Population statistics without a Census or register
Abstract
The Office for National Statistics (ONS) is exploring models for the future of population statistics. The UK does not have a population register or a set of coherent identifiers across administrative datasets held by government. The current population statistics system is underpinned by the decennial Census, which is expensive and is arguably becoming increasingly unwieldy as a source of data in a rapidly evolving society and with ever increasing demands for more timely, relevant statistics. The system is also highly reliant on a port-based survey to measure migrant flows to and from UK, with the result that the intercensal population size estimates tend to have an increasing element of bias. The ONS is therefore researching how the population statistics system can be transformed within that context. This paper outlines the current plan, focusing on some of the methodological challenges underpinning the transformation.
1.Introduction
The Office for National Statistics (ONS) is exploring models for the future of population and migration statistics using a range of new and existing data sources to meet user needs. The UK does not have a population register or a set of coherent identifiers across administrative datasets held by government, unlike many European countries such as the Netherlands. The current population statistics system is underpinned by the decennial Census, which is expensive and is arguably becoming increasingly unwieldy as a source of data in a rapidly evolving society with increasing demands for more timely, relevant statistics. The system is also highly reliant on a port-based International Passenger Survey (IPS) to measure migrant flows to and from UK, with the result that the intercensal population size estimates tend to have an increasing element of bias particularly at local levels. The ONS is therefore researching how it can transform the population statistics system within that context [1]. This paper outlines the current plans for England and Wales, focusing on some of the methodological challenges underpinning the transformation. National Records for Scotland (NRS) and Northern Ireland Statistics and Research Agency (NISRA) are separately exploring the same challenges, although all three organisations are closely collaborating.
2.Context
Population and migration statistics underpin a wide variety of other statistics (such as unemployment rates) and inform a vast range of decisions. For example, the ability to accurately forecast pensions, make decisions about local services (such as the number of school places or the provision of health services for an ageing population) and decisions about where to site new businesses. These statistics are also used to help inform public debate. It is therefore essential that these statistics are accurate and timely. Figure 1 shows a conceptual picture of how population and migration statistics sit within the wider framework of social and economic statistics, and how the decennial census interfaces with these.
Figure 1.
What is the population statistics system?
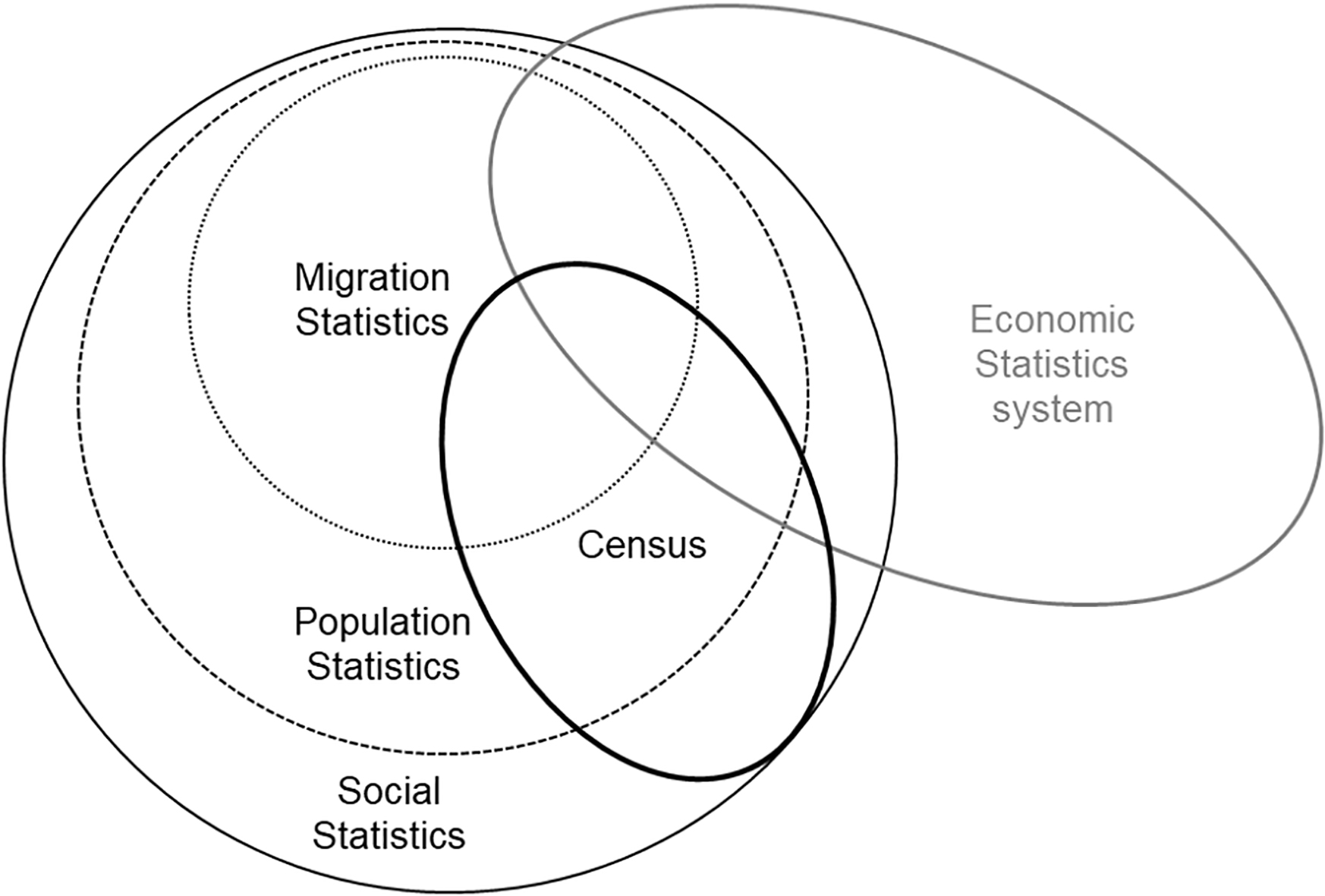
Population statistics in England and Wales are currently underpinned by a population census but there is an ambition to reinforce and ultimately replace this core with administrative data. In March 2014, the UK National Statistician recommended that the 2021 Census in England and Wales should be predominantly online, making increased use of administrative data and surveys to both enhance the statistics from the 2021 Census and improve statistics between censuses. The government’s response to this recommendation was an ambition that “censuses after 2021 will be conducted using other sources of data” [2].
