Multivariate small area estimation of undernutrition for children under five using official statistics
Abstract
Surveys are mainly used to obtain reliable estimates for planned domains at national and regional levels. However, the unplanned domains (lower administrative layers) with small sample sizes must be estimated. The direct survey estimates of the non-planned domains with small sample sizes lead to large sampling variability. Thus, small area estimations dealt with managing this variability by borrowing the strength of neighboring areas. The target variables of the study were obtained from the 2016 Ethiopian demographic and health survey (EDHS) and the auxiliary variables taken from the 2007 population and housing census data. Multivariate Fay Herriot (MFH) model was used by incorporating the correlations among the target variables. The model diagnostic measures assured the normality assumption, and the consistency of multivariate small area estimates are valid. Multivariate EBLUPs of the target variables produced the lowest percent coefficient of variation (CV) and root mean square error (MSE). Therefore, multivariate EBLUP has improved the direct survey estimates of undernutrition (stunting, wasting, and underweight) for small sample sizes (even zero sample sizes). It also provided better estimates compared to the univariate EBLUPs. Generally, multivariate EBLUPs of undernutrition produced the best reliable, efficient, and precise estimates for small sample sizes in all zones. Zones are essential domains for planning and monitoring purposes in the country, and therefore these results provide valuable estimates for policymakers, planners, and legislative organs of the government. One of the novelties of this paper is estimating the non-sampled zones, and therefore the policymakers will give equal attention similar to the sampled zones.
1.Introduction
Sample surveys are mainly designed to produce estimates of population characteristics of interest for larger domains at regional and national levels with large sample sizes [1]. In these large domains, reliable, efficient, and precise direct survey estimates can be produced from the survey data. However, in many practical cases, estimates are often required for areas with small sample sizes for which the survey was not addressed. Recently the demand for reliable, consistent, and precise small area statistics is significantly increased across the globe. Unfortunately, for most surveys, the sample size is not large enough to guarantee reliable direct estimates for all characteristics of interest. Small area estimation (SAE) overwhelmed the problems of producing reliable estimates of parameters of interest for which the samples sizes are too small for adequate precisions [1].
Small area estimations based on area-level aggregated population data is a Fay Herriot model since it was introduced by [2]. Many researchers studied small area estimation under the univariate Fay Herriot (UFH) model, which overlooks the correlation between related target variables of interest [3, 4, 5]. However, surveys may be appropriate in practical cases to consider many correlated target variables. In such cases, the multivariate Fay-Herriot (MFH) model can be used to integrate the correlation among target variables. The MFH extends the UFH model introduced by [6] and developed by considering different covariance structures of the random effects by [7]. The MFH model replicated the joint modeling of more than one target variable, in contrast to the UFH model [8]. MFH models have become popular methods to produce reliable parameter estimates of multiple related characteristics of interest that are commonly produced from many surveys [8].
Statisticians are often applied to assess correlated measures of target variables, such as undernutrition indicators (stunting, wasting, and underweight). Multivariate models consider the correlation of different variables and are typically applied to this situation. There are few publications in the literature on small area estimates that use multivariate small area estimations under the FH model. Studies by [7, 8, 9, 10] evaluated the precision of small area estimators produced from univariate models to those generated from multivariate models for each target variable.
In this study, we used a multivariate extension of the Fay-Herriot model to help the estimate of mean vectors of the z scores of undernutrition indicators in small areas for small or zero sample sizes. When multiple dependent variables are considered correlated, the MFH model may produce better results than the univariate FH model [1], but these models have received little attention.
The mean squared error (MSE) is integral to small area estimation research [1]. MSE estimation depends on the method of model parameter estimation, but the assumptions made about the distributions of the random model components. It is essential to obtain an accurate estimator of MSE to reflect the true variability associated with the EBLUP estimators. MSE estimators have been studied using variance component estimators under the MFH model [11, 12]. The performance of small area estimates under the MFH model was assessed by the coefficient of variation (CV %) and root MSE [10, 13].
Undernutrition remains the leading public health problem in different continents, especially in eastern Africa and southern Asia [14, 15]. In Ethiopia, undernutrition is a severe problem for children under five and table among the worst countries in the world [16, 17]. Even though there has been significant recent progress in the country, there are still problems in different parts [16]. The target variable for this study has been the z-score of the undernutrition status of children under five, which are underweight (weight-for-age), stunting (height for age), and wasting (weight for height) from the 2016 Ethiopian demographic and health survey (EDHS) data.
The standardized measures of stunting, wasting, and underweight were calculated using the new child growth standards released by the world health organization [18]. Children whose height-for-age
According to the report in [15], globally, an estimated 144 million and 47 million children under five were stunted and wasted, respectively. Most of the world’s stunted, underweight and wasted children under five were lived in Asia and Africa [15]. In Ethiopia, 38%, 10%, and 24% of children under five were stunted, wasted, and underweight [16, 17].
Many studies in Ethiopia examined undernutrition at the regional level [16, 19, 20, 21, 22, 23, 24]. These analyses were carried out utilizing survey data from planned domains at the national and regional levels. Furthermore, some studies have recently been undertaken at the zonal level [25, 26, 27]. However, the small area estimation ideas that boost effective sample sizes were overlooked in these investigations. As a result, unplanned areas that are zones of undernutrition in Ethiopia (the third administrative level) must be assessed using auxiliary variables to overcome the problem of small sample sizes. Because of the small sample size and the fact that the zones are unplanned domains for the Ethiopian survey, traditional direct survey estimation methods cannot be employed. As a result, under the MFH model, small area estimations are applied for under five age child undernutrition indicators.
The health service uses decentralization as the most influential administrative determinant [28]. The federal ministry of health decentralized the health service parallel to the government structures (regions, zones, and woredas). These administrative hierarchies are the key institutions involved in health care delivery in the country [28, 29]. Among them, the zonal governments serve as a bridge between regional and woreda (district) governments. The zonal health department is responsible for monitoring and evaluating health activities in the districts [28], which means that zones have more policy implications than districts. Therefore, zonal level estimates of undernutrition are invaluable for legislative organs at any level of structures in the country.
