The impact of artificial intelligence in the diagnosis and management of acoustic neuroma: A systematic review
Abstract
BACKGROUND:
Schwann cell sheaths are the source of benign, slowly expanding tumours known as acoustic neuromas (AN). The diagnostic and treatment approaches for AN must be patient-centered, taking into account unique factors and preferences.
OBJECTIVE:
The purpose of this study is to investigate how machine learning and artificial intelligence (AI) can revolutionise AN management and diagnostic procedures.
METHODS:
A thorough systematic review that included peer-reviewed material from public databases was carried out. Publications on AN, AI, and deep learning up until December 2023 were included in the review’s purview.
RESULTS:
Based on our analysis, AI models for volume estimation, segmentation, tumour type differentiation, and separation from healthy tissues have been developed successfully. Developments in computational biology imply that AI can be used effectively in a variety of fields, including quality of life evaluations, monitoring, robotic-assisted surgery, feature extraction, radiomics, image analysis, clinical decision support systems, and treatment planning.
CONCLUSION:
For better AN diagnosis and treatment, a variety of imaging modalities require the development of strong, flexible AI models that can handle heterogeneous imaging data. Subsequent investigations ought to concentrate on reproducing findings in order to standardise AI approaches, which could transform their use in medical environments.
1.Introduction
Acoustic neuromas (AN) alternatively referred to as acoustic neurinoma, vestibular schwannoma, or acoustic neurofibroma is gradually developing tumours that arise from the Schwann cell sheaths. They often reside in the cerebellopontine angle and are juxtaposed to either extra-axially or intracranially next to the vestibular or cochlear nerve [1, 2]. AN is the most common benign tumor and its incidence has been reported to increase in recent years [3, 4]. The occurrence of AN has been found more in women than men [4]. In the US, the prevalence of AN is
The importance of AI and machine learning in the medical sciences is highlighted by these developments, which open the door to more precise, effective, and scalable healthcare solutions.
Given its power and flexibility of usage, in the current systematic review, we explore the role of AI in changing the landscape of diagnosis and subsequent management of AN in future.
2.Methods
The systematic review was performed according to the Preferred Reporting Items for Systematic Reviews and Meta-Analyses [23].
2.1Search strategy
A systematic review of peer-reviewed literature in databases including PubMed, Embase, Cochrane Library, Web of Science, Library and Information Science Abstracts (LISA), Scopus, and the National Library of Medicine was carried out until December 2023. We employed a search strategy utilizing a combination of search words, including ‘Acoustic Neuroma’ AND ‘Artificial Intelligence’ OR ‘machine learning’. Besides, another search strategy with the keywords acoustic neuroma’ AND ‘artificial intelligence’ OR ‘machine learning’ OR ‘deep learning’ along with Saudi Arabia as ‘affiliation’ or ‘title’ to search for the papers published from the Kingdom of Saudi Arabia. Based on the title and abstract, post-screening, all the eligible articles were reviewed further for data extraction. The literature search followed PICO strategy (P: any population irrespective of gender diagnosed with acoustic neuroma, I: neuroimaging for the diagnosis and management of AN, C: traditional methods, O: usefulness of AI in the diagnosis of AN.
2.2Inclusion criteria and exclusion criteria
The included studies were selected on the following criteria: (i) published as an original article irrespective of ethnicity and gender, using any AI or deep learning tools for the diagnosis and the management of AN reporting their clinical, epidemiological, molecular, and genetic features. The published articles with irrelevant or incomplete information, or in languages other than English, were excluded from the analysis.
2.3Data extraction
The following keywords were used to thoroughly screen the eligible papers: “acoustic neuroma”, “vestibular schwannoma”, “acoustic neurofibroma”, “artificial intelligence”, “years”, “Saudi Arabia”, “incidence”, “imaging”, “MRI”, “CT scan”, “rate”, “diagnosis”, “management”, “deep learning”, “machine learning”, “neurological tumour”.
2.4Risk of bias assessment
The quality of all selected studies was assessed using modified Joanna Briggs Institute’s (JBI) Critical Appraisal Checklists for Studies (Fig. 1). The risk of bias in a study was considered high if the “yes” score was
Table 1
Details of the studies included in the systematic review with risk of bias assessment
S. No | Author (year) | Studt type (N) | Main finding | RBA score |
---|---|---|---|---|
1 | Shapey et al. (2019) [28] | P ( | The authors report an AI framework for delineating and calculating the volume of AN tumour with accuracy to an independent human annotator. | 4 |
2 | Lee et al. 2020 [33] | R ( | The authors developed a single-pathway U-Net and a two-pathway U-Net model and found that later outperformed the former. | 4.5 |
3 | Windisch et al. 2020 [36] | P ( | The authors report a neural network that differentiates between MRI slices containing either a glioblastoma, a AN, or no tumour. | 4 |
4 | Abouzari, et al. 2020 [31] | P ( | The authors report that, unlike logistic regression models, their constructed ANN model was superior in sensitivity and specificity in predicting patient-answered AN recurrence. | 4.5 |
5 | Lee et al. (2021) [24] | P ( | The authors report a framework for evaluating the treatment responses using a novel volumetric measurement algorithm, that can also be used longitudinally in patients with AN following GKRS. | 4.5 |
