Linking statistical literacy and data stewardship in Public Universities of Niger: Lessons learned from the collaboration with the national statistics institute
Abstract
This paper aims at highlighting the lessons learned from recent initiatives between public universities of Niger and the national statistics institute.
Our investigation of the existing national statistical system revealed the need to increase the number of qualified human resources with advanced skills in open data, big data, data visualisation, machine learning, mathematical modeling and data-driven innovations.
Moreover, the existing statistical literacy and data crowdsourcing activities need to be validated and upscaled; and we have found a lack of experience in managing big data and in the development of mathematical methods and fast computational algorithms to analyze them.
Finally, the aforementioned collaboration can be improved by working closely with private sector, civil society and the data science community to generate new approaches to emerging issues including climate change and sustainable development.
1.Introduction
Statistical literacy and data stewardship are important for citizens, academia, journalists, industry or policy makers; particularly in the context of the ongoing data revolution which is changing how we access and use data and information related to global, continental or national development agendas.
National statistical offices (NSO) and universities are among the key actors of this process particularly in terms of the definition, implementation, monitoring progress or evaluation of these agendas. Facts that require to develop new skills for harnessing the potential of this data revolution.
In Niger, public universities are collaborating with the national statistics institute (NSI) on many activities including empirical studies [1, 2, 3], research projects, surveys, national censuses, statistical literacy and data stewardship, capacity development and training. During the last two decades these activities fitted under the framework of the United Nations (UN) Millenium Development Goals (MDGs), the National Strategies for the Development of Statistics (NSDS), the New Partnership for Africa’s Development (NEPAD), the UN Sustainable Development Goals (SDGs, Agenda 2030) and the African Union (AU) Agenda 2063.
The first National Strategy for the Development of Statistics (NSDS I) [4] in Niger, covered the period from 2008 to 2012 and the second one (NSDS II) was supposed to cover the period from 2014 to 2018 [5]. As mentioned in [6], the partial implementation of NSDS II (due to its non adoption by the goverment) led to its modification (in 2016) and extension for covering the 2017–2021 period. This delay in adoption of the initial NSDS II was motivated by the integration of the needs of: the upcoming Economic and Social Development Plan (ESDP, 2017–2021), the aforementioned Agendas 2030 and 2063.
The NSI recently conducted (in July 2019) a revision of the policy document for dissemination of the National Statistical System(NSS) in order to integrate the aspects relating to the access to microdata.
The SDGs report 2017 [7] highlighted the importance of harnessing the power of data for sustainable development. In this context, there is a growing demand for data and statistics that are relevant, accurate, timely, sufficiently disaggregated, accessible and easy to use. Facts that require to pursue coordinated efforts between data producers and end-users; via finding innovative ways to produce and apply data and statistics in addressing the growing complexity of sustainable development.
Two(2) SDGs National Voluntary Reviews (NVRs) for Niger were respectively conducted in 2018 [8] and 2020 [9]; based on the 2017 baseline reference situation for indicators of the targets identified as priorities for the implementation of the SDGs [10].
Although the NVRs revealed some data gaps that can be bridged by harnessing the potential of the data generated by citizens, private sector or academia, it is important to have a critical mass of data scientists and statisticians who are able to control the quality of the collected data and who are able to analyze it and extract relevant information using data science and big data approaches. Unfortunately, these competences are not available or very scarce in the majority of the NSO in Africa.
Young generations are at the center of these (short to long term) agendas for instance SDGs Target 4.4 aims at “By 2030, substantially increase the number of youth and adults who have relevant skills, including technical and vocational skills, for employment, decent jobs and entrepreneurship”. Furthermore as “digital natives” they are able to acquire the new skills which are necessary to compile SDGs indicators in new and exciting ways.
Our fundamental research question can be formulated as follow: How can we develop competences for the university students in the context of the current data revolution in Niger?
The rest of this paper is organized as follows: Section 2 is about methodology; Section 3 investigates the concepts of statistical literacy and data stewardship in the context of the ongoing data revolution; Section 4 analyzes human resources and competences within the Nigerien National Statistical System; Section 5 is dedicated to the collaboration between the NSI and Public Universities of Niger. Section 6 is about discussion and concluding remarks are given in Section 7.
