OBO Foundry food ontology interconnectivity
Abstract
Since its creation in 2016, the FoodOn food ontology has become an interconnected partner in various academic and government projects that span agricultural and public health domains. This paper examines recent data interoperability capabilities arising from food-related ontologies belonging to, or compatible with, the encyclopedic Open Biological and Biomedical Ontology Foundry (OBO) ontology platform, and how research organizations and industry might utilize them for their own projects or for data exchange. Projects are seeking standardized vocabulary across many food supply activities ranging from agricultural production, harvesting, preparation, food processing, marketing, distribution and consumption, as well as more indirect health, economic, food security and sustainability analysis and reporting tools. To satisfy this demand for controlled vocabulary requires establishing domain specific ontologies whose curators coordinate closely to produce recommended patterns for food system vocabulary.
1.Introduction
Ontologists and semantic web advocates envision a future in which stakeholders in all sectors will be able to take advantage of a harmonious federated data landscape built on the interoperability prowess of ontologies. This data interconnectivity vision has been spearheaded by life science related academic and government research agencies in an effort to describe research datasets and papers. To this end, the multilateral Open Biological and Biomedical Ontology Foundry (OBO) [1] curation consortium manages a collection of domain specific ontologies – taxonomy, chemistry, anatomy, genomics, disease, etc. – that facilitate interoperability by adhering to a set of term curation and logical relation patterns. A current review [2] of farm-to-fork data harmonization approaches reinforces the importance of ontologies such as the OBO FoodOn [3] ontology for food product description and processing, and how much more work needs to be done. While a multi-ontology framework for describing and guiding agriculture, food, diet and health was proposed in 2007 [4] around the time that OBO was launched, only in the last few years have OBO ontologies emerged to provide vocabulary and design patterns for food-related domains such as agriculture, diet and nutrition.
In this paper we provide an overview of food-related OBO ontologies and their connections to FoodOn and other core OBO ontologies, as well as their current pitfalls and future development aims. We aim to satisfy an audience that understands the basics of ontology, and wants to be aware of what the OBO foundry recently has to offer in the food domain for terminology and object properties without having to examine each of the given ontologies directly. The Appendix includes an abbreviation dictionary and URLs where more information about referenced ontologies can be found, including ontology GitHub repositories where versions can be retrieved.
1.1.The FoodOn food ontology
FoodOn is described in detail in its inaugural paper [3] and in the https://foodon.org website, so here we provide a brief introduction, followed by overviews of the other food related ontologies. Generally, we seek to show how the collective community of older and newer OBO ontology curators is supporting the harmonization effort required for cross-domain food systems analysis. FoodOn’s mandate is to describe and provide precomposed terms for generic (non-branded) food products that a food producer, food manufacturer / processor, or consumer can find in the supply chain, ranging from wild or farmed food, to processed, wholesale, retail, prepared, restaurant or home-cooked food. FoodOn now offers over 24,000 terms in its extensive food product hierarchy and description facets, such as applied cooking treatments, preservation methods, and packaging, along with another roughly 10,000 OBO ontology terms including chemistry, anatomy and food source organism (plant, animal, fungi or algae) taxonomy domains.
FoodOn entered the OBO Foundry in 2016, transforming and evolving the basic structure of LanguaL [5], a popular food composition database (FCD) vocabulary created by the U.S. Food and Drug Administration (FDA) Center for Food Safety and Applied Nutrition (CFSAN) in 1975. During FoodOn’s inception, it adopted hundreds of Environment Ontology (ENVO) [6] food terms, and it integrated with OBO’s Chemical Entities of Biological Interest (ChEBI) [7] ontology which organizes molecular entities – mainly ‘small’ chemical compounds (natural or synthetic) – pertinent to the processes of living organisms. FoodOn imports anatomical terms from the Plant Ontology (PO) [8] and UBERON multi-species anatomy ontology [9], taxonomy from NCBITaxon [10], and imports dietary and nutritional terms from the Compositional Dietary Nutrition Ontology (CDNO) [11] and Ontology for Nutritional Studies (ONS) [12]. Finally, FoodOn organises food related data items and processes under the Information Artifact Ontology (IAO) [13] and Ontology for Biomedical Investigation (OBI) [14] classes respectively, and draws mainly on existing Relation Ontology (RO) relations [15], with just a few others introduced, like “has ingredient” and “has defining ingredient”.
1.2.Food related ontology use cases
Use case examples of how FoodOn and other OBO ontologies are being used can be found in a number of standards and database projects expressed in knowledge graph or tabular formats.
Food sample composition, nutrition and bioactive analytics:
The Rockefeller Foundation supported Periodic Table of Food Initiative is coordinating data collection and mass spectrometry analysis of the nutrients and other bioactive compounds of over 1000 foods that are representative of consumption patterns around the world. Ontologies such as FoodOn and AgrO [16] are being utilized to describe the biosample context of sampled foods.
The U.S. Department of Agriculture (USDA) FoodData Central website provides FoodOn identifiers and categories for its Foundation Foods database of single-ingredient foods and their nutritional analytic data. FoodOn mapping to all single ingredient SR Legacy database foods is also underway.
WikiFCD, a wikibase database of food composition (FCD) and nutrient information mainly dedicated to standardizing and bringing online the FCD’s of developing nations, references FoodOn terms.
FoodKG [17], a knowledge graph launched in 2019 representing over 1 million recipes, is constructed with an ontology combining FoodOn, ChEBI and other resources like the USDA Nutrient Database [18], and the Im2recipe photograph-recipe matching project (http://im2recipe.csail.mit.edu/). It enables querying of recipes by ingredient, cook time, course type, and meal type. Competency questions (factoid: “How much fat is in butter?”, comparison: “Which has more fat, butter or olive oil?” and constraint: “Which dish has chicken, onion, and garlic?”) can be applied to its knowledge graph.
With the support of the FDA, the FDA Seafood Product List is included in FoodOn to expand the mapping of common language fish names to scientific taxonomy names to improve food traceability and authentication.
Foodborne disease investigation:
The FDA GenomeTrakr database [19,20] which contains over 45,000 foodborne pathogen genomic sequences and their metadata, are matched to FoodOn, National Center for Biotechnology Information (NCBI) taxon (NCBITaxon) and ENVO ontology terms using a textual-sample description-to-ontology-term matching software called LexMapr [21]. GenomeTrakr records are then submitted to the NCBI Biosample sequence repository to assist in foodborne outbreak and antimicrobial resistance research.
The Genomic Standards Consortium (GSC) of minimum information standards (MIxS) is adding a food package [22] for agriculture and industry-situated sampling metadata for pathogen and metagenomic analysis.
The draft ISO/TC 34/SC 9 standard “Microbiology of the Food Chain – Whole Genome Sequencing, Typing and Genomic Characterization of Foodborne Bacteria” makes extensive reference to FoodOn, NCBITaxon, ENVO and other ontologies for particular fields.
NCBI’s One Health Enteric Package specification for US surveillance of foodborne pathogens [23], available as part of its BioSample specification since 2022, provides data-entry spreadsheets along with guidelines to use FoodOn, ENVO and AGRO terms for selected fields. A detailed study of surface swab site metadata requirements that utilized OBO Foundry ontologies was integrated into this specification. [24]
Fig. 1.
Key food-related ontologies within the OBO sphere, and some leading organizations and consortiums that curate them. The direction of ontology term import and export is indicated by the arrows of dotted lines; solid lines convey curation participation. Each ontology has a count of terms it introduces in proportion to all its terms including ones reused from other ontologies. Data provided by https://ontobee.org/ontostat as of October 25, 2022.
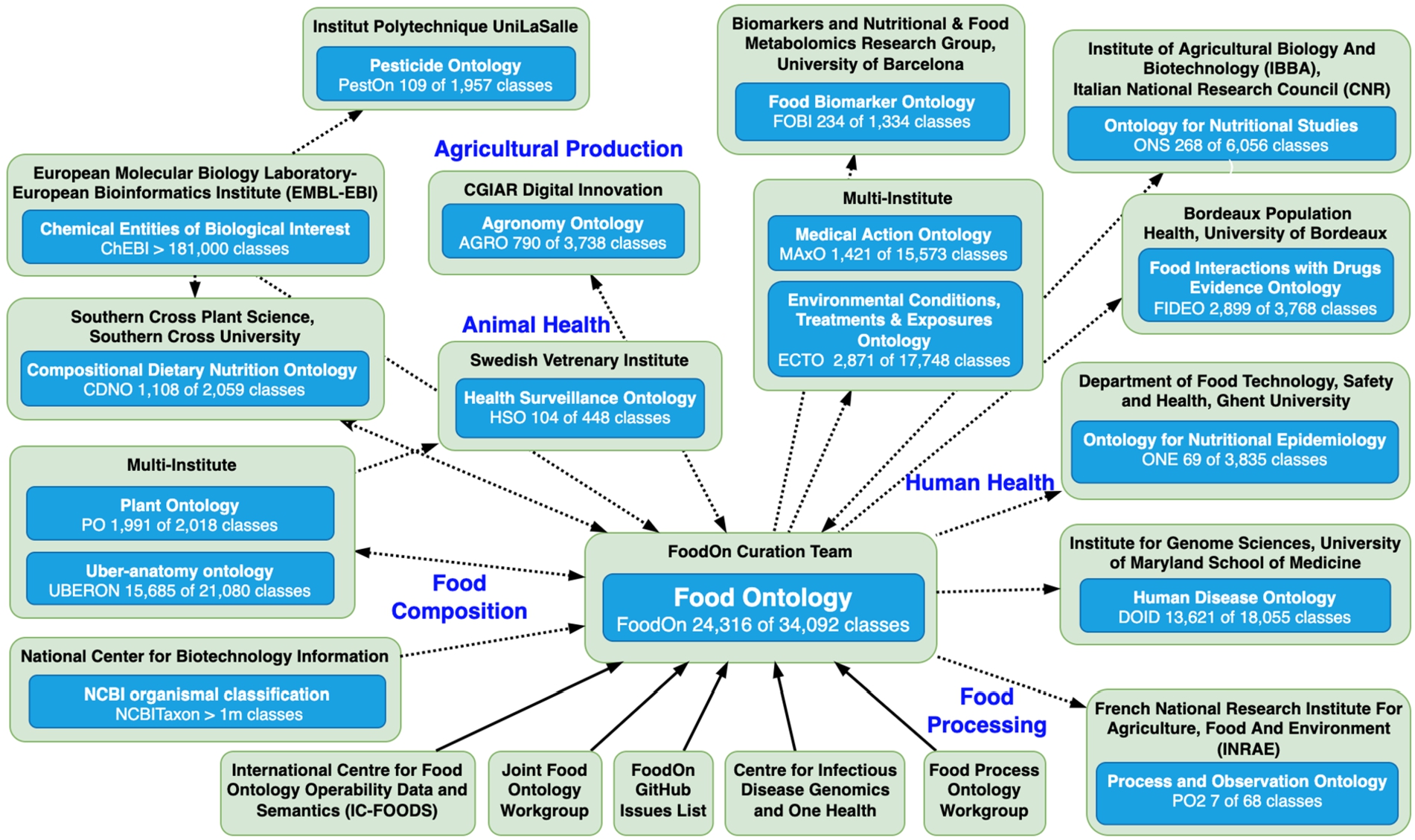
Figure 1 illustrates the emergence or expansion of food-related OBO ontologies to meet the needs of projects like these, describing food composition, agricultural production, food processing and animal and human health factors. The One Health [25] paradigm of interrelated human, animal, and environmental health that has emerged in the last decade also motivates interdisciplinary vocabulary, such as the Health Surveillance Ontology [26].
2.Methods
The co-authors of this paper led the development of most of the food-related ontologies reviewed here, and so have informed a summary description and future direction of their respective ontology. Some are newer to ontology development, and so have focused mainly on using the open-source Stanford Protégé editor [27] to curate their ontology in W3C Web Ontology Language (OWL) 2.0 format [28], with perhaps an initial round of imported terms from third party ontologies. Each author has made an effort to curate their ontology according to OBO Foundry curation principles [29]. A minimum requirement, called The Minimum Information to Reference an External Ontology Term (MIREOT) [30], is the reuse of classes and relations from other OBO ontologies to avoid the costly and confusing situation where term entities having similar or identical semantics but different identifiers exist in multiple ontologies. Our authors have often included the MIREOT import of individual terms or branches of third party ontology hierarchies, in some cases by using a tool like OntoFox [31] to generate an OWL import file. Some have merged this file directly into their main ontology, others have more advantageously kept it separately so it can be refreshed over time. Figure 1 shows how many terms are introduced by a given ontology, out of a total number filled out by imported, reused terms. Other OBO term management best practices they adhere to include:
New term requests (NTR) are handled usually via Github requests or larger bulk review and import projects.
