Preliminary research of the classification of the brain acute stroke lesions by the Diffusion Kurtosis Imaging (DKI) and Diffusion Weighted Imaging (DWI) parameters
Abstract
BACKGROUND:
Diffusion-weighted magnetic resonance imaging (DWI) is a mature scanning technique. With high sensitivity in detecting cerebral infractions, it has become an essential part of the clinical evaluation of acute stroke. However, with the update in medical ideals and treatment, clinicians are now focusing on distinguishing between reversible and irreversible brain tissue damage rather than detecting ischaemic lesions alone.
OBJECTIVE:
We supposed that Diffusion Kurtosis Imaging (DKI) could classify heterogeneous DWI lesions, deepening the understanding of tissue injury. We systematically studied the different parameters of DKI in acute stroke patients in the literature.
METHODS:
We collected 41 patients (26 male, 15 female), including different infarctions with acute cerebral infarction in different brain regions. Of all patients, 20 were single-infarction, while others were multi-infarctions. In this paper, we categorized acute cerebral infarction lesions into two types according to the parametric characteristics of both DKI and DWI. Type I means the DKI and DWI were matched, and Type II means the DKI and DWI were mismatched. Based on each parametric map, the region of interest (ROI) is outlined in each most severe lesion area (as large as possible in the center of the lesion). In the control group, ROIs of the same size are located in the corresponding regions of the contralateral hemisphere.
RESULTS:
In both Type I and Type II, all parameters conform to a normal distribution. An independent sample T-test was used to compare the differences between each group. In Type I, we found the FA, MD, Da, Dr, MK and Ka values were statistically different (
CONCLUSION:
DKI, compared to DWI, can provide more imaging information about intracranial ischemic infarction, which can deepen the understanding of the mechanism of ischemic tissue damage. Our classification of the brain acute stroke lesions by DKI parameters and DWI may help us rediscover the real core of infraction.
1.Introduction
Diffusion-weighted magnetic resonance imaging (DWI) is a mature scanning technique. With high sensitivity in detecting cerebral infractions, it has become an essential part of the clinical evaluation of acute stroke [1, 2]. However, with the update in medical ideals and treatment, clinicians are now focusing on distinguishing between reversible and irreversible brain tissue damage rather than detecting ischaemic lesions alone.
The former research has shown that the mismatch between diffusion-weighted imaging (DWI) and perfusion-weighted imaging (PWI) shows superior sensitivity to ischemic brain injury [1, 2]. Nevertheless, the significance of mismatched tissues is unclear. The status of mismatched tissues depends on many complex factors, such as the duration and nature of the ischemic injury and the proximity of patent vessels. In a word, perfusion diffusion mismatch only approximates a genuine ischemic hemi-dark band. Despite the drawbacks, this mismatch is usually used to guide clinical decision-making in diagnosing and treating acute stroke [3, 4, 5]. Thus, more and more methods are explored to help clinicians better understand ischemic brain injury.
Diffusion kurtosis imaging (DKI) is a new diffusion-weighted sequence approach that measures the non-Gaussianity of water diffusion, which can be used to characterize the complexity or heterogeneity of the tissue microenvironment. Compared with 3D q-space imaging, DKI has low requirements in imaging time, hardware requirements, and post-processing [4]. Thus, DKI has been shown to detect microstructural brain tissue changes in the ageing brain, acute stroke, and tumors.
We systematically studied the different parameters of DKI in acute stroke patients and the normal experiments in the literature. DKI, compared with DWI, has more parameters that can provide more information about the infarct core. We also followed the prognosis of patients with different types. We supposed that DKI could stratify heterogeneous DWI lesions, improving the characterization of tissue injury. In the literature, the characteristics of DKI have been systematically studied in acute stroke patients.
2.Method
2.1Ethics statement
The study was approved by the institutional review board (CWO) of the Second Affiliated Hospital of Xiamen Medical University. All patients provided written informed consent.
2.2Subjects
We collected 41 patients (26 male, 15 female) with acute cerebral infarction, including 86 different infarctions in different areas of the brain. All in all, 20 patients were single-infarction, and others were multi-infarctions. All patients received magnetic resonance imaging (MRI) scans within 72 h of stroke onset. Acute cerebral infarction patients were included. However, the patients with cerebral hemorrhage (original or secondary), cerebral tumors, degenerative brain disease, craniocerebral trauma, post-craniocerebral operation, dyspnea, and coma were excluded.
