Logical Imputation to Optimize Prognostic Risk Classification in Metastatic Renal Cell Cancer
Abstract
BACKGROUND:
Application of the MSKCC and IMDC models is recommended for prognostication in metastatic renal cell cancer (mRCC). Patient classification in MSKCC and IMDC risk groups in real-world observational studies is often hampered by missing data on required pre-treatment characteristics.
OBJECTIVE:
To evaluate the effect of application of easy-to-use logical, or deductive, imputation on MSKCC and IMDC risk classification in an observational study setting.
PATIENTS AND METHODS:
We used data on 713 mRCC patients with first-line sunitinib treatment from our observational European multi-centre study EuroTARGET. Pre-treatment characteristics and follow-up were derived from medical files. Hospital-specific cut-off values for laboratory measurements were requested. The effect of logical imputation of missing data and consensus versus hospital-specific cut-off values on patient classification and the subsequent models’ predictive performance for progression-free and overall survival (OS) was evaluated.
RESULTS:
45% of the patients had missing data for≥1 pre-treatment characteristic for either model. Still, 72% of all patients could be unambiguously classified using logical imputation. Use of consensus instead of hospital-specific cut-offs led to a shift in risk group for 12% and 7% of patients for the MSKCC and IMDC model, respectively. Using logical imputation or other cut-offs did not influence the models’ predictive performance. These were in line with previous reports (c-statistic ∼0.64 for OS)
CONCLUSIONS:
Logical imputation leads to a substantial increase in the proportion of patients that can be correctly classified into poor and intermediate MSKCC and IMDC risk groups in observational studies and its use in the field should be advocated.
INTRODUCTION
The Memorial Sloan Kettering Cancer Center (MSKCC) model and the International Metastatic Renal Cell Carcinoma Database Consortium (IMDC) model were developed to facilitate prognostication in metastatic renal cell carcinoma (mRCC). Given the absence of markers that predict therapy response, the treatment guideline of the European Association of Urology advocates the use of either the MSKCC or the IMDC model for patient counselling and choice of therapy in mRCC [1]. These models are also used for mRCC patient selection in clinical studies and for prognostic characterization of patients in real-world observational studies. Both models categorize patients into a favourable, intermediate, and poor risk group. Categorization of patients in the MSKCC model is based on the presence or absence of five risk factors derived from the following pre-treatment characteristics: Karnofsky performance status (KPS), time between diagnosis and treatment, levels of lactate dehydrogenase (LDH), serum hemoglobin and corrected serum calcium [2]. The IMDC model additionally includes risk factors based on platelet and neutrophil counts while LDH is omitted [3]. For ease of use in routine clinical practice, the cut-offs for the laboratory-based characteristics are based on institution-specific lower limits of normal (LLN) and upper limits of normal (ULN) [4].
Data on these risk factors are generally available for mRCC patients in randomized and prospective clinical trials. However, in real-world and observational study settings, values for one or more of these risk factors are often lacking for a substantial number of study participants. A non-systematic literature search showed that a variety of methods is used to deal with these missing values in MSKCC or IMDC risk classification. These include a case-deletion approach with analysis of patients with complete data on all underlying risk factors only (e.g. [3, 5]) and statistical multiple imputation techniques (e.g. [6, 7]). But many papers do not mention any procedure (e.g. [8–10]). Each of these methods has its own disadvantages. Use of a case-wise deletion approach leads to loss of data and possibly to bias in study results. And statistical imputation procedures require advanced skills and software packages and may also lead to the introduction of bias, depending on missingness patterns [11]. To our knowledge, none of the studies that apply the MSKCC or IMDC model report the use of logical imputation, also known as deductive imputation, to classify patients with missing risk factor values. In this approach, missing values are replaced by values that can be unambiguously derived from observed values based on logical or mathematical relationships between these variables. This methodology is suited for imputation of composite scores, like the MSKCC and IMDC models, and can be performed on an individual patient level and without the need for advanced statistical techniques. The fraction of missing data that can be imputed using this approach, will depend on the actual logic that can be applied and the missing data pattern in the data at hand. Nevertheless, using this approach can simplify the process of resolving the remaining missing data [12]. Here, we apply such logical imputation for MSKCC and IMDC risk classification in data of sunitinib-treated mRCC patients from EuroTARGET, a European, multi-centre observational study [13] and evaluate its effect on patient risk classification and the models’ predictive performance.