ONS are progressing a programme of work to put administrative data at the core of evidence on international migration (for the UK) and population (for England and Wales). The aim is to deliver a fully transformed system for producing population and migration statistics by 2023. The programme intends to deliver continuous improvement to existing statistics along the way as more administrative data becomes available and supports the ambitions set out above. Most importantly, the outputs should be coherent and meet user needs [3]. Alongside work exploring user needs, definitions, concepts and data is the overarching statistical design and methods to derive the required statistics. This paper focuses on that aspect, outlining the statistical framework and some of the methodological components which are being explored. As the approach has developed, many challenges have emerged from the research.
3.Statistical framework and methods
Figure 2 shows the high level view of transformation plans in the lead up to and immediately after the 2021 Census. The principle is to try to produce the best possible population statistics every year using the best available sources. Research into the overall statistical design of such an approach continues, based on using administrative data either alone or in combination to form the basis of an Administrative based Population Estimate (ABPE). An important feature is the requirement for a survey to measure coverage, in this context referred to as a Population Coverage Survey (PCS). This is required because exploratory work to date shows that approximately unbiased population size estimates are not currently possible with administrative records alone [4].
Figure 2.
Proposed transformation of population statistics system in England and Wales.
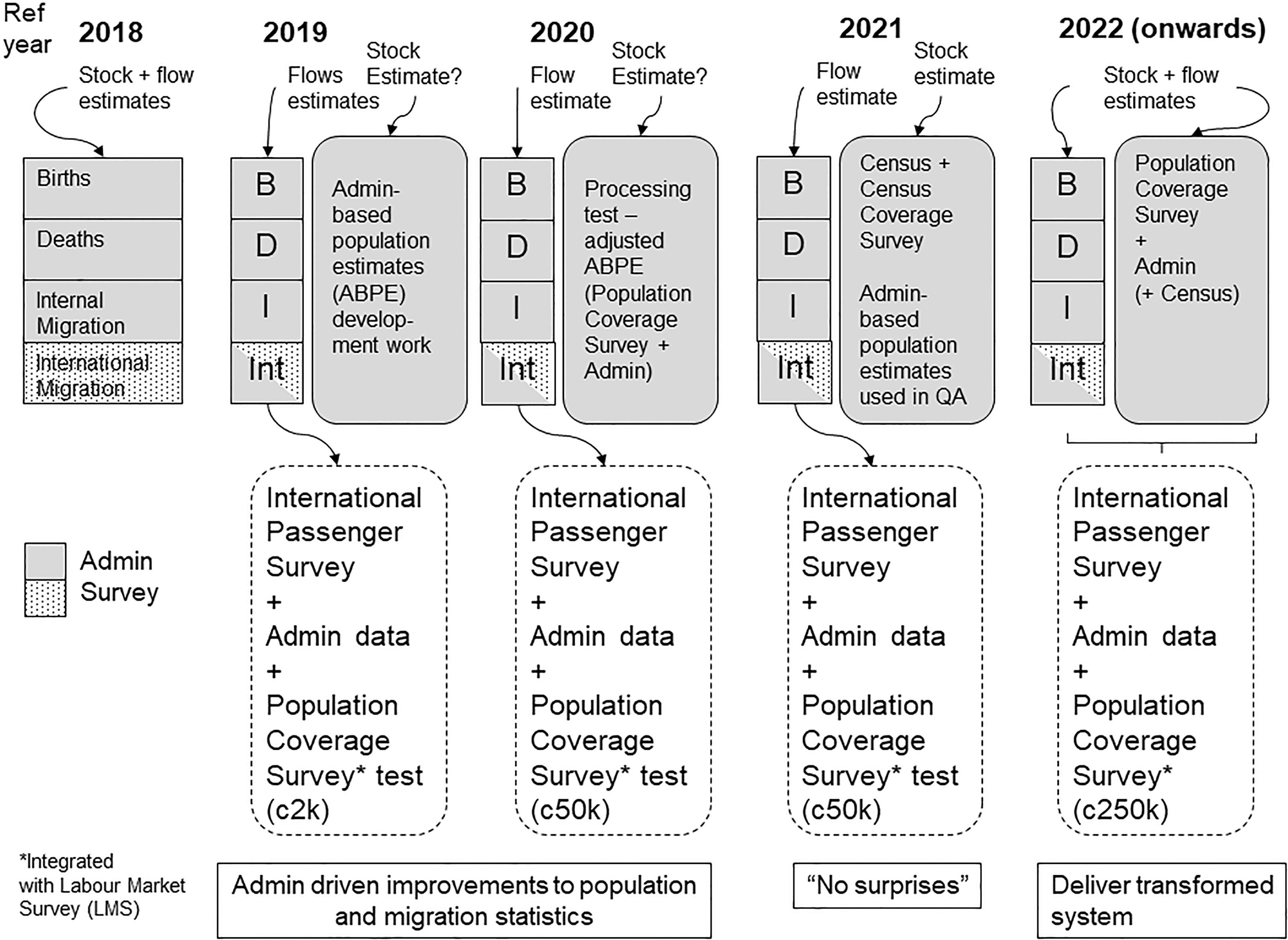
The key part of the transformation is exploring how population statistics can be produced without reliance on a census or a population register. Many other countries without a population register are considering this problem as well, including Canada, New Zealand and Ireland. The idea is to combine administrative data and existing or new surveys to estimate the quantities of interest. This is an ambitious undertaking with many challenges. This section focuses on the key methodological challenges, summarising the work undertaken on each.
These methodological challenges include:
1. Understand the administrative data
2. Linkage without a common identifier
3. Population Coverage Survey sample design
4. Estimation of population size
5. Estimation of population flows (and consistency with 4)
6. Estimation of population characteristics (and consistency with 4 and 5)
7. Measuring uncertainty
