Genomic and Transcriptomic Characteristics of Tumors of Patients with Metastatic Clear Cell Renal Cell Carcinoma Clinically Benefiting from First-Line Treatment with Ipilimumab Plus Nivolumab
Abstract
Background:
Ipilimumab plus nivolumab is approved as a first-line treatment for intermediate or poor risk metastatic renal cell carcinoma (mRCC). However, ∼35% of patients progress within six months on ipilimumab plus nivolumab, and no validated genomic biomarkers predict the benefit. In this study, we explore the genomic and transcriptomic differences among patients with clear cell mRCC patients who either did or did not experience clinical benefit from first-line ipilimumab plus nivolumab therapy.
Method:
Patients with clear cell mRCC intermediate or poor IMDC risk scores, with available tumor whole exome with/without transcriptome sequencing before starting systemic therapy were included. Patients who developed a complete response, partial response, or stable disease for at least six months after initiating treatment were categorized into the ‘clinical benefit’ group, whereas the rest were classified as ‘no clinical benefit.’ Genomic alteration frequencies between the groups were assessed with a chi-square test. Differentially expressed genes and gene sets were identified via DeSeq2 and GSEA v4.2.3, respectively.
Result:
53 patients with clear cell mRCC (37 clinical benefit and 16 no clinical benefit) were eligible and included. No significant difference was found in the genomic alteration frequencies between these groups. Baseline tumor transcriptomic data were available for 14 patients (9 clinical benefit and 5 no clinical benefit). The apical surface and pathways downregulated by KRAS signaling were enriched in the clinical benefit group, whereas inflammatory pathways were enriched in the no clinical benefit group.
Conclusion:
These findings suggest that tumor specific gene expression as assessed by RNA sequencing could serve as a potential biomarker of response to ipilimumab plus nivolumab therapy.
INTRODUCTION
The therapeutic landscape of metastatic renal cell carcinoma (mRCC) has evolved rapidly in the past few years. Combination therapy incorporating dual immune checkpoint inhibitors targeting programmed cell death protein 1 (PD-1) and cytotoxic T-lymphocyte-associated protein 4 (CTLA-4) or combined PD-1 and vascular endothelial growth factor (VEGF) inhibition has become the standard of care after demonstrating significant improvement in overall survival (OS) compared to monotherapy with the VEGF receptor tyrosine kinase inhibitor, sunitinib, in randomized clinical trials [1–4]. In the CheckMate 214 trial, patients with intermediate or poor risk disease per the International mRCC Database Consortium (IMDC) risk stratification had significantly better objective response rate (ORR) and OS with the combination of ipilimumab and nivolumab as compared to sunitinib leading to its regulatory approval [5, 6]. Though ipilimumab plus nivolumab is one of the commonly used first-line treatment regimens, not all patients benefit [6, 7]. For instance, although durable partial and complete responses were seen in a subset of patients with intermediate or poor-risk disease in the CheckMate 214 study, a significant proportion (20%) of patients had primary progressive disease as the best response and ∼35% progressed within 6 months [6]. The median progression-free survival is 11.6 months [6].
To date, there does not exist a clinically actionable biomarker to guide therapy selection in front-line mRCC, and treatment selection is made based on IMDC risk stratification, patient disease volume, comorbidities, and patient and clinician’s preferences [8]. Several putative biomarkers derived from comprehensive genomic profiling (CGP) are currently under investigation, such as tumor mutational burden (TMB), somatic alterations in PBRM1, along with RNA-based molecular subtypes and gene expression signatures characterizing tumor microenvironment and anti-tumor immune response [9–17]. For example, whole-transcriptome analysis of IMmotion151 trial tumor samples revealed seven unique molecular patient subgroups. Atezolizumab (anti-PD-L1) combined with bevacizumab (VEGF monoclonal antibody) showed improved results in clusters 4 (T-effector/proliferative), 5 (proliferative), and 7 (snoRNA), while sunitinib monotherapy (VEGF Tyrosine kinase inhibitor) seemed more effective in clusters 1 (angiogenic/stromal) and 2 (angiogenic) [18]. However, none of these biomarker candidates have been validated prospectively. Therefore, there continues to be a significant unmet need to develop and validate biomarkers to predict immune checkpoint inhibitor-based therapy outcomes in mRCC. This multi-institution real-world retrospective study evaluated the genomic and transcriptomic characteristics associated with clinical benefit in mRCC patients receiving first-line therapy with ipilimumab and nivolumab.
METHODS
Patient selection
This retrospective study included patients with a histologically confirmed diagnosis of mRCC receiving treatment at two National Cancer Institute-Designated Comprehensive Cancer Centers (NCI-CCC; Huntsman Cancer Institute at the University of Utah, Salt Lake City, Utah, and the City of Hope Comprehensive Cancer Center, Duarte, California) between 2017 through 2022. Eligible patients had clear cell histology, intermediate or poor disease per IMDC score, received ipilimumab plus nivolumab as first-line treatment for metastatic disease, and had genomic with/without transcriptomic data from the treatment naïve tumor tissue (described below).
Patient-level clinical data were collected from the patient’s electronic medical records. Collected data included baseline demographic, clinical, and disease characteristics such as age at diagnosis, gender, race, body mass index (BMI), smoking status, history of nephrectomy prior to initiation of systemic therapy, presence of sarcomatoid features in the histopathological specimen, and IMDC risk score. In addition, results from comprehensive genomic profiling (CGP) with or without available transcriptomic data were obtained as part of their routine clinical care.