2.Methodology
The conceptualization of statistical literacy, data stewardship and data revolution is very important as we have a lot of definitions or interpretations related to these concepts.
Moreover, we will investigate the linkages between statistical literacy and data stewardship in the context of the existing collaboration between the NSI and public universities of Niger (particularly Abdou Moumouni University) over the 2014–2020 period.
Our analysis will use data from online Google forms based quizzes, competition application forms, reports, etc.
In terms of development agendas, the focus will be made on the UN SDGs.
3.Statistical literacy, data stewardship and data revolution for SDGs22
Ben-Zvi and Garfield [11] define statistical literacy as “a set of skills that students may actively use in understanding statistical information – among them, organising data, constructing tables or being able to work with different varieties of data representations”.
Furthermore, the concepts of data literacy and statistical literacy are often used without distinction; and sometimes data literacy is considered as a sub-component of statistical literacy [12].
Some institutions like the Australian Bureau of Statistics’ Education Services consider four criteria essential for statistical literacy:
1. data awareness;
2. the ability to understand statistical concept;
3. the ability to analyse, interpret and evaluate statistical information;
4. the ability to communicate statistical information and understandings
Moreover, as highlighted by Rosenbaum [13] “Data stewardship can be thought of as a collection of data management methods covering acquisition, storage, aggregation, and deidentification, and procedures for data release and use”.
The Africa Data Revolution Report [14] conceptualized the “African data revolution” and characterized it by:
1. the exponential increase in the volume, types and speed of data available in African countries;
2. the increased availability and use of new types of data as well as new uses of old or conventional types of data;
3. new principles of data governance, including the principles of inclusion and openness; and
4. new data-related rights and freedoms.
Finally, the UN Independent Expert Advisory Group on the Data Revolution for Sustainable Development defines the data revolution as an “explosion in the volume of data, the speed with which data are produced, the number of producers of data, the dissemination of data, and the range of things on which there is data, coming from new technologies such as mobile phones and the ‘Internet of things’, and from other sources, such as qualitative data, citizen-generated data and perceptions data”.
In this context, it is important to investigate the needs for human resources and competences in the National Statistical System of Niger.
4.National Statistical System and human resources and competences
The first National Strategy for the Development of Statistics (NSDS I) in Niger was adopted in 2008 and covered the period from 2008 to 2012. The second one (NSDS II) which was initially supposed to cover the period from 2014 to 2018; was modified in 2016 and extended for covering the 2017–2021 period.
The weaknesses of the NSS identified by the NSDS include:
• insufficient human resources in quantity and quality;
• operational problems of data collection, processing, analysis, publication and dissemination, due to the insufficiency of human resources in quantity and quality, but also of material and financial resources;
The NSDS priority programs include:
• Human resources development and applied research;
• Dissemination of productions and promotion of a statistical culture.
The types of profiles to be valued in the human resources development and operational research plan are essentially related to three (3) related fields:
(i) statistics/economics;
(ii) demography;
(iii) computer science.
In addition, all levels of qualification (PhD, Engineering, Master, Bachelor, etc) in these three areas are sought by both the NSI and the sectoral statistical services.
Although the NSI have had to evolve to become “data steward”, the concepts of data revolution, citizen-generated data, data science, big data (categories and sources), open data and data visualization were not mentioned or detailed in NSDS I and NSDS II.
Citizen-generated data, earth observations and satellite imagery can be used as complement to traditional data sources. Particularly in the context of the SDGs that require real-time monitoring and the need to address reliability and geographic coverage challenges associated with traditional data methods in hard to reach areas. Results from these newer data sources can be validated by classical census and household surveys.
Finally, collaboration between NSO and universities has been identified among means for acquiring skilled statisticians and supporting data-science-driven work [15].
5.Collaboration between NSI and Public Universities of Niger
5.1Overview of activities
Although the NSI of Niger regularly collaborates with local research institutions and public universities; we will highlight the collaboration with Abdou Moumouni University (the first national university created in 1971 and located in the Niamey capital city of Niger).
