living Alone Status | Outcome Expectations | 0.0518 (0.0299) [–0.0001, 0.1172] | 0.5604 (0.2807) [0.0076, 1.1131] | 0.0924 (0.0200) [0.0190, 0.2513] | |
Self-Efficacy | 0.0051 (0.0112) [–0.0123, 0.0343] | 0.2193 (0.2926) [–0.3569, 0.7954] | 0.0231 (0.0192) [0.0529, 0.1319] | ||
0.1351 (0.0590) [0.0190, 0.2513] | |||||
Community Type: Rural vs. Urban | Outcome Expectations | –0.0276 (0.0282) [–0.0906, 0.0216] | –0.2725 (0.2767) [–0.8172, 0.2722] | ||
Self-Efficacy | –0.0028 (0.0089) [–0.0235, 0.0140] | –0.2888 (0.2881) [–0.8560, 0.2783] | |||
–0.0986 (0.0563) [–0.2076, 0.0140] | –0.0968 (0.0563) [–0.2076, 0.0140] | ||||
Community Type: | Outcome Expectations | –0.0640 (0.0377) [–0.1463, 0.0013] | –0.6319 (0.3523) [–1.3255, 0.0616] | ||
Suburban vs. Urban | Self-Efficacy | –0.0079 (0.0195) [–0.0520, 0.0282] | –0.8089 (0.3668) [–1.5311, –0.0868] | ||
–0.2679 (0.0722) [–0.4100, –0.1257] | –0.2679 (0.0722) [–0.4100, –0.1257] |
Note. PDDS: Patient Disease Determinant Steps; CHIEF-SF: Craig Hospital Inventory of Environmental Factors–Short Form; SE: standard error.
4Discussion
Few studies to date have identified fixed or modifiable variables that may predict engagement in fatigue self-management behaviors. This study advances the literature on MS by exploring five categories of factors that may directly or indirectly influence engagement in fatigue self-management behaviors, which has potential to help inform the development of personalized fatigue self-management interventions. For instance, we found that outcome expectations had the most significant direct effect on fatigue self-management behaviors at 6 weeks. People with MS who believe that fatigue self-management behaviors are ineffective may benefit from cognitive-behavioral strategies that help them reframe how they think about fatigue in relation to their behaviors.
Among the four categories of facilitators and barriers (Fig. 2), factors reflecting health status (e.g. PDDS, Symptoms of MS Scale), resources and environment (e.g., CHIEF-SF, living alone, community living type), and the healthcare system (e.g. utilization of rehab or wellness services) were significantly associated with fatigue self-management behaviors. No personal and lifestyle characteristics measured (e.g., race, Neuro-QOL Depression, Neuro-QOL Anxiety) were found to be significant correlates of fatigue self-management behaviors. Our findings are consistent with studies that have utilized similar frameworks to explicate relationships between facilitators, barriers, and engagement in self-management behaviors. For instance, research on fatigue self-management behaviors demonstrate that Social Cognitive Theory constructs, particularly self-efficacy and outcome expectations, may serve as a positive influence on behavior change in reducing MS and cancer-related fatigue (Chiba et al., 2019; Plow et al., 2020).
4.1Health status
A higher PDDS and Symptoms of MS scale score at baseline were associated with more frequent engagement in fatigue self-management behaviors at 6-weeks. These findings may reflect that greater disability and symptom severity is associated with a greater need to engage in self-management behaviors. A lack of perceived symptoms has been identified as a factor of diminishing self-management efforts, either due to decreased regard in seriousness or benefit of self-management tasks (Costantini et al., 2008). An individual who experiences more severe MS symptoms may be more motivated to learn and engage in self-management behaviors to relieve the impact of these symptoms.