Patients were classified into two groups based on investigator-assessed responses to ipilimumab plus nivolumab therapy. Patients with a complete or partial response or stable disease lasting at least six months from treatment initiation were included in the ‘clinical benefit’ group. In contrast, patients experiencing disease progression within six months were included in the ‘no clinical benefit’ group.
Genomic and transcriptomic analysis
Patients included in the study had available CGP with or without associated transcriptomic data obtained as part of routine clinical care. CGP and transcriptomic sequencing was done on tumor samples using archival formalin-fixed, paraffin embedded tissue obtained from nephrectomy, partial nephrectomy, nephroureterectomy, or diagnostic tissue biopsy done as a standard of care prior to the initiation of systemic therapy. The CGP was done by a Clinical Laboratory Improvement Amendments (CLIA) certified next-generation sequencing assay covering a limited panel of genes (324 genes in Foundation Medicine Inc., Cambridge, MA, or 648 genes in Tempus xT, Chicago, IL) or whole exome sequencing (Caris Life Sciences, Irving, TX, or Exact Sciences, Phoenix, AZ). Two hundred eighty-six shared genes among the genomic testing platforms were identified and included in the genomic analysis. Research analysis of the tumor whole transcriptome data was performed for samples with clinical whole transcriptome sequencing available.
Statistical analysis
Pathogenic variants in the prespecified genes identified to be shared between the assays were included in the genomic analysis, and variants of unknown significance were excluded. The frequency of genomic alterations was summarized using descriptive statistics and compared between the two outcomes groups using the chi-square test. Multivariate regression assessed the difference in the two groups’ baseline demographic and clinical variables for disease response.
For the whole transcriptome analysis, FASTQ files were aligned using STAR. Transcript abundance estimates were generated using Salmon (v0.14.1), run in quasimapping mode using the GRCh38 genome build as the reference and additional options of validate Mappings, seqBias, and gcBias. Gene level abundance estimates were produced using Salmon’s geneMap function to map transcript ID to gene name. Transcript abundance normalization and differential gene expression analysis were performed using DESeq2 [19]. Expression profiles were compared between the ‘clinical benefit’ and ‘no-clinical benefit’ groups, with a p-adjusted value of less than 0.1 considered significant after multiple testing corrections. Additionally, to interpret the pathway enrichment in transcriptional data between the cohorts, Gene set enrichment analysis was performed with GSEA v4.2.3 using phenotype classes (clinical benefit and no-clinical benefit), with permutation on gene sets using the cancer hallmarks gene sets from MSigDB [20, 21]. Multiple testing correction was performed using a false discovery rate (FDR) of q < 0.1 to indicate significant enrichment within one phenotype class.
