The International Program in Survey and Data Science (IPSDS): A modern study program for working professionals
Abstract
The International Program in Survey and Data Science (IPSDS) is an online educational program, which can be attended through the Joint Program in Survey Methodology (JPSM) at the University of Maryland (UMD) and a part-time Master of Applied Data Science & Measurement (MDM) at the University of Mannheim and Mannheim Business School (MBS). It is targeted towards and attended by working professionals involved or interested in data collection and data analysis including those working in official statistics. The program conveys competencies in the areas of data collection, data analysis, data storage, and data visualization. The faculty of the program includes researchers and lecturers from both the University of Maryland and the University of Mannheim as well as other organizations such as destatis and Statistics Netherlands in the field of official statistics. The program was awarded the label of ‘European Master in Official Statistics (EMOS)’ under conditions in May 2021.
In the article, we summarize the methodological and statistical competencies needed in official statistics and show how IPSDS covers this set of skills. We will present the flipped classroom design used for the IPSDS program and demonstrate that it is especially suited for students who are working professionals at the same time.
1.Introduction
Data on the characteristics and behaviors of individuals often serve as a basis for decision-making in the public sector. These data can be divided into data obtained with a particular survey design and those that arise organically as a byproduct of administrative and digital processes. While the use of data from administrative procedures has been well-established in Europe, the use of new digital trace data from, for example, websites collected through web scraping or through cooperations with mobile network operators, is still in an early phase in official statistics [1, 2]. These digital trace data can be roughly defined as “records of activity (trace data) undertaken through an online information system (thus, digital)” [3]. Recent years have shown that these new digital data sources will not replace traditional surveys completely, as these data often cannot cover all aspects: Important socio-demographic variables in the social sciences like household size, education, income, or employment status are in general not part of digital trace data [4]. Still, the increased interest in the use of new and big data sources in official statistics [5] and the linking and matching of data [6, 7] has led to an increased demand for well-trained data analysts. These data analysts need both the skills to collect and analyze data from surveys and work with large semi-structured or even unstructured data sets. These skills are often not taught or are taught only on a basic level in social science or economic programs. At the same time, there is a rapidly growing number of data science programs (see [8] for a recent overview of Data Science programs in German-speaking countries). Nevertheless, the training in Data Science programs is often very tech-oriented, and the link to methodological issues in official statistics is usually neglected. This is where the International Program in Survey and Data Science (IPSDS) comes into play.
IPSDS meets the demand for experts in the diverse fields of new and old forms of data collection, data analysis, data storage, and data output through a part-time master’s degree program. The starting point for the program was the two-year Joint Program in Survey Methodology (JPSM), which has been offered at the University of Maryland since 1993 together with the University of Michigan and the Westat cooperation. IPSDS was anchored in Germany at the University of Mannheim in 2016, facilitated through strong ties between the faculty in Maryland and Mannheim and based on the experiences from the JPSM program. IPSDS provides a flexible online learning environment and courses are held by instructors from both universities (as well as other universities and organizations). After several years of constant development and testing, the curriculum developed by IPSDS is now offered as the Mannheim Master in Applied Data Science and Measurement (MDM) by the Mannheim Business School. Students can take IPSDS courses through two full Master’s programs, JPSM at the University of Maryland and MDM at the University of Mannheim and the Mannheim Business School.
Unlike many continuing education programs, the program draws heavily on flipped classroom format where students learn asynchronously in advance of the class with prerecorded videos and other materials. The synchronous class time is reserved for problem-based, collaborative learning [9, 10]. This design allows the students to arrange their time resources more freely. IPSDS implemented this flipped classroom design into an online program accessible from anywhere in the world and students can adapt their studies to their professional and personal responsibilities and combine profession, studies and family.
The target group for the Master’s program are working professionals from all over the world who are professionally involved or interested in data collection and data analysis. They are employed in various fields, such as applied demographic, sociological, and economic research. Former and current students include professionals working in official statistics in different counties, data analysts at research institutes, and lecturers in various substantive fields at universities. They use data or are in charge of data collection from surveys or new data sources. Most students expect IPSDS to provide them with the necessary contents and skills to further develop in their careers in survey and data science [11].
IPSDS courses also cover the set of learning outcomes defined by the European Master in Official Statistics (EMOS) network. EMOS is a label of European Master’s programs providing post-graduate education in official statistics, which is awarded by the European Statistical System Committee (ESSC). The MDM program at the University of Mannheim was awarded the EMOS label under conditions and recommendations in May 2021. Several IPSDS faculty members are also working for official statistics institutions like Statistics Netherlands, Destatis, or the Institute for Employment Research (IAB), the Research Institute of the Federal Employment Agency in Germany.
We will start this article with an overview of the skills needed from data science, statistics, and survey methodology in official statistics and how these skills are covered in the IPSDS curriculum. We will also present the “inverted classroom” learning format used in the IPSDS program and demonstrate that it is especially suited for working professionals. We will also present a sample course from the curriculum and possibilities to receive so-called micro-credentials, that is, certificates for single courses or course bundles through IPSDS.
2.Methodological and statistical competencies needed in official statistics today
As Radermacher [2, p. 22] noted: “The decisive factor for the quality of statistics is the staff of the statistical institution.” The fast-changing world of data constitutes high requirements to the skill set of people working with it. The demand for data literacy is accelerated by the large amount of data that is amassed in the digital age and the expectations of organizations to make use of it [8]. Based on the American Association for Public Opinion Research (AAPOR) task force report on big data in survey research [12, 13], IPSDS identified five different areas of competence for working successfully with data from different sources under current developments in the world of data (Keusch and Kreuter 2020). These five areas will be presented in the following.
