Integrating network pharmacology and Mendelian randomization to explore potential targets of matrine against ovarian cancer
Abstract
BACKGROUND:
Matrine has been reported inhibitory effects on ovarian cancer (OC) cell progression, development, and apoptosis. However, the molecular targets of matrine against OC and the underlying mechanisms of action remain elusive.
OBJECTIVE:
This study endeavors to unveil the potential targets of matrine against OC and to explore the intricate relationships between these targets and the pathogenesis of OC.
METHODS:
The effects of matrine on the OC cells (A2780 and AKOV3) viability, apoptosis, migration, and invasion was investigated through CCK-8, flow cytometry, wound healing, and Transwell analyses, respectively. Next, Matrine-related targets, OC-related genes, and ribonucleic acid (RNA) sequence data were harnessed from publicly available databases. Differentially expressed analyses, protein-protein interaction (PPI) network, and Venn diagram were involved to unravel the core targets of matrine against OC. Leveraging the GEPIA database, we further validated the expression levels of these core targets between OC cases and controls. Mendelian randomization (MR) study was implemented to delve into potential causal associations between core targets and OC. The AutoDock software was used for molecular docking, and its results were further validated using RT-qPCR in OC cell lines.
RESULTS:
Matrine reduced the cell viability, migration, invasion and increased the cell apoptosis of A2780 and AKOV3 cells (
CONCLUSIONS:
We identified six matrine-related targets against OC, offering novel insights into the molecular mechanisms underlying the therapeutic effects of matrine against OC. These findings provide valuable guidance for developing more efficient and targeted therapeutic approaches for treating OC.
1.Introduction
Ovarian cancer (OC) is the most devastating malignancy among female reproductive carcinomas, tragically ranking as the fifth leading cause of cancer-related deaths among women worldwide [1]. Shockingly, merely a quarter of women with OC receive an early diagnosis at Stage I/II, and a staggering majority (75%) are confronted with the challenges of advanced stages (Stage III/IV) at the time of diagnosis [2]. A clinical investigation unequivocally underscores the pivotal role of metastasis in determining unfavorable survival outcomes for OC patients [3]. Despite the aggressive implementation of frontline treatments, such as surgical interventions and adjuvant chemotherapy, the 10-year survival rates for women diagnosed with OC at Stage III and Stage IV remain a mere 21% and less than 5%, respectively [4]. Consequently, the pressing need to explore more therapeutic agents and their precise targets for combating OC becomes ever more imminent.
Matrine (C15H24N2O) is an intriguing alkaloid extracted from the Sophora family, which has been shown to have potential therapeutic effects on human diseases associated with epithelial cells [5, 6]. Previous study has demonstrated that matrine modulates various biological activities, such as apoptosis, oxidative stress, inflammation, fibrosis, and the immune response [7]. Notably, a published study has underscored the role of matrine role in promoting OC cell apoptosis by modulating the ERK/JNK signaling pathway [8]. Additionally, the efficacy of matrine in suppressing OC cell progression and development has been attributed to its inhibition of phosphorylation signaling pathways [9]. However, despite these findings, the specific targets through which matrine exerts its effects on OC remain unclear, warranting further investigation.
Mendelian randomization (MR) is a rapidly advancing field grounded in Mendel’s law of inheritance. MR utilizes single nucleotide polymorphisms (SNPs) as instrumental variables (IVs) to uncover causal relationships between modifiable exposures and clinically important outcomes [10]. By utilizing genetic variants as instrumental variables, MR provides a robust approach to estimating the causal associations between exposures and their impacts on outcomes, lending valuable insights into the realm of causality in epidemiological research. MR can be employed to investigate the causal links between particular risk factors and tumor risk [11, 12, 13]. However, exploring the causal relationships using MR between matrine-related core targets and OC risk has not been reported.
The primary objective of this study is to identify potential targets of matrine in OC and to investigate their associations with the disease. To achieve this, we conducted a comprehensive analysis, including differential expression analysis and protein-protein interaction (PPI) network analysis, to uncover the core targets of matrine against OC. Remarkably, six core genes were identified: TP53, STAT3, CCND1, VEGFA, IL1B, and CCL2. Furthermore, the causal associations between core targets and OC were investigated using an MR study. Finally, molecular docking analysis was performed to investigate the molecular docking site and binding energy, and the results were further validated in vitro. The pictorial representation of the methodology is shown in Supplementary Fig. 1.
Figure 1.
Effects of matrine on OC cell lines A2780 and SKOV3 cells. (A) CCK-8 assay CCK-8 detected the cell viability of A2780 and SKOV3 cells. (B) Flow cytometry detected cell apoptosis. (C) Wound healing detected cell migration. (E) Transwell assay detected cell invasion (scale bar
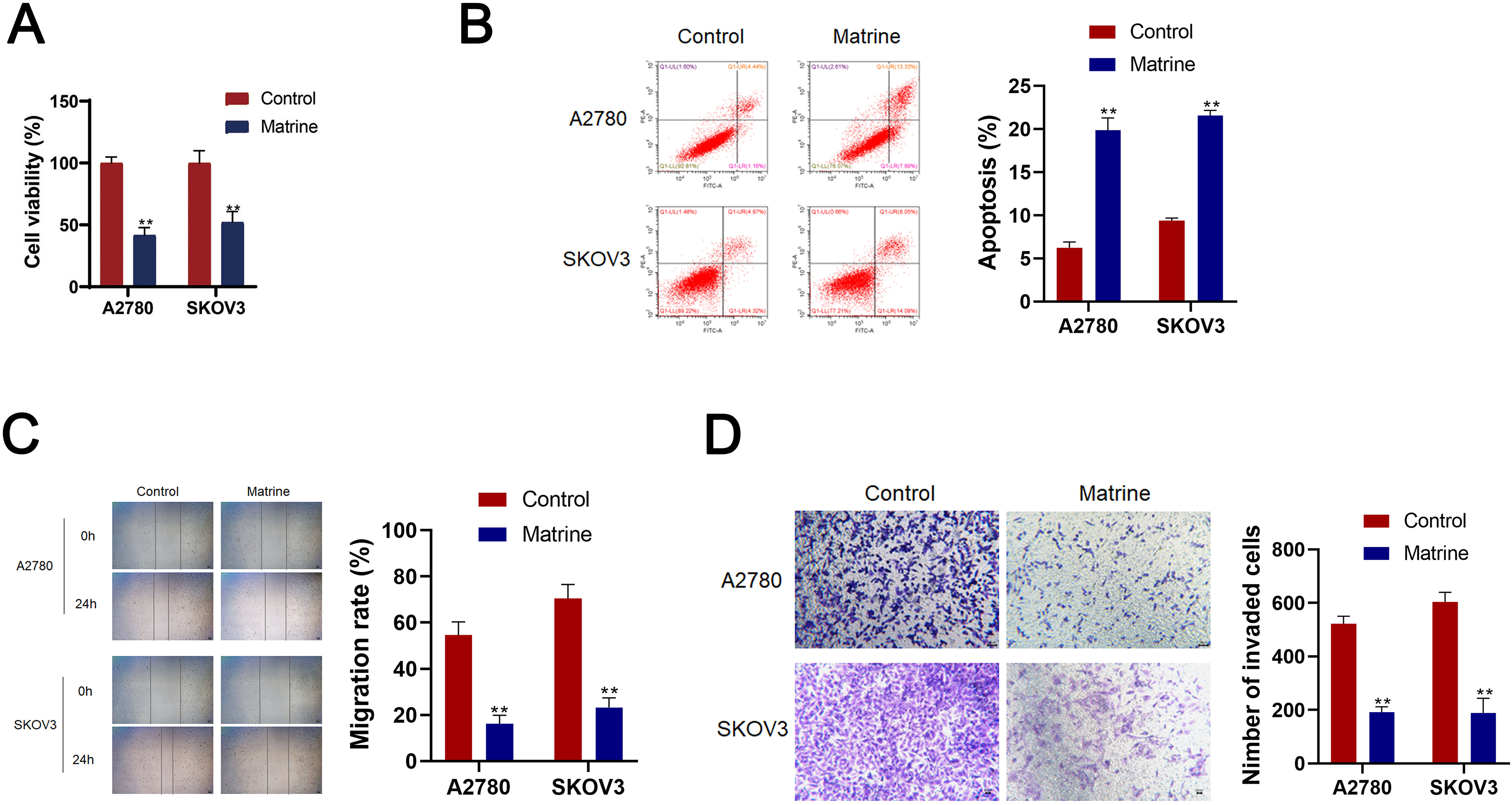
2.Materials and methods
2.1Cells culture and treatment
OC cell lines A2780 (#CL-0013, Procell, Wuhan, China) and SKOV3 cells (#CL-0215, Procell) were cultured in RPMI-1640 medium (Gibco, CA, USA) at 37∘C with 5% CO2. Matrine purchased from Wuhan Zhongbiao Science & Technology CO. (Wuhan, China) was diluted into 2 mg/mL and then used to treat cells for 24 h.