RESULTS
Baseline demographic characteristics
Fifty-three patients met the eligibility criteria and were included in this study. Of those, 37 patients were assigned to the ‘clinical benefit’ group and 16 to the ‘no clinical benefit’ group. Figure 1 shows a flowchart for the patient selection for our study. Clinical characteristics of the patients were similar between the two groups, and no significant differences in age, gender, and race were found (Table 1).
Fig. 1
Figure showing patient selection for the study.
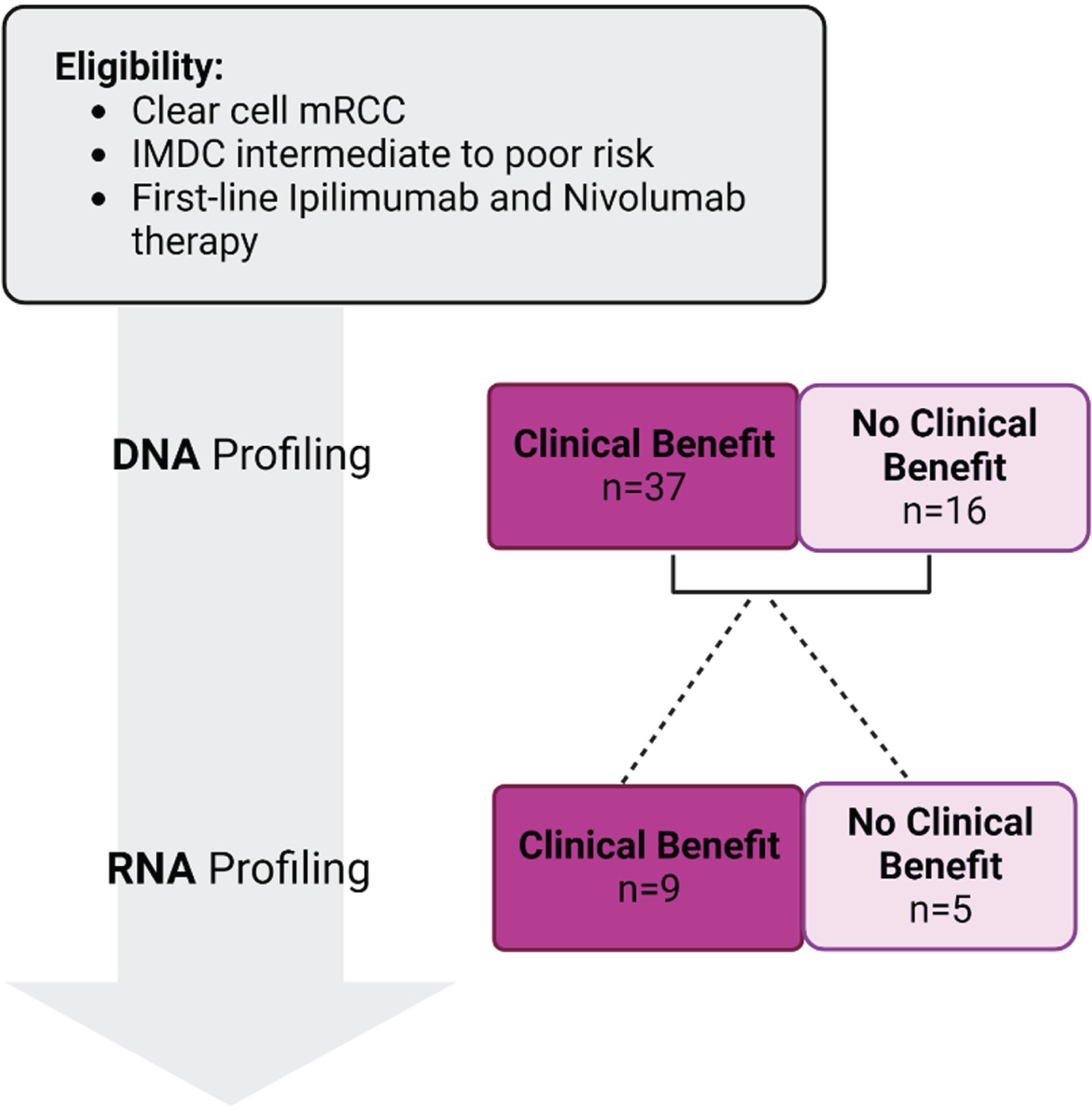
Table 1
Baseline demographic and clinical characteristics of clinical benefit and no clinical benefit cohorts
Clinical Benefit | No Clinical Benefit | P value | |
(N = 37) | (N = 16) | ||
Age at diagnosis, years | |||
Median (range) | 59 (41–82) | 64 (31–82) | 0.43 |
Gender, n (%) | |||
Male | 27 (73) | 12 (75) | 0.28 |
Female | 10 (27) | 4(25) | |
Race, n (%) | |||
White | 32 (86.5) | 14 (87.5) | 0.31 |
Non-White | 5 (13.5) | 2 (12.5) | |
Body mass index, n (%) | |||
≤30 | 17 (46) | 4 (25) | 0.72 |
>30 | 20 (54) | 12 (75) | |
Prior history of smoking, n (%) | |||
Yes | 14 (37.9) | 4 (25) | 0.69 |
No | 23 (62.1) | 12 (75) | |
Nephrectomy, n (%) | |||
Yes | 23 (62.1) | 13 (82.3) | 0.49 |
No | 14 (37.9) | 3 (18.7) | |
Sarcomatoid histology, n (%) | |||
Yes | 3 (8.1) | 2 (12.5) | 0.67 |
No | 34 (91.9) | 14 (87.5) | |
Brain metastases, n (%) | |||
Yes | 3 (8.1) | 0 (0) | 0.25 |
No | 34 (91.9) | 16 (100) | |
Lung metastases, n (%) | |||
Yes | 26 (70.2) | 13 (81.3) | 0.95 |
No | 11 (29.8) | 3 (18.7) | |
IMDC prognostic risk, n (%) | |||
Intermediate | 4 (10.9) | 2 (12.5) | 0.61 |
Poor | 33 (89.1) | 14 (87.5) |
Genomic characteristics
The most frequently altered genes in the overall cohort were VHL (56.6%), PBRM1 (47.1%), and SETD2 (20.7%). The frequency of genomic alterations was not significantly different between the ‘clinical benefit’ and ‘no clinical benefit’ groups. The prevalence of recurrent genomic alterations in VHL (54.1% vs. 62.5%, p = 0.57), PBRM1 (45.9% vs. 50%, p = 0.79), SETD2 (18.9% vs. 25%, p = 0.62) and KDM5C (18.9% vs. 18.8%, p = 0.99) were similar between the ‘clinical benefit and ‘no-clinical benefit’ cohorts. All patients in our cohort had a TMB ≤10 Mut/Mb and did not have evidence of microsatellite instability based on CGP. Supplementary Table 1 and Fig. 2 summarize the frequency of genomic alterations observed among patients in the two groups.
Fig. 2
Genomic alteration frequency among ccRCC patients with clinical benefit compared to no clinical benefit from first line Ipilimumab plus Nivolumab. Abbreviations: CB, clinical benefit; NCB, no clinical benefit.
