Base price determination for IPL mega auctions: A player performance-based approach
Abstract
Indian Premier League (IPL) is the most popular T20 domestic league in the world. An essential aspect of this league is the “Mega-Auction”, which is of focus in this study. The mega auction occurs once every three years, and it is found that the auction process is inefficient as the time taken is long (∼2 days). This is because players specify their base price. Thus, this study focuses on the efficiency of the auction process and addresses it by prescribing the base price for players. The base prices are prescribed such that they are as close to the actual auction price of a player. Accordingly, in the past, only two mega auctions occurred in 2014 and 2018, and both are considered in this work. Here, a two-stage algorithm to determine the base prices of players is proposed. In the first stage, K-Means clustering is used to group players. The base price for players allocated to a cluster is proposed using a developed assignment logic in the second stage. An empirical demonstration of the proposed algorithm indicates that the auction process has been made efficient as the time taken decreases by ∼17.6% and ∼31.1% for Indian and foreign players, respectively.
1Introduction
Sports analytics is a field that applies analysis techniques to past performance data that can provide a competitive advantage to an individual or a team. It became famous following the release of the film Moneyball in 2011. The global sports analytics market was valued at $42.76 million in 2018, and it is projected to hit $6.376 billion by 2026, growing at a CAGR of 40.40% from 2019 to 2026 (Allied Market Research, 2019). There are two types of sports analytics: (1) on-field and (2) off-field. On-field analytics deals with tracking and monitoring a player’s performance on the field to predict future performance, strengths, weaknesses, and injuries. This can improve the performance of a team or an individual in a game. Off-field analytics deals with the business side of sports, where a sports organization or a team owner finds patterns or insights in data that could improve their revenue or competitive advantage. This assists in making critical strategic decisions that can improve the profitability of the sports organization.
Cricket, a globally popular team sport, has also incorporated sports analytics in recent times (Kargal, 2021). Currently, the three-game formats at the international level are Test Matches, One-Day Internationals, and Twenty20 (T20). T20 is the newest and shortest version of the three formats. This format of 20 overs per side was introduced in 2005 by the International Cricket Council (ICC). A typical T20 match is for three hours, bringing in new audiences due to its shorter duration. As the game is fast-paced, it demands skill sets like power-hitting, skilful bowling, and outstanding fielding. Therefore, T20 has had the highest incorporation of analytics (ICC, 2022).
T20 games are played at the international level and in several leagues worldwide. The Indian Premier League (IPL) is one of the most famous annual Twenty20 cricket leagues. IPL began in the year 2008. It was founded by then BCCI vice-president Lalit Modi seeing the popularity boom of T20 in India when the national team won the ICC World T20 (TNN, 2013). The IPL has a global audience, and it is currently the most-watched cricket league in the world (India Today, 2021).
At present, the IPL has eight teams, each representing an Indian city. In the league phase, each team plays against the other twice in a round-robin fashion, and the top four qualify for the playoffs. The playoffs then decide the two teams that will face each other in the IPL Finals, and the winner is crowned “IPL Champions” for that year. IPL is a franchise-based tournament that invites private firms to own them. Investors see value in investing in the tournament as they believe that IPL will continue to grow, given that it is a source of entertainment to millions (Business Today, 2021).
The different franchises will have to create squads for participating in the IPL. The squad creation is through the IPL auction, an exciting part of the tournament. The BCCI conducts the auction to select players from a pool of Indian and overseas players for the eight franchises. The BCCI ensures a fair auction by setting a cap on the budget for all the participating franchises before the auction. In the 2021 IPL auction, the budget for each franchise was Rs. 85 crores. Additionally, a franchise must spend at least 75 percent of the budget. The budget may or may not get revised before an auction. A franchise can have players ranging from 18 to 25 in their respective squads. However, a squad must have at least 17 Indian players and at most 8 overseas players. The Indian players are categorized as capped and uncapped based on whether they have represented their country or not.
We have two types of IPL auctions –mini-auction and mega auction. A mega auction takes place once every three years, while mini auctions are held every year in those three years. In a mini-auction, every franchise has the right to retain or release any number of players. Nevertheless, in a mega auction, a franchise can only retain a maximum of five players by a combination of direct retention and Right To Match (RTM). Direct retention allows a team to retain a maximum of three players before the auction. RTM is another option to retain a player by matching the highest bid amount by another team during the auction. Teams that have already retained three players by direct retention can use two RTM cards, while teams with less than three can opt for three RTM cards. Each team can retain a maximum of 3 capped Indian players, 2 overseas players, and 2 uncapped Indian players.
The IPL auction is a one-day or a two-day long event, depending on whether it is a mini or mega auction that year. Before the process, an initial list of players who register for the auction will be shortlisted based on the lists provided by each franchise. Players come in sets on the first day of the auction. The auction starts with marquee players who are top performers in T20 cricket. This lot is followed by separate lots of batsmen, all-rounders, wicketkeepers, fast bowlers, and spinners. Each of these lots contains both capped and uncapped Indian players and overseas players. Once these players have been presented in the auction, the eight franchises can select a set of players from the remaining unsold players by the end of the first day. These players will go under the hammer the next day as part of the accelerated bidding process. Before such a long process, an important aspect is that the base price of players must be decided. The base price ranges between Rs. 20 Lakhs to Rs. 200 Lakhs. Players can decide upon their base prices, but they must be carefully chosen considering their performance history and recent form. Else they might risk getting unsold (Katewa, 2021).
This study aims to assist the auction process of IPL by suggesting a player’s base price. For the suggestion of the base price, past IPL performance data are utilized. If the suggested base price is as close to the actual auctioned price, then the auction duration is shortened. Moreover, since the base price is suggested based on past IPL performance, it will ensure that good-performing players have improved base prices, while players with average performance (even if they are big names in the sport) have a base price adjusted based on recent performance.
The following section presents a literature review related to the problem. Section 3 discusses the problem of focus in detail. The methodology to address the focused problem, along with an illustration, is presented in Section 4. The illustration is done using real-life data collected from past IPL tournaments. Hence, the results from the illustration are also presented in the same section. The paper concludes in Section 5, where the significance and limitations of this work, along with the future scope, are presented.
2Literature review
Since the new millennium, data analytics has taken over nearly every sport at almost every level (Navarro, 2020). There have been several studies on the analysis of sports. A study by Apostolou and Tjortjis (2019) attempted on predicting the performance of individual soccer players based on the previous season’s data. In the game of soccer, another research, Pantzalis and Tjortjis, 2020, studied two fundamental cases of sports analytics: team performance prediction and player performance prediction. Here, advanced statistics are used on the previous season’s data for prediction.
Plumley et al. (2018) analyse the competitive balance in the English Football League system using the Herfindahl Index of Competitive Balance (HICB). The study identified that “English Premier League” stands out as the least balanced league in English football among the four leagues. Coluccia et al. (2018) attempt to financially evaluate a goalkeeper of a Series A-League club using an option-pricing model. Mu (2016) proposes an Analytical Hierarchy Process (AHP) for selecting the Golden Ball winner. This is applied to the 2014 FIFA Golden Ball selection, and the potential winners are suggested based on their attack, defence, and fair play performance. Easton & Newell (2019) took an altogether different problem of fantasy sports and analysed whether daily fantasy sports are gambling or not.
Coming to cricket, a study by Anik et al. (2018) does performance prediction of players in ODI cricket using machine learning algorithms. Studies by Barot et al. (2020), Kapadia et al. (2020), and Senevirathne and Manage (2021) focus on predicting the outcome of a game, specifically in limited-overs cricket. Jayanth et al. (2018) proposed a model for match outcome prediction, team structure analysis, and player recommendation systems. Support vector machine (SVM) is used for ranking the players and k-means clustering is used for the player recommendation system. Wickramasinghe (2020) uses the naive bayes approach to predict the winner of an ODI match and achieves its highest accuracy of 85.71% using the univariate feature selection method. Davis et al. (2015) introduce a new metric for player evaluation called “expected run differential” in T20 cricket.
The niche area of the auction in IPL has been studied from time to time. Dey et al. (2014) designed a model based on Analytical Hierarchy Process (AHP) and Artificial Neural Network (ANN) for estimating players’ prices in IPL. First, AHP measures the weights of the attributes responsible for player price estimation, and following that ANN is used to forecast the player price with the weights calculated by AHP.
