Training official statisticians for adaptive statistical practice
Abstract
Statistics is undergoing what feels like an evolutionary jump, i.e., a period of rapid and sweeping developments brought about by dramatic shifts in its environment. The repercussions are felt strongly by official statistics, which operates at the forefront of societal and economic change. In this paper, we look at the implications of the recent developments for the training of official statisticians and highlight key knowledge areas for successfully navigating the emerging landscape. In addition, we employ the concept of adaptive expertise to help us identify three qualities that support the independent and lifelong development of practicing statisticians, and propose five teaching strategies for fostering these qualities in the classroom.
1.Introduction
During the past two decades, the world has experienced momentous change in the way it produces, processes, and uses information. This transformation has been fuelled by technological progress in data storage, electronic communications, and computational power, and its effects are manifest in our everyday lives, from targeted advertising to personalized medicine. All together these developments are often characterized as the Data Revolution.
The digital and data revolutions have had important implications for official statistics. The monopoly held by official statistics, to provide timely and high-quality statistics for free is being challenged by the proliferation of readily available, ad-hoc and fragmentary data [1]. This and other social, environmental and economic changes, such as, globalisation, digitalisation, climate change, ideological battles as to whether data are a public or a proprietary good and changing attitudes to data privacy pose significant challenges for official statistics [2]. The continued relevance of official statistics will rely on its capacity to adapt in the midst of constant change, and a critical factor in this is the training of new official statisticians to ensure they understand the political – social – economic environment in which they operate.
University training of official statisticians has traditionally focused on mathematical statistics, especially core topics such as probability theory, statistical modelling, and methods for sampling and inference. This is typically performed in the context of a Bachelors’ and/or Masters’ degree in Statistics, although specialized programs such as the recent European Masters in Official Statistics (EMOS) have more targeted curricula [3]. Although the term ‘statistics’ started as synonymous with official demographic and economic data, the scientific field of Statistics eventually assumed a much broader scope, becoming the foremost discipline tasked with extracting knowledge from general data. This primacy, however, is now being challenged from emerging fields such as Machine Learning, Data Mining, Data Science, and Data Analytics, which claim similar goals. This has caused considerable soul-searching within the discipline, as Statistics is trying to rediscover its place in this new competitive world. But there is also a growing consensus that Statistics needs to embrace recent developments, such as algorithmic learning, while upholding its traditional strengths and, most importantly, its principled approach to data collection and inference. For example, the American Statistical Association (ASA) issued a statement on the role of Statistics in Data Science [4] calling for a ‘sustained and substantial collaborative effort’ in which ‘statisticians must engage, learn from, teach, and work with ‘researchers with expertise in data management and computation.’ In their epilogue, Efron and Hastie [5] offer a historical perspective of Statistics’ shifting focus between applications, mathematics, and computation, and provide examples of recent developments fuelled by applications and computation, the type of which will guarantee the sustained importance of the field.
Besides recent developments in statistical research and practice, there are significant efforts underway to update statistical education. There have been calls to broaden undergraduate curricula going back to at least Cobb [6], who recommends emphasizing statistical thinking using real data and active learning. More recently, Horton and Hardin [7] recognized that ‘the traditional statistics curriculum with mathematical foundations has not kept up with pressing demands for students who can make sense of data’ and call for curricula that ‘prepare students to engage in the entire data analysis process’. Specifically, Nolan and Temple Lang [8] argue that ‘computational literacy and programming are as fundamental to statistical practice and research as mathematics’ and propose a more central role for computing in teaching statistics. While these calls have been around for some time, the emergence of ‘Data Science’ has given new impetus and urgency to their adoption. Many academic departments are now implementing reforms aimed at balancing theory and methodology with computing and statistical practice, along the lines summarised in the most recent ASA curriculum guidelines [9]. These reforms are facilitated by the ready availability of data, computational power, and open-source software, which have opened up new ways of teaching statistics (e.g., simulation-based inference) and integrating empirical work in the classroom (e.g., collaborative projects).
A major motivation for the ongoing reforms is not just to teach students new skills and procedures, but to fundamentally develop their ability to understand, evaluate, produce, and communicate statistical results and arguments. The terms statistical literacy, statistical reasoning, and statistical thinking have all been used to describe overlapping aspects of this ability; see Garfield [10] for definitions and delMas [11] for a comparison. For the purposes of this paper, we adopt an expanded view of literacy, which is closer to that put forward by Gal [12], who uses literacy to suggest ‘a broad cluster not only of factual knowledge and certain formal and informal skills, but also of desired beliefs, habits of mind, or attitudes, as well as general awareness and a critical perspective’. Obviously, statistical literacy is a primary learning outcome for educational programmes in statistics, and a critical requirement for any aspiring official statistician working in a National Statistical Office (NSO) or as part of the National Statistical System (NSS). But statistical literacy also relies upon other abilities, more fundamentally on general literacy and numeracy, as well as technological and information literacy. General literacy includes reading and writing, but also a wider appreciation of history, geography, legal issues, ethics and culture. Gore [13] describes reading and writing as ‘democracy’s calisthenics’, and argues that the decline in literacy has impacted our ability to reason and think. More generally, a statistician should understand the broader context of what is being measured – including the history and the appropriateness of the measurement tool. Walter Radermacher [14], former Director General of Eurostat, correctly notes that ‘statistical competence and literacy must not be reduced to mathematical-technical skills. Rather, it also requires an understanding of the fundamentals of the humanities.’ Moreover, information and technological literacy, or the ability to access, evaluate, and use information while making effective use of technology, are becoming essential prerequisites for other forms of literacy.