2.2Cells counting kit 8 (CCK-8) analysis for cell viability
SKOV3 and A2780 cells were inoculated in a 96-well plate (100
2.3Flow cytometry for cell apoptosis
Flow cytometry was performed to assess the cell apoptosis in SKOV3 and A2780 cells. After culture for 24 h, cells were gathered and suspended in 195
2.4Cell migration and invasion
Would healing was used to assess of cell migration. SKOV3 and A2780 cells were lated in 6-well plates (5
Transwell were employed to assess cell invasion. SKOV3 and A2780 cells were seeded into upper chamber, and DMEM containing 10% FBS was added to the lower chamber. After 24 h incubation, cells were washed with PBS, fixed with methanol, and stained with 0.1% crystal violet.
2.5Data acquisition and preprocessing
The GeneCards (www.genecards.org) [14], Herbal Ingredients’ Targets Platform (HIT; http://hit2.badd-cao.net) [15], SwissTargetPrediction (www.swisstargetprediction.ch) [16], and SuperPred (http://prediction. charite.de/index.php) [17] databases were adopted to explore and predict the possible matrine-related targets. OC-related genes were downloaded from GeneCards and DisGeNET (http://www.disgenet.org) [18] online platforms. The GSE66957 containing mRNA profile information of 57 OC cases and 12 controls and GSE26712 including mRNA profile information of 185 OC cases and 10 controls were searched from the Gene Expression Omnibus (GEO, http://www.ncbi.nlm.nih.gov/geo/) database.
2.6Identification of potential matrine-related targets against OC
The limma package was implemented to obtain differentially expressed genes (DEGs) in OC with detecting conditions of
2.7PPI network development and core targets selection
Search Tool for the Retrieval of Interacting Genes (STRING; https://string-db.org) [19] platform was adopted to explore the molecular interactions of intersecting genes with score_cutoff
2.8Mendelian randomization (MR)
TwoSampleMR package in R software (Version 4.2.2) was employed to conduct MR analysis. The exposure-related drug-targeting instrumental variables were harmonized with the outcome datasets. Four statistical methods, including inverse variance weighting (IVW), weighted mode, weighted median, and simple mode, were applied to explore potential causal relationships between core targets and OC.
2.9Molecular docking
The three-dimensional (3D) protein structure of the core targets was obtained from the RCSB Protein Data Bank (RCSB) using the website http://www.pdb.org/. To isolate the modified and ligand components and eliminate water molecules, the Pymol software was utilized. Additionally, the 3D structure of matrine was acquired from PubChem, hydrogen atoms were added, and charges were calculated. Subsequently, Autodock (Version 4.2.6) software was employed to perform docking simulations with the core targets. A stable connection site was determined based on a threshold of limited binding energy
2.10Quantitative real-time PCR (qRT-PCR)
Total RNA was isolated using Trizol (Invitrogen, CA, USA). Reverse transcription utilized the cDNA Reverse Transcription Kit (TIANGEN, Beijing, China) to synthesize cDNA. Quantitative PCR was conducted using SYBR Green PCR Master Mix (Lifeint, Xiamen, China) on the StepOnePlus Real-Time PCR System (Bio-rad, CA, USA). Specific primers designed for CCND1, IL1B, p53, and GAPDH (internal control) were used in a 20
2.11Statistical analysis
Statistical analysis of the RT-qPCR data was performed using GraphPad Prism software 8.0. After confirming that the data met the assumptions of normality and equal variance, we employed Student’s t-test to compare the means between the two groups. Each set of experiments was conducted in triplicate, and results were expressed as mean
3.Results
3.1Effects of martrine in cell viability, apoptosis, migration, and invasion in vitro
To investigate the effects of matrine on OC, A2780 and AKOV3 cells were cultured. Significantly, matrine reduced the cell viability, migration, invasion and increased the cell apoptosis of A2780 and AKOV3 cells (
3.2Potential targets of matrine against OC
To further investigate the targets of matrine on OC, network pharmacology and mendelian randomization were conducted. Totally 635 possible matrine targets and 5,513 OC-related genes were obtained from the online websites after removing duplicates (Fig. 2A–B, Supplementary Table 1). A total of 8,001 DEGs were detected from the GSE66957 cohort, which comprised 4,990 up-regulated genes and 3011 down-regulated genes (Fig. 2C–D, Supplementary Table 2). Finally, 105 potential targets of matrine against OC were confirmed by overlapping 635 possible targets of matrine, 5,513 OC-related genes, and 8,001 DEGs (Fig. 2E, Supplementary Table 3).
Figure 2.
Identification of potential targets of matrine against OC. (A) The possible targets of matrine are from HIT, SwissTargetPrediction, SuperPred, and GeneCards databases, respectively. (B) OC-related genes were obtained from the GeneCards and DisGeNET online websites, respectively. (C) Volcanic map of DEGs in GSE66957 cohort. (D) Heat map of the expression levels of DEGs between OC cases and controls from GSE66957 cohort. Blue represents down-regulation, and red represents up-regulation. (E) Venn diagram shows 105 interacting genes among OC-related genes, targets of matrine, and DEGs. OC: ovarian cancer; DEGs: differentially expressed genes.