Thus, our study was focused on the multivariate estimates of the
The successive sections of this research paper are organized as follows: Section 2 describes the data sources, the sampling design and methodologies of the multivariate Fay Herriot model, Section 3 contains the results, discussions are presented in Section 4, and finally, Section 5 contains conclusions.
2.Methods and materials
In this section, the secondary source of the data used in the MFH model was the 2016 EDHS combined with the 2007 population and housing census data. These were the latest round of available data being used for policymakers in Ethiopia. A wide gap could exist between the 2007 census and 2016 survey data since the census in this country takes place every ten years. Note that using auxiliary covariates to model the undernutrition data from 2016 data are not expected to change significantly over a short period of time [32]. However, we used the 2016 census projection for sex, urban and rural residence auxiliary variables to all zones [33].
These data are used for estimating the z-scores of undernutrition at zonal levels in Ethiopia. The 2016 EDHS used a sampling frame designed for the Ethiopian population and housing census, which was carried out in 2007 by the Ethiopian central statistical agency (CSA). The 2016 sample survey was designed to provide reliable estimates of key indicators at the national and regional levels. Similarly, the sample survey was designed to provide estimates for urban and rural areas [17].
In the 2016 EDHS, a two-stage stratified random sampling technique was applied to collect data from nine regions, two administrative cities, and urban and rural areas. There were 21 strata selected for sampling. Enumeration areas (EAs) samples were collected independently from each stratum in two stages. At every lower level of administration, implicit stratification and proportional allocation were achieved by sorting the sampling frame within each sampling stratum before sampling, according to administrative units at different levels, and using a probability proportional to sample size selection at the first sampling stage [17].
In the first stage, 645 EAs were independently selected in each stratum with probability proportional to the EAs size. Among 645 EAs, 202 EAs were for urban, and 443 EAs were for rural areas. In the second stage, an equal probability systematic sampling was used to select 28 households per cluster from the newly created household lists. The height and weight measurements were collected from children 0–59 months, women aged 15–49 years, and men aged 15–59 years [17] in all the selected households. In this study, we comprised 8441 households of children under five within 87 zones of Ethiopia. The sample sizes are ranged from 5 to 457 households, with an average of 97 households in all 87 surveyed areas (zones special zones and special woredas). And also, there were eight non-sampled zones (special zones and special woredas).
2.1Variables of the study
2.1.1Target variables
The target variables to estimate using small area estimation techniques have been the
2.1.2Auxiliary variables
The auxiliary variables used in this study were obtained from Ethiopia’s 2007 population and housing census. They were derived from census data in two ways: through characteristics associated with children under five and parents. In one instance, the sex (male or female) and age (below one year, 1–2 years, and 4–5 years) of children under five years of age were collected. On the other hand, the auxiliary variables of parents were: sex (male and female), place of residence (urban and rural), age (15–24, 25–34, 35–44, and 45–49), source of drinking water (improved and unimproved), educational levels (non-educated, primary and secondary and above), literacy (literate and illiterate), marital status (married, never married and others), type of toilet facility (have toilet facility and doesn’t have toilet facility), the number of sons died (no died, one died and two and more died ), the number of daughters died (no died, one died and two and more died ), the number of families in the household (less than five, and five and more), and disability (disabled and not disabled), and employment status (government-employed, private employed, self-employed, employer, unemployed and other employment [1, 2, 3, 4, 5].
These variables are aggregated at the zonal level to be used as auxiliary variables in small area estimations. In the study, 41 auxiliary variables were taken into account. However, the suitable variables are chosen using principal component analysis (PCA), which reduces the number of variables to a few key components with minor information loss [10].
2.2Multivariate Fay Herriot model
Various extensions of the basic area-level model have been developed in the literature [1, 7, 8, 11, 12]. One type of extension leads to extending the UFH model to a multivariate Fay-Herriot model to take advantage of the correlations between different characteristics of interest [7, 8]. Suppose the population is decomposed into m areas. Let
(1)
Where the vector
(2)
where
(3)
where
We can rewrite model (3) in matrix form as follows
(4)
where
In our study the characteristics interest of target variables were stunting
Therefore, the direct estimator of the variance covariance matrix (R) of the multivariate data for z scores of stunting
where
This study considered three particularizations of models under the Fay Herriot model in Eq. (4) concerning the arguments of correlated characteristics of interest. The first model is assumed to have zero correlation between the target variables (stunting, wasting, and underweight). This is particularly the UFH model. The second model is a model that assumed the existence of correlations with the homogeneity of the covariance matrix of the area-level random effects. Therefore, in model two, the model variance component parameters are the correlation (
The area level (zonal level in our case) random component
Correlations (
The last model is the heteroscedastic version of the second model, such that there exist correlations with heterogeneous random variances components. Model 3 is known as the heteroscedastic autoregressive multivariate Fay-Herriot model (HAR(1)), and its random error component is as follows [7, 8]:
2.2.1Multivariate small area estimates under Fay Herriot model
The MFH in model (4) can be written in the form of general linear mixed model [6]. The multivariate empirical best linear unbiased prediction (EBLUP) of area level random effects are (
(5)
The most common practical problem in small area estimation is measuring the variability associated with EBLUP under the MFH model. The variability in these estimators is measured using mean squared error. The MSE of the multivariate EBLUP defined under the MFH model (4) is followed [7]. The MSE of multivariate EBLUP estimator is obtained by taking the diagonal covariance of matrix
Where
(6)
Where,
Figure 1.
Normal Q-Q plots of the residuals for stunting, wasting and underweight.