Each accepted term gets an OBO standardized Permanent Uniform Resource Locator (PURL) [32] that resolves to a term detail page provided by an ontology search engine like Ontobee.org [33].
Terms may be marked obsolete, and may include replacement references, but are never deleted.
Along with the MIREOT principle, this functionality fulfills FAIR data principles [34] (especially the interoperability and reusability aims) insofar as any dataset annotated with a collection of OBO ontologies should have unambiguous semantic references to terms that can evolve and remain translatable over time. Generally, all OBO ontologies are asked to reuse existing relationship terms – the “data structure glue” – of object properties between entities, which are mainly found in the Relation Ontology, and a handful of data properties between an entity and some literal data value. However, some “application ontologies” are under pressure to support quick development of databases and knowledge graphs and so introduce their own relations which are often very specific to their data models and thus confound the interoperability model of shared relations that OBO espouses. OBO is increasingly excluding such ontologies from joining the foundry, and encouraging existing members to translate their more general relations to RO or to have their more specialized relations added to RO.
Ontology maintenance and build technology is not detailed in this paper, but briefly some of the summarized ontologies, such as FoodOn, employ more automated curation tools, while others are manually constructed. FoodOn and others use the “ROBOT” [35] command line tool’s “template” option to manage terms that conform to the same tabularized pattern of annotations and simple axioms. ROBOT’s “reason” command may be run to ensure OBO ontologies and statements expressed with them are consistent with shared RO logical relations, along with the Basic Formal Ontology (BFO) [36] or upcoming Core Ontology for Biology and Biomedicine (COB) [37] compatibility that many aspire to. Both ROBOT and OntoFox can be introduced into a more automated Ontology Development Kit [38] ontology build pipeline that includes ontology recompilation based on file changes, and logical and syntactic checks. OBO also now provides a suite of external quality tests via the OBO Dashboard [39].
Due to their start-up phase, a number of this paper’s ontologies are single agency/single team efforts. Eventually, an OBO ontology is expected to attract more than one user, thus encouraging other stakeholders to be identified and encouraged to join the curation team, thereby improving an ontology’s standing as a de facto or more official standard. A second approach is to have ontology curators involved in regular multilateral discussion with other ontology projects in a similar domain. To this end, the Joint Food Ontology Workgroup (JFOW) [40] was launched in the spring of 2020 as an informal methodology for term development within the food systems domain. JFOW was formed after FoodOn received batches of requests for diet and nutrition terms from a few other ontologies, but was aware that the Ontology for Nutritional Studies was under development and targeting membership in OBO, and could be an opportune niche home for the requested terms. The workgroup convenes once a month with representation from the NTR-requesting ontologies as well as USDA, FDA, and other academic and research agencies keen to help and to assess the appropriate reuse of ontologies within their operations. During its initial 4 months a corpus of over 60 diet and dietary pattern terms was discussed, reviewed, approved and then handed to ONS for implementation. A similar discussion group, the Food Process Ontology Workgroup, convened to discuss food process related ontologies and create a generic food processing model and closely related recipe and ingredient model. [41]
3.Overview of food related ontologies
Our overview begins with agriculture related ontologies and the bioactive compounds encountered there, then moves into pesticides and chemical exposures, then food processing and dietary pattern, and biomarkers arising from that, followed by diseases and medical treatments, nutritional research study annotation, and finally animal health and foodborne pathogen surveillance.
In a number of ontology overviews, we touch on the role selenium (ChEBI:27568) plays in human health [42] as an example. Selenium intake arising from its concentration in plants – a function of many agricultural factors including soil concentration, supplementation, pH, drainage and microbiome – may lead to micronutrient under or over-nutrition (of the amino acid selenocysteine and derived selenoproteins), and knock-on health effects, many of which require more research to clarify. Diseases such as Keshan disease are highly corelated to selenium deficiency stemming from diet, agricultural soil and environmental conditions [43]. Toxic selenium ingestion by both animals and humans via natural overabundance or improper use or formulation error of a nutritional supplement can lead to selenosis [44], while proper supplement use in thyroid disease therapy leads to improved clinical scores [45]. The analysis of bioactive compounds such as selenium as factors in human and animal health can now benefit from ontologies that name biosample sources – from soil, plant and animal material, human blood, and stool – as well as their contextual data – agricultural conditions and treatments, plant phenotypes, human diseases and symptoms.
3.1.AgrO, CO, TO, and PECO: Agriculture related ontologies
The Agronomy Ontology (AgrO) [16], launched in 2017 in the context of the CGIAR Platform for Big Data in Agriculture, describes agronomic management practices, implements, and variables used during crop plot experiments. It includes over 200 plant morphology traits from the Plant Trait Ontology (TO) [46], parameters identified by agronomists and crop modelers from the pre-existing Crop Ontology (CO) [46,47], and a treatment menu of 15 terms from the Plant Experimental Conditions Ontology (PECO) [48]. AgrO contains over 600 geography and geology related terms from ENVO, as well as 550 ChEBI terms and 155 Phenotype And Trait Ontology (PATO) [49] terms. Along with harvested food product references, AgrO “crop residue” terms are imported from FoodOn to support research on reuse of such materials. Continuing the selenium example, AgrO references various forms of selenium for sampling measurements, TO provides a “selenium content” biochemical plant trait; and PECO provides a “selenium nutrient exposure” treatment.
AgrO is used in the CGIAR Agronomy Field Information Management System, a software tool that enables the digital collection of agronomic trial data [50]. AgrO, ENVO and other ontologies can be used within the MIxS package standard [51] for soil biosample metadata collection [52]. In the near future, to support the Periodic Table of Food Initiative, AgrO will import FoodOn food and nutrition terms which are important to measure at the post-harvest stage. As well, AgrO term language will be adjusted to cover commercial production crop treatments. There is a future opportunity to further align TO biochemical traits and CDNO nutrient chemical concentrations.
3.2.CDNO: Compositional dietary nutrition ontology
CDNO [53], launched in 2020, provides a hierarchy of over 1,000 terms for nutritional attributes – vitamins, carbohydrates, lipids, minerals, proteins, etc. – and matching bioactive chemical concentrations from crops, livestock, and fisheries that contribute to human diet and which are referenced in precision food commodity laboratory analytics. Rather than directly mirror ChEBI’s hierarchy of molecular entities and their roles, CDNO provides a more diet-and-nutrition community friendly arrangement (Fig. 2) by essentially flattening ChEBI hierarchies like “mineral nutrient” and “protein” to selected elements, and adding CDNO groupings like “plant secondary metabolite”. Initially, CDNO, FoodOn and Plant Ontology (PO) curators worked together in a collaborative effort to establish a set of over 58 food raw materials entities. They additionally defined sources of plant samples and crop production processes or stages from which the food raw material was taken.
Fig. 2.
The top level CDNO ‘dietary chemical component” class and its subclasses, most of which are imported from ChEBI.
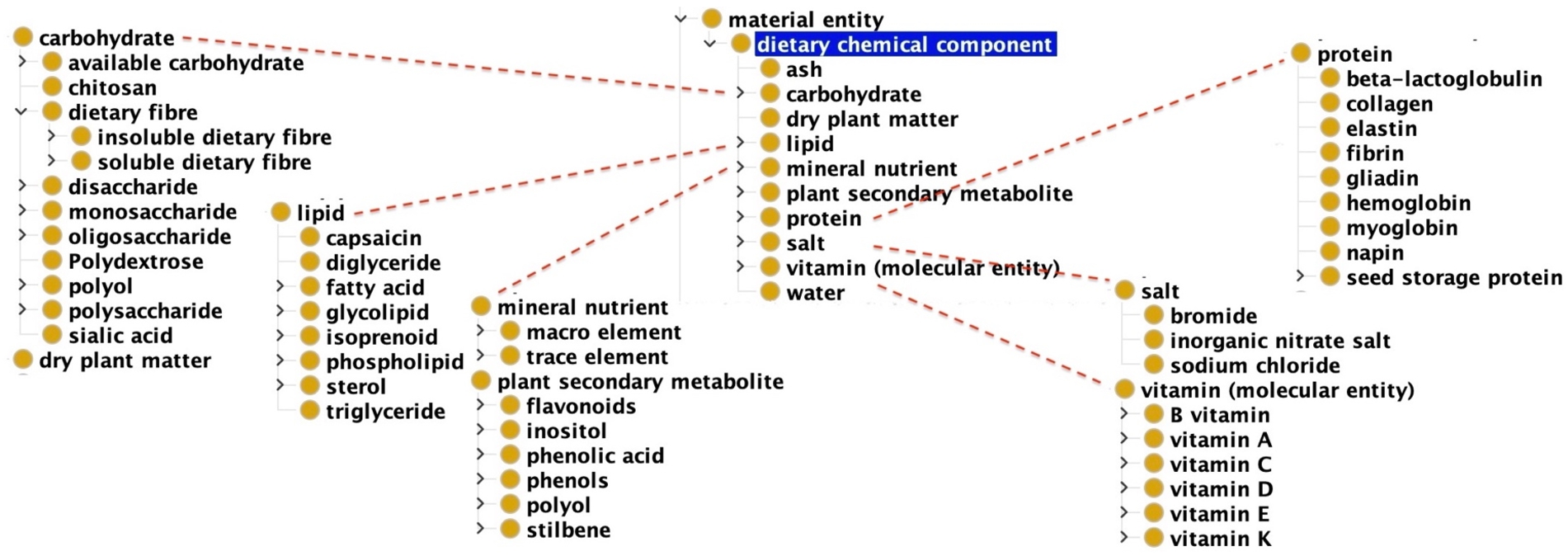
Fig. 3.
Visualization of how CDNO and FOODON classes can be brought together in a graph database statement about selenium concentration in a whole wheat kernel sample.
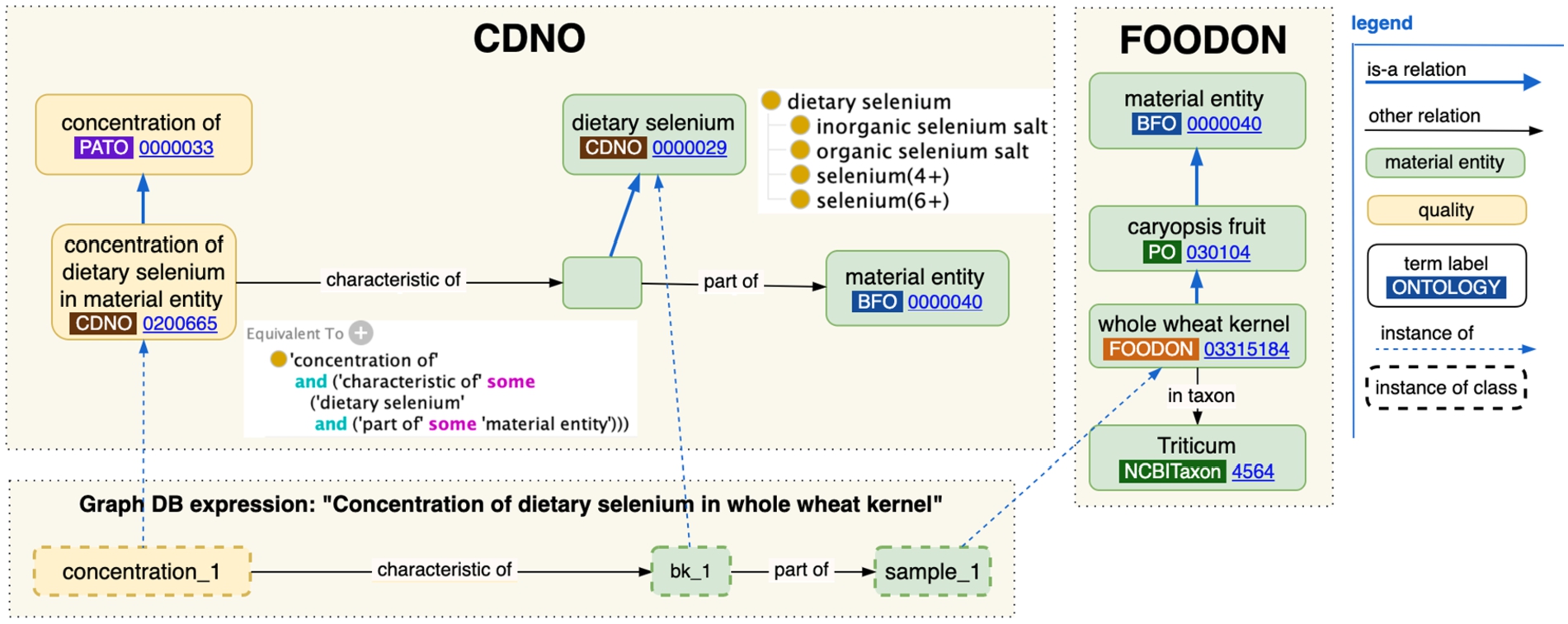
CDNO’s “nutritional component concentration” classes have a design pattern that uses PATO’s “concentration of” term that references a particular analyte in proportion to the BFO “material entity” specimen that is being measured in. A specimen can be a plant or animal species’ anatomical part, yielding a comprehensive way to measure nutritional attributes of biosamples (like seeds) throughout the food production lifecycle. Following the selenium example, Fig. 3 shows the “concentration of dietary selenium in material entity” class defines a concentration characteristic of an analyte as part of some material entity biosample. The term’s OWL logical “Equivalent To” definition is shown, as well as CDNO’s dietary selenium and its ChEBI subclasses which may be measurement targets. To express selenium concentration in a whole wheat kernel, we need to reference “whole wheat kernel” a FoodOn reference that helpfully indicates (axiomatizes) the kind of plant anatomy part it is, and its taxonomic origin. These classes can combine in a graph database data structure (aka A-Box or instance data) as shown in the “Graph DB expression” box of Fig. 3, where each dashed box is an instance of a class, with its own identifier (not shown are data properties for specifying the concentration measurement itself).