Table 1
Overview of the collaboration between the NSI and Abdou Moumouni University during the 2014–2020 period. Source: author
Activity | Period/frequency |
– Capacity Building for admission to regional specialized higher schools of statistics, applied economics and demography | Yearly |
– Household surveys (nutrition, health, employment, youth ICT skills, …) | Yearly |
– Human Development Index Analysis | 2014 |
– African Statistics Day | Yearly |
– AYOROU Macroeconomic framing Model | 2014 |
– Study of Middle Class Emergency and Inequality reduction | 2014–2016 |
– Study of the Quality of Public Expense and impact on economic growth and employment | 2015 |
– Creation of the National Higher School of Statistics | 2016–2019 |
– Social Accounting Matrix Validation Workshop | 2017 |
– Café Statistique | Quarterly |
– Application to the World Bank Collaborative Data for Sustainable Development call for proposals | 2018 |
– Analysis of the National Priorities for the UN SDGs | 2016–2017 |
– Development of an Open Data Portal | 2018–2019 |
– Participation to the International Statistical Poster Competition | 2018–2019 |
– 60 th anniversary of the National Statistics Institute | 2019 |
– National Statistical System dissemination policy document | 2019 |
– Panel on Open Data and SDGs as part of the Innovation for Development (I4Dev) International Conference (side event of the African Union Head of State and Government Summit in Niger) | 2019 |
– COVID-19 official information management and dissemination | 2020 |
– Development of an Android mobile app for the dissemination of official statistical data | 2020 |
Table 1 gives an overview of the activities conducted from 2014 to 2020. This period corresponds to the end of the UN Millenium Development Goals and the adoption and implementation of the Sustainable Development Goals agenda.
These activities include capacity building and training, surveys, empirical studies, mathematical and statistical modeling, collaborative research projects, open data initiatives, policy documents, internships, conference panels, statistical literacy competitions and outreach as well as the recent COVID-19 pandemic management.
In 2018 Abdou Moumouni University collaborated with the NSI and other partners (business, public adminsitration, students union, national coordination of the international statistical literacy project, …) on a project entitled “Leveraging crowdsourcing and citizen science for bridging data gaps on information and communication technologies’ skills in public universities of Niger” as part of the World Bank Development Data Group and The Global Partnership for Sustainable Development Data call for Proposals 2018.33 The types of data proposed for this project are available in Table 2.
Table 2
Types of data proposed for the World Bank Collaborative Data for Sustainable Development call for proposals 2018. Source: author
Types of data |
---|
Administrative data |
Commercially licensed data |
Geospatial data |
Metadata (e.g. call detail records) |
Satellite imagery |
Sensor data |
Paradata(e.g geographic location of the respondents, the used devices, browsers and platforms; level of vocabulary in a text) |
5.2Assessing students awareness of the SDGs
Outreach activities identified in Table 1 include the assessment of students awareness about the SDGs, civic statistics and other topics related to global citizenship. In 2017 and 2019 two (2) assessments were conducted via online quizzes (as part of the homeworks for bachelor students enrolled within the ICT course at the faculty of sciences and techniques) with respectively 337 respondents (86.1% males and 13.9% females) and 306 respondents (78.1% males and 21.9% females). Figure 1 illustrates the first question of these quizzes (in french language) which is “1. What do the illustrations below show?”.
Figure 1.
First question of the SDGs awareness quizzes conducted in 2017 and 2019. Source: author.
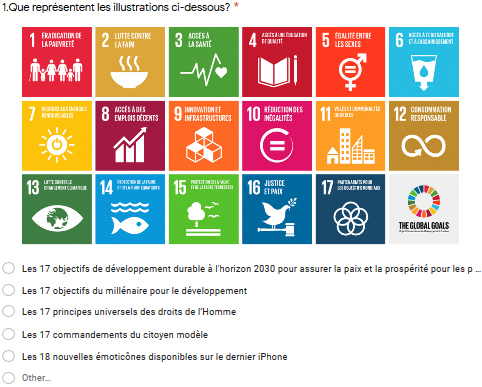
Figure 2.
a. Responses to the first question of the SDGs awareness quizz (2017). Correct response is in green color (67.7% of the respondents); b. Responses to the first question of the SDGs awareness quizz (2019). Correct response is in green color (65.4% of the respondents).