3.1Understand the administrative data
ONS research has established that population size estimates for local government areas can be derived from administrative sources alone, but the accuracy varies and is poorer at lower levels of disaggregation. The approach used is to link sources at individual level (this is described in Section 3.2) and apply rules which determine whether a record is included in the population estimate or not (and their characteristics should they differ between sources) [4].
However, this is not straightforward as some sources are derived from administrative processes that only service a sub-population, for instance the Higher Education Statistics Agency (HESA) data only covers students who register on a higher education course. In addition, individual data sources often contain over-coverage (records that do not belong to the usually resident population) and have some under-coverage (usually resident people who are not included). Over-coverage can also be caused by people (or administrative data holders) not updating their information in a timely manner when a situation changes, e.g. death, emigration or a change of address. For instance, there is no obligation to de-register from the National Health Service (NHS) Patient Register (PR) when emigrating. These administrative data lags can result in both under-coverage and over-coverage. On an individual source, records can be removed if there is an indication that they may not be present, for instance, an ‘FP69’ is a flag given to a patient on the PR when there is doubt about the patient registration. It gives a local doctors practice six months to validate the registration.
These records can be removed from the population estimate based on the data held on a single source. Linking multiple data sources is another way to identify potential over-coverage and rules can then be applied to include records in or out of the population based on the linkage outcomes and the likelihood of recent interactions with the individual data source. The first attempt at this kind of rule was only including records that could be found on both broad coverage data sources available to ONS:
• PR data, which contains a list of all patients who are registered by the NHS in England and Wales
• The Department for Work and Pensions Customer Information System (CIS) data, which contains a list of people who have a National Insurance Number.
Having decided to include a record into the population it may not be obvious at which location to include them if the address on the data sources differ. Rules can be determined that account for this such as:
Figure 3.
Percentage difference between 2011 Census and CIS, PR and Linkage-based ABPE by single year of age, males.