2.1Research question
To successfully conduct a data project, it is important to formulate relevant and adequate research questions and assess which type of data can be used to find answers to the corresponding questions. Expert knowledge from the respective disciplines and work contexts is essential here. Professionals working in survey and data science usually acquired this substantive expertise in their respective fields during their previous undergraduate and graduate studies and through their professional careers. However, they also need knowledge about different types of research questions (descriptive, explorative, inferential, predictive, causal) and how to formulate them (see [14] for a classification of research questions).
2.2Data generating process
The next question is which data can be used to answer the research question. Researchers can potentially choose from an increasing variety of data (survey data, administrative data, digital trace data). However, this rising variety in possible data sources leads to increasing demands in knowing the advantages and disadvantages of these sources. In addition, different data generating processes require different skills for controlling and applying these processes.
When conducting a survey, researchers need skills at least in the area of questionnaire design as well as sampling and weighting. They need a solid understanding of the total survey error (TSE) framework for improving surveys by optimizing data quality. Even though the methodological basics are taught in many undergraduate social science programs, it is crucial to stay informed on new developments. Surveys and the (technical) environment in which they are conducted have changed in recent years. Researchers conducting surveys now also need to know about web survey design, surveys conducted on smartphones and user experience research. Plus, there has been a shift away from complex and expensive probability-based sampling to less costly non-probability-based sampling. Results regarding the analytical potential of the latter non-probability samples have, however, been mixed [15, 16].
Administrative data (e.g., data on developments in the economy and labor market, admission and graduation data of educational institutions, data originating from the healthcare system) also plays a critical part in official statistics. Many public organizations now provide administrative data also for research purposes. As a rule, administrative data are very large data sets and differ from survey data in terms of structure and complexity as they are “not originally collected for the purpose of research” [17, p. 4]. It is essential to understand the data-generating process to comprehend the data and its capabilities and, in particular, to identify potential sources of error [18].
Another important type of organic data is digital trace data. These require yet another different set of skills like extracting data from existing platforms through APIs [19] or web-scraping [20]. They also require the ability to evaluate and implement legal regulations and possible error sources like, for example, algorithmic confounding, that is, the tendency of digital systems to induce specific behaviors like ad clicking, posting content, or network building [21].
As the different data types come with different benefits and drawbacks, another essential skill is matching and combining data from different sources to leverage their individual strengths [6, 7]. Eurostat has also recognized the importance of integrating new forms of data by starting ESSnet Big Data II, a project and continuation of ESSnet Big Data I, which aims at evaluating the potential of selected big data sources and through building and implementing applications.11
2.3Data curation and storage
Once the relevant data for answering a research question have been identified and collected, they must be processed, organized, and stored before analysis. In larger organizations such as statistical offices, these tasks are often solved with the help of I.T. specialists [22]. For analysts, basic SQL knowledge is vital to load data from a database into the analysis software. The further needed data wrangling and munching skills will differ by type of data. Survey data might require consistency checks [23] or the imputation of missing data [24], non-tabular data might require restructuring, and text data often need several pre-processing steps before analysis [25].
Figure 1.
MDM curriculum.

2.4Data analysis
After data wrangling and munching, the next step is to analyze the data. Analyzing data requires the skills to evaluate the data, build and examine models, and interpret the results. To accomplish these tasks, profound knowledge of various statistical methods, hypothesis testing, inference statistics, and the statistical control of confounding variables is needed. This skill portfolio extends continuously with recent and current developments. In official statistics, machine learning [26, 27, 28] and the increasing use of small area estimation [29] and geospatial data [5] have been significant new developments.
2.5Data output and access
After analysis, the final steps are to create data visualization and data documentation and to guarantee reproducibility. Data visualization should make data and results understandable and interpretable for their respective target groups, regardless of whether people from this target group possess deep statistical knowledge [30]. The documentation of a data project should allow reproducibility, transparency, and collaboration [31]. The FAIR (Findablity Accessibility, Interoperability, Reusability) principles have become guidelines for scientific data management, which demands specific knowledge and skills [32]. For Official Statistics, the UN Fundamental Principles of Official Statistics and the UN Statistical Quality Assurance Frameworks also serve as an important framework for producing high-quality statistics [33, 34]. When data are shared, (legal) questions of data privacy quickly arise [35].
All presented skill areas are usually only partially covered in undergraduate study programs. In the IPSDS program, however, they build the core of the curriculum as the five so-called focus areas (see Fig. 1). IPSDS follows a practice-oriented approach that aims to equip the students with the necessary skills for handling whole projects as part of a team or on their own. The focus areas mark the core technical skills for successfully working with data. Nevertheless, IPSDS also stresses the improvement of soft skills. This is achieved by implementing teamwork in a range of courses and culminates in the project consulting course, where students tackle a data project from a real-world client in teams.
3.Curriculum
The IPSDS program has been offered as the accredited Mannheim Master of Applied Data Science & Measurement (MDM) at the Mannheim Business School since 2020. MDM is a 30 months, 90 ECTS22 credits program taught in English and leads to a Master of Science Degree (see Fig. 1).
The 90 ECTS credits include 60 ECTS credits to be acquired through courses, from which 54 ECTS credits are mandatory courses that cover the essential competencies from the five focus areas. The students can select courses worth additional 6 ECTS credits as electives according to their personal preferences and professional needs. Every course belongs to one or several of the focus areas presented in the previous section. Following the constructive alignment principle [36], the syllabus of each course states the targeted learning outcome, teaching and learning methods used, and type of assignments and grading. The students also have to complete a project report worth 10 ECTS credits, using the skills and knowledge they gained from the program within their field of work. The program concludes with final written and oral exams and the Master’s project of 20 ECTS credits. The elements of the program will be briefly presented in the following.