PATIENTS AND METHODS
Patient population
The EuroTARGET project focused on identifying sunitinib-response biomarkers and started in March 2011. EuroTARGET included 748 mRCC patients who were prospectively recruited after March 2011 in 62 hospitals from the Netherlands, Spain, United Kingdom, Romania, and Germany, and 462 mRCC patients from previous retrospective and prospective studies (‘historical’ patients) resulting in 1210 participants. From these 1210 participants, 713 participants with established mRCC who received sunitinib as first-line tyrosine-kinase inhibitor (TKI) treatment and who had at least 6 months of outcome assessment were selected [13]. The study was approved by the ethics committee at each participating center and all patients gave written informed consent.
Data collection
Design and data collection procedures within EuroTARGET are extensively described elsewhere [13]. Clinical information (baseline and follow-up) from all patients was collected from medical files via web-based case record forms (CRFs). Missing and inconsistent data were assessed and resolved by reviewing the CRFs or the medical files. Exact cleaning steps are described in the supplementary files of van der Zanden et al. [13]. For this study, we extracted data on baseline clinical characteristics including all pre-treatment characteristics of the MSKCC and IMDC models (see Supplementary Table 1), treatment lines, tumour response according to RECIST v1.1, and death dates.
MSKCC and IMDC model classification
Patients with complete data on all pre-treatment characteristics were scored according to the MSKCC and IMDC models (Supplementary Table 1) [2, 3]. Next, we performed logical imputation in which we also classified patients with missing values on pre-treatment characteristics but with sufficient information for an unambiguous classification for either of the models. The applied logic for the IMDC model is presented in Fig. 1. In short, if a patient had one known risk factor and only one missing factor he/she could be unambiguously classified into the intermediate risk group. If a patient had three known risk factors, then, regardless of the number of remaining missing factors, the patient could be classified as poor risk. Only for a favourable risk group classification, all risk factors have to be known. Depending on the combination of known risk factors and remaining missing factors, additional classifications of patients can be obtained (see Fig. 1 for IMDC and Supplementary Figure 1 for the MSKCC risk model). Some illustrative examples of patients for the IMDC model are given in Supplementary Table 2 and for the MSKCC model in Supplementary Table 3.
Fig. 1
Rules for logical imputation of the International Metastatic Renal Cell Carcinoma Database Consortium (IMDC) risk groups. The bold outlined boxes are the additional classifications obtained by using this imputation procedure. Abbreviations: LLN, lower limit of normal; ULN, upper limit of normal.
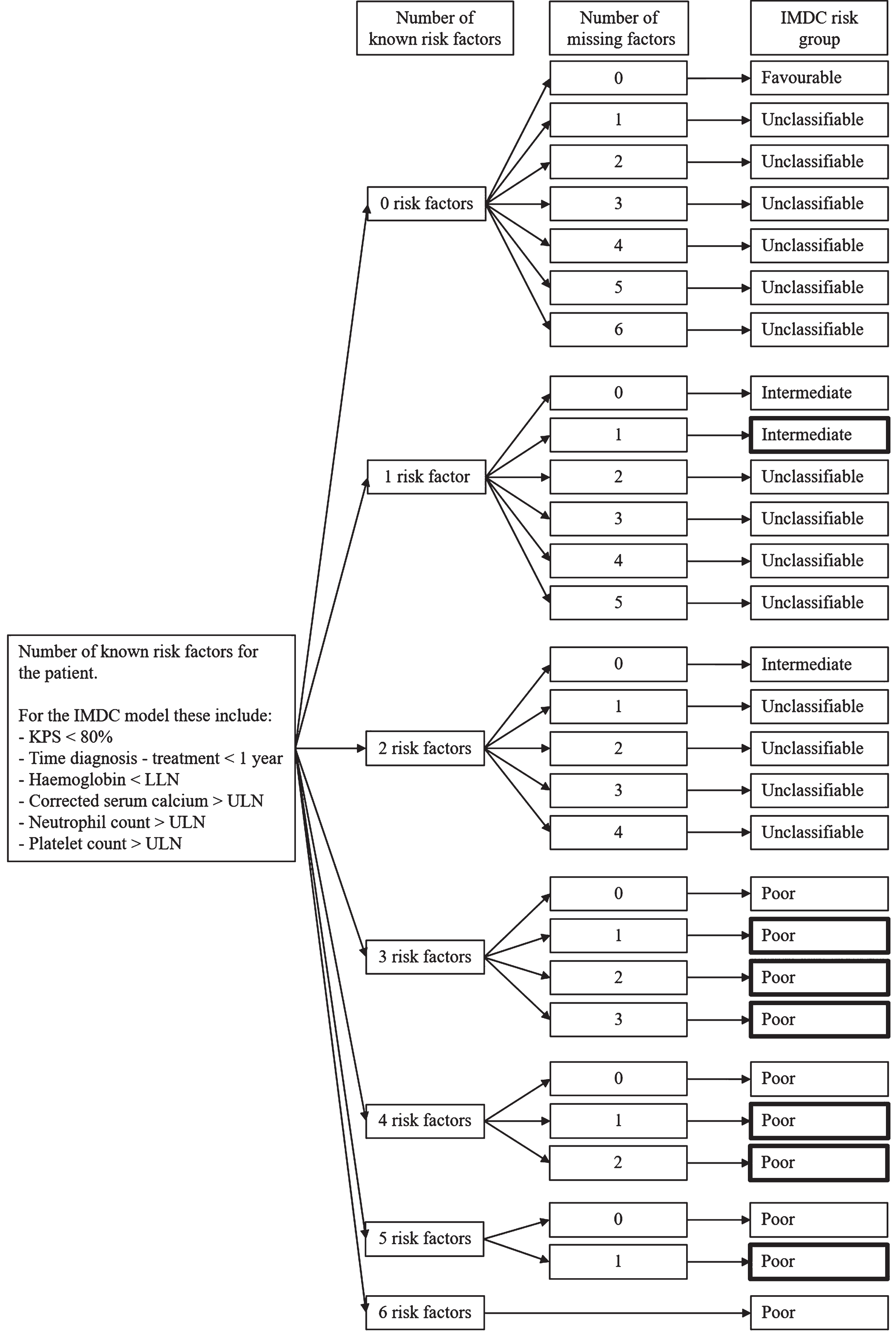