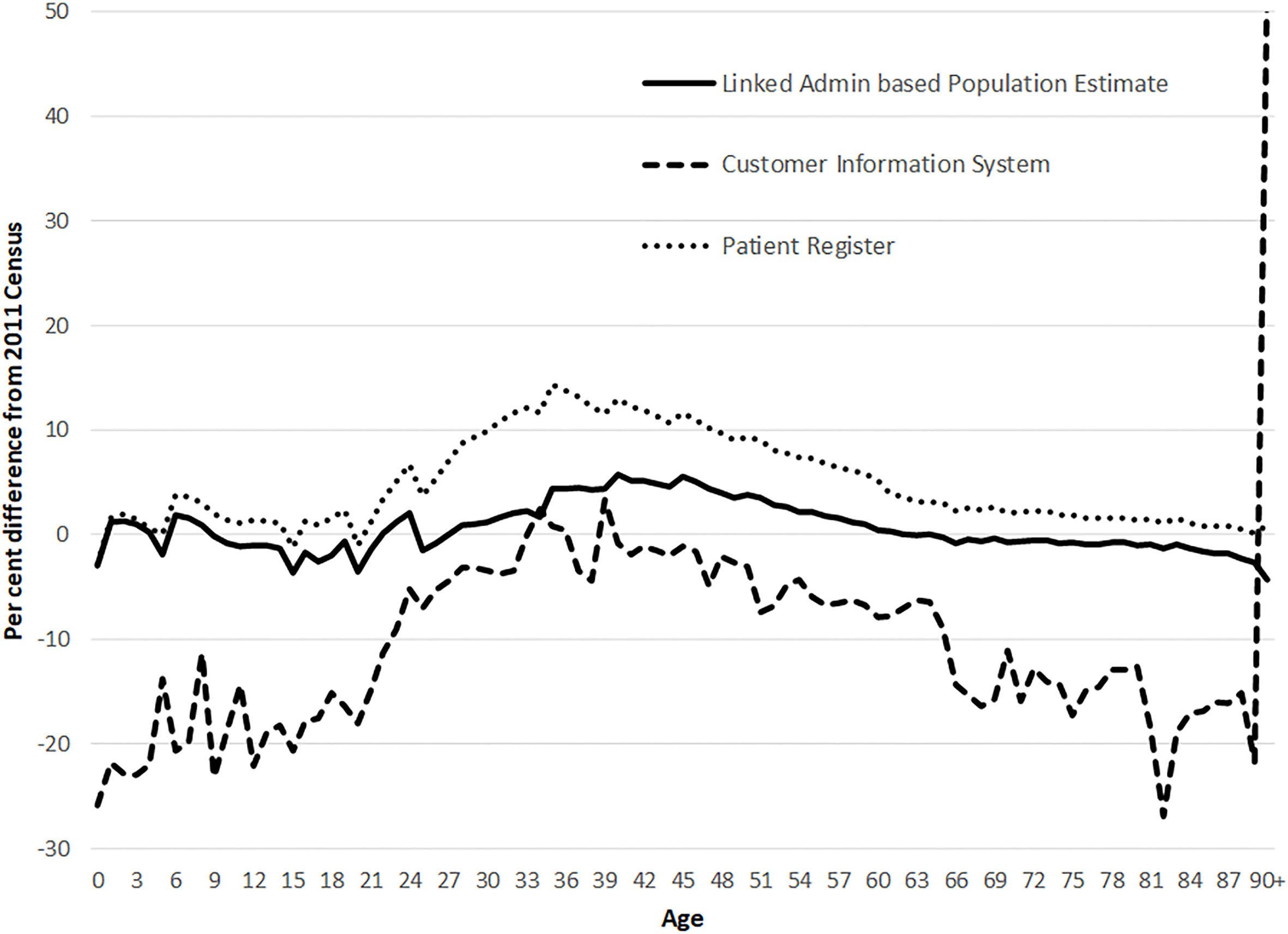
• Remove the record from the population
• Split the record and assign a weight (e.g. half) to represent the person in both locations
• Choose which source to believe is the most likely to be up to date based on the characteristics of the individual or the administrative variables (e.g. last update).
The first of these increases under-coverage. The second results in a weighted database which balances under-coverage and over-coverage, but the impact will not be uniform as particular area types (e.g. university locations) might have large clusters of these cases. The third approach, which could also result in weights, can also be complemented by using additional data sources where the individual also appears. For example, HESA data or English and Welsh School Census data, which contains a list of all pupils registered at a state school in England and Wales, are believed to have more up to date location information due to the frequency of updates. Under this assumption, these sources can be used to infer geographical location and to include additional records which are highly likely to indicate usual residence. This type of approach produces reasonable population estimates at national and local authority level but at lower levels of disaggregation, such as age and sex, biases due to coverage and linkage errors are significant [4]. Figure 3 shows the percentage difference between the 2011 Census and two key administrative sources, the PR and the CIS, as well as the result of using a linkage approach to produce a national ABPE.
Figure 4.
Percentage difference between 2011 Census and Activity-based and Linkage-based estimate by single year of age, males.
