Improvement of differential diagnosis of lung cancer by use of multiple protein tumor marker combinations
Abstract
BACKGROUND:
Differential diagnosis of non-small cell lung cancer (NSCLC) and small cell lung cancer (SCLC) in hospitalized patients is crucial for appropriate treatment choice.
OBJECTIVE:
To investigate the relevance of serum tumor markers (STMs) and their combinations for the differentiation of NSCLC and SCLC subtypes.
METHODS:
Between 2000 and 2003, 10 established STMs were assessed retrospectively in 311 patients with NSCLC, 128 with SCLC prior systemic first-line therapy and 51 controls with benign lung diseases (BLD), by automatized electrochemiluminescence immunoassay technology. Receiver operating characteristic (ROC) curves and logistic regression analyses were used to evaluate the diagnostic efficacy of both individual and multiple STMs with corresponding sensitivities at 90% specificity. Standards for Reporting of Diagnostic Accuracy (STARD guidelines) were followed.
RESULTS:
CYFRA 21-1 (cytokeratin-19 fragment), CEA (carcinoembryonic antigen) and NSE (neuron specific enolase) were significantly higher in all lung cancers vs BLD, reaching AUCs of 0.81 (95% CI 0.76–0.87), 0.78 (0.73–0.84), and 0.88 (0.84–0.93), respectively. By the three marker combination, the discrimination between benign and all malignant cases was improved resulting in an AUC of 0.93 (95% CI 0.90–0.96). In NSCLC vs. BLD, CYFRA 21-1, CEA and NSE were best discriminative STMs, with AUCs of 0.86 (95% CI 0.81–0.91), 0.80 (0.74–0.85), and 0.85 (0.79–0.91). The three marker combination also improved the AUC: 0.92; 95% CI 0.89–0.96). In SCLC vs. BLD, ProGRP (pro-gastrin-releasing peptide) and NSE were best discriminative STMs, with AUCs of 0.89 (95% CI 0.84–0.94) and 0.96 (0.93–0.98), respectively, and slightly improved AUC of 0.97 (95% CI 0.95–0.99) when in combination. Finally, discrimination between SCLC and NSCLC was possible by ProGRP (AUC 0.86; 95% CI 0.81–0.91), NSE (AUC 0.83; 0.78–0.88) and CYFRA 21-1 (AUC 0.69; 0.64–0.75) and by the combination of the 3 STMs (AUC 0.93; 0.91–0.96), with a sensitivity of 88% at 90% specificity.
CONCLUSIONS:
The results confirm the power of STM combinations for the differential diagnosis of lung cancer from benign lesions and between histological lung cancer subtypes.
1Introduction
Lung cancer is one of the most frequent cancer types and a leading cause of cancer-related deaths worldwide [1, 2]. With demographic changes in industrial countries, a growing global population and an increasing smoking prevalence in less developed countries, along with the possible adverse effects by the coronavirus disease (COVID-19) on the diagnosis and prognosis of cancer, the burden of lung cancer remains high and continues to be a significant public health issue [3–5], both in industrialized and developing countries [6, 7]. The imminent need to improve lung cancer risk assessment is reflected in the promotion of detection at an early stage through low-dose computed tomography (LDCT) screening and refined by clinical risk models and biomarker assessments [8, 9]. Although novel treatment options, such as immune checkpoint inhibitors, expand therapeutic strategies, success rates remain relatively low in advanced stages [10], and early diagnosis and resulting appropriate intervention is crucial. In addition to the conventional approach of invasive lung biopsy, which often requires surgery and imaging that depend on experienced and adept interpretation, serum tumor markers provide a non-invasive and reproducible option for detecting lung cancer [11]. While elevated protein tumor markers can be observed in both healthy individuals and patients with other diseases, they are particularly associated with malignant processes and hold the potential for clinical application in the diagnosis of lung cancer [12].
The two primary types of lung cancer, non-small cell lung cancers (NSCLC) and small cell lung cancers (SCLC), exhibit diverse histological subtypes with distinct clinical features, growth patterns, molecular and biochemical characteristics, as well as sensitivities to systemic, targeted, and immunological therapies [13–16]. In addition to imaging and histopathological diagnostic procedures, serum tumor markers (STMs) may provide supplementary histological information to assist in treatment decisions and improve outcomes by optimizing the efficacy of therapy, while reducing the likelihood of adverse effects.
While a panoply of protein and molecular markers are applied for immunohistological diagnosis in cancer tissue, additional blood-based biomarkers are needed to support differential diagnosis in both NSCLC and SCLC. Classical blood-based biomarkers primarily used in NSCLC include carcinoembryonic antigen (CEA), cytokeratin-19 fragment (CYFRA21-1), and squamous cell carcinoma antigen (SCC). For SCLC, neuron specific enolase (NSE), and pro-gastrin-releasing peptide (ProGRP) are commonly employed [17]. Overexpression of these tumor markers has been associated with the diagnosis and histologic differentiation of lung cancer. In the recent years, there has been a growing interest in the use of serum tumor marker panels and algorithmic scores to improve diagnostic efficacy of lung cancer diagnosis in a non-invasive manner [18]. These panels combine the levels of multiple STMs to enhance their sensitivity and specificity for detecting cancer. In addition, STMs are included in lung-cancer prediction models to improve eligibility criteria for lung cancer screening [8]. Beyond established lung cancer markers, carbohydrate antigen markers CA 125, CA 15-3 and CA 19-9 have been included in some combined STM evaluations [12].
However, the available data on individual STM results exhibit discrepancies and have limited sensitivity and specificity, particularly in the case of early-stage lung cancer. Although none of these protein tumor markers are specific to lung cancer and can be elevated in other diseases or malignancies [19], their association with lung cancer and histological subtypes suggests a need for further assessment of their suitability for differential diagnosis. While several studies have proven the added value of different tumor marker combinations and scores for the differential diagnosis of lung cancer, independent validation and expansion with novel promising markers is warranted to fill the still existing diagnostic gaps.
The current study aimed to investigate the clinical relevance and ability of serum tumor markers, CYFRA 21-1, CEA, NSE, ProGRP, SCC, CA 125, CA 15-3, CA 19-9 and CA 72-4, both individually and in combination, to detect and discriminate lung cancer from benign lung disease, as well as to differentiate between various histological lung cancer subtypes.
With NSE and ProGRP, excellent diagnostic tumor markers are available for SCLC. However, there is still a lack of convincing markers for squamous and adenocarcinoma. Therefore, we included tumor markers CA 19-9, CA 72-4, CA 125 and CA 15-3 which have shown diagnostic potential in adenocarcinoma of the pancreas, the stomach, the ovaries and the breast, to investigate a possible additive value also in lung cancer. The melanoma marker S100 was included as negative marker. Additionally, combinational STM investigations were conducted to assess a potential additive benefit in the diagnosis and management of lung cancer.
2Patients and methods
2.1Study population and design
In a retrospective case-control approach, serum samples of 439 patients suffering from newly diagnosed lung cancer and receiving systemic therapy were investigated. The study cohort comprised 308 patients with NSCLC, 128 SCLC and 3 with mixed histology type. NSCLC and SCLC groups did not differ significantly in terms of age (U test, p = 0.78) or sex (χ2 test, p = 0.55). Consecutively collected serum samples of 51 patients with benign lung diseases (BLD), who were admitted to and diagnosed at the Asklepios Lung Speciality Hospital Gauting, were included in the evaluation as the control group. Diseases that were considered in the control cohort were pneumonia, sarcoidosis, fibrosis, tuberculosis, and respiratory insufficiency. Histopathological classification was determined in accordance with the revised 2001 World Health Organization (WHO) classification of lung tumors [20].
The evaluation study was conducted in accordance with the ethical standards outlined in the Declaration of Helsinki and the use of anonymized laboratory data for statistical evaluation was approved by the Ethics Committee of the Ludwig Maximilians-University (LMU) Munich (UE-Nr 114-13), as specified in the IRB approval.
2.2Methods and materials
Blood samples were consecutively collected in the Asklepios Lung Speciality Hospital Gauting (Germany) between 2000 and 2003 as part of the clinical routine setting at the time of diagnosis of active tumor disease and prior to the start of systemic first-line chemotherapy. Preanalytic handling included venous puncture and blood collection in S-Monovette® vials (Sarstedt, Nürmbrecht, Germany), followed by centrifugation at 3000 rpm for 15 minutes. Subsequently, STMs were routinely measured at the central laboratory of the Hospital Gauting or stored in cryogenic vials at temperatures below –70°C, until measurement at the Institute of Clinical Chemistry of the University Hospital Munich-Grosshadern.
The quantification of CEA, CYFRA21-1, NSE, SCC, CA 125, CA 15-3, CA 19-9, CA 72-4 and S100 was performed by electrochemiluminescence immunoassay (ECLIA) technology on an automated Cobas Elecsys E411 analyzer (Roche Diagnostics, Mannheim, Germany), while an enzyme-linked immunosorbent assay (ELISA, IBL, Hamburg, Germany) was used for ProGRP measurement.
2.3Statistical analysis
Medians, interquartile ranges (IQRs) and ranges are used in tables to depict the distribution of values. Differences in STMs between groups were calculated with Mann-Whitney U tests. Correlations between biomarkers were assessed with Spearman’s rank-order correlation. The diagnostic power of single and combined markers was evaluated by areas under the receiver operating characteristic (ROC) curves (AUCs) as well as by sensitivity at 90% specificity (Sens90). Logistic regression analysis was performed for all combinations of two, three and four markers. Log-values of the markers were used in logistic regression to account for the right-skewed distribution of the markers. The scores or probabilities obtained from logistic regression for ROC analysis are based on leave-one-out cross-validation to avoid overfitting. The marker combinations with the highest AUCs are presented. It should be noted that the other marker combinations provided similar results. Confidence intervals for the ROC analysis and sensitivity were computed based on 5000 bootstrap samples. Statistical significance was set at p < 0.05. Data analysis was performed using R (version 4.2.0; https://www.R-project.org (accessed on 11 March 2023), USA).
3Results
3.1Patient characteristics
As depicted in Table 1, there were 308 patients with non-small cell lung cancer (NSCLC), comprising 210 males and 98 females. Additionally, there were 128 patients with small cell lung cancer (SCLC), consisting of 93 males and 35 females. Among NSCLC patients, one each had stage I and stage II disease, while 111 had stage 3 localized disease and 195 had metastatic disease. Staging information was not available for 2 cases. The predominant histopathology in NSCLCs was adenocarcinoma (N = 129, 41.9%), squamous cell carcinoma (N = 88, 28.6%), large cell carcinoma (N = 8, 2.6%) and non-specified NSCLC (N = 83, 26.9%). SCLC patients comprised 61 (47.7%) with limited disease (among them 55 with stage 3) and 67 (52.3%) with extended disease.