Even with such bold reforms underway, the rapidly changing landscape of Statistics and Data Science, coupled with fundamental social, economic, environmental and other changes, guarantee that new generations of official statisticians will face conditions and challenges never seen before. In such a dynamic environment, it is important to anticipate the direction of future developments, but also to be able to adapt to unforeseen conditions; in this paper, we try to address both challenges. The following section identifies some of the core skills and central themes that will be essential in the training of official statisticians going forward. Next, we claim that alongside new content and skills, our programs should also cultivate an adaptive mindset, one that will prepare students to apply their knowledge flexibly and creatively, and to learn on their own. Finally, we present strategies for cultivating both core skills and an adaptive mindset, with examples in the context of university courses in Statistics.
2.Requirements of official statistics
It is very difficult to anticipate what specific skills NSOs and NSSs will require in the future. But at this juncture it is hard to imagine that the ‘hard’ statistical skills required of a statistician today will not be as relevant tomorrow. Arguably, these core statistical skills will become more important, rather than less, as graduates become more accustomed to using ‘blackbox’ software packages, but perhaps less accustomed to thinking about the underlying concepts and methodologies. While hard skills will most likely remain an essential ingredient for any statistician in the future, perhaps the necessary skills mix will change. We anticipate that three essential skills will be required: numerical skills; statistical skills; and increasingly, technological skills. Irrespective of whether we are discussing a data scientist, an official statistician or a professional statistician in another field, the requirement for these basic skills is universal. Mathematical and numerical skills should be, more or less, self-explanatory, but crucially a statistician should be able to spot patterns, understand differences between stocks and flows and be comfortable reading and writing in scientific notation. Having statistical skills means being able to work with real, often messy or incomplete data and understanding bias; both the likely sources and what remedial actions can be taken. Statisticians should understand the subtle but important differences between accuracy and precision and also develop a good understanding of concepts like uncertainty and risk. A competent statistician should be able to select and use appropriate statistical techniques and models. Future technological skills are the area hardest to predict. Technology is changing rapidly, with consequences not only for the applications we will use, but also the types of data we may have access to. Here it is very hard for a university to prepare courses for the future and for statistical offices to say with any certainty what will be required. If current trends have anything useful to say, then they suggest a greater use to ‘freeware’ and combining packages. They also suggest a commitment to lifelong learning will be essential.
Any discussion of future requirements should be broadened beyond skills to also include competencies. Statisticians, like any other professionals will need to continually update their skills over the lifetime of their careers. What are less likely to change over time are the basic characteristics or competencies necessary to be a good statistician (see Fig. 1). Specifically, a statistician must be creative, curious, critical, skeptical and resourceful. These are self-explanatory and do not require any elaboration. Perhaps less obvious, a statistician should also be aware of the cultural and civic or political environment in which they operate. It is very important that a statistician not only understands the context in which previous indicators and statistics were compiled, but also that they properly understand the opportunities and constraints that their environment imposes on their practice. For example, when contemplating the use of big data, NSOs may be forced to confront issues before the law is clear or cultural norms have been established. Given the importance of public trust for an NSO, it is essential that statisticians are sensitive to these issues and understand what is acceptable by the public they serve. The challenge for universities is how to nurture and develop competencies.
Statisticians must understand the underlying logic of theory, so that having acquired skills, they can apply them and put theory into practice in a variety of real-life situations (all invariably more complex and messy than the scenarios presented in text books). Other skills, perhaps neglected in the past, but now universally recognized as important, is the ability to communicate well and to present statistics in context. In a world awash with data and cluttered, incoherent messaging, the ability to translate data into comprehensible statistics and digestible messages is absolutely essential. Data visualisation is an important element subset of this skill, but perhaps one where too much emphasis is being placed at the moment. A statistician can only design an effective visualization if they are clear themselves what the key messages are.
A university course that prepares statisticians for a career in official statistics would ideally cover four broad domains: statistical systems; statistical techniques; policy issues; and for want of a better heading, the political economy of data. Thus, ‘official statistics literacy’ incorporates a wider set of domains than the more general concept of statistical literacy presented above. Including this wider mix of issues would enrich the content of university courses, stimulate discussion and give statistics students a better flavor of what their future career might involve.
A.
A. Statistical Systems. A modeule in statistical systems would include: an introduction to the official statistics eco-system that comprises national, regional and global statistical systems; an outline of the mandate and functions of the UN Statistical Commission, the UN regional Commissions and UN specialised agencies, funds and programmes and other important international organisations, such as, the World Bank Group, the International Monetary Fund (IMF), the Organisation for Economic Cooperation and Development (OECD) and Eurostat. The module would explain how these institutions coordinate their work; it would also provide an explanation of the importance of internationally recognized classifications, such as ISIC, CPC, SITC, COICOP, or EBOPS11 and framework methodologies, such as, the System of National Accounts (SNA), Balance of Payments (BoP), System of Environmental-Economic Accounting (SEEA) or International Merchandise Trade Statistics (IMTS), and how they have been developed and continue to evolve.
Statisticians should also be introduced to: the UN Fundamental Principles of Official Statistics [16], the Principles Governing International Statistical Activities [17] and any regionally or nationally appropriate adaptations; professional principles, such as, the ISI Declaration of Professional Ethics [18]; and internationally recognized statistical quality assurance frameworks and codes of practice, such as, the UN Statistical Quality Assurance Framework [19], or the UN National QAF [20] and any regionally or nationally appropriate adaptations. An important element of any statistician’s work (whether official or otherwise) will be dealing with legislation. Official statisticians will need to be conversant with the national statistical law (and those in Europe will also need to know their way around European statistical legislation and GDPR22). Increasingly, concerns regarding privacy and confidentiality (terms often treated as synonymous but in fact very different things) mean that all statisticians and data scientists must understand the legal environment in which they work, in particular, data protection and freedom of information legislation and how they interrelate. But equally, statisticians must understand the human rights arguments regarding access to information (Resolution 59 of the UN General Assembly adopted in 1946 and Article 19 of the 1948 Universal Declaration of Human Rights). As we expect more and more secondary digital data to be generated, understanding of the legal issues of accessing and using these data are critical to ensuring a statistician does no harm [21]. Statistical courses could also usefully devote some time to discussing terms like ‘harm’ and ‘good’ and what they mean.