2.3Classification
In this paper, according to the manifestations of DKI and DWI parameters, the acute cerebral infarction lesions were classified into two categories. Type I means both the signals and areas on DKI and DWI were matched and Type II means either the signals and areas on DKI and DWI were mismatched. We collected 45 Type I and 41 Type II respectively (Figs 1 and 2).
Figure 1.
Type I: both DWI and DKI were positive, which means matched. A: DWI; B: DKI; C: ADC.
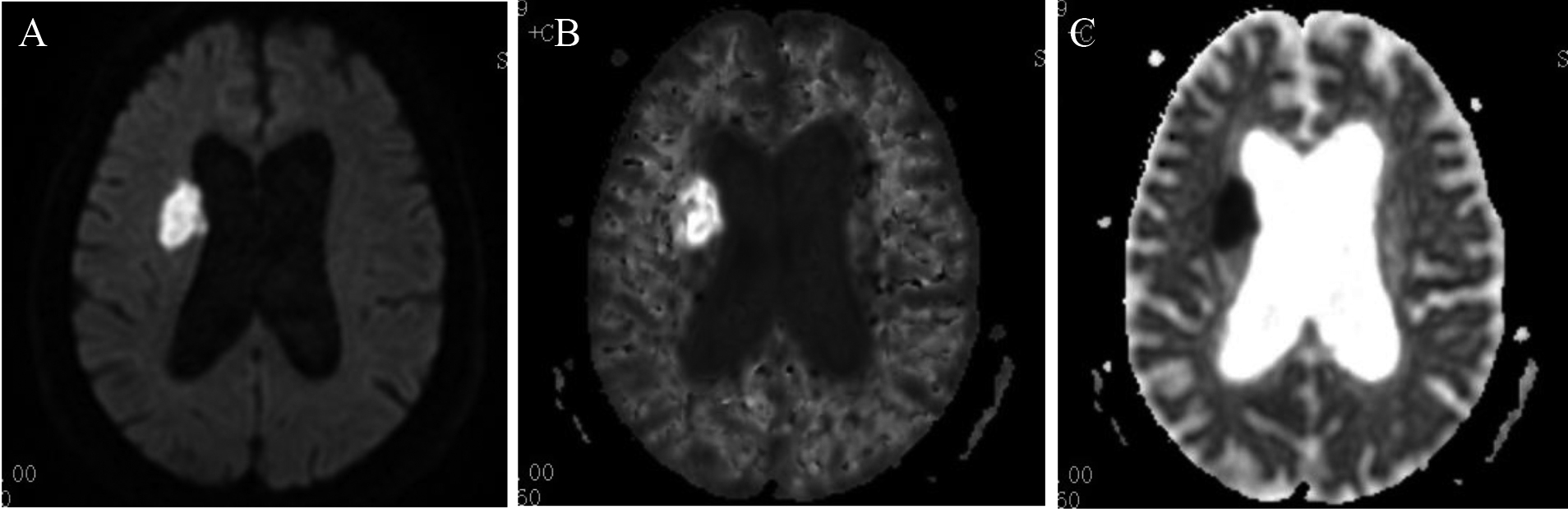
Figure 2.
Type II: DWI was positive, while DKI was negative, which means mismatched. A: DWI; B: DKI; C: ADC.