Absolute values for pre-treatment laboratory-based characteristics were entered via CRFs. Lower and upper levels of normal are required to obtain the individual patients’ risk factors for LDH, haemoglobin, calcium, and platelet and neutrophil counts. Hereto, we followed two strategies: (1) obtain hospital-specific LLN and ULN cut-offs from the 62 hospitals that contributed to the prospective patient recruitment after March 2011; for historical patients, this was not feasible; (2) come to a consensus on EuroTARGET-wide cut-offs. We obtained relevant cut-offs for 49 out of 62 hospitals, with other hospitals not responding to our repeated requests. The values showed large variation among the hospitals, especially for corrected calcium levels (5.24 to 11.0 g/dL), neutrophil count (4.8 to 9.0×109/L), and LDH (190 to 495 IU/L) (see Supplementary Table 4). For consensus EuroTARGET cut-offs, we reviewed reported cut-off values in publications on the predictive performance of MSKCC and IMDC models up to the year 2016 and those described in national guidelines on the use of pharmaceuticals [6, 9, 14–16]. Literature showed no variation for haemoglobin (LLN 13 g/dL males, 11.5 g/dL females) and corrected serum calcium (ULN 10 mg/dL). However, values varied for platelet count (ULN 400–450×103), neutrophil count (ULN 4.5–7.2×109/L) and LDH (ULN 225–230 IU/L) [6, 9, 14, 16–18]. Four experts from the EuroTARGET team, including a medical oncologist, a pharmacologist, an epidemiologist, and a molecular biologist, agreed upon the consensus cut-off values for the current study based on both the values found in literature and on the hospital-specific cut-off values (see Supplementary Table 4). We classified patients using both consensus and hospital-specific cut-off values.
Clinical outcomes
Overall survival (OS) was defined as the time (in months) between the first day of sunitinib treatment and the date of death or censoring. Progression-free survival (PFS) was defined as the time (in months) between first day of sunitinib treatment and disease progression, relapse, development of a new lesion, death, or censoring (whichever happened first), regardless of the duration of sunitinib treatment. Tumour response was defined according to RECIST version 1.1 and based on patient evaluation by local caregivers as given in the radiology report or medical record (no review of imaging). Patients without events were censored at the date at which patients were last known to be alive or end of study, whichever came first [13].
Statistical analyses
Patient characteristics were described using medians and ranges, or total numbers and percentages. Both prognostic models were examined for associations with OS and PFS using log-rank tests. Survival curves with median survival times and interquartile ranges (IQR) were created using Kaplan-Meier analyses. Univariable Cox proportional hazards regression analyses were performed on all risk groups. Proportional hazards were examined using log-log plots (not presented). Discriminative performance was evaluated using Harrell’s Concordance statistic (c-statistic); 95% confidence interval (CI) for c-statistics were created by bootstrapping 250 simulated datasets, using sampling with replacement. Calibration was assessed by comparing observed Kaplan-Meier curves with predicted Cox regression curves [19]. All statistical analyses were performed in STATA 11.2 for Windows.