These results contrast some findings in the current literature which indicate that increased symptoms and disability can serve as barriers to engagement in self-management behaviors. For example, MS symptoms and disability are well-documented barriers to participating in physical activity, which can be considered a fatigue self-management behavior (Plow et al., 2015; Schulman-Green et al., 2016). Therefore, experiencing greater disability and more severe symptoms may make someone more likely to engage in certain types of fatigue self-management behaviors but not others. Fatigue self-management behaviors involve a broad range of activities from using adaptive equipment to communicating one’s needs to family and friends, and the performance of these behaviors may be influenced by different individual factors. A person with MS fatigue may be more likely to take rest breaks throughout the day but may be less likely to engage in physical activity. Future research should subcategorize the various type of fatigue self-management behaviors to further delineate the relationship between health status indicators and fatigue self-management behaviors.
4.2Environment and resources
Several aspects of one’s surrounding environment were found to be significant facilitators or barriers of fatigue self-management behaviors. Living alone was significantly associated with increased engagement in fatigue self-management behaviors. A systemic review performed by Schulman-Green revealed that individuals who live with others may experience disruptions in self-management due to conflicting demands of their own self-management routines and those of others in their household (Schulman-Green et al., 2016). For example, people with MS who live with others and have a caregiving role may prioritize the health needs of those whom they live with, which may make them less likely to focus on their own personal self-management care.
The results of the final model indicated that living in an urban environment is negatively associated with fatigue-self management behaviors. Urban living can present a mix of challenges and benefits to engaging in self-management behaviors. For instance, urban environments are associated with increased barriers to healthcare access, exposure to occupational hazards, and poor nutrition (Alirol et al., 2011). The spontaneity of living in an urban environment may serve as a self-management barrier and make it more difficult to maintain consistent daily habits compared to rural counterparts. Nonetheless, the role of urban living as a barrier or facilitator to self-management is complex, and future research is needed to delineate the nuanced role that community types have on modifying health and self-management behaviors.
Our finding that increased environmental barriers (CHIEF-SF) is significantly associated with increased fatigue self-management behaviors may provide supporting evidence that people with MS engage in fatigue self-management more often due to increased perceived health needs. People with MS who report increased disability may be more likely to experience environmental barriers (Harrison-Felix, 2001). Those who face environmental barriers (e.g. “how often has the natural environment –temperature, terrain, climate –made it difficult to do what you want or need to do?”) may need to be more mindful of their symptoms and self-manage them accordingly.
4.3Self-management processes
As a part of the self-management processes, outcome expectations had the largest positive direct effect on fatigue-self management behaviors. Additionally, our mediation analysis revealed that outcome expectations for fatigue self-management behaviors at baseline mediated the effects of PDDS on fatigue self-management behaviors at 6-weeks. This suggests that people with MS scoring higher on PDDS may be more likely to engage in fatigue self-management behavior and tend to be influenced by their outcome expectations of the behavior. This is consistent with other study results demonstrating that outcome expectations may have a causative role in promoting self-management behaviors (Plow et al., 2020).
Self-efficacy was found to be a positive correlate with fatigue self-management behaviors. Current literature widely supports that self-efficacy is associated with greater self-management behaviors (Hwu & Yu, 2006; Marks et al., 2005; Wilski & Tasiemski, 2016). Nonetheless, we found that self-efficacy was not the strongest correlate of fatigue self-management behaviors, nor did not it serve as a mediator in our research sample. It is possible that outcome expectations of a behavior exerts greater influence on self-management than self-efficacy because the belief that a certain behavior will result in a desired outcome may outweigh one’s confidence in executing the behavior (Wilski et al., 2020). Someone who is less confident in their ability to adjust workspaces to manage their fatigue may still attempt to perform the behavior if they have high outcome expectations that it will be beneficial.
4.4Limitations
Limitations of this study include the use of self-report measures as opposed to using performance-based measures of function (e.g. cognitive). Additionally, the results may have limited external validity due to our sample of largely white, middle-class females, and an under-representation of ethnic minority with MS. Due to the study’s exclusion criteria, our study results have limited generalizability to people with MS with more severe disability who are non-ambulatory. The Self- and Family Management Model may also present with several limitations due to conceptually overlapping categories, which may under or over-estimate the impact of some facilitators and barriers on fatigue self-management behaviors.