3.1Core courses
The core curriculum is designed to cover the five focus areas while moving from introductory courses to courses that convey more advanced skills and knowledge (see the Appendix for a list of offered courses). It is central to take the participants’ different knowledge and skill levels into account. As the students have varied educational and professional backgrounds and are specialized in different topics, they first have the opportunity to refresh their knowledge of basic statistical concepts, to improve their R programming skills and their data management skills in introductory courses. Another introductory course offers a broad overview of the essential topics in survey and data science and different types of research questions.
Several courses cover the four main focus areas. Data generating processes are addressed in courses on questionnaire design, record linkage focusing on big data applications, and sampling. A course on modern workflows in data science is the core of the focus area on data curation and storage. Students deepen their data analysis skills in a course on generalized linear models. They can also choose from different elective courses, focusing on other data curation and storage and data analysis methods (see next section). Courses on data visualization and on legal and ethical aspects of data dissemination cover the area of data output and access.
The curriculum is designed to be practice-oriented. Courses not only convey theoretical knowledge and single competencies, but they enable students to handle whole data projects properly on their own. The skillset of the core courses come together in the course on project consulting, where students work in teams on a data project with real-world clients. The course offers the opportunity to experience all steps of a data consulting project including data analysis of real-world data, interacting with clients, and communicating the analysis results in written and oral form. Different partnering institutions like, for example, the German Federal Bank, have contributed projects. Thus, students often have the possibility to choose a project from official statistics.
3.2Elective courses
Elective courses complement the mandatory core curriculum. The program requires students to acquire 6 ECTS from elective courses. The possible electives cover all focus areas (see the Appendix for a full list of offered courses).
The introductory course on official statistics, currently taught by the former general director of Eurostat Walter Radermacher, is specifically designed to introduce students to the system of official statistics in the modern age. It is primarily targeted at students not yet working in official statistics. For students already familiar with the system of official statistics, the course on more advanced topics in official statistics addresses newer and more specialized areas such as big data, geospatial data, and microsimulations in official statistics. Another elective course of particular interest to students employed in official statistics is data confidentiality and statistical disclosure control.
Apart from courses of particular interest to those employed in official statistics, students can use their elective credits to receive training in other programming languages such as Python or SQL. They can also broaden their skills in new data generation (web scraping/APIs) and analysis methods (machine learning).
3.3Project report
IPSDS encourages students to transfer their newly acquired knowledge from the courses to their workplace and apply those acquired competencies under real-world circumstances through internships or work projects. Since IPSDS students complete the study program while being employed, they can use their new skills at their own workplace. These work or external projects are rewarded with credits. Students reflect in their project reports on how they have been able to apply the skills and methods learned in their place of work. For students who cannot use their own place of work for creating the project report (due to data protection or other reasons) or who want to use the opportunity to work in a different environment, the program provides the students with a project, e.g. through traineeships. This is secured due to established cooperation with practice partners, mainly from official statistics, like the German Federal Bank and the Institute for Employment Research. They will introduce students to current research projects on which students can work as well as provide support and counseling during the project. This close collaboration between students and program partners is also generating valuable results for the cooperating institutions.
3.4Final exams and master’s project
The final exams of the program consist of a written exam on research design and oral exams covering the other focus areas. During the oral exams, the students work on a case study. Examiners ask questions structured along the four focus areas data generating process, data curation and storage, data analysis, and data output and access. The students have to describe how they would plan and execute each step of the research project and respond to challenges in this scenario. By spanning the complete workflow of a research project, the students have to showcase their whole skill set in working with data.
After completing the final exams, the students can begin their Master’s project. It consists of a written master’s thesis and an oral defense. The Master’s project is done in teams with up to five students. In addition to two supervisors coaching the student teams, the program established monthly milestone meetings with each team and two additional instructors. During the monthly meetings, all students report their contributions and progress to the instructors and receive feedback, which helps them complete their Master’s project successfully. The topics of the projects can be related to the field of official statistics, for example, a master’s thesis completed in 2021 evaluating the utility of linked administrative data for nonresponse bias adjustment in longitudinal survey data.
4.Learning format
Traditionally, education in survey methodology and social research has been the domain of established universities, and these have also responded to the increasing demand for expertise in data science and big data [8]. However, many non-university providers have started to offer online education and training in this area as well. Online learning platforms such as Coursera (https://www.coursera.org/), edX (https://www.edx.org/), Khan Academy (https://www.khanacademy.org/) or Udacity (https://eu.udacity.com/) offer a wide range of courses from different disciplines, including courses on working with data from new but also traditional sources. DataCamp (https://www.datacamp.com/) allows students to interactively learn how to program with R, Python, and SQL. Courses offered by these organizations are so-called massive open online courses (MOOCs) and direct interaction with the lecturers is usually not part of the course. Engagement with the learning content is typically limited to watching prerecorded videos and solving standardized, small tasks. This self-directed learning requires high motivation on the part of students. Thus, many MOOCs struggle with extremely high dropout rates [37, 38]. Some MOOC providers have tried to move towards paid certificates and offered more direct interaction with lecturers in recent years. In contrast, universities have moved towards recognizing the importance of life-long learning and offering certificates for singular courses without requiring the participants to complete a full bachelor’s or master’s degree [8].
The IPSDS program is already situated between traditional study programs and MOOC courses. It heavily relies on the flipped classroom design (also known as inverted classroom). The flipped classroom has two main characteristics: All course materials including prerecorded video lectures and readings are put online. This allows students to learn asynchronously at their own pace and to coordinate one’s employer (or family) demands and studies. In addition to those asynchronous lectures, students use discussion forums and have live meetings in regular intervals with their lecturers. This allows students to work on specific content together with lecturers and other students [10]. Each week, students participate in a 50-minute online session hosted by the instructor (see Fig. 2). IPSDS courses usually include only eight to fifteen students and the online sessions enable the discussion of students’ questions as well as difficulties in assignments and projects. They also motivate students to continue to participate in the course actively. Screen-capture software, desktop sharing, and virtual meeting rooms form a technical foundation with which instructors can effectively implement these forms of teaching. Instructors can track the progress and understanding of all students at each stage of learning.