RESULTS
Patient characteristics
Patient characteristics of the 713 included EuroTARGET patients are summarized in Table 1. The median OS was 27.5 months (IQR 10.8–51.2) and the median PFS was 11.1 months (IQR 4.6–26.0).
Table 1
Characteristics of the included EuroTARGET population (n = 713)
Variable | Number of patients (%) |
Age (years) at initiation of sunitinib treatment; median (range) | 63 (20–87) |
Sex | |
Males | 525 (73.6) |
Females | 188 (26.4) |
Histology subtype* | |
Clear cell carcinoma | 620 (87.0) |
Non-clear cell carcinoma | 66 (9.2) |
Unknown | 27 (3.8) |
Nr of metastases at start sunitinib | |
1 | 268 (37.6) |
2 | 248 (34.8) |
3 or more | 195 (27.3) |
Unknown | 2 (0.3) |
Prior nephrectomy | |
Yes | 534 (74.9) |
No | 170 (25.1) |
Time from diagnosis to sunitinib treatment≤1 year | |
Yes | 414 (58.1) |
No | 299 (41.9) |
WHO status≥1 | |
Yes | 310 (43.5) |
No | 259 (36.3) |
Unknown | 144 (20.2) |
Haemoglobin < lower limit of normal# | |
Yes | 272 (38.2) |
No | 400 (56.1) |
Unknown | 41 (5.7) |
Corrected calcium > upper limit of normal# | |
Yes | 119 (16.7) |
No | 369 (51.7) |
Unknown | 225 (31.6) |
Neutrophil count > upper limit of normal# | |
Yes | 75 (10.5) |
No | 514 (72.1) |
Unknown | 124 (17.4) |
Platelet count > upper limit of normal# | |
Yes | 107 (15.0) |
No | 557 (78.1) |
Unknown | 49 (6.9) |
High LDH > 1.5 times upper limit of normal# | |
Yes | 121 (17.0) |
No | 496 (69.6) |
Unknown | 96 (13.4) |
Time on sunitinib (months); median (IQR) | 9.0 (4.0–20.0) |
Median follow-up time in months; median (IQR) | 17.0 (9.0–33.4) |
Median OS time in months; median (IQR) | 27.5 (10.8–51.2) |
Median PFS time in months; median (IQR) | 11.1 (4.6–26.0) |
Abbreviations: LDH, lactate dehydrogenase; WHO, World Health Organization; IQR, interquartile range; OS, Overall Survival; PFS, Progression-free Survival. *Histology subtype as defined by a central pathologists; if not available, subtype as defined by a local pathologist was used. #Based on EuroTARGET consensus cut-offs as presented in Supplementary Table 4.
Missing values and patient classification
Information on corrected serum calcium was missing for 225 patients (31.6%), on WHO performance status for 144 patients (20.2%), and on neutrophil count for 124 patients (17.4%) (Table 1). Data on all pre-treatment characteristics (‘complete cases’) for MSKCC and IMDC models were available for 396 (55.5%) and 387 (54.3%) patients, respectively (see Supplementary Table 5). A total of 117 (16.4%) and 135 (18.9%) patients had at least one missing variable for MSKCC and IMDC pre-treatment model characteristics, respectively, but could nonetheless be unambiguously classified into the intermediate or poor risk group via logical imputation. This resulted in 513 (72%) classifiable patients for the MSKCC and 522 (73%) for the IMDC model (Table 2).
Table 2
MSKCC and IMDC risk group classification based on a complete case and logical imputation approach and using consensus cut-off values for laboratory measurements*
MSKCC risk group, number of patients (%) | ||||
Favourable | Intermediate | Poor | Total | |
Complete cases only | 58 (15%) | 206 (52%) | 132 (33%) | 396 (56% of 713) |
Logical imputation | 58 (11%) | 272 (53%) | 183 (36%) | 513 (72% of 713) |
IMDC risk group, number of patients (%) | ||||
Favourable | Intermediate | Poor | Total | |
Complete cases only | 65 (17%) | 188 (48%) | 134 (35%) | 387 (54% of 713) |
Logical imputation | 65 (13%) | 247 (47%) | 210 (40%) | 522 (73% of 713) |
Abbreviations: MSKCC, Memorial Sloan Kettering Cancer Center; IMDC, International Metastatic Renal Cell Carcinoma Database Consortium. *Based on consensus cut-off values as presented in Supplementary Table 4.