Table 1
Patient characteristics
Benign lung disease | Lung cancer | NSCLC | SCLC | |
N | 439 | 308 | 128 | |
Age/gender | ||||
Age (years), median (range) | 61 | 63 | 63 | 61 |
(19–86) | (25–86) | (25–86) | (40–86) | |
Female N (%) | 24 (47.1) | 134 (30.5) | 98 (31.8) | 35 (27.3) |
Male N (%) | 27 (52.9) | 305 (69.5) | 210 (68.2) | 93 (72.7) |
Histology, N (%) | ||||
NSCLC – Adenocarcinoma | 129 (29.4) | 129 (41.9) | ||
NSCLC – Squamous cell carcinoma | 88 (20) | 88 (28.6) | ||
NSCLC – Large cell carcinoma | 8 (1.8) | 8 (2.6) | ||
NSCLC – not further classified | 83 (18.9) | 83 (26.9) | ||
SCLC | 128 (29.2) | 128 (100) | ||
mixed histology | 3 (0.7) | |||
Stage, N (%) | ||||
UICC stage 1 | 5 (1.1) | 1 (0.3) | 4 (3.1) | |
UICC stage 2 | 3 (0.7) | 1 (0.3) | 2 (1.6) | |
UICC stage 3 | 166 (37.8) | 111 (36.1) | 55 (43) | |
UICC stage 4 | 265 (60.4) | 195 (63.3) | 67 (52.3) |
NSCLC (non-small cell lung cancer); SCLC (small cell lung cancer); UICC (Union for International Cancer Control).
3.2Distribution of tumor marker values in disease groups
Table 2 illustrates the concentrations, ranges, median values, interquartile ranges (ICR) and 95th percentiles of STM values in BLD and lung cancer. The value distribution of tumor markers in benign lung diseases showed median and 95% levels within the range or only slightly elevated compared to the reference values provided by the manufacturers for the lung cancer markers, CEA, CYFRA 21-1, NSE, ProGRP, and SCC. For the carbohydrate antigens, CA 125, CA 15-3, CA 19-9, and CA 72-4, 95th percentiles were twice or even more elevated than the reference values reported by the manufacturers. As a negative control, S100 exhibited very low levels in both benign and malignant lung diseases and was not considered for the subsequent evaluations.
Table 2
Value distribution of tumor markers with median values, interquartile ranges (ICR), minimal and maximal values and 95th percentiles in benign lung disease (BLD) and lung cancer patients
CEA | CYFRA | NSE | PROGRP | SCC | CA 125 | CA 15-3 | CA 19-9 | CA 72-4 | S100 | ||
ng/mL | ng/mL | ng/mL | pg/mL | ng/mL | U/mL | U/mL | U/mL | U/mL | ng/mL | ||
Benign Lung Diseases | N | 51.0 | 51.0 | 51.0 | 41.0 | 54.0 | 51.0 | 51.0 | 51.0 | 51.0 | 51.0 |
Min | 0.4 | 0.1 | 0.4 | 2.0 | 0.1 | 6.9 | 4.3 | 0.6 | 0.3 | 0.005 | |
Q1 | 1.2 | 0.7 | 6.1 | 4.9 | 0.5 | 13.0 | 13.1 | 2.9 | 0.7 | 0.024 | |
Med | 2.3 | 1.1 | 8.2 | 14.1 | 0.8 | 27.1 | 21.6 | 8.5 | 1.2 | 0.037 | |
Q3 | 3.6 | 2.0 | 11.6 | 19.0 | 1.1 | 55.7 | 35.4 | 18.1 | 3.9 | 0.055 | |
Max | 8.2 | 7.5 | 21.7 | 131.0 | 5.9 | 164.0 | 78.1 | 117.0 | 23.9 | 0.121 | |
Lung Cancer | N | 438.0 | 438.0 | 438.0 | 438.0 | 432.0 | 431.0 | 431.0 | 431.0 | 431.0 | 425.0 |
Min | 0.2 | 0.1 | 3.6 | 2.0 | 0.1 | 4.0 | 8.1 | 0.5 | 0.3 | 0.001 | |
Q1 | 2.7 | 1.7 | 12.5 | 10.0 | 0.4 | 24.6 | 18.5 | 6.5 | 0.6 | 0.011 | |
Med | 5.5 | 3.8 | 16.4 | 19.2 | 0.7 | 52.4 | 26.1 | 13.5 | 1.5 | 0.020 | |
Q3 | 25.8 | 9.6 | 29.1 | 45.0 | 1.4 | 130.9 | 44.4 | 39.5 | 6.5 | 0.031 | |
Max | 77695.0 | 1017.9 | 1053.5 | 109316.0 | 176.0 | 25084.4 | 3465.0 | 54417.0 | 22743.0 | 0.167 | |
SCLC | N | 128.0 | 128.0 | 128.0 | 128.0 | 127.0 | 127.0 | 127.0 | 127.0 | 127.0 | 125.0 |
Min | 0.3 | 0.1 | 6.6 | 3.0 | 0.1 | 4.4 | 8.3 | 0.5 | 0.3 | 0.001 | |
Q1 | 2.7 | 1.2 | 20.2 | 32.6 | 0.3 | 22.4 | 16.6 | 7.4 | 0.5 | 0.010 | |
Med | 3.8 | 2.2 | 40.0 | 344.5 | 0.6 | 38.3 | 21.8 | 13.2 | 0.7 | 0.017 | |
Q3 | 9.5 | 4.1 | 94.1 | 1035.5 | 0.8 | 80.9 | 32.3 | 35.0 | 2.1 | 0.026 | |
Max | 3643.0 | 282.7 | 1053.5 | 109316.0 | 12.1 | 2161.5 | 112.9 | 1435.0 | 35.4 | 0.167 | |
NSCLC | N | 307.0 | 307.0 | 307.0 | 307.0 | 302.0 | 301.0 | 301.0 | 301.0 | 301.0 | 297.0 |
Min | 0.2 | 0.1 | 3.6 | 2.0 | 0.1 | 4.0 | 8.1 | 0.5 | 0.3 | 0.001 | |
Q1 | 2.7 | 2.4 | 11.3 | 8.5 | 0.4 | 26.8 | 19.8 | 5.9 | 0.8 | 0.011 | |
Med | 6.4 | 4.9 | 14.6 | 15.0 | 0.7 | 64.5 | 28.7 | 13.7 | 2.5 | 0.021 | |
Q3 | 37.6 | 10.7 | 18.8 | 24.0 | 1.9 | 160.3 | 54.8 | 44.6 | 10.5 | 0.034 | |
Max | 77695.0 | 1017.9 | 355.0 | 130.0 | 176.0 | 25084.4 | 3465.0 | 54417.0 | 22743.0 | 0.154 | |
NSCLC_ADC | N | 129.0 | 129.0 | 129.0 | 129.0 | 128.0 | 127.0 | 127.0 | 127.0 | 127.0 | 126.0 |
Min | 0.2 | 0.4 | 3.6 | 2.0 | 0.1 | 7.4 | 9.7 | 0.5 | 0.4 | 0.001 | |
Q1 | 3.5 | 2.5 | 11.2 | 10 | 0.3 | 40.15 | 23.8 | 6.1 | 1.25 | 0.012 | |
Med | 12.9 | 4.4 | 14.6 | 15.0 | 0.6 | 94.1 | 41.7 | 16.7 | 5.3 | 0.025 | |
Q3 | 83.9 | 10.2 | 17.7 | 25.6 | 1.1 | 261.1 | 85.5 | 75.7 | 30.2 | 0.038 | |
Max | 1437.0 | 255.6 | 275.3 | 64.0 | 16.6 | 25084.4 | 3465.0 | 54417.0 | 22743.0 | 0.154 | |
NSCLC_SQC | N | 87.0 | 87.0 | 87.0 | 87.0 | 86.0 | 86.0 | 86.0 | 86.0 | 86.0 | 84.0 |
Min | 0.3 | 0.1 | 5.4 | 2.0 | 0.1 | 5.1 | 10.5 | 0.5 | 0.3 | 0.001 | |
Q1 | 2.25 | 3.35 | 11 | 9 | 0.75 | 20.73 | 17.7 | 5.88 | 0.8 | 0.012 | |
Med | 4.3 | 6.6 | 14.5 | 15.0 | 1.7 | 38.9 | 22.4 | 11.0 | 1.3 | 0.017 | |
Q3 | 7.65 | 13 | 18.9 | 24.6 | 3.95 | 86.85 | 30.2 | 21.63 | 4.5 | 0.028 | |
Max | 187.7 | 388.2 | 63.0 | 130.0 | 176.0 | 385.2 | 98.3 | 1546.0 | 169.9 | 0.063 | |
NSCLC loc | N | 112.0 | 112.0 | 112.0 | 112.0 | 111.0 | 111.0 | 111.0 | 111.0 | 111.0 | 110.0 |
Min | 0.3 | 0.1 | 5.4 | 2.0 | 0.1 | 5.1 | 9.8 | 0.5 | 0.4 | 0.001 | |
Q1 | 2.6 | 1.7 | 10.6 | 7.0 | 0.4 | 23.0 | 18.5 | 5.3 | 0.8 | 0.012 | |
Med | 5.1 | 3.7 | 13.2 | 13.0 | 0.7 | 47.1 | 26.7 | 10.8 | 2.0 | 0.022 | |
Q3 | 17.1 | 7.5 | 16.4 | 21.2 | 1.8 | 120.4 | 43.2 | 32.7 | 9.3 | 0.032 | |
Max | 1437.0 | 266.2 | 63.0 | 106.0 | 176.0 | 8130.0 | 1995.0 | 25826.9 | 415.4 | 0.154 | |
NSCLC met | N | 195.0 | 195.0 | 195.0 | 195.0 | 191.0 | 190.0 | 190.0 | 190.0 | 190.0 | 187.0 |
Min | 0.2 | 0.2 | 3.6 | 2.0 | 0.1 | 4.0 | 8.1 | 0.5 | 0.3 | 0.001 | |
Q1 | 2.8 | 2.8 | 11.9 | 9.0 | 0.4 | 30.4 | 20.6 | 6.4 | 0.9 | 0.011 | |
Med | 8.7 | 6.2 | 15.3 | 16.0 | 0.7 | 78.4 | 29.9 | 15.5 | 2.9 | 0.021 | |
Q3 | 41.6 | 12.2 | 22.2 | 25.3 | 1.9 | 189.1 | 62.9 | 52.4 | 11.3 | 0.035 | |
Max | 77695.0 | 1017.9 | 355.0 | 130.0 | 27.1 | 25084.4 | 3465.0 | 54417.0 | 22743.0 | 0.092 | |
SCLC lim | N | 61.0 | 61.0 | 61.0 | 61.0 | 60.0 | 60.0 | 60.0 | 60.0 | 60.0 | 60.0 |
Min | 0.3 | 0.1 | 6.6 | 3.0 | 0.1 | 4.4 | 8.5 | 0.5 | 0.4 | 0.001 | |