6 | Yang et al. (2021) [25] | R ( | Based on the pre-radio-surgical radiomics, the authors proposed a machine-learning model potentiated to predict the pseudo-progression and AN outcome following GKRS. | 4 |
7 | Chakrabarty et al. 2021 [45] | R ( | Authors developed a CNN model capable of accurately classifying 6 different types of brain tumours, and discriminating between the images from pathologic and healthy tissue. | 3.5 |
8 | George-Jones et al. 2021 [37] | P ( | Authors developed a CNN, that detects AN growth with its potential application in AN surveillance. | 3.5 |
9 | Huang et al. 2021 [38] | R ( | The authors created an algorithm that automatically distinguishes between the solid and cystic tumour components of AN. | 4 |
10 | Yao et al. 2022 [51] | R ( | The authors demonstrated that using deep learning, postoperative AN can be accurately segmented without human intervention. | 3 |
11 | Kujawa et al. (2022) [26] | P ( | The authors report an AI framework beneficial for automatically classifying AN based on Koos scale with an accuracy comparable to that of trained neurosurgeons. | 4 |
12 | Neve et al. (2022) [27] | R ( | The authors report a CNN model that could accurately detect and delineate AN, and differentiate the clinically relevant variance between extra and intra-meatal tumour fragments. | 4 |
13 | Chai et al. 2022 [32] | R ( | The authors proposed DPGAN, a three-stage hierarchical generative adversarial network that performs better for generating quality compared to other cutting-edge GAN-based techniques. In addition, it effectively improves semantic segmentation of biological images as well. | 3 |
14 | Cas et al. 2022 [44] | R ( | In order to calculate vestibular schwannoma volumes without operator input, authors, built a deep learning system by combining transformers and convolutional neural networks. | 3.5 |
15 | Va Bechem et al. 2022 [30] | P ( | The authors developed an AI-PREM tool that organized and quantified patient feedback and reduced the time invested by healthcare professionals to evaluate and prioritize patient experiences without any limitation of closed-ended questions. | 4.5 |
16 | Dorent et al. 2023 [34] | P ( | The authors established an unsupervised cross-modality segmentation model to perform unilateral AN and bilateral cochlea segmentation automatically. | 4 |
17 | Zhang et al. 2023 [39] | P ( | The authors report a novel AI model named ACP-TransUNet based on the improved TransUNet structure, using data from MRI for segmentation of AN in the cerebellopontine angle region. | 4 |
18 | Wang et al. 2023 [40] | P ( | Authors report a deep learning model associated with clinical manifestations and multi-sequence MRI for short-term postoperative functioning of facial nerve in patients with AN. | 3.5 |
Table 1, continued | ||||
---|---|---|---|---|
S. No | Author (year) | Studt type (N) | Main finding | RBA score |
19 | Wang et al. 2023 [41] | P ( | The authors reported a deep multi task model with an advanced learning effectiveness achieving promising performance on tumour enlargement prediction and segmentation. | 4 |
20 | Wang 2023 [42] | R ( | The authors developed a 3D CNN model for automated AN segmentation with a reasonably good performance. | 4.5 |
21 | Lee 2023 [43] | R ( | Lee et al. proposed a deep learning-based tumour segmentation method applicable for multiple types of intracranial tumours including AN, meningioma and brain metastasis undergoing GKRS. | 4 |
22 | Teng et al. 2023 [35] | P ( | Authors have presented a novel, high-performance deep learning model for brain extraction on T1CE MR scans. | 3.5 |
23 | Neve et al. 2023 [47] | R ( | The authors report automated 2D diameter measurements of AN on MRI and found it as accurate as human 2D measurements. | 3.5 |
24 | Caio Neves et al. 2023 [48] | P ( | The authors used deep learning method to evaluate ANs and their spatial relationships with the ipsilateral inner ear in MRI and found that deep learning system can segment AN and inner ear structures in high-resolution MRI scans with a promising accuracy. | 4 |
25 | Wu et al. 2023 [50] | R ( | The authors present a unique brain tumour image synthesis and segmentation network (TISS-Net) that achieves high-performance end-to-end brain tumour segmentation and synthesised target modality. | 3.5 |
26 | Yu et al. 2023 [46] | P ( | The authors proposed a DNN that shows that, unlike brain tissue oedema, predictors of tumour diameter, volume, and surface area had significant prognostic value in AN surgical outcome. | 3 |
27 | Neve et al. 2023 [29] | P ( | Based on a survey study authors reported that compared to the close-ended question PREM, the open-ended question, PREM provides more precise and in-depth details on the patient experience in AN care. | 4 |
28 | Shapey et al. 2021 [49] | P ( | Here, we provide the first publicly available annotated imaging dataset of VS by releasing the data and annotations used in our prior work. | 2.5 |
AI: artificial intelligence; ANN: artificial neural network; CNN: convolutional neural network; DNN: deep neural network; DPGAN: data pair generative adversarial network; GKRS: Gama Knife radio-surgery; PREM: Patient-reported experience measures, TISS: Tumor Image Synthesis and Segmentation network; P: Prospective study; R: Retrospective study. The risk bias assessment (RBA) score was quantified with these questions: Q1. Were the criteria for inclusion in the sample clearly defined? Q2. Were the study subjects and the setting described in detail? Q3. Was the exposure measured in a valid and reliable way? Q4. Were objective, standard criteria used for measurement of the condition? Q5. Were confounding factors identified? Q6. Were strategies to deal with confounding factors stated? Q7. Were the outcomes measured in a valid and reliable way? Q8. Was appropriate statistical analysis used? Q9. Is the model used publicly available? Q10. Is sample size reasonably good?
Figure 1.
PRISMA flow diagram for study selection process.