Consensus versus hospital-specific cut-off values
Supplementary Table 4 presents the consensus cut-off values and the minimum, mode and maximum cut-off values of participating hospitals. The frequency of MSKCC and IMDC risk factors according to the EuroTARGET consensus cut-offs for all available 713 patients is given in Supplementary Table 6. A total of 245 and 242 patients could be classified using the hospital-specific cut-off values and MSKCC and IMDC model, respectively. The use of hospital-specific instead of consensus cut-off values resulted in a shift in risk group classification for 29 of the 245 patients (11.8%) for MSKCC, and 17 of the 242 patients (7.0%) for IMDC (Supplementary Table 6).
Predictive value and impact of missing values and different cut-off values
Table 3 presents c-statistics for OS and PFS for both MSKCC and IMDC models. These were comparable for MSKCC and IMDC. When using only patients without any missing data on pre-treatment characteristics (complete cases) and consensus cut-off values, c-statistics for MSKCC were 0.64 (95% CI: 0.60–0.67) for OS and 0.58 (0.55–0.62) for PFS, and for IMDC 0.64 (95% CI: 0.60–0.67) and 0.59 (0.56–0.63), respectively. Use of logical imputation (and consensus cut-off values) resulted in highly similar c-statistics. Also, the c-statistics for consensus and for hospital-specific cut-offs were the same (Table 3).
Table 3
Discriminative performance of the MSKCC and IMDC models in relation to overall survival and progression-free survival in different scenarios with regard to missing values and consensus versus hospital-specific cut-off values
MSKCC | OS | PFS | |||
Scenarios: | c-statistic | 95% CI | c-statistic | 95% CI | |
Complete cases only and consensus cut-offs* | 0.64 | 0.60–0.67 | 0.58 | 0.55–0.62 | n = 396 |
Logical imputation and consensus cut-offs* | 0.64 | 0.61–0.67 | 0.59 | 0.56–0.61 | n = 513 |
Complete cases only and hospital-specific cut-offs** | 0.63 | 0.59–0.67 | 0.57 | 0.52–0.61 | n = 245 |
Complete cases only and consensus cut-offs for the subset of patients for which hospital-specific cut-offs were available as well* | 0.63 | 0.59–0.67 | 0.57 | 0.52–0.61 | n = 245 |
IMDC | OS | PFS | |||
Scenarios: | c–statistic | 95% CI | c-statistic | 95% CI | |
Complete cases only and consensus cut-offs* | 0.64 | 0.60–0.67 | 0.59 | 0.56–0.63 | n = 387 |
Logical imputation and consensus cut-offs* | 0.64 | 0.61–0.67 | 0.60 | 0.58–0.63 | n = 522 |
Complete cases only and hospital-specific cut-offs** | 0.62 | 0.58–0.66 | 0.57 | 0.53–0.61 | n = 242 |
Complete cases only and consensus cut-offs for the subset of patients for which hospital-specific cut-offs were available as well* | 0.63 | 0.58–0.67 | 0.57 | 0.53–0.61 | n = 242 |
Abbreviations: MSKCC, Memorial Sloan Kettering Cancer Center; IMDC, International Metastatic Renal Cell Carcinoma Database Consortium; OS, Overall Survival; PFS, Progression Free Survival; CI, Confidence Interval. *Consensus cut-off values as presented in Supplementary Table 4. **Hospital-specific cut-off values as presented in Supplementary table 4.
The related calibration plots for OS and PFS are presented in Supplementary Figures 2–7. All models showed a good match between predicted and observed curves for OS and PFS, except for the favourable OS risk group; there, the predicted curve underestimated survival until ∼42 months and overestimated it afterwards.
DISCUSSION
Using data from the EuroTARGET study, we showed that MSKCC and IMDC risk stratification profits from an easy-to-apply logical imputation procedure in which patients with one up to three missing model characteristics can still be classified correctly into the intermediate or poor risk group, depending on the classification model used.
EuroTARGET is a large-scale European observational study including 62 centres from 5 countries. Extensive effort was put in retracing missing values on the pre-treatment characteristics of the MSKCC and IMDC models by scrutinizing medical files. Nevertheless, 45% of the patients had at least one missing value on the pre-treatment characteristics for either model. We are convinced that we optimized the extraction of data available in the medical files and that the current dataset is a realistic representation of the reporting of these characteristics in clinical practice. We saw variability in missingness between countries and centres, reflecting differences in reporting standards. For example, corrected serum calcium was missing for 45% of the Spanish patients and 16% of the Dutch patients. Indeed, variable frequencies of missing data on MSKCC and IMDC model parameters are also seen in other studies [6, 7, 20]. Using logical imputation, we were able to classify ∼17% of EuroTARGET patients with at least one missing value to the poor or intermediate risk group. Logical imputation did not affect the predictive performance in this study on a group level compared to a case-deletion approach; the observed c-statistics in this study for OS for both the MSKCC and IMDC model was 0.64, in line with previous reports [3, 5–7, 9, 17, 21, 22], and indicating low to moderate discriminative performance. The gain of using logical imputation in terms of classifiable patients and predictive performance in other cohorts will vary, depending on the distribution of number of known risk factors and number of missing risk factor variables for the patients in the dataset of study.