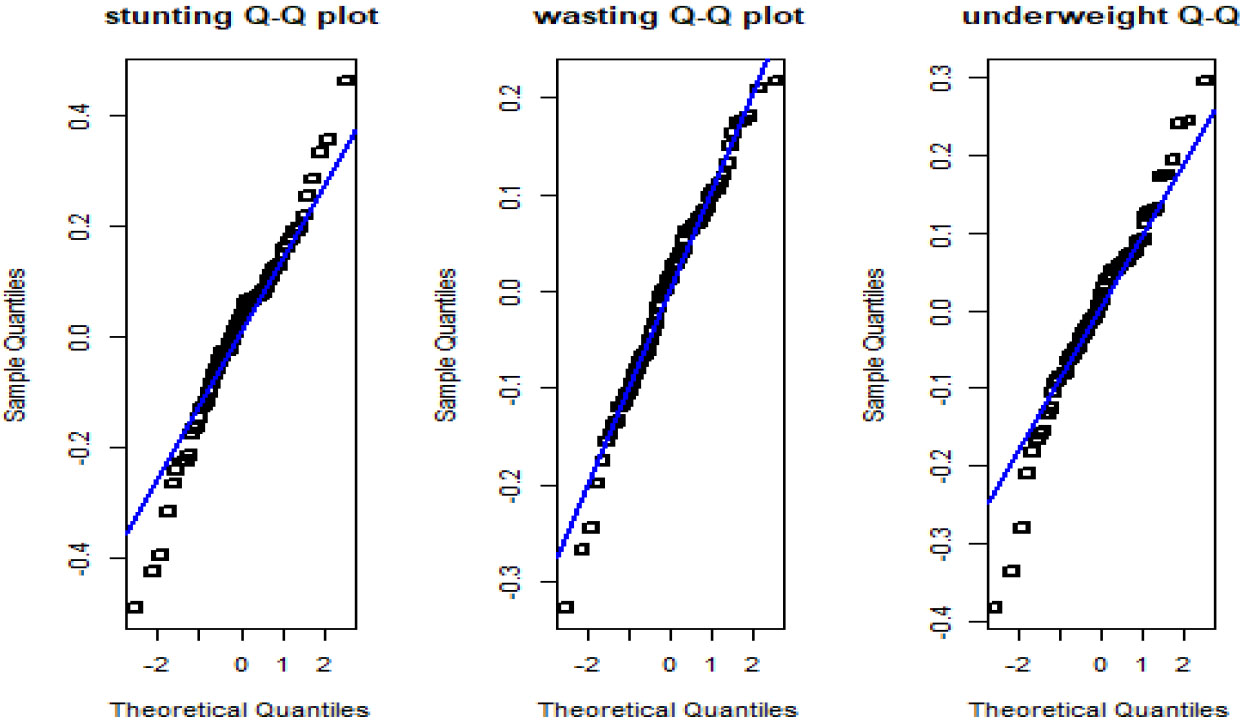
3.Results
3.1Variables selection and model comparison
This study took into account many auxiliary variables (approximately 41). On the other hand, the appropriate variables are chosen using principal component analysis (PCA), which reduces the number of variables to a few key components with minimal information loss [10]. PCA generates components that concentrate more explained variation in the first few principal components than any other variable in the original data. PCA uses an orthogonal transformation process to turn a set of observations of possibly correlated variables into a set of principal component values, which are uncorrelated variables. The number of primary components produced was 41, the same as the number of auxiliary variables. Nonetheless, the first eight principal components were kept (Table 1) since their eigenvalues were greater than one, and they explained 85.67 percent of the overall fluctuations [37].
Table 1
The first 8 principal components with eigenvalues
Eigenvalue | Difference | Proportion | Prin (%) | |
---|---|---|---|---|
1 | 16.23 | 9.57 | 0.3959 | 39.59 |
2 | 6.66 | 2.35 | 0.1624 | 55.83 |
3 | 4.31 | 1.91 | 0.1051 | 66.34 |
4 | 2.41 | 0.57 | 0.0587 | 72.20 |
5 | 1.83 | 0.29 | 0.0446 | 76.66 |
6 | 1.54 | 0.43 | 0.0376 | 80.42 |
7 | 1.11 | 0.06 | 0.0270 | 83.12 |
8 | 1.05 | 0.15 | 0.0255 | 85.67 |
In this result, we compared different multivariate Fay Herriot models to check the existence of correlations among target variables and the heteroscedasticity of covariance for random area effects. Accordingly, we equated the models using z scores of undernutrition to check the existence of correlations and to decide relatively the best model for further analysis. The estimates of the model variance component estimators (
Figure 2.