“Concentration of dietary selenium in material entity” and “whole wheat kernel” are each an example of a “precomposed term” – a term that has an equivalency axiom describing a set of relations to other things that together are the criteria for recognizing something of its kind. In databases one may also encounter a “post-composed” term, one which doesn’t have a preassigned ontology id, but which has the same searchable sentence structure as a precomposed term, to match more detailed needs of researchers, for example comparing concentration of dietary selenium in the endosperm versus germ part of a Titicum kernel. Note that for projects such as CDNO’s CropStoreDB (https://www.cropstoredb.org/) that use tabular datasets rather than graph representations, a biosample table can simply have columns for biosample identifiers, dietary component measure, FoodOn product term or PO anatomical term and taxonomy, and any other agricultural context metadata. With the addition of some column semantics, tabular views can be converted back into appropriate graph representations.
Upcoming CDNO work is focused on a hierarchy of classes for physical and functional attributes and dietary functional roles, as well as nomenclature required to describe variation in analytic measures. This will allow harmonization of the nutrient measures used for international standards such as the FAO sponsored International Network of Food Data Systems (INFOODS) [54] system used in many food composition databases, as well as the commonly used USDA nutrient codes in FoodData Central [55] (where for example selenium is listed in the “Supporting data for CSV Downloads” archive nutrient.csv table with id 317 and a microgram unit).
3.3.ECTO: The environmental conditions, treatments, and exposures ontology
ECTO [56], launched in 2016, provides language to describe experimental and environmental factors for a wide range of public health and environmental monitoring objectives, including modeling components of human disease and nutritional health, environmental toxin exposure, and alteration of biological function. It focuses on documenting precomposed experimental treatments, non-experimental exposures, and environmental conditions that may impact organisms. For modelling experimental designs, ECTO contains hundreds of “exposure to chemical” terms such as “exposure to chlorpyrifos” (a moderately toxic pesticide that has been banned since 2020 in the European Union, and since 2022 in the USA) which can be used to model human and other organism diseases and phenotypes that arise from exposure, and the underlying metabolic activity at work, as illustrated in Fig. 4. The RO relations “has exposure medium”, “has exposure stimulus”, “has exposure route” and “realized in response to” all connect processes which summarize a chain of exposure events, for example, exposure to an instance of an apple which itself has been exposed to chlorpyrifos.
Fig. 4.
Instance-level ECTO data representation of exposure to chlorpyrifos by way of food-ingestion, including the disease and phenotype associated with it.
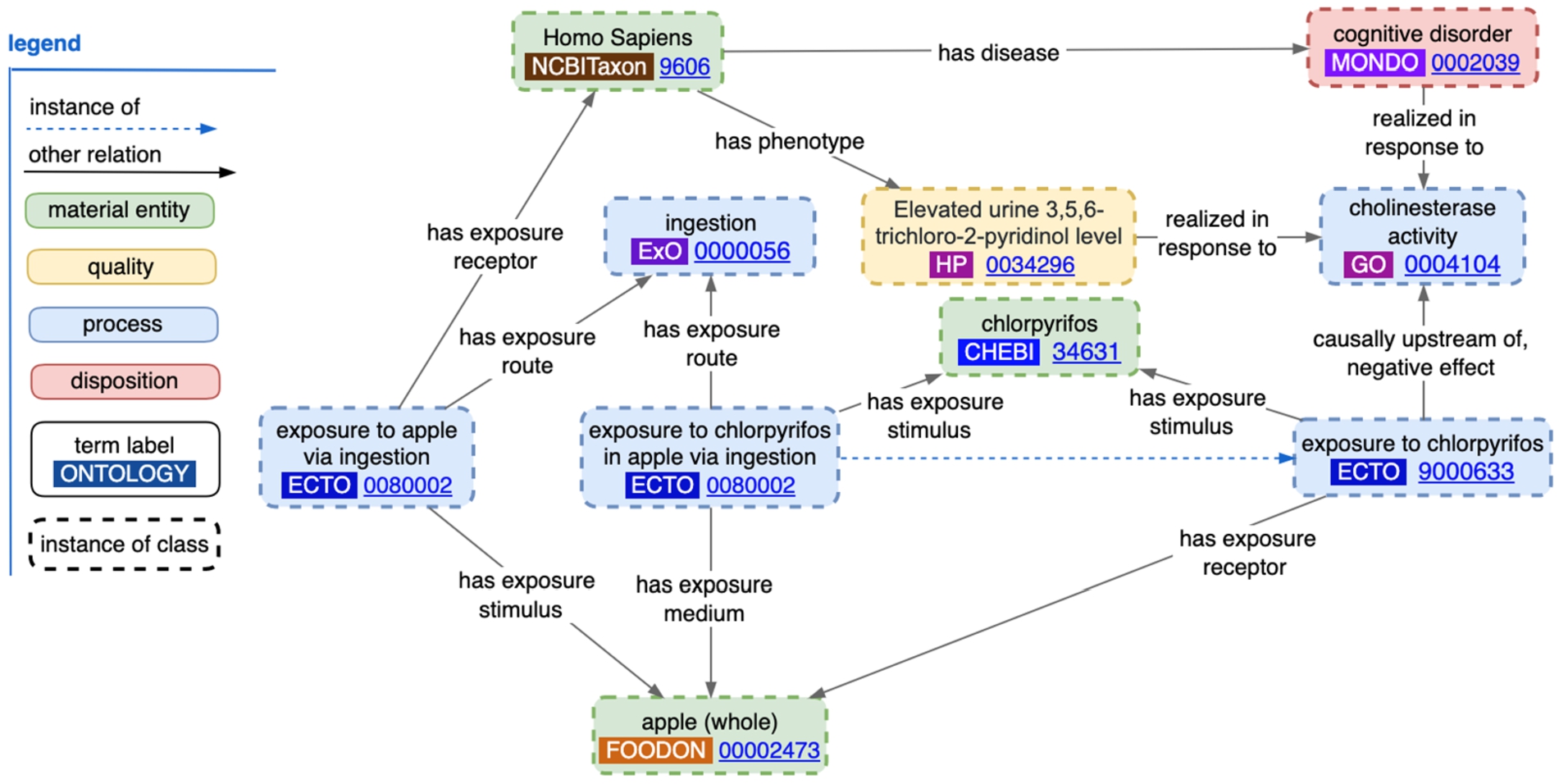
ECTO currently has over 160 food ingestion terms such as “exposure to skim milk via ingestion”, and 17 vitamin and mineral ingestion terms including an “exposure to selenium via ingestion” process. An upcoming Dead Simple OWL Design Pattern (DOSDP) [57] will integrate exposures to specific diets that refer to terms found within the Ontology for Nutritional Studies.
3.4.PESTON: The pesticide ontology
PestOn [58], launched in 2022, aims to provide an ontological framework for organizing branded pesticide product regulatory information. Regulation of pesticides (mostly chemical agents used to protect crops from disease, pests, and weeds, and improve plant growth) and their reporting requirements and other publicly-mandated information is uneven among nations and geopolitical regions, leaving uncertainty about their responsible use, and challenges in evaluating health and environmental impacts. PestOn was developed by ontologizing The Italian Ministry of Health’s pesticide register dataset, comprising over 16,000 pesticide products used over several decades. Pesticide brand names, their active ingredients (including chemical name and concentration), production company information, pesticide by chemical role (e.g. fungicide, insecticide, herbicide, plant growth regulator, adjuvant, herbicide, etc. product classes linked to ChEBI chemical role where possible), type of associated hazards (e.g. dangerous for the environment, flammable, toxic, etc.) and regulatory events (issue date, expiry, revocation) and derived authorization status are all part of the PestOn model.
More than 99% of pesticide active ingredients were successfully matched against CHeBI ontology, with the remainder either chemicals that the authors then suggested to ChEBI curators, or were organisms like fungi and bacteria which PESTON does not currently model. At launch, PestOn involves 795 active ingredients contained in 16,458 pesticide products and their 532 production companies. PestOn is divided into a core ontology that can be used for describing pesticides in general, but it also comes with import files which supply an RDF graph of all the Italian instance data for the pesticide products, companies and regulatory events. The SPARQL querying language [59] can be used for example to retrieve the current available products in each pesticide role category, as well as a summary of products containing a given active ingredient. Taking the chlorpyrifos example in the ECTO overview, PestOn has it as an active ingredient in over 190 brand name pesticides offered in the Italian market, along with issue and revocation dates, enabling one to determine compliance with the European chlorpyrifos ban.
PestOn’s initial corpus of Italian regulatory data should be expandable to other jurisdictions although different regulatory events (besides application / revocation, etc.) may need to be added. PestOn is not yet a member of OBO, and does not reference foods or crops that are applicable to pesticide application, but it could be useful in research linking branded pesticide products to exposure effects as described in ECTO.
3.5.ONS: Ontology for nutritional studies
ONS [12], first published in 2018, focuses on vocabulary for modeling nutritional studies, including diet and dietary pattern variations. A central concept in ONS is “diet” (ONS:1000001, having 58 subclasses), a datum defined as “the sum of food consumed by a person or other organism”. Diet is currently distinguished from “dietary pattern” (ONS:0000094, having 34 subclasses), defined as “the quantity, proportion, variety and combination of different foods and drinks consumed in meals, and the frequency with which they are habitually consumed”. Dietary pattern was intended to represent a dataset typically resulting from assays in the context of nutritional epidemiology (for example, an ONS “Food Frequency Questionnaire”), containing a specification of foods consumed and, as a result, denoting the type of diet to which a subject has adhered. Curation and development revolving around the initial diet concept in ONS has greatly benefited from the collaboration with the Joint Food Ontology Workgroup. Thanks to this interaction, multiple different subclasses of the diet (and related dietary pattern) were defined.
Various flavours of diet and related dietary patterns currently have axioms that include or exclude FoodOn products by way of an RO “eats” relation. As an example, the “vegan diet” (ONS:1000021) and the “vegan dietary pattern” (ONS:2000021) are axiomatized as eating vegetables and excluding the consumption of animal products; however, these axioms need to be revised as “eats” can only connect from an organism to a relevant food, rather than from diet, which is currently a kind of IAO Information Content Entity (which is logically disjoint from material entities like food). Additionally, the distinction between diet and dietary pattern needs to be re-examined. While diet as a descriptive term (the ONS “descriptive diet” class) details what an organism has actually eaten, it appears that the remaining diet terms could be considered eating patterns, and thus be consolidated under dietary pattern.
3.6.PO2: Process and observation ontology
Fig. 5.
PO2 uses similar classes for both production line actuation and observation.