B. Statistical (and technological) Techniques. Traditional topics like distribution theory, probability, sampling/weighting, multivariate analyses and regression must be included, but so too should some basic, but often ignored, statistical techniques. Unfortunately, NSOs rarely get to work with the clean datasets favoured by university courses – real life data are typically very messy. Therefore, data cleaning (including treatment of outliers, imputation and interpolation) is an essential skill. Statisticians must also be aware of the limitations of abstract models and in particular, the Ricardian vice of oversimplification but also avoid the temptation to overfit. Another technique, too often ignored, is seasonal adjustment. This is essential for analyzing and presenting sub-annual time series, yet frequently young career statisticians do not know how to seasonally adjust time series or how to test time series models to ensure they are appropriate. More could be done to examine real life issues, for example, how to seasonally adjust series in the aftermath of shocks, such as, the 2008 financial crisis or COVID-19. Another area deserving of more attention is index theory and practice – understanding the characteristics and strengths and weaknesses of different index formulae and when it is appropriate to use them. These are essential skills for statisticians working in price, business and macroeconomic statistics.
Increasingly NSOs and international organisations (IOs) are compiling Leading, Composite and Sentiment (LCS) indices, such as, the UNDP Human Development Index; the OECD Better Life Index; the WEF Global Competitiveness Index; or the UNCTAD Productive Capacities Index. Thus the acquisition of factor or Principal Component Analyses skills will be important. The merits and technical challenges of such indices (for example, selection and transformation of sub-indices and weighting) could usefully be discussed, as could their usefulness in the formation of policy.
In a ‘big data’ world, with massive computing power facilitating the linking of records, safeguarding confidentiality and maintaining public trust are becoming greater challenges. The importance of these subjects deserves a prominent place in any statistics curriculum. As noted above, statistical software is likely to come and go with increasing rapidity, and therefore students should be introduced to programming logic, not just how to use the most fashionable software packages. Preparation for lifelong learning should be emphasised.
C. Policy Issues. Beyond technical statistical techniques and tools, universities could play an important role in highlighting some of policy issues that pose significant challenges for public policy (and by extension NSOs). For the purposes of this paper, four interconnected issues are discussed: globalisation; wellbeing; digitalisation; and jobless growth. This selection is by no means exhaustive.33
Understanding the impacts of globalisation is a major policy issue and properly measuring those is a priority issue for statisticians. In an age of deep or hyperglobalisation [22], the traditional, country-based approaches to macroeconomic measurement have reached their limits. With hyperglobalisation, the relationships between national economies are no longer the driving force of globalisation but rather the relationships between large multinational enterprises. Economic and social globalisation is also impacting employment, crisis contagion, trade policy, protectionism and migration. These are problems that nationally based statistics are insufficient to solve [23] and why Karabell has stated that ‘our statistical map … is showing signs of age’ [24]. Consequently, globalisation poses a challenge for the relevance of traditional trade, labour market, price and macro-economic statistics [1].
Figure 2.
Dimensions of official statistical literacy. Source: Author’s presentation.
Measuring wellbeing (sometimes also described or bundled with progress) can be approached from an individual or societal perspective. Since the 1970’s there have been several attempts to supplement GDP with other indicators of progress, such as, the Measure of Economic Welfare [25]; the Genuine Progress Index [26]; the Human Development Index [27]; or the index of Gross National Happiness [28] to name a few. After the 2008 financial crisis those efforts intensified with a raft of new indices being developed, most notably: the European Commission ‘Beyond GDP’;44 OECD Better Life Index;55 and UNEP Inclusive Wealth Index [29]; and more recently, the Eurasian Economic Union ‘Inclusive Growth Index’ [30]. These composite indices attempt to blend elements of human, natural and production capital or the social, economic and environmental pillars of development together. There is growing pressure to incorporate dimensions of climate crisis and environmental degradation into the mix, such as, the Chinese Green GDP Quality index [31]. Equally there is a demand for greater representation of non-economic elements that impact on the human experience. The rationale is obvious but presents a number of measurement challenges. For example, how should the environment, biodiversity, natural ecosystems, spirituality or aesthetic be valued? How can double counting be avoided? How should a single valuation methodology be designed so that it works equally well across all domains? How best to deal with data availability problems, especially for developing countries or marginalised communities [1]?
Digitalisation raises a wide range of policy issues, ranging from robot and data taxation, inclusivity and inequality, investment in infrastructure, implications of blockchain technology, competition, and consumer protection. It also raises issues for personal privacy, autonomy and security, something many are beginning to consider as impossible [2] – Goodman [32] has likened digital security to a ’digital Maginot line’. The digital revolution has led to a data deluge, where large digital platforms like Amazon, Google, Facebook and Baidu monitor our every move and led Gore to warn of ‘a stalker economy’ [33]. A large challenge for official statistics is both to measure this new economy and also capitalise on these new data sources. In 1987, the economist Robert Solow identified a ‘productivity paradox’ where computers had become ubiquitous everywhere but in productivity statistics [34]. Arguably the same could be said of digitalisation. More broadly, it is not clear that the full impact of digitalisation is properly understood or is being captured in productivity statistics, or for that matter, in labour or real income statistics either.