IPSDS thus uses a hybrid format between traditional university programs and completely self-paced learning. Due to the shortened synchronous in-class time, it is also especially suitable for online classes. At the same time, the (short) meetings still allow guidance and counsel for the students, contrary to MOOCs where students study primarily on their own.
IPSDS also allows students to access a wide network of peers in the private and public sectors; a significant part of the student body works in official statistics, for example. The kick-off event Connect@IPSDS, which takes place once a year in Mannheim, plays a crucial role in that regard. This event with courses and lectures offers the opportunity to get to know each other in person before the first classes take place online or catch up with other students later.
5.An example from the IPSDS curriculum: The Data Confidentiality and Statistical Disclosure Control course
To provide a better insight into the study program, we will present one of the courses and the skills taught in more detail. The course Data Confidentiality and Statistical Disclosure Control was developed and is currently taught by Jörg Drechsler from the Institute for Employment Research (IAB), the Research Institute of the Federal Employment Agency in Germany. Most data collecting and providing institutions in official statistics like the IAB face the dilemma between giving broad data access to researchers and the general public and at the same time ensuring data confidentiality. While statistical disclosure control is an integral part of data dissemination in official statistics, few courses or workshops are available as part of regular statistics study programs. Thus, the course has been an important part of the curriculum, especially for those interested in official statistics, and is currently offered every spring.
The prerecorded lectures of the course offer a broad overview of traditional statistical disclosure control methods like local suppression, rounding, microaggregation, swapping or sampling [39]. The instructor also introduces a relatively new approach to statistical disclosure control: synthetic data sets [40]. In the online meetings, the instructor and the students typically discuss open questions from the discussion forums, the assignments, and programming examples. The discussions in the online forum benefit from the sharing of experiences of those employed at institutions that need to find the right balance between data confidentiality, analytical validity of the data, and data access. By the end of the course, students know which measures are typically taken by statistical agencies in their own and other countries to guarantee confidentiality and are aware of potential limitations. They are informed about the concept of synthetic data and can also generate synthetic data sets themselves and evaluate the disclosure risk of the generated data. Grading in the course is based on participation as well as quizzes on the more theoretical aspects of data confidentiality and assignments, which in part require practical R programming.
6.Micro-credentials
One of the key concerns in post-graduate programs for working professionals is that students have a limited time budget for further education. While the IPSDS learning format was specifically developed to accommodate the busy schedule of working professionals, part of the targeted group is still unable to complete an entire master’s program. Nevertheless, professionals employed in official statistics, research institutes, universities, or other organizations, are often interested in broadening their skill set and knowledge. And while traditional university degrees remain very important, ‘there is an increasing need for alternative credentials that can better document the informal, online, and lifelong development of skills and knowledge both inside and outside the university’ [41, p. 593]. So-called “micro-credentials” have thus become much more important and common in recent years. Those smaller learning units represent the mastery of a limited set of skills or competencies rather than broader and interrelated set of skills, like full bachelor degrees” [42, p. 2].
The increasing interest in micro-credentials can also be observed in official statistics: The topic was discussed in the January 2021 board meeting for the European Master in Official Statistics (EMOS).33 While the board stated some caution regarding the minimum requirements and the quality of the material, which is also discussed controversially in educational literature [43], the EMOS-Board acknowledged micro-credentials in their January discussion as “one way forward for personalizing teaching and providing certificates for smaller amounts of learning, especially for already employed professionals.”
Figure 3.
MDM course bundles.
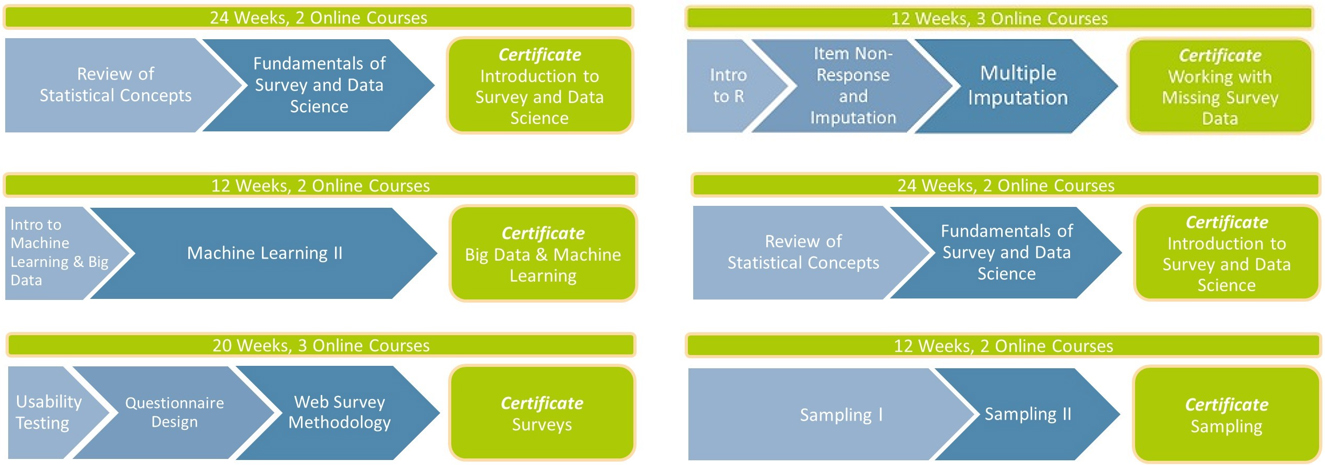
IPSDS has registered many requests from people who are very interested in one or a few IPSDS courses but are not able to commit to the whole Master’s program. Therefore, since summer 2021, IPSDS offers micro-credentials through the Mannheim Business School as so-called open courses. External participants can book all courses from the IPSDS curriculum individually. The open course participants join the students of the Master’s program for their selected course(s) and receive the same qualified learning experience in a proven online environment. After passing the course, they receive a certification of completion. For people interested in developing their skill set in a specific area, the program also offers course bundles (see Fig. 3), such as the course bundle on working with missing survey data. This bundle includes the two courses on item nonresponse and multiple imputation and an introductory R course since the other courses require a certain degree of R programming skills.