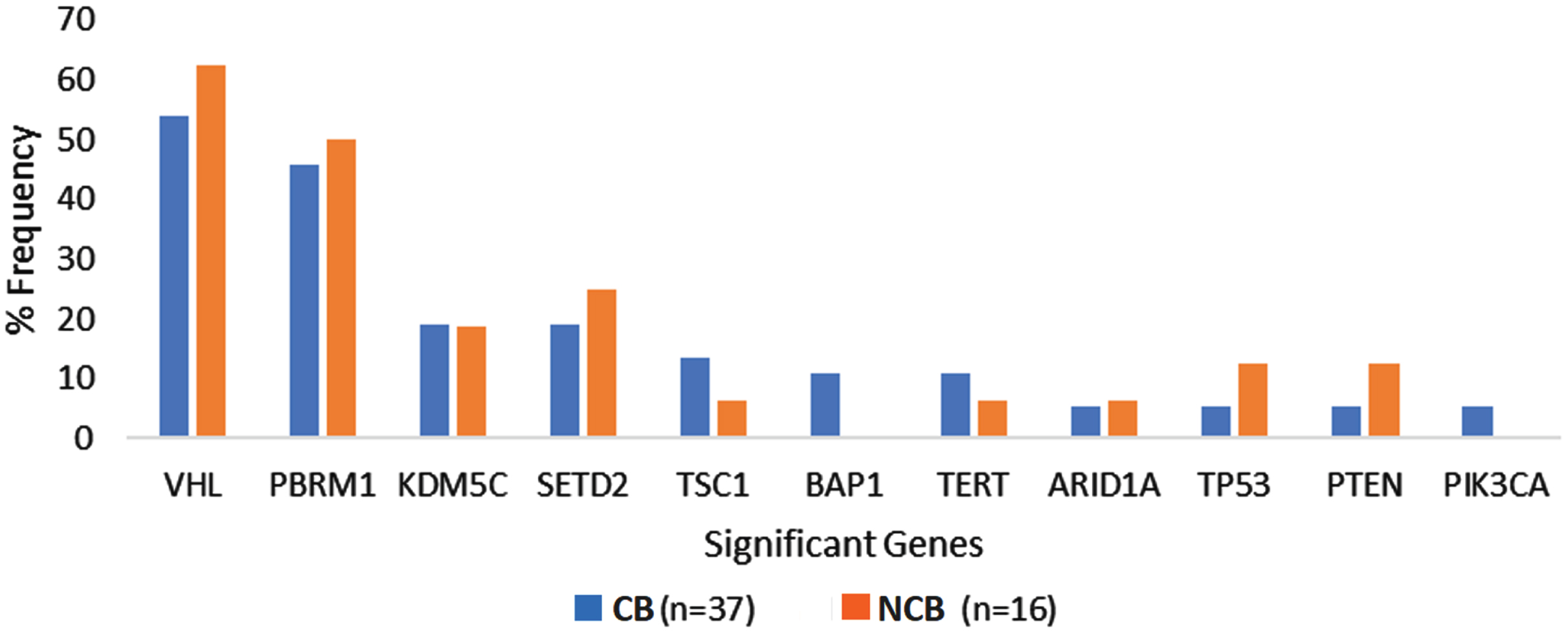
Gene expression analysis
Tumor-based RNA-sequencing data were available for 14 patients. Among these, 9 derived ‘clinical benefit’, while 5 had ‘no clinical benefit’. Forty protein-coding genes were identified as differentially expressed between the two groups (q < 0.1).
Thirty-two genes were overexpressed in the ‘clinical benefit’ group, while eight were overexpressed in the ‘no clinical benefit’ cohort. The most frequently overexpressed genes in patients with ‘clinical benefit’ were NACA2, CTSE, RGS11, MYLK3, and PROX1 (p-value <0001, all), while WFDC12 (p < 0.001), EDN2 (p < 0.001), GJB2 (p < 0.001), XPNPEP2 (p = 0.002), and SCG5 (p = 0.001) were enriched in patients without clinical benefit (Supplementary Table 2 and Supplementary Figure 1). In patients with clinical benefit, pathway analysis utilizing GSEA showed enrichment in the apical surface pathway (NES = 1.67, q = 0.009) and pathways downregulated by KRAS-signaling (NES = 1.68, q = 0.01). In contrast, patients without clinical benefit had upregulation of immunoregulatory pathways such as interferon (IFN) α response (NES = 1.38, q = 0.04), IFN-γ response (NES = 1.49, q = 0.03), TNF-α signaling via NFKB (NES = 2.01, q = 0.001), IL6-JAK-STAT3 signaling (NES = 1.56, q = 0.02), TGF-β signaling (NES = 1.52, q = 0.02), inflammatory response (NES = 1.42, q = 0.03) and reactive oxygen species (NES = 1.75, q = 0.005; Fig. 3 and Supplementary Table 3).
Fig. 3
Signaling pathways enriched in clinical benefit and no clinical benefit groups. Enrichment plot present (A) Apical surface (B) KRAS Signaling (C) Interferon-γ (D) IL6JAK-STAT3 signaling (E) Reactive Oxygen Species (F) TNF-α Signaling via NFKB.