Q1 | 2.5 | 0.8 | 15.7 | 23.2 | 0.4 | 17.7 | 17.1 | 8.5 | 0.5 | 0.009 | |
Med | 3.2 | 1.6 | 27.8 | 331.0 | 0.6 | 35.8 | 20.2 | 11.9 | 0.8 | 0.019 | |
Q3 | 5.2 | 2.9 | 55.8 | 847.0 | 1.0 | 92.4 | 25.8 | 21.6 | 2.2 | 0.026 | |
Max | 157.6 | 48.1 | 591.0 | 10502.0 | 12.1 | 849.0 | 54.1 | 248.0 | 24.0 | 0.167 | |
SCLC ext | N | 67.0 | 67.0 | 67.0 | 67.0 | 67.0 | 67.0 | 67.0 | 67.0 | 67.0 | 65.0 |
Min | 0.9 | 0.1 | 8.6 | 3.0 | 0.1 | 9.3 | 8.3 | 0.5 | 0.3 | 0.001 | |
Q1 | 3.2 | 1.5 | 28.2 | 36.2 | 0.3 | 24.6 | 15.6 | 7.3 | 0.5 | 0.011 | |
Med | 6.1 | 2.6 | 53.8 | 356.0 | 0.6 | 38.3 | 23.9 | 15.5 | 0.7 | 0.017 | |
Q3 | 14.6 | 7.9 | 133.9 | 1974.0 | 0.7 | 77.6 | 38.2 | 59.2 | 1.5 | 0.024 | |
Max | 3643.0 | 282.7 | 1053.5 | 109316.0 | 2.7 | 2161.5 | 112.9 | 1435.0 | 35.4 | 0.098 |
CEA (carcinoembryonic antigen); CYFRA (cytokeratin-19 fragment); NSE (neuron specific enolase); ProGRP (pro-gastrin-releasing peptide); SCC (squamous cell cancer antigen); CA 19-9 (carbohydrate antigen 19-9); CA 15-3 (cancer antigen 15-3); CA 125 (cancer antigen 125); CA 72-4 (cancer antigen 72-4); NSCLC (non-small cell lung cancer); SCLC (small cell lung cancer); adenocarcinoma (ADC); squamous cell carcinoma (SQC); localized (loc); metastasized (met); limited disease (lim); extensive disease (ext); Q1 (first quartile); Q3 (third quartile); med (median).
Significant differences in STM levels (Tables 2, 3, Fig. 1) were seen between patients with BLD and lung cancer, with higher values for CEA, CYFRA 21-1, NSE, ProGRP, CA 125 (p < 0.001 for all) as well as for CA 15-3 and CA 19-9 (p≤0.01, both). Only SCC and CA 72-4 showed similar values in both groups. In the comparison between BLD and NSCLC patients (Tables 2, 3, Fig. 1), significantly higher values were observed for CEA, CYFRA 21-1, NSE, CA 125, CA 15-3 (p < 0.001 for all), CA 19-9 (p < 0.01) and CA 72-4 (p < 0.05). When comparing patients with BLD to those with SCLC, significantly higher values were particularly observed for ProGRP and NSE (p < 0.001 for both) as well as for CEA, CYFRA 21-1 (p < 0.001 for all), CA 125 (p < 0.05), CA 19-9 and CA 72-4 (p < 0.01, both), while SCC values (p < 0.01) were lower in SCLC (Tables 2, 3, Fig. 1).
Table 3
Power of discrimination of different groups by single tumor markers and combinations
CEA | CYFRA | NSE | PROGRP | SCC | CA 125 | CA 15-3 | CA 19-9 | CA 72-4 | Best double marker combi | Best triple marker combi | Best four marker combi | ||
CEA+ NSE | CEA + NSE + CYFRA | CEA + NSE + CYFRA+ | |||||||||||
ProGRP | |||||||||||||
Benign* vs lung cancer | AUC | 0.78 | 0.81 | 0.88 | 0.67 | 0.52** | 0.67 | 0.62 | 0.64 | 0.53 | 0.92 | 0.93 | 0.95 |
95% CI | 0.73–0.84 | 0.76–0.87 | 0.84–0.93 | 0.59–0.74 | 0.45–0.59 | 0.6–0.75 | 0.54–0.71 | 0.56–0.72 | 0.45–0.6 | 0.89–0.95 | 0.90–0.96 | 0.92–0.97 | |
Sens90 | 56% | 52% | 66% | 43% | 16% | 33% | 28% | 25% | 19% | 72% | 79% | 84% | |
95% CI | 0.48–0.62 | 0.45–0.69 | 0.55–0.77 | 0.24–0.52 | 0.10–0.27 | 0.21–0.48 | 0.16–0.33 | 0.13–0.42 | 0.15–0.29 | 0.68–0.84 | 0.68–0.88 | 0.78–0.91 | |
p-value | <0.001 | <0.001 | <0.001 | <0.001 | 0.6 | <0.001 | <0.01 | <0.01 | 0.56 | ||||
CEA + | CEA + | CEA + | |||||||||||
CYFRA | CYFRA+ | CYFRA+ | |||||||||||
NSE | NSE+ | ||||||||||||
ProGRP | |||||||||||||
Benign* vs NSCLC | AUC | 0.8 | 0.86 | 0.85 | 0.57 | 0.48** | 0.71 | 0.66 | 0.63 | 0.6 | 0.90 | 0.92 | 0.94 |
95% CI | 0.74–0.85 | 0.81–0.91 | 0.79–0.91 | 0.48–0.66 | 0.41–0.55 | 0.63–0.78 | 0.58–0.74 | 0.56–0.71 | 0.53–0.67 | 0.86–0.94 | 0.89–0.96 | 0.90–0.97 | |
Sens90 | 60% | 62% | 57% | 27% | 16% | 39% | 33% | 27% | 26% | 73% | 74% | 80% | |
95% CI | 0.51–0.68 | 0.53–0.76 | 0.43–0.72 | 0.05–0.38 | 0.1–0.26 | 0.24–0.54 | 0.21–0.39 | 0.14–0.42 | 0.19–0.37 | 0.62–0.83 | 0.63–0.89 | 0.71–0.91 | |
p-value | <0.001 | <0.001 | <0.001 | 0.15 | 0.63 | <0.001 | <0.001 | <0.01 | <0.05 | ||||
NSE + | NSE + | NSE + | |||||||||||
ProGRP | ProGRP+ | ProGRP+ | |||||||||||
CEA | CEA+ | ||||||||||||
CYFRA | |||||||||||||
Benign* vs SCLC | AUC | 0.75 | 0.69 | 0.96 | 0.89 | 0.62** | 0.61 | 0.53 | 0.65 | 0.65** | 0.97 | 0.97 | 0.97 |
95% CI | 0.67–0.82 | 0.61–0.78 | 0.93–0.98 | 0.84–0.94 | 0.54–0.71 | 0.51–0.7 | 0.43–0.63 | 0.56–0.74 | 0.56–0.73 | 0.95–0.99 | 0.95–0.99 | 0.95–0.99 | |
Sens90 | 44% | 28% | 88% | 81% | 15% | 21% | 15% | 21% | 29% | 92% | 94% | 94% | |
95% CI | 0.35–0.54 | 0.2–0.51 | 0.82–0.94 | 0.67–0.88 | 0.09–0.32 | 0.12–0.38 | 0.02–0.24 | 0.08–0.43 | 0.19–0.45 | 0.88–0.98 | 0.89–0.98 | 0.89–0.98 | |
p-value | <0.001 | <0.001 | <0.001 | <0.001 | <0.01 | <0.05 | 0.5 | <0.01 | <0.01 | ||||
NSE + | NSE + | NSE + | |||||||||||
ProGRP | ProGRP+ | ProGRP+ | |||||||||||
CYFRA | CYFRA+ | ||||||||||||
CA72-4 | |||||||||||||
NSCLC* vs SCLC | AUC | 0.59** | 0.69** | 0.83 | 0.86 | 0.6** | 0.61** | 0.65** | 0.49** | 0.71** | 0.9 | 0.93 | 0.94 |
95% CI | 0.53–0.64 | 0.64–0.75 | 0.78–0.88 | 0.81–0.91 | 0.55–0.66 | 0.56–0.67 | 0.59–0.7 | 0.44–0.55 | 0.65–0.76 | 0.86–0.94 | 0.91–0.96 | 0.92–0.97 | |
Sens90 | 9% | 27% | 58% | 70% | 10% | 18% | 18% | 6% | 14% | 80% | 88% | 87% | |
95% CI | 0.04–0.16 | 0.19–0.38 | 0.5–0.73 | 0.63–0.8 | 0.05–0.16 | 0.1–0.27 | 0.11–0.28 | 0.02–0.12 | 0.14–0.34 | 0.73–0.88 | 0.80–0.93 | 0.81–0.93 | |
p-value | <0.01 | <0.001 | <0.001 | <0.001 | <0.001 | <0.001 | <0.001 | 0.84 | <0.001 |
Areas under the receiver operating characteristic (ROC) curves (AUCs), 95% confidence intervals (95% CI) and sensitivities at 90% specificities (Sens90). Differences of values between different groups presented as p-values (Mann-Whitney U test). CEA (carcinoembryonic antigen); CYFRA (cytokeratin-19 fragment); NSE (neuron specific enolase); ProGRP (pro-gastrin-releasing peptide); SCC (squamous cell cancer antigen); CA 19-9 (carbohydrate antigen 19-9); CA 15-3 (cancer antigen 15-3); CA 125 (cancer antigen 125); CA 72-4 (cancer antigen 72-4); Small cell lung cancer (SCLC); non-small cell lung cancer (NSCLC); adenocarcinoma (ADC); squamous cell carcinoma (SQC); localized (loc); metastasized (met); limited disease (lim); extensive disease (ext). Classifier for logistic regression (*). Biomarker has a negative correlation with classifier (**).