Bias diagnostic plot with
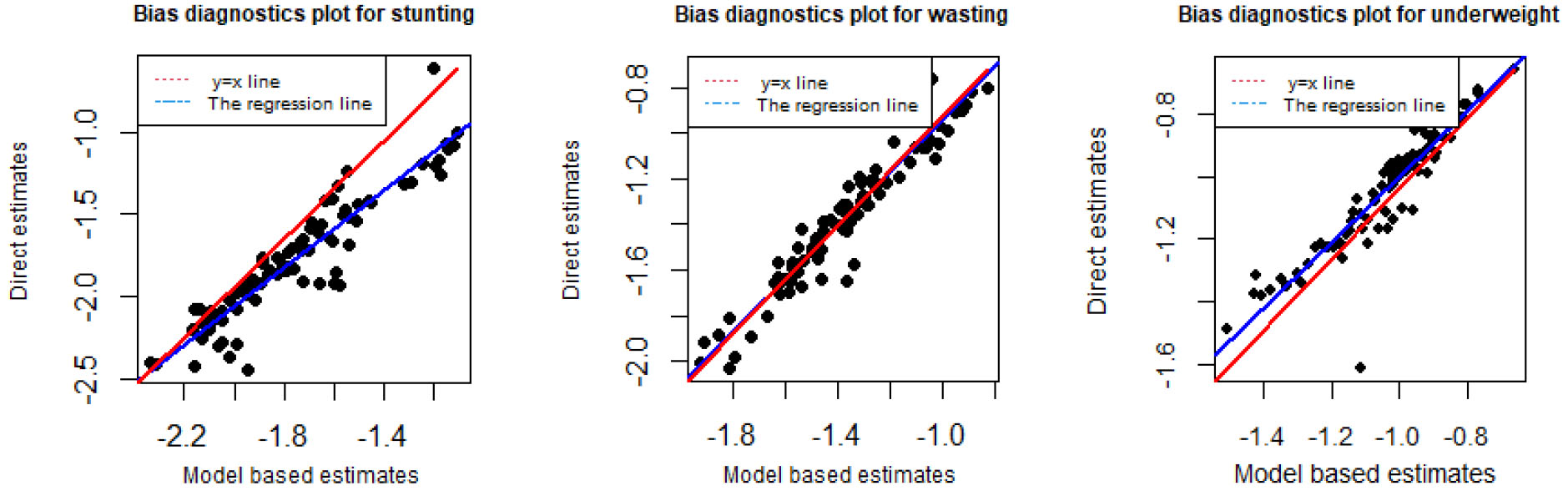
3.2Model diagnostics measures
The normality assumptions of the sampling error under the homogeneous variance in the MFH model have been detected by the normal probability (Q-Q) plots (Fig. 1) and the Shapiro-Wilk test. The assumptions of normality were validated by probability (Q-Q) plots of the sampling error residuals for the three target variables of stunting, wasting, and underweight (Fig. 1). In addition to this, the Shapiro-Wilk test was carried out to measure the normality assumptions of the target variables. The
3.3The performance measures and improvements
The model diagnostics measures of small area estimates under the homogeneous variance MFH model are based on the arguments that model-based small area estimates should be consistent, unbiased, and more precise than direct survey estimates. The model-based small area estimates should provide an approximation to the direct survey estimates, so these values are consistent and close to the expected values of direct estimates. The small area estimates under the MFH model should have less variability than the corresponding direct survey estimates. The bias diagnostics, CV, and root MSE measures were performed in this research for the reliabilities, validities, and precisions of the model-based multivariate small area estimate for the three target variables.
The direct survey estimates on the y-axis vs. the model-based multivariate small area estimates on the x-axis are plotted (Fig. 2). The bias diagnostic plots in (Fig. 2) tested the argument that the deviation of the multivariate small area estimates regression line (blue line) from the line of equality
Furthermore, we can compute the Wald test statistic for the goodness of fit diagnostic. Following [38], the difference between the model-based estimates and the direct survey estimates should not be significant. The value of the Wald test statistic for the goodness of fit diagnostic follows
Table 2
Summary statistics of CV (%) for direct, UFH and MFH model estimates for the three target variables (the
Values | Sample sizes | Stunting | Wasting | Underweight | ||||||
---|---|---|---|---|---|---|---|---|---|---|
Direct | UFH | MFH | Direct | UFH | MFH | Direct | UFH | MFH | ||
Minimum | 3 | 3.26 | 3.19 | 3.02 | 3.84 | 3.65 | 3.58 | 3.40 | 3.30 | 3.16 |
Q1 | 38 | 5.41 | 4.89 | 4.34 | 6.93 | 5.73 | 5.44 | 5.79 | 5.18 | 4.60 |
Median | 78 | 7.57 | 6.42 | 5.28 | 9.54 | 7.60 | 6.66 | 8.53 | 6.77 | 5.79 |
Mean | 97 | 9.45 | 6.80 | 5.55 | 11.37 | 7.88 | 6.98 | 9.54 | 6.79 | 6.14 |
Q3 | 126 | 11.16 | 7.98 | 6.35 | 13.47 | 9.61 | 7.81 | 10.91 | 8.00 | 7.20 |
Maximum | 457 | 32.93 | 12.63 | 9.65 | 34.02 | 15.18 | 10.33 | 41.60 | 12.11 | 11.47 |