Another, but related, policy concern is the risk of a ‘jobless future’ or the ‘end of work’ [35] emerging from the disruptive blend of technology, automation and task-based labour that has been dubbed the 4th industrial revolution [36]. The shift towards non-standard work patterns is blurring the distinction between formal and informal employment. The variety of new non-standard or on-demand employment contracts mixed with robotics and other technological advances are posing challenges for those trying to classify and measure this new gig economy. Another link between labour and digitalisation is the nascent but fascinating discussion on the ownership of data held by social media and search platforms that are essentially the product of users’ labour and who benefits from this ‘work’ [2]. Ferguson [37] has likened this to a ‘sharecropping economy’ where the tools of production are available to many, but the rewards are concentrated in the hands of a few. Zuboff [38], in a throwback to Marxism, calls it ‘behavioural surplus.’
D. Political Economy of data. Statisticians should also be aware of the wider context of how data are being used and the important debates underway in which data or statistics are in one way or another at the heart. At the core of official statistics is the ambition to provide impartial information, yet no statistic is truly neutral. Every statistic is the product of many decisions and assumptions, conscious or subconscious [39]. It is important that students understand that statistics are manufactured, not discovered, and that the decisions and choices they make have an impact. The selection of variables in a composite index; whether to weight or not; or the treatment of outliers, all affect not only the basic result but perhaps also the alignment to a political or economic ideology. This realisation becomes especially important when thinking about what ‘evidence informed decision making’ really means or the concerns expressed as ‘Governance by numbers’ [40]. This is a growing worry for many as algorithms (or what Tufekci has called ‘semi-savant mini-Frankensteins’ [41]) are playing an increasingly greater role in our lives, from deciding whether we get a loan to whether we are shortlisted for an interview [42, 43].
Many other interesting debates are underway, not least, the ‘End of Theory’ argument posited by Anderson [44] that with the emergence of big data, correlation has superseded causation. In other words, hypothesis driven science is redundant. Whether one agrees or not, this is an argument worth exploring. Should ‘open data’ exclusively target public sector data, or should it also push for more openness with private sector data. Are there risks to not pursuing a more balanced policy? Is ‘Fake News’ undermining the credibility of science and official statistics and how can NSOs tackle it without themselves becoming politicised? Has official statistics failed in its role – why for example, in the face of overwhelming statistical evidence is the climate crisis not being taken as seriously as it should? There are interesting ideas, such as data infrastructure, to be discussed where state information can be organized into registers supported by unique identifiers [45]. This is a very logical, effective and efficient approach but requires some sacrifice of privacy to the state. What are the cultural or other barriers to this? There are so many fascinating debates that students could and should be introduced to, so that they understand the fascinating range of opportunities available to them. For example, there is much talk today of Data Revolution [46], but what does that mean? Has there really been a revolution or is the data deluge just another step in the natural evolution of data [2]?
3.The case for adaptive expertise
We have seen how advances in the production and processing of data are catalysing changes in statistical education, placing increased emphasis on computation and applications. And in the last section, we identified specific requirements for training of official statisticians, both traditional and with an eye on the future. Nevertheless, as Zheng [47] points out, ‘for the everexpanding skill set and application of Data Science, we can never be able to teach our students enough “coping skills” to handle each situation’. In a world that is always changing, the objectives and function of Official Statistics need constant readjustment [48]. Of course, curriculum renewal is also a continuous process, but it is typically slow and trails developments in the field. So instead of trying to anticipate change, it is better to prepare students for future learning, that is to cultivate their ‘capacity to learn new information, to use resources effectively and innovatively, and to invent new strategies for learning and problem solving in practice’ [49]. This should be an obvious requirement as statisticians, like any other professional, will need to continually update their practice and skills over the lifetime of their career [15]. Nevertheless, this becomes critically important in a fast-changing discipline. In this section we examine how to prepare for lifelong development through the lens of adaptive expertise, a notion that will help us identify key traits and how to foster them.
The concept of adaptive expertise was introduced by Hatano and Inagaki [50] to describe the ability to aptly address new types of problems. It is used in juxtaposition to routine expertise, which is the ability to solve familiar problems in an optimal way. Adaptive expertise encompasses routine expertise, that is, any expert must be able to perform optimally and consistently in common representative domain tasks. This requires domain-specific knowledge as well as procedural competency, accumulated through repeated practice with standard tasks. But the defining characteristic of adaptive experts is that they can exhibit superior performance in new and unfamiliar tasks as well. This requires a deeper conceptual understanding of their area, combined with ingenuity and creativity. Undoubtedly, these are desirable characteristics, so the main question is how to cultivate them within programs of study or training in statistics. We first review the literature for a general framework of developing adaptive expertise, before proposing specific strategies for statistical education.
Figure 3.
Trajectories to adaptive expertise through the innovation-efficiency space.
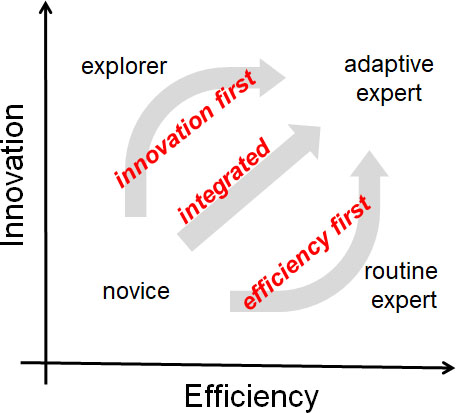
Adaptive expertise calls for superior performance in novel situations, something that requires both domain-specific proficiency and general inventiveness or creativity/resourcefulness (see Fig. 1). Schwartz et al. [51] discuss the trade-offs involved in developing such skills with the help of a two-dimensional space of Efficiency and Innovation, an adapted version of which appears in Fig. 3. The authors consider different trajectories to adaptive expertise: one which prioritizes efficiency, one which prioritizes innovation, and one which combines the two, which we refer to as an “integrated” trajectory. Most formal education has traditionally been geared towards efficiency, or what Hatano and Inagaki [50] call “procedural knowledge”. This certainly rings true for Statistics, which is still frequently taught as a collection of methodological solutions, accompanied by a set of decision rules for when to use them. Thus, a typical trajectory for a university student is to first acquire procedural skills and knowledge in standard settings, and to then develop adaptive expertise through projects, internships, capstones, or, eventually, work experience. On the other hand, Schwartz et al. [51] note that content-free activities in critical thinking and problem-solving (i.e., innovation) can help develop the relevant skills, but these might be ineffective for tackling larger, more complex problems. This trajectory is typical for those approaching Statistics from other disciplines and learning on-the-job, often at the cost of having to reinvent the wheel. Schwartz et al. advocate for a more balanced approach, one that supports simultaneous development along both dimensions. We also believe in fostering progress along both dimensions, even at the expense of delaying proficiency, since adaptability is at least as important as specialized skills in a rapidly changing environment [52].