Chauhan et al. (2018) focus on applying operations research to the concept of IPL auction. The objective of this research is to use operations research in player valuation. Various methods are used to develop the model, such as performance-based index, hedonic pricing, ordinary least squares regression technique, and AHP-ANN model. The Performance-Based Index combines batting, bowling, and wicket-keeping skills to form one index and quantify their performances to find the price they deserve. The Hedonic Pricing model considers the internal and external factors in developing the valuation. The Least Square Regression model accounts for the players’ experience, performance, and characteristics to evaluate the players. The AHP-ANN model assists in handling the complexity and selecting the attributes for player price calculation.
Rani et al. (2020) focused on applying various machine learning algorithms to predict the bid price of a player participating in an IPL auction. The bid price for a player is estimated using a player’s past performance parameters. The machine learning algorithms in the paper are Decision Tree Regressor, K-Nearest Neighbours (KNN), Linear Regression, Stochastic Logistic Regression, Random Forest Regressor, and Support Vector Regression (SVR). SVR and Linear Regression stood out for giving the best results.
The study by Das et al. (2021) shows how a modified hedonic model and a robust machine learning technique, i.e. XGBoost Learning, can be efficient in the price prediction of players in an IPL auction. This modified hedonic model extends the classic hedonic model, which aims to find the coefficients of independent variables and output a new price for cricketers.
Till now, the research in IPL auctions is all about predicting a player’s bid price. The study by Kansal et al. (2014) addresses the base price prediction in IPL auctions. The study predicts the base price of players in IPL using the data mining technique. Several predictive models have been built to predict a player’s selection in the Indian Premier League based on each player’s past performance. The data used consists of playing factors from all the two formats of the game, namely ODI and T20 cricket. The playing factors such as average, strike rate, number of wickets, catches, etc., were utilized to predict the selection of batsmen, bowlers, and all-rounders in the teams. The core idea of the study is to assign players to a base group among a set of fixed five base groups. The base groups range from Rs. 30 Lakh to Rs. 200 Lakh. Data mining tools were used to predict the base price group for players. Three algorithms are applied, namely decision tree, naïve Bayes, and Multi-Layer Perceptron (MLP), on the data set to predict the base price of players. The algorithms are applied to batsmen, bowlers, and all-rounder data set, respectively. For the batsmen dataset, the highest accuracy is shown by MLP, which is 94.18%. Similarly, for bowlers and all-rounders datasets, the highest accuracies are shown by MLP, and they are 94.44% and 97.95%, respectively. The study concludes that MLP had shown the best accuracy for any category of players.
Kansal et al. (2014) can be considered the basis for this study. However, in this current study, the scope for base price prediction is far more extensive than the one addressed by Kansal et al. (2014). This is because the study by Kansal et al. (2014) only assigns players to base groups based on past performance. In this work, the past performance of players is used to arrive at a specific base price for the player in the current auction. This base price is also expected to be closer to the actual auction price of the player. Moreover, new base groups may be created using the arrived base prices if required.
3Problem formulation
The IPL Auctions are the forum where different franchises engage in an open bidding process to acquire players for creating the respective squads. In this auction, the base price for players is declared before the auction process. A player’s base price is the price at which a franchise starts bidding for that player. The base price range is between Rs. 20 Lakhs to Rs. 200 Lakhs. The large range is because of the different types of players: Overseas and Indian players being auctioned. Overseas players are non-Indian citizens who represent different national teams across the globe. These overseas players can set their base price within the given range. Indian players are Indian citizens playing at the international level as part of the Indian National Team and/or playing domestic cricket in India as part of different state associations. The Indian players who have represented the country as a part of the country’s international team are called “Capped players”, while those who have not been are called “Uncapped players”. The base price of Indian players is communicated to the BCCI by the players themselves (Sportskeeda, 2021). Currently, the base price for uncapped players can be one of the following: Rs. 20 Lakhs, Rs. 30 Lakhs, or Rs. 40 Lakhs. In the case of capped players, the base price can be Rs. 50 Lakh, Rs. 75 Lakh, Rs. 100 Lakh, Rs. 150 Lakh, and Rs. 200 Lakh, (Malvania, 2019). To the best of our knowledge, no source in the open forum specifies how the base prices are determined for the different players by the BCCI.
The different franchise bid for players in the bidding process, and bids increase by Rs. 5 Lakh for every bid till Rs. 100 Lakh. After Rs. 100 Lakhs, the bid increases by Rs. 10 Lakh till Rs. 200 Lakh. Beyond Rs. 200 Lakh, the increase is by Rs. 20 Lakh for every bid till Rs. 1000 Lakh. After Rs. 1000 Lakhs, bids increase by Rs. 50 Lakh till the player gets sold. (Cricbuzz, 2018)
The above IPL auction is an extravagant event, and it is of two types: “Mega-auction” and “Mini-auction”. More than 500 players will go under the hammer in a mega-auction, and around 300 players can be expected in a mini-auction. A franchise uses the mega auction to completely rebuild their squad, except for a few retained players who are retained. Mini auctions are meant to fill small gaps in the existing squad of a franchise’s team. A mini-auction can be conducted in a day, while mega-auctions take double the time to get completed, excluding the time taken by franchises to get prepared before those two days. BCCI has two entire days before the IPL to conduct the mega auction. However, from an IPL management perspective, auction conduct time can be considerably reduced if the base price for the player is as close to the actual selling price. Thus, determining an ideal base price would translate to a reduction in the number of bids for a player. This can drastically reduce the total time taken for an auction. From an IPL management perspective, the determination of base price for a player is more imperative than determining the actual auctioned price for the same player. This is because, player auction prices are driven by non-tangible factors such as “Player Popularity”, “Franchise Requirements”, etc. Hence, an error in determining the auction price would drastically affect the revenue streams.
Therefore, this study aims to bridge the gap in determining the base prices for players in an analytic and data-driven fashion. To this end, an algorithm to predict the base price close to a player’s auctioned price is proposed. The proposed algorithm uses past IPL performance and auction data of players. The core of this proposed algorithm consists of two stages, the first is clustering, and the second is base price prediction. Players are grouped into clusters in the clustering stage based on their overall IPL experience. After this clustering, the base price of a player participating in an upcoming mega auction is determined using a developed assignment logic. This assignment logic identifies a similar player within the same cluster as the current player by utilizing past IPL performance characteristics. Once a similar player is identified, the previous year’s auction price of this similar player is assigned as the base price for the current player. The above algorithm is explained in detail in the subsequent sections.
4Methodology and illustration
4.1Algorithm
To determine the base price, an algorithm with two stages is proposed. The first stage of the algorithm focuses on clustering players of the auction. The second stage determines the base price for players, within a cluster, by using a developed assignment logic.
Past mega-auction data is utilized in the clustering stage to create player clusters based on “IPL experience”. In this study, IPL experience is quantitively measured as the total number of balls a player has bowled and/or faced irrespective of their role (Batsman, Bowler, All-Rounder, or Wicketkeeper). This IPL experience is computed for the duration after the past mega auction and until the current mega-auction. This implies that the IPL experience for a mega-auction conducted in 2018 is computed using 2015, 2016, and 2017. This is because the previous mega auction occurred in 2014. In IPL history, only two mega auctions have occurred. One in 2014 and the other in 2018. Hence, in the clustering stage, clusters are created based on the IPL experience for 2014.
For 2014, “n1” players auctioned are grouped into “k” clusters based on the IPL experience computed from the data of 2013, 2012, and 2011. Here, intuitively, the IPL experience indirectly captures the different player types. For determining the k-clusters, a K-Means clustering is adopted. This is a non-hierarchical clustering technique, and k-clusters are created such that the total intra-cluster variance is minimal. Say,
P = {p | p is a player auctioned off in the past IPL mega Auction (here it is the 2014 IPL auction), and 1≤p≤n1}
Z = {z | z is a cluster of players auctioned off in the past IPL mega Auction (here it is the 2014 IPL auction), and 1≤z≤k}
PEp –Past IPL Experience of a player-p in IPL seasons before the past mega auction (here it is from 2011 to 2013)
APp –Auction Price for player-p in the past IPL mega Auction (here it is for the 2014 IPL mega Auction)
Cz –Centroid of a cluster-z
Apz –Assignment of player-p to cluster-z for the past mega auction (here it is 2014 IPL mega Auction)
where
Then, the objective of minimizing total intra-cluster variance can be computed as given in (1)
(1)
K-Means clustering is a nonhierarchically clustering technique, and the following procedure is adopted to identify the ideal number of clusters-k.