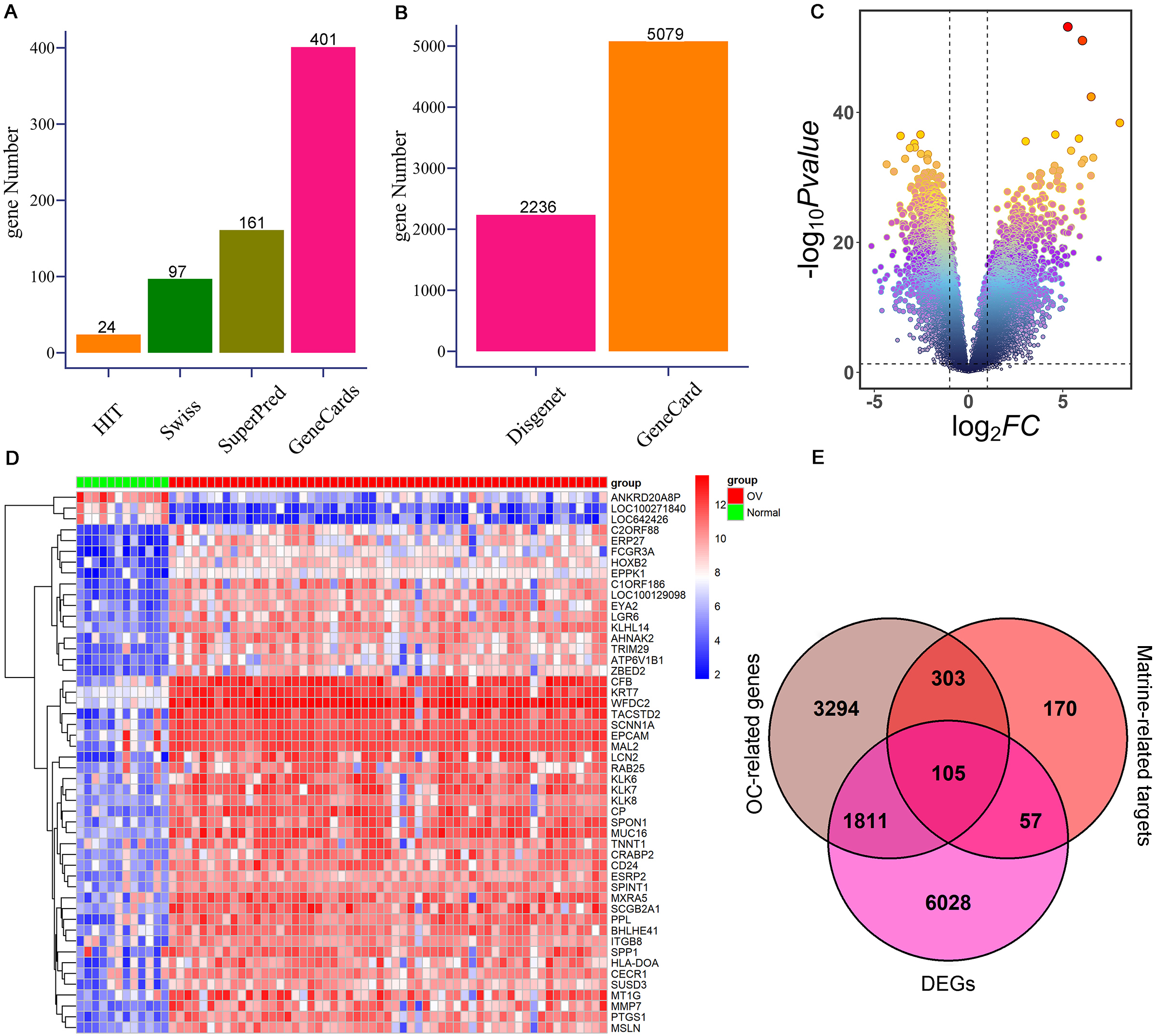
Figure 3.
Screening of hub targets. (A) PPI network of 105 intersecting genes. (B) Four core modules (Degree, EPC, MCC, and MNC) in the PPI network. (C) Venn diagram shows the six hub targets among four core modules. PPI: protein-protein interaction.
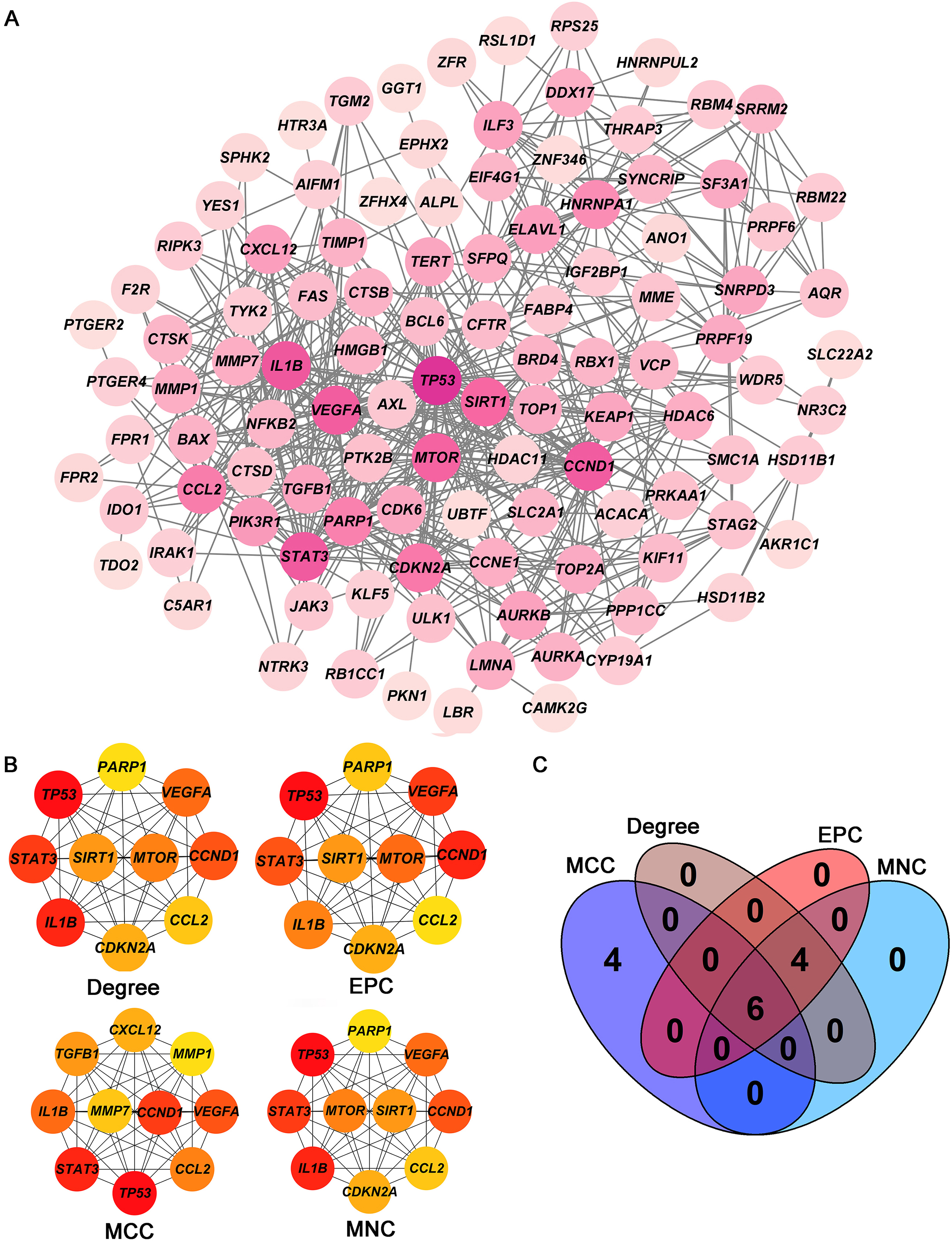
3.3Identification of core targets
The PPI network including 578 interactions among 105 potential targets of matrine was developed and shown in Fig. 3A. In addition, four core modules in the PPI network consisted of genes with the top 10 scores calculated by MCC, MNC, EPC, and Degree algorithms exhibited in Fig. 3B. By intersecting the four core modules, six core targets, consisting of TP53, STAT3, CCND1, VEGFA, IL1B, and CCL2, were further obtained (Fig. 3C). Moreover, the expression levels of six core targets were verified in the GEPIA database, and the results suggested that the expression of TP53, CCND1, VEGFA, IL1B, and CCL2 was remarkably higher in the OC samples than in the control group, whereas there was no significant difference in STAT3 (Fig. 4). Moreover, validation of the core targets differential expression in the GSE26712 dataset further revealed that TP53, CCND1, VEGFA, IL1B, and CCL2 levels were elevated in OC samples (Fig. 5).