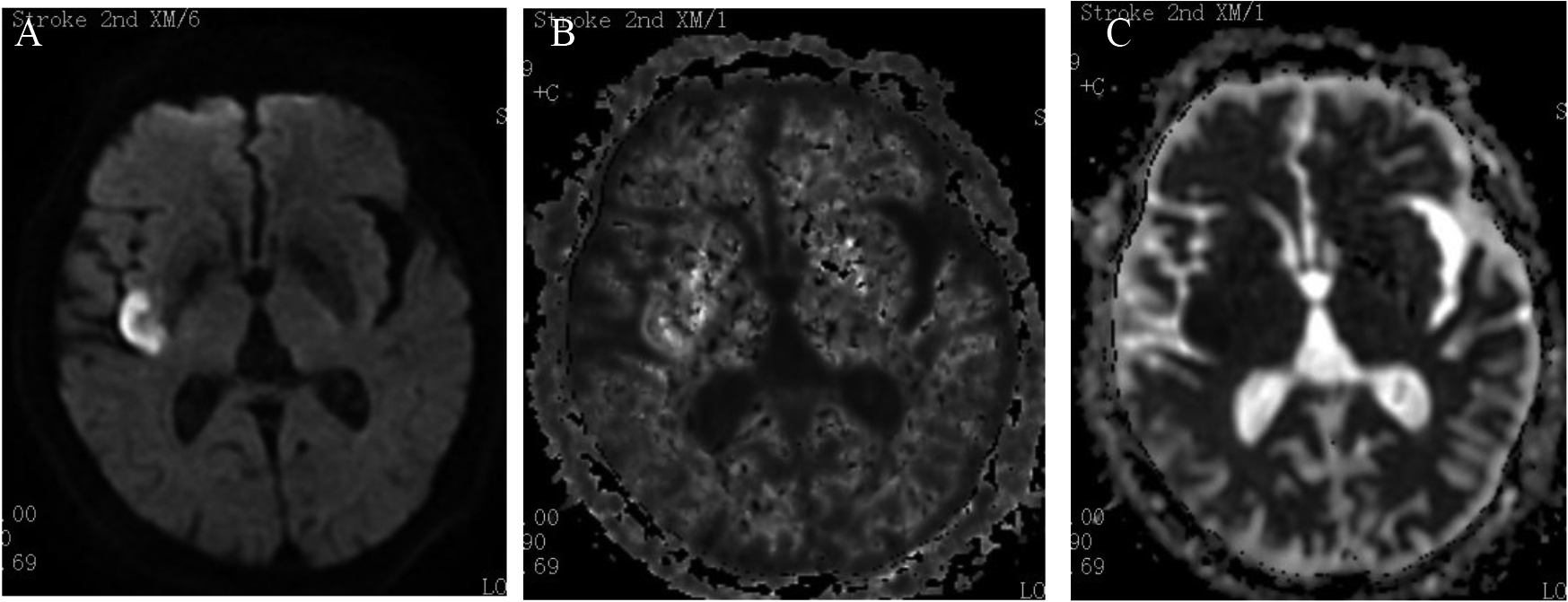
2.4Imaging acquisition
We used a GE 1.5T HDx Echo Speed Plus MRI scanner and an 8-channel head coil (GE Healthcare Life Sciences, Chalfont, UK). The scanning sequences included T1 weighted image (T1WI), T2 weighted image (T2WI), T2 fluid attenuated inversion recovery (T2 FLAIR) imaging, DWI and DKI. DWI sequence b has a value of 1000 SEC/mm2. The DKI sequence scanning parameters are as follows: repetition time (TR) is 6000 ms, echo time (TE) is the minimum, the layer thickness is 5 mm, layer spacing is 1.5 mm, the field of view (FOV) is 240
2.5Imaging analysis
DKI post-processing software provided by GE company was used to post-process DKI images. Regions of interest (ROIs) (as large as possible in the focal center) were outlined within the most severe areas of each lesion according to each independent parametric map. The same size ROIs were located in the corresponding region of the contralateral hemisphere in the control groups (Fig. 3). Measure all ROIs’ multiple DKI parameters (MK, Kr and Ka). The diagnosis and image analysis of ischemic lesions by two radiologists is a single-blind study. A third is consulted when two leading radiologists disagree.
Table 1
The means of different parameters of DKI in the experiment group and the control group
Mean | FA | MD | Da | Dr | MK | Ka | Kr |
---|---|---|---|---|---|---|---|
Tpye I, experiment | 0.23 | 0.66 | 0.82 | 0.58 | 1.22 | 1.33 | 1.16 |
Type I, control | 0.41 | 1.02 | 1.50 | 0.78 | 0.78 | 0.82 | 0.93 |
Type II, experiment | 0.32 | 0.96 | 1.24 | 0.82 | 1.12 | 1.17 | 1.08 |
Type II, control | 0.44 | 0.97 | 1.51 | 0.74 | 0.79 | 0.79 | 1.08 |
Figure 3.
Regions of interest (ROIs) (as large as possible in the focal center) were outlined within the most severe areas of each lesion according to each independent parametric map. The same size ROIs were located in the corresponding region of the contralateral hemisphere in control groups.