5Conclusion
This study identified several facilitators and barriers that influence fatigue self-management behaviors in people with MS. Health status (i.e., disability and symptom severity), environmental factors (e.g., living situation) and self-management processes (i.e., self-efficacy and outcome expectations) may play an important role in influencing engagement in fatigue self-management behaviors. Understanding factors that influence fatigue self-management behaviors in the population with MS will help inform future fatigue self-management interventions that are better tailored to individuals’ needs by considering aspects of their health status, environment, and self-management processes.
Conflict of interest
None to report.
Funding
This work was supported by the Office of the Assistant Secretary of Defense for Health Affairs, through the Multiple Sclerosis Research Program under Award No. W81XWH-17-l-0089. Opinions, interpretations, conclusions and recommendations are those of the authors and are not necessarily endorsed by the Department of Defense.
References
1 | Alirol, E. , Getaz, L. , Stoll, B. , Chappuis, F. , & Loutan, L. ((2011) ). Urbanisation and infectious diseases in a globalised world. Lancet Infect Dis, 11: (2), 131–141. https://doi.org/10.1016/S1473-3099(10)70223-1 |
2 | Asano, M. , & Finlayson, M. L. ((2014) ). Meta-analysis of three different types of fatigue management interventions for people with multiple sclerosis: Exercise, education, and medication. Multiple Sclerosis International, 2014: , 798285. https://doi.org/10.1155/2014/798285 |
3 | Bandura, A. , ((1977) , Mar). Self-efficacy: toward a unifying theory of behavioral change. Psychol Rev, 84: (2), 191–215. https://doi.org/10.1037//0033-295x.84.2.191 |
4 | Benedict, R. H. , Cox, D. , Thompson, L. L. , Foley, F. , Weinstock-Guttman, B. , & Munschauer, F. , ((2004) , Dec). Reliable screening for neuropsychological impairment in multiple sclerosis. Mult Scler, 10: (6), 675–678. https://doi.org/10.1191/1352458504ms1098oa |
5 | Cella, D. , Lai, J. S. , Nowinski, C. J. , Victorson, D. , Peterman, A. , Miller, D. , Bethoux, F. , Heinemann, A. , Rubin, S. , Cavazos, J. E. , Reder, A. T. , Sufit, R. , Simuni, T. , Holmes, G. L. , Siderowf, A. , Wojna, V. , Bode, R. , McKinney, N. , Podrabsky, T. , Wortman, K. , Choi, S. , Gershon, R. , Rothrock, N. , & Moy, C. , (2012, Jun 5). Neuro-QOL: Brief measures of health-related quality of life for clinical research in neurology. Neurology, 78: (23), 1860–1867. https://doi.org/10.1212/WNL.0b013e318258f744 |
6 | Chiba, I. , Sasahara T. , & Mizuno, M. , (2019, Jul-Sep). Factors in Cancer-Related Fatigue Self-Management Behaviors of Outpatients Undergoing Chemotherapy. Asia Pac J Oncol Nurs, 6: (3), 209–211. https://doi.org/10.4103/apjon.apjon_1_19 |
7 | Constantino, M. J. ((2012) ). Believing is seeing: an evolving research program on patients’ psychotherapy expectations. Psychother Res, 22: (2), 127–138. https://doi.org/10.1080/10503307.2012.663512 |
8 | Costantini, L. , Beanlands, H. , McCay, E. , Cattran, D. , Hladunewich, M. , & Francis, D. , ((2008) , Mar-Apr). The selfmanagement experience of people with mild to moderate chronic kidney disease. Nephrol Nurs J, 35: (2), 147–155; quiz 156. https://www.ncbi.nlm.nih.gov/pubmed/18472683 |