Figure 4.
Box plots show the expression levels of CCL2, CCND1, IL1B, STAT3, TP53, and VEGFA between OC cases and controls based on the GEPIA database. Red indicates OC samples, while blue indicates normal samples. OC: ovarian cancer. * P < 0.05.
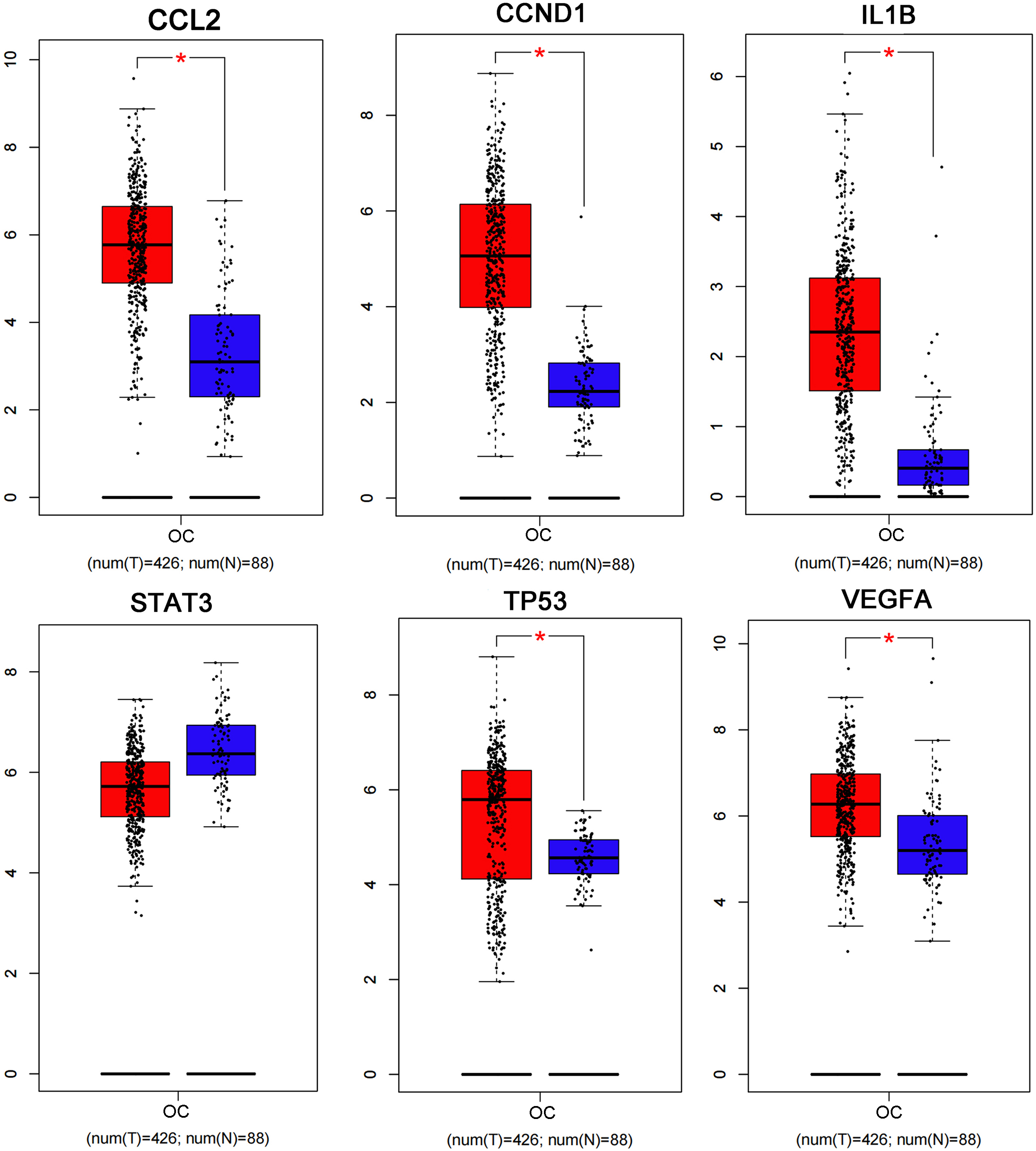
Figure 5.
Box plots show the expression levels of CCL2, CCND1, IL1B, STAT3, TP53, and VEGFA between OC cases and controls in validation dataset GSE26712. Red indicates OC samples, while blue indicates normal samples. OC: ovarian cancer. *P< 0.05, **P < 0.01, *** P< 0.001, ***** P< 0.0001.