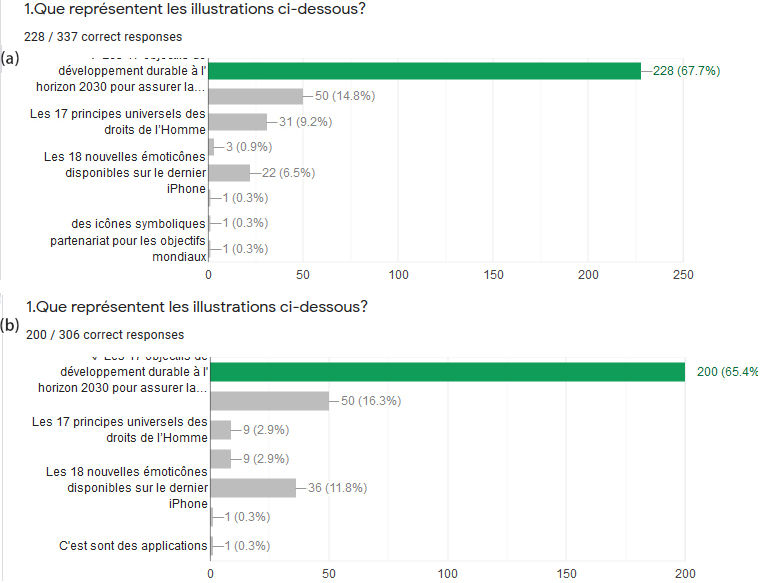
Figure 2a and b show that 67.7% of the respondents (respectively 65.4% of respondents) have found the correct response in 2017 (respectively in 2019). These students have diverse backgrounds (Biology, Biochemistry, Theoretical Chemistry, Electronics Electrotechnics and Automatics, Geology, Computer Science, Mathematics and Theoretical Physics).
The remaining questions of this quiz (multiple choice, checkboxes, dropdown, multiple choice grid, checkbox grid) are related to the WHO ambient (outdoor) air pollution factsheet.44
For example 96.1% of the respondents in 2017 (respectively 88.6% of respondents in 2019) have answered correctly that stroke, heart disease, lung cancer and respiratory conditions, chronic or acute are the four(4) main diseases caused by air pollution.
Additionally, the 2017 quiz (respectively the 2019 quiz) revealed that 72.1% of the respondents (respectively 69% of the respondents) have found the correct response about the number and causes of premature deaths related to outdoor air pollution in urban and rural areas in 2012 (respectively in 2016). In fact it is estimated that in 2012 around 3.7 million premature deaths have been caused by exposure to PM
It is important to recall that the WHO is custodial agency for the following air pollution-related SDGs indicators:
• 3.9.1 Mortality from air pollution
• 7.1.2 Access to clean fuels and technologies
• 11.6.2 Air quality in cities.
The students also uploaded photos (crowdsourced database) about local cooking fuels and technologies. Sidi Zakari [16, 17] provided more details about this crowdsourcing activity; which can be upscaled so that it can complement existing data sources and official statistics for the definition and monitoring of the aforementioned SDGs indicators 7.1.2 and 11.6.2.
5.3Participation to statistical and data literacy competitions
5.3.1International Statistical Poster Competition 2018–2019
Abdou Moumouni University(AMU) collaborated with the NSI for the organization of the national round of the International Statistical Poster Competition 2018–2019 organized as part of the International Statistical Literacy Project(ISLP).55
Around 450 bachelor students from AMU and 50 students (from local high schools) were involved in this competition.
The national award winning posters in the university and high school categories were respectively entitled “Understanding internet use in public universities of Niger”66 and “Accidents in Niger”.77
Although high school students have only used secondary data sources (particularly a website with information on accidents in Niger) for their poster; bachelor students’ poster combined primary and secondary data sources to respond to the following questions:
• What are the various uses of internet within public universities in Niger?