Logical imputation allows for increase in the number of correctly classified patients in the poor and intermediate MSKCC/IMDC risk groups but not in the favourable risk group. Its application may hence lead to a study sample with complete data which is not reflective of the originally identified sample. Whether and to what extent omission of unclassified patients in a subsequent complete-case analysis may affect the internal and/or external validity of results, depends on the research question and statistical analysis at hand. Researchers should always carefully examine the effect of missing data on study results and consider if statistical multiple imputation techniques are necessary. In the logical imputation approach, patients will only be classified if an unambiguous decision can be derived from their available data. This in contrast with statistical multiple imputation that creates and uses multiple datasets to deal with uncertainties of imputation. The use of logical imputation prior to statistical multiple imputation techniques will result in a reduction of the missing values that have to be statistically imputed and can simplify the process of analyzing the remaining missing data [12].
The MSKCC and IMDC models require the definition of lower and upper limits of normal for laboratory-based parameters to determine risk factors. These limits may vary among institutes and over time due to differences in measurement methods and reference populations. We indeed saw a large variation for cut-offs for neutrophil count, corrected calcium and LDH. Unfortunately, we experienced that these limits are hard to retrieve in a multi-centre observational study setting. Relying on consensus-based cut-offs instead, led to changes in individual patient classification but only marginally affected the discriminative performance and calibration. Different cut-off values are used between published studies, especially for neutrophil count which ranged from 4.5 to 7.2×109 [6, 9, 14–16]. Various studies also noticed different cut-off values between participating hospitals and mention the use of hospital-specific values for classification of patients [2, 3, 5, 7, 21, 22]. However, several other studies did either not report on this issue [8, 20, 23] or were single centre studies [9, 24].
One limitation of our study is the use of data that is based on sunitinib which is no longer standard of care. However, the presented procedure can be used in any situation where the MSKCC or IMDC models are applied, regardless of treatments.
In summary, MSKCC and IMDC model classification of mRCC patients in observational studies is often hampered by missing data. Here, we demonstrate an easy-to-apply logical imputation procedure. It can be applied prior to application of a statistical multiple imputation procedure and in situations where such a statistical imputation procedure is not possible or appropriate. Real-world, off-protocol cohorts where key variables are missing in substantial numbers of patients are increasingly used to generate evidence for health care decision making, also in mRCC. This underlines the importance of the use of imputation techniques, including the logical one.
ACKNOWLEDGMENTS
We would like to thank all patients who participated and their treating physicians for inviting them.
FUNDING
The research leading to these results has received funding from the European Union’s Seventh Framework Programme (FP7/2007–2013) under grant agreement no 259939.
AUTHOR CONTRIBUTIONS
Jake S.F. Maurits: Conception of the study, analysis and interpretation of the data, writing draft and revisions, final approval of the version submitted
Loes F.M. van der Zanden: Conception of the study, interpretation of the data, writing draft and revisions, supervision, final approval of the version submitted
Meta H.M. Diekstra: Acquisition of the data, revision, final approval of the version submitted
Valentin Ambert: Acquisition of the data, revision, final approval of the version submitted
Daniel Castellano: Acquisition of the data, revision, final approval of the version submitted
Jesus Garcia-Donas: Acquisition of the data, revision, final approval of the version submitted
Rosa Guarch Troyas: Acquisition of the data, revision, final approval of the version submitted
Henk-Jan Guchelaar: Acquisition of the data, revision, final approval of the version submitted
Ulrich Jaehde: Acquisition of the data, revision, final approval of the version submitted
Kerstin Junker: Acquisition of the data, revision, final approval of the version submitted
Anna Martinez-Cardus: Acquisition of the data, revision, final approval of the version submitted
Marius T. Radu: Acquisition of the data, revision, final approval of the version submitted
Cristina Rodriguez-Antona: Acquisition of the data, revision, final approval of the version submitted
Max Roessler: Acquisition of the data, revision, final approval of the version submitted
Anne Warren: Acquisition of the data, revision, final approval of the version submitted
Tim Eisen: Conception of the study, acquisition of the data, revision, final approval of the version submitted
Egbert Oosterwijk: Conception of the study, revisions, final approval of the version submitted
Lambertus A. L. M. Kiemeney: Conception of the study, revisions, supervision, final approval of the version submitted
Sita H. Vermeulen; Conception of the study, interpretation of the data, writing draft and revisions, supervision, final approval of the version submitted
All authors had access to the study data.