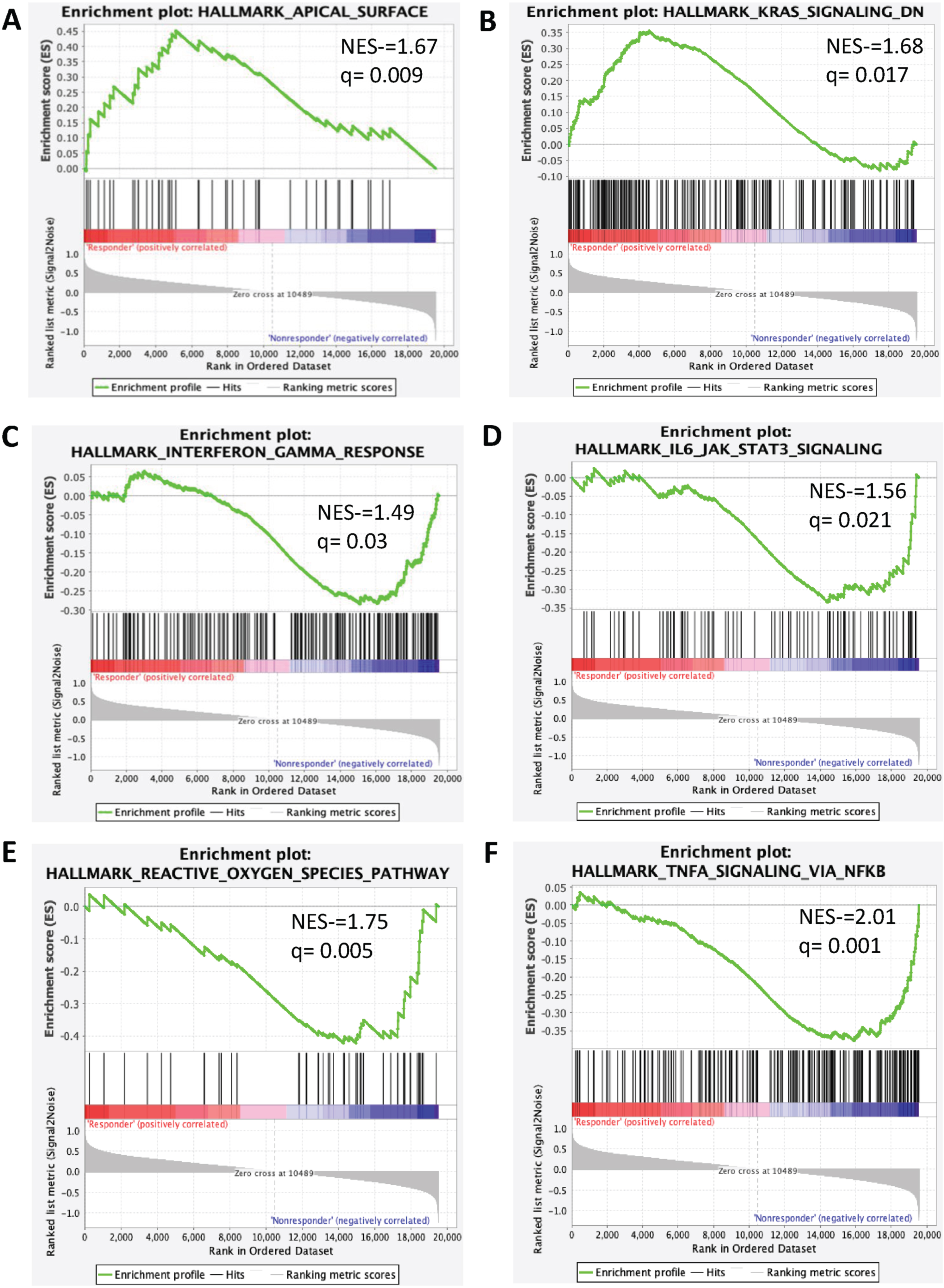
DISCUSSION
Through our study, we evaluate both DNA and RNA based biomarkers in real-world metastatic ccRCC patient population receiving treatment with first-line ipilimumab and nivolumab therapy. In our study, no significant differences in baseline and clinical characteristics or the frequency of genomic alterations were observed between patients deriving benefit or no benefit from ipilimumab plus nivolumab. However, we did observe distinct transcriptomic profiles in mRCC patients who experienced clinical benefit with ipilimumab and nivolumab compared to those who did not.
The frequency of most prevalent genomic alterations described in patients with mRCC, such as PBRM1 and VHL alterations were similar to the frequency observed in the overall cohort of our study [22, 23]. Further, the lack of difference in the frequency of genomic alterations between the two cohorts mirrored the findings of previous reports where the efficacy of ICIs containing regimens were independent of genomic alterations among mRCC patients [12, 16, 22, 23].
Our gene expression analyses showed that patients who benefited clinically from ipilimumab and nivolumab therapy exhibited enrichment of the apical surface signaling and pathways downregulated by KRAS signaling. The apical surface pathway codes for membrane proteins in the apical domain of cells, which maintain cellular polarity [24]. Although there is a paucity of evidence correlating the role of the apical surface pathway in mRCC, loss of cellular polarity has been linked to oncogenesis and cancer progression [24, 25]. On the other hand, KRAS, a member of the RAS family of genes, plays a crucial role in cellular proliferation, differentiation, and survival [26]. Pathways downregulated due to KRAS signaling such as AKT-mTOR and MAPK signaling, are known to upregulate PD-L1 expression in cancer cells [26]. This may potentially explain the fact that tumors enriched with pathways downregulated by KRAS signaling are more sensitive to immune checkpoint inhibitors [27]. However, KRAS alteration is rarely observed in mRCC, unlike in other tumor types, such as colorectal, lung, and melanoma tumors [28]. Further studies are needed to explore the role of KRAS signaling and the response to immune checkpoint inhibitors in the context of mRCC.
Our study identified several pathways, including interferon (IFN)-α, IFN-γ, reactive oxygen species, MYC target V1, and adipogenesis, which were enriched in the ‘no clinical benefit’ cohort. The IFN signaling system is essential in modulating tumor immunogenicity and has been known to enhance tumor sensitivity to ICIs by inducing PD-L1 expression [29]. While PD-L1 expression may promote tumor susceptibility to ICIs, aberrant IFN-γ signaling-induced adaptive PD-L1 expression on cancer cells also results in T cell inactivation, which mediates resistance to anti-CTLA-4 therapy or PD1/PD-L1 inhibitors [30]. Further, our study provided insight into several metabolic pathways, including glycolysis, adipogenesis, and reactive oxygen species, which were enriched in the ‘no clinical benefit’ cohort. Our study’s findings align with the exploratory analysis from the CheckMate214 trial, which reported the enrichment of pathways such as adipogenesis, Myc target V1, reactive oxygen species, and IFN γ and IFN α pathways among ipilimumab and nivolumab-treated patients who had a shorter PFS of less than 18 months [31].