• What are the obstacles to the use of the internet by university students?
• What are the proportions (by sex) of internet users within public universities in Niger?
This poster is a good example of the integration of Statistics and ICT to enlighten opinion on the use of the internet in public universities of Niger.
The national award ceremony took place on the sidelines of the 3rd edition of the Club of the students of the Department of Mathematics and Computer Science(CEDMI)’s day. This CEDMI’s day, under the high sponsorship of the Minister of Hydraulics, Hygiene and Sanitation had as its theme “Contribution of CEDMI to the achievement of the Sustainable Development Goals”.
A special mention has been awarded to a second team of bachelor students for their poster entitled “The use of medicinal plants in Niger: the case of moringa, tephrosia lupinifolia and panicum subaldidum”.
5.3.2The ReSAKSS Data Challenge99
This competition organized by the Regional Strategic Analysis and Knowledge Support System (ReSAKSS) is for “enthusiastic data users to showcase their creativity and analytical skills using data and other resources on the ReSAKSS website to develop an innovative knowledge product or project that addresses a development challenge faced by Africa”.
Each submission should fit under one of the following four(4) categories: (a) Essay and Report; (b) Visual Arts; (c) IT products and Services; (d) High School Projects.
During the first edition of this challenge in 2018, a team of three(3) students from Lycée d’Excellence High School of Niamey proposed a paper entitled “Agriculture and Development in Africa: the West Africa case study”. This paper has been drafted under the supervision of one of the AMU researcher.
Moreover, in order to promote national and regional data as well as short to long term agendas; a data literacy tool (auto-grading quiz with answers feedback)1010 has been proposed by a researcher from AMU. For instance, Fig. 3 gives an overview of a multiple choice grid that links the ReSAKSS data and information to: UN SDGs (2016–2030), African Union Agenda (2013–2063), the Niger Economic and Social Development Plan (2012–2015, 2017–2021) and the Comprehensive Africa Agriculture Development Programme (CAADP, 2015–2025). This data literacy tool is still under development through the integration of feedbacks from respondents who are participating to a pilot phase. One of the challenges identified during this pilot phase was related to the language barrier as the current version of the tool is in english instead of french (which is the official language in Niger). Additional feedbacks highlighted the need to use the data ReSAKSS provides, though it could easily be extended into that direction and it might be interesting to evaluate if it is possible to let people generate their own questions and maybe share with others (flash cards/spaced repetition). This would generate a growing question library with possibly country specific questions and use more of the data provided by ReSAKSS.
Figure 3.
A question from a data literacy tool proposed as part of the first edition of the ReSAKSS Data Challenge.
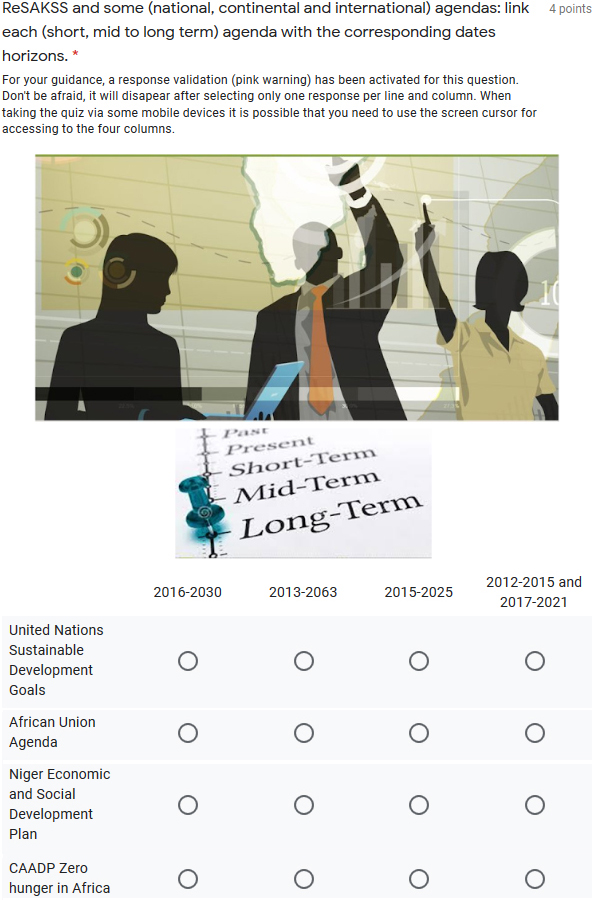
5.3.3Preparation of the UN Data for Climate Action Challenge 20171212
This competition highlighted the importance of Big Data (from private sector) for climate action with focus on climate mitigation (e.g energy, transportation), climate adaptation (e.g food and agriculture, community resilience) and the linkages between climate (SDG 13) and other SDGs (e.g. zero poverty, good health and well being, reducing inequality).