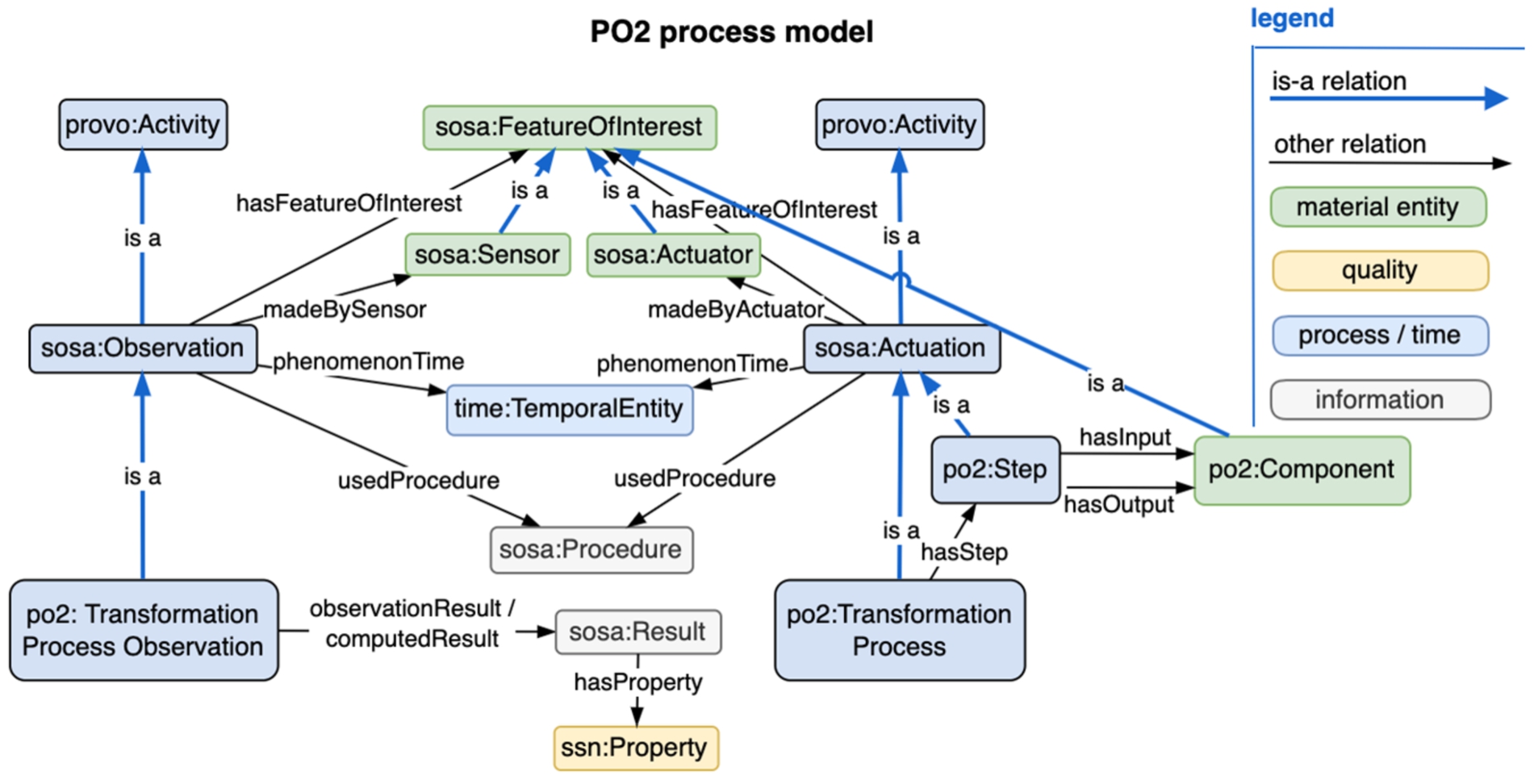
PO2 [60] details processing steps, characteristics and sampling regimes of foods during their industrial manufacturing process, as well as their food formulation (Fig. 5). The ontology contains a small core layer of 68 classes dedicated to the generic modeling of both transformation and characterization processes, while domain specific sub-ontologies specialize the PO2 core model for different projects. PO2 can represent a food transformation process described by a set of experimental observations available at different scales and evolving in time as a production process composed of unit operations. PO2 is based partly on the Sensor, Observation, Sample, and Actuator (SOSA) ontology [61], but also has BFO, OWL-Time and IAO classes. It has been implemented in database-driven software that covers dairy, meat, and biorefinery production, to represent the unique characteristics of foods during their manufacturing process. The PO2 team participates in the Food Process Ontology Workgroup which is helping to build FoodOn’s food transformation process branch.
Future directions for PO2 include the development of a PO2 specialization called TransformON [62] which will cover various bioresource transformation processes that produce food and biobased products. It includes three component subclasses, two for food and feed taken directly from FoodEx 2, as well as a branch of non-food substances, including organic wastes or residues (e.g. wastewater) and bio-based products. A future objective is to align the PO2 component branch with FoodOn, and in this way map between FoodEx 2 and FoodOn. Processing equipment (actuators) and measuring instruments (sensors), as well as branches for analytical procedures and measured properties will be mapped to or sourced from OBO ontologies. PO2, available at https://doi.org/10.15454/XSVVBW, is not registered with OBO as it is tightly integrated with several other non-OBO ontologies, but it has collaborated on a comparison of it and the OBO process model [41].
3.7.FOBI: The food-biomarker ontology
FOBI [63], launched in 2020, is aimed at describing the relationships between a food and its metabolome, the collection of all metabolites in the body directly derived from the digestion and biotransformation of foods and their constituents. These biomarkers are often measured in stool or urine samples. FOBI, currently with a total of 1197 terms, is composed of two interconnected branches: a food branch consisting of over 300 FoodOn raw single-ingredient and multi-component foods; and a food intake “Biomarkers” branch of about 968 chemicals (Figure 6), many from ChEBI but with some FOBI specific terms. Connections between the two are made with FOBI’s “BiomarkerOf” object property, with 590 connections made. Figure 6 illustrates two biomarkers that can be left in humans as a result of consuming apple directly, or in some processed form such as a baked apple pie. (Note that the FOBI paper [63] refers to its own “Food Ontology […] created on the basis of dietary data obtained from self-reported surveys for dietary assessment […]”, but this references FOBI’s own branch of food items that was later mainly converted to FoodOn terms).
Fig. 6.
Example class-level FOBI linkage between FoodOn “apple (whole)” and FOBI BiomarkerOf object properties.
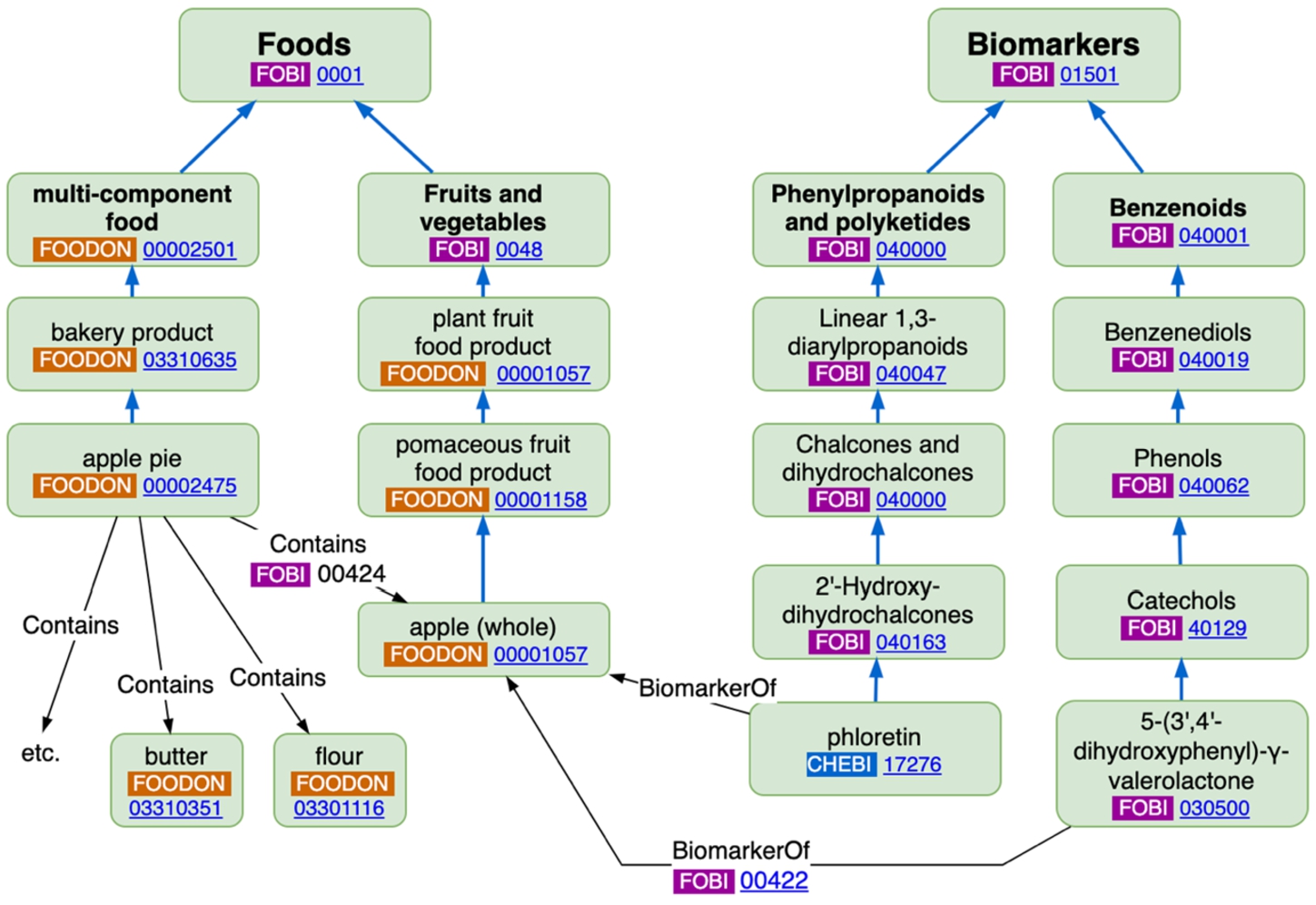
Various tasks could advance FOBI from an inaugural ontology. There are some lingering inconsistent PURLs (e/g. for Benzodioxoles, http://purl.obolibrary.org/obo/fobi.owl#FOBI:050222 should be standardized to http://purl.obolibrary.org/obo/FOBI_050222). FoodOn and ChEBI could be reused more: FOBI has apple juice as a subclass of “apple (whole)” rather than more correctly as a sibling product; ChEBI has a “catechol” class that could be reused. Remaining FOBI chemical classes should migrate into ChEBI if they are missing from that resource. FOBI class names are often pluralized, which OBO usually discourages as it conveys an instance of a class could point to a plurality of a thing rather than a single countable thing. FOBI has a number of classes such as “Phenylpropanoids and polyketides” which have disjunct subclasses, and which may be desired in a user interface menu but the question arises whether such umbrella classes are needed within an ontology itself, or would a hierarchy containing separate (in this case ChEBI) phenylpropanoid and polyketide classes and their branches suffice?
FOBI contains some example multi-component foods, like apple pie, which not directly connected to biomarkers, but which, by itemizing ingredients with a “Contains” (and inverse “IsIngredientOf”) object property, convey the possibility of recipe-ingredient-biomarker or biomarker-ingredient-recipe deduction, and related nutritional implications. However, it is rare that a recipe can be defined as a class with an exhaustive list of ingredients, since non-essential ingredient substitution is usually allowed (for example, shortening for butter in apple pie crust). FOBI could convert its multi-component food examples to test-sample instance-data (a particular apple pie’s constituents) and employ FoodOn’s “has ingredient” and “has defining ingredient” object properties. Alternately FOBI could consider leaving multi-component food definitions to the domain of a recipe database. There is also the fidelity question of which part of a plant or animal is precisely responsible for a biomarker – perhaps it should be axiomatized that only an apple hypanthium is the source of a biomarker residue, then we leave it to a reasoner to infer that ingesting a peeled, cored apple could lead to the biomarker being present. Also, “BiomarkerOf” is a shortcut relation representing a more complex digestion model which would be helpful to elaborate on, so that models could be offered for describing how biomarkers’ concentrations trail off time-wise after digestion, thus enabling prediction of how much related food may have been consumed.
3.8.DO and MONDO disease ontologies
The Human Disease Ontology (DO, with term prefix DOID) [64] has 28 “nutrition disease” (DOID:374) categories devoted to nutritional deficiency, for example “Keshan disease” arising from selenium deficiency, as well as overnutrition-triggered diseases. DO also includes allergies arising from food product consumption. The RO object property “has allergic trigger” (RO:0001022) has been used to attach an allergic disease to the class of food that triggers it. Food allergy (positioned under “gastrointestinal allergy”, an “An allergic disease that is located in the gastrointestinal tract”) currently has 33 categories including fish and shellfish, fruit, milk, wheat and vegetable subclasses, many with corresponding relations to FoodOn food products that cause them. DO also supports the Immune Epitope Database (IEDB) [65] database by enabling a selection of DO terms – ones with an “oboInOwl#inSubset DO_IEDB_slim” annotation – to be filtered into a separate file for export, and this includes the entire food allergy branch.