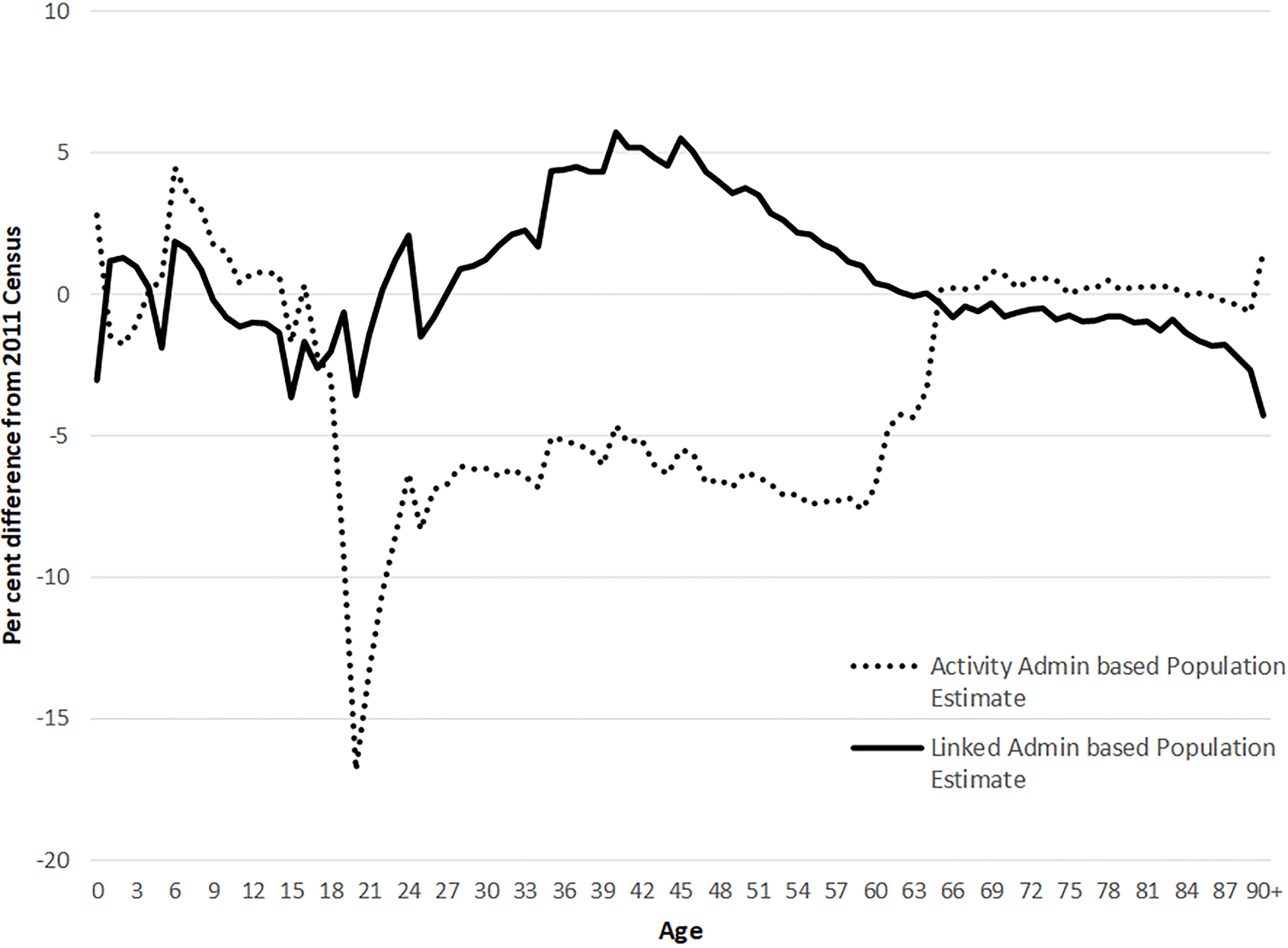
An alternative approach is to remove the over-coverage. Under-coverage is less of a problem as it is a familiar issue that census estimation tackles through using a second source, such as a survey. One approach to remove over-coverage is to use datasets that contain ‘activity’ information such as having paid tax or received benefits within a time period, for example within 12 months of the reference date of interest. Countries like Germany and Estonia, who use administrative data to compile census-type statistics, use this approach. This was prototyped using two basic principles:
• all records included should have a sign of activity within the 12 months prior to the reference date, or appear in the same address and have a relationship with an active person
• activity and appearance on a single data source is sufficient for inclusion, with data linkage only necessary to deduplicate records that appear on multiple sources.
This approach can use different data sources to best represent different population groups. Figure 4 shows the percentage difference between the 2011 Census and the two administrative based population estimates – one using a linkage approach and the other utilising activity data described above. This shows that the activity-based approach helped to tackle the specific problem of a large over-coverage of working age males in the estimates which only use linkage status. This is likely due to working age males leaving the country but not de-registering from services, and thus the activity data helps to remove them. However, this activity-based set of estimates still shows over-coverage at smaller geographies, and thus is still an issue that needs further work, and this reinforces the need for a PCS and estimation method to adjust for coverage errors [4].
3.2Linkage without a common identifier
Administrative datasets in England and Wales do not have a common unique identifier. Instead, data collected consistently across sources such as name, date of birth and locational information are used for linkage. Although these variables exist on the sources, they may be collected in different ways for different purposes and may contain discrepancies. This is a well-known problem, with many deterministic and probabilistic algorithms existing for achieving linkage [5]. Where the data is available, these algorithms can be used to link data with good accuracy. However, due to public perception concerns from both ONS and data suppliers, a decision was taken in 2013 to anonymise administrative data prior to linkage to ensure that high levels of anonymity and privacy are maintained [6]. This used a one-way encryption technique to encode the administrative data. As a result, all existing ‘fuzzy’ or probabilistic algorithms would not work, as the encryption removes any similarity between two similar strings. However, ONS was permitted to create match-keys by concatenating different parts of the identifiers in a string that can be used for automatic deterministic matching. The intention was to allow for certain values not to need to match exactly while maintaining high confidence that the matches made are true positives. For example, a match-key can be constructed from the initials of an individual’s forename and surname, combined with their date of birth, sex and postcode district. The resulting string is then encrypted and can be used to link records between datasets. A match would only be made where the match-key string is unique on both datasets. The approach is to construct a series of match-keys, each designed to resolve a type of inconsistency often occurring between records belonging to the same individual. The match-keys are used as matching fields in a stepwise linkage process, each forming a separate deterministic ‘match pass’ [7]. Samples of clerically checked matched pairs estimated a false positive (matches made that are not true matches) rate of around 1 percent and a false negative (missed matches) rate of 2.5 percent. These errors contribute to the biases seen in the estimates from linked administrative sources in Figs 3 and 4, as they can look like over-coverage and under-coverage respectively.