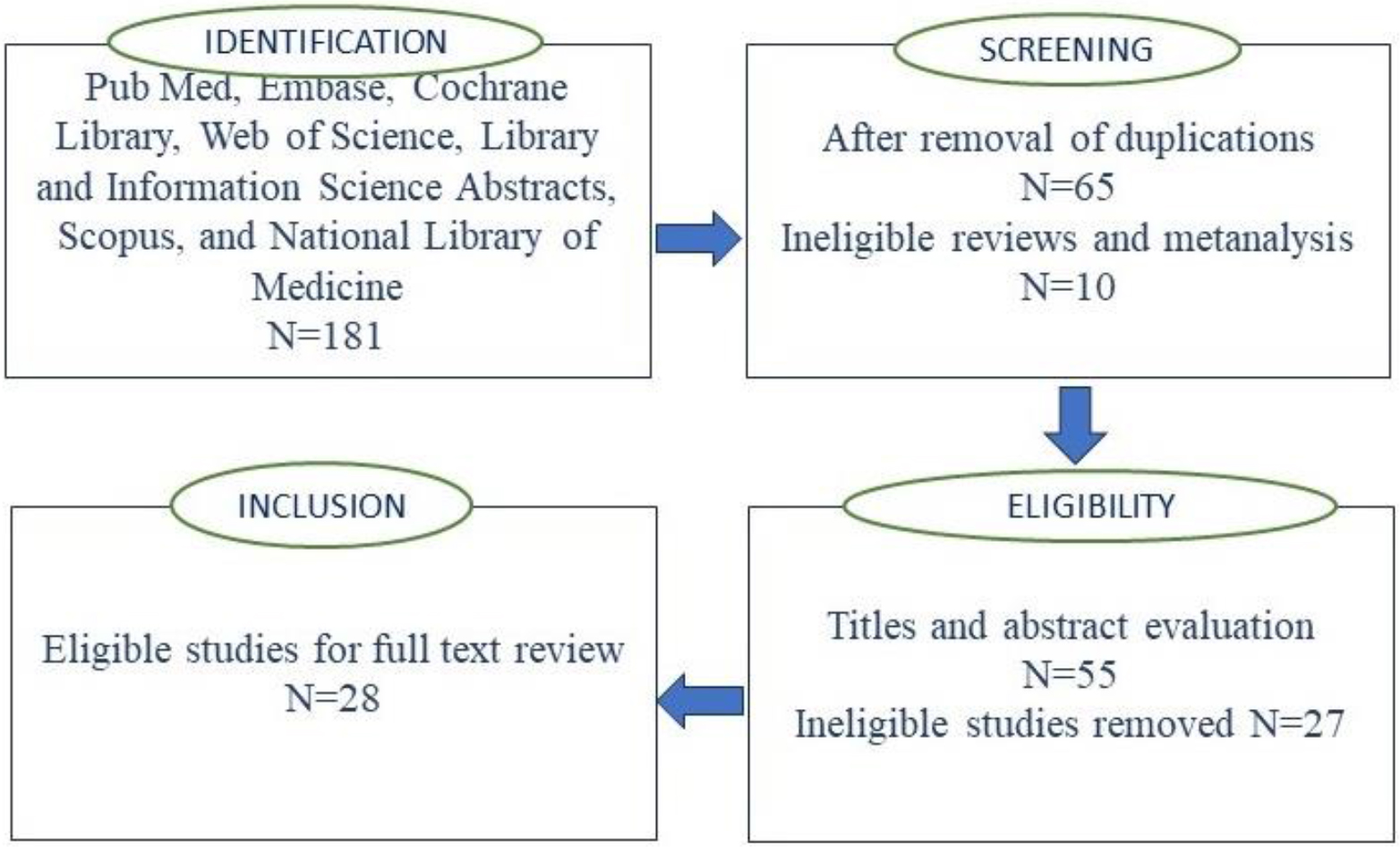
3.Results
Our search retrieved a total of 181 citations, and after the removal of duplicates, a total of 55 articles were retained. After abstract and title screening, based on incomplete and/or irrelevant information, 27 articles were excluded, and the remaining 28 studies [24, 25, 26, 27, 28, 29, 30, 31, 32, 33, 34, 35, 36, 37, 38, 39, 40, 41, 42, 43, 44, 45, 46, 47, 48, 49, 50, 51] were considered after a full evaluation of the manuscript. A flow diagram (following PRISMA guidelines of the search strategy are presented in Fig. 1. The details of the articles selected for the current systematic review are presented in Table 1. Among 28 studies reviewed and included in the current systematic review, 15 were based on the data obtained from prospective studies [24, 26, 28, 29, 30, 31, 34, 35, 36, 37, 39, 40, 41, 46, 48, 49] while 12 were based on the data obtained from the patients retrospectively and/or publicly available data sets [25, 27, 32, 33, 38, 42, 43, 44, 45, 47, 50, 51]. The artificial intelligence, and machine learning models proposed by all the authors suggest that such tools have a potential for non-invasive prediction of diagnosis, therapeutic and treatment outcomes for AN. Three studies evaluating the patient experiences, using Patient-Reported Experience Measures (PREMs) in predicting patient patient-answered outcomes found that the use of AI can play a pivotal role in AN management [31]. Reported that, unlike logistic regression models, their constructed AI model was superior in sensitivity and specificity in predicting patient-answered AN recurrence [30]. Developed an AI-PREM tool that organized and quantified patient feedback and reduced the time invested by healthcare professionals to evaluate and prioritize patient experiences without any limitation of closed-ended questions. A survey study by Neve et al. [29] found that unlike close-ended question PREM, the open-ended question PREM provides more precise and in-depth details on the patient experience in AN care.