The Mondo Disease Ontology (MONDO), which seeks to cover and standardize disease vocabulary found in a number of large disease thesauri) has a “nutritional disorder” (MONDO:0005137) term branch containing cross references to DO and other ontologies and thesauri, and under which 5 “food-related allergic diseases”, 68 categories of “overnutrition” diseases (mainly obesity variants), and 67 categories of “nutritional deficiency disease” (including Keshan disease) are listed.
For food-related diseases, symptoms such as obesity and allergic inflammation can be found in the Human Phenotype Ontology (HP). Although it is problematic in a disease ontology to directly link diseases to symptoms with object properties (since OWL logic can only express certainty rather than probability that a symptom might be associated to a disease) this could be accomplished in a more context-sensitive database or knowledge graph of fuzzy logic or probabilistic connections between the two.
3.9.MAxO: The medical action ontology
MAxO [66], launched in 2020, is a broad ontology that provides a structured vocabulary for medical procedures, interventions, therapies, treatments, or clinical recommendations, including nutritional recommendations. MAxO was designed to annotate diseases, particularly rare diseases where management of nutrients is often critical. MAxO provides a lexicon of 77 dietary intake avoidance behaviour terms (Fig. 7) such as “selenium intake avoidance”, and an “avoided food” object property in the case where FoodOn products are being avoided. For example if a person has a strong fish odor, and Trimethylaminuria (the increased presence of trimethylamine in urine) is detected, then a MAxO “broccoli intake avoidance” treatment may be recommended to reduce trimethylamine abundance [67]. Additionally, over 70 nutritional supplementation process terms are included, including “selenium supplementation”, with linkages to directly to ChEBI chemicals as inputs (and in one case, “multivitamin supplementation” which uses the FoodOn “vitamin supplement”).
Fig. 7.
A small portion of the nutrition intervention hierarchy within MAxO.
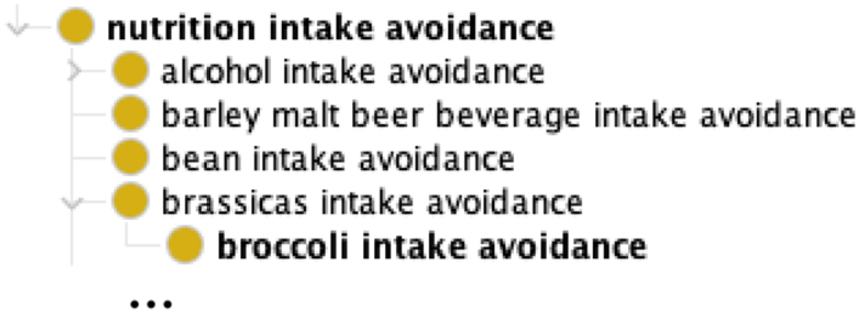
In order to capture the relationship between treatments and diseases, the Phenotypic Observation Explication Tool (POET, https://poet.jax.org/) was developed to establish a relationship between MAxO, HP, and MONDO terms. It allows researchers to actively participate in annotating diseases in their area of expertise.
3.10.FIDEO: Food interactions with drugs evidence ontology
FIDEO [68], launched in 2020, and providing over 170 FoodOn food categories, and over 260 ChEBI chemicals, is used in a database containing annotation and retrieval of scientific articles about food-drug interactions. Other domain-specific food categories such as “vitamin k-rich food product” are locally defined. While initial efforts were focused on the design of the ontology based on the Basic Formal Ontology (BFO) and the OBO Foundry principles, more recent efforts have resulted in a user-friendly visual interface that allows search and exploration of interactions [69] and currently focus on the automatic integration of food-drug interactions from DrugBank (https://go.drugbank.com) and Hedrine (https://hedrine.univ-grenoble-alpes.fr) to FIDEO using ROBOT’s [35] template command.
3.11.ONE: Ontology for nutritional epidemiology
ONE [70] details nutritional epidemiology manuscript and dataset characteristics, including the document structure of research manuscripts, dietary surveys, and food-based dietary guidelines (FBDGs). FBDGs represent a wealth of accumulated diet knowledge summarized from nutrition studies [71,72], and are important documents for policy makers, healthcare workers, educators and ultimately the general public to glean dietary policy and advice from. ONE brings into ontology form an established set of “Strengthening the Reporting of Observational Studies in Epidemiology” (STROBE) minimal requirements for the reporting of nutritional epidemiology research [73]. The first version of ONE extends IAO document parts to cover research paper structure, and as well description of food surveys which form the underlying datasets for many studies. Abstract, discussion, ethics, methods, results and supplementary methods specific to dietary studies are defined, as well as quality assessment classes of study designs and dietary recall methods (Fig. 8). FBDG terms were added into ONE in 2021 to support potential applications such as automated analysis of dietary trends, along with a graph database application for holding semi-automated assessments of nutritional epidemiology manuscripts [74].
Fig. 8.
ONE’s hierarchy of dietary survey quality indicators.
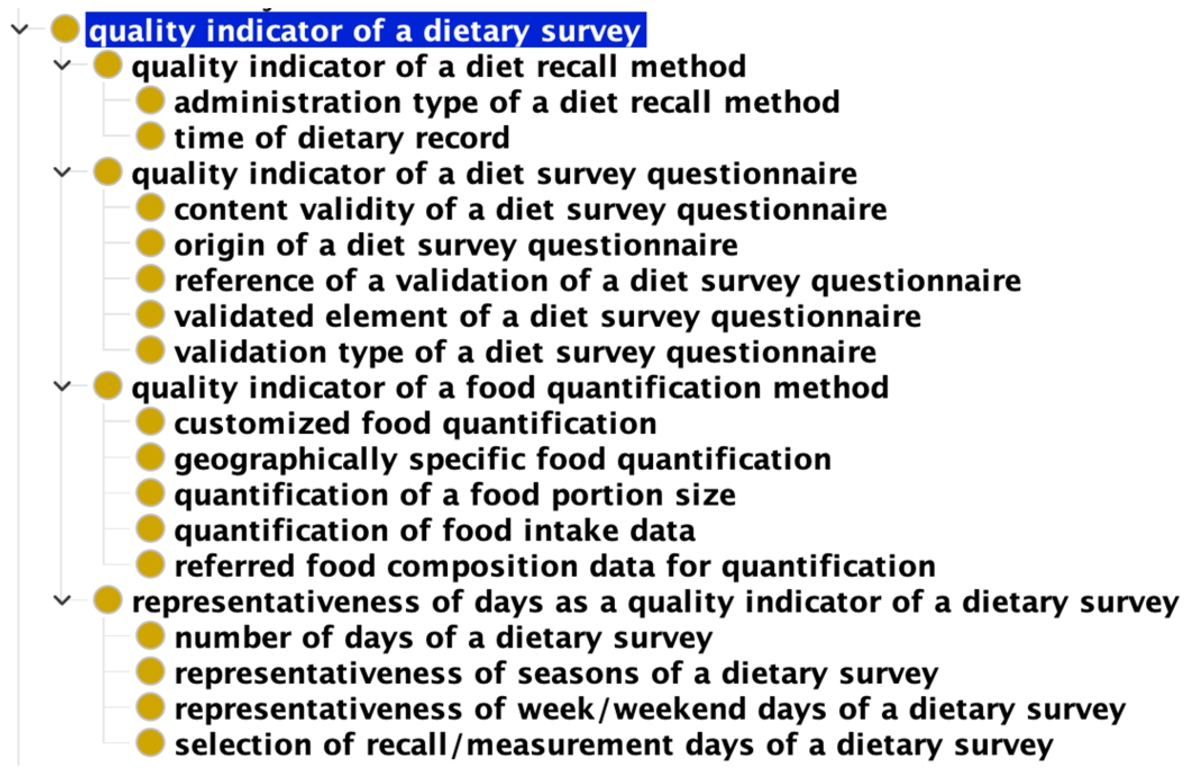
ONE curators began development of a Natural Language Processing (NLP) query language interface to enable plain language retrieval of relevant nutritional studies as encoded in a knowledge graph, as well as dashboard development to visualize nutritional knowledge contained in research manuscripts and population based recommendations [75].
3.12.HSO: The health surveillance ontology
HSO [76] is a knowledge model for data collection, collation and reporting of animal health, public health and food safety surveillance systems, including proactive surveillance and reactive investigation methods and objectives. While detailed surveillance data may not be easily harmonizable and/or shareable due to specific national agency reporting requirements, a top-down semantic harmonization of reporting elements is still required to pool summary data for international / multi-agency contexts. After engaging multilaterally about what the essential components of One Health surveillance data are, the HSO curation team developed vocabulary and a model centered around a planned process “surveillance activity” and related protocols, along with new vocabulary of over 200 classes, 40 object properties, and 20 data properties, for sampling strategies, host and pathogen taxonomy, specimen device type, and anatomical origin or substance. HSO is an outcome of the One Health suRveillance Initiative on harmOnization of data collection and interpretatioN (ORION) project and is currently interoperable with various catalogues in the European Food and Safety Administration’s (EFSA) Standard Sample Description. HSO can be naturally paired with the recently OBO-launched Vertebrate Breed Ontology (VBO) [77] of over 16,000 region-specific domestic animal breeds and wild lineages to pinpoint taxa beyond what NCBITaxon can offer.
Future HSO integration with OBI sample types would be beneficial. There may be opportunity to reduce the number of object properties, for example the HSO “Surveillance Activity” has “has surveillance actions” object properties which allow a “surveillance methods specification” – a diagnostic/reporting/sampling design/specimen collection protocol – in their range. Instead, in a Surveillance Activity’s plan specification could directly link to these protocols via “has part” without losing any reasoning or query power.
4.Discussion
Information is accruing within OBO ontologies as each adds term axioms that reference other terms inside or outside its own ontology. The wealth of this federated activity exists precisely because these ontologies are not constrained by the narrower mandate of any particular curation organization. Figure 9 shows an example of how FoodOn, DO and NCBITaxon ontology terms (including their axioms) combine without any extra work to express the fact that parsley, dill and celery allergies each arise from different food products which themselves derive from species contained within the same Tribe-level taxonomic hierarchy. Even from this short relationship chain, one could hypothesize potential allergies for consumers who are allergic to any one of these products. One can imagine similar hypotheses being generated about foods that have been produced or processed with similar classes of exogenous chemicals.
Our collective vision is to bridge the span of food-related activity – from agriculture and ecosystem context to harvest, distribution, consumption and related health effect analytics – with a set of entities and relationships between them, that would add up to an entire history or provenance trail of products. Accomplishing this however, requires extra multi-disciplinary thoughtfulness and work on the part of ontology curation teams, to in effect invent the language that makes this possible. This is an evolving, iterative process that has technical and multilateral challenges.
Fig. 9.
An allergy-food product-taxonomy pattern arising from the relations existing across 3 ontologies.