9 | Faul, F. , Erdfelder, E. , Buchner, A. , & Lang, A. G. , (2009, Nov). Statistical power analyses using G.Power 3.1: tests for correlation and regression analyses. Behavior research methods, 41: (4), 1149–1160. https://doi.org/10.3758/brm.41.4.1149 |
10 | Fisk, J , Pontefract, A , Ritvo, P , Archibald, C , & Murray, T. ((1994) ). The impact of fatigue on patients with multiple sclerosis, Can J Neurol Sci, 21: (1), 9–14. |
11 | Fritz, M. S. , & Mackinnon, D. P. , ((2006) , Sep-Oct). A framework for the study of self- and family management of chronic conditions. Nurs Outlook, 54: (5), 233–239. https://doi.org/10.1016/j.outlook.2006.06.004 |
12 | Grey, M. , Knafl, K. , & McCorkle, R. , ((2015) , Mar-Apr). A revised Self- and Family Management Framework. Nurs Outlook, 63: (2), 278–286. https://doi.org/10.1016/j.outlook.2014.10.003 |
13 | Grey, M. , Schulman D. , Knafl, K. , & Reynolds, N. R. , ((2015) , Mar-Apr). A revised Self- and Family Management Framework. Nurs Outlook, 63: (2), 162–170. https://doi.org/10.1016/j.outlook.2014.10.003 |
14 | Harrison-Felix, C. L. (2001). The Craig Hospital Inventory of EnvironmentalFactors. Retrieved February 5 from http://www.tbims.org/combi/chief |
15 | Hayes, A. F. ((2018) ). Introduction to mediation, moderation, and conditional process analysis: A regression-based approach 2nd ed>. Guilford Press. |
16 | Hohol, M. J. , Orav, E. J. , & Weiner, H. L. , ((1999) , Oct). Disease steps in multiple sclerosis: a longitudinal study comparing disease steps and EDSS to evaluate disease progression. Mult Scler, 5: (5), 349–354. https://doi.org/10.1177/135245859900500508 |
17 | Horton, M. , Rudick, R. A. , Hara-Cleaver, C. , & Marrie, R. A. , ((2010) , Aug). Validation of a self-report comorbidity questionnaire for multiple sclerosis. Neuroepidemiology, 35: (2), 83–90. https://doi.org/10.1159/000311013 |
18 | Hwu, Y. J. , & Yu, C. C. , ((2006) , Dec). Exploring health behavior determinants for people with chronic illness using the con- structs of planned behavior theory. J Nurs Res, 14: (4), 261–270. https://doi.org/10.1097/01.jnr.0000387585.27587.4e |
19 | Katzman, R. , Brown, T. , Fuld, P. , Peck, A. , Schechter, R. , & Schimmel, H. , ((1983) , Jun). Validation of a short Orientation-Memory Concentration Test of cognitive impairment. Am J Psychiatry, 140: (6), 734–739. https://doi.org/10.1176/ajp.140.6.734 |
20 | Khaodhiar, L , McCowen, K. C , & Blackburn, G. L. ((1999) ). Obesity and its comorbid conditions. Clin Cornerstone, 2: (3), 17–31. https://doi.org/10.1016/s1098-3597(99)90002-9 |
21 | Kobelt, G. , Thompson, A , Berg, J. , Gannedahl, M. , & Eriksson, J. , Group, M. S., & European Multiple Sclerosis, P. ((2017) , Jul). New insights into the burden and costs of multiple sclerosis in Europe. Mult Scler, 23: (8), 1123–1136. https://doi.org/10.1177/1352458517694432 |
22 | Krupp, L. , ((2006) , Aug). Fatigue is intrinsic to multiple sclerosis (MS) and is the most commonly reported symptom of the disease. Mult Scler, 12: (4), 367–368. http://www.ncbi.nlm.nih.gov/entrez/query.fcgi?cmd=Retrieve&db=PubMed&dopt=Citation&list_uids=16900749 |
23 | Kurtzke, J. F. , ((1983) , Nov). Rating neurologic impairment in multiple sclerosis: an expanded disability status scale (EDSS). Neurology, 33: (11), 1444–1452. https://doi.org/10.1212/wnl.33.11.1444 |