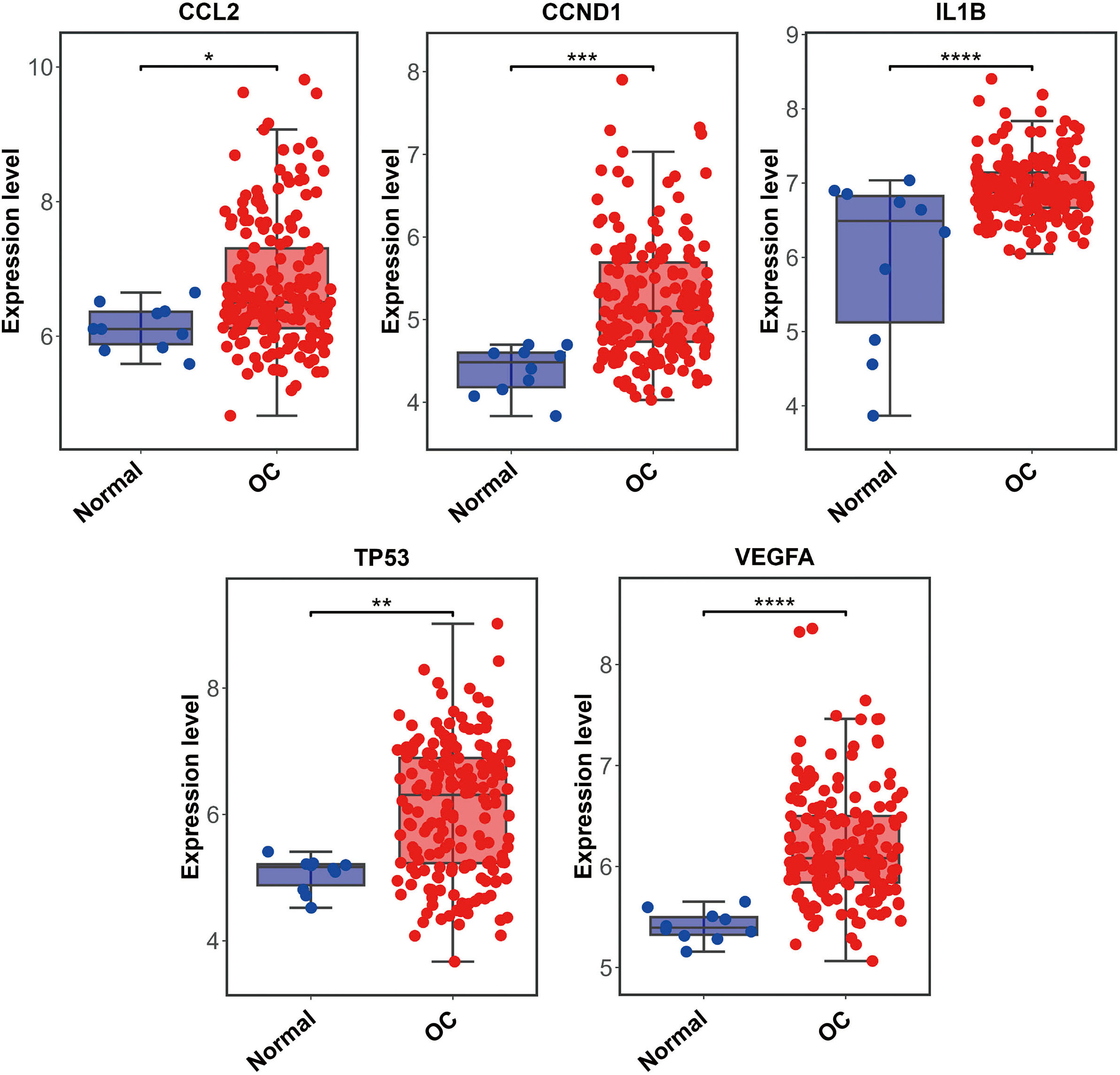
3.4Evaluating the potential association between core targets and OC
The SNP characteristics of CCND1, IL1B, TP53, VEGFA, and OC were meticulously presented in
Supplementary Table 4, providing comprehensive insights into their genetic attributes. The causal correlations between the levels of core targets (CCND1, IL1B, TP53, and VEGFA) and the risk of OC were evaluated and shown in Fig. 6A–D, the results demonstrated that VEGFA and IL1B were protective factors for OC, while CCND1 and TP53 were risk factors for OC. The funnel plot exhibited a roughly symmetrical causal effect (Fig. 6E–H).
Supplementary Table 5 demonstrated that CCND1 (Weighted median,
3.5Matrine-targets docking analysis
To gain deeper insights into the molecular interactions, four genes (CCND1, IL1B, TP53, and VEGFA) detected by MR analysis were subjected to molecular docking as protein receptors interacting with matrine (CID: 91466). Based on the selection criteria of binding energy less than
Table 1
Results of molecular docking
GeneName | UniprotID | PDB ID | Compound | PubchemID | Bind energy (kcal/mol) |
---|---|---|---|---|---|
TP53 | P04637 | 6ggc | Matrine | CID: 91466 | |
CCND1 | P24385 | 2w96 | |||
IL1B | P01584 | 5r8e |
Figure 6.
Mendelian randomization study results. (A-D) Scatter plot showing the causal effect of CCND1, IL1B, TP53, and VEGFA on the risk of OC. (E-H) Funnel plots show overall heterogeneity of MR estimates of the effect of CCND1, IL1B, TP53, and VEGFA on OC. OC: ovarian cancer.
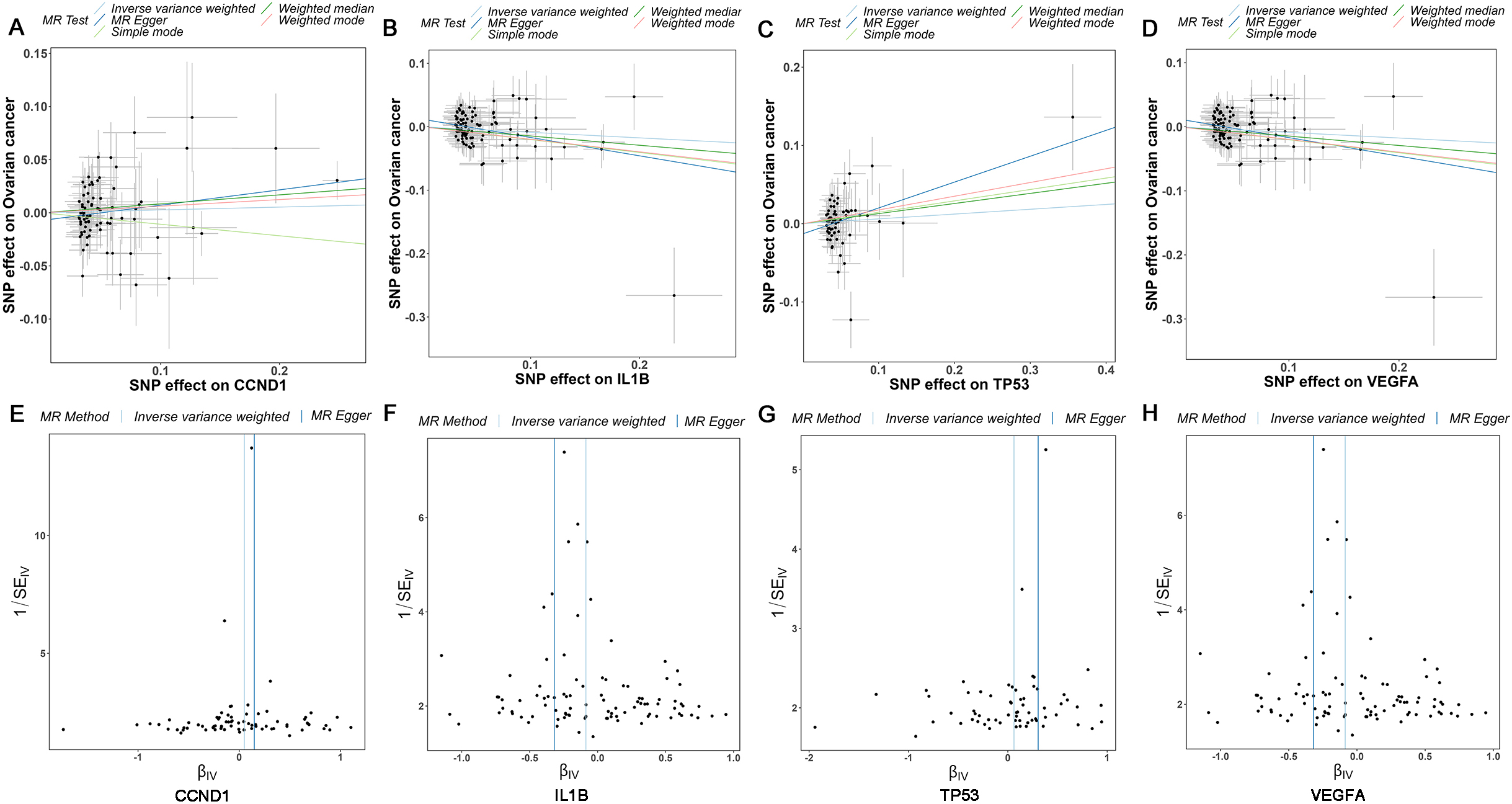
Figure 7.