Fig. 1
Log Transformed Serum Tumor Marker Values in Benign Lung Disease and Lung Carcinoma Patients. Benign (benign lung disease); LuCa (lung carcinoma); SCLC (small cell lung cancer); NSCLC (non-small cell lung cancer); Adeno (adenocarcinoma); SQC (squamous cell carcinoma); CEA (carcinoembryonic antigen); CYFRA (cytokeratin-19 fragment); NSE (neuron specific enolase); ProGRP (pro-gastrin-releasing peptide); SCC (squamous cell cancer antigen); CA199 (carbohydrate antigen 19-9); CA153 (cancer antigen 15-3); CA125 (cancer antigen 125); CA724 (cancer antigen 72-4).
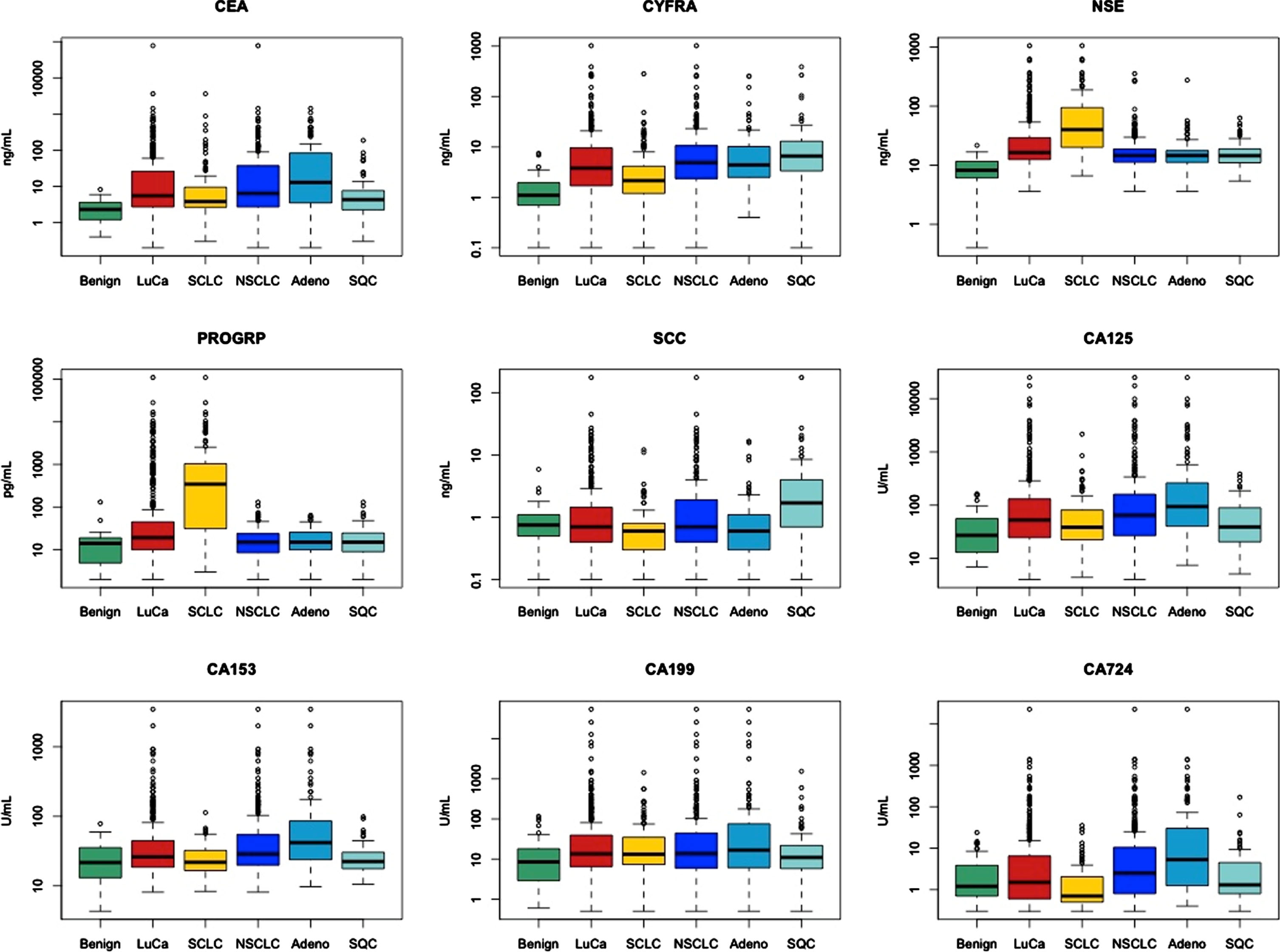
Regarding histologies, NSE and ProGRP were significantly (both p < 0.001) higher in SCLC than in NSCLC, while CYFRA 21-1, SCC, CA 125, CA 15-3, CA 72-4 (p < 0.001 for all) and CEA (p < 0.01) were significantly higher in NSCLC than in SCLC.
In more detail, CYFRA 21-1 (p < 0.05) and SCC (p < 0.001) were significantly higher in squamous cell NSCLC, while CEA, CA 125, CA 15-3, CA 72-4 (p < 0.001, all) as well as CA 19-9 (p < 0.05) were significantly higher in adenocellular NSCLC.
In the comparison of localized and metastatic disease in NSCLC, significantly higher levels of CYFRA 21-1 (p < 0.01), NSE (p < 0.001) as well as of CEA, CA125 NSE and CA 15-3 (p < 0.05, all three) were observed in metastatic disease. In the comparison of limited and extended disease in SCLC, significantly higher levels of CYFRA 21-1, NSE (p < 0.001, both) and CEA (p < 0.01) were observed in extended disease. Remarkably, ProGRP was already strongly elevated in limited SCLC disease demonstrating its potential for early diagnosis of SCLC.
3.3Correlation of tumor markers in disease groups
Some markers showed distinct correlations in different disease groups (Fig. 2). In our analysis, we have considered as noteworthy correlations between biomarkers with an R-value exceeding 0.25, as the studied biomarkers are related to different biochemical aspects and functions of cancer cells, i.e. they represent different surface epitopes (carbohydrate antigens), structural components (cytokeratins) or enzymatic (NSE) and endocrine (ProGRP) properties. Therefore, a significant correlation of the biomarkers – even with low correlation coefficients – appears to be relevant in this context. In BLD, highest correlations were observed for CYFRA with CA 125 (R = 0.45), NSE (R = 0.28), CA 15-3 (R = 0.29), NSE with ProGRP (R = 0.33) and CA 125 with CA 15-3 (R = 0.28), while NSE and CA 19-9 were negatively correlated (R = –0.28). In all lung cancers, the highest correlations were observed for CEA with CA 125 (R = 0.26), CA 15-3 (R = 0.30), CA 19-9 (R = 0.26), CA 72-4 (R = 0.29), CYFRA with SCC (R = 0.27), CA 125 (R = 0.32), CA 15-3 (R = 0.27), NSE with ProGRP (R = 0.38), CA 125 with CA 15-3 (R = 0.39), CA 19-9 (R = 0.30), CA 15-3 with CA 19-9 (R = 0.26) and CA 72-4 (R = 0.32). A similar picture was seen for the histological NSCLC subtype with even higher correlations between CYFRA 21-1 and NSE (R = 0.38) and between the carbohydrate antigen markers, while in SCLC, correlations between CYFRA 21-1 and NSE (R = 0.47), as well as between NSE and ProGRP (R = 0.34) were most pronounced.
Fig. 2
Correlations of serum tumor markers in A) patients with benign lung diseases, B) patients with lung cancer, C) patients with non-small cell lung cancer (NSCLC), and D) patients with small cell lung cancer (SCLC).