Data linkage generally comes down to a decision between searching for these extra good matches by relaxing how precise one wants the data to match, versus relaxing the precision too much and starting to include incorrect matches (false positives). More recently, ONS has been working closely with data suppliers in migrating to a new ONS secure data environment. This environment enables unencrypted identifiers to be used for data linkage, while maintaining security of the data as an ONS priority by separating person identifiers (names, dates of birth and addresses) from information about their characteristics. Record linkage can then use standard techniques, allowing improved linkage quality, while still adhering to the principle of not holding identifiable information in one place for longer than required [1]. This process is still underway, with work to determine how much improvement this makes still to be completed.
3.3Population coverage survey sample design
It was recognised early on that in order to produce unbiased population size estimates, a coverage survey of some sort would be required. As the administrative data research progressed as described in Section 3.1, over-coverage in the sources was highlighted as a major obstacle. Dependent interviewing, where a sample of administrative records are drawn and traced in the field, is in theory the best way of estimating over-coverage. However, due to ethical concerns, only dependent sampling – disproportionately sampling from areas with known coverage error but not using identifiable data in the field – is being considered. The same approach can be used to estimate under-coverage as it is like the methodology for measuring census under-enumeration. Therefore, Census Coverage Survey (CCS) sample design principles can be used (for a complete description see [8]).
A high-quality frame of addresses is being used for the 2021 Census, which will result in a known quality frame after the event. This can be used as an address sampling frame for subsequent surveys. The survey operation itself would be like the CCS. The questionnaire should be short, collecting basic demographic information from everyone at the address plus other variables for linkage or measurement of administrative data coverage. However, it need not be a primarily face-to-face survey and could use self-response modes. It is anticipated that the survey would have to achieve a respondent sample size similar to the CCS of 300,000 addresses (around 1 percent of the resident population), assuming it is required to obtain population size estimates for all local authorities with a reasonable degree of precision. However, the PCS would be a voluntary survey which would achieve a response rate of around 60 percent. The impact on the quality of population size estimates requires further investigation as described in Section 3.4. Consequently, the sample size required is likely to be 500,000 addresses to provide the respondent sample size required.
The sample design problem is to measure each coverage component with acceptable precision through stratification and allocation using proxy measures of coverage error. Initial design work has focused on obtaining national level population estimates, including:
• Assuming coverage measurement of the linked Administrative Based Population Estimate (ABPE) stock file
• Finding covariates of the under and over-coverage measures in administrative data and characterising each Output Area (OA – small areas used across the statistical system with population mean of 120 households) by its under and over-coverage rate
• Using the covariates to build a stratification index at OA level
• Simulating sample sizes necessary to achieve confidence intervals of given size around national population estimates.
The stratification index was constructed by comparing the 2011 based ABPE to 2011 Census at record level and characterising each OA by its under and over-coverage rate. These were used as outcome variables when attempting to find covariates. There was a poor correlation between over-coverage and under-coverage rates and so separate covariates had to be found for each measure. Research focused on finding variables within the administrative datasets which have high coverage of the population, are expected to be available in the future and which are regularly updated. For under-coverage, a form of principle component regression modelling found that the best covariates comprised a linear combination of variables that measured new international migration to an area, ethnicity and a measure of administrative records which do not pass the rules/evidential threshold for inclusion in the ABPE. For over-coverage, a standard linear regression indicated that this was best predicted by a derived variable which is a proxy for the levels of migration into and out of an area. This compares people living at addresses in consecutive years’ ABPEs and created a standardised measure of moves into and out of all addresses irrespective of household size.
Based on the CCS design principles and some simulation, the proposed sample design is a stratified two-stage cluster design, with OAs as the first stage primary sampling units (PSUs) and addresses as the secondary sampling units (SSUs). The OA level stratification was formed using Dalenius-Hodges minimum variance stratification [9] for both under-coverage and over-coverage, resulting in six groups for each. However, the survey can collect information on both types of coverage from every address sampled, and therefore to reduce sample size the initial sample allocation uses the over-coverage stratification, and then checks the sample size allocation for the under-coverage strata. Additional sample is added into any small strata to ensure the final sample sizes will achieve the precision required for both over and under-coverage.
In order to build up to a full survey by 2023, options for designing a smaller scale survey to start in 2020 were explored. Simulations show that to achieve a 95 percent confidence interval width of 1 percent around a national population estimate would require an issued sample size of approx. 50,000 households, assuming a 50 percent response rate. Some initial tests of PCS questions, operations and data linkage were performed between 2017 and 2019 and have suggested response rates of 50–60 percent.