NB: all CV (%) are in absolute values.
Figure 3.
Zones (sorted by decreasing sample size) root MSE’s of direct, EBLUP UFH and EBLUP MFH model estimates of stunting, wasting and underweight for children under age five.
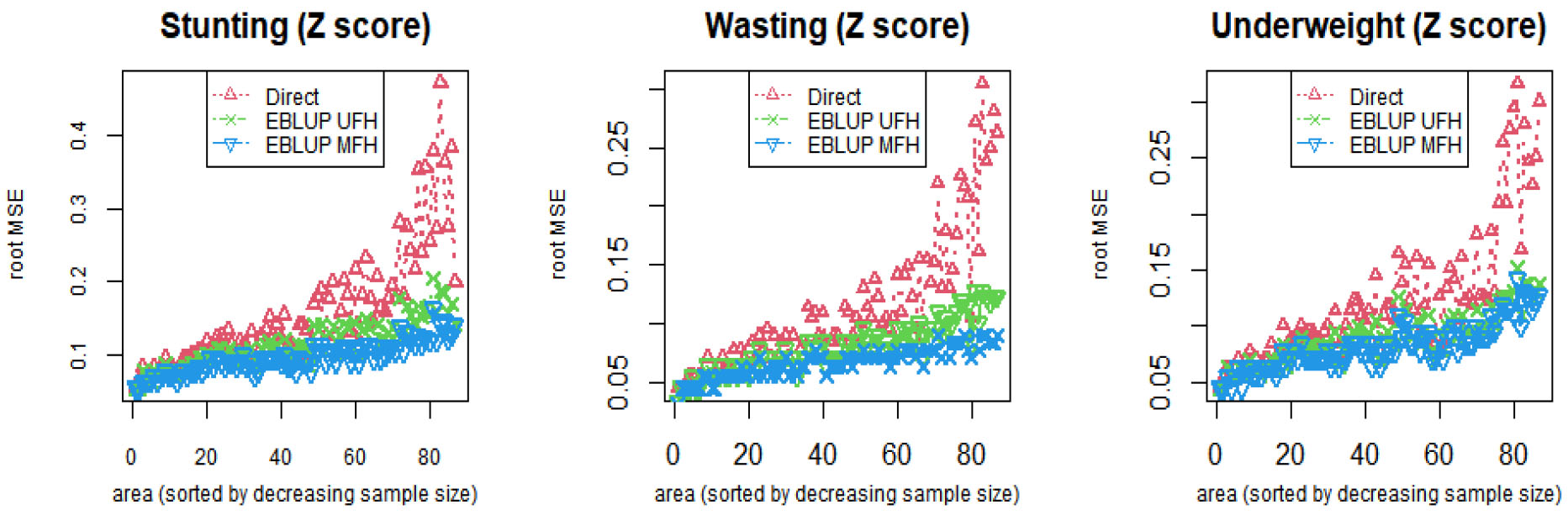
A summary statistics of the CV (%) of the direct, univariate EBLUP and multivariate EBLUP of stunting, wasting, and underweight were presented in (Table 2). The CV (%) of the multivariate small area estimates (both UFH and MFH) is less than the direct survey estimates for all target variables. The CV (%) of direct survey estimates varied from 3.26 to 32.93 with a mean value of 9.45 for stunting, 3.84 to 34.02 with a mean value of 11.37 for wasting, and 3.40 to 41.60 with a mean value of 9.54 for underweight (Table 2). On the other hand, the CV (%) of univariate small area estimates under the UFH model ranged from 3.19 to 12.63 with a mean value of 6.80 for stunting, 3.65 to 15.18 with a mean value of 7.88 for wasting, and 3.30 to 12.11 with a mean value of 6.79 for underweight. In addition, the multivariate small area estimates of CV (%) were varied from 3.84 to 9.65 with a median value of 6.80 for stunting, 3.58 to 10.33 with a median value of 6.66 for wasting, and 3.16 to 11.47 with a median value of 5.79 for underweight in (Table 2). The summary statistics of the first and the third quartiles show that the CV (%) of the direct survey estimates are more than the model-based estimates (both in UFH and MFH models) in all target variables.
Figure 4.
Zones (sorted by decreasing sample size) CV (%) of direct, EBLUP UFH and EBLUP MFH estimators of stunting, wasting and underweight for children under age five.
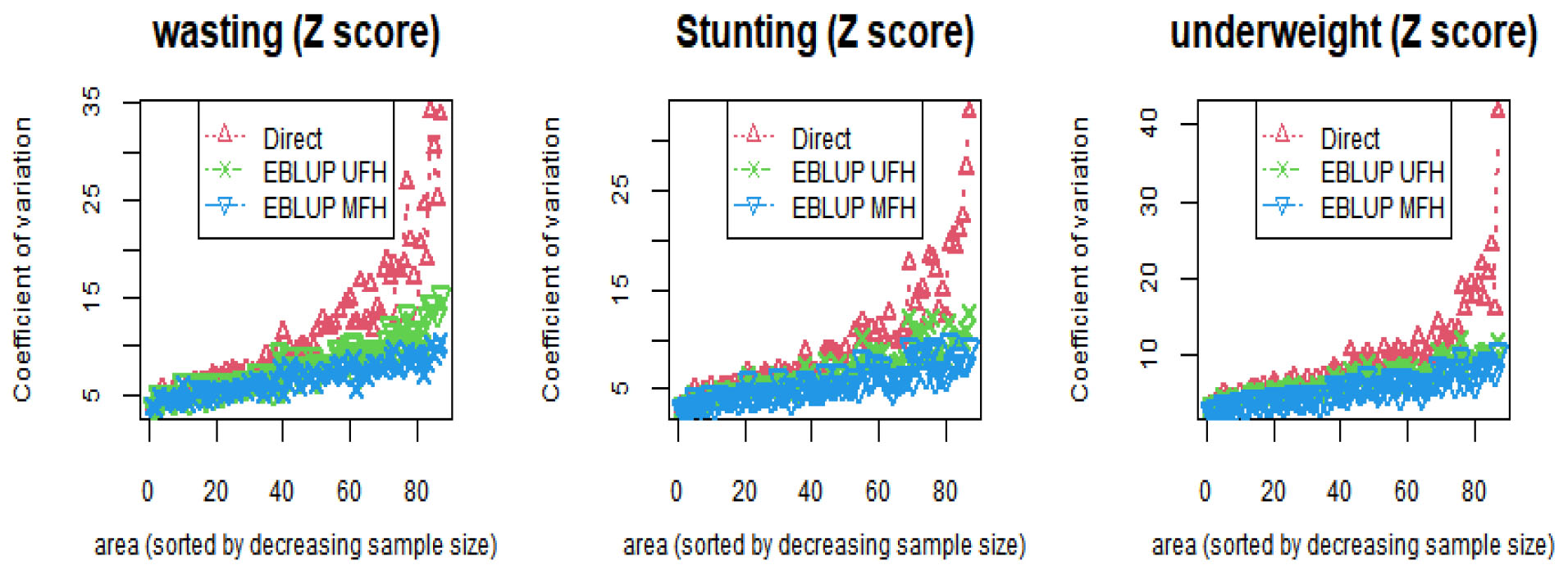
The root MSEs and the CV (%) of the direct survey estimate, univariate and multivariate EBLUPs of stunting, wasting, and underweight were reported in (Figs 3 and 4) respectively. In (Fig. 3), the zone-specific (with decreasing sample size) root MSE of multivariate small area estimates (blue) is smaller than the direct survey estimates (red) and the univariate small area estimates (green) for stunting, underweight, and wasting. The direct survey estimates have unacceptably high standard errors for all target variables as the sample size decreases (Fig. 3). However, the root MSE of the direct survey estimates are improved by model-based multivariate small area estimates due to the strong correlations among stunting, wasting, and underweight. Therefore, the results indicated that the direct survey estimates of all the three target variables are unstable (Table 2), (Fig. 3), and (Fig. 4). On the other hand, multivariate EBLUP with the smallest CVs is considered more reliable and precise estimates of undernutrition.