Setting an integrated approach as the preferred way of developing adaptive expertise, the next question is how to attain it. Since educational programs are conventionally geared towards efficiency, we examine how to promote progress along the innovation dimension. As a starting point, Hatano [53] lists three generic factors that support the development of adaptive vs routine expertise:
1.
1. Exposure to variation: Learners should face “natural randomness” in the types of problems they encounter.
2. Encouragement to experiment: The learning environment should motivate active experimentation, without requiring external reinforcement.
3. Emphasis on comprehension: Learners should develop a deeper conceptual understanding of the methods and tools they are using.
Hatano advocates a process model for adaptive expertise, whereby learners are regularly confronted with new situations which do not perfectly conform to prior knowledge/skills. They are then prompted to engage in experimentation without the need or expectation of extrinsic rewards, which typically favour efficiency. Lastly, students are encouraged to reflect on, compare, and assess their approach, refining their understanding and mental models of the subject at hand. This process model reflects aspects of other known strategies for building expertise, such as problem-based learning [54] and deliberate practice [55].
The factors advocated by Hatano are rather generic, so other researchers have built upon these ideas, especially in the context of professional education in fields such as medicine and engineering. Bohle-Carbonell et al. [56] provide a comprehensive review of characteristics and learning environments for adaptive expertise, while Mylopoulos et al. [57] offer twelve practical recommendations for designing curricula that support adaptive expertise. Alongside Hatano’s original principles and generic methods for adaptive expertise in the literature, we identify three relevant traits and attitudes that are particular to Statistics:
1.
1. Inquisitiveness: Statistics is a domain-free scientific discipline, or as Tukey famously put it “[statisticians] get to play in everyone’s backyard”. Therefore, statisticians should be characterized by intellectual curiosity and a spirit of inquiry (see Fig. 1). This is especially true of official statisticians, who are called upon to work on an ever-expanding range of social, economic, and scientific issues. In order to engage productively in such diverse applications, statisticians must have broad interests and an appetite for knowledge. This will help them identify important questions and motivate them to pursue answers beyond conventional beliefs and practices.
2. Statistical Thinking: What differentiates statisticians from other scientists working with data is the conceptual framework through which they approach problems, rather than the methods or tools they apply. The term statistical thinking is used to describe the thought processes that distinguish our discipline. There have been several definitions of the term [58, 59], and while details vary they all share a core set of factors which include: a) taking into account variation and uncertainty throughout the analysis, b) recognizing the importance of what is measured and how data are produced, and c) being able to appropriately reason using statistical models about the phenomenon at hand.
3. Extroversion: Since Statistics does not have a dedicated domain of study, it is by nature and necessity an outward-facing discipline. It is crucial for statisticians, and especially official ones, to interact constructively with other scientists and professionals. It is through these interactions that our discipline draws energy and stimulation, and maintains its relevance in a changing world. This should be reflected in the way Statistics is taught, by preparing and encouraging students to engage in such interactions see (Section 2).
Taken together, these three features constitute what we call an adaptive statistical mindset, i.e. a way of thinking that is essential to the independent and sustained development of learners and practitioners of statistics. The term describes the desired mindset with which a statistician should approach new situations. Specifically, they should first develop a deep understanding of the problem at hand, appreciating its context, requirements, and peculiarities. Then, they should be willing to try out new approaches beyond off-the-shelf solutions, while maintaining clear objectives and applying methods for comparing, assessing, and adapting these approaches. The latter is facilitated by the effective exchange of information and feedback among the various collaborators and stakeholders. Lastly, a statistician with an adaptive mindset should be able to constructively reflect on their experiences, which will result in continually improving their analytical and critical thinking, as well as their creativity and confidence.
The traits and attitudes of an adaptive statistical mindset parallel the model investigation process as outlined in Wild and Pfannkuch [59], from problem definition, to planning, collecting, and analyzing data, until drawing conclusions. The core of the process relies on statistical thinking, the need for which is well documented and dates to at least Cobb [6]. The other two characteristics of inquisitiveness and extroversion are complementary to the statistical investigation process, in that they incite and promote its development. More specifically, they help identify interesting problems, interpret and communicate the results, as well as iterate and refine the investigation process. All three features include higher-order thinking skills which, although difficult to teach, should nevertheless be integrated and cultivated throughout the curriculum. In the next section, we propose relevant teaching strategies in the context of statistics courses that promote such an adaptive statistical mindset.