In 2019 [28] developed the first fully automatic AI framework for delineating and calculating the volume of AN tumour with accuracy to an independent human annotator, while George-Jones et al. [37] developed a convolutional neural network (CNN), that detects AN growth with its potential application in AN surveillance. Three follow-up studies, by employing used advanced deep learning models to calculate AN volumes and tumour enlargement prediction by achieving promising performance as accurate as humans. Measurements [41, 44, 47, 36], developed a neural network that differentiated between MRI slices containing either a glioblastoma, a AN, or no tumor. The AI models developed by different groups could differentiate between solid and cystic tumour components of AN [38] delineate AN [27, 45], classify 6 different types of brain tumours, and healthy tissue [45] or differentiate variance between extra and intra-meatal tumour fragments [27]. Patient management and clinical workflow could be greatly enhanced by automatically segmenting AN from MRI [49, 51, 34] and Wang et al. [41] established an unsupervised cross-modality segmentation model to perform unilateral AN and bilateral cochlea segmentation automatically [39], report a novel AI model named ACP-TransUNet based on the improved TransUNet structure, and Wu, et al. [50] presented a high-performance brain tumour image synthesis and segmentation network (TISS-Net). Wang et al. [38] developed a 3D CNN model for automated AN segmentation only, while the segmentation model proposed by Lee et al. [43] is applicable for multiple types of intracranial tumours including AN, meningioma and brain metastasis undergoing gamma knife radio surgery (GKRS). Moreover, the AI model proposed by [26] could classify AN based on Koos scale with an accuracy comparable to that of trained neurosurgeons. AI has now also been used for evaluating the treatment responses [24] predict the pseudo-progression in patients with AN following GKRS [25]. The AI model reported by Wang et al. could delineate clinical manifestations and short-term postoperative functioning of facial nerve [41], or segment AN and ipsilateral inner ear structures in patients with AN [48]. Unlike brain tissue oedema, the deep neural network (DNN) proposed by Yu et al. [46] showed that, predictors of tumour diameter, volume, and surface area had significant prognostic value in AN surgical outcome. Chai et al. [32] proposed data pair generative adversarial network (DPGAN), a three-stage hierarchical generative adversarial network that performs better for generating quality compared to other cutting-edge GAN-based techniques and semantic segmentation of biological images as well.
4.Discussion
In the current systematic review, we observed that AI and deep learning methods and have been successfully developed and used for not only the estimations of AN volume and segmentations, but differentiating it from other tumor types and healthy tissues. More and more studies with uniformity in AI methods is likely to revolutionize its applications in the near future. Patient experiences are crucial to the standard of care. Limited studies have demonstrated the benefit of using open-ended PREM questions to gauge both positive and bad experiences to identify actionable targets for quality improvement. With a minimal human element, an important component in assessing the practicability of using open-source PREMs in clinical settings is the automated analysis of the documents to minimize labour. Use of AI in PREM has been found very helpful for the management of patients with AN as well [29, 30, 52]. However, more replicative studies are required to substantiate these findings in the future. Even if our results demonstrate the potential of AI in the detection and treatment of brain tumors, including AN, some limitations of this study should be taken into account:
1. Possible publication bias: Because studies with favorable results are more likely to be published than those with negative or inconclusive outcomes, our systematic review may have been influenced by publication bias. The evidence base may overrepresent research showing substantial or positive effects of AI applications, which might have an impact on the overall findings and interpretations.
2. Exclusion of non-English studies: Because we only considered studies written in English, relevant studies written in other languages might not have made it into the analysis. This language barrier may skew our results and restrict how far the findings may be applied. In order to offer a more thorough analysis, future evaluations ought to think about include non-English studies.
3. Certain included studies were retrospective: A sizable portion of the studies we included in our analysis used data that was collected in the past. In addition to frequently lacking the control over variables that prospective research offer, retrospective studies can introduce a variety of biases, including selection and recall bias. The results’ dependability and suitability for clinical practice are impacted by this constraint.
4. Generalization of AI models: Variations in imaging modalities and non-uniform datasets provide considerable hurdles to the generalization of AI models. We suggest using consistent imaging data standards and using a variety of datasets when training AI models to get over these restrictions. The robustness and practicality of AI in clinical practice can be improved by standardizing the procedures for gathering data and training models.
4.1Restrictions in clinical integration and implementation
There are various practical challenges to incorporating AI into healthcare practice, including:
1. Training needs for healthcare professionals: Healthcare personnel must receive training in the use of AI technology in order for it to be implemented effectively. This entails comprehending AI outputs, correctly interpreting findings, and incorporating AI tools into daily operations. It will be crucial to create initiatives for continual education and training programs.
2. Infrastructure requirements: In order to implement AI in healthcare settings, a significant amount of infrastructure is needed. This includes secure networks, sophisticated processing power, and data storage options. It is imperative to guarantee that healthcare institutions have the requisite technical infrastructure.
3. Patient acceptance: It’s critical that people trust and accept AI-driven healthcare solutions. In order to ensure transparency in the processes used to make AI decisions, efforts should be undertaken to educate patients about the advantages and limitations of AI. Successful integration will depend on addressing patient concerns and making sure AI tools are utilized to enhance rather than replace the clinician-patient connection.
4. Data collection and model training standardization: The standardization of data collection and model training is essential to overcome the challenges posed by variations in imaging modalities and non-uniform datasets, which hinder the ability to generalize AI models. We recommend adhering to standardized imaging data protocols and utilizing diverse datasets during the training of AI models to overcome these limitations. Standardizing the procedures for data collection and model training can enhance the resilience and effectiveness of AI in clinical practice.