It has mobilized business leaders (who provided the challenge datasets) and the data science community to generate new approaches to climate action and sustainable development.
Companies contributing data for the challenge include:
• BBVA Data and Analytics, a financial data analytics company
• Crimson Hexagon, an enterprise social media analytics company
• Earth Networks, a weather and lightning sensor network operator
• Nielsen, a global measurement and analytics company
• Orange, a global telecommunications provider
• Planet, an earth-imaging satellite network operator
• Plume Labs, an environmental data sciences company
• Schneider Electric, a global specialist in energy management and automation
• Waze, a free, real-time community-based traffic and navigation app
When an individual or a team requested data for this challenge, it was possible to specify geographic, temporal, and other scope parameters. In addition, researchers may apply to receive cloud-computing support from Microsoft, and visual analytics support from Tableau. The competitors could also use additional open and public datasets and proprietary or other datasets.The final research submission include research paper or poster and application or tool.
This challenge required:
1. General academic and professional background in: Earth and Environmental Sciences, Mathematics and Statistics, Engineering, Computer Science and InformationTechnologies, Data Science, Policy and/or Social Sciences, etc.
2. Research communication skills in: Academic/ Scientific writing, Policy analysis, Data visualization, Dashboard/Application development, etc.
3. Knowledge and skills in: Computer Science, Data Science, Statistical and Machine Learning methods (see Table 3).
Table 3
Knowledge and skills in Computer Science, Data Science, Statistical and Machine Learning methods identified as part of the UN Data Challenge for Climate Action 2017. Source: author
Computer science |
Programming |
Software engineering |
System administration |
Database management |
Managing structured data (e.g., parsing, cleaning, joining, etc.) |
Managing unstructured data (e.g., parsing, cleaning, joining, etc.) |
Big data and distributed data processing frameworks |
Managing or developing cloud-based infrastructures (e.g., Azure, Google Cloud) |
Scientific computing |
Knowledge and skills in the field of data science |
Information extraction using Natural Language Processing techniques (e.g., named entity recognition, sentiment analysis, topic modeling) |
Computational linguistics (machine translation, speech recognition, human-computer interaction) |
Machine learning methods (Decision trees, SVM, neural networks, Kmeans, etc.) |
Search and Information retrieval (Lucene, SOLR, ElasticSearch) |
Image processing and classification |
Geospatial data integration |
Statistical and machine learning methods |
Bayesian statistics |
Classification |
Clustering |
Deep learning/Neural networks |
Maximum likelihood analysis |
Natural language processing/Text mining |
Optimization |
Prediction |
Regression |
Simulation |
Survival analysis |
Anomaly detection |
During the application process, the challenges faced by the research team from the department of mathematics and computer science of AMU were related to the lack of experience in the management of Big Data as well as the related expertise in terms of Computer Science, Data Science, Statistical and Machine Learning methods (which are not also available among the staff of the NSI).
The effective interpretation and the creation of new knowledge to solve the world’s problems (like climate change) are important for making useful the vast amount of data collected every day.
Moreover, in many cases the existing classical statistical methods do not work for such complex structured data; and the stochastic nature of the data (caused by model assumptions, measurement errors, …) requieres the development of mathematical methods and fast computational algorithms to analyze them.