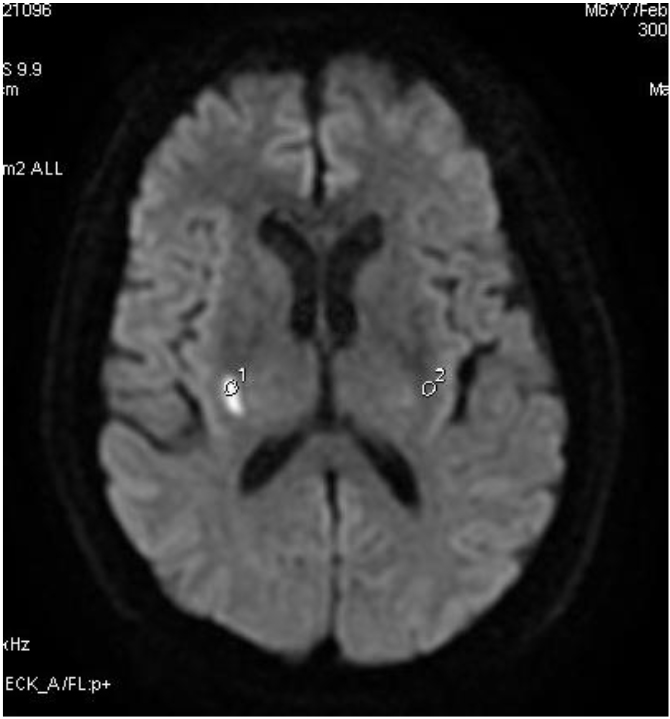
2.6Statistical analysis
The measured DKI parameters were analyzed by independent sample t-test. We used SPSS 13.0 software (SPSS, Inc., Chicago, IL, USA) to analyze all data.
3.Results
3.1Patient characteristics.
In this paper, we collected 41 acute ischemic stroke patients, including 26 males and 15 females aged 63.4
3.2Parametric maps of DKI in different types
In both Type I and Type II, all parameters follow the normal distribution, and the independent sample t-test was used to compare the differences between each group. The FA, MD, Da and Dr values were decreased compared with the normal contralateral group, while the values of MK, Ka and Kr were increased remarkably in Table 1.
In Type I, we found the FA, MD, Da, Dr, MK and Ka values were statistically different (
4.Discussion
Diffusion-weighted imaging (DWI) is the most reliable neuroimaging technique to assess acute ischemic stroke [5, 6]. Nowadays, DWI has been extensively used in acute stroke patients because of its high sensitivity. DWI has its deficit: it cannot reflect the actual tissue damage of the acute stroke lesions, which are uneven and may partially recover during treatment [7]. The ischemic core manifests that the diffusion-weighted image showed high intensity, and the apparent diffusion coefficient was significantly reduced (ADC). ADC is usually maintained at low levels 4 days after stroke and then gradually pseudo-normalized. In the subacute or chronic stage, the value of ADC becomes normal or high [8, 9]. There is a fundamental limitation of ADC which can only analyze the date approximating Gaussian distribution. However, the injury tissue of cerebral infarction conforms to non-Gaussian distribution.
DKI is an extension of DWI and DTI technology, an emerging MR technology based on the principle of non-Gaussian diffusion movement of water molecules in vivo. It can quantitatively describe the non-Gaussian diffusion characteristics of water molecules inside and outside cells and provide more prosperous, more authentic and accurate tissue microstructure information than DWI and DTI technology [8, 9]. DKI technology was first proposed by Professor Jenson of New York University in 2005. In recent years, DKI has been gradually applied to study diseases of various systems, especially in the brain, prostate and other tumors have achieved preliminary results, showing good clinical value [8, 9, 10].
DKI introduces the fourth-order kurtosis K value to quantify the degree of restriction of the non-Gaussian diffusion movement of water molecules in tissues. The reference of higher-order statistics can better reflect the signal characteristics of the tissue itself [11]. In addition to the diffusion parameters, the main parameters that the DKI model can obtain include: mean kurtosis, MK, a parameter reflecting the degree of diffusion restriction of water molecules in the tissue; indicators reflecting anisotropy-kurtosis anisotropy (KA); indicators that reflect the direction of parallel or vertical maximum eigenvalues-axial kurtosis, AK or K//) and radial kurtosis (RK or K). Due to the high sensitivity and specificity of DKI in reflecting the microstructure characteristics of tissues, it has excellent clinical application potential [12].