Molecular docking site. (A) CCND1-matrine. (B) IL1B-matrine. (C) TP53-matrine. (D) RT-qPCR detected the mRNA expression of CCND1, IL1B, and P53. OC cell line A2780 and SKOV3 cells were treated with matrine. * P < 0.05, **P < 0.01.
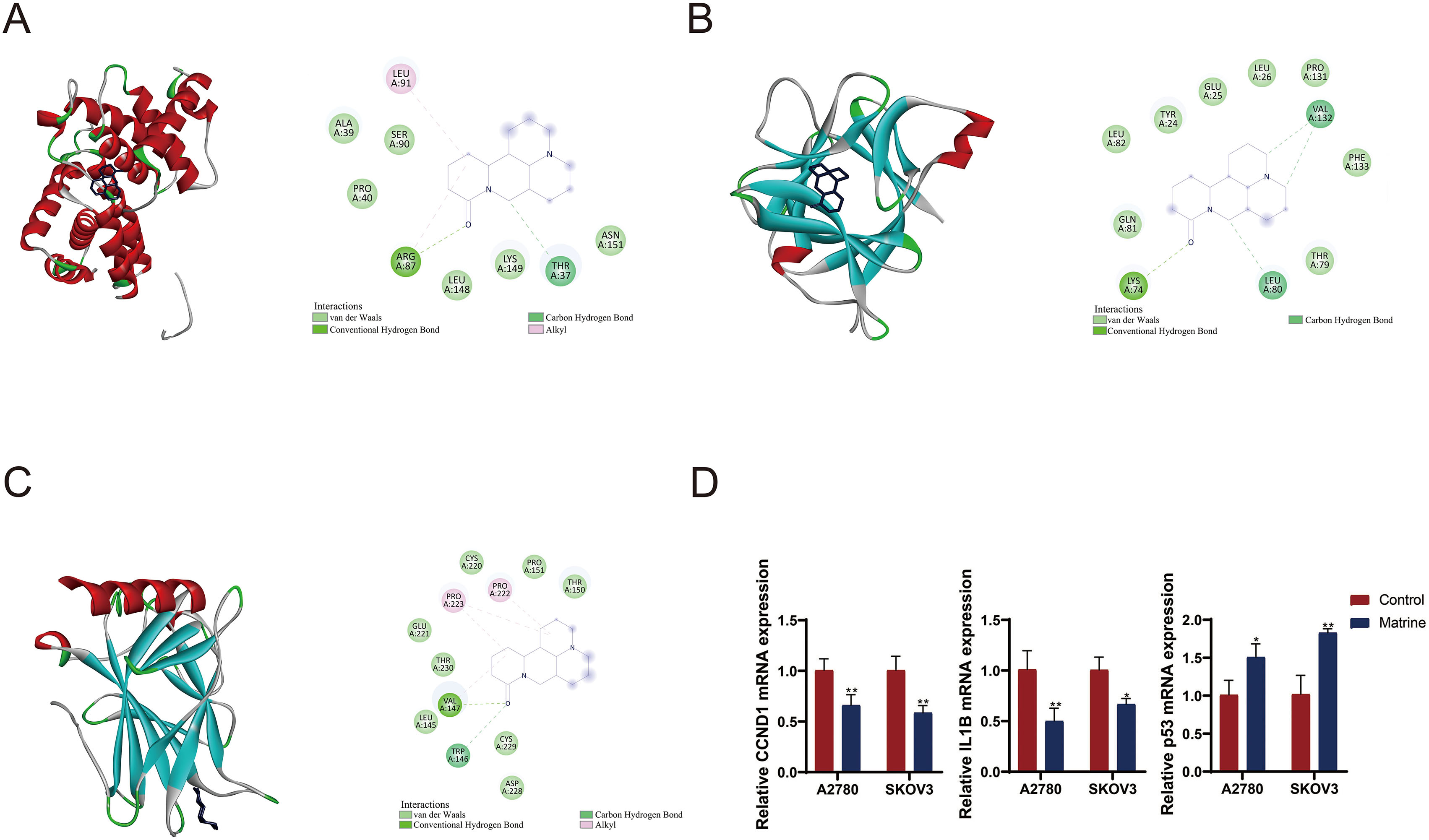
3.6Validation of molecular docking in vitro
We then validated the effect of matrine on levels of target genes CCND1, IL1B, and TP53 in OC cell lines A2780 and SKOV3.The expression levels of CCND1 and IL1B were decreased while P53 expression was increased in the marine-treated cells compared to control cells (
4.Discussion
OC is the second leading cause of gynecologic cancer deaths in women, with approximately 239,000 new cases reported annually (3.6% of cancer-related cases), resulting in approximately 152,000 deaths (4.3% of cancer-related deaths) annually worldwide [21]. Despite notable progress in medical research, patients with OC still face a formidable prognosis, with an approximate five-year survival rate of 59.6% after diagnosis in Asian countries [22]. Studies have demonstrated that matrine has anti-inflammatory properties [23] and inhibits progression and apoptosis in OC cells [8, 9]. Therefore, the present study applied network pharmacology, MR, and molecular docking analyses to explore the mechanism of matrine against OC, thus providing reference ideas and strategies for future OC prevention and treatment.
Firstly, we verified the effects of matrine on the OC in vitro, suggesting that matrine decreased the cell viability, migration, invasion and increased cell apoptosis in OC cell line A2780 and SKOV3. This result is coincidence with the previous publications [8, 24, 25, 26]. Next, we detected six core matrine-related targets (TP53, CCND1, VEGFA, IL1B, CCL2, and STAT3), in which five genes (TP53, CCND1, VEGFA, IL1B, and CCL2) were remarkable differenttially expressed between OC and normal samples. Subsequent MR analyses provided valuable insights, suggesting that VEGFA and IL1B were protective factors for OC, whereas CCND1 and TP53 were risk factors for OC.
CCND1 is a pivotal cell cycle regulatory protein frequently upregulated in human tumors and is associated with poor prognosis [27, 28, 29, 30]. MR analyses have shown a positive correlation between CCND1 and OC risk. Mechanistically, this may be due to CCND1 contributing to enhanced cell proliferation and division by forming a functional complex with cell cycle protein-dependent kinases (CDKs), effectively promoting the transition from the G1 to the S phase of the cell cycle [30].