7.Summary
New sources of data and analysis methods provide opportunities to deliver more efficient and effective official statistical services. However, extracting relevant and reliable information from new and traditional sources and incorporating new and traditional data analysis methods into the statistical production process is a challenging task. These developments require those in charge of data generating processes, data storage, data analysis, and data output to periodically update their skills and knowledge in the area of survey and data science.
For working professionals in official statistics, IPSDS offers a genuine online learning environment accessible from anywhere in the world since 2016. Both a master’s program, as well as certificates for single courses and bundles of courses, are available. One of the most important fields the students can apply new skills to is the field of official statistics. When the Master’s program was formally institutionalized as Master of Applied Data Science and Measurement at the Mannheim Business School and the University of Mannheim, program management applied to become certified as European Master in Official Statistics (EMOS)44 and was able to receive the label in May 2021 under conditions and recommendations.
The program emphasizes interaction between faculty and students as well as networking and working in (international) groups. A significant part of the faculty and the student body is currently working or has worked in statistical agencies in Europe and the US. The coordination of the IPSDS training with work and leisure time is possible through the flipped classroom design. Classes are a mix of synchronous and asynchronous learning in small groups of no more than fifteen students. Additional courses like the project consulting course, internships, work projects, and the Master’s project complement the Master’s program focused on applications in real-world data settings. The program covers data collection, data analysis, dissemination, and data quality, taking into account various data sources and their combination. Elective courses offer students insights into new developments like big data or geospatial data in official statistics complementing the skills they can gain in mandatory courses.
In the evaluations, Students have mentioned several aspects of the program that they appreciate. The supporting network of students who are all professionals in the same or adjacent fields is often stressed as an asset, as well as the courses that are divided into small segments that allow flexible learning. The variety of classes and cutting-edge topics that are covered are another strength that is frequently emphasized.
For education and training, there is now a wide range of offerings from universities and providers from the field of online learning. Formats range from single, free-of-charge online courses that can be taken with thousands of other students from around the world (MOOCs) to traditional face-to-face academic bachelor’s or master’s programs. In between, however, we believe there is also demand for hybrid programs such as IPSDS, that combine the flexibility of online learning with interaction in an online classroom setting, especially regarding training in official statistics.
Notes
1 https://ec.europa.eu/eurostat/cros/content/essnet-big-data-1_en [Last Access: May 1, 2021].
2 ‘The European Credit Transfer System (ECTS) is a point system used by universities and agreed by governments […]. ECTS points, or ECTS credits indicate the required workload to complete a study programme, or a module within a study programme. […] The typical “full course load” at an American university implies 15 U.S. credits per Semester, which is equal to 30 ECTS credits at a European university. So the factor between American and European credits is usually 2 […].’ https://www.study.eu/article/what-is-the-ects-european-credit-transfer-and-accumulation-system [Last accessed: April 25, 2021].
3 See meetings conclusion and agenda: https://ec.europa.eu/eurostat/cros/content/2021-01-28emos-board-no-13conclusions-and-agenda_en [Last access: April 25, 2021].
4 https://ec.europa.eu/eurostat/cros/content/emos-explained_en [Last Accessed: May 2, 2021].
Acknowledgments
The IPSDS project on which this report is based was funded by the German Federal Ministry of Education and Research under the number [16OH22064].
References
[1] | Wiengarten L, Zwick M. Neue digitale Daten in der amtlichen Statistik. In: König C, Schröder J, Wiegand E, eds. Big Data [Internet]. Wiesbaden: Springer Fachmedien Wiesbaden; (2018) [cited 2021 Apr 25]. pp. 43–60. Available from: doi: 10.1007/978-3-658-20083-1_5. |
[2] | Radermacher WJ. Official Statistics 4.0: Verified Facts for People in the 21st Century [Internet]. Cham: Springer International Publishing; (2019) [cited 2021 Apr 25]. Available from: doi: 10.1007/978-3-030-31492-7. |
[3] | Howison J, Crowston K, Wiggins A. Validity issues in the use of social network analysis with digital trace data. J Assoc Inf Syst. (2011) ; 12(12). |
[4] | Schnell R. “Big Data” aus wissenschaftssoziologischer Sicht: Warum es kaum sozialwissenschaftliche Studien ohne Befragungen gibt (Big Data From a Sociological Point of View: Why There Are Few Studies in the Social Sciences Not Using Surveys). SSRN Electron J [Internet]. (2018) [cited 2021 Apr 25]; Available from: https://www.ssrn.com/abstract=3548537. |
[5] | Daas PJH, Puts MJ, Buelens B, van den Hurk PAM. Big data as a source for official statistics. J Off Stat. (2015) Jun 1; 31: (2): 249–62. |