Table 3
Summary results of efficiency gain of MFH over UFH and direct survey estimates
Efficiency gain MFH over Direct estimate | Efficiency gain MFH over UFH | |||||
Statistics | Stunting | Wasting | Underweight | Stunting | Wasting | Underweight |
Minimum | 6.89 | 4.48 | 5.94 | 3.87 | 2.27 | |
1 | 21.94 | 13.86 | 18.70 | 12.96 | 6.42 | 7.94 |
Median | 30.63 | 19.82 | 25.77 | 17.32 | 10.77 | 9.69 |
Mean | 33.44 | 23.66 | 28.43 | 17.05 | 12.72 | 9.53 |
3 | 43.66 | 29.91 | 33.73 | 21.28 | 18.44 | 11.16 |
Maximum | 72.14 | 57.99 | 72.42 | 29.93 | 36.49 | 16.72 |
Table 4
Summary statistics for small area estimation under MFH Model for non-sampled zones
Statistic | Direct | EBLUP | CV for direct estimate | CV for EBLUP | ||||||||
---|---|---|---|---|---|---|---|---|---|---|---|---|
Stunt | Wast | Under weight | Stunt | Wast | Under weight | Stunt | Wast | Under weight | Stunt | Wast | Under weight | |
Min | – | – | – | 1.61 | 1.37 | 1.04 | – | – | – | 4.51 | 4.69 | 7.01 |
Q1 | – | – | – | 1.78 | 1.48 | 1.08 | – | – | – | 8.20 | 8.27 | 11.73 |
Mean | – | – | – | 1.90 | 1.59 | 1.18 | – | – | – | 13.66 | 13.49 | 17.21 |
Q3 | – | – | – | 2.04 | 1.71 | 1.26 | – | – | – | 19.33 | 18.29 | 21.60 |
Max | – | – | – | 2.09 | 1.73 | 1.43 | – | – | – | 23.28 | 23.13 | 29.45 |
The summary efficiency gain results for multivariate EBLUP estimates over the univariate EBLUP estimates, and the direct survey estimates were presented in (Table 3). The maximum efficiency gain for multivariate EBLUP over direct survey estimates was recorded 72.14 in Bahir Dar, 57.99 in Derashe special woreda, and 72.42 in Hawasa for stunting, wasting, and underweight, respectively. Similarly, the multivariate EBLUP over the univariate EBLUP was 29.93 for Bahir Dar, 39.49 for Huru guduru, Wollega, and 16.72 for Mao komo special zone for the target variables stunting, wasting, and underweight, respectively. The maximum improvements in efficiency have been recorded for zones with small sample sizes. For instance, Hawasa city and Bahir Dar city have the smallest sample sizes, 5 and 7, respectively. Whereas the minimum efficiency gain in CV was recorded for large sample sizes. The minimum efficiency gains in CV for multivariate over univariate small area estimates have been recorded: 3.87 in Korahe,
Table 4 reported the summary statistics of the non-sampled zones (zero sample size for each zone) under the MFH model for stunting wasting and underweight. There were eight non-sampled zones (Adama special zone, Amaro, Argoba, Basketo, Burayu, Fiq, Jimma special zone, and Konso special woreda) under 2016 Ethiopian DHS. Small area estimate is the optimum approach for non-sampled zones or zones with zero sample sizes [32]. The small area estimates and CV (%) of stunting, wasting, and underweight were estimated based on the MFH model for non-sampled zones using auxiliary covariates from the census (Table 4). We cannot establish a comparison of CV for non-sampled zones since there are no direct survey estimates for them. The small area estimates and the CV of the non-sampled zones, on the other hand, are consistent with the results of the sampled zones.
4.Discussion
The target variables of the study were obtained from the 2016 Ethiopian demographic and health survey (EDHS), and the auxiliary variables are taken from the 2007 population and housing census data. Principal component analysis was used to reduce the number of auxiliary variables to a few informative variables. Unlike the survey study by [16, 40], this study was the small area estimations of unplanned domains with small sample sizes for the correlated target variables by borrowing strength from the census data. In contrast to the univariate FH model, which ignores the correlation among the target variables [41, 42, 43], this study considered the correlations among three target variables for a small sample size. Following the correlations of the target variables, the univariate model becomes the MFH model [7, 8, 11]. We considered three particularizations of the model in [7] to detect the existence or nonexistence of a correlation and heteroscedasticity. Likewise, we discussed the three models in detail, and the homogeneous variance MFH model was considered appropriate.
The normality assumption of the selected model is valid via the Shapiro-Wilk test and normal Q-Q plot. The Q-Q plots and the Shapiro-Wilk test have concluded that the sampling errors are expected to be normally distributed [10, 11]. The bias diagnostic measures and the Wald test statistic for the goodness of fit indicated that the model-based multivariate small area estimates are likely to be consistent with the direct survey estimates for all target variables. These diagnosis checking were coherently agreed with the previous studies [10, 11, 12, 38].
The performance measures of the multivariate EBLUP over the univariate small area estimates and the direct survey estimates were measured by CV and root MSE [7, 8, 9]. The direct survey estimates of the target variables (stunting, wasting, and underweight) had the largest variabilities with small sample sizes. The root MSE of the direct survey estimates were more than the corresponding model-based multivariate EBLUP [7]. This is because of considering the correlation between related target variables of interest in the model-based multivariate EBLUP estimates [10].
The best efficiency gains were recorded for the model-based multivariate EBLUPs over the direct survey estimates in all zones for small sample sizes. The Multivariate EBLUP improved the univariate small area estimates and direct estimates. These improvements were obtained because of the correlations of the target variables [10]. Thus, multivariate EBLUP under the MFH model is the best reliable, efficient, and precise for all target variables stunting, wasting, and underweight [8, 10, 44, 45]. Multivariate small area estimates improve the univariate small area estimates and direct survey estimates due to the correlations among the target variables. The summary statistics of the non-sampled zones for all target variables were produced under the MFH model [1, 5, 32, 36]. The estimation of non-sampled zones under the MFH model is precise and adequate with similar approaches to the results of sampled zones [32]. The availability of reliable and adequate estimates of non-sampled zones will help the government give them equal attention to 87 sampled zones.