Table 1
Strategies for cultivating the three components of an adaptive statistical mindset
Strategy | Component | ||
---|---|---|---|
Inquisitiveness | Statistical | Extroversion | |
thinking | |||
Open-ended investigations | |||
Complex, real-world data | |||
Collaborative work | |||
Facilitated problem-solving | |||
Authentic assessment |
4.Teaching strategies for adaptive statistical practice
Adaptive expertise in any field is developed through sustained engagement in activities that promote efficiency and innovation, and for official statisticians, like most professionals, such experiences will span their entire career. In this section, we focus on post-secondary education and in particular university courses offered as part of study or training programs in Statistics. We present five strategies that are generic enough to be employed at any stage of the curriculum, from introductory to advanced methodological courses, at either the undergraduate or graduate level. The goal of these strategies is to encourage progress along the innovation dimension, which is to be pursued in tandem with the goal of developing procedural skills and knowledge. Table 1 lists the five teaching strategies and highlights their correspondence to the three components of an adaptive statistical mindset. While these strategies are not new and some have appeared in similar contexts [9, 58], we have not seen them compiled or examined through the lens of adaptivity before. Below we provide more details on each strategy, and its relation to adaptive statistical practice.
A.
A. Open-ended statistical investigations: Students should be exposed to problems that are intentionally vague and under-specified, and which require them to think about and formulate meaningful statistical questions. Problems should lend themselves to different perspectives, inciting students to invent and evaluate alternative approaches. The context of the investigation is also important for coming up with good questions, and topics that are close to students’ experiences and interests, and those with broader social significance typically work better at capturing student interest. Such investigations can form the basis for other strategies, and their benefits span all three components.
B. Complex, real-world data: Data should be pervasive in the teaching of Statistics, but the form in which they come in is just as important. We should not condition our students to work with curated and groomed data, but we should expose them to a variety of real-world data in their raw and oftentimes messy form, collected for different purposes and in different contexts. These data should also be complex, consisting of multiple variables arranged over different data types and structures. And students should also be able to combine data from several sources in order to compile the relevant information, all the while considering issues of data quality.
C. Collaborative work: Collaboration should be integrated into the practical components of courses, i.e., from in-class problem-solving, to assignments and projects. Earlier in the curriculum, this would typically be in the form of working with peers, but in advanced courses there should be opportunities for more formal, external statistical consultations or collaborations. The latter can range from assisting students from other disciplines with their theses, consulting for external clients, or working within a group on a research project. By distributing such opportunities at different levels of the curriculum, students will get to gradually build up soft skills required for effective collaboration.
D. Facilitated problem-solving: Many students find working on open-ended problems, and in particular the lack of definitive answers, to be disconcerting. Facilitation can help structure and guide the process, as well as model desired behavior. Exploring problems with support from the teaching team in a low-stakes environment allows students to try out ideas without fear of failure, while receiving constructive feedback. In turn, this helps build students’ conceptual understanding and confidence, as well as honing their communication skills.
E. Authentic assessment: Assessments should be aligned with the desired learning outcomes and overall program objectives, reflecting what is valued and expected from students. But for reasons of either convenience or tradition, assessments are frequently performed in ways that are artificial, favouring efficiency and correctness at the expense of ingenuity and comprehension. If we want to reinforce an adaptive statistical practice, we should assess students in a more wholistic way, one which is as close as possible to how they would analyse data in real life. For example, we could ask students to conduct an entire statistical investigation on new data, including open-ended questions that require contextual understanding, probing, and creativity.
Specific examples of these strategies in the context introductory courses at two campuses of the University of Toronto are provided in Gibbs and Damouras [60] and an overview of a complete program designed with such considerations in mind is given in Gibbs [61]. Sustained progress along the path of adaptive statistical practice will require integration of novel situations and learning that emphasizes understanding, exploration and discovery.
5.Conclusion
We have argued that statisticians require a broad range of skills. One can anticipate that technological skills will play a greater role in the toolbox of a future statistician, while all the core statistical skills will remain essential. In a rapidly changing environment, the requirement for particular technological skills may change or evolve, whereas the core competencies will not. We have also argued that a statistician, whether today or in fifty years’ time, will need to be curious and inquisitive. They must naturally critically analyse trends and be sceptical of results. The world is a complex, messy and resource constrained place, and so they must be creative, inventive and resourceful if they are to get the job done.
The role of universities should not only be to impart knowledge and skills, but also ignite curiosity and stimulate discussion. Too often statistics is presented or taught as a black and white set of rules or solutions, but in reality the application of statistics to the complex world we inhabit is at best grey. Official statistics, in particular, has not been historically well covered in academia – perhaps as many academics themselves do not fully appreciate or understand their role. We have argued that, in addition to teaching the traditional elements of statistics, more practical, applied and contextual statistics could be taught.
The bigger challenge for universities is how to cultivate and nurture competencies. This might be tackled by introducing students to what we have termed policy issues and the political economy of data. These issues are fascinating and could be used to encourage debate, stimulating students to think about the complex role that data and statistics play in today’s data driven world. The role of the Professional Diploma in Official Statistics and Policy Evaluation [62] in Ireland or the European Master in Official Statistics [3] are interesting case studies as they were designed with input from official statisticians and take a broader perspective than traditional statistics courses.
Another way to meet current and future demands for our profession is by fostering an adaptive statistical mindset through university education and training programs. The concept comprises three traits and attitudes, namely inquisitiveness, statistical thinking, and extroversion, which promote adaptive practice and lifelong professional development in the field. We describe both general principles for promoting adaptivity, as well as five specific teaching strategies tailored to statistics. All our suggestions combined can widen the scope of statistics courses, making them more interesting, linking them to the great debates of the day, better preparing students to tackle new problems as they arise, and showcasing what a fascinating and rewarding career may be awaiting graduates.
Notes
2 GDPR or the General Data Protection Regulation is the primary data protection legislation for the European Union. It came into force in May 2018.