4.2Ethical consideration
The application of AI technology in healthcare presents significant ethical issues in addition to technical ones. Prioritizing patient privacy and data security is necessary to guarantee the security of sensitive health information. To protect patient data, AI use in healthcare contexts should adhere to current laws and policies. Furthermore, in order to make sure that AI decision-making systems adhere to the values of justice, accountability, and openness, it is imperative that their ethical implications be thoroughly considered. It takes a team effort to solve these moral issues and successfully and ethically incorporate AI technologies into clinical practice in order to include them into AI.
Considering these drawbacks, our analysis shows how AI has the potential to have a big influence on AN diagnosis and treatment. Advanced AI models that can process diverse imaging data can be integrated to improve diagnosis accuracy and create individualized treatment regimens. To validate these results and guarantee their safe and efficient application in clinical settings, more study with standardized techniques and prospective designs is required.
AI algorithms have been trained to analyse MRI scans to detect and diagnose AN and identify patterns and characteristics associated with the presence of the tumour. Previous work published so far suggests that AI has the potential to significantly change current clinical practice by altering the way ANs are measured and managed. Irrespective of the tumour’s clinical manifestation, the differences between the outcomes of the suggested AI models developed by the researchers and the clinical measurements made by qualified radiologists have been found to be within the range considered clinically acceptable [24, 28]. Using MR images, studies have persistently addressed the issue of AN segmentation. Based on probability statistics, using Bayesian partial volume segmentation scheme [53] or deep learning like CNN, AI has been successfully employed in AN diagnosis and segmentation [28]. With excellent dice coefficients [28, 54] developed a 2.5D CNN and 2.5D U-Net, respectively, capable of generating automatic AN segmentation methods that did not require any user interaction.
Studies have shown that unlike conventional linear measurements for estimating tumour size, and volumetric measurement is more accurate and dependable for calculating the size of AN [55, 56]. Using AI to calculate AN volume, can be pivotal in clinical management, contouring tumours for radiosurgery treatment, and subsequent swift treatment planning [28, 42]. Unlike other methods, the TISS-Net AI tool [50], not only produced substantially higher and more accurate segmentation tumor assessment even without gadolinium-based scanning. This time-efficient tool minimizes the potentially harmful side effects of the gadolinium contrast agent. Moreover, CNN model proposed are capable of accurately classifying 6 different types of brain tumours, and discriminate between the images from pathologic and a healthy tissue [45]. While AI is likely to revolutionize the future diagnosis and management of most of diseases including AN, certain limitations associated with it also need to be considered. The primary limitation shared by most of the deep learning methods on image analysis is non-uniform datasets created with different imaging modalities and properties [28, 45]. The partial volume effects and the variation in the contrast intensity of AN and other tumors limit the use of AI models in generalisability [24]. The models proposed employ binary segmentation based on the synthesis of a missing modality, its efficacy on multi-class segmentation needs further research [50]. A significant number (44%) of studies included in the study were based on the data collected retrospectively. The inherent bias associated with these studies cannot be entirely ruled out. Moreover, while most of the studies were based on a reasonably large sample size, few included data from a few subjects only, warranting studies with larger sample sizes to verify relevant models. These results suggest that AI is a promising approach for the diagnosis and management of brain tumours including AN. The advances in computational biology strongly advocate that AI can be used in image recognition and diagnosis, radionics and feature extraction, clinical decision support, treatment planning, robot-assisted surgery, monitoring and follow-up, rehabilitation, and quality of life monitoring in most health conditions including AN. However, it is noteworthy that although AI presents significant potential in healthcare, its implementation should be undertaken in collaboration with healthcare professionals. The validation and incorporation of AI technologies need to be established and validated in the healthcare workflow to guarantee their safety, effectiveness, and ethical use.
5.Conclusion and future research recommendations
The development of advanced artificial intelligence models capable of integrating various imaging modalities is imperative for improving the diagnosis and treatment of acoustic neuromas, given the diverse imaging characteristics. Furthermore, it is anticipated that carrying out additional research using standardised AI methodologies will greatly expand the uses and efficacy of these technologies in the medical industry.
Even though our review shows a lot of progress in the use of AI to manage acoustic neuromas, there are still a few holes in the literature that need to be filled in order to improve this field even more: 1- standardization of data collection and model training as it uniform techniques for imaging data collection and the integration of varied datasets for AI model training must be developed. The robustness and generalizability of AI applications in clinical contexts might be improved by this standardization, 2- prospective studies; retrospective studies make up the majority of the research conducted nowadays, thus prospective studies should be the main emphasis of future research in order to reduce biases and produce more accurate results, 3- incorporating non-English studies where broadening the focus of systematic reviews to encompass non-English studies might yield a more all-encompassing comprehension of worldwide research endeavors and discoveries, 4- ethical considerations; that additional investigation is required to examine the ethical implications of artificial intelligence (AI) in healthcare, with a focus on patient privacy, data security, and the impartiality and openness of AI decision-making procedures, and 5- clinical implementation and validation; to guarantee the efficacy and safety of AI models, research should concentrate on their actual clinical application, including validation in real-world settings.
Future research can advance the field’s understanding and move towards more reliable and therapeutically useful AI solutions by filling up these gaps in the knowledge base.
Consent for publication
All authors reviewed the results, approved the final version of manuscript and agreed to publish it.
Data availability
The data come from literary studies that were searched, reviewed and published until December 2023.
Funding
This work is funded by Princess Nourah bint Abdulrahman University Researchers Supporting Project number (PNURS2024R 284), Princess Nourah bint Abdulrahman University, Riyadh, Saudi Arabia. With Regards.
Acknowledgments
The authors would like to show sincere thanks to those techniques who have contributed to this research. The authors extend their appreciation to Princess Nourah bint Abdulrahman University Researchers Supporting Project number (PNURS2024R 284), Princess Nourah bint Abdulrahman University, Riyadh, Saudi Arabia.