24 | Liepold, A , & Mathiowetz, V. ((2005) ). Reliability and validity of the Self-Efficacy for Performing Energy Conservation Strategies Assessment for persons with multiple sclerosis. Occupational Therapy International, 12: (4), 234–249. https://www.ncbi.nlm.nih.gov/pubmed/16485510 |
25 | Lorig, K. R. , & Holman, H. , ((2003) , Aug). Self-management education: history, definition, outcomes, and mechanisms. Ann Behav Med, 26: (1), 1–7. https://doi.org/10.1207/S15324796ABM2601_01 |
26 | Mallik, P. S. , Finlayson, M. , Mathiowetz, V. , & Fogg, L. , ((2005) , Aug). Psychometric evaluation of the Energy Conservation Strategies Survey. Clinical Rehabilitation, 19: (5), 538–543. http://www.ncbi.nlm.nih.gov/entrez/query.fcgi?cmd=Retrieve&db=PubMed&dopt=Citation&list_uids=16119410 |
27 | Marks, R. , Allegrante, J. P. , & Lorig, K , ((2005) , Apr). A review and synthesis of research evidence for self-efficacy-enhancing interventions for reducing chronic disability: implications for health education practice (part II). Health Promot Pract, 6: (2), 148–156. https://doi.org/10.1177/1524839904266792 |
28 | Mathiowetz, V. , Matuska, K. M. , & Murphy, M. E. , ((2001) , Apr). Efficacy of an energy conservation course for persons with multiple sclerosis. Archives of Physical Medicine and Rehabilitation, 82: (4), 449–456. http://www.ncbi.nlm.nih.gov/entrez/query.fcgi?cmd=Retrieve&db=PubMed&dopt=Citation&list_uids=11295003 |
29 | McMillan, L. , & Moore, K. A. , ((2006) , Jun). The development and validation of the impact of multiple sclerosis scale and the symptoms of multiple sclerosis scale. Archives of Physical Medicine and Rehabilitation, 87: (6), 832–841. http://www.ncbi.nlm.nih.gov/entrez/query.fcgi?cmd=Retrieve&db=PubMed&dopt=Citation&list_uids=16731220 |
30 | Miller, D. M. , Bethoux, F. , Victorson, D. , Nowinski, C. J. , Buono, S. , Lai, J. S. , Wortman, K. , Burns, J. L. , Moy, C. , & Cella, D. , ((2016) , May). Validating Neuro-QoL short forms and targeted scales with people who have multiple sclerosis. Mult Scler, 22: (6), 830–841. https://doi.org/10.1177/1352458515599450 |
31 | Penner, I. K. , & Paul, F. , ((2017) , Nov). Fatigue as a symptom or comorbidity of neurological diseases. Nat Rev Neurol, 13: (11), 662–675. https://doi.org/10.1038/nrneurol.2017.117 |
32 | Plow, M. , Motl, R. W. , Finlayson, M. , & Bethoux, F. , ((2020) , Feb 21). Intervention mediators in a randomized controlled trial to increase physical activity and fatigue self-management behaviors among adults with multiple sclerosis. Annals of Behavioral Medicine, 54: (3), 213–221. https://doi.org/10.1093/abm/kaz033 |
33 | Plow, M. A. , Finlayson, M. , Gunzler, D. , & Heinemann, A. W. , ((2015) , Jun). Correlates of participation in meaningful activities among people with multiple sclerosis. J Rehabil Med, 47: (6), 538–545. https://doi.org/10.2340/16501977-1948 |
34 | Plow, M. A. , Finlayson, M. , & Rezac, M. , ((2011) , Mar). A scoping review of self-management interventions for adults with multiple sclerosis. PM R, 3: (3), 251–262. https://doi.org/10.1016/j.pmrj.2010.11.011 |
35 | Ryan, P. , & Sawin, K. J. , ((2009) , Jul-Aug). The Individual and Family Self-Management Theory: background and perspectives on context, process, and outcomes. Nurs Outlook, 57: (4), 217–225 e216. https://doi.org/10.1016/j.outlook.2008.10.004 |