CONFLICT OF INTERESTS
T.E. is currently employed by Roche, has received research support from AstraZeneca, Bayer and Pfizer and holds stock in AstraZeneca and Roche; is an Editorial Board Member of this journal, but was not involved in the peer-review process of this paper, nor had access to any information regarding its peer-review. J.G.-D. has received research funding from AstraZeneca, Pierre Fabre, and Pfizer; received personal fees from Clovis Oncology, Astellas, Pierre Fabre, Janssen, MSD, IPSEN, Roche, BMS, and Pfizer; and received nonfinancial support from Astellas, Pierre Fabre, and Pfizer. D.C. is part of the advisory board and received personal fees from Astellas, AstraZeneca, BMS, GSK, IPSEN, Janssen, MSD, Novartis, Pfizer and Roche; and received nonfinancial support from Astellas AstraZeneca, Bayer, BMS, Clovis, Eisai, Exelisis, GSK, IPSEN, Janssen, Lilly, MSD, Pfizer, QED Therapeutics, Roche; and is executive member of the SOGUG foundation with nonfinancial interests. E.O. is an Associate Editor of this journal, but was not involved in the peer-review process of this paper, nor had access to any information regarding its peer-review.
J.M. has no conflict of interest to report. L.vd.Z. has no conflict of interest to report. M.D. has no conflict of interest to report. V.A. has no conflict of interest to report. R.G.T. has no conflict of interest to report. H-J.G. has no conflict of interest to report. U.J. has no conflict of interest to report. K.J has no conflict of interest to report., A.M-C. has no conflict of interest to report. M.R. has no conflict of interest to report. C. R-A. has no conflict of interest to report. M.R. has no conflict of interest to report. A.W. has no conflict of interest to report. L.K. has no conflict of interest to report. S.V. has no conflict of interest to report.
SUPPLEMENTARY MATERIAL
[1] The supplementary material is available in the electronic version of this article: https://dx.doi.org/10.3233/KCA-220007.
REFERENCES
[1] | Ljungberg B , Albiges L , Bedke J , Bex A , Capitanio U , Giles RH , et al. EAU Guidelines on Renal Cell Carcinoma. European Association of Urology. 2021; European Association of Urology. |
[2] | Motzer RJ , Bacik J , Murphy BA , Russo P , Mazumdar M . Interferon-alfa as a comparative treatment for clinical trials of new therapies against advanced renal cell carcinoma. Journal of clinical oncology: official journal of the American Society of Clinical Oncology. (2002) ;20: (1):289–96. |
[3] | Heng DY , Xie W , Regan MM , Warren MA , Golshayan AR , Sahi C , et al. Prognostic factors for overall survival in patients with metastatic renal cell carcinoma treated with vascular endothelial growth factor-targeted agents: results from a large, multicenter study. Journal of clinical oncology: official journal of the American Society of Clinical Oncology. (2009) ;27: (34):5794–9. |
[4] | Donskov F , Heng DY , Rini BI . On-treatment biomarkers in metastatic renal cell carcinoma: towards individualization of prognosis?. Expert Rev Anticancer Ther .(2017) ;17: (2):97–9. |
[5] | Ko JJ , Xie W , Kroeger N , Lee JL , Rini BI , Knox JJ , et al. The International Metastatic Renal Cell Carcinoma Database Consortium model as a prognostic tool in patients with metastatic renal cell carcinoma previously treated with first-line targeted therapy: a population-based study. The Lancet Oncology. (2015) ;16: (3):293–300. |
[6] | Bamias A , Tzannis K , Beuselinck B , Oudard S , Escudier B , Diosynopoulos D , et al. Development and validation of a prognostic model in patients with metastatic renal cell carcinoma treated with sunitinib: a European collaboration. British Journal of Cancer. (2013) ;109: (2):332–41. |
[7] | Heng DY , Xie W , Regan MM , Harshman LC , Bjarnason GA , Vaishampayan UN , et al. External validation and comparison with other models of the International Metastatic Renal-Cell Carcinoma Database Consortium prognostic model: a population-based study. The Lancet Oncology. (2013) ;14: (2):141–8. |
[8] | Gore ME , Szczylik C , Porta C , Bracarda S , Bjarnason GA , Oudard S , et al. Final results from the large sunitinib global expanded-access trial in metastatic renal cell carcinoma. British Journal of Cancer. (2015) ;113: (1):12–9. |