[6] | Tokle J, Bender S. Record linkage. In: Foster I, Ghani R, Jarmin R, Kreuter F, Lane J, eds. Big data and social science research: theory and practical approaches. Boca Raton: Chapman and Hall/CRC Press; (2016) . pp. 23–70. |
[7] | Sakshaug JW, Antoni M. Errors in Linking Survey and Administrative Data. In: Biemer PP, de Leeuw E, Eckman S, Edwards B, Kreuter F, Lyberg LE, et al., eds. Total Survey Error in Practice [Internet]. Hoboken, NJ, USA: John Wiley & Sons, Inc.; (2017) [cited 2021 Apr 25]. pp. 557–73. Available from: doi: 10.1002/9781119041702.ch25. |
[8] | Keusch F, Kreuter F. Zukunft der Aus-und Weiterbildung in der Markt-und Sozialforschung. In: Keller B, Klein H-W, Wachenfeld-Schell A, Wirth T, eds. Marktforschung für die Smart Data World [Internet]. Wiesbaden: Springer Fachmedien Wiesbaden; (2020) [cited 2021 Apr 25]. pp. 3–25. Available from: doi: 10.1007/978-3-658-28664-4_1. |
[9] | Tucker B. The Flipped Classroom [Internet]. Education Next. (2011) [cited 2021 Apr 30]. Available from: https://www.educationnext.org/the-flipped-classroom/. |
[10] | Bates JE, Almekdash H, Gilchrest-Dunnam MJ. The Flipped Classroom: A Brief, Brief History. In: Santos Green L, Banas JR, Perkins RA, eds. The Flipped College Classroom: Conceptualized and Re-Conceptualized [Internet]. Cham: Springer International Publishing; (2017) [cited 2021 Apr 30]. pp. 3–10. (Educational Communications and Technology: Issues and Innovations). Available from: doi: 10.1007/978-3-319-41855-1_1. |
[11] | Samoilova E. Target group and accepted test-cohorts (1-3) [Internet]. Vol. 5, IPSDS Assessment Report. Mannheim: Universität Mannheim; (2018) [cited 2021 May 1]. Available from: https://madoc.bib.uni-mannheim.de/59079. |
[12] | Japec L, Kreuter F, Berg M, Biemer P, Decker P, Lampe C, et al. Big data in survey research: AAPOR task force report. Public Opin Q. (2015) ; 79: (4): 839–80. |
[13] | Usher A. Skills required to integrate big data into public opinion research. 70th Annual Conference of the American Association for Public Opinion Research; (2015) ; Hollywood, Florida. |
[14] | Leek JT, Peng RD. What is the question? Science. (2015) Mar 20; 347: (6228): 1314–5. |
[15] | Sakshaug JW, Wisniowski A, Perez Ruiz DA, Blom AG. Supplementing small probability samples with nonprobability samples: a bayesian approach. J Off Stat. (2019) ; 35: (3): 653–81. |
[16] | Cornesse C, Blom AG, Dutwin D, Krosnick JA, De Leeuw ED, Legleye S, et al. A review of conceptual approaches and empirical evidence on probability and nonprobability sample survey research. J Surv Stat Methodol. (2020) Feb 1; 8: (1): 4–36. |
[17] | Connelly R, Playford CJ, Gayle V, Dibben C. The role of administrative data in the big data revolution in social science research. Soc Sci Res. (2016) Sep; 59: : 1–12. |
[18] | Groen J. Sources of error in survey and administrative data: the importance of reporting procedures. J Off Stat. (2012) Jun 1; 28: : 173–98. |
[19] | Neylon C. Working with web data and APIs. In: Foster I, Ghani R, Jarmin R, Kreuter F, Lane J, eds. Big data and social science research: theory and practical approaches [Internet]. Boca Raton: Chapman and Hall/CRC; (2020) [cited 2021 Apr 25]. pp. 25–42. Available from: https://www.taylorfrancis.com/https://www.taylorfrancis.com/chapters/edit/10.1201/9780429324383-2/working-web-data-apis-cameron-neylon. |
[20] | Munzert S, Rubba C, Meißner P, Nyhuis D. Automated data collection with R: a practical guide to Web scraping and text mining. Chichester, West Sussex, United Kingdom: Wiley; (2015) . |
[21] | Salganik MJ. Bit by Bit: Social Research in the Digital Age. Reprint edition. Princeton University Press; (2017) . 448. |
[22] | Harms C, Schmidt S. Marktforschung im Daten-Zeitalter: Software ist nur die halbe Miete [Internet]. (2019) [cited 2021 Apr 25]. Available from: https://www.marktforschung.de/dossiers/themendossiers/plattformen-und-datensysteme-2019/dossier/marktforschung-im-daten-zeitalter-software-ist-nur-die-halbe-miete/. |
[23] | Curran PG. Methods for the detection of carelessly invalid responses in survey data. J Exp Soc Psychol. (2016) Sep 1; 66: : 4–19. |
[24] | Carpenter JR, Kenward MG. Multiple Imputation and its Application: Carpenter/Multiple Imputation and its Application [Internet]. Chichester, UK: John Wiley & Sons, Ltd; (2013) [cited 2021 Apr 25]. Available from: doi: 10.1002/9781119942283. |
[25] | Haddi E, Liu X, Shi Y. The role of text pre-processing in sentiment analysis. Procedia Comput Sci. (2013) ; 17: : 26–32. |
[26] | Hassani H, Saporta G, Silva ES. Data mining and official statistics: the past, the present and the future. Big Data. (2014) Mar; 2: (1): 34–43. |
[27] | Tam S-M, Clarke F. Big data, official statistics and some initiatives by the australian bureau of statistics: big data and the ABS1. Int Stat Rev. (2015) Dec; 83: (3): 436–48. |
[28] | Jahani E, Sundsøy P, Bjelland J, Bengtsson L, Pentland A ‘Sandy’, de Montjoye Y-A. Improving official statistics in emerging markets using machine learning and mobile phone data. EPJ Data Sci. (2017) Dec; 6: (1): 3. |
[29] | Tzavidis N, Zhang L, Luna A, Schmid T, Rojas-Perilla N. From start to finish: a framework for the production of small area official statistics. J R Stat Soc Ser A Stat Soc. (2018) Oct; 181: (4): 927–79. |