Conflict of interest
The authors report there are no competing interests to declare.
References
[1] | Greene J, Al-Dhahir MA. Acoustic neuroma (vestibular schwannoma). StatPearls, Treasure Island (FL): StatPearls. Available online: https://www.ncbi.nlm.nih.gov/books/NBK470177/(accessed on 20 December 2023), (2023) . |
[2] | Braunstein S, Ma L. Stereotactic radiosurgery for vestibular schwannomas. Cancer management and research. (2018) Sep 20: : 3733-40. |
[3] | Carlson ML, Link MJ. Vestibular schwannomas. New England Journal of Medicine. (2021) Apr 8; 384: (14): 1335-48. |
[4] | Propp JM, McCarthy BJ, Davis FG, Preston-Martin S. Descriptive epidemiology of vestibular schwannomas. Neuro-oncology. (2006) Jan 1; 8: (1): 1-1. |
[5] | Fisher JL, Pettersson D, Palmisano S, Schwartzbaum JA, Edwards CG, Mathiesen T, Prochazka M, Bergenheim T, Florentzson R, Harder H, Nyberg G. Loud noise exposure and acoustic neuroma. American journal of epidemiology. (2014) Jul 1; 180: (1): 58-67. |
[6] | Koo M, Lai JT, Yang EY, Liu TC, Hwang JH. Incidence of vestibular schwannoma in Taiwan from 2001 to 2012: a population-based national health insurance study. Annals of Otology, Rhinology & Laryngology. (2018) Oct; 127: (10): 694-7. |
[7] | Lin D, Hegarty JL, Fischbein NJ, Jackler RK. The prevalence of “incidental” acoustic neuroma. Archives of otolaryngology–head & neck surgery. (2005) Mar 1; 131: (3): 241-4. |
[8] | Gupta VK, Thakker A, Gupta KK. Vestibular schwannoma: what we know and where we are heading. Head and neck pathology. (2020) Dec; 14: (4): 1058-66. |
[9] | Berkowitz O, Iyer AK, Kano H, Talbott EO, Lunsford LD. Epidemiology and environmental risk factors associated with vestibular schwannoma. World neurosurgery. (2015) Dec 1; 84: (6): 1674-80. |
[10] | Chen M, Fan Z, Zheng X, Cao F, Wang L. Risk factors of acoustic neuroma: systematic review and meta-analysis. Yonsei medical journal. (2016) May 5; 57: (3): 776. |
[11] | Brodhun M, Stahn V, Harder A. Pathogenesis and molecular pathology of vestibular schwannoma. Hno. (2017) May; 65: : 362-72. |
[12] | Halliday J, Rutherford SA, McCabe MG, Evans DG. An update on the diagnosis and treatment of vestibular schwannoma. Expert review of neurotherapeutics. (2018) Jan 2; 18: (1): 29-39. |
[13] | Lees KA, Tombers NM, Link MJ, Driscoll CL, Neff BA, Van Gompel JJ, Lane JI, Lohse CM, Carlson ML. Natural history of sporadic vestibular schwannoma: a volumetric study of tumor growth. Otolaryngology–Head and Neck Surgery. (2018) Sep; 159: (3): 535-42. |
[14] | Carlson ML, Vivas EX, McCracken DJ, Sweeney AD, Neff BA, Shepard NT, Olson JJ. Congress of neurological surgeons systematic review and evidence-based guidelines on hearing preservation outcomes in patients with sporadic vestibular schwannomas. Neurosurgery. (2018) Feb 1; 82: (2): E35-9. |
[15] | Sheikh MM. De Jesus O. Vestibular schwannoma. Treasure Island, FL: StatPearls Publishing, (2021) . |
[16] | Ahmad RA, Sivalingam S, Topsakal V, Russo A, Taibah A, Sanna M. Rate of recurrent vestibular schwannoma after total removal via different surgical approaches. Annals of Otology, Rhinology & Laryngology. (2012) Mar; 121: (3): 156-61. |
[17] | Basu K, Sinha R, Ong A, Basu T. Artificial intelligence: How is it changing medical sciences and its future? Indian Journal of Dermatology. (2020) Sep 1; 65: (5): 365-70. |
[18] | Ahuja AS. The impact of artificial intelligence in medicine on the future role of the physician. Peer J. (2019) Oct 4; 7: : e7702. |
[19] | Ghaderzadeh M, Asadi F, Ramezan Ghorbani N, Almasi S, Taami T. Toward artificial intelligence (AI) applications in the determination of COVID-19 infection severity: considering AI as a disease control strategy in future pandemics. Iranian Journal of Blood and Cancer. (2023) Aug 30; 15: (3): 93-111. |
[20] | Fasihfar Z, Rokhsati H, Sadeghsalehi H, Ghaderzadeh M, Gheisari M. AI-driven malaria diagnosis: developing a robust model for accurate detection and classification of malaria parasites. Iranian Journal of Blood and Cancer. (2023) Aug 30; 15: (3): 112-24. |
[21] | Gheisari M, Ghaderzadeh M, Li H, Taami T, Fernández-Campusano C, Sadeghsalehi H, Afzaal Abbasi A. Mobile Apps for COVID-19 Detection and Diagnosis for Future Pandemic Control: Multidimensional Systematic Review. JMIR mHealth and uHealth. (2024) Feb 22; 12: : e44406. |
[22] | Jamshidi S, Mohammadi M, Bagheri S, Najafabadi HE, Rezvanian A, Gheisari M, Ghaderzadeh M, Shahabi AS, Wu Z. Effective Text Classification using BERT, MTM LSTM, and DT. Data & Knowledge Engineering. (2024) Apr 21: : 102306. |