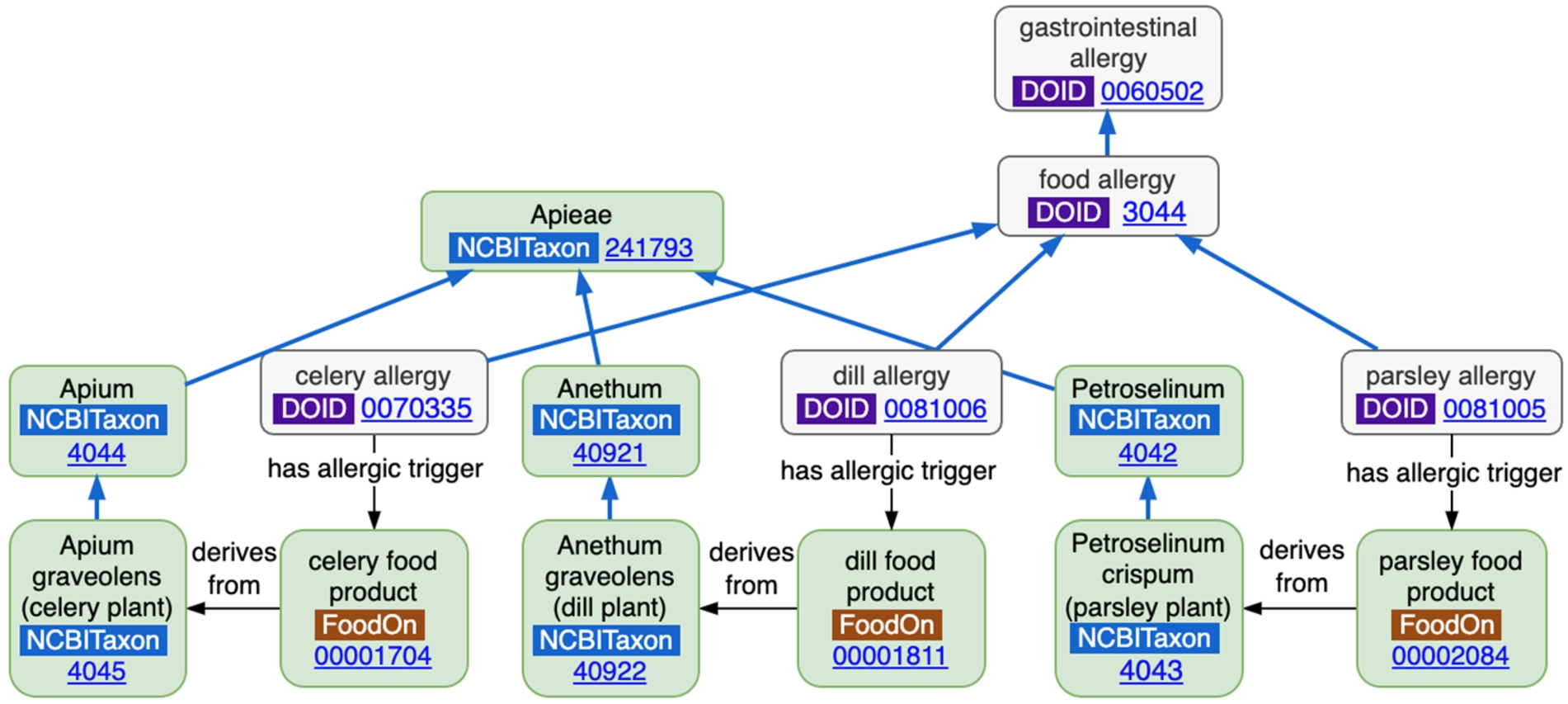
For example, AgrO connects various measurement protocols for crop area, row length, mulch thickness and color, well depth, etc. by a “measurement method of” object property to the characteristic they capture. To honor the OBO principle that RO should house most relationships, AgrO has initiated a request to add “measurement method of” to RO, with a proposed definition now under critique. RO has in turn asked OBI (because it leads planned process model relationship work) for recommendations about the definition and label of this property. Most of this dialogue is offered on a volunteer basis, with at best informal pro-bono offers of time. This exposes an education and communication problem: interoperability requires the expense of liaising between people and organizations about term definitions, and object and data property design patterns. A prerequisite to this work is to have curators understand the same paradigm for appropriate modelling of relations beyond a class-subclass hierarchy. Training programs are emerging, for example the OBOOK (https://oboacademy.github.io/obook/), to address this.
In general, more work needs to be done between food-related ontology curators to establish design patterns (as exemplified by nanopublications [78]) that congregate into larger knowledge graphs about food. By connecting biosamples, their context and bioactive constituents, and exposures, we could more easily see how over one-thousand CDNO dietary chemicals (including selenium) leave their mark on organism and environmental health. This will greatly facilitate dataset comparison and analysis within a clinical, veterinarian, or agricultural collection context, and would facilitate a cross-domain standard for human, animal, plant, or environmental biosample description, including its collection context, handling provenance, and analysis.
The Joint Food Ontology Workgroup has fostered teamwork to solve a variety of these problems. Longstanding issues about the presentation of vitamins as chemicals or as chemical roles have been resolved in 2021 with the help of some JFOW members and ChEBI; as well JFOW discussion has clarified the domain coverage and scope between ONE and ONS. Many modeling problems remain – standardizing the modeling of datasets holding biosamples for example, to detail time of observation and other contextual data. Ideally JFOW work would be supported by a full-time liaison to oversee modelling synchronization among the group agency’s projects.
Some OBO ontologies could be better integrated with FoodOn, Plant Ontology, ChEBI or NCBITaxon term references. For example DRON “cucumber allergenic extract” has no axiom connecting to cucumber; MONDO’s Keshan disease doesn’t connect to ChEBI selenium; PECO’s “selenium nutrient exposure” treatment could use an axiom indicating it is about, or has as a process input, selenium. FIDEO and FOBI could shift their native food terms into FoodOn. Some of the newer ontology entrants such as ONS launched with the manual editing a single term file, and now a few years later have to face the problem of catching up to changes in third party ontology content – namely, hierarchy changes. However, even for ontologies with term import and build systems, term curation coordination becomes more difficult the moment others are involved to distribute the technical load. This shows up for example in FoodOn situations where a new food term added to a ROBOT template table involves taxon or anatomy terms, referenced by name, that need to be imported in upstream OntoFox import files first before their names can even be recognized by the ROBOT tools, leading to a multi-stage compilation process. This error-prone complexity could be eliminated by the development of an elegant multi-user, multi-ontology and import file curation platform.
A larger challenge is to see how OBO might evolve to support domains that are outside of the life sciences. OBO would seem to have a natural counterpart in the Industrial Ontology Foundation (IOF), launched in 2016, but IOF’s recently launched core ontology [79,80] does not yet contain subdomains to trade terms with. ENVO’s “manufactured product” remains the tentative home for a large influx of food industry and other equipment useful for laboratory and food process modelling, including over 350 food related equipment terms via the Institute for Food Safety at Cornell University.
OBO ontologies and Simple Knowledge Organization System [81] or basic Resource Definition Framework [82] based vocabularies in business operations or strategy and sustainability policy sectors can potentially add value together in a knowledge graph. The GS1 Global Product Classification for Food/Beverage/Tobacco [83] could be linked to OBO food related ontologies for describing nutrients, allergens, ingredients and serving sizes for example. This is critical for supporting traceability requirements of many blockchain projects under development since GS1 standardized vocabulary is pervasive in business. On the horizon, the European Food and Safety Administration will be creating a pan-European food composition database presumably supported by the EFSA FoodEx2 vocabulary thesaurus, and this would benefit from integration with or mapping to OBO and other ontology-based knowledge.
5.Conclusion
The development of the OBO food related ontologies is occurring in a semi-autonomous parallel fashion, with interconnectivity issues arising on a weekly basis, and with the need to fund and train new talent to help curate the growing volume of what is a catch-up exercise to standardize and digitize vocabulary being used in research, policy and industry across the food spectrum. Taken together, the above work represents an explosion of knowledge and data harmonization about food systems, and is emerging as the language for a federated database model. The successful reuse of terms is demonstrated, and the methodology of inter-agency curation points the way to faster de-facto standardization of vocabulary. The semantic web way of thinking about vocabulary through OWL ontologies that easily generalize and specialize about food related data in the world still needs development work, but is proving to be a success in managing the complexity of life science knowledge, and a promising model for describing activity in health and sustainability policy and business domains as well.
Acknowledgements
This work is primarily supported by the USDA Non-Assistance Cooperative Agreement 58-8040-8-014-F and Genome Canada Grant 286GET to W. Hsiao, and NSF Awards OAC–2112606. Intelligent cyberinfrastructure with computational learning in the environment (ICICLE) AI institute, and CNS 1737573; SCC-RCN: Developing an Informational Infrastructure for Building Smart Regional Foodsheds.
Appendices
Appendix
Table 1
Abbreviations
Acronym | Resource | URL |
AgrO | Agronomy Ontology | https://github.com/AgriculturalSemantics/agro |
BFO | Basic Formal Ontology | http://ifomis.org/bfo |
CDNO | Compositional Dietary Nutrition Ontology | https://cdno.info |
CFSAN | Center for Food Safety and Applied Nutrition | https://www.fda.gov/about-fda/fda-organization/center-food-safety-and-applied-nutrition-cfsan |
ChEBI | Chemical Entities of Biological Interest | https://www.ebi.ac.uk/chebi |
CO | Crop Ontology | https://cropontology.org |
COB | Core Ontology for Biology and Biomedicine | https://obofoundry.org/COB |
DO | Disease Ontology | https://disease-ontology.org |
ECTO | Environmental conditions, treatments and exposures ontology | https://github.com/EnvironmentOntology/environmental-exposure-ontology |
ENVO | Environment Ontology | http://environmentontology.org |
PO | Plant Ontology | https://wiki.plantontology.org |
PTFI | Periodic Table of Food Initiative | https://foodperiodictable.org |
FAIR | FAIR Guiding Principles for scientific data management and stewardship | https://www.go-fair.org/fair-principles |
FBDG | Food-based dietary guideline | |
FCD | Food composition database | |
FDA | Food and Drug Administration | https://www.fda.gov |
FDC | FoodData Central website | https://fdc.nal.usda.gov |
FOBI | Food-Biomarker Ontology | https://github.com/pcastellanoescuder/FoodBiomarkerOntology |
FoodEx 2 | The food classification and description system | https://www.efsa.europa.eu/en/data/data-standardisation |
FoodKG | A Semantics-Driven Knowledge Graph for Food Recommendation | https://foodkg.github.io |
FoodOn | Food Ontology | https://foodon.org |
GenomeTrakr | GenomeTrakr Network | https://www.fda.gov/food/whole-genome-sequencing-wgs-program/genometrakr-network |
GSC | Genomic Standards Consortium | https://www.gensc.org |
HP | Human Phenotype Ontology | https://hpo.jax.org/app/ |
IAO | Information Artifact Ontology | https://github.com/information-artifact-ontology/IAO |
JFOW | Joint Food Ontology Workgroup | https://github.com/FoodOntology/joint-food-ontology-wg |
LanguaL | Langua aLimentaria | https://www.langual.org |
MIREOT | Minimum Information to Reference an External Ontology Term | https://doi.org/10.1038/npre.2009.3576.1 |
MIxS | GSC Minimum Information about any Sequence | https://www.gensc.org/pages/standards-intro.html |
MONDO | Mondo Disease Ontology | https://mondo.monarchinitiative.org |
NCBITaxon | National Center for Biotechnology Information Taxon Ontology | https://github.com/obophenotype/ncbitaxon |
NTR | New term request | |
OBI | Ontology for Biomedical Investigations | http://obi-ontology.org |
OBO | Open Biological and Biomedical Ontology Foundry | https://obofoundry.org |
ONS | Ontology for Nutritional Studies | https://github.com/enpadasi/Ontology-for-Nutritional-Studies |
OWL-Time | Time Ontology | https://www.w3.org/TR/owl-time |
PATO | Phenotype And Trait Ontology | https://github.com/pato-ontology/pato |
Table 1
(Continued)
Acronym | Resource | URL |
PECO | Plant Experimental Conditions Ontology | https://github.com/Planteome/plant-experimental-conditions-ontology |
PestOn | Pesticide Ontology | https://github.com/marco-medici/peston |
PO2 | Process and Observation Ontology | https://doi.org/10.15454/XSVVBW |
PURL | Permanent Uniform Resource Locator | https://en.wikipedia.org/wiki/Persistent_uniform_resource_locator |
RDF | Resource Description Framework | https://www.w3.org/RDF |
RO | Relation Ontology | https://oborel.github.io |
TO | Plant Trait Ontology | https://github.com/Planteome/plant-trait-ontology |
UBERON | Multi-species anatomy ontology | http://obophenotype.github.io/uberon |