For the purposes of efficiency and cost saving, the intention in the longer term is to integrate the survey with the Labour Market Survey, the PCS acting as a short first wave with a sub-sample used for subsequent waves which provide labour market information. The PCS survey sample will likely be issued on a quarterly basis across each reference year for field efficiency. A rolling reference date will mean more complex estimation procedures which have not yet been fully investigated. The first test using this survey design is planned to start mid-2020. Results of the test will inform future survey design and the scaling of the integrated survey to enable, at minimum, Local Authority level population estimates with acceptable confidence intervals.
3.4Estimation of population size
From a methodological perspective, this is the largest challenge. The aim is to estimate population size by age-sex and local authority with precision levels that are close to those obtained by a census, and to maintain this level of quality each year. ONS has extensive experience of dealing with under-coverage (in censuses), using a focused coverage survey and capture-recapture techniques [10]. However, the challenge with administrative data are the presence of over-coverage and linkage errors, which cause bias in capture-recapture estimators. Essentially there are two strategies:
(a) Use strong inclusion rules to compile administrative records to eliminate over-coverage, and focus methods to measure under-coverage. The risk is that under-coverage becomes too large, reducing precision;
(b) Use mild inclusion rules when compiling administrative records, and then attempt to measure both under-coverage and over-coverage simultaneously. This should minimise under-coverage, but it risks high over-coverage and the feasibility of estimating both biases.
ONS is currently exploring the approaches for estimating population size under these options. The estimators under consideration are all essentially forms of capture-recapture, albeit with a different set of assumptions for each. Under option (a), a classical dual-system estimator could work well although it would be reliant on the inclusion rules’ ability to remove over-coverage from the administrative data. This essentially depends on having high quality ‘signs of life’ information as explored in Section 3.1. Alternatives under option (b) include a weighting class approach [11] which is insensitive to over-coverage and person-level linkage error, or a Bayesian framework currently being developed by Statistics New Zealand [12]. They are developing a Bayesian hierarchical model, where the observed data comes from administrative data linked to a coverage survey. This model seeks to infer the “true” location in a population-administrative data union, allowing for both under and over-coverage. The key input is the use of prior information that provides reasonable bounds on the total population size N, the capture probabilities for the survey and the administrative data coverage probabilities.
Both strategies have challenges that would need addressing. For instance, the standard DSE based approach is still likely to require some over-coverage assessment to provide protection against bias, perhaps using the same method used in the Census [13]. But how would this affect the variance? Weighting-class and the Bayesian approach both assume the survey has zero within-household non-response, so would require something to mitigate against this.
A simulation framework is being developed that would allow comparisons across methods for different scenarios, evaluating levels of bias and variance and how robust each option is against failure of its assumptions. This will help guide whether strategy (a) or (b) is preferred, together with the ongoing exploration of the administrative data. The work is drawing on international experience and developments in other National Statistical Institutes who are also moving towards an administrative based system. For example, the Central Statistics Office in Ireland are exploring trimmed Dual System Estimation which further mitigates against over-coverage [14].
3.5Estimation of population flows and characteristics
Population size estimation is not the only estimation challenge. Statistics on international migration flows and ethnicity are required by users. Most of these are underpinned by the census providing point-in-time small area detail and ongoing social surveys providing timely estimates nationally, but rarely sub-nationally.
For estimating flows, ONS are currently considering all available sources to fully understand international migration and determine the best way to integrate survey and administrative data [3]. The estimation of flows will need to be considered alongside estimates of the population size, as they need to be coherent over time, and are important components for population projections. Methods for calibration based on quality will be required, but this research has not started. Current efforts are focused on understanding the quality of new administrative sources that can feed into the future transformed system.
For estimating characteristics, ONS are exploring whether the 2021 Census can be rolled forward by integrating administrative, survey and other data to construct a modelled database, based on a concept of fractional counting. The idea is to treat a person’s location (and perhaps other characteristics) as probabilistic over time; these probabilities will change dependent on the signals across various data sources. Small area estimates can be derived by summing the probabilities across the database. The estimation of the fractional counters might use methodologies such as incremental learning algorithms [15]. The key question is whether such modelled outputs would have lower bias than that which accumulates in census statistics due to population change. This research will also provide hints as to whether large scale data collections might be required in the future to benchmark or calibrate such outputs.
3.6Measuring uncertainty
In order to assess the different options and how all of these methods come together to produce statistics, measures of uncertainty will need to be derived. Where a sampling process is involved, this is relatively straightforward using established techniques. However, as the transformed statistics are likely to involve multiple sources which are not samples then new methods are needed to obtain accurate representations of the levels of error in the resulting statistics. For example, work has already been carried out to estimate quality for mid-year population estimates which use a combination of sources [16]. Further work is required to explore whether that framework can be extended, or whether additional replication or model-based methods can be developed. However, this challenge must not be underestimated given the relative lack of information about the data generating mechanisms for many of the administrative sources being explored, and how error can propagate through a complex linkage and estimation process.