[23] | Moher D, Liberati A, Tetzlaff J, Altman DG, PRISMA Group* T. Preferred reporting items for systematic reviews and meta-analyses: the PRISMA statement. Annals of internal medicine. (2009) Aug 18; 151: (4): 264-9. |
[24] | Lee CC, Lee WK, Wu CC, Lu CF, Yang HC, Chen YW, Chung WY, Hu YS, Wu HM, Wu YT, Guo WY. Applying artificial intelligence to longitudinal imaging analysis of vestibular schwannoma following radiosurgery. Scientific reports. (2021) Feb 4; 11: (1): 3106. |
[25] | Yang HC, Wu CC, Lee CC, Huang HE, Lee WK, Chung WY, Wu HM, Guo WY, Wu YT, Lu CF. Prediction of pseudoprogression and long-term outcome of vestibular schwannoma after Gamma Knife radiosurgery based on preradiosurgical MR radiomics. Radiotherapy and Oncology. (2021) Feb 1; 155: : 123-30. |
[26] | Kujawa A, Dorent R, Connor S, Oviedova A, Okasha M, Grishchuk D, Ourselin S, Paddick I, Kitchen N, Vercauteren T, Shapey J. Automated koos classification of vestibular schwannoma. Frontiers in radiology. (2022) Mar 10; 2: : 837191. |
[27] | Neve OM, Chen Y, Tao Q, Romeijn SR, de Boer NP, Grootjans W, Kruit MC, Lelieveldt BP, Jansen JC, Hensen EF, Verbist BM. Fully Automated 3D vestibular schwannoma segmentation with and without gadolinium-based contrast material: A multicenter, multivendor study. Radiology: Artificial Intelligence. (2022) Jun 22; 4: (4): e210300. |
[28] | Shapey J, Wang G, Dorent R, Dimitriadis A, Li W, Paddick I, Kitchen N, Bisdas S, Saeed SR, Ourselin S, Bradford R. An artificial intelligence framework for automatic segmentation and volumetry of vestibular schwannomas from contrast-enhanced T1-weighted and high-resolution T2-weighted MRI. Journal of neurosurgery. (2019) Dec 6; 134: (1): 171-9. |
[29] | Neve OM, van Buchem MM, Kunneman M, van Benthem PP, Boosman H, Hensen EF. The added value of the artificial intelligence patient-reported experience measure (AI-PREM tool) in clinical practise: Deployment in a vestibular schwannoma care pathway. PEC Innovation. (2023) Dec 15; 3: : 100204. |
[30] | van Buchem MM, Neve OM, Kant IM, Steyerberg EW, Boosman H, Hensen EF. Analyzing patient experiences using natural language processing: development and validation of the artificial intelligence patient reported experience measure (AI-PREM). BMC Medical Informatics and Decision Making. (2022) Jul 15; 22: (1): 183. |
[31] | Abouzari M, Goshtasbi K, Sarna B, Khosravi P, Reutershan T, Mostaghni N, Lin HW, Djalilian HR. Prediction of vestibular schwannoma recurrence using artificial neural network. Laryngoscope investigative otolaryngology. (2020) Apr; 5: (2): 278-85. |
[32] | Chai L, Wang Z, Chen J, Zhang G, Alsaadi FE, Alsaadi FE, Liu Q. Synthetic augmentation for semantic segmentation of class imbalanced biomedical images: A data pair generative adversarial network approach. Computers in Biology and Medicine. (2022) Nov 1; 150: : 105985. |
[33] | Lee WK, Wu CC, Lee CC, Lu CF, Yang HC, Huang TH, Lin CY, Chung WY, Wang PS, Wu HM, Guo WY. Combining analysis of multi-parametric MR images into a convolutional neural network: Precise target delineation for vestibular schwannoma treatment planning. Artificial Intelligence in Medicine. (2020) Jul 1; 107: : 101911. |
[34] | Dorent R, Kujawa A, Ivory M, Bakas S, Rieke N, Joutard S, Glocker B, Cardoso J, Modat M, Batmanghelich K, Belkov A. CrossMoDA 2021 challenge: Benchmark of cross-modality domain adaptation techniques for vestibular schwannoma and cochlea segmentation. Medical Image Analysis. (2023) Jan 1; 83: : 102628. |
[35] | Teng, Y., et al., Automated, fast, robust brain extraction on contrast-enhanced T1-weighted MRI in presence of brain tumors: an optimized model based on multi-center datasets. European Radiology, (2023) :pp. 1-10. |
[36] | Windisch P, Weber P, Fürweger C, Ehret F, Kufeld M, Zwahlen D, Muacevic A. Implementation of model explainability for a basic brain tumor detection using convolutional neural networks on MRI slices. Neuroradiology. (2020) Nov; 62: : 1515-8. |
[37] | George-Jones NA, Wang K, Wang J, Hunter JB. Automated detection of vestibular schwannoma growth using a two-dimensional U-Net convolutional neural network. The Laryngoscope. (2021) Feb; 131: (2): E619-24. |
[38] | Huang CY, Peng SJ, Wu HM, Yang HC, Chen CJ, Wang MC, Hu YS, Chen YW, Lin CJ, Guo WY, Pan DH. Quantification of tumor response of cystic vestibular schwannoma to Gamma Knife radiosurgery by using artificial intelligence. Journal of Neurosurgery. (2021) Oct 1; 1: (aop): 1-9. |