5.Conclusions
This study applied the MFH models using the 2016 survey by linking with 2007 population and housing census data. Under the three MFH models, the homogeneous variance MFH model is appropriate to obtain relatively reliable, efficient, and precise estimates. The model diagnostic measures directed that the model-based multivariate small area estimates are reliable to the direct survey estimates. Furthermore, the MFH model’s sampling errors are normally distributed for all target variables. The CV and the root MSE indicated that the model-based multivariate small area estimations are the most reliable, efficient, and precise under the homogeneous MFH model. The performance measures of CV and root MSE under the MFH model provided a significant gain in efficiency to obtain zonal level estimates of stunting, wasting, and underweight. The direct survey estimates are some high standard errors with small sample sizes, but these high standard errors are reduced by using multivariate small area estimates. Multivariate small area estimates highly improve the direct survey estimates because they have significant correlations between stunting, wasting and underweight.
In Ethiopia, surveys are planned to produce national and regional level estimates, while zonal levels are not considered in the survey. However, zones are an essential domain for the planning and monitoring process, and therefore the availability of zonal level statistics plays a prominent role for planning and policymakers. This study has produced the best reliable, efficient, and precise statistics with sampled and non-sampled zones and provides valuable information to policymakers, planners, and legislative organs of the government. One of the novelties of this paper is estimating the non-sampled zones, and therefore the policymakers will give equal attention similar to the sampled zones.
Acknowledgments
We would like to express our gratitude to the paper’s editors and reviewers. We would like to thank the DHS Program and the Ethiopian CSA for providing us access to the data. We would also like to thank Bahir Dar, and Debre Tabor Universities.
References
[1] | Rao JNK, Molina I. Small area estimation. 2nd ed. Hoboken: (2015) . |
[2] | Fay RE, Herriot RA. Estimates of income for small places: an application of james-stein procedures to census data. J Am Stat Assoc (1979) ; 74: : 269-77. doi: 10.1080/01621459.1979.10482505. |
[3] | Datta GS, Rao JNK, Smith DD. On measuring the variability of small area estimators under a basic area level model. Biometrika Trust (2005) ; 92: : 183-96. |
[4] | Shiferaw Y, Galpin J. A corrected confidence interval for a small area parameter through the weighted estimator under the basic area level model. J Iran Stat Soc. (2019) ; 18: : 17-51. doi: 10.29252/jirss.18.1.17.A. |
[5] | Shiferaw Y, Galpin J. Improved Confidence Intervals for a Small Area Mean Under The Fay-Herriot Model. University of the Witwatersrand, (2016) . |
[6] | Fay RE. Application of multivariate regression to small domain estimation. Small Area Stat (1987) ; 91-102. |
[7] | Benavent R, Morales D. Multivariate Fay-Herriot models for small area estimation. Comput Stat Data Anal. (2016) ; 94: : 372-90. doi: 10.1016/j.csda.2015.07.013. |
[8] | Ubaidillah A, Notodiputro KA, Kurnia A, Mangku IW. Multivariate Fay-Herriot models for small area estimation with application to household consumption per capita expenditure in Indonesia. J Appl Stat. (2019) ; 46: : 2845-61. doi: 10.1080/02664763.2019.1615420. |
[9] | Guha S, Chandra H. Measuring disaggregate level food insecurity via multivariate small area modelling: evidence from rural districts of Uttar Pradesh, India. Food Secur. (2021) ; 13: : 597-615. doi: 10.1007/s12571-021-01143-1. |
[10] | Guha S, Chandra H. Measuring and mapping disaggregate level disparities in food consumption and nutritional status via multivariate small area modelling. Soc Indic Res. (2021) ; 154: : 623-46. doi: 10.1007/s11205-020-02573-8. |
[11] | Moretti A, Shlomo N, Sakshaug JW. Multivariate small area estimation of multidimensional latent economic well-being indicators. Int Stat Rev. (2019) ; 88: : 1-28. doi: 10.1111/insr.12333. |
[12] | Moretti A, Shlomo N, Sakshaug JW. Parametric bootstrap mean squared error of a small area multivariate EBLUP. Commun Stat Simul Comput. (2018) ; 49: : 1474-86. doi: 10.1080/03610918.2018.1498889. |
[13] | Ito T, Kubokawa T. On measuring the variability of small area estimators in a multivariate fay-herriot model. (2018) : 1-21. |
[14] | Muller O, Krawinkel M. Malnutrition and health in developing countries. Cmaj. (2005) ; 173: : 279-86. |
[15] | UNICEF, WHO, World Bank. Levels and trends in child malnutrition: Key findings of the 2020 Edition of the Joint Child Malnutrition Estimates. Geneva WHO. (2020) ; 24: : 1-16. |
[16] | Tekile AK, Woya AA, Basha GW. Prevalence of malnutrition and associated factors among under-five children in Ethiopia: evidence from the 2016 Ethiopia Demographic and Health Survey. BMC Res Notes. (2019) ; 12: : 1-6. doi: 10.1186/s13104-019-4444-4. |
[17] | CSA, ICF. Ethiopia Demographic Health Survey. Addis Ababa, Ethiopia, and Rockville, Maryland, USA: CSA and ICF, (2016) . |
[18] | De Onis M. WHO child growth standards. (2006) . |