Several pro-tumor immunoregulatory pathways, including IL-6-JAK-STAT3, TNFα via NFKB signaling, TGF-β signaling, inflammatory response, heme metabolism, epithelial-mesenchymal transition, and myogenesis, were also enriched among patients who did not benefit clinically from ipilimumab and nivolumab therapy, in our study.
Enhanced IL-6-JAK-STAT3 in conjunction with IFN α and IFN γ signaling is known to impair cytotoxic T-cell activation resulting in a diminished response to ICIs in clear cell renal cell carcinoma [32]. Gene expression analyses have reported the overexpression of inflammatory and immune-related signatures among patients who respond to immunotherapy or immunotherapy-containing regimens across different cancers [33–35]. Interestingly, our findings contrasted with the biomarker analysis of the CheckMate 214 trial, where the upregulation of these pathways was associated with prolonged progression-free survival in the ipilimumab and nivolumab arm [36]. Possible grounds for the observed discrepancy could be intratumor heterogeneity and a small sample size of patients with available pre-treatment tumor-based RNA sequencing. These findings necessitate the need to investigate such correlation in further biomarker studies. Prospective interrogation and clinical validation of these findings may help identify biomarkers predictive of a durable response to ipilimumab and nivolumab therapy.
The results from our study provide insight into the role of biological processes occurring in the tumor microenvironment of renal cell tumors and the response to ipilimumab and nivolumab therapy. Our study was limited by its retrospective nature and the small sample size.
Notwithstanding these limitations, the strength of our study lies in the patient population. Our study is the first to date to have interrogated RNA-based markers to predict response to ipilimumab and nivolumab therapy among real-world mRCC patients.
CONCLUSION
Our study aimed to explore the genomic and transcriptomic profiles of mRCC patients as biomarkers of response to front-line ipilimumab and nivolumab. Patients who derived clinical benefit did not have any significant difference in the frequency of genomic alterations compared to those who did not. However, our gene expression analysis identified distinct pathways upregulated in the ‘clinical benefit’ group, suggesting that transcriptomic analysis could help identify patients who could selectively benefit from this doublet ICI-based therapy. While further studies are necessary to validate these findings, identifying predictive biomarkers for treatment selection and designing clinical trials for this patient population is crucial.
ACKNOWLEDGMENTS
The authors have no acknowledgments.
FUNDING
The authors report no funding.
AUTHOR CONTRIBUTIONS
All authors contributed to the study conception and design. Material preparation, data collection and analysis were performed by Nishita Tripathi, Luis Meza, Sara Byron, and Jiaming Zhang. The first draft of the manuscript was written by Nishita Tripathi and all authors commented on previous versions of the manuscript. All authors read and approved the final manuscript. All authors had access to the data used for the manuscript.
ETHICS APPROVAL
The study was approved (or granted exemption) by the appropriate institutional and/or national research ethics committee (Huntsman Cancer Institute, University of Utah, and City of Hope Comprehensive Cancer Center IRB).
CONFLICTS OF INTEREST
Sumanta K. Pal reports consulting/advisory role for Novartis, Medivation, Astellas Pharma, Pfizer, Aveo, Myriad, Genentech, Exelixis, Bristol-Myers Squibb, and Astellas. Sumanta K. Pal is an Editorial Board Member of this journal, but was not involved in the peer-review process of this paper, nor had access to any information regarding its peer-review.
Neeraj Agarwal (lifetime disclosures): Consultancy to Astellas, Astra Zeneca, Aveo, Bayer, Bristol Myers Squibb, Calithera, Clovis, Eisai, Eli Lilly, EMD Serono, Exelixis, Foundation Medicine, Genentech, Gilead, Janssen, Merck, MEI Pharma, Nektar, Novartis, Pfizer, Pharmacyclics, and Seattle Genetics. Research funding to Neeraj Agarwal’s institution: Arnivas, Astellas, Astra Zeneca, Bavarian Nordic, Bayer, Bristol Myers Squibb, Calithera, Celldex, Clovis, Crispr, Eisai, Eli Lilly, EMD Serono, Exelixis, Genentech, Gilead, Glaxo Smith Kline, Immunomedics, Janssen, Lava, Medivation, Merck, Nektar, Neoleukin, New Link Genetics, Novartis, Oric, Pfizer, Prometheus, Rexahn, Roche, Sanofi, Seattle Genetics, Takeda, and Tracon. Neeraj Agarwal is an Editorial Board Member of this journal, but was not involved in the peer-review process of this paper, nor had access to any information regarding its peer-review.
Umang Swami reports consultancy to Astellas, Exelixis and Seattle Genetics and research funding to institute from Janssen, Exelixis and Astellas/Seattle Genetics.
Benjamin L. Maughan reports consulting/advisory role to Janssen Oncology, Exelixis, Tempus, Bristol-Myers Squibb, Astellas Medivation, Bayer, AVEO, Clovis Oncology, Merck, Peloton Therapeutics, Pfizer, Bavarian Nordic, Sanofi. Travel, accommodation, expenses: Exelixis. Research funding to institution: Clovis Oncology, Bristol-Myers Squibb, Bavarian Nordic, Exelixis.
Arpita Desai reports consulting/advisory role to Janssen Oncology, Exelixis, Merck, Dendreon. Research funding to institution: Janssen Oncology, Merck Nishita Tripathi, Luis Meza, Nicolas Sayegh, Ameish Govindarajan, Sara A. Byron, Jiaming Zhang, Beverly Chigarira, Yeonjung Jo, Zeynep B. Zengin, Haoran Li, Georges Gebrael have no conflicts of interest to disclose.