[9] | Kwon WA , Cho IC , Yu A , Nam BH , Joung JY , Seo HK , et al. Validation of the MSKCC and Heng risk criteria models for predicting survival in patients with metastatic renal cell carcinoma treated with sunitinib. Annals of Surgical Oncology. (2013) ;20: (13):4397–404. |
[10] | Motzer RJ , Escudier B , Bukowski R , Rini BI , Hutson TE , Barrios CH , et al. Prognostic factors for survival in patients treated with sunitinib for metastatic renal cell carcinoma. British Journal of Cancer. (2013) ;108: (12):2470–7. |
[11] | Donders AR , van der Heijden GJ , Stijnen T , Moons KG . Review: a gentle introduction to imputation of missing values. J Clin Epidemiol. (2006) ;59: (10):1087–91. |
[12] | Naeim A , Keeler EB , Mangione CM . Options for handling missing data in the Health Utilities Index Mark 3. Med Decis Making. (2005) ;25: (2):186–98. |
[13] | van der Zanden LF , Vermeulen SH , Oskarsdottir A , Maurits JS , Diekstra MH , Ambert V , et al. Description of the EuroTARGET cohort: A European collaborative project on TArgeted therapy in renal cell cancer-GEnetic- and tumor-related biomarkers for response and toxicity. Urologic Oncology. 2017. |
[14] | Beuselinck B , Job S , Becht E , Karadimou A , Verkarre V , Couchy G , et al. Molecular subtypes of clear cell renal cell carcinoma are associated with sunitinib response in the metastatic setting. Clinical cancer research: an official journal of the American Association for Cancer Research. (2015) ;21: (6):1329–39. |
[15] | Oken MM , Creech RH , Tormey DC , Horton J , Davis TE , McFadden ET , et al. Toxicity and response criteria of the Eastern Cooperative Oncology . Group American Journal of Clinical Oncology. (1982) ;5: (6):649–55. |
[16] | Yuasa T , Tsuchiya N , Urakami S , Horikawa Y , Narita S , Inoue T , et al. Clinical efficacy and prognostic factors for overall survival in Japanese patients with metastatic renal cell cancer treated with sunitinib. BJU International. (2012) ;109: (9):1349–54. |
[17] | Beuselinck B , Vano Y-A , Oudard S , Wolter P , De Smet R , Depoorter L , et al. Prognostic impact of baseline serum C-reactive protein in patients with metastatic renal cell carcinoma (RCC) treated with sunitinib. BJU International. (2014) ;114: (1):81–9. |
[18] | Nederlandse Vereniging voor Klinische Chemie en Laboratoriumgeneeskunde. Algemeen overzicht refer- entiewaarden (10 Nov 2017). Available at: https://www.nvkc.nl/algemeen-overzicht-referentiewaarden (accessed 20 Nov 2017) [cited 2017 20 Nov]. Available from: Available at: https://www.nvkc.nl/algemeen-overzicht-referentiewaarden. |
[19] | Pencina MJ , D’Agostino RB Sr . Evaluating Discrimination of Risk Prediction Models: The C Statistic. Jama. (2015) ;314: (10):1063–4. |
[20] | Heng DY , Chi KN , Murray N , Jin T , Garcia JA , Bukowski RM , et al. A population-based study evaluating the impact of sunitinib on overall survival in the treatment of patients with metastatic renal cell cancer. Cancer. (2009) ;115: (4):776–83. |
[21] | Kubackova K , Melichar B , Bortlicek Z , Pavlik T , Poprach A , Svoboda M , et al. Comparison of Two Prognostic Models in Patients with Metastatic Renal Cancer Treated with Sunitinib: a Retrospective, Registry-Based Study. Targeted Oncology. 2015. |
[22] | Tanaka N , Mizuno R , Ito K , Shirotake S , Yasumizu Y , Masunaga A , et al. External Validation of the MSKCC and IMDC Risk Models in Patients Treated with Targeted Therapy as a First-line and Subsequent Second-line Treatment: A Japanese Multi-institutional Study. Eur Urol Focus. (2016) ;2: (3):303–9. |
[23] | Molina AM , Jia X , Feldman DR , Hsieh JJ , Ginsberg MS , Velasco S , et al. Long-term response to sunitinib therapy for metastatic renal cell carcinoma. Clinical Genitourinary Cancer. (2013) ;11: (3):297–302. |
[24] | De Lichtenberg TH , Hermann GG , Rorth M , Hojer Larsen MJ , Mansourvar Z , Holm ML , et al. Overall survival after immunotherapy, tyrosine kinase inhibitors and surgery in treatment of metastatic renal cell cancer: outcome of 143 consecutive patients from a single centre. Scandinavian Journal of Urology. (2014) ;48: (4):379–86. |