[30] | Ridgway J, Nicholson J, Sutherland S, Hedgers S. Strategies for public engagement with official statistics. In: Sorto MA, ed. Advances in statistics education: developments, experiences and assessments Proceedings of the Satellite conference of the International Association for Statistical Education (IASE), July 2015, Rio de Janeiro, Brazil [Internet]. Rio de Janeiro, Brazil: International Association for Statistical Education (IASE); (2015) [cited 2021 Apr 25]. Available from: http://iase-weborg/Conference_Proceedings.php?p=Advances_in_Stats_Education_2015. |
[31] | Luhmann, Sybille, Grazzini, Jacopo, Ricciato, Fabio, Meszaros, Matyas, Giannakouris, Konstantinos, Museux, Jean-Marc, et al. Promoting reproducibility-by-design in statistical offices. In Zenodo; (2019) [cited 2021 Apr 25]. Available from: https://zenodo.org/record/3240198. |
[32] | Wilkinson MD, Dumontier M, Aalbersberg IJJ, Appleton G, Axton M, Baak A, et al. The FAIR guiding principles for scientific data management and stewardship. Sci Data. (2016) Mar 15; 3: : 160018–160018. |
[33] | United Nations. Resolution adopted by the General Assembly on 29 January 2014 – 68/261 Fundamental Principles of Official Statistics. General Assembly sixty-eighth session, Agenda item 9; (2014) . |
[34] | United Nations Statistics Division. United Nations nationalquality assurance frameworks manual for official statistics: including recommendations, the framework and implementation guidance. New York: United Nations; (2019) . |
[35] | Lane J, Stodden V, Bender S, Nissenbaum H, eds. Privacy, Big Data, and the Public Good: Frameworks For Engagement. 10.1017/CBO9781107590205. Cambridge: Cambridge University Press; (2014) . |
[36] | Biggs J. Aligning Teaching and Assessment to Curriculum Objectives. Chicago: Imaginative Curriculum Project, LTSN Generic Centre; (2003) . |
[37] | Samoilova E, Keusch F, Kreuter F. Integrating Survey and Learning Analytics Data for a Better Understanding of Engagement in MOOCs. In: Jiao H, Lissitz RW, Van Wie A, eds. Data Analytics and Psychometrics Informing Assessment Practices. Charlotte, NC: Informatione Age Publishing, INC.; (2018) . (The MARCES Book Series). |
[38] | Reich J, Ruipérez-Valiente JA. The MOOC pivot. Science. (2019) Jan 11; 363: (6423): 130–1. |
[39] | Reiter JP. Statistical approaches to protecting confidentiality for microdata and their effects on the quality of statistical inferences. Public Opin Q. (2012) Mar 1; 76: (1): 163–81. |
[40] | Raghunathan TE, Reiter JP, Rubin DB. Multiple imputation for statistical disclosure limitation. J Off Stat. (2003) ; 19: (1): 1. |
[41] | West RE, Newby T, Cheng Z, Erickson A, Clements K. Acknowledging All Learning: Alternative, Micro, and Open Credentials. In: Bishop MJ, Boling E, Elen J, Svihla V, eds. Handbook of Research in Educational Communications and Technology [Internet]. Cham: Springer International Publishing; (2020) [cited 2021 Apr 25]. pp. 593–613. Available from: doi: 10.1007/978-3-030-36119-8_27. |
[42] | Ehlers U. Higher creduation-degree or education? The rise of micro-credentials and its consequences for the university of the future. In Genoa; (2018) . |
[43] | Ralston SJ. Higher education’s microcredentialing craze: a postdigital-deweyan critique. Postdigital Sci Educ. (2021) Jan 1; 3: (1): 83–101. |
[44] | Kreuter F, Keusch F, Samoilova E, Frößinger K. International Program in Survey and Data Science. In: König C, Schröder J, Wiegand E, eds. Big Data: Chancen, Risiken, Entwicklungstendenzen [Internet]. Wiesbaden: Springer Fachmedien Wiesbaden; (2018) . pp. 27–41. Available from: doi: 10.1007/978-3-658-20083-1_4. |
Appendices
Appendix
Table A1
Courses offered as part of the IPSDS curriculum in 2021
Course | Type | Focus area | Ects credits | Instructor(s) | |
---|---|---|---|---|---|
Name | Affiliation | ||||
Review of Statistical Concepts | Core | Data Analysis | 6 | Brian Kim | Lecturer at the University of Maryland |
Privacy Law | Core | Data Output/ Access | 2 | Thomas Fetzer | Professor and Chair for Public Law, Regulation and Tax Law at the University of MannheimAdjunct Professor of Law at the University of Pennsylvania Law School |
Introduction to R course | Tutorial | – | – | Self-paced | |
Fundamentals of Survey and Data Science | Core | Research Design | 6 | Alexander Wenz | Postdoctoral Researcher at Professorship for Statistics and Methodology at University of Mannheim |
Introduction to Real World Data Management | Core | Data Curation/Storage | 4 | Alexandru Cernat | Senior Lecturer in Social Statistics at the University of Manchester |
Questionnaire Design | Core | Data Generating Process | 4 | Gina Walejko | Senior User Experience Researcher at Google |
Modern Workflow in Data Science | Core | Data Curation/ Storage | 4 | Alexandru Cernat | Senior Lecturer in Social Statistics at the University of Manchester |
Ethical Considerations for Data Science Research | Core | Data Output/ Access | 2 | Jessica Vitak | Associate Professor at College of Information Studies of the University of Maryland |
Introduction to Record Linkage with Big Data Applications | Core | Data Generating Process | 4 | Manfred Antoni | Senior Researcher, Research Data Center (FDZ) of the Federal Employment Agency at the Institute for Employment Research (IAB) |
Stefan Bender | Head of the Research Data and Service Center of the Deutsche BundesbankChair of INEXDAHonorary Professor at School of Social Sciences, University of Mannheim | ||||
Christian Borgs | Statistics Department of I.T.NRW | ||||
Joe Sakshaug | Distinguished Researcher, Deputy Head of Research/Head of the Data Collection and Data Integration Unit, Statistical Methods Research Department at the IABUniversity Professor of Statistics, LMU MunichHonorary Professor at School of Social Sciences, University of MannheimAdjunct Research Assistant Professor, University of Michigan | ||||