Mathematical methods such as statistics, probability theory and optimisation theory applied in the field of machine learning are useful for extracting structural information from the data and for using it effectively (based on the assumption that even very complex data have a certain structure).
More details about statistical and data literacy activities conducted by Abdou Moumouni University in collaboration with private sector, public administration, civile society and NGOs can be found in [18].
6.Discussion
All of the aforementioned initiatives highlighted the importance of:
1. the assessment of the understanding of statistical concepts, and then being able to tell a story that is based on these concepts; and
2. the act of data stewardship (e.g. assessing ethics, open data/information management principles, standards etc.).
In terms of building a “profile for statistical understanding”, the context of this paper aligns better with the Gall’s model for evaluating adults’ statistical literacy [19]. This model assumes that “people’s statistical literacy involves both a knowledge component (comprised of five cognitive elements: literacy skills, statistical knowledge, mathematical knowledge, context knowledge, and critical questions) and a dispositional component (comprised of two elements: critical stance, and beliefs and attitudes)”.
Moreover, in this framework, “statistical literacy not only focuses on aspects necessary to establish an awareness of data and critical thinking that must take place in order to consume data, but also focuses on the dispositional aspects of statistical literacy, a form of enquiry and action that an individual takes as a result of processing the information”.
Furthermore, it is important to mention that one of the challenges identified in the aforementioned context was about the measurement of statistical literacy and data stewardship as all of the initiatives which have been reported in this paper are not part of a specific framework but can fit under the umbrella of the NSDS. This is why the paper is articulated around the NSDS and their linkages with the skills needed for agendas like the SDGs. Unfortunately the NSDS didn’t provide metrics for measuring statistical literacy in the context of public universities nor make specific reference to data stewardship aspects related to data ethics, open data, etc. In fact all of these stewardship aspects fall under the African Charter of Statistics which didn’t take into account new terminologies and emerging concepts like open data, big data etc.
7.Conclusion
The collaboration between the public universities of Niger (particularly Abdou Moumouni University) and the national statistics institute revealed the need to increase the number of qualified human resources (particularly young generations) with advanced skills in open data, big data, data visualisation, machine learning, mathematical modeling and data-driven innovations.
Moreover, the existing statistical literacy and data crowdsourcing activities need to be validated and upscaled. Lessons learned include also the lack of experience in managing big data and in the development of mathematical methods and fast computational algorithms to analyze them.
Alternative solutions include greater collaboration and coordination with private sector, civil society and the data science community to reduce the costs associated to real-time data collection and processing and help to enhance data quality and use. Facts that require significant investment in diverse data technologies, platforms, internet and other digital technologies.
Finally, it seems that the SDGs awareness is decreasing over time among bachelor students and it is important to continue regular assessments and to conduct regular capacity needs assessments in terms of data requirements for the UN SDGs and the AU Agenda 2063 and the Niger readiness for different types of data and platforms.
Notes
6 Proposed by a team of five(5) bachelor students from the department of mathematics and computer science of AMU.
7 Proposed by a team of three(3) students from Lycée d’Excellence High School of Niamey.
10 Available at https://goo.gl/forms/WKPyGy7ZGxzcbKC63.
Acknowledgments
The author wish to thank the National Statistics Insitute for the collaboration and continuous support in promoting statistical literacy and data stewardship in public universities of Niger.