[19] | Amare D, Negesse A, Tsegaye B, Assefa B, Ayenie B. Prevalence of undernutrition and its associated factors among children below five years of age in Bure Town, West Gojjam Zone, Amhara National Regional State, Northwest Ethiopia. Adv Public Heal. (2016) ; 2016: : 8. doi: 10.1155/2016/7145708. |
[20] | Endris N, Asefa H, Dube L. Prevalence of malnutrition and associated factors among children in rural Ethiopia. Biomed Res Int. (2017) ; 2017: : 6. doi: 10.1155/2017/6587853. |
[21] | Gebre A, Reddy PS, Mulugeta A, Sedik Y, Kahssay M. Prevalence of malnutrition and associated factors among under-five children in pastoral communities of Afar Regional State, Northeast Ethiopia: a community-based cross-sectional study. J Nutr Metab. (2019) ; 2019: : 13. doi: 10.1155/2019/9187609. |
[22] | Tadesse S, Alemu Y. Urban-rural differentials in child undernutrition in Ethiopia. Int J Nutr Metab. (2015) ; 7: : 15-23. doi: 10.5897/IJNAM2014.0171. |
[23] | Woodruff BA, Wirth JP, Bailes A, Matji J, Timmer A, Rohner F. Determinants of stunting reduction in Ethiopia 2000–2011. Matern Child Nutr. (2017) ; 13: . doi: 10.1111/mcn.12307. |
[24] | Yeshaw Y, Kebede SA, Liyew AM, Tesema GA, Agegnehu CD, Teshale AB, et al. Determinants of overweight/obesity among reproductive age group women in Ethiopia: multilevel analysis of Ethiopian demographic and health survey. BMJ Open. (2020) ; 10: : e034963. doi: 10.1136/bmjopen-2019-034963. |
[25] | Fenta HM, Zewotir T, Muluneh EK. Spatial data analysis of malnutrition among children under-five years in Ethiopia. BMC Med Res Methodol. (2021) ; 21: : 1-13. doi: 10.1186/s12874-021-01391-x. |
[26] | Fenta HM, Zewotir T, Muluneh EK. A machine learning classifier approach for identifying the determinants of under – five child undernutrition in Ethiopian administrative zones. BMC Med Inform Decis Mak. (2021) ; 21: : 1-12. doi: 10.1186/s12911-021-01652-1. |
[27] | Fenta HM, Zewotir T, Muluneh EK. Disparities in childhood composite index of anthropometric failure prevalence and determinants across Ethiopian administrative zones. PLoS One. (2021) ; 16: : 1-17. doi: 10.1371/journal.pone.0256726. |
[28] | Woldie M, Jirra C, Azene G. Presence and use of legislative guidelines for the distribution of decentralized decision making authority in the Jimma zone health system, Southwest Ethiopia. Ethiop J Health Sci. (2011) ; 21: : 29. |
[29] | Kitaw Y, Teka G-E, Meche H, Damen H, Fentahun M. The evolution of public health in Ethiopia. 2nd ed. (2012) . |
[30] | Klaver W. Underweight or stunting as an indicator of the MDG on poverty and hunger. (2010) . |
[31] | Chubb H, Simpson JM. The use of Z-scores in paediatric cardiology. Ann Pediatr Cardiol. (2012) ; 5: . doi: 10.4103/0974-2069.99622. |
[32] | Chandra H. Exploring spatial dependence in area-level random effect model for disaggregate-level crop yield estimation. J Appl Stat. (2013) ; 40: : 823-42. doi: 10.1080/02664763.2012.756858. |
[33] | CSA. Federal Democratic Republic of Ethiopia Central Statistical Agency Population Projection of Ethiopia for All Regions At Wereda Level from 2014–2017. (2013) . |
[34] | Gizaw Z, Woldu W, Bitew BD. Acute malnutrition among children aged 6–59 months of the nomadic population in Hadaleala district, Afar region, northeast Ethiopia. Ital J Pediatr. (2018) ; 44: : 1-10. doi: 10.1186/s13052-018-0457-1. |
[35] | Wirth JP, Rohner F, Petry N, Onyango AW, Matji J, Bailes A, et al. Assessment of the WHO Stunting Framework using Ethiopia as a case study. Matern Child Nutr. (2017) ; 13: : e12310. doi: 10.1111/mcn.12310. |
[36] | Permatasari N, Ubaidillah A. Msae: An R Package of Multivariate Fay Herriot Models for Small Area Estimation. R J (2021) ; 1-12. |
[37] | Warnholz S. Small area estimation using robust estenstions to area level models theory, implementation and simulation studies. Freie Universität Berlin, (2016) . |
[38] | Mukhopadhyay PK, McDowell A. Small area estimation for survey data analysis using SAS software. SAS Glob. Forum, vol. 2011, (2011) , p. 96. |
[39] | Ogwu MC, Osawaru M. Principal component analysis: A tool for multivariate analysis of genetic variability. African J Plant Sci. (2016) . |
[40] | Brown G, Chambers R, Heady P, Heasman D. Evaluation of small area estimation methods – an application to unemployement estimats from the UK LFS. Proc. Stat. Canada Symp. (2001) ; pp. 1-10. doi: 10.1201/9780203166314.ch1. |
[41] | Li H. Small Area Estimation: An Empirical Best Linear Unbiased Prediction Approach. University of Maryland, (2007) . |
[42] | Rajkumar AS, Gaukler C, Tilahun J. Combating Malnutrition in Ethiopia: An Evidence-Based Approach for Sustained Results: World Bank Publications. (2012) . doi: 10.1596/978-0-8213-8765-8. |
[43] | Rao J. Small-area estimation. Wiley StatsRef Stat Ref Online. (2014) ; 1-8. doi: 10.1002/9781118445112.stat03310.pub2. |
[44] | Datta GS. Model-based approach to small area estimation. Sample Surv Inference Anal. (2009) ; 29B: : 251-88. doi: 10.1016/S0169-7161(09)00232-6. |
[45] | Islam S, Chandra H. Small area estimation combining data from two surveys. Commun Stat Comput. (2019) ; 36: : 1-22. doi: 10.1080/03610918.2019.1588308. |
[46] | Islam S, Chandra H, Aditya K, Lal SB. Small area estimation under a spatial model using data from two surveys. Int J Agric Stat Sci. (2018) ; 14: : 231-7. |
[47] | Esteban MD, Morales D, Pérez A, Santamaría L. Area-level time models for small area estimation of poverty indicators. Comb Soft Comput Stats Methods. (2010) ; 77: : 233-7. doi: 10.1007/978-3-642-14746-3_29. |