3 For example, one could add climate change or environmental degradation.
References
[1] | MacFeely S. The continuing evolution of official statistics: Some challenges and opportunities. Journal of Official Statistics. (2016) ; 32: : 789-810. |
[2] | MacFeely S. In search of the data revolution: Has the official statistics paradigm shifted? Statistical Journal of the International Association of Official Statistics. (2020) ; 32: : 1075-1094. |
[3] | EMOS Board. European Master in Official Statistics (EMOS) Learning Outcomes, https://ec.europa.eu/eurostat/cros/system/files/emos_learning_outcomes_2019_adopted.pdf (2019, accessed May 2, 2021). |
[4] | van Dyk D, Fuentes M, Jordan MI, et al. ASA statement on the role of statistics in data science. Amstat News. (2015) ,https://magazine.amstat.org/blog/2015/10/01/asa-statement-on-the-role-of-statistics-in-data-science/ (2015, accessed May 2, 2021). |
[5] | Efron B, Hastie T. Computer age statistical inference: Algorithms, evidence, and data science. Cambridge University Press, (2016) . |
[6] | Cobb G. Teaching statistics. In: Steen LA (ed) Heeding the call for change: Suggestions for curricular action. The Mathematical Association of America, (1992) ; 3-43. |
[7] | Horton NJ, Hardin JS. Teaching the next generation of statistics students to “Think With Data”: Special issue on statistics and the undergraduate curriculum. Am Stat. (2015) ; 69: : 259-265. |
[8] | Nolan D, Temple Lang D. Computing in the Statistics Curricula. Am Stat. (2010) ; 64: : 97-107. |
[9] | American Statistical Association Undergraduate Guidelines Workgroup. 2014 Curriculum Guidelines for Undergraduate Programs in Statistical Science, https://www.amstat.org/asa/education/Curriculum-Guidelines-for-Undergraduate-Programs-in-Statistical-Science.aspx (2014, accessed May 2, 2021). |
[10] | Garfield J. Statistical literacy, reasoning, and thinking. International Encyclopedia of Statistical Science. (2014) ; 1439-1441. |
[11] | delMas RC. Statistical literacy, reasoning, and thinking: A commentary. Journal of Statistics Education. (2002) ; 10. |
[12] | Gal I. Adults’ statistical literacy: Meanings, components, responsibilities. International Statistical Review. (2002) ; 70: : 1-25. |
[13] | Gore A. The assault on reason. Penguin, (2007) . |
[14] | Radermacher WJ. Governing-by-the-numbers/Statistical governance: Reflections on the future of official statistics in a digital and globalised society. Statistical Journal of the IAOS. (2019) ; 35: : 519-537. |
[15] | MacFeely S. Teaching Data Science – A User Perspective (or Preparation for a Career as an Official Statistician). in: Proceedings of the 62nd ISI World Statistics Congress. KUALA LUMPUR, MALAYSIA, https://2019.isiproceedings.org/Files/5.Special-Topic-Session(STS)-Volume-3.pdf (2019, accessed May 2, 2021). |
[16] | United Nations (Resolution 68/261 adopted by the General Assembly on 29 January 2014). United Nations Fundamental Principles of Official Statistics, https://unstats.un.org/unsd/dnss/gp/FP-New-E.pdf (2014, accessed April 19, 2021). |
[17] | United Nations Committee for the Coordination of Statistical Activities. Principles Governing International Statistical Activities, https://unstats.un.org/unsd/ccsa/principles_stat_activities/, (2016) . |
[18] | International Statistical Institute. Declaration of Professional Ethics, https://www.isi-web.org/images/about/Declaration-EN2010.pdf, (2010) . |
[19] | United Nations. Statistical Quality Assurance Framework, https://unstats.un.org/unsd/unsystem/documents/UNSQAF-2018.pdf, (2018) . |
[20] | United Nations Statistics Division. Generic National Quality Assurance Framework (NQAF), https://unstats.un.org/unsd/dnss/QualityNQAF/nqaf.aspx, (2012) . |
[21] | MacFeely S. The big (data) bang: Opportunities and challenges for compiling sdg indicators. Global Policy. (2019) ; 10: : 121-133. |
[22] | United Nations Conference on Trade and Development. Trade and development report: Beyond austerity – towards a global new deal, https://unctad.org/en/PublicationsLibrary/tdr2017_en.pdf, (2017) . |
[23] | Tooze A. Crashed: How a decade of financial crises changed the world. Penguin, (2018) . |
[24] | Karabell Z. The leading indicators: A short history of the numbers that rule our world. Simon and Schuster, (2014) . |
[25] | Nordhaus WD, Tobin J. Is growth obsolete? in: Economic Research: Retrospect and Prospect, Nordhaus WD, Tobin J, eds, Volume 5, Economic Growth. NBER, https://www.nber.org/system/files/chapters/c7620/c7620.pdf (1972, accessed July 7, 2021). |
[26] | Talberth J, Cobb C, Slattery N. The Genuine Progress Indicator 2006: A Tool for Sustainable Development. Redefining Progress, https://d3pcsg2wjq9izr.cloudfront.net/files/24200/articles/12128/GPI202006.pdf (2007, accessed July 7, 2021). |
[27] | United Nations Development Programme (UNDP). Human Development Report 1990. Concept and Measurement of Human Development. New York, http://hdr.undp.org/en/reports/global/hdr1990 (1990, accessed July 7, 2021). |
[28] | Alkire S. Bhutan’s Gross National Happiness Index 2008. OPHI Research In Progress Series, https://www.ophi.org.uk/wp-content/uploads/Alkire_Bhutan_GNH_index_2008_OPHIRP5a.pdf (2008, accessed July 7, 2021). |
[29] | United Nations University International Human Dimensions Programme on Global Environmental Change, United Nations Environment Programme. Inclusive wealth report 2012? Measuring progress toward sustainability. Cambridge: Cambridge University Press, (2012) . |