References
[1] | Institut National de la Statistique du Niger (INS). Emergence d’une classe moyenne et réduction de la pauvreté et des inégalités au Niger, 2 |
[2] | Institut National de la Statistique du Niger (INS). Emergence d’une classe moyenne et réduction de la pauvreté et des inégalités au Niger, 1 |
[3] | Institut National de la Statistique du Niger (INS). Qualité de la dépense publique et impact sur la croissance économique et l’emploi au Niger. (2015) . http://www.stat-niger.org/statistique/file/compte/Etude_Qualite_Depense.pdf. |
[4] | Institut National de la Statistique du Niger (INS). Stratégie Nationale de Développement de la Statistique (SNDS). (2007) . http://www.stat-niger.org/statistique/file/snds/SNDS_niger.pdf. |
[5] | Institut National de la Statistique du Niger (INS). Stratégie Nationale de Développement de la Statistique(SNDS). (2014) . https://paris21.org/sites/default/files/Niger%20SNDSII%202014-2018%20VER%20FINALE%2013%207%202014.pdf. |
[6] | Institut National de la Statistique du Niger (INS). Stratégie Nationale de Développement de la Statistique(SNDS). (2017) . http://www.stat-niger.org/statistique/file/Autres_Rapports/SNDSII_2017_2021.pdf. |
[7] | UN DESA Statistics Division. The Sustainable Development Goals Report (2017) . https://unstats.un.org/sdgs/report/2017/harnessing. |
[8] | Ministère du Plan. Revue Nationale Volontaire sur les Objectifs de Développement Durable au Niger. Juillet (2018) . https://sustainabledevelopment.un.org/content/documents/19886Niger_Revue_national_volontaire_ODD_Niger_Final40718.pdf. |
[9] | Ministère du Plan. Deuxieme Rapport National Volontaire sur les Objectifs de Développement Durable au Niger. Juin (2020) . https://sustainabledevelopment.un.org/content/documents/26454VNR_2020_Niger_Report_French.pdf. |
[10] | National Statistics Institute. Baseline Reference of Sustainable Development Goals (SDG) Niger. December (2017) . http://www.stat-niger.org/statistique/file/ODDNIGER/ODD_EN.pdf. |
[11] | Ben-Zvi D, Garfield JB. Statistical Literacy, Reasoning and Thinking: Goals, Definitions, and Challenges. In D. Ben-Zvi, J.B. Garfield (Eds.), The Challenge of Developing Statistical Literacy, Reasoning, and Thinking. Kluwer Publishers. (2004) , pp. 3–16. |
[12] | Dodge Y. Oxford Dictionary of Statistical Terms. Oxford University Press. (2003) . |
[13] | Rosenbaum S. Data governance and stewardship: designing data stewardship entities and advancing data access. Health Serv Res. (2010) ; 45: (5 Pt 2): 1442-1455. doi: 10.1111/j.1475-6773.2010.01140.x |
[14] | ECA, UNDP, OD, 3W foundation. The Africa Data Revolution Report. (2016) . |
[15] | Conference of European Statisticians. The next generation of statisticians and data scientists. Skills for the new generation of statisticians. Geneva: UN Economic and Social Council. (2017) . www.unece.org/fileadmin/DAM/stats/documents/ece/ces/2017/CES_23_E_Next_generation_Finland___Eurostat.pdf. |
[16] | Sidi Zakari I. Reducing the impact of household air pollution: Challenges and opportunities for achieving the Sustainable Development Goals. The Global Young Academy (GYA) yearly magazine GYA Connections, Issue 6, (2018) , pp. 11-14. https://globalyoungacademy.net/wp-content/uploads/2018/10/Connections-issue-6_print.pdf. |
[17] | Sidi Zakari I. Bridging data gap for understanding emerging issues in Niger: challenges and opportunities in the era of smart cities and smart villages. Proceedings of the 1st IEEE International Conference on Natural and Engineering Sciences for Sahel’s Sustainable Development-Impact of Big data application on society and environment. Ouagadougou, Burkina Faso, 4–6 February (2020) . https://ieeexplore.ieee.org/document/9069593. |
[18] | Sidi Zakari I. Promoting Statistics in the era of Data Science and Data Driven Innovations. Statistics Education Research Journal. (2020) ; 19: (1): 226-237. https://iaseweb.org/documents/SERJ/SERJ19(1)_Sidizakari.pdf?1583007717. |
[19] | Gal I. ((2004) ). Adults’ statistical literacy: Meanings, components, responsibilities. In D. Ben-Zvi & J. Garfield (Eds.), The challenge of developing statistical literacy, reasoning and thinking. Dordrecht, The Netherlands: Kluwer, pp. 47-78. |