Generalized Linear Models | Core | Data Analysis | 4 | Thomas Gautschi | Professor and Chair in Sociological Methodology at the University of Mannheim |
Sampling I | Core | Data Generating Process | 4 | Raphael Nishimura | Director of Sampling Operations at the University of Michigan |
Introduction to Data Visualization | Core | Data Output/Access | 2 | Richard Traunmüller | Full Visiting Professor at University of MannheimJunior Professor at Goethe University Frankfurt (on leave) |
Project Consulting Course | Core | All Areas | 12 | Helmut Küchenhoff | Professor at the Department of Statistics at theLudwig-Maximilians-Universitaät München (LMU)Head of Consulting Unit (StaBLab) at the LMU |
Stefan Bender | Head of the Research Data and Service Center of the Deutsche BundesbankChair of INEXDA Honorary Professor at School of Social Sciences, University of Mannheim | ||||
Introduction to Official Statistics | Elective | Data Output/Access | 2 | Walter Rademacher | Visiting Researcher at Sapienza Università di Roma President of the Federation of European National Statistical Societies FENStatS |
Table A1, continued | |||||
---|---|---|---|---|---|
Course | Type | Focus area | Ects credits | Instructor(s) | |
Name | Affiliation | ||||
Advanced Topics in Official Statistics | Elective | Data Output/Access | 2 | Hanna Brenzel | Head of Department’ Methods of Data Analysis’ at Federal Statistical Office of Germany |
Piet Daas | Senior Methodologist & Data Scientist for Big Data, Statistics Netherlands Part-time professor by special appointment at Eindhoven University of Technology | ||||
Marco Puts | Methodologist and Lead Data Scientist at the Center for Big Data, Statistics in Netherlands Guest Researcher at Radbound University | ||||
Data Confidentiality and Statistical Disclosure Control | Elective | Data Output/Access | 4 | Jörg Drechsler | Distinguished researcher at IAB Honorary Professor at the School of Social Sciences of the University of Mannheim Adjunct Associate Professor, Joint Program in Survey Methodology, University of Maryland |
Web Scraping and APIs | Elective | Data Generating Process | 2 | Simon Munzert | Assistant Professor in Data Science and Public Policy at Hertie School Adjunct Assistant Professor at the University of Maryland |
Experimental Design for Surveys | Elective | Data Generating Process | 4 | Ashley Amaya | Senior Research Survey Methodologist at RTI International |
Sampling II | Elective | Data Generating Process | 2 | Raphael Nishimura | Director of Sampling Operations at the University of Michigan |
Usability Testing for Survey Research | Elective | Data Generating Process | 2 | Emily Geisen | Senior Experience Management Scientist at Qualtrics Lecturer at University of North Carolina |
Web Survey Methodology and Online Panels with Practical Survey Programming | Elective | Data Generating Process | 4 | Nejc Berzelak | Statistician and methodologist at Faculty of Social Sciences, University of Ljubljana |
Survey Design and Implementation in International Contexts | Elective | Data Generating Process | 2 | Zeina Mneimneh | Director of the International Unit at the Survey Research Center of the University of Michigan Assistant Research Scientist at the University of Michigan |
Introduction to Python and SQL | Elective | Data Curation/Storage | 2 | Brian Kim | Lecturer at the University of Maryland |
Multiple Imputation – Why and How | Elective | Data Curation/Storage | 2 | Jörg Drechsler | Distinguished researcher at IAB Honorary Professor at the School of Social Sciences of the University of Mannheim Adjunct Associate Professor, Joint Program in Survey Methodology, University of Maryland |
Introduction to Big Data and Machine Learning | Elective | Data Analysis | 2 | Frauke Kreuter | Professor of Statistics and Data Science for the Social Sciences and Humanities at the Ludwig-Maximilians-University of Munich Co-director of Data Science Centers at the University of Maryland (USA) and Mannheim (Germany) Head of Statistical Methods Research Department (KEM) at the IAB |
Trent Buskirk | Novak Family Professor of Data Science and Chair of the Applied Statistics and Operations Research Department at Bowling Green State University | ||||
Machine Learning II | Elective | Data Analysis | 4 | Trent Buskirk | Novak Family Professor of Data Science and Chair of the Applied Statistics and Operations Research Department at Bowling Green State University |
Christoph Kern | Post-Doctoral Researcher at the Professorship for Statistics and Methodology at the University of Mannheim | ||||
Analysis of Complex Survey Data | Elective | Data Analysis | 4 | Stefan Zins | Specialist at Competence Center for Empirical Methods (KEM) at Institute for Employment Research (IAB) of the Federal Agency of Employment Germany |
Table A1, continued | |||||
Course | Type | Focus area | Ects credits | Instructor(s) | |
Name | Affiliation | ||||
Item Nonresponse and Imputation | Elective | Data Analysis | 2 | Jörg Drechsler | Distinguished researcher at IAB Honorary Professor at the School of Social Sciences of the University of Mannheim Adjunct Associate Professor, Joint Program in Survey Methodology, University of Maryland |
Step by Step in Survey Weighting | Elective | Data Analysis | 2 | Stefan Zins | Specialist at Competence Center for Empirical Methods (KEM) at Institute for Employment Research (IAB) of the Federal Agency of Employment Germany |