[39] | Zhang Z, Zhang X, Yang Y, Liu J, Zheng C, Bai H, Ma Q. Accurate segmentation algorithm of acoustic neuroma in the cerebellopontine angle based on ACP-TransUNet. Frontiers in Neuroscience. (2023) May 24; 17: : 1207149. |
[40] | Wang MY, Jia CG, Xu HQ, Xu CS, Li X, Wei W, Chen JC. Development and validation of a deep learning predictive model combining clinical and radiomic features for short-term postoperative facial nerve function in acoustic neuroma patients. Current Medical Science. (2023) Apr; 43: (2): 336-43. |
[41] | Wang K, George-Jones NA, Chen L, Hunter JB, Wang J. Joint vestibular schwannoma enlargement prediction and segmentation using a deep multi-task model. The Laryngoscope. (2023) Oct; 133: (10): 2754-60. |
[42] | Wang H, Qu T, Bernstein K, Barbee D, Kondziolka D. Automatic segmentation of vestibular schwannomas from T1-weighted MRI with a deep neural network. Radiation Oncology. (2023) May 8; 18: (1): 78. |
[43] | Lee WK, Yang HC, Lee CC, Lu CF, Wu CC, Chung WY, Wu HM, Guo WY, Wu YT. Lesion delineation framework for vestibular schwannoma, meningioma and brain metastasis for gamma knife radiosurgery using stereotactic magnetic resonance images. Computer Methods and Programs in Biomedicine. (2023) Feb 1; 229: : 107311. |
[44] | Cass ND, Lindquist NR, Zhu Q, Li H, Oguz I, Tawfik KO. Machine learning for automated calculation of vestibular schwannoma volumes. Otology & Neurotology. (2022) Dec 1; 43: (10): 1252-6. |
[45] | Chakrabarty S, Sotiras A, Milchenko M, LaMontagne P, Hileman M, Marcus D. MRI-based identification and classification of major intracranial tumor types by using a 3D convolutional neural network: a retrospective multi-institutional analysis. Radiology: Artificial Intelligence. (2021) Aug 11; 3: (5): e200301. |
[46] | Yu Y, Song G, Zhao Y, Liang J, Liu Q. Prediction of vestibular schwannoma surgical outcome using deep neural network. World Neurosurgery. (2023) Aug 1; 176: : e60-7. |
[47] | Neve OM, Romeijn SR, Chen Y, Nagtegaal L, Grootjans W, Jansen JC, Staring M, Verbist BM, Hensen EF. Automated 2-Dimensional Measurement of Vestibular Schwannoma: Validity and Accuracy of an Artificial Intelligence Algorithm. Otolaryngology–Head and Neck Surgery. (2023) Dec; 169: (6): 1582-9. |
[48] | Neves CA, Liu GS, El Chemaly T, Bernstein IA, Fu F, Blevins NH. Automated Radiomic Analysis of Vestibular Schwannomas and Inner Ears Using Contrast-Enhanced T1-Weighted and T2-Weighted Magnetic Resonance Imaging Sequences and Artificial Intelligence. Otology & Neurotology. (2023) Sep 1; 44: (8): e602-9. |
[49] | Shapey J, Kujawa A, Dorent R, Wang G, Dimitriadis A, Grishchuk D, Paddick I, Kitchen N, Bradford R, Saeed SR, Bisdas S. Segmentation of vestibular schwannoma from MRI, an open annotated dataset and baseline algorithm. Scientific Data. (2021) Oct 28; 8: (1): 286. |
[50] | Wu J, Guo D, Wang L, Yang S, Zheng Y, Shapey J, Vercauteren T, Bisdas S, Bradford R, Saeed S, Kitchen N. TISS-net: Brain tumor image synthesis and segmentation using cascaded dual-task networks and error-prediction consistency. Neurocomputing. (2023) Aug 1; 544: : 126295. |
[51] | Yao P, Shavit SS, Shin J, Selesnick S, Phillips CD, Strauss SB. Segmentation of Vestibular Schwannomas on Postoperative Gadolinium-Enhanced T1-Weighted and Noncontrast T2-Weighted Magnetic Resonance Imaging Using Deep Learning. Otology & Neurotology. (2022) Dec 1; 43: (10): 1227-39. |
[52] | Abouzari M, Goshtasbi K, Sarna B, Khosravi P, Reutershan T, Mostaghni N, Lin HW, Djalilian HR. Prediction of vestibular schwannoma recurrence using artificial neural network. Laryngoscope investigative otolaryngology. (2020) Apr; 5: (2): 278-85. |
[53] | Vokurka EA, Herwadkar A, Thacker NA, Ramsden RT, Jackson A. Using Bayesian tissue classification to improve the accuracy of vestibular schwannoma volume and growth measurement. American journal of neuroradiology. (2002) Mar 1; 23: (3): 459-67. |
[54] | Wang G, Li W, Ourselin S, Vercauteren T. Automatic brain tumor segmentation based on cascaded convolutional neural networks with uncertainty estimation. Frontiers in computational neuroscience. (2019) Aug 13; 13: : 56. |
[55] | MacKeith S, Das T, Graves M, Patterson A, Donnelly N, Mannion R, Axon P, Tysome J. A comparison of semi-automated volumetric vs linear measurement of small vestibular schwannomas. European Archives of Oto-Rhino-Laryngology. (2018) Apr; 275: : 867-74. |
[56] | Walz PC, Bush ML, Robinett Z, Kirsch CF, Welling DB. Three-dimensional segmented volumetric analysis of sporadic vestibular schwannomas: comparison of segmented and linear measurements. Otolaryngology–Head and Neck Surgery. (2012) Oct; 147: (4): 737-43. |