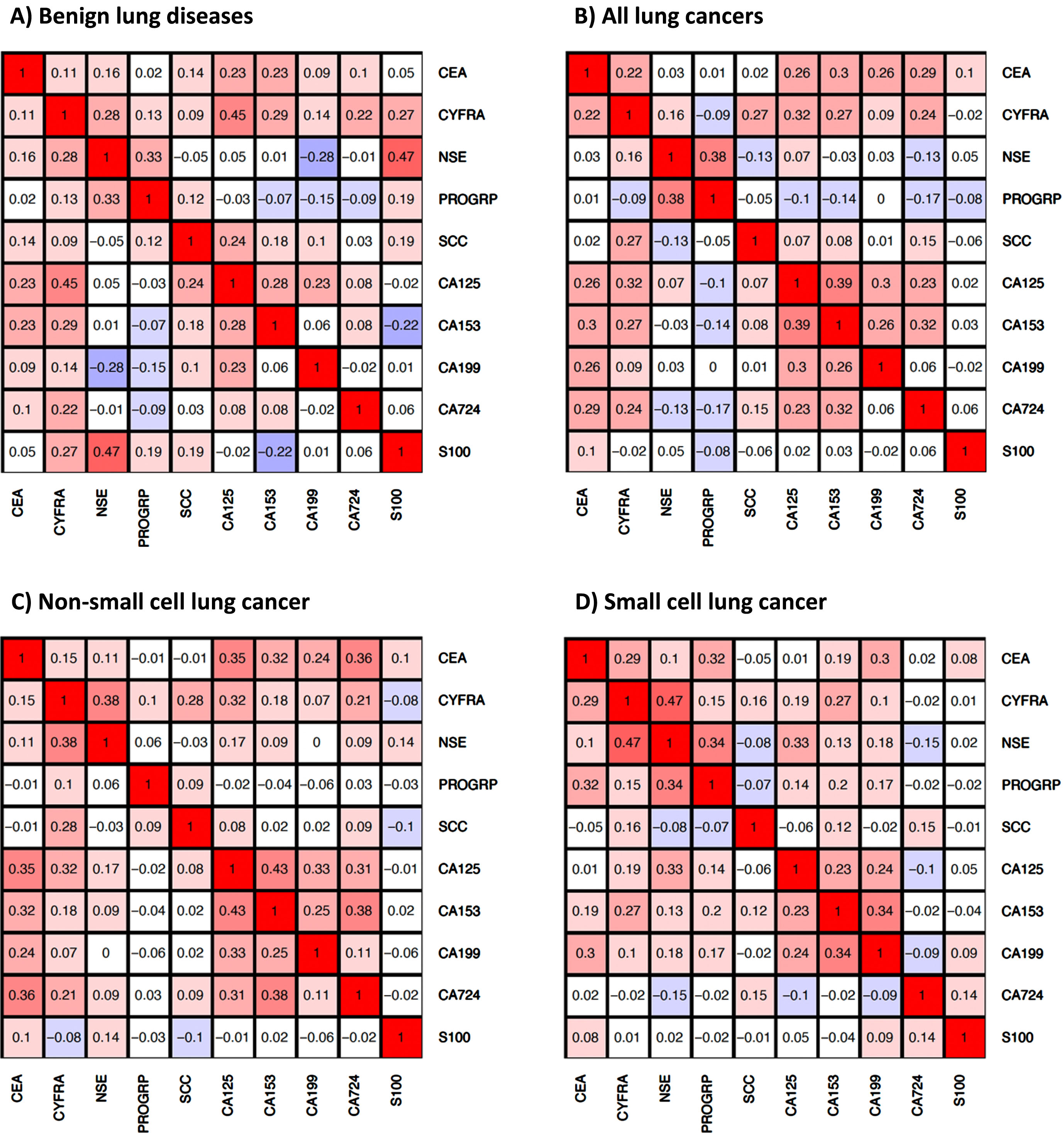
3.4Discrimination of lung cancer and benign lung diseases
The power of discrimination of lung cancer from BLD was calculated by AUCs under the ROC curves for all single STMs providing a comprehensive evaluation of sensitivity and specificity over the whole range of possible cutoffs (Table 3, Fig. 3). Thereby, the highest AUCs were achieved by CYFRA 21-1 (AUC 0.81; 95% CI 0.76–0.87; p < 0.001), CEA (AUC 0.78; 95% CI 0.73–0.84; p < 0.001) and NSE (AUC 0.88; 95% CI 0.84–0.93; p < 0.001). Remarkably, AUC was further increased by the two-marker combination of CYFRA 21-1 and NSE to 0.91 (95% CI 0.87–0.95; p < 0.001), NSE and CEA to 0.92 (95% CI 0.89–0.95; p < 0.001) and by the three-marker combination of CYFRA 21-1, CEA and NSE to 0.93 (95% CI 0.90–0.96; p < 0.001) (Fig. 3). The best sensitivities at 90% specificity for single markers were 52% for CYFRA 21-1, 56% for CEA and 66% for NSE. Combinations of NSE and CEA reached a sensitivity of 72% and for CYFRA 21-1, CEA and NSE of 79% at 90% specificity. The four marker combinations ProGRP, NSE, CYFRA 21-1 and CEA reached the highest sens90 of 84%.
Fig. 3
Power of discrimination between patients with lung cancer and benign lung diseases by use of single tumor markers, and combinations, illustrated by receiver operating characteristic (ROC) curves, providing a complete profile of sensitivity and specificity over the whole range of possible cutoffs, with areas under the curves (AUCs) and respective 95% confidence intervals (95% CI).
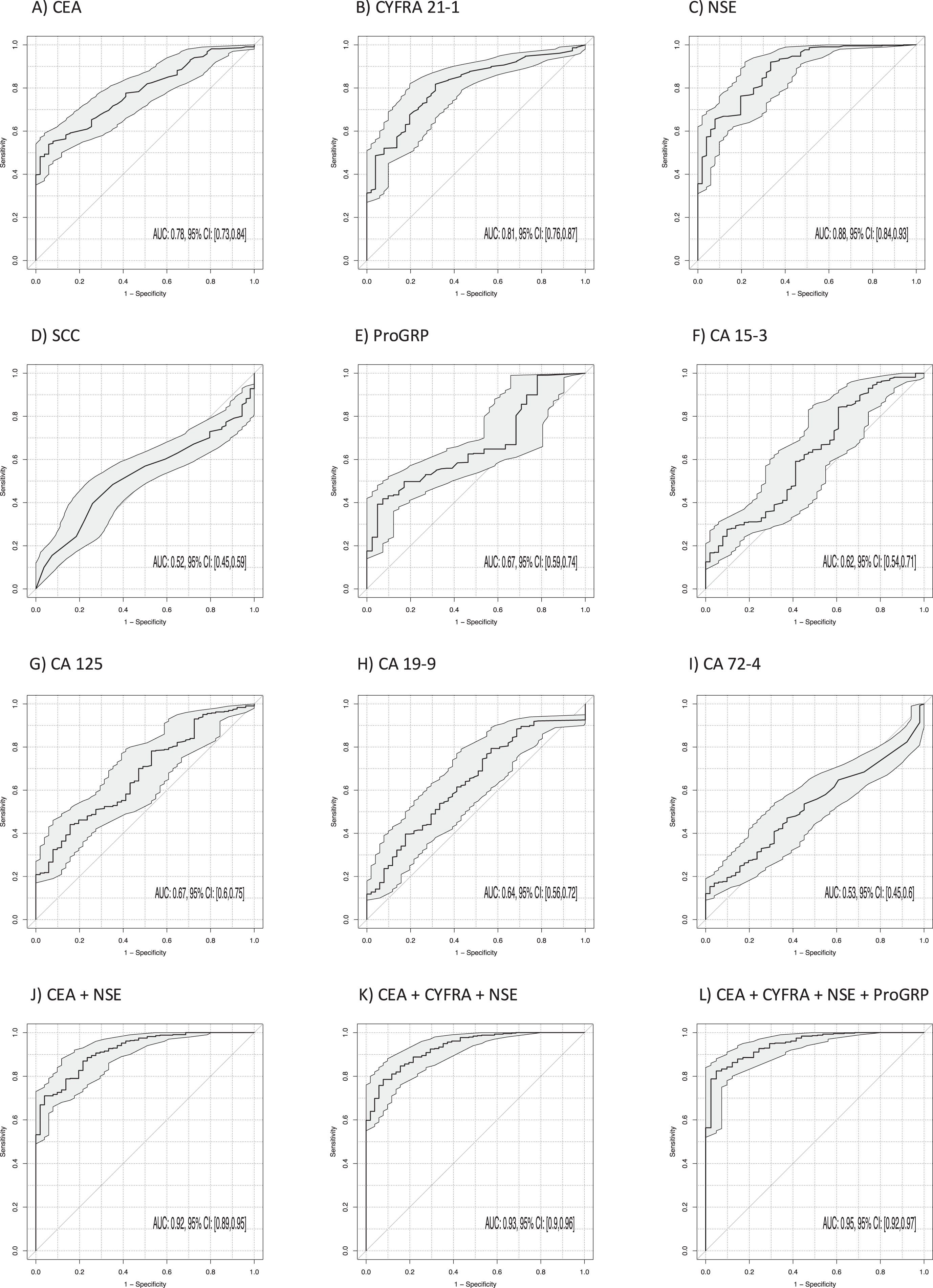
3.5Discrimination of NSCLC and benign lung diseases
The best discrimination between NSCLC and BLD (Table 3, Fig. 4) was achieved by CYFRA 21-1 (AUC 0.86; 95% CI 0.81–0.91; p < 0.001), NSE (AUC 0.85; 95% CI 0.79–0.91; p < 0.001) and CEA (AUC 0.80; 95% CI 0.74–0.85; p < 0.001). AUC was further increased by the two-marker combination of CEA and NSE to 0.90 (95% CI 0.86–0.94; p < 0.001) and CEA and CYFRA 21-1 (AUC 0.90; 95% CI 0.86–0.94; p < 0.001), and by the three-marker combination of CYFRA 21-1, CEA and NSE to 0.92 (95% CI 0.89–0.96; p < 0.001). The best sensitivities at 90% specificity for single markers were 62% for CYFRA 21-1, 60% for CEA and 57% for NSE. Particularly for the combinations of CEA and CYFRA 21-1, the sensitivity reached 73%, and for the three-marker combination CYFRA 21-1, CEA and NSE it was 74% (p < 0.001). A four-marker combination with ProGRP increased sensitivity to 80%.
Fig. 4
Power of discrimination between patients with non-small cell lung cancer (NSCLC) and benign lung diseases (BLD) by use of single tumor markers and combinations illustrated by receiver operating characteristic (ROC) curves with areas under the curves (AUCs) and respective 95% confidence intervals (95% CI).