4.Conclusions
The methodological challenges of transforming population statistics without a census or population register should not be underestimated. ONS is devoting significant resources to research in this area. This paper only touches the surface of all possibilities, and those which are being addressed. For instance, other methodological challenges not covered here include longitudinal data linkage, disclosure control, demographic analysis, imputation and the output definitions. Lastly, there are many practical issues to explore and resolve including timing of administrative data access, processing platforms and the implementation of a large population coverage survey. However, the research has shown that there is value in the administrative data, and whilst replicating a full set of high-quality census-type statistics may not be achievable in the short term, there are opportunities to produce statistics with improved timeliness and reduced cost and burden. There are methodological challenges, but the rewards are potentially significant.
References
[1] | Office for National Statistics. Annual assessment of ONS’s progress on the Administrative Data Census: July 2018 [cited 2019 Oct 9]. Available from: www.ons.gov.uk/census/censustransformationprogramme/administrativedatacensusproject/administrativedatacensusannualassessments/annualassessmentofonssprogressontheadministrativedatacensusjuly2018. |
[2] | Office for National Statistics. The census and future provision of population statistics in England and Wales: Recommendation from the National Statistician and Chief Executive of the UK Statistics Authority, and the government’s response, March 2014 [cited 2019 Oct 9]. Available from: www.ons.gov.uk/census/censustransformationprogramme/beyond2011censustransformationprogramme/thecensusandfutureprovisionofpopulationstatisticsinenglandandwalesrecommendationfromthenationalstatisticianandchiefexecutiveoftheukstatisticsauthorityandthegovernmentsresponse. |
[3] | Office for National Statistics. Transformation of the population and migration statistics system: an overview [cited 2019 Oct 9]. Available from: www.ons.gov.uk/peoplepopulationandcommunity/populationandmigration/internationalmigration/articles/transformationofthepopulationandmigrationstatisticssystemoverview/2019-06-21. |
[4] | Office for National Statistics. Developing our approach for producing admin-based population estimates, England and Wales: 2011 and 2016 [cited 2019 Oct 9]. Available from: www.ons.gov.uk/peoplepeoplexlationandcommunity/populationandmigration/populationestimates/articles/developingourapproachforproducingadminbasedpopulationestimatesenglandandwales2011and2016/2019-06-21. |
[5] | Harron K, Goldstein H, Dibben C, editors. Methodological Developments in Data Linkage. First edition. Chichester: Wiley; (2016) . |
[6] | Office for National Statistics. Beyond 2011: Safeguarding Data for Research: Our Policy [cited 2019 Oct 9]. Available from: https://webarchive.nationalarchives.gov.uk/20180903191659/https://www.ons.gov.uk//ons/about-ons/who-ons-are/programmes-and-projects/beyond-2011/reports-and-publications/beyond-2011-safeguarding-data-for-research-our-policy–m10-.pdf. |
[7] | Abbott O, Jones P, Ralphs M. Large-scale linkage for total populations in official statistics. In: Harron K, Goldstein H, Dibben C, editors. Methodological Developments in Data Linkage. First edition. Chichester: Wiley; (2016) . pp. 170-200. |
[8] | Brown J, Abbott O, Smith PA. Design of the 2001 and 2011 census coverage surveys for England and Wales. Journal of the Royal Statistical Society Series A. (2011) ; 174: : 881-906. |
[9] | Dalenius T, Hodges JL. Minimum Variance Stratification. Journal of the American Statistical Association. (1959) ; 54: : 88-101. |
[10] | Office for National Statistics. Coverage assessment and adjustment evaluation. [cited 2019 Oct 9]. Available from: www.ons.gov.uk/ons/guide-method/census/2011/how-our-census-works/how-did-we-do-in-2011-/evaluation-coverage-assessment-and-adjustment.pdf. |
[11] | Office for National Statistics. Coverage-adjusted administrative based population estimates for England and Wales 2011. [cited 2019 Oct 10]. Available from: www.ons.gov.uk/census/censustransformationprogramme/administrativedatacensusproject/methodology/researchoutputscoverageadjustedadministrativedatapopulationestimatesforenglandandwales2011. |
[12] | Bryant JR, Graham P. A Bayesian Approach to Population Estimation with Administrative Data. Journal of Official Statistics. 31: (3): 475-487. |
[13] | Office for National Statistics. Overcount estimation and adjustment [cited 2019 Oct 10]. Available from: www.ons.gov.uk/ons/guide-method/census/2011/census-data/2011-census-data/2011-firstrelease/first-release–quality-assurance-and-methodology-papers/overcount-estimation-andadjustment.pdf. |
[14] | Dunne J. The Irish PECADO project: population estimates compiled from administrative data only [cited 2019 Oct 10]. Available from: www.cso.ie/en/media/csoie/newsevents/documents/administrativedataseminar/7thadministrativedataseminar/Methodology_Report.pdf. |
[15] | Gepperth A, Hammer B. Incremental learning algorithms and applications. Proceedings of the European Symposium on Artificial Neural Networks, Computational Intelligence and Machine Learning, Bruges, (2016) . |
[16] | Office for National Statistics. Methodology for measuring uncertainty in ONS local authority mid-year population estimates: 2012 to 2016. [cited 2019 Oct 10]. Available from: www.ons.gov.uk/methodology/methodologicalpublications/generalmethodology/onsworkingpaperseries/methodologyformeasuringuncertaintyinonslocalauthoritymidyearpopulationestimates2012to2015. |