Because of different cell membranes and biochemical characteristics, biological tissues shift the diffusion of water molecules to the normal distribution and become non-Gaussian. The magnitude of water molecule diffusion deviation from Gaussian distribution can be quantified by a dimensionless scale, that is, excess kurtosis. This parameter can quantify the degree of water molecule diffusion restriction and diffusion inhomogeneity, thereby reflecting the situation of water molecule diffusion hindered or limited. If
The diffusion kurtosis coefficient (K) is a quantitative measure of non-Gaussian properties of white matter (WM) and grey matter (GM); Because of the multiple barriers like cellular/axonal membranes, organelles, and or chambers, the water diffusion in biological tissue is limited; thus, it results in positive K [13].
The increase in K of the ischemic injuries may indicate an increase in the water microenvironment’s complexity or heterogeneity in WM and GM [14]. MK is the most critical parameter of DKI technology, defined as the average of diffusion kurtosis in all directions, and is considered to be an indicator of the complexity of tissue microstructure [15]. Compared with DWI, MK has the advantage of not relying on the spatial orientation of the tissue structure and can be used in both the grey and white matter in the brain.
The size of MK depends on the complexity of the tissue microstructure in the region of interest. The more complex the structure (e.g., the lower the degree of differentiation of cancer cells and the greater the cell density), the more significant the spread of non-Gaussian water molecules, and the larger the MK [16].
KA is somewhat similar to FA, evolving according to the standard deviation of kurtosis. The KA value represents the degree to which water molecules tend to diffuse anisotropy. That is, the larger the value, the more obvious the degree of tendency [17].
The former study showed that, in experimental models of traumatic brain injury, in which nerve tissue was impacted in the subacute phase, MK was elevated in the pseudo-normalization of MD and FA [18]. Studies have shown that an increase in MK is associated with higher reactive astrocyte proliferation, confirming K as a tissue heterogeneity biomarker. Similar results have been observed in previous patient and animal stroke DKI preliminary studies.
In other words, K is an in vivo measure of microenvironmental complexity and inhomogeneity, which can provide complementary information to the traditional diffusion index and may be a more sensitive biomarker for exploring pathophysiological changes [17, 18].
In diagnosing of acute ischemic stroke, DKI can further reflect the hemodynamic and tissue metabolic damage severity information based on DWI. At the same time, it can effectively judge the changes and prognosis of brain tissue structure in patients with acute ischemic stroke in short-term treatment. DKI indexes can potentially reflect tumor cell density and cell proliferation characteristics. The indicators obtained by DKI imaging can be used as a non-invasive clinical tool with high diagnostic accuracy to identify low and high-grade gliomas or distinguish different histological subtypes of tumors.
5.Conclusion
Our findings suggest that DWI/DKI mismatches may reflect mild injury and potentially recoverable ischemic lesions, while matched regions may indicate irreversible cell damage. In our studies, MK and Ka were significantly different in Type II, which may mean the real infraction core in the mismatch acute store patients.
DKI, compared to DWI, provides more helpful information about acute ischemic cerebral infraction, which can deepen the understanding of ischemic tissue injury. Our classification of the brain acute stroke lesions by DKI parameters and DWI may do a favor to a new understanding of the real infraction core.
Ethics statement
The study was approved by the institutional review board (CWO) of the Second Affiliated Hospital of Xiamen Medical University. All patients provided written informed consent.
Availability of data and material
All datasets related to the current study are available from the corresponding author upon reasonable request.
Author contributions
Xin Liu and Gen Yan conceived and designed the experiments; Xin Liu, Fang Chen and Lei Wang performed the experiments and collected the data; Xin Liu wrote the paper; Bo Zheng help analyze the data. All authors read and approved the final manuscript.
Acknowledgments
This research was supported by the Natural Science Foundation of Fujian Province (No. 2022J01139).
Conflict of interest
The authors have no conflicts of interest to declare.