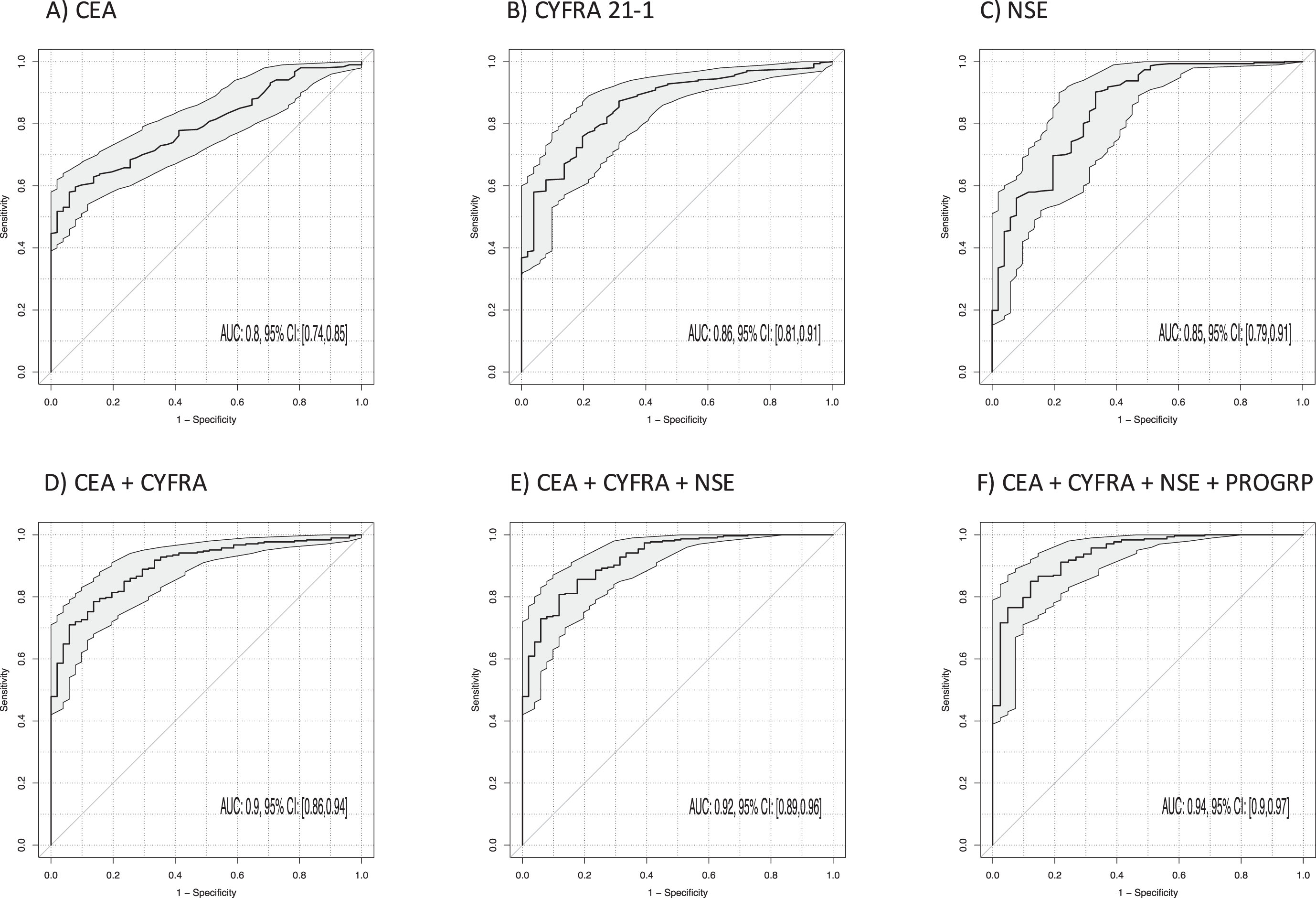
3.6Discrimination of SCLC and benign lung diseases
The best discrimination between SCLC and BLD (Table 3, Fig. 5) was achieved by NSE (AUC 0.96; 95% CI 0.93–0.98; p < 0.001) and ProGRP (AUC 0.89; 95% CI 0.84–0.94; p < 0.001). The AUC was slightly increased by the two-marker combination of NSE and ProGRP to 0.97 (95% CI 0.95–0.99; p < 0.001). The three-marker combination of NSE, ProGRP and CEA did not show improvement in discrimination further (AUC 0.97; 95% CI 0.95–0.99; p < 0.001). The best sensitivities at 90% specificity for single markers were 88% for NSE, 81% for ProGRP, and 92% for the combination of NSE and ProGRP. The addition of more biomarkers yielded only a minor improvement in sensitivity, reaching 94%.
Fig. 5
Power of discrimination between patients with small cell lung cancer (SCLC) and benign lung diseases (BLD) by use of single tumor markers and combinations illustrated by receiver operating characteristic (ROC) curves with areas under the curves (AUCs) and respective 95% confidence intervals (95% CI).
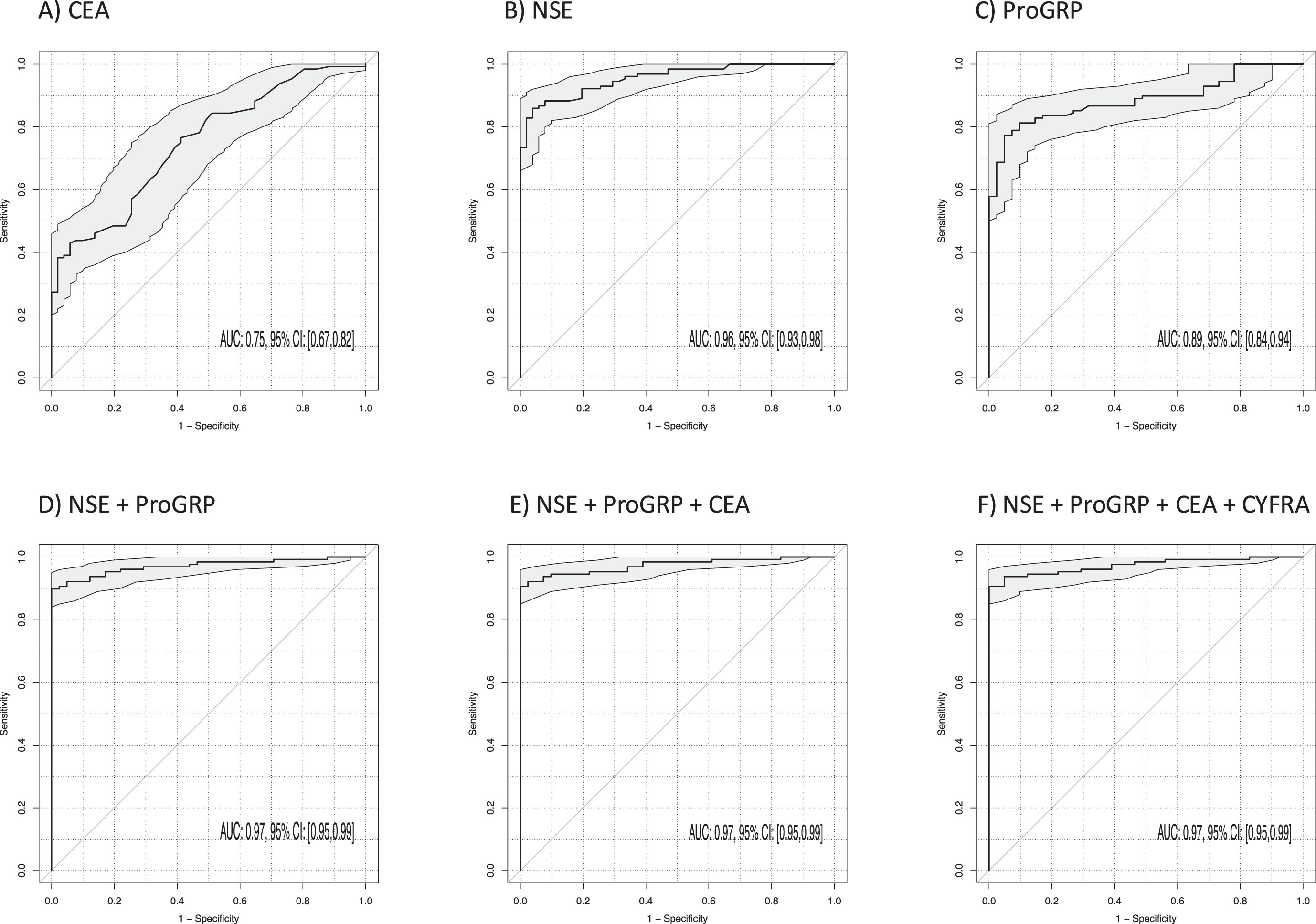
3.7Discrimination of NSCLC and SCLC
The most relevant for the support of the histological subtyping in clinical differential diagnosis is the discrimination of SCLC from NSCLC. The best discrimination between SCLC and NSCLC (Table 3, Fig. 6) was achieved by ProGRP (AUC 0.86; 95% CI 0.81–0.91; p < 0.001) and NSE (AUC 0.83; 95% CI 0.78–0.88; p < 0.001). The AUC was increased by the two-marker combination of ProGRP and NSE to 0.90 (95% CI 0.86–0.94; p < 0.001) and further increased to 0.93 by the three-marker combination of ProGRP, NSE and CYFRA 21-1 (95% CI 0.91–0.96; p < 0.001) (Fig. 6). The best sensitivities at 90% specificity for single markers were 70% for ProGRP, 58% for NSE and 80% for the combinations of ProGRP and NSE as well as 88% for ProGRP, NSE and CYFRA 21-1. The sensitivity was not increased by a four-marker combination with CA 72-4 (87%) and an AUC of 0.94 (95% CI 0.92–0.97; p < 0.001).
Fig. 6
Power of discrimination between patients with small cell lung cancer (SCLC) and non-small cell lung cancer (NSCLC) by use of single tumor markers and combinations illustrated by receiver operating characteristic (ROC) curves with areas under the curves (AUCs) and respective 95% confidence intervals (95% CI).
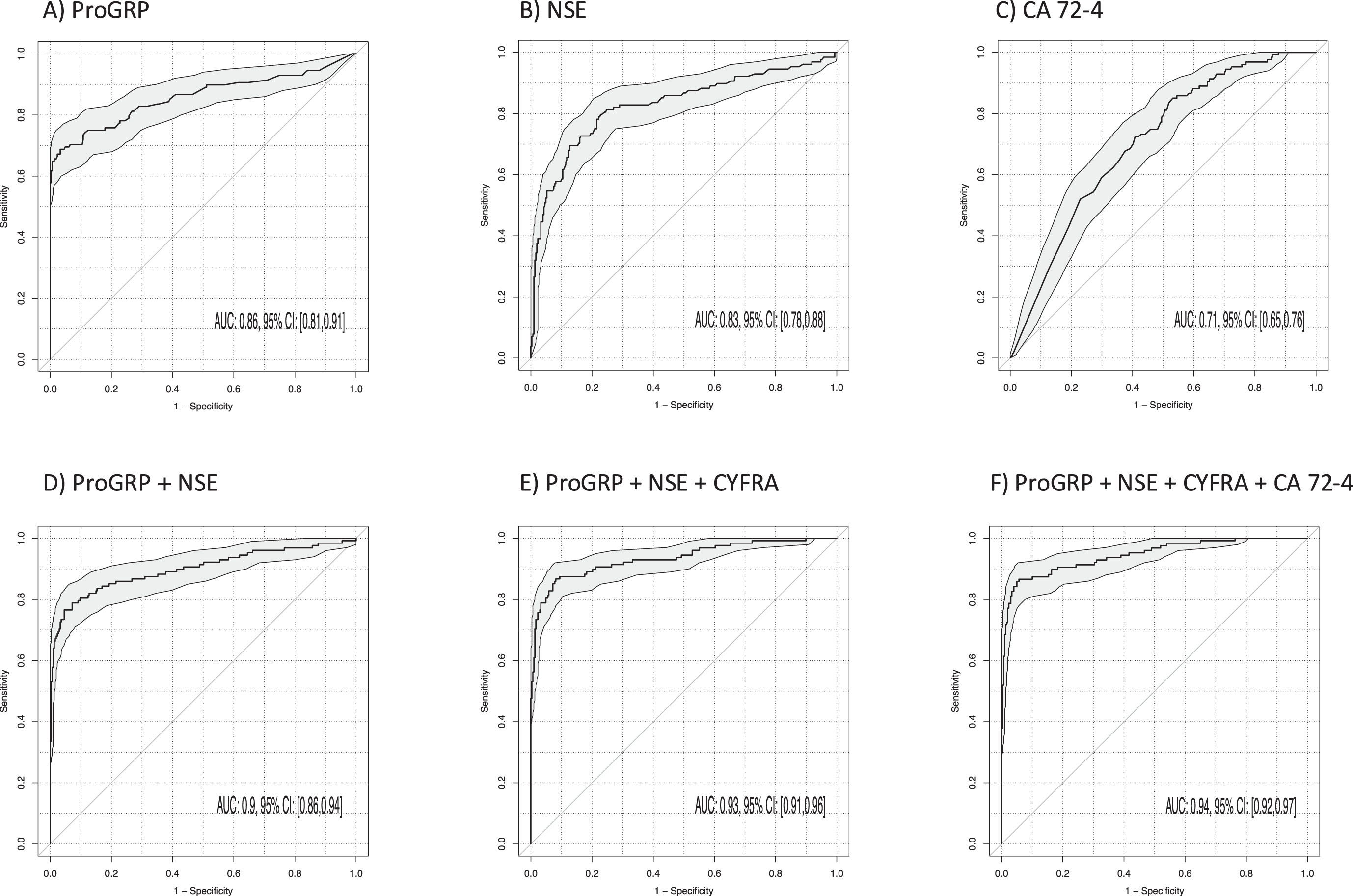
3.8Discrimination of squamous and adenocellular NSCLC
To further classify NSCLC into squamous cell carcinoma and adenocarcinoma, the discriminative capability was found to be moderately effective, as indicated in Supplementary Table 1. The best markers for the classification of adenocarcinoma were low SCC and high CA 15-3 values, both showing an area under the curve of 0.75 (95% CI 0.69–0.82; p < 0.001). However, their sensitivity at a 90% specificity threshold was only 37% for SCC and 24% for CA 15-3. Their combination enhanced the discriminative ability to an AUC of 0.86 (95% CI 0.81–0.91; p < 0.001), with a sensitivity of 60% at 90% specificity.