Say,
X = {x | x is a player in the training set and x ∈ P}
Y = {y | y is a player in the testing set, and y ∈ P, such that Y-X = {}}
APx –Auction Price for player-x in the training set
APy –Auction Price for player-p in the testing set
Then, since three possible player roles exist, the algorithm is set to have a minimum of 3 clusters (initial value for k is 3). “k” is then increased up to 14, because after 14 clusters less than five players will be allocated to different clusters which are undesirable for the next stage of the algorithm. For each value of k, the following procedure is followed to identify the players in each cluster.
Step-1: Split the past IPL mega auction data in an 80:20 ratio, where 80% of the dataset goes into the training set-X and the remaining 20% goes into the testing set-Y.
Step-2: Apply K-Means clustering on the training set-X and assignment logic on the testing set-Y.
Step-3: Calculate the ratio of the number of correct predictions and the total number of predictions. A prediction is said to be correct when the predicted auction price for a player (in Y) is less than or equal to the actual auction price for the player (in P).
Fig. 1
Cluster accuracy ratios for Indian and Foreign players in 2014 IPL mega auction.
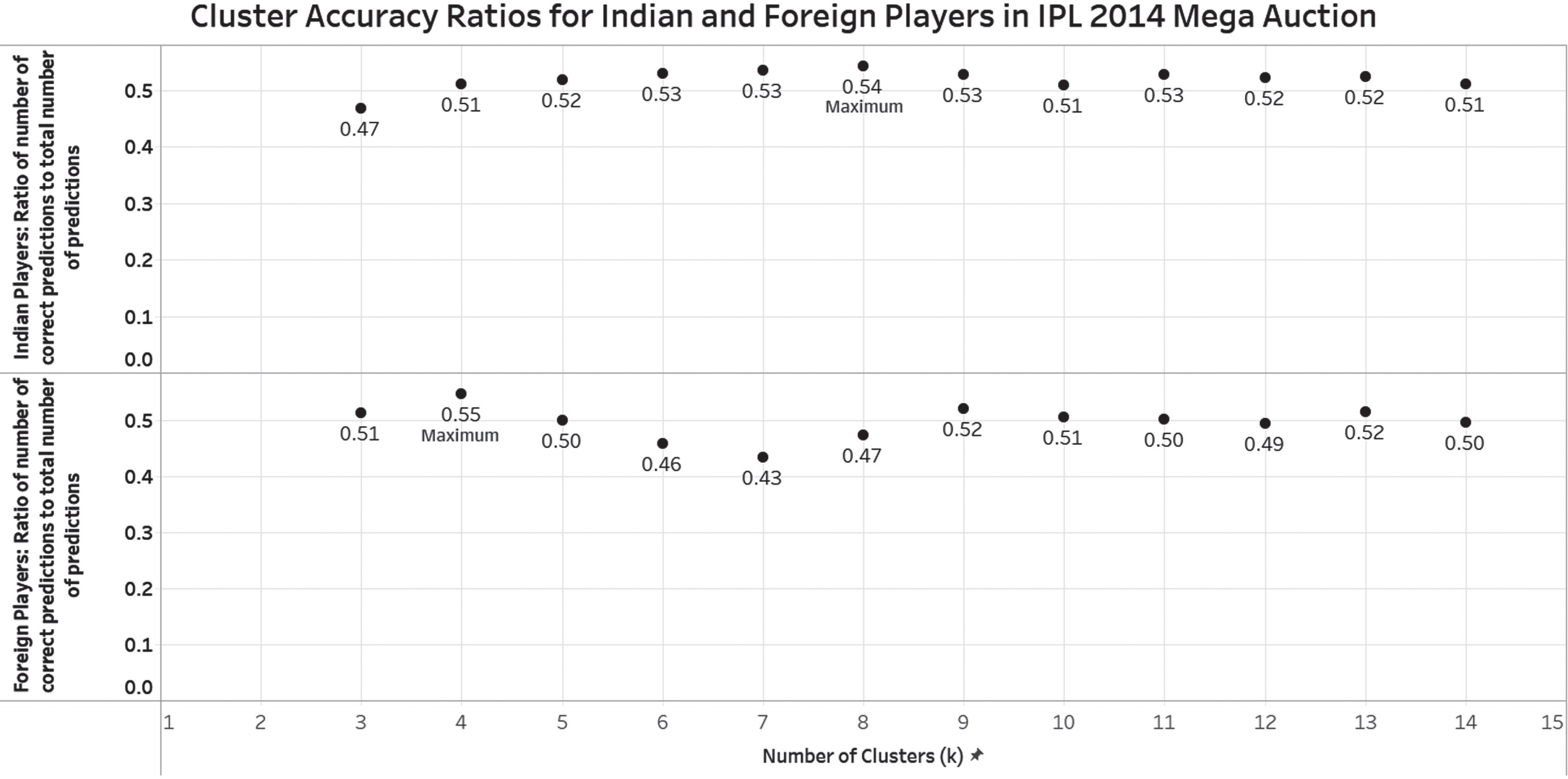
Step-4: Repeat the above three steps a large number of times (say 100 times) and compute the average of ratios calculated in Step-3.
The results from the above algorithm are plotted as a graph as shown in Fig. 1, with the number of clusters on the x-axis and the average of the ratios on the y-axis. The number of clusters which has the maximum average ratio is the ideal number of clusters for the data set at hand.
By adopting the above procedure, “n1” players who appeared in the past IPL mega auction can be grouped into k-clusters based on the past IPL experience “PEp”. Using the identified clusters, in the second stage of the algorithm, the base price for players being auctioned in the current mega auction is assigned based on player attributes. Player attributes refer to the different measurable performances such as the number of balls faced, fours scored, wickets taken by a player, etc. These attributes enable the algorithm to identify similar players and assign base prices accordingly. For the base price assignment, a rule-based approach is developed. The assignment stage of the algorithm consists of 5-steps.
Say,
Q = {q | q is a player to be auctioned in the current mega auction (here it is 2018 IPL mega auction), and 1≤q≤n2} L = {l | l is an attribute of a player’s performance, and 1≤l≤14}
CEq –Current IPL Experience of a player-q in IPL seasons between the past and current mega auctions (Here it is from 2015 to 2017)
BPq –Base Price for player-q in the current mega auction (here it is 2018 IPL mega auction)
CAqz –Assignment of player-q to cluster-z for the current mega auction (here it is 2018 IPL mega auction)
VAlp –Value of attribute-l for player-p
VAlq –Value of attribute-l for player-q
Then the 5-steps in the assignment stage of the algorithm are as follows:
Step-1: Player-q is assigned to a cluster-z such that the Euclidean distance between “Cz” and IPL experience “CEq” is minimal.
Step-2: Within the assigned cluster-z, player-q is matched to the nearest similar player-p. This is achieved by minimizing the Euclidean distance (given in (2)) across all attributes.
(2)
Note: If the player-q is already in cluster-z as player-THORN based on PETHORN, then the above matching is conducted after removing player-THORN from the cluster. This is done to ensure that the mapping is unbiased.
Step-3: APp for player-p is assigned as the BPq for player-q.
Step-4: Repeat Steps 1 to 4 for all the n2 players in set-Q.
Once the above procedure is completed, the base prices for all the players being auctioned will be available for the IPL management. Therefore, the proposed two-stage algorithm can be summarised as:
Clustering stage (Stage-1): Create clusters using IPL experience from the immediate past mega auction.
Assignment stage (Stage-2): Allocate current players being auctioned to previously determined clusters by using IPL experience. Identify the most similar player within the allocated cluster, and assign their auction price as the base price for the current player.
Both accuracy and efficiency need to be verified to establish the utility of the proposed algorithm. The accuracy of the proposed algorithm can be measured by comparing the actual IPL auction price and the proposed base price for a player. If the proposed base price is less than or equal to the actual auction price, then the algorithm is accurate. Efficiency can be established by calculating the difference between the number of actual bids and the number of bids when the base price equals the one suggested by the algorithm. The above difference must be positive or zero for the algorithm to be efficient. This indicates that the algorithm has saved time by proposing a base price closer to the actual auction price than the existing policy for assigning a base price.