DATA AVAILABILITY
The datasets generated during and/or analyzed during the current study are available from the corresponding author on reasonable request.
SUPPLEMENTARY MATERIAL
[1] The Supplementary Figures and Tables are available in the electronic version of this article: https://dx.doi.org/10.3233/KCA-230011.
REFERENCES
[1] | Rini BI , Plimack ER , Stus V , Gafanov R , Hawkins R , Nosov D , et al. Pembrolizumab plus Axitinib versus Sunitinib for Advanced Renal-Cell Carcinoma, N Engl J Med (2019) ;380: (12):1116–1127. |
[2] | Choueiri TK , Powles T , Burotto M , Escudier B , Bourlon MT , Zurawski B , et al. Nivolumab plus Cabozantinib versus Sunitinib for Advanced Renal-Cell Carcinoma, N Engl J Med (2021) ;384: (9):829–841. |
[3] | Motzer R , Alekseev B , Rha S-Y , Porta C , Eto M , Powles T , et al. Lenvatinib plus pembrolizumab or everolimus for advanced renal cell carcinoma, N Engl J Med (2021) ;384: (14):1289–1300. |
[4] | Choueiri TK , Motzer RJ , Rini BI , Haanen J , Campbell MT , Venugopal B , et al. Updated efficacy results from the JAVELIN Renal 101 trial: First-line avelumab plus axitinib versus sunitinib in patients with advanced renal cell carcinoma, Ann Oncol Off J Eur Soc Med Oncol (2020) ;31: (8):1030–1039. |
[5] | FDA approves nivolumab plus ipilimumab combination for intermediate or poor-risk advanced renal cell carcinoma [Internet]. Available from: https://www.fda.gov/drugs/resources-informationapproved-drugs/fda-approves-nivolumab-plusipilimumab-combination-intermediate-or-poor-riskadvanced-renal-cell |
[6] | Motzer RJ , Tannir NM , McDermott DF , Arén Frontera O , Melichar B , Choueiri TK , et al. Nivolumab plus Ipilimumab versus Sunitinib in Advanced Renal-Cell Carcinoma, N Engl J Med (2018) ;378: (14):1277–1290. |
[7] | Shah NJ , Sura SD , Shinde R , Shi J , Singhal PK , Robert NJ , et al. Real-world treatment patterns and clinical outcomes for metastatic renal cell carcinoma in the current treatment era, Eur Urol open Sci (2023) ;49: :110–118. |
[8] | Ernst MS , Navani V , Wells JC , Donskov F , Basappa N , Labaki C , et al. Outcomes for international metastatic renal cell carcinoma database consortium prognostic groups in contemporary first-line combination therapies for metastatic renal cell carcinoma. Eur Urol. 2023. |
[9] | Miao D , Margolis CA , Gao W , Voss MH , Li W , Martini DJ , et al. Genomic correlates of response to immune checkpoint therapies in clear cell renal cell carcinoma, Science (2018) ;359: (6377)801–6. |
[10] | Braun DA , Ishii Y , Walsh AM , Van Allen EM , Wu CJ , Shukla SA , et al. Clinical validation of PBRM1 alterations as a marker of immune checkpoint inhibitor response in renal cell carcinoma, JAMA Oncol (2019) ;5: (11):1631–1633. |
[11] | Liu X-D , Kong W , Peterson CB , McGrail DJ , Hoang A , Zhang X , et al. PBRM1 loss defines a nonimmunogenic tumor phenotype associated with checkpoint inhibitor resistance in renal carcinoma, Nat Commun (2020) ;11: (1):2135. |
[12] | McDermott DF , Huseni MA , Atkins MB , Motzer RJ , Rini BI , Escudier B , et al. Clinical activity and molecular correlates of response to atezolizumab alone or in combination with bevacizumab versus sunitinib in renal cell carcinoma, Nat Med (2018) ;24: (6):749–757. |
[13] | Becht E , Giraldo NA , Beuselinck B , Job S , Marisa L , Vano Y , et al. Prognostic and theranostic impact of molecular subtypes and immune classifications in renal cell cancer (RCC) and colorectal cancer (CRC), Oncoimmunology (2015) ;4: (12):e1049804. |
[14] | Beuselinck B , Job S , Becht E , Karadimou A , Verkarre V , Couchy G , et al. Molecular subtypes of clear cell renal cell carcinoma are associated with sunitinib response in the metastatic setting, Clin cancer Res an Off J Am Assoc Cancer Res (2015) ;21: (6):1329–1339. |
[15] | Vano Y-A , Elaidi R , Bennamoun M , Chevreau C , Borchiellini D , Pannier D , et al. Nivolumab, nivolumab-ipilimumab, and VEGFR-tyrosine kinase inhibitors as first-line treatment for metastatic clear-cell renal cell carcinoma (BIONIKK): A biomarker-driven, open-label, non-comparative, randomised, phase 2 trial, Lancet Oncol (2022) ;23: (5):612–624. |
[16] | Motzer RJ , Robbins PB , Powles T , Albiges L , Haanen JB , Larkin J , et al. Avelumab plus axitinib versus sunitinib in advanced renal cell carcinoma: Biomarker analysis of the phase 3 JAVELIN Renal 101 trial, Nat Med (2020) ;26: (11):1733–1741. |