3.9Discrimination between localized and metastatic cancer disease
The serum tumor markers investigated for discriminating between localized and metastatic disease in NSCLC (Supplementary Table 1, Supplementary Figure 2), as well as between limited and extensive disease in SCLC (Supplementary Table 1, Supplementary Figure 3), demonstrated low AUC and sensitivity values at a specificity threshold of 90%, and were slightly enhanced by a combination of markers.
4Discussion
While elevated protein tumor markers can be observed in both healthy individuals and patients with other diseases, their association is particularly strong with malignant processes [12]. In this setting, the well-known lung cancer markers, CYFRA 21-1, CEA, NSE and ProGRP, but also the adenocellular markers, CA 125, CA 15-3 and CA 19-9, are significantly elevated in lung cancer patients compared to patients with benign lung disease, serving as differentiating, diagnostically relevant control group. Our results demonstrated that a combination of three markers, namely CYFRA 21-1, CEA, and NSE is more effective than any of these markers used individually, with a sensitivity of 79% for lung cancer detection at 90% specificity with an AUC of 0.93. Interestingly, the combination of CYFRA 21-1, ProGRP and NSE performed best for discriminating between NSCLC and BLD with a sens90 of 74%. Even higher is the AUC reaching 0.97 and a sens90 of 92% for discriminating between SCLC and BLD for the combination of NSE and ProGRP, outperforming single STM measurements. The most remarkable finding is the excellent discrimination of the histological subtypes of NSCLC and SCLC by the three-marker combination of ProGRP, NSE and CYFRA 21-1, with an AUC of 0.93 and a sensitivity of 88% at 90% specificity. The differentiation is of great significance as it guides the administration of distinct treatments, and lung cancer tissue biopsy may either be impractical or yield inconclusive outcomes [21–23]. Hence, the use of serum biomarkers provides valuable support in the histological subtyping of a tumor. In our study, we observed that the AUC and the sensitivity at a clinically relevant high specificity of 90% vs controls often further improved when we examined an increasing number of biomarkers in combination. Nevertheless, it is important to consider a specific clinical question and find a balance between cost-effectiveness and the gain in diagnostic information when considering the inclusion of additional biomarkers in clinical practice.
A recent study [24] explored the value of five STMs in 390 patients younger than 45 years with pulmonary nodules. No significant discrimination of malignant from benign nodules was evident and single or combined investigation showed limited value for diagnosis of lung cancer. In our study, patients were older with a median age of 62, which better reflects the overall situation of lung cancer patients. In line with our results, Wang et al. [25] examined a panel of six tumor markers and found significantly higher levels of CEA, CYFRA 21-1, NSE, CA 125 and ProGRP in lung cancer patients compared to BLD and healthy controls, however this was not observed for SCC. Furthermore, especially ProGRP and NSE showed a good discriminative ability between NSCLC and SCLC, in accordance with our results. Barchiesi et al. [26] conducted a small prospective investigation which validated the distinguishing capacity of NSE and, particularly, ProGRP (with a sensitivity of 82.4% and a specificity of 93.3%). This capability was further improved when both were used in combination (86.7% sensitivity at 96.6% specificity). Another study by Gruber et al. [27] highlighted the excellent discrimination between lung cancer and benign diseases using a score consisting of age, CYFRA 21-1, CEA and NSE, outperforming single markers alone. In addition, this study also found the best discrimination between NSCLC and SCLC by a score of ProGRP, NSE and CYFRA 21-1. One of the most comprehensive studies [28] involving 3144 patients admitted to the Hospital Clinic at Barcelona with suspicion of having lung cancer, used a set of six STMs, CYFRA 21-1, CEA, NSE, ProGRP, SCC and CA 15-3, combined in a score. This score achieved better sensitivity, specificity, negative predictive value, and positive predictive value (88.5%, 82%, 83.7%, and 87.3%, respectively) than each STM considered individually. This increased the diagnostic performance of a clinical model that included tumor size, age, and smoking status. In addition, the impressive performance of ProGRP and NSE was proven for the discrimination between NSCLC and SCLC.
Our study confirms these results and extends the approach to other STMs, such as CA 125, CA 19-9 and CA 72-4, that are more often used for adenocellular cancers of other origins [11, 29].
Interestingly, they show satisfactory performance for the differentiation between squamous and adenocellular subtypes of lung cancer, as values are significantly elevated in adenocarcinoma – similarly to CEA and CA 15-3 – while CYFRA 21-1 and SCC values are higher in the squamous cell subtype. This may become relevant in the future, as targeted tyrosine kinase inhibitor therapies are mainly applied in adenocarcinoma with molecular changes in tumor cell growth pathways, such as EGFR or ALK mutations, while squamous cell cancers are more susceptible to immune checkpoint inhibitor therapies [30–32]. However, the discriminatory ability displayed by AUCs and sensitivities at 90% specificity is only moderate, highlighting the need for further investigation in larger cohorts in future studies. Disease severity is best demonstrated by NSE and CYFRA 21-1, with significantly higher levels in both NSCLC and SCLC, however the discriminative ability in ROC curve analysis was limited. It should be noted that patients with limited or localized disease were primarily UICC stage 3B, and the discriminative ability for disease severity may potentially be stronger between patients with stage 1 or 2 and those with metastatic disease.
The study has several limitations, such as its retrospective design, the inclusion of a larger lung cancer group and a smaller benign control group, the small size of the histological subgroups, as well as the absence of a validation cohort. Since the time of data collection, noteworthy modifications have been made to the WHO classification of lung tumors [33, 34], reflecting the evolving understanding of lung tumor biology, diagnostic techniques, and treatment approaches. As our investigation primarily focused on distinguishing between cancer and benign disease, identifying subtypes, as well as differentiating between localized and metastatic disease (limited and extensive disease), and the WHO classifications of 2001 and 2015 have not changed for these aspects. Therefore, the core issues of our study were not substantially affected by the changes in the classification system, as the main groups and parameters we examined remained generally consistent. Furthermore, we primarily included late-stage lung cancer patients, where higher STM levels are expected. However, this reflects the current situation in which lung cancer is mainly diagnosed and systemically treated in an internistic oncologicalsetting.
On the other hand, it has to be emphasized that we included patients with benign lung disease as the clinically most relevant control cohort for differential diagnosis. Furthermore, samples were handled and analyzed in a standardized procedure regarding collection, storage, treatment prior to measurement and quality-controlled analysis and evaluation. In addition, the data interpretation was conducted independently of clinical and laboratory data acquisition, and guidelines for Reporting Diagnostic Accuracy studies (STARD) [35, 36] have been applied.
Nonetheless, the multifaceted potential of tumor markers in lung cancer, ranging from diagnostic and utility and disease progression monitoring to treatment response prediction and prognostic significance, highlights the need for further research in this field, to guide treatment decisions and follow-up care [37]. For instance, with the advent of liquid profiling and multianalyte blood testing methodologies, such as Cancer SEEK [38], a promising strategy for early detection and monitoring by evaluating the levels of circulating proteins alongside cell-free DNA mutations has been presented.
5Conclusion
The present study demonstrated the power of combinations of multiple tumor markers for enhanced diagnostic accuracy of lung cancer, especially when analyzing specific histological subgroups. This could help improve the detection, histological differentiation, and management of lung cancer, which is essential when biopsy results are not available or heterogenous cancers with mixed histologies are present. Alongside well-established lung cancer tumor markers, the study demonstrated the ability of additional adenocellular biomarkers to aid in improved histological subtyping.
Acknowledgments
The authors have no acknowledgments.
Author contributions
CONCEPTION: IT, SH
DATA ACQUISITION: JP, SH
INTERPRETATION OR ANALYSIS OF DATA: IT, FK, SH
PREPARATION OF THE MANUSCRIPT: IT, SH
REVISION FOR IMPORTANT INTELLECTUAL CONTENT: IT, FK, JP, SH
SUPERVISION: SH
Conflict of interest
IT, FK and JP have no conflict of interest to report. SH has received research funding or honoraria from Roche, BMS, Merck, Sysmex, Thermo, Volition and Instand. SH is also an editorial board member of Tumor Biology and an editor of the special issue Lung Cancer Tumor Markers but had no involvement in the peer review process of this manuscript.
Supplementary material
[1] The supplementary material is available in the electronic version of this article: https://dx.doi.org/10.3233/TUB-230021.