USDA | U.S. Department of Agriculture | https://www.usda.gov |
VBO | Vertebrate Breed Ontology | https://github.com/monarch-initiative/vertebrate-breed-ontology |
WikiFCD | Wikidata Food Composition Data | https://wikifcd.wiki.opencura.com |
References
[1] | B. Smith, M. Ashburner, C. Rosse, J. Bard, W. Bug, W. Ceusters, L.J. Goldberg, K. Eilbeck, A. Ireland, C.J. Mungall, N. Leontis, P. Rocca-Serra, A. Ruttenberg, S.-A. Sansone, R.H. Scheuermann, N. Shah, P.L. Whetzel and S. Lewis (OBI Consortium), The OBO foundry: Coordinated evolution of ontologies to support biomedical data integration, Nat. Biotechnol. 25: ((2007) ), 1251–1255. doi:10.1038/nbt1346. |
[2] | A. Zeb, J.-P. Soininen and N. Sozer, Data harmonisation as a key to enable digitalisation of the food sector: A review, Food Bioprod. Process. 127: ((2021) ), 360–370. doi:10.1016/j.fbp.2021.02.005. |
[3] | D.M. Dooley, E.J. Griffiths, G.S. Gosal, P.L. Buttigieg, R. Hoehndorf, M.C. Lange, L.M. Schriml, F.S.L. Brinkman and W.W.L. Hsiao, FoodOn: A harmonized food ontology to increase global food traceability, quality control and data integration, NPJ Sci Food 2: ((2018) ), 23. doi:10.1038/s41538-018-0032-6. |
[4] | M.C. Lange, D.G. Lemay and J.B. German, A multi-ontology framework to guide agriculture and food towards diet and health, J. Sci. Food Agric. 87: ((2007) ), 1427–1434. doi:10.1002/jsfa.2832. |
[5] | J.D. Ireland and A. Møller, LanguaL food description: A learning process, Eur. J. Clin. Nutr. 64: (3) ((2010) ), S44–S48. doi:10.1038/ejcn.2010.209. |
[6] | P.L. Buttigieg, N. Morrison, B. Smith, C.J. Mungall and S.E. Lewis (ENVO Consortium), The environment ontology: Contextualising biological and biomedical entities, J. Biomed. Semantics 4: ((2013) ), 43. doi:10.1186/2041-1480-4-43. |
[7] | P. de Matos, R. Alcántara, A. Dekker, M. Ennis, J. Hastings, K. Haug, I. Spiteri, S. Turner and C. Steinbeck, Chemical entities of biological interest: An update, Nucleic Acids Res. 38: ((2010) ), D249–D254. doi:10.1093/nar/gkp886. |
[8] | R.L. Walls, L. Cooper, J. Elser, M.A. Gandolfo, C.J. Mungall, B. Smith, D.W. Stevenson and P. Jaiswal, The Plant Ontology facilitates comparisons of plant development stages across species, Front. Plant Sci. 10: ((2019) ), 631. doi:10.3389/fpls.2019.00631. |
[9] | C.J. Mungall, C. Torniai, G.V. Gkoutos, S.E. Lewis and M.A. Haendel, Uberon, an integrative multi-species anatomy ontology, Genome Biol. 13: ((2012) ), R5. doi:10.1186/gb-2012-13-1-r5. |
[10] | S. Federhen, The NCBI taxonomy database, Nucleic Acids Res. 40: ((2012) ), D136–D143. doi:10.1093/nar/gkr1178. |
[11] | R. Azman Halimi, B.J. Barkla, L. Andrés-Hernandéz, S. Mayes and G.J. King, Bridging the food security gap: An information-led approach to connect dietary nutrition, food composition and crop production, J. Sci. Food Agric. 100: ((2020) ), 1495–1504. doi:10.1002/jsfa.10157. |
[12] | F. Vitali, R. Lombardo, D. Rivero, F. Mattivi, P. Franceschi, A. Bordoni, A. Trimigno, F. Capozzi, G. Felici, F. Taglino, F. Miglietta, N. De Cock, C. Lachat, B. De Baets, G. De Tré, M. Pinart, K. Nimptsch, T. Pischon, J. Bouwman and D. Cavalieri, ENPADASI consortium, ONS: An ontology for a standardized description of interventions and observational studies in nutrition, Genes Nutr. 13: ((2018) ), 12. doi:10.1186/s12263-018-0601-y. |
[13] | IAO: Information artifact ontology, Github, n.d., https://github.com/information-artifact-ontology/IAO (accessed November 2, 2022). |
[14] | A. Bandrowski, R. Brinkman, M. Brochhausen, M.H. Brush, B. Bug, M.C. Chibucos, K. Clancy, M. Courtot, D. Derom, M. Dumontier, L. Fan, J. Fostel, G. Fragoso, F. Gibson, A. Gonzalez-Beltran, M.A. Haendel, Y. He, M. Heiskanen, T. Hernandez-Boussard, M. Jensen, Y. Lin, A.L. Lister, P. Lord, J. Malone, E. Manduchi, M. McGee, N. Morrison, J.A. Overton, H. Parkinson, B. Peters, P. Rocca-Serra, A. Ruttenberg, S.-A. Sansone, R.H. Scheuermann, D. Schober, B. Smith, L.N. Soldatova, C.J. Stoeckert Jr., C.F. Taylor, C. Torniai, J.A. Turner, R. Vita, P.L. Whetzel and J. Zheng, The ontology for biomedical investigations, PLoS One 11: ((2016) ), e0154556. doi:10.1371/journal.pone.0154556. |
[15] | obo-relations: RO is an ontology of relations for use with biological ontologies, Github, n.d., https://github.com/oborel/obo-relations (accessed March 5, 2022). |
[16] | C. Aubert, P.L. Buttigieg, M.A. Laporte, M. Devare and E. Arnaud, CGIAR Agronomy Ontology, (2017) , https://github.com/AgriculturalSemantics/agro (accessed October 17, 2017). |
[17] | S. Haussmann, O. Seneviratne, Y. Chen, Y. Ne’eman, J. Codella, C.-H. Chen, D.L. McGuinness and M.J. Zaki, FoodKG: A Semantics-Driven Knowledge Graph for Food Recommendation, Lecture Notes in Computer Science, (2019) , pp. 146–162. doi:10.1007/978-3-030-30796-7_10. |
[18] | USDA national nutrient database for standard reference, legacy release, (n.d.), https://data.nal.usda.gov/dataset/usda-national-nutrient-database-standard-reference-legacy-release (accessed November 3, 2022). |
[19] | Whole genome sequencing (WGS) program, U.S. Food and Drug Administration. (n.d.), https://www.fda.gov/food/science-research-food/whole-genome-sequencing-wgs-program (accessed October 25, 2022). |
[20] | Standardizing the isolation source metadata for the genomic epidemiolo, FDA, ((2021) ), https://www.fda.gov/science-research/fda-science-forum/standardizing-isolation-source-metadata-genomic-epidemiology-foodborne-pathogens-using-lexmapr (accessed October 25, 2022). |
[21] | G. Gosal, E. Griffiths, D. Dooley, I. Gill, D. Fornika, H. Tate, M. Sanchez, R. Timme and W. Hsiao, LexMapr: A rule-based text mining tool for ontology-driven harmonization of short biomedical specimen descriptions, Research 8: ((2019) ), F1000. doi:10.7490/F1000RESEARCH.1117323.1. |
[22] | C.J. Grim, A.M. Windsor, B. Kocurek, S.R. Leonard, T.K.S. Richter, G. Gopinath, M. Balkey, P. Ramachandran, A. Ottesen, K. Jarvis and R. Timme, Development of a MIxS (Minimum Information about any (x) Sequence) food environmental metadata standard, 2020, https://bit.ly/MIxS_Food_Dev (accessed June 22, 2021). |
[23] | BioSample attributes, (n.d.), https://submit.ncbi.nlm.nih.gov/biosample/template/?organism-organism_name=&organism-taxonomy_id=&package-0=OneHealthEnteric.1.0&action=definition (accessed May 10, 2023). |
[24] | J. Feng, D. Daeschel, D. Dooley, E. Griffiths, M. Allard, R. Timme, Y. Chen and A.B. Snyder, A schema for digitized surface swab site metadata in open-source DNA sequence databases, MSystems 8: ((2023) ), e0128422. doi:10.1128/msystems.01284-22. |
[25] | Avma, One health: A new professional imperative, American Veterinary Medical Association Schaumburg, IL, (2008) , https://www.avma.org/sites/default/files/resources/onehealth_final.pdf. |
[26] | F.C. Dórea, F. Vial, K. Hammar, A. Lindberg, P. Lambrix, E. Blomqvist and C.W. Revie, Drivers for the development of an animal health surveillance ontology (AHSO), Prev. Vet. Med. 166: ((2019) ), 39–48. doi:10.1016/j.prevetmed.2019.03.002. |
[27] | M.A. Musen (Protégé Team), The protégé project, AI Matters. 1: ((2015) ), 4–12. doi:10.1145/2757001.2757003. |
[28] | OWL – semantic web standards, (n.d.), https://www.w3.org/OWL/ (accessed November 1, 2022). |
[29] | OBO principles, OBO foundry. (n.d.), http://www.obofoundry.org/principles/fp-000-summary.html (accessed June 24, 2021). |
[30] | Y. He, Z. Xiang, J. Zheng, Y. Lin, J.A. Overton and E. Ong, The eXtensible ontology development (XOD) principles and tool implementation to support ontology interoperability, J. Biomed. Semantics 9: ((2018) ), 3. doi:10.1186/s13326-017-0169-2. |
[31] | Z. Xiang, M. Courtot, R.R. Brinkman, A. Ruttenberg and Y. He, OntoFox: Web-based support for ontology reuse, BMC Res. Notes. 3: ((2010) ), 175. doi:10.1186/1756-0500-3-175. |
[32] | J.A. Overton, M. Cuffaro and C.J. Mungall, The OBO foundry operations committee technical working groupc, string of PURLs – frugal migration and maintenance of persistent identifiers, Data Sci. 3: ((2020) ), 3–13. doi:10.3233/DS-190022. |
[33] | Z. Xiang, C. Mungall, A. Ruttenberg and Y. He, Ontobee: A linked data server and browser for ontology terms, in: ICBO, (2011) , https://www.academia.edu/download/39270744/0deec5393d9ae86155000000.pdf. |
[34] | FAIR principles, GO FAIR, (2017) , https://www.go-fair.org/fair-principles/ (accessed November 3, 2022). |
[35] | R.C. Jackson, J.P. Balhoff, E. Douglass, N.L. Harris, C.J. Mungall and J.A. Overton, ROBOT: A tool for automating ontology workflows, BMC Bioinformatics 20: ((2019) ), 407. doi:10.1186/s12859-019-3002-3. |
[36] | R. Arp, B. Smith and A.D. Spear, Building Ontologies with Basic Formal Ontology, MIT Press, (2015) , https://market.android.com/details?id=book-AUxQCgAAQBAJ. |
[37] | Core ontology for biology and biomedicine, (n.d.), https://obofoundry.org/COB/ (accessed October 15, 2022). |
[38] | N. Matentzoglu, D. Goutte-Gattat, S.Z.K. Tan, J.P. Balhoff, S. Carbon, A.R. Caron, W.D. Duncan, J.E. Flack, M. Haendel, N.L. Harris, W.R. Hogan, C.T. Hoyt, R.C. Jackson, H. Kim, H. Kir, M. Larralde, J.A. McMurry, J.A. Overton, B. Peters, C. Pilgrim, R. Stefancsik, S.M. Robb, S. Toro, N.A. Vasilevsky, R. Walls, C.J. Mungall and D. Osumi-Sutherland, Ontology development kit: A toolkit for building, maintaining and standardizing biomedical ontologies, Database (Oxford) 2022: ((2022) ), baac087. doi:10.1093/database/baac087. |
[39] | R.C. Jackson, N. Matentzoglu, J.A. Overton, R. Vita, J.P. Balhoff, P.L. Buttigieg, S. Carbon, M. Courtot, A.D. Diehl, D. Dooley, W. Duncan, N.L. Harris, M.A. Haendel, S.E. Lewis, D.A. Natale, D. Osumi-Sutherland, A. Ruttenberg, L.M. Schriml, B. Smith, C.J. Stoeckert, N.A. Vasilevsky, R.L. Walls, J. Zheng, C.J. Mungall and B. Peters, OBO foundry in 2021: Operationalizing open data principles to evaluate ontologies, BioRxiv. (2021) , 2021.06.01.446587. doi:10.1101/2021.06.01.446587. |
[40] | JFOW, Joint Food Ontology Workgroup. (n.d.), https://github.com/FoodOntology/joint-food-ontology-wg (accessed June 24, 2021). |
[41] | D. Dooley, M. Weber, L. Ibanescu, M. Lange, L. Chan, L. Soldatova, C. Yang, R. Warren, C. Shimizu, H.K. McGinty and W. Hsiao, Food process ontology requirements, Semant. Web. ((2022) ), 1–32, preprint. doi:10.3233/sw-223096. |
[42] | R. Stoffaneller and N.L. Morse, A review of dietary selenium intake and selenium status in Europe and the Middle East, Nutrients 7: ((2015) ), 1494–1537. doi:10.3390/nu7031494. |
[43] | Y. Shi, W. Yang, X. Tang, Q. Yan, X. Cai and F. Wu, Keshan disease: A potentially fatal endemic cardiomyopathy in remote mountains of China, Front. Pediatr. 9: ((2021) ), 576916. doi:10.3389/fped.2021.576916. |