The TP53 protein functions as a crucial DNA-binding transcription factor, exerting its influence on hundreds of different promoter elements in the genome and thereby orchestrating the expression of numerous genes [31]. Playing a pivotal role in key cellular processes that regulate cell proliferation and preserve genomic integrity and stability [32], TP53 serves as a guardian of the genome. In human cancers, TP53 gene undergoes alterations in all but 5 residues of its 393 amino acids, making it truly exceptional among all cancer-associated genes [33]. Loss of heterozygosity at the TP53 motif is a common occurrence in OC [34, 35]. Considering these findings, TP53 emerges as a compelling candidate and potential risk factor in the context of OC.
VEGFA serves as a pivotal controller in angiogenesis, acting as a specific mitogen for endothelial cells, an initiator of angiogenesis, and a mediator that enhances vascular permeability [36]. The significant role of VEGFA in promoting angiogenesis and its common upregulation in human cancers has led to the development of therapies targeting VEGF and VEGF receptors [37]. The role of VEGFA in promoting tumorigenesis and cancer cell survival in cancer remains contentious, likely attributed to its tissue-specific effects [38, 39, 40, 41]. A meta-analysis suggested that the VEGF
We employed molecular docking analysis to investigate the therapeutic potential of matrine against OC. The results demonstrated promising binding interactions with three pivotal targets: TP53, CCND1, and IL1B. Furthermore, in vitro experiments revealed that matrine reduced expression levels of CCND1 and IL1B whereas increased the TP53 expression in OC cells. Thess indicate that matrine may exert its effects through targeted interactions with these critical proteins, shedding light on its molecular mechanisms of action in the context of OC treatment.
Despite the valuable insights obtained from our research, it is important to acknowledge several limitations that warrant consideration. Primarily, the sample size utilized in this investigation was relatively small, and the reliance on a single dataset may introduce inherent biases that could impact the generalizability of our results. Moreover, this investigation only employed bioinformatics approaches and further in vitro and in vivo experiments are needed to validate our results. Next, the potential synergistic effects of matrine in combination with existing chemotherapeutic or targeted therapies needed to be explored. Finally, how matrine modulates the targets and potential pathways needed to be deeply delved.
5.Conclusions
Altogether, this study identified six pivotal matrine-related core targets (TP53, CCND1, STAT3, IL1B, VEGFA, and CCL2) against OC. MR analysis suggested intriguing insights into the potential roles of CCND1 and TP53 as risk factors in OC. Molecular docking analysis demonstrated that matrine had good potential to bind to TP53, CCND1, and IL1B. These findings collectively advance our understanding of the mechanism of action of matrine in OC and provide valuable insights into the potential efficacy of matrine.
Funding
The authors report no funding.
Data availability statement
The data that support the findings of this study are available from the GEO (https://www.ncbi.nlm.nih. gov/geo/) database.
Ethical considerations
This study do not involve human and animal studies and is exempt from Institutional Review Board approval.
Acknowledgments
The authors have no acknowledgments.
Conflict of interest
The authors declare that they have no conflict of interest.
References
[1] | Sung H, Ferlay J, Siegel RL, Laversanne M, Soerjomataram I, Jemal A, et al. Global Cancer Statistics 2020: GLOBOCAN Estimates of Incidence and Mortality Worldwide for 36 Cancers in 185 Countries. CA Cancer J Clin. (2021) ; 71: (3): 209-49. |
[2] | Ebell MH, Culp MB, Radke TJ. A Systematic Review of Symptoms for the Diagnosis of Ovarian Cancer. American Journal of Preventive Medicine. (2016) ; 50: (3): 384-94. |
[3] | Tempfer CB, El Fizazi N, Ergonenc H, Solass W. Metastasis of ovarian cancer to the breast: A report of two cases and a review of the literature. Oncology Letters. (2016) ; 11: (6): 4008-12. |
[4] | Jelovac D, Armstrong DK. Recent progress in the diagnosis and treatment of ovarian cancer. CA Cancer J Clin. (2011) ; 61: (3): 183-203. |
[5] | Zhang Z, Wang X, Wu W, Wang J, Wang Y, Wu X, et al. Effects of matrine on proliferation and apoptosis in gallbladder carcinoma cells (GBC-SD). Phytotherapy Research: PTR. (2012) ; 26: (6): 932-7. |
[6] | Zhang P, Wang Z, Chong T, Ji Z. Matrine inhibits proliferation and induces apoptosis of the androgen-independent prostate cancer cell line PC-3. Molecular Medicine Reports. (2012) ; 5: (3): 783-7. |
[7] | Lao Y. [Clinical study on effect of matrine injection to protect the liver function for patients with primary hepatic carcinoma after trans-artery chemo-embolization (TAE). Zhong Yao Cai = Zhongyaocai = Journal of Chinese Medicinal Materials. (2005) ; 28: (7): 637-8. |
[8] | Liang X, Ju J. Matrine inhibits ovarian cancer cell viability and promotes apoptosis by regulating the ERK/JNK signaling pathway via p38MAPK. Oncology Reports. (2021) ; 45: (5). |
[9] | Zhang X, Hou G, Liu A, Xu H, Guan Y, Wu Y, et al. Matrine inhibits the development and progression of ovarian cancer by repressing cancer associated phosphorylation signaling pathways. Cell Death & Disease. (2019) ; 10: (10): 770. |
[10] | Holmes MV, Ala-Korpela M, Smith GD. Mendelian randomization in cardiometabolic disease: challenges in evaluating causality. Nature Reviews Cardiology. (2017) ; 14: (10): 577-90. |
[11] | Zhou W, Liu G, Hung RJ, Haycock PC, Aldrich MC, Andrew AS, et al. Causal relationships between body mass index, smoking and lung cancer: Univariable and multivariable Mendelian randomization. International Journal of Cancer. (2021) ; 148: (5): 1077-86. |
[12] | Chen X, Kong J, Diao X, Cai J, Zheng J, Xie W, et al. Depression and prostate cancer risk: A Mendelian randomization study. Cancer Medicine. (2020) ; 9: (23): 9160-7. |
[13] | Chong M, Sjaarda J, Pigeyre M, Mohammadi-Shemirani P, Lali R, Shoamanesh A, et al. Novel Drug Targets for Ischemic Stroke Identified Through Mendelian Randomization Analysis of the Blood Proteome. Circulation. (2019) ; 140: (10): 819-30. |
[14] | Safran M, Dalah I, Alexander J, Rosen N, Iny Stein T, Shmoish M, et al. GeneCards Version 3: the human gene integrator. Database (Oxford). (2010) ; 2010: : baq020. |