References
[1] | Wittsack H-J, Ritzl A, Fink GR, et al., MR Imaging in Acute Stroke: Diffusion-weighted and Perfusion Imaging Parameters for Predicting Infarct Size. Radiology. (2002) ; 222: : 397-403. |
[2] | Yin J, Sun H, Wang Z, et al. Diffusion Kurtosis Imaging of Acute Infarction: Comparison with Routine Diffusion and Follow-up MR Imaging. Radiology. (2018) ; 287: (2): 651-657. |
[3] | Chen Y, Zhao X, Ni H, Feng J, Ding H, Qi H, Wan B, Ming D. Parametric mapping of brain tissues from diffusion kurtosis tensor. Comput Math Methods Med. (2012) ; 820847: : 2012. |
[4] | Jensen JH, Falangola MF, Hu C, Tabesh A, Rapalino O, Lo C, Helpern JA. Preliminary observations of increased diffusional kurtosis in human brain following recent cerebral infarction. NMR Biomed. (2011) ; 24: : 452-457. |
[5] | Zhu L-H, Zhang Z-P, Wang F-N, et al., Diffusion kurtosis imaging of microstructural changes in brain tissue affected by acute ischemic stroke in different locations. Artical Research. (2019) ; 14: (2): 272-279. |
[6] | Hui ES, Fieremans E, Jensen JH, Tabesh A, Feng W, Bonilha L, Spampinato MV, Adams R, Helpern JA. Stroke assessment with diffusional kurtosis imaging. Stroke. (2012) ; 43: : 2968-2973. |
[7] | Guo Y-L, Li S-J, Zhang Z-P, et al., Parameters of diffusional kurtosis imaging for the diagnosis of acute cerebral infarction in different brain regions. Experimental and Therapeutic Medicine. (2016) ; 12: : 933-938. |
[8] | Zhou IY, Guo Y, Igarashi T, et al., Fast diffusion kurtosis imaging (DKI) with Inherent COrrelation-based Normalization (ICON) enhances automatic segmentation of heterogeneous diffusion MRI lesion in acute stroke. NMR in Biomedicin. (2016) ; 29: (12): 1670-1677. |
[9] | Steven AJ, Zhuo J, Melhem ER. Diffusion kurtosis imaging: an emerging technique for evaluating the microstructural environment of the brain. AJR Am J Roentgenol. (2014) ; 202: (1): W26-33. |
[10] | Weber RA, Hui ES, Jensen JH, Nie X, Falangola MF, Helpern JA, et al., Diffusional kurtosis and diffusion tensor imaging reveal different time-sensitive strokeinduced microstructural changes. Stroke. (2015) ; 46: (2): 545-50. |
[11] | Zhang S, Yao Y, Shi J, et al., The temporal evolution of diffusional kurtosis imaging in an experimental middle cerebral artery occlusion (MCAO) model. Magn Reson Imaging. (2016) ; 34: (7): 889-95. |
[12] | Hui ES, Du F, Huang S, et al., Spatiotemporal dynamics of diffusional kurtosis, mean diffusivity and perfusion changes in experimental stroke. Brain Res. (2012) ; 1451: : 100-9. |
[13] | Wu EX, Cheung MM. MR diffusion kurtosis imaging for neural tissue characterization. NMR Biomed. (2010) ; 23: : 836-848. |
[14] | Zhuo J, Xu S, Proctor JL, et al., Diffusion kurtosis as an in vivo imaging marker for reactive astrogliosis in traumatic brain injury. Neuroimage. (2012) ; 59: : 467-477. |
[15] | Kamagata K, Tomiyama H, Motoi Y, et al., Diffusional kurtosis imaging of cingulate fibers in Parkinson disease: comparison with conven-tional diffusion tensor imaging. Magn Reson Imaging. (2013) ; 31: : 1501-1506. |
[16] | Hui ES, Du F, Huang S, et al., Spatiotemporal dynamics of diffusional kurtosis, mean diffusivity and perfusion changes in experimental stroke. Brain Res. (2012) ; 1451: : 100-109. |
[17] | Raab P, Hattingen E, Franz K, et al., Cerebral gliomas: diffusional kur-tosis imaging analysis of microstructural differences. Radiology. (2010) ; 254: : 876-881. |
[18] | Rosenkrantz AB, Pahani AR, Chenevert TL, et al., Body diffusion kur-tosis imaging: basic principles, applications, and considerations for cli-nical practice. J Magn Reson Imaging. (2015) ; 42: : 1190-1202. |