[44] | J.K. MacFarquhar, D.L. Broussard, P. Melstrom, R. Hutchinson, A. Wolkin, C. Martin, R.F. Burk, J.R. Dunn, A.L. Green, R. Hammond, W. Schaffner and T.F. Jones, Acute selenium toxicity associated with a dietary supplement, Arch. Intern. Med. 170: ((2010) ), 256–261. doi:10.1001/archinternmed.2009.495. |
[45] | J. Köhrle, Selenium and the thyroid, Curr. Opin. Endocrinol. Diabetes Obes. 22: ((2015) ), 392–401. doi:10.1097/MED.0000000000000190. |
[46] | L. Cooper, M.-A. Laporte, J. Elser, V.C. Blake, T.Z. Sen, C. Mungall and E. Arnaud, The plant trait ontology links wheat traits for crop improvement and genomics, (n.d.), http://ceur-ws.org/Vol-2807/abstractT.pdf (accessed October 29, 2022). |
[47] | R. Shrestha, L. Matteis, M. Skofic, A. Portugal, G. McLaren, G. Hyman and E. Arnaud, Bridging the phenotypic and genetic data useful for integrated breeding through a data annotation using the crop ontology developed by the crop communities of practice, Front. Physiol. 3: ((2012) ), 326. doi:10.3389/fphys.2012.00326. |
[48] | L. Cooper, A. Meier, M.-A. Laporte, J.L. Elser, C. Mungall, B.T. Sinn, D. Cavaliere, S. Carbon, N.A. Dunn, B. Smith, B. Qu, J. Preece, E. Zhang, S. Todorovic, G. Gkoutos, J.H. Doonan, D.W. Stevenson, E. Arnaud and P. Jaiswal, The Planteome database: An integrated resource for reference ontologies, plant genomics and phenomics, Nucleic Acids Res. 46: ((2018) ), D1168–D1180. doi:10.1093/nar/gkx1152. |
[49] | G.V. Gkoutos, P.N. Schofield and R. Hoehndorf, The anatomy of phenotype ontologies: Principles, properties and applications, Brief. Bioinform. 19: ((2018) ), 1008–1021. doi:10.1093/bib/bbx035. |
[50] | M. Devare, C. Aubert, O.E. Benites Alfaro, I.O. Perez Masias and M.-A. Laporte, AgroFIMS: A tool to enable digital collection of standards-compliant FAIR data, Front. Sustain. Food Syst. 5: ((2021) ). doi:10.3389/fsufs.2021.726646. |
[51] | P. Yilmaz, R. Kottmann, D. Field, R. Knight, J.R. Cole, L. Amaral-Zettler, J.A. Gilbert, I. Karsch-Mizrachi, A. Johnston, G. Cochrane, R. Vaughan, C. Hunter, J. Park, N. Morrison, P. Rocca-Serra, P. Sterk, M. Arumugam, M. Bailey, L. Baumgartner, B.W. Birren, M.J. Blaser, V. Bonazzi, T. Booth, P. Bork, F.D. Bushman, P.L. Buttigieg, P.S.G. Chain, E. Charlson, E.K. Costello, H. Huot-Creasy, P. Dawyndt, T. DeSantis, N. Fierer, J.A. Fuhrman, R.E. Gallery, D. Gevers, R.A. Gibbs, I.S. Gil, A. Gonzalez, J.I. Gordon, R. Guralnick, W. Hankeln, S. Highlander, P. Hugenholtz, J. Jansson, A.L. Kau, S.T. Kelley, J. Kennedy, D. Knights, O. Koren, J. Kuczynski, N. Kyrpides, R. Larsen, C.L. Lauber, T. Legg, R.E. Ley, C.A. Lozupone, W. Ludwig, D. Lyons, E. Maguire, B.A. Methé, F. Meyer, B. Muegge, S. Nakielny, K.E. Nelson, D. Nemergut, J.D. Neufeld, L.K. Newbold, A.E. Oliver, N.R. Pace, G. Palanisamy, J. Peplies, J. Petrosino, L. Proctor, E. Pruesse, C. Quast, J. Raes, S. Ratnasingham, J. Ravel, D.A. Relman, S. Assunta-Sansone, P.D. Schloss, L. Schriml, R. Sinha, M.I. Smith, E. Sodergren, A. Spor, J. Stombaugh, J.M. Tiedje, D.V. Ward, G.M. Weinstock, D. Wendel, O. White, A. Whiteley, A. Wilke, J.R. Wortman, T. Yatsunenko and F.O. Glöckner, Minimum information about a marker gene sequence (MIMARKS) and minimum information about any (x) sequence (MIxS) specifications, Nat. Biotechnol. 29: ((2011) ), 415–420. doi:10.1038/nbt.1823. |
[52] | Class: Soil, (n.d.), https://genomicsstandardsconsortium.github.io/mixs/Soil/ (accessed October 28, 2022). |
[53] | L. Andrés-Hernández, K. Blumberg, R.L. Walls, D. Dooley, R. Mauleon, M. Lange, M. Weber, L. Chan, A. Malik, A. Møller, J. Ireland, L. Segovia, X. Zhang, B. Burton-Freeman, P. Magelli, A. Schriever, S.M. Forester, L. Liu and G.J. King, Establishing a common nutritional vocabulary – from food production to diet, Front. Nutr. 9: ((2022) ), 928837. doi:10.3389/fnut.2022.928837. |
[54] | S.P. Murphy, U.R. Charrondiere and B. Burlingame, Thirty years of progress in harmonizing and compiling food data as a result of the establishment of INFOODS, Food Chem. 193: ((2016) ), 2–5. doi:10.1016/j.foodchem.2014.11.097. |
[55] | FoodData central, (n.d.), https://fdc.nal.usda.gov/download-datasets.html (accessed November 2, 2022). |
[56] | L. Chan, A. Thessen, W.D. Duncan, N. Matentzoglu, C. Schmitt, C. Grondin, N. Vasilevsky, J. McMurry, P. Robinson, C.J. Mungall, M.A. Haendel, The Environmental Conditions, Treatments, and Exposures Ontology (ECTO): Connecting toxicology and exposure to human health and beyond, Journal of Biomedical Semantics 14: (3) ((2023) ). doi:10.1186/s13326-023-00283-x. |
[57] | D. Osumi-Sutherland, M. Courtot, J.P. Balhoff and C. Mungall, Dead simple OWL design patterns, J. Biomed. Semantics 8: ((2017) ), 18. doi:10.1186/s13326-017-0126-0. |
[58] | M. Medici, D. Dooley and M. Canavari, PestOn: An ontology to make pesticides information easily accessible and interoperable, Sustainability 14: ((2022) ), 6673. doi:10.3390/su14116673. |
[59] | SPARQL 1.1 query language, (n.d.), https://www.w3.org/TR/sparql11-query/ (accessed November 4, 2022). |
[60] | L. Ibanescu, J. Dibie, S. Dervaux, E. Guichard and J. Raad, PO2 – a process and observation ontology in food science. Application to dairy gels, in: Metadata and Semantics Research, Springer International Publishing, (2016) , pp. 155–165. doi:10.1007/978-3-319-49157-8_13. |
[61] | K. Janowicz, A. Haller, S.J.D. Cox, D. Le Phuoc and M. Lefrançois, SOSA: A lightweight ontology for sensors, observations, samples, and actuators, Journal of Web Semantics 56: ((2019) ), 1–10. doi:10.1016/j.websem.2018.06.003. |
[62] | M. Weber, P. Buche and L. et al. Ibanescu, PO2/TransformON, an ontology for data integration on food, feed, bioproducts and biowaste engineering, npj Sci Food 7: ((2023) ), 47. doi:10.1038/s41538-023-00221-2. |
[63] | P. Castellano-Escuder, R. González-Domínguez, D.S. Wishart, C. Andrés-Lacueva and A. Sánchez-Pla, FOBI: An ontology to represent food intake data and associate it with metabolomic data, Database 2020: ((2020) ). doi:10.1093/databa/baaa033. |
[64] | L.M. Schriml, E. Mitraka, J. Munro, B. Tauber, M. Schor, L. Nickle, V. Felix, L. Jeng, C. Bearer, R. Lichenstein, K. Bisordi, N. Campion, B. Hyman, D. Kurland, C.P. Oates, S. Kibbey, P. Sreekumar, C. Le, M. Giglio and C. Greene, Human disease ontology 2018 update: Classification, content and workflow expansion, Nucleic Acids Res. ((2018) ). doi:10.1093/nar/gky1032. |
[65] | R. Vita, S. Mahajan, J.A. Overton, S.K. Dhanda, S. Martini, J.R. Cantrell, D.K. Wheeler, A. Sette and B. Peters, The Immune Epitope Database (IEDB): 2018 update, Nucleic Acids Res. 47: ((2019) ), D339–D343. doi:10.1093/nar/gky1006. |
[66] | MAxO, Medical Action Ontology (MAxO). (n.d.), https://github.com/monarch-initiative/MAxO (accessed June 24, 2021). |
[67] | I.R. Phillips, E.A. Shephard, Primary Trimethylaminuria, (1993) , https://pubmed.ncbi.nlm.nih.gov/20301282/ (accessed October, 2022). |
[68] | G. Bordea, J. Nikiema, R. Griffier, T. Hamon and F. Mougin, FIDEO: Food interactions with drugs evidence ontology, in: 11th International Conference on Biomedical Ontologies, (2020) , https://hal.archives-ouvertes.fr/hal-03185166/. |
[69] | F. Lalanne, P. Bedouch, C. Simonnet, V. Depras, G. Bordea, R. Bourqui, T. Hamon, F. Thiessard and F. Mougin, Visualizing food-drug interactions in the Thériaque database, Stud. Health Technol. Inform. 281: ((2021) ), 253–257. doi:10.3233/SHTI210159. |
[70] | C. Yang, H. Ambayo, B.D. Baets, P. Kolsteren, N. Thanintorn, D. Hawwash, J. Bouwman, A. Bronselaer, F. Pattyn and C. Lachat, An ontology to standardize research output of nutritional epidemiology: From paper-based standards to linked content, Nutrients 11: ((2019) ). doi:10.3390/nu11061300. |
[71] | N. Teicholz, The scientific report guiding the US dietary guidelines: Is it scientific?, BMJ. 351: ((2015) ), h4962. doi:10.1136/bmj.h4962. |
[72] | C. Montagnese, L. Santarpia, M. Buonifacio, A. Nardelli, A.R. Caldara, E. Silvestri, F. Contaldo and F. Pasanisi, European food-based dietary guidelines: A comparison and update, Nutrition 31: ((2015) ), 908–915. doi:10.1016/j.nut.2015.01.002. |
[73] | C. Lachat, D. Hawwash, M.C. Ocké, C. Berg, E. Forsum, A. Hörnell, C. Larsson, E. Sonestedt, E. Wirfält, A. Åkesson, P. Kolsteren, G. Byrnes, W. De Keyzer, J. Van Camp, J.E. Cade, N. Slimani, M. Cevallos, M. Egger and I. Huybrechts, Strengthening the reporting of observational studies in epidemiology – nutritional epidemiology (STROBE-nut): An extension of the STROBE statement, PLOS Medicine 13: ((2016) ), e1002036. doi:10.1371/journal.pmed.1002036. |
[74] | C. Yang, D. Hawwash, B. De Baets, J. Bouwman and C. Lachat, Perspective: Towards automated tracking of content and evidence appraisal of nutrition research, Adv. Nutr. 11: ((2020) ), 1079–1088. doi:10.1093/advances/nmaa057. |
[75] | C. Yang, Nutritional-epidemiologic-ontologies: Machine-readable nutritional epidemiologic (meta-)data, Github, n.d., https://github.com/cyang0128/Nutritional-epidemiologic-ontologies (accessed November 4, 2022). |
[76] | M. Filter, T. Buschhardt, F. Dórea, E. Lopez de Abechuco, T. Günther, E.M. Sundermann, J. Gethmann, J. Dups-Bergmann, K. Lagesen and J. Ellis-Iversen, One health surveillance codex: Promoting the adoption of one health solutions within and across European countries, One Health 12: ((2021) ), 100233. doi:10.1016/j.onehlt.2021.100233. |
[77] | Vertebrate breed ontology, (n.d.), https://monarch-initiative.github.io/vertebrate-breed-ontology/ (accessed November 4, 2022). |
[78] | E. Mina, M. Thompson, R. Kaliyaperumal, J. van Zhao, E. der Horst, Z. Tatum, K.M. Hettne, E.A. Schultes, B. Mons and M. Roos, Nanopublications for exposing experimental data in the life-sciences: A Huntington’s disease case study, J. Biomed. Semantics 6: ((2015) ), 5. doi:10.1186/2041-1480-6-5. |
[79] | M. Drobnjakovic, F. Ameri, C. Will, B. Smith and A. Jones, The Industrial Ontologies Foundry (IOF) core ontology, (n.d.), https://philarchive.org/archive/DROTIO-2 (accessed November 2, 2022). |
[80] | Ontology, Github, n.d., https://github.com/iofoundry/ontology (accessed November 8, 2022). |
[81] | SKOS Simple Knowledge Organization System – home page, (n.d.), https://www.w3.org/2004/02/skos (accessed May 9, 2023). |
[82] | RDF 1.1 concepts and abstract syntax, (n.d.), https://www.w3.org/TR/rdf11-concepts/ (accessed May 9, 2023). |
[83] | GS1 food, GS1 global product classification for food/beverage/tobacco, (2018) , https://www.gs1.org/voc/FoodBeverageTobaccoProduct (accessed June 25, 2021). |