[15] | Yan D, Zheng G, Wang C, Chen Z, Mao T, Gao J, et al. HIT 20: an enhanced platform for Herbal Ingredients’ Targets. Nucleic Acids Research. (2022) ; 50: (D1): D1238-d43. |
[16] | Daina A, Michielin O, Zoete V. SwissTargetPrediction: updated data and new features for efficient prediction of protein targets of small molecules. Nucleic Acids Research. (2019) ; 47: (W1): W357-w64. |
[17] | Gallo K, Goede A, Preissner R, Gohlke BO. SuperPred 30: drug classification and target prediction-a machine learning approach. Nucleic Acids Research. (2022) ; 50: (W1): W726-w31. |
[18] | Piñero J, Saüch J, Sanz F, Furlong LI. The DisGeNET cytoscape app: Exploring and visualizing disease genomics data. Computational and Structural Biotechnology Journal. (2021) ; 19: : 2960-7. |
[19] | Szklarczyk D, Gable AL, Nastou KC, Lyon D, Kirsch R, Pyysalo S, et al. The STRING database in 2021: customizable protein-protein networks, and functional characterization of user-uploaded gene/measurement sets. Nucleic Acids Research. (2021) ; 49: (D1): D605-D12. |
[20] | Feng C, Zhao M, Jiang L, Hu Z, Fan X. Mechanism of Modified Danggui Sini Decoction for Knee Osteoarthritis Based on Network Pharmacology and Molecular Docking. Evidence-Based Complementary and Alternative Medicine: eCAM. (2021) ; 2021: : 6680637. |
[21] | Lheureux S, Braunstein M, Oza AM. Epithelial ovarian cancer: Evolution of management in the era of precision medicine. CA Cancer J Clin. (2019) ; 69: (4): 280-304. |
[22] | Maleki Z, Vali M, Nikbakht HA, Hassanipour S, Kouhi A, Sedighi S, et al. Survival rate of ovarian cancer in Asian countries: a systematic review and meta-analysis. BMC Cancer. (2023) ; 23: (1): 558. |
[23] | Huang WC, Chan CC, Wu SJ, Chen LC, Shen JJ, Kuo ML, et al. Matrine attenuates allergic airway inflammation and eosinophil infiltration by suppressing eotaxin and Th2 cytokine production in asthmatic mice. Journal of Ethnopharmacology. (2014) ; 151: (1): 470-7. |
[24] | Wei R, Cao J, Yao S. Matrine promotes liver cancer cell apoptosis by inhibiting mitophagy and PINK1/Parkin pathways. Cell Stress & Chaperones. (2018) ; 23: (6): 1295-309. |
[25] | Xu PL, Cheng CS, Jiao JY, Chen H, Chen Z, Li P. Matrine injection inhibits pancreatic cancer growth via modulating carbonic anhydrases- a network pharmacology-based study with in vitro validation. Journal of Ethnopharmacology. (2022) ; 287: : 114691. |
[26] | Zhao B, Hui X, Wang J, Zeng H, Yan Y, Hu Q, et al. Matrine suppresses lung cancer metastasis via targeting M2-like tumour-associated-macrophages polarization. American Journal of Cancer Research. (2021) ; 11: (9): 4308-28. |
[27] | Rudas M, Lehnert M, Huynh A, Jakesz R, Singer C, Lax S, et al. Cyclin D1 expression in breast cancer patients receiving adjuvant tamoxifen-based therapy. Clinical Cancer Research: An Official Journal of the American Association for Cancer Research. (2008) ; 14: (6): 1767-74. |
[28] | Feng Z, Guo W, Zhang C, Xu Q, Zhang P, Sun J, et al. CCND1 as a predictive biomarker of neoadjuvant chemotherapy in patients with locally advanced head and neck squamous cell carcinoma. PloS One. (2011) ; 6: (10): e26399. |
[29] | Albasri AM, Elkablawy MA, Ansari IA, Alhujaily AS. Prognostic Significance of Cyclin D1 Over-expression in Colorectal Cancer: An Experience from Madinah, Saudi Arabia. Asian Pacific Journal of Cancer Prevention: APJCP. (2019) ; 20: (8): 2471-6. |
[30] | Qie S, Diehl JA. Cyclin D1, cancer progression, and opportunities in cancer treatment. Journal of Molecular Medicine (Berlin, Germany). (2016) ; 94: (12): 1313-26. |
[31] | Silwal-Pandit L, Langerød A, BØrresen-Dale AL. TP53 Mutations in Breast and Ovarian Cancer. Cold Spring Harbor Perspectives in Medicine. (2017) ; 7: (1). |
[32] | Lane D, Levine A. p53 Research: the past thirty years and the next thirty years. Cold Spring Harbor Perspectives in Biology. (2010) ; 2: (12): a000893. |
[33] | Leroy B, Anderson M, Soussi T. TP53 mutations in human cancer: database reassessment and prospects for the next decade. Human Mutation. (2014) ; 35: (6): 672-88. |
[34] | Godwin AK, Vanderveer L, Schultz DC, Lynch HT, Altomare DA, Buetow KH, et al. A common region of deletion on chromosome 17q in both sporadic and familial epithelial ovarian tumors distal to BRCA1. American Journal of Human Genetics. (1994) ; 55: (4): 666-77. |
[35] | Saretzki G, Hoffmann U, Röhlke P, Psille R, Gaigal T, Keller G, et al. Identification of allelic losses in benign, borderline, and invasive epithelial ovarian tumors and correlation with clinical outcome. Cancer. (1997) ; 80: (7): 1241-9. |
[36] | Brustmann H, Naudé S. Vascular endothelial growth factor expression in serous ovarian carcinoma: relationship with high mitotic activity and high FIGO stage. Gynecologic Oncology. (2002) ; 84: (1): 47-52. |
[37] | Goel HL, Pursell B, Chang C, Shaw LM, Mao J, Simin K, et al. GLI1 regulates a novel neuropilin-2/α6β1 integrin based autocrine pathway that contributes to breast cancer initiation. EMBO Molecular Medicine. (2013) ; 5: (4): 488-508. |
[38] | Aktaş SH, Akbulut Yazici HO, Zengin N, Akgün HN, Üstüner Z, Içli F. A new angiogenesis prognostic index with VEGFA, PlGF, and angiopoietin1 predicts survival in patients with advanced gastric cancer. Turkish Journal of Medical Sciences. (2017) ; 47: (2): 399-406. |
[39] | Baba H, Baba Y, Uemoto S, Yoshida K, Saiura A, Watanabe M, et al. Changes in expression levels of ERCC1, DPYD, and VEGFA mRNA after first-line chemotherapy of metastatic colorectal cancer: results of a multicenter study. Oncotarget. (2015) ; 6: (32): 34004-13. |
[40] | Tian T, Guo T, Zhen W, Zou J, Li F. BET degrader inhibits tumor progression and stem-like cell growth via Wnt/β-catenin signaling repression in glioma cells. Cell Death & Disease. (2020) ; 11: (10): 900. |
[41] | Braicu EI, Gasimli K, Richter R, Nassir M, Kümmel S, Blohmer JU, et al. Role of serum VEGFA, TIMP2, MMP2 and MMP9 in monitoring response to adjuvant radiochemotherapy in patients with primary cervical cancer–results of a companion protocol of the randomized NOGGO-AGO phase III clinical trial. Anticancer Research. (2014) ; 34: (1): 385-91. |
[42] | Zhang X, Qin J, Qin A. Three Polymorphisms of Vascular Endothelial Growth Factor (+936C > T, -460C > T, and -2578C > A) and Their Susceptibility to Ovarian Cancer: A Meta-Analysis. International Journal of Gynecological Cancer: Official Journal of the International Gynecological Cancer Society. (2015) ; 25: (5): 779-85. |