References
[1] | International Agency for Research on Cancer (IARC) [homepage on the Internet]. Lyon: Global Cancer Observatory, 2008 [cited 2023 Jan 28]. Available from: https://gco.iarc.fr/. |
[2] | World Health Organization (WHO) [homepage on the Internet]. Geneva: Global Health Estimates: Life expectancy and leading causes of death and disability [cited 2022 April 27]. Available from: https://www.who.int/data/gho/data/themes/mortality-and-global-health-estimates. |
[3] | Bade BC , Dela Cruz CS Lung cancer Epidemiology, etiology, and prevention. Clin Chest Med. (2020) ;41: (1):1–24. doi: 10.1016/j.ccm.2019.10.001. |
[4] | Siegel RL , Miller KD , Fuchs HE , Jemal A Cancer statistics, 2022. CACancer J Clin. (2022) ;72: :7–33. doi:10.3322/caac.21708. |
[5] | Smith BD , Smith GL , Hurria A , Hortobagyi GN , Buchholz TA Future of cancer incidence in the United States: Burdens upon an aging, changing nation. J Clin Oncol. (2009) ;27: (17):2758–65. doi:10.1200/JCO.2008.20.8983. |
[6] | National Cancer Institute (NIH) [homepage on the Internet]. USA: SEER Cancer Statistics Review, 1975-2018 [cited 2022 May 17]. Available from: https://seer.cancer.gov/csr/19752018/index.html. |
[7] | Duma N , Santana-Davila R , Molina JR Non–small cell lung cancer: Epidemiology, screening, diagnosis, and treatment. Mayo Clin Proc. (2019) ;94: (8):1623–40 doi:10.1016/j.mayoc2019.01.013 |
[8] | Guida F , Sun N , Bantis LE , Muller DC , Li P , Taguchi A , et al. Assessment of lung cancer risk on the basis of a biomarker panel of circulating proteins. JAMA Oncol. (2018) ;4: (10):e182078. doi:10.1001/jamaoncol.2018.2078. |
[9] | Becker N , Motsch E , Trotter A , Heussel CP , Dienemann H , Schnabel PA ,et al. Lung cancer mortality reduction by LDCT screening—Results from the randomized German LUSI trial. Int J Cancer. (2020) ;146: (6):1503–13. doi:10.1002/ijc.32486. |
[10] | Blons H , Garinet S , Laurent-Puig P , Oudart J-B Molecular markers and prediction of response to immunotherapy in non-small cell lung cancer, an update. J Thorac Dis. (2019) ;11: :S25–36. doi:10.21037/jtd.2018.12.48. |
[11] | Holdenrieder S Biomarkers along the continuum of care in lung cancer. Scand J Clin Lab Invest Suppl. (2016) ;245: :S40–5. doi:10.1080/00365513.2016.1208446. |
[12] | Molina R , Holdenrieder S , Auge JM , Schalhorn A , Hatz R , Stieber P Diagnostic relevance of circulating biomarkers in patients with lung cancer. Holdenrieder S, Stieber P, Herausgeber. Cancer Biomark. (2010) ;6: (3-4):163–78. doi:10.3233/CBM-2009-0127. |
[13] | Raso MG , Bota-Rabassedas N , Wistuba II Pathology and classification of SCLC. Cancers. (2021) ;13: (4):820. doi:10.3390/cancers13040820. |
[14] | Nicholson AG , Tsao MS , Beasley MB , Borczuk AC , Brambilla E , Cooper WA , et al. The WHO classification of lung tumors: Impact of advances since 2015. J Thorac Oncol Off Publ Int Assoc Study Lung Cancer. (2022) ;17: (3):362–87. doi:10.1016/j.jtho.2021.11.003. |
[15] | Galli G , Rossi G Lung cancer histology-driven strategic therapeutic approaches. Shanghai Chest. (2020) ;4: :29–29. doi:10.21037/shc.2020.01.03. |
[16] | Liu L , Teng J , Zhang L , Cong P , Yao Y , Sun G , et al. The combination of the tumor markers suggests the histological diagnosis of lung cancer. BioMed Res Int. . (2017) ;2017: :1–9. doi:10.1155/2017/2013989. |
[17] | Mauro C , Passerini R , Spaggiari L , Galetta D , Radice D , Lentati P , et al. New and old biomarkers in the differential diagnosis of lung cancer: Pro-gastrin-releasing peptide in comparison with neuron-specific enolase, carcinoembryonic antigen, and CYFRA 21-1. Int J Biol Markers. (2019) ;34: (2):163–7. doi:10.1177/1724600819834235. |
[18] | Yang D-W , Zhang Y , Hong Q-Y , Hu J , Li C , Pan B-S , et al. Role of a serum-based biomarker panel in the early diagnosis of lung cancer for a cohort of high-risk patients: Biomarker Panel for Lung Cancer Diagnosis. Cancer. (2015) ;121: :3113–21. doi:10.1002/cncr.29551. |
[19] | Yang Y , Xu M , Huang H , Jiang X , Gong K , Liu Y , et al. Serum carcinoembryonic antigen elevation in benign lung diseases. Sci Rep. (2021) ;11: (1):19044. doi:10.1038/s41598-021-98513-8. |
[20] | Brambilla E , Travis WD , Colby TV , Corrin B , Shimosato Y The new World Health Organization classification of lung tumours. Eur Respir J. (2001) ;18: (6):1059–68. doi:10.1183/09031936.01.00275301. |
[21] | Chouaid C , Dujon C , Do P , Monnet I , Madroszyk A , Le Caer H , et al. Feasibility and clinical impact of re-biopsy in advanced non small-cell lung cancer: A prospective multicenter study in a real-world setting (GFPC study 12-01). Lung Cancer. (2014) ;86: (2):170–3. doi:10.1016/j.lungcan.2014.08.016. |
[22] | Jamal-Hanjani M , Wilson GA , McGranahan N , Birkbak NJ , Watkins TBK , Veeriah S , et al. Tracking the evolution of non–small-cell lung cancer. N Engl J Med. (2017) ;376: :2109–21. doi:10.1056/NEJMoa1616288. |
[23] | Rolfo C , Mack PC , Scagliotti GV , Baas P , Barlesi F , Bivona TG , et al. Liquid biopsy for advanced non-small cell lung cancer (NSCLC): A statement paper from the IASLC. J Thorac Oncol. (2018) ;13: (9):1248–68. doi:10.1016/j.jtho.2018.05.030. |
[24] | Xu L , Su Z , Xie B Diagnostic value of conventional tumor markers in young patients with pulmonary nodules. J Clin Lab Anal. (2021) ;35: (9):e23912. doi:10.1002/jcla.23912. |
[25] | Wang L , Wang D , Zheng G , Yang Y , Du L , Dong Z , et al. Clinical evaluation and therapeutic monitoring value of serum tumor markers in lung cancer. Int J Biol Markers. (2016) ;31: :80–7. doi:10.5301/jbm.5000177. |
[26] | Barchiesi V , Simeon V , Sandomenico C , et al. Circulating progastrin-releasing peptide in the diagnosis of Small Cell Lung Cancer (SCLC) and in therapeutic monitoring. J Circ Biomark. (2021) ;10: :9–13. doi:10.33393/jcb.2021.2212. |
[27] | Gruber C , Hatz R , Reinmiedl J , Nagel D , Stieber P CEA, CYFRA 21-1, NSE, and ProGRP in the diagnosis of lung cancer: A multivariate approach / CEA, CYFRA 21-1, NSE und ProGRP in der Diagnostik des Lungenkarzinoms: Eine multivariate Analyse. J Lab Med. (2008) ;32: (5):361–71. doi:10.1515/JLM.2008.050. |
[28] | Molina R , Marrades RM , Augé JM , Escudero JM , Viñolas N , Reguart N , et al. Assessment of a combined panel of six serum tumor markers for lung cancer. Am J Respir Crit Care Med. (2016) ;193: (4):427–37. doi:10.1164/rccm.201404-0603OC. |
[29] | Stieber P , Heinemann V Sinnvoller Einsatz von Tumormarkern / Sensible use of tumor markers. J Lab Med. (2008) ;32: (5):339–60. doi:10.1515/JLM.2008.015. |
[30] | Santos ES , Rodriguez E Treatment considerations for patients with advanced squamous cell carcinoma of the lung. Clin Lung Cancer. (2022) ;23: (6):457–66. doi:10.1016/j.cllc.2022.06.002. |
[31] | Friedlaender A , Banna G , Malapelle U , Pisapia P , Addeo A Next generation sequencing and genetic alterations in squamous cell lung carcinoma: Where are we today? Front Oncol. (2019) ;9: :166. doi:10.3389/fonc.2019.00166. |
[32] | Niu Z , Jin R , Zhang Y , Li H Signaling pathways and targeted therapies in lung squamous cell carcinoma: Mechanisms and clinical trials. Signal Transduct Target Ther. (2022) ;7: (1):353. doi:10.1038/s41392-022-01200-x. |
[33] | Nicholson AG , Tsao MS , Beasley MB , et al. The WHO classification of lung tumors: Impact of advances since 2015. J Thorac Oncol. (2022) ;17: (3):362–87. 10.1016/j.jtho.2021.11.003. |
[34] | Travis WD , Brambilla E , Nicholson AG , et al. The World HealthOrganization classification of lung tumors: Impact of genetic,clinical and radiologic advances since the classification. JThorac Oncol. (2015) ;10: (9):1243–60. doi:10.1097/JTO.0000000000000630. |
[35] | Cohen JF , Korevaar DA , Gatsonis CA , Glasziou PP , Hooft L , Moher D , et al. STARD for Abstracts: Essential items for reporting diagnostic accuracy studies in journal or conference abstracts. BMJ. (2017) j3751: . doi:10.1136/bmj.j3751. |
[36] | Cohen JF , Korevaar DA , Altman DG , Bruns DE , Gatsonis CA , Hooft L , et al. STARD guidelines for reporting diagnostic accuracy studies: Explanation and elaboration. BMJ Open. (2016) ;6: (11):e012799. doi:10.1136/bmjopen-2016-012799. |
[37] | Holdenrieder S , Wehnl B , Hettwer K , Simon K , Uhlig S , Dayyani F Carcinoembryonic antigen and cytokeratin-19 fragments for assessment of therapy response in non-small cell lung cancer: A systematic review and meta-analysis. Br J Cancer. (2017) ;116: (8):1037–45. doi:10.1038/bjc.2017.45. |
[38] | Cohen JD , Li L , Wang Y , Thoburn C , Afsari B , Danilova L , et al. Detection and localization of surgically resectable cancers with a multi-analyte blood test. Science. (2018) ;359: (6378):926–30. doi:10.1126/science.aar3247. |