[30] | Eurasian Economic Commission, United Nations Conference on Trade and Development. Inclusive Growth of the Eurasian Economic Union Member States: Assessments and opportunities, http://www.eurasiancommission.org/ru/act/integr_i_makroec/dep_makroec_pol/Documents/Inclusive_growth_in_EAEU_Member.pdf (2019, accessed July 7, 2021). |
[31] | Philipsen D. The little big number. Princeton University Press, (2015) . |
[32] | Goodman M. Future Crimes: Inside the Digital Underground and the Battle for Our Connected World. (2015) . |
[33] | Schneier B. “Stalker economy” here to stay, https://www.cnn.com/2013/11/20/opinion/schneier-stalker-economy (accessed April 21, 2021). |
[34] | Solow RM. “We’d Better Watch Out” Review of manufacturing matters: The myth of the post-industrial economy. The New York Times, (1987) , https://economicsociologydotorg.files.wordpress.com/2018/07/wed-better-watch-out.pdf (1987, accessed April 21, 2021). |
[35] | Eurofound and the International Labour Office. Working anytime, anywhere: The effects on the world of work, http://eurofound.link/ef1658 (2017, accessed April 21, 2021). |
[36] | Schwab K. The fourth industrial revolution. Penguin Books. (2017) . |
[37] | Ferguson N. The square and the tower: Networks and power, from the freemasons to Facebook. Penguin Books. (2019) . |
[38] | Zuboff S. The age of surveillance capitalism the fight for a human future at the new frontier of power. Public Affairs. (2019) . |
[39] | MacFeely S, Nastav B. You say you want a [data] revolution: A proposal to use unofficial statistics for the SDG Global Indicator Framework. Statistical Journal of the IAOS. (2019) ; 35: : 309-327. |
[40] | Fukuda-Parr S, McNeill D. Knowledge and politics in setting and measuring the SDGs: Introduction to special issue. Global Policy. (2019) ; 10: : 5-15. |
[41] | Tenner E. The efficiency paradox: What big data can’t do. Alfred A Knopf. (2018) . |
[42] | O’Neil C. Weapons of math destruction: How big data increases inequality and threatens democracy. Allen Lane, London UK. (2016) . |
[43] | Sumpter D. Outnumbered: From Facebook and Google to Fake News and Filter-bubbles – The Algorithms That Control Our Lives. Bloomsbury Publishing. (2018) . |
[44] | Anderson C. The end of theory: The data deluge makes the scientific method obsolete. Wired magazine. (2008) . |
[45] | MacFeely S, Dunne J. Joining up public service information: The rationale for a national data infrastructure. Administration; 61. |
[46] | United Nations Secretary-General’s Independent Expert Advisory Group on a Data Revolution for Sustainable Development. A world that counts: Mobilizing the data revolution for sustainable development, http://www.undatarevolution.org/wp-content/uploads/2014/11/A-World-That-Counts.pdf (2014, accessed May 2, 2021). |
[47] | Zheng T. Teaching data science in a statistical curriculum: Can we teach more by teaching less? Journal of Computational and Graphical Statistics. (2017) ; 26: : 772-774. |
[48] | MacFeely S. Big data and official statistics. in: Big Data Governance and Perspectives in Knowledge Management. IGI Global. Strydom Kruger S, Strydom M, eds, (2019) ; 25-54. |
[49] | Mylopoulos M, Brydges R, Woods NN, et al. Preparation for future learning: A missing competency in health professions education? Medical Education. (2016) ; 50: : 115-123. |
[50] | Giyoo Hatano Kayoko Inagaki. Two courses of expertise. in: Child development and education in Japan. W.H. Freeman, NY. Stevenson HW, Azuma H, Hakuta K, eds, (1986) ; 262-272. |
[51] | Schwartz DL, Bransford JD, Sears D, et al. Efficiency and innovation in transfer. in: Transfer of Learning from a Modern Multidisciplinary Perspective. Mestre J, ed., Greenwich, CT: Information Age Publishing, 2005. |
[52] | Levin HM. The importance of adaptability for the 21st century. Society. (2015) ; 52: : 136-141. |
[53] | Hatano G. Social and motivational bases for mathematical understanding. New Directions for Child and Adolescent Development. (1988) ; 55-70. |
[54] | Barrows HS. Problem-based learning in medicine and beyond: A brief overview. New Directions for Teaching and Learning. (1996) ; 1996: : 3-12. |
[55] | Ericsson KA, Krampe RT, Tesch-Römer C. The role of deliberate practice in the acquisition of expert performance. Psychological Review. (1993) ; 100: : 363-406. |
[56] | Bohle Carbonell K, Stalmeijer RE, Könings KD, et al. How experts deal with novel situations: A review of adaptive expertise. Educational Research Review. (2014) ; 12: : 14-29. |
[57] | Mylopoulos M, Steenhof N, Kaushal A, et al. Twelve tips for designing curricula that support the development of adaptive expertise. Med Teach. (2018) ; 40: : 850-854. |
[58] | Chance BL. Components of statistical thinking and implications for instruction and assessment. Journal of Statistics Education. (2002) ; 10. |
[59] | Wild CJ, Pfannkuch M. Statistical thinking in empirical enquiry. International Statistical Review. (1999) ; 67: : 223-248. |
[60] | Gibbs AL, Damouras S. Evolving statistics education for a data science world. In: Proceedings of the 62nd ISI World Statistics Congress. KUALA LUMPUR, MALAYSIA, https://2019.isiproceedings.org/Files/5.Special-Topic-Session(STS)-Volume-3.pdf (2019, accessed May 2, 2021). |
[61] | Gibbs AL. Building a foundation in statistics in the era of data science. In: Proceedings of the Tenth International Conference on Teaching Statistics (ICOTS10, July 2018), Kyoto, Japan. Voorburg, The Netherlands: International Statistical Institute. |
[62] | Institute for Public Administration. Professional diploma in official statistics for policy evaluation, https://www.ipa.ie/public-management/professional-diploma-in-official-statistics-for-policy-evaluation.1763.html (2019, accessed May 2, 2021). |