4.2Data collection and pre-processing
To demonstrate the two-stage algorithm proposed for base price determination, primary data is collected from “cricsheet.org” and web scrapped from “iplt20.com”. From the two websites, two data sets are obtained. The first data set, obtained from “cricsheet.org”, contains ball-by-ball information on every IPL match played from 2008 to 2017. The second dataset from “ipl20.com” contains the auction information of players sold in two (2014 and 2018) IPL mega auctions. Snapshots of the above two datasets are presented respectively in Tables 1 and 2.
The ball-by-ball information in the first dataset is processed to consolidate three-year values, before the respective mega auction, for each player’s attribute. Hence, for a player being auctioned in 2014, consolidation consists of values in each attribute for the years 2011, 2012, and 2013. Similarly, for the 2018 mega auction, consolidation in each attribute is for the years 2015 to 2017. Here, attributes are the characteristics that best depict a player’s performance. The chosen 14 attributes and their descriptions are presented in Table 3. The 14 attributes are chosen as they are (1) commonly referred to when introducing players during an IPL game, and (2) they capture player characteristics. The processed first data set is merged with the auction data for the respective mega auctions. This generates a data set, henceforth referred to as “Player Dataset“, which is utilized by the algorithm. A snapshot of the player dataset is provided in Table 4. The player dataset consists of 149 Indian players, out of which 76 and 73 are the number of players sold in the mega auction of 2014 and 2018, respectively. Additionally, there are 69 Overseas players, out of which 33 and 36 are the number of players sold in the mega auction 2014 and 2018, respectively. The above data processing is carried out through a developed python script.
Table 1
Sample ball-by-ball data obtained from
Match ID | Inning | Batting team | Bowling team | Over | Ball | Batsman | Non-striker | Bowler | Is it a super over | ||
60 | 1 | Kolkata Knight Riders | Royal Challengers Bangalore | 1 | 1 | SC Ganguly | BB McCullum | P Kumar | 0 | ||
60 | 1 | Kolkata Knight Riders | Royal Challengers Bangalore | 1 | 2 | BB McCullum | SC Ganguly | P Kumar | 0 | ||
60 | 1 | Kolkata Knight Riders | Royal Challengers Bangalore | 1 | 3 | BB McCullum | SC Ganguly | P Kumar | 0 | ||
60 | 1 | Kolkata Knight Riders | Royal Challengers Bangalore | 1 | 4 | BB McCullum | SC Ganguly | P Kumar | 0 | ||
60 | 1 | Kolkata Knight Riders | Royal Challengers Bangalore | 1 | 5 | BB McCullum | SC Ganguly | P Kumar | 0 | ||
60 | 1 | Kolkata Knight Riders | Royal Challengers Bangalore | 1 | 6 | BB McCullum | SC Ganguly | P Kumar | 0 | ||
60 | 1 | Kolkata Knight Riders | Royal Challengers Bangalore | 1 | 7 | BB McCullum | SC Ganguly | P Kumar | 0 | ||
60 | 1 | Kolkata Knight Riders | Royal Challengers Bangalore | 2 | 1 | BB McCullum | SC Ganguly | Z Khan | 0 | ||
60 | 1 | Kolkata Knight Riders | Royal Challengers Bangalore | 2 | 2 | BB McCullum | SC Ganguly | Z Khan | 0 | ||
60 | 1 | Kolkata Knight Riders | Royal Challengers Bangalore | 2 | 3 | BB McCullum | SC Ganguly | Z Khan | 0 | ||
Wide runs | Bye runs | Leg bye runs | No-ball runs | Penalty runs | Batsman runs | Extra runs | Total runs | Player dismissed | Dismissal kind | Fielder | Year |
0 | 0 | 1 | 0 | 0 | 0 | 1 | 1 | 2008 | |||
0 | 0 | 0 | 0 | 0 | 0 | 0 | 0 | 2008 | |||
1 | 0 | 0 | 0 | 0 | 0 | 1 | 1 | 2008 | |||
0 | 0 | 0 | 0 | 0 | 0 | 0 | 0 | 2008 | |||
0 | 0 | 0 | 0 | 0 | 0 | 0 | 0 | 2008 | |||
0 | 0 | 0 | 0 | 0 | 0 | 0 | 0 | 2008 | |||
0 | 0 | 1 | 0 | 0 | 0 | 1 | 1 | 2008 | |||
0 | 0 | 0 | 0 | 0 | 0 | 0 | 0 | 2008 | |||
0 | 0 | 0 | 0 | 0 | 4 | 0 | 4 | 2008 | |||
0 | 0 | 0 | 0 | 0 | 4 | 0 | 4 | 2008 |
Table 2
A sample data on player-wise auction price in previous mega auctions
Player name | Auction year | Country | Auction price |
A Ashish Reddy | 2014 | Indian | 20,00,000 |
A Choudhary | 2018 | Indian | 30,00,000 |
A Dananjaya | 2018 | Overseas | 50,00,000 |
A Mishra | 2014 | Indian | 4,75,00,000 |
A Mishra | 2018 | Indian | 4,00,00,000 |
A Nehra | 2014 | Indian | 2,00,00,000 |
AB Dinda | 2014 | Indian | 1,50,00,000 |
AD Nath | 2018 | Indian | 1,00,00,000 |
AD Russell | 2014 | Overseas | 60,00,000 |
AG Paunikar | 2014 | Indian | 20,00,000 |
Table 3
List of player attributes considered in this study
S. no | Attribute | Attribute description |
1 | Total_runs | Total runs scored by the player |
2 | 0s | Total number of dot balls faced by the player |
3 | Running | Total number of ones and twos run by the player |
4 | 4s | Total fours scored by the player |
5 | 6s | Total sixes hit by the player |
6 | Dots_bowl | Total number of balls bowled by the player without conceding runs |
7 | Wides | Total wide balls bowled by the player |
8 | No_balls | Total no balls bowled by the player |
9 | Runs_conceded | Total number of runs that the opposing side have scored while the player was bowling, excluding any byes, leg byes, or penalty runs. |
10 | Extra_runs_conceded | Total number of runs that the opposite side have scored through wides and no balls |
11 | Wkts | Total number of wickets taken by the player |
12 | Bowled | Total number of wickets taken when the player’s legitimate delivery hit the stumps |
13 | Caught | Total number of wickets taken when the ball is caught by either the player who bowled or the fielder, after the opponent player hits the ball |
14 | Boundaries_conceded | Total number of fours and sixes scored by the opposing side while the player was bowling |
Table 4
A sample data on the consolidated three-year values, before the respective mega auction, for each attribute of a player
Name | Auction year | Balls faced | Total runs | 0s | Running | 1s | 2s | 4s | 6s | Balls bowled | Dots bowled | Wides |
A Ashish Reddy | 2014 | 118 | 160 | 38 | 80 | 51 | 13 | 11 | 6 | 203 | 65 | 7 |
A Choudhary | 2018 | 20 | 25 | 4 | 15 | 13 | 1 | 1 | 1 | 101 | 42 | 6 |
A Dananjaya | 2018 | 0 | 0 | 0 | 0 | 0 | 0 | 0 | 0 | 0 | 0 | 0 |
A Mishra | 2014 | 163 | 173 | 76 | 91 | 69 | 11 | 19 | 1 | 974 | 370 | 10 |
A Mishra | 2018 | 78 | 61 | 42 | 41 | 33 | 4 | 2 | 2 | 750 | 246 | 23 |
A Nehra | 2014 | 20 | 12 | 13 | 8 | 6 | 1 | 1 | 0 | 504 | 183 | 10 |
AB Dinda | 2014 | 21 | 11 | 13 | 7 | 7 | 0 | 1 | 0 | 540 | 207 | 22 |
AD Nath | 2018 | 28 | 20 | 14 | 16 | 10 | 3 | 1 | 0 | 0 | 0 | 0 |
AD Russell | 2014 | 40 | 58 | 14 | 28 | 16 | 6 | 3 | 3 | 138 | 38 | 3 |
AG Paunikar | 2014 | 57 | 49 | 40 | 13 | 9 | 2 | 9 | 0 | 0 | 0 | 0 |
No. of | Runs balls | Extra runs conceded | Total runs conceded | Wickets conceded | Bowled | Caught | Boundaries conceded | 4s conceded | 6s conceded | Country | Role | Price |
2 | 298 | 9 | 307 | 14 | 4 | 7 | 36 | 21 | 15 | Indian | Bowler | 20,00,000 |
1 | 137 | 7 | 144 | 5 | 0 | 5 | 19 | 13 | 6 | Indian | Bowler | 30,00,000 |
0 | 0 | 0 | 0 | 0 | 0 | 0 | 0 | 0 | 0 | Overseas | Bowler | 50,00,000 |
6 | 1113 | 16 | 1129 | 53 | 9 | 29 | 114 | 65 | 49 | Indian | Bowler | 4,75,00,000 |
2 | 972 | 25 | 997 | 32 | 4 | 15 | 108 | 65 | 43 | Indian | Bowler | 4,00,00,000 |
2 | 706 | 12 | 718 | 22 | 4 | 17 | 103 | 76 | 27 | Indian | Bowler | 2,00,00,000 |
8 | 756 | 30 | 786 | 30 | 6 | 23 | 113 | 88 | 25 | Indian | Bowler | 1,50,00,000 |
0 | 0 | 0 | 0 | 0 | 0 | 0 | 0 | 0 | 0 | Indian | All-rounder | 1,00,00,000 |
1 | 225 | 4 | 229 | 1 | 0 | 1 | 35 | 29 | 6 | Overseas | All-rounder | 60,00,000 |
0 | 0 | 0 | 0 | 0 | 0 | 0 | 0 | 0 | 0 | Indian | Wicket keeper | 20,00,000 |
4.3Demonstration
The player dataset created in the previous sub-section is utilized to demonstrate the proposed algorithm’s workability and establish its effectiveness. Here, the player dataset is separated into Indian and Foreign players, and the algorithm is implemented separately for the two types of players. This is because the base price of Indian and Foreign players is significantly different in IPL. Accordingly, for the split datasets, the mega auction conducted in 2014 is utilized to create the clusters at the clustering stage of the proposed algorithm. A plot of player experiences (PEp) for 76 Indian and 33 Foreign players is presented in Fig. 2 and Fig. 3, respectively. With this, the clustering stage is executed, and the accuracies for the different number of clusters are presented in Table 5. The ideal number of clusters for Indian players is 8, and that of Foreign players is 4. The number of players in each of these clusters is presented in Table 6.
Fig. 2
Indian players IPL experience (from IPL 2011 to IPL 2013).
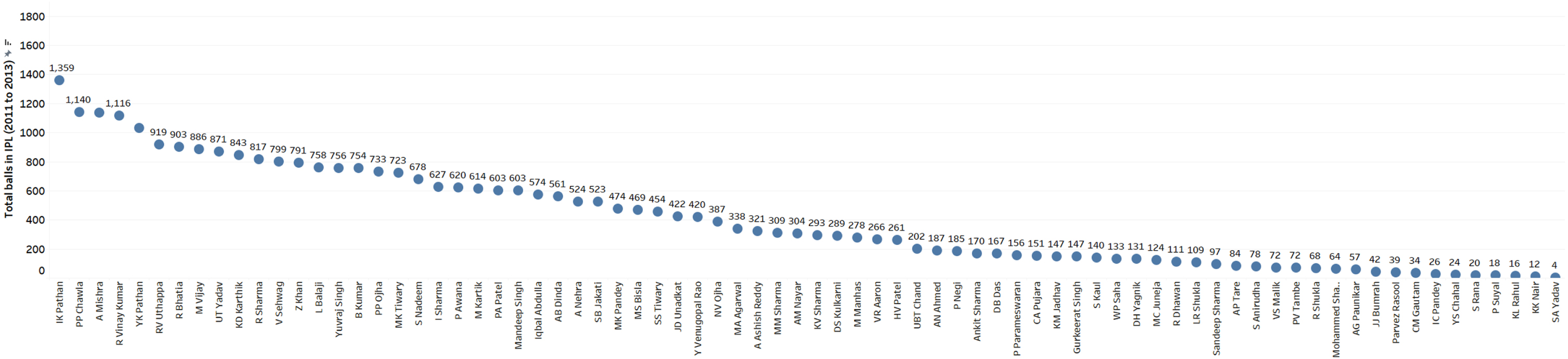
Fig. 3
Foreign players IPL experience (from IPL 2011 to IPL 2013).
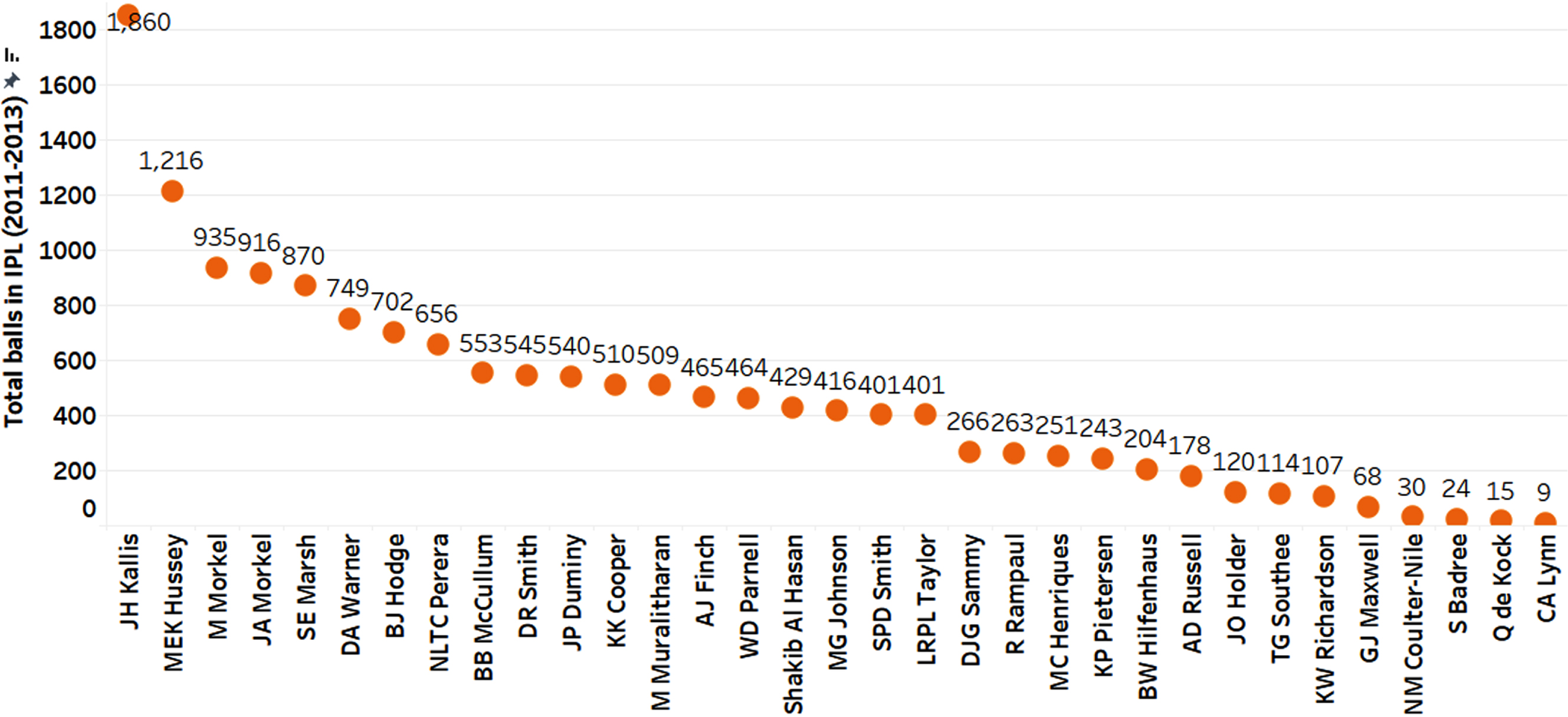
Table 5
Summary of the number of clusters and the accuracies for Indian and Foreign players
Number of clusters (k) | Accuracy for Indian players | Accuracy for Foreign players |
3 | 0.4668 | 0.5143 |
4 | 0.5097 | 0.5486 |
5 | 0.5172 | 0.4999 |
6 | 0.5289 | 0.4593 |
7 | 0.5338 | 0.4343 |
8 | 0.5430 | 0.4743 |
9 | 0.5265 | 0.5214 |
10 | 0.5093 | 0.5057 |
11 | 0.5266 | 0.5028 |
12 | 0.5212 | 0.4943 |
13 | 0.5231 | 0.5161 |
14 | 0.5112 | 0.4975 |
Table 6
Summary of number of Indian and Foreign players in the cluster
Cluster number for Indian players | Number of Indian players auctioned in 2018 mapped to that cluster | Cluster number for Foreign players | Number of Foreign players auctioned in 2018 mapped to that cluster |
0 | 12 | 0 | 17 |
1 | 14 | 1 | 0 |
2 | 5 | 2 | 14 |
3 | 0 | 3 | 5 |
4 | 7 | Only 4 clusters for foreign players | |
5 | 20 | ||
6 | 10 | ||
7 | 5 |
At the 2018 mega auction, 73 Indian and 36 Foreign players were auctioned. These players are assigned to the 8 Indian clusters and 4 Overseas clusters based on their experience (CEq) in the IPL seasons from 2015 to 2017. It is interesting to note that the number of clusters for Indian and Foreign players differs. A possible reason for this could be the squad requirement (at least 17 players must be Indian, and at most 8 players are Foreign). There is a larger and more closely associated pool of Indian players. Thus, a franchise may be more liberal when bidding for Indian players. On the contrary, a franchise may be more cautious in picking a foreign player because both the number of available slots and the number of available players are lesser.
Next, the base price for each player is determined by identifying similar players based on the attributes. The base price for a player in the 2018 mega auction is set to be the auction price of a similar player in the 2014 mega auction. The similar players identified for 73 Indian and 36 Foreign players are presented in Table 7. Table 7 also provides the proposed base price for 73 Indian and 36 Foreign players and their actual base and actual auction prices in the 2018 mega auction. The implementation of the proposed algorithm is through a developed python script.
From Table 7, out of 73 Indian players and 36 Foreign players:
• The algorithm has improved the base price for 54 Indian players and 21 Foreign players, i.e., the predicted base price is less than or equal to the actual auction price for these players. Therefore, the base price is not higher than the actual auction price for ∼74% of the Indian players and ∼58% of the Foreign players. This indicates the accuracy of the algorithm.
• Among the 54 players, the algorithm underpredicted the base prices for 21 Indian players and 5 Foreign players, i.e., the predicted base price is less than the actual base price and actual auction price. For the remaining 33 players, the new predicted base price is improved compared to the actual base price.
• The algorithm has decreased the time taken for the entire auction process by ∼17.6% and ∼31.1% for Indian and Foreign players, respectively. The time taken for the auction process is calculated in terms of the number of auction calls. The actual time taken for Indian and Foreign players is 1237 and 494, respectively. If the proposed base prices were used, the time taken is 1019 and 340 for Indian and Foreign players, respectively. This decrease in the time taken proves the efficiency of the algorithm.
• A deeper analysis of the 19 Indian players whose proposed base price is higher than their actual auction price indicates the following:
∘ Out of the 19 players, 10 were uncapped players, and 9 were capped players.
∘ The uncapped players have performed well, and hence they are matched with players with reputations and higher auction prices. However, all uncapped players have gone either at their base price or very low incremental prices.
Table 7
Proposed base prices, actual base & auction prices and algorithm efficiency for players participating in 2018 IPL Mega Auction
S. no | Player | Country | Identified most similar player | Actual base price (in million Rupees) | Proposed base price (in million Rupees) | Actual auction price (in million Rupees) | Actual time taken for auction | Time taken with the proposed base price |
1 | A Choudhary | Indian | AN Ahmed | 3000000 | 3000000 | 3000000 | 0 | 0 |
2 | A Mishra | Indian | UT Yadav | 15000000 | 26000000 | 40000000 | 15 | 7 |
3 | AD Nath | Indian | Parvez Rasool | 2000000 | 9500000 | 10000000 | 16 | 1 |
4 | AM Rahane | Indian | YK Pathan | 20000000 | 32500000 | 40000000 | 10 | 4 |
5 | AP Tare | Indian | AG Paunikar | 2000000 | 2000000 | 2000000 | 0 | 0 |
6 | AS Rajpoot | Indian | S Kaul | 3000000 | 4500000 | 30000000 | 29 | 26 |
7 | AT Rayudu | Indian | Mandeep Singh | 5000000 | 8000000 | 22000000 | 21 | 15 |
8 | Ankit Sharma | Indian | AN Ahmed | 2000000 | 3000000 | 2000000 | 0 | 0 |
9 | Anureet Singh | Indian | A Nehra | 3000000 | 20000000 | 3000000 | 0 | 0 |
10 | Avesh Khan | Indian | VS Malik | 2000000 | 2000000 | 7000000 | 10 | 10 |
11 | BB Sran | Indian | HV Patel | 5000000 | 4000000 | 22000000 | 21 | 23 |
12 | Basil Thampi | Indian | A Ashish Reddy | 3000000 | 2000000 | 9500000 | 13 | 15 |
13 | Bipul Sharma | Indian | HV Patel | 2000000 | 4000000 | 2000000 | 0 | 0 |
14 | DJ Hooda | Indian | JD Unadkat | 4000000 | 28000000 | 36000000 | 30 | 4 |
15 | DL Chahar | Indian | R Shukla | 2000000 | 4000000 | 8000000 | 12 | 8 |
16 | DS Kulkarni | Indian | P Awana | 5000000 | 6500000 | 7500000 | 5 | 2 |
17 | G Gambhir | Indian | YK Pathan | 20000000 | 32500000 | 28000000 | 4 | 0 |
18 | Gurkeerat Singh | Indian | P Negi | 5000000 | 2000000 | 7500000 | 5 | 11 |
19 | HV Patel | Indian | A Nehra | 2000000 | 20000000 | 2000000 | 0 | 0 |
20 | Harbhajan Singh | Indian | PP Chawla | 20000000 | 42500000 | 20000000 | 0 | 0 |
21 | IR Jaggi | Indian | KK Nair | 2000000 | 7500000 | 2000000 | 0 | 0 |
22 | Ishan Kishan | Indian | MA Agarwal | 4000000 | 16000000 | 62000000 | 43 | 25 |
23 | J Yadav | Indian | LR Shukla | 5000000 | 15000000 | 5000000 | 0 | 0 |
24 | JD Unadkat | Indian | VR Aaron | 15000000 | 20000000 | 115000000 | 48 | 43 |
25 | KD Karthik | Indian | Mandeep Singh | 20000000 | 8000000 | 74000000 | 27 | 41 |
26 | KH Pandya | Indian | Yuvraj Singh | 4000000 | 140000000 | 88000000 | 56 | 0 |
27 | KK Nair | Indian | Mandeep Singh | 5000000 | 8000000 | 56000000 | 38 | 32 |
28 | KL Rahul | Indian | Y Venugopal Rao | 20000000 | 5500000 | 110000000 | 42 | 61 |
29 | KM Jadhav | Indian | A Ashish Reddy | 20000000 | 2000000 | 78000000 | 29 | 55 |
30 | KV Sharma | Indian | M Kartik | 20000000 | 10000000 | 50000000 | 15 | 25 |
31 | Kuldeep Yadav | Indian | HV Patel | 15000000 | 4000000 | 58000000 | 24 | 41 |
32 | M Ashwin | Indian | S Kaul | 2000000 | 4500000 | 22000000 | 27 | 22 |
33 | M Vijay | Indian | PA Patel | 20000000 | 14000000 | 20000000 | 0 | 6 |
34 | M Vohra | Indian | MA Agarwal | 2000000 | 16000000 | 11000000 | 17 | 0 |
35 | MA Agarwal | Indian | M Manhas | 2000000 | 3000000 | 10000000 | 16 | 14 |
36 | MK Pandey | Indian | Mandeep Singh | 10000000 | 8000000 | 110000000 | 52 | 56 |
37 | MK Tiwary | Indian | DS Kulkarni | 5000000 | 11000000 | 10000000 | 10 | 0 |
38 | MM Sharma | Indian | R Vinay Kumar | 15000000 | 28000000 | 24000000 | 7 | 0 |
39 | Mandeep Singh | Indian | MA Agarwal | 5000000 | 16000000 | 14000000 | 14 | 0 |
40 | Mohammed Shami | Indian | A Ashish Reddy | 10000000 | 2000000 | 30000000 | 15 | 31 |
41 | Mohammed Siraj | Indian | P Parameswaran | 10000000 | 3000000 | 26000000 | 13 | 27 |
42 | N Rana | Indian | MA Agarwal | 2000000 | 16000000 | 34000000 | 33 | 11 |
43 | NV Ojha | Indian | KV Sharma | 7500000 | 37500000 | 14000000 | 9 | 0 |
44 | P Negi | Indian | M Kartik | 5000000 | 10000000 | 10000000 | 10 | 0 |
45 | P Sahu | Indian | AN Ahmed | 2000000 | 3000000 | 2000000 | 0 | 0 |
46 | PA Patel | Indian | Mandeep Singh | 10000000 | 8000000 | 17000000 | 7 | 11 |
47 | PJ Sangwan | Indian | P Parameswaran | 3000000 | 3000000 | 15000000 | 19 | 19 |
48 | PP Chawla | Indian | M Kartik | 10000000 | 10000000 | 42000000 | 21 | 21 |
49 | R Ashwin | Indian | Iqbal Abdulla | 20000000 | 6500000 | 76000000 | 28 | 45 |
50 | R Tewatia | Indian | Parvez Rasool | 2000000 | 9500000 | 30000000 | 31 | 16 |
51 | R Vinay Kumar | Indian | HV Patel | 10000000 | 4000000 | 10000000 | 0 | 12 |
52 | RA Tripathi | Indian | DS Kulkarni | 2000000 | 11000000 | 34000000 | 33 | 16 |
53 | RD Chahar | Indian | Mohammed Shami | 2000000 | 42500000 | 19000000 | 25 | 0 |
54 | RV Uthappa | Indian | V Sehwag | 20000000 | 32000000 | 64000000 | 22 | 16 |
55 | S Dhawan | Indian | YK Pathan | 20000000 | 32500000 | 52000000 | 16 | 10 |
56 | S Gopal | Indian | PV Tambe | 2000000 | 2000000 | 2000000 | 0 | 0 |
57 | S Kaul | Indian | P Parameswaran | 3000000 | 3000000 | 38000000 | 33 | 33 |
58 | S Nadeem | Indian | VR Aaron | 4000000 | 20000000 | 32000000 | 28 | 6 |
59 | SA Yadav | Indian | A Ashish Reddy | 3000000 | 2000000 | 32000000 | 30 | 32 |
60 | SN Thakur | Indian | VR Aaron | 7500000 | 20000000 | 26000000 | 18 | 3 |
61 | SS Tiwary | Indian | MA Agarwal | 5000000 | 16000000 | 8000000 | 6 | 0 |
62 | STR Binny | Indian | JD Unadkat | 5000000 | 28000000 | 5000000 | 0 | 0 |
63 | SV Samson | Indian | Mandeep Singh | 10000000 | 8000000 | 80000000 | 40 | 44 |
64 | Sachin Baby | Indian | AN Ahmed | 2000000 | 3000000 | 2000000 | 0 | 0 |
65 | Sandeep Sharma | Indian | Z Khan | 5000000 | 26000000 | 30000000 | 25 | 2 |
66 | T Natarajan | Indian | YS Chahal | 4000000 | 2000000 | 4000000 | 0 | 4 |
67 | UT Yadav | Indian | Z Khan | 10000000 | 26000000 | 42000000 | 21 | 8 |
68 | V Shankar | Indian | CM Gautam | 4000000 | 2000000 | 32000000 | 28 | 32 |
69 | WP Saha | Indian | Mandeep Singh | 10000000 | 8000000 | 50000000 | 25 | 29 |
70 | Washington Sundar | Indian | LR Shukla | 15000000 | 15000000 | 32000000 | 11 | 11 |
71 | YK Pathan | Indian | R Bhatia | 7500000 | 17000000 | 19000000 | 14 | 2 |
72 | YS Chahal | Indian | UT Yadav | 20000000 | 26000000 | 60000000 | 20 | 17 |
73 | Yuvraj Singh | Indian | Mandeep Singh | 20000000 | 8000000 | 20000000 | 0 | 14 |
74 | AJ Finch | Foreign | DR Smith | 15000000 | 45000000 | 62000000 | 26 | 9 |
75 | AJ Tye | Foreign | DJG Sammy | 10000000 | 35000000 | 72000000 | 36 | 19 |
76 | B Stanlake | Foreign | TG Southee | 5000000 | 12000000 | 5000000 | 0 | 0 |
77 | BA Stokes | Foreign | BJ Hodge | 20000000 | 24000000 | 125000000 | 45 | 43 |
78 | BB McCullum | Foreign | DA Warner | 20000000 | 55000000 | 36000000 | 8 | 0 |
79 | BCJ Cutting | Foreign | KP Pietersen | 10000000 | 90000000 | 22000000 | 11 | 0 |
80 | C Munro | Foreign | KW Richardson | 5000000 | 10000000 | 19000000 | 19 | 9 |
81 | CA Lynn | Foreign | KP Pietersen | 20000000 | 90000000 | 96000000 | 38 | 3 |
82 | CH Gayle | Foreign | DA Warner | 20000000 | 55000000 | 20000000 | 0 | 0 |
83 | CJ Jordan | Foreign | GJ Maxwell | 10000000 | 60000000 | 10000000 | 0 | 0 |
84 | CR Brathwaite | Foreign | KP Pietersen | 10000000 | 90000000 | 20000000 | 10 | 0 |
85 | CR Woakes | Foreign | KW Richardson | 20000000 | 10000000 | 74000000 | 27 | 37 |
86 | DA Miller | Foreign | NLTC Perera | 15000000 | 16000000 | 30000000 | 10 | 9 |
87 | DJ Bravo | Foreign | JA Morkel | 20000000 | 24000000 | 64000000 | 22 | 20 |
88 | DT Christian | Foreign | DJG Sammy | 10000000 | 35000000 | 15000000 | 5 | 0 |
89 | GJ Maxwell | Foreign | NLTC Perera | 20000000 | 16000000 | 90000000 | 35 | 39 |
90 | Imran Tahir | Foreign | NLTC Perera | 10000000 | 16000000 | 10000000 | 0 | 0 |
91 | JC Buttler | Foreign | DR Smith | 15000000 | 45000000 | 44000000 | 17 | 0 |
92 | JJ Roy | Foreign | KP Pietersen | 15000000 | 90000000 | 15000000 | 0 | 0 |
93 | JP Duminy | Foreign | Shakib Al Hasan | 10000000 | 28000000 | 10000000 | 0 | 0 |
94 | K Rabada | Foreign | KW Richardson | 15000000 | 10000000 | 42000000 | 16 | 21 |
95 | KA Pollard | Foreign | JA Morkel | 20000000 | 24000000 | 54000000 | 17 | 15 |
96 | KS Williamson | Foreign | Shakib Al Hasan | 15000000 | 28000000 | 30000000 | 10 | 1 |
97 | MA Starc | Foreign | R Rampaul | 20000000 | 9000000 | 94000000 | 37 | 49 |
98 | MG Johnson | Foreign | JP Duminy | 20000000 | 22000000 | 20000000 | 0 | 0 |
99 | MP Stoinis | Foreign | JP Duminy | 20000000 | 22000000 | 62000000 | 21 | 20 |
100 | Mohammad Nabi | Foreign | R Rampaul | 5000000 | 9000000 | 10000000 | 10 | 2 |
101 | Mustafizur Rahman | Foreign | M Muralitharan | 10000000 | 10000000 | 22000000 | 11 | 11 |
102 | NM Coulter-Nile | Foreign | BJ Hodge | 15000000 | 24000000 | 22000000 | 6 | 0 |
103 | PJ Cummins | Foreign | NLTC Perera | 20000000 | 16000000 | 54000000 | 17 | 21 |
104 | Q de Kock | Foreign | DR Smith | 20000000 | 45000000 | 28000000 | 4 | 0 |
105 | SR Watson | Foreign | JA Morkel | 10000000 | 24000000 | 40000000 | 20 | 8 |
106 | SW Billings | Foreign | MC Henriques | 10000000 | 10000000 | 10000000 | 0 | 0 |
107 | Shakib Al Hasan | Foreign | NLTC Perera | 10000000 | 16000000 | 20000000 | 0 | 0 |
108 | TA Boult | Foreign | JP Duminy | 15000000 | 22000000 | 22000000 | 10 | 4 |
109 | TG Southee | Foreign | JP Duminy | 10000000 | 22000000 | 10000000 | 6 | 0 |
Furthermore, the results obtained for the 2022 Mega Auction through the proposed algorithm are presented in Annexure 1 (2022 mega auction data was unavailable at the time of submission). From Exhibits 1 and 2 (in Annexure-1), the following observations are made for the 72 Indian and 38 Foreign players auctioned in 2022:
• The algorithm has improved the base price for 44 Indian players and 22 Foreign players, i.e., the predicted base price is less than or equal to the actual auction price for these players. Therefore, the base price is not higher than the actual auction price for ∼61% of the Indian players and ∼58% of the Foreign players. This indicates the accuracy of the algorithm.
• The algorithm has decreased the time taken for the entire auction process by ∼34.4% and ∼27% for Indian and Foreign players, respectively. The time taken for the auction process is calculated based on the number of auction calls. The actual auction calls for Indian and Foreign players are 1317 and 621, respectively. If the proposed base prices were used, the auction calls would be 864 and 453 for Indian and Foreign players, respectively. This decrease proves the efficiency of the algorithm.
The demonstration for the 2022 mega auction further strengthens the reliability of the proposed model.
Therefore, from the above demonstration, it is evident that the proposed algorithm assists in predicting the base price for the players in an auction.
5Conclusion
This study focuses on the problem of base price determination for an IPL mega auction. The problem is addressed from the perspective of improving the efficiency of the auction process by reducing the time of the auction. Accordingly, the study proposes a two-stage algorithm for base price prediction. The first stage of the algorithm is clustering. The second stage is a developed assignment logic. The players are clustered into groups at the clustering stage based on the IPL experience. The IPL experience is computed based on the number of balls a player has played (batsman have faced and bowlers have bowled) in the time frame of three years between the previous two IPL mega auctions. The clusters are formed using the K-Means clustering technique. Next, the players about to be auctioned in the current IPL mega auction are assigned to the determined clusters by computing their IPL experience in the IPLs between the previous mega-auction and the current mega auction (three IPL years). After this assignment, the second stage of the algorithm is utilized. In this stage, the players assigned to the clusters are matched to the closest player in that cluster based on IPL performance. Once the closest player has been matched, the previous mega auction’s actual price is allocated as the base price for the player in the current auction.
The proposed two-stage algorithm is demonstrated using real-life IPL data. The mega-auction data is collected for the past two mega-auctions in 2014 and 2018. The player performance is computed from data collected for IPL matches between 2011 to 2018. Using the above data, two performance measures for the proposed algorithm are estimated. The first is accuracy, and the second is efficiency. Here accuracy refers to the ability of the algorithm to arrive at a base price that is not higher than the actual auction price of the player. The efficiency refers to the ability of the algorithm to reduce the auction time by suggesting a base price that is as close to the actual auction price of the player. Accordingly, from the demonstration, it is evident that the proposed approach has an accuracy of ∼74% and ∼58% for predicting the base price of Indian and Foreign players, respectively. Moreover, the algorithm decreases the time taken for the auction process by ∼17.6% and ∼31.1% for Indian and Foreign players, respectively. Analysis of the 2022 mega auction data aligns with the results of the 2018 mega auction.
Therefore, from the demonstration, it is evident that the proposed algorithm effectively addresses the concern of base price determination. Nevertheless, it is also evident that accuracy (∼74%) needs attention. As there are only three past IPL mega auctions as references for this study, the concrete establishment of accuracy and efficiency is not yet possible. Moreover, to the best of our knowledge, this is the first study that focuses on base price determination. This is in contrast to the present practice where the players themselves set the base price in IPL. Thus, it is believed that future work can focus on improving accuracy. Further, the current work is suitable only for determining the base price for players with IPL experience. It is not applicable for new entrants without IPL experience. Therefore, to address these concerns, experience from other sources (T20 leagues in other countries for foreign players and domestic T20 leagues for uncapped Indian players) can be explored. Additionally, future work may also consider the exploration of modelling the IPL as a Vickrey auction so that true player values may be determined.
References
1 | Allied Market Research (2019) Sports Analytics Market [online] https://www.alliedmarketresearch.com/sports-analyticsmarket (accessed 27 January 2022). |
2 | Anik A.I. , Yeaser S. , Hossain A.G , Chakrabarty A. Player’s performance prediction in ODI cricket using machine learning algorithms, 2018 4th International Conference on Electrical Engineering and Information & Communication Technology (ICEEiCT), available at: http://doi.org/10.1109/ceeict.2018.8628118. |
3 | Apostolou K. , Tjortjis C. 2019, Sports analytics algorithms for performance prediction, 2019 10th International Conference on Information, Intelligence, Systems and Applications (IISA), available at: http://doi.org/10.1109/iisa.2019.8900754. |
4 | Barot H. , Kothari A. , Bide P. , Ahir B. , Kankaria R. 2020, Analysis and prediction for the Indian Premier League, 2020 International Conference for Emerging Technology (INCET), available at: http://doi.org/10.1109/incet49848.2020.9153972. |
5 | Business Today 2021, ‘How IPL owners, teams, franchises make money? All you need to know’, Business Today, 27 April [online] https://www.businesstoday.in/latest/trends/story/howipl-owners-teams-franchises-make-money-all-you-need-to-know-294481-2021-04-27 (accessed 27 January 2022) |
6 | Chauhan N. , Jain P. , Gupta P. , Kumar N. , Bajaj N. (2018) A study of the application of operations research in the valuation of players in IPL, International Journal of Advance Research, Ideas and Innovations in Technology 4: (5) pp. 719–723. |
7 | Coluccia D. , Fontana S. , Solimene S. (2018) An application of the option-pricing model to the valuation of a football player in the ‘Serie A League’, International Journal of Sport Management and Marketing 18: (1/2) pp. 155–168. |
8 | Cricbuzz 2018, IPL Auction 2018 [online] https://www.cricbuzz.com/ (accessed 27 January 2022). |
9 | Das N.R. , Priya R. , Mukherjee I. , Paul G. 2021, Modified hedonic based price prediction model for players in IPL auction, 2021 12th International Conference on Computing Communication and Networking Technologies (ICCCNT), available at: http://doi.org/10.1109/icccnt51525.2021.9580108. |
10 | Davis J. , Perera H. , Swartz T.B. Player evaluation in twenty cricket, Journal of Sports Analytics 1: (1) pp. 19–31. |
11 | Dey P.K. , Banerjee A. , Ghosh D.N. , Mondal A.C. AHP-neural network-based player price estimation in IPL, International Journal of Hybrid Information Technology 7: (3) pp. 15–24. |
12 | Easton T. , Newell S. Are daily fantasy sports gambling? Journal of Sports Analytics 5: (1) pp. 35–43. |
13 | ICC 2022, The Three Formats of Cricket [online] https://www.icccricket.com/about/cricket/game-formats/the-three-formats (accessed 27 January 2022). |
14 | India Today 2021, IPL is a revelation, the camaraderie amongst cricketers from all over the world is amaz-ing: Aftab Shivdasani, India Today, 9 April [online] https://www.indiatoday.in/sports/ipl-2021/story/ipl-is-a-revelation-the-camaraderie-amongst-cricketers-from-all-over-the-world-is-amazing-aftab-shivdasani-1789062-2021-04-09 (accessed 27 January 2022) |
15 | Jayanth, S.B. , Anthony, A. , Abhilasha, G. , Shaik N. , Srinivasa G. A team recommendation system and outcome prediction for the game of Cricket, Journal of Sports Analytics 4: (4) pp. 263–273. |
16 | Kansal, P. , Kumar, P. , Arya, H. , Methaila A. 2014, Player valuation in Indian Premier League auction using data mining technique, 2014 International Conference on Con-temporary Computing and Informatics (IC3I), available at: http://doi.org/10.1109/ic3i.2014.7019707. |
17 | Kapadia, K. , Abdel-Jaber, H. , Thabtah, F. , Hadi W. 2020, Sport analytics for cricket game results using machine learning: An experimental study, Applied Computing and Informatics, In Press, available at: http://doi.org/10.1016/j.aci.2019.11.006. |
18 | Kargal R. IPL 2021: How data analytics is changing cricket, The Bridge, 13 April [online] https://thebridge.in/ipl-2021/ipl-2021-how-data-analytics-changing-cricket-20753#:∼:text=The%20on%2Dfield%20possibilities%20with,captured%20from%20every%20cricket%20match.&text=The%20larger%20the%20sample%20size,to%20a%20bowler%20as%20well (accessed 27 January 2022). |
19 | Katewa S. 2021, How does the IPL auction work? (with Rules & Process), Cricket Mastery, 