[17] | Krishna C , DiNatale RG , Kuo F , Srivastava RM , Vuong L , Chowell D , et al. Single-cell sequencing links multiregional immune landscapes and tissue-resident T cells in ccRCC to tumor topology and therapy efficacy, Cancer Cell (2021) ;39: (5):662–677.e6. |
[18] | Motzer RJ , Banchereau R , Hamidi H , Powles T , McDermott D , Atkins MB , et al. Molecular Subsets in Renal Cancer Determine Outcome to Checkpoint and Angiogenesis Blockade, Cancer Cell (2020) ;38: (6):803–817.e4. |
[19] | Love MI , Huber W , Anders S Moderated estimation of fold change and dispersion for RNA-seq data with DESeq2, Genome Biol (2014) ;15: (12):550. |
[20] | Mootha VK , Lindgren CM , Eriksson K-F , Subramanian A , Sihag S , Lehar J , et al. PGC-1alpha-responsive genes involved in oxidative phosphorylation are coordinately downregulated in human diabetes, Nat Genet (2003) ;34: (3):267–273. |
[21] | Subramanian A , Tamayo P , Mootha VK , Mukherjee S , Ebert BL , Gillette MA , et al. Gene set enrichment analysis: A knowledge-based approach for interpreting genome-wide expression profiles, Proc Natl Acad Sci U S A (2005) ;102: (43):15545–15550. |
[22] | Braun DA , Hou Y , Bakouny Z , Ficial M , Sant’ Angelo M , Forman J , et al. Interplay of somatic alterations and immune infiltration modulates response to PD-1 blockade in advanced clear cell renal cell carcinoma, Nat Med (2020) ;26: (6):909–918. |
[23] | Gerlinger M , Horswell S , Larkin J , Rowan AJ , Salm MP , Varela I , et al. Genomic architecture and evolution of clear cell renal cell carcinomas defined by multiregion sequencing, Nat Genet (2014) ;46: (3):225–233. |
[24] | Coradini D , Casarsa C , Oriana S . Epithelial cell polarity and tumorigenesis: New perspectives for cancer detection and treatment, Acta Pharmacol Sin (2011) ;32: (5):552–564. |
[25] | Gandalovicová A , Vomastek T , Rosel D , Brábek J . Cell polarity signaling in the plasticity of cancer cell invasiveness, Oncotarget (2016) ;7: (18):25022–25049. |
[26] | Hamarsheh S , Groß O , Brummer T , Zeiser R . Immune modulatory effects of oncogenic KRAS in cancer, Nat Commun (2020) ;11: (1):5439. |
[27] | Sciortino C , Viglialoro V , Nucci M , Polito MG , Cortesi E , Gelibter A et al. Response to immunotherapy in KRAS G12C mutated NSCLC: A single-centre retrospective observational study, Oncotarget (2022) ;13: :686–693. |
[28] | Lee JK , Sivakumar S , Schrock AB , Madison R , Fabrizio D , Gjoerup O , et al. Comprehensive pan-cancer genomic landscape of KRAS altered cancers and real-world outcomes in solid tumors, NPJ Precis Oncol (2022) ;6: (1):91. |
[29] | Benci JL , Johnson LR , Choa R , Xu Y , Qiu J , Zhou Z , et al. Opposing functions of interferon coordinate adaptive and innate immune responses to cancer immune checkpoint blockade, Cell (2019) ;178: (4):933–948.e14. |
[30] | Nikoo M , Rabiee F , Mohebbi H , Eghbalifard N , Rajabi H , Yazdani Y , et al. Nivolumab plus ipilimumab combination therapy in cancer: Current evidence to date, Int Immunopharmacol (2023) ;117: :109881. |
[31] | Motzer RJ , Choueiri TK , McDermott DF , Powles T , Yao J , Ammar R ,et al. Biomarker analyses from the phase III CheckMate 214 trial of nivolumab plus ipilimumab (N+I) or sunitinib (S) in advanced renal cell carcinoma (aRCC), J Clin Oncol [Internet] (2020) ;38: (15_suppl):5009. Available from: https://doi.org/10.1200/JCO.2020.38.15_suppl.5009. |
[32] | Kruk L , Mamtimin M , Braun A , Anders H-J , Andrassy J , Gudermann T , et al. Inflammatory networks in renal cell carcinoma. Cancers. 2023;15. |
[33] | Riaz N , Havel JJ , Makarov V , Desrichard A , Urba WJ , Sims JS , et al. Tumor and microenvironment evolution during immunotherapy with nivolumab, Cell (2017) ;171: (4):934–949.e16. |
[34] | Lei M , Siemers NO , Pandya D , Chang H , Sanchez T , Harbison C et al. Analyses of PD-L1 and inflammatory gene expression association with efficacy of nivolumab±ipilimumab in gastric cancer/gastroesophageal junction cancer, Clin cancer Res an Off J Am Assoc Cancer Res (2021) ;27: (14):3926–3935. |
[35] | Hodi FS , Wolchok JD , Schadendorf D , Larkin J , Long GV , Qian X , et al. TMB and inflammatory gene expression associated with clinical outcomes following immunotherapy in advanced melanoma, Cancer Immunol Res (2021) ;9: (10):1202–1213. |
[36] | Motzer RJ , Choueiri TK , McDermott DF , Powles T , Vano Y-A , Gupta S , et al. Biomarker analysis from CheckMate 214: Nivolumab plus ipilimumab versus sunitinib in renal cell carcinoma, J Immunother cancer (2022) ;10: (3). |