36 | Sav, A , King, M. A , Kelly, F , McMillan, S. S , Kendall, E , Whitty, J. A , & Wheeler, A. J. ((2015) ). Self-management of chronic conditions in a rural and remote context. Aust J Prim Health, 21: (1), 90–95. https://doi.org/10.1071/PY13084 |
37 | Schoemann, A. M , Boulton, A. J , & Short, S. D. ((2017) ). Determining power and sample size for simple and complex mediation models, Social Psychological and Personality Science, 8: (4), 379–386. |
38 | Schulman D. , Jaser, S. , Martin, F. , Alonzo, A. , Grey, M. , McCorkle, R. , Redeker, N. S. , Reynolds, N. , & Whittemore, R. , ((2012) , Jun). Processes of self-management in chronic illness. J Nurs Scholarsh, 44: (2), 136–144. https://doi.org/10.1111/j.1547-5069.2012.01444.x |
39 | Schulman D. , Jaser, S. S. , Park, C. , & Whittemore, R. , ((2016) , Jul). A metasynthesis of factors affecting self-management of chronic illness. J Adv Nurs, 72: (7), 1469–1489. https://doi.org/10.1111/jan.12902 |
40 | Sherbourne, C. D , Stewart, A. L. ((1991) ). The MOS social support survey. Soc Sci Med, 32: (6), 705–714. https://doi.org/10.1016/0277-9536(91)90150-b |
41 | Skerrett, T. N. , & Moss-Morris, R. , ((2006) , 2006/11/01/). Fatigue and social impairment in multiple sclerosis: The role of patients’ cognitive and behavioral responses to their symptoms. Journal of Psychosomatic Research, 61: (5), 587–593. https:// doi.org/https://doi.org/10.1016/j.jpsychores.2006.04.018 |
42 | Smith, M. M. , & Arnett, P. A. , ((2005) , Oct). Factors related to employment status changes in individuals with multiple sclerosis. Mult Scler, 11: (5), 602–609. http://www.ncbi.nlm.nih.gov/pubmed/16193900 |
43 | Thompson, A. J. , Baranzini, S. E. , Geurts, J. , Hemmer, B. , & Ciccarelli, O. , ((2018) , Apr 21). Multiple sclerosis. Lancet, 391: (10130), 1622–1636. https://doi.org/10.1016/S0140-6736(18)30481-1 |
44 | Vanage, S. M. , Gilbertson, K. K. , & Mathiowetz, V. , ((2003) , May-Jun). Effects of an energy conservation course on fatigue impact for persons with progressive multiple sclerosis . American Journal of Occupational Therapy, 57: (3), 315–323. http://www.ncbi.nlm.nih.gov/entrez/query.fcgi?cmd=Retrieve&db=PubMed&dopt=Citation&list_uids=12785670 |
45 | Vucic, S. , Burke, D. , & Kiernan, M. C. , ((2010) , Jun). Fatigue in multiple sclerosis: mechanisms and management. Clin Neurophysiol, 121: (6), 809–817. https://doi.org/10.1016/j.clinph.2009.12.013 |
46 | Whiteneck, G. G. , Harrison-Felix, C. L. , Mellick, D. C. , Brooks, C.A. , Charlifue, S. B. , & Gerhart, K. A. , ((2004) , Aug). Quantifying environmental factors: A measure of physical, attitudinal, service, productivity, and policy barriers. Archives of Physical Medicine and Rehabilitation, 85: (8), 1324–1335. https://doi.org/10.1016/j.apmr.2003.09.027S0003999304000073[pii]. |
47 | Wilski, M. , Kocur, P. , Brola, W. , & Tasiemski, T. , ((2020) , Jul). Psychological factors associated with self-management in multiple sclerosis. Acta Neurol Scand, 142: (1), 50–57. https://doi.org/10.1111/ane.13236 |
48 | Wilski, M. , & Tasiemski, T. , ((2016) , May). Illness perception, treatment beliefs, self-esteem, and self-efficacy as correlates of self-management in multiple sclerosis. Acta Neurol Scand, 133: (5), 338–345. https://doi.org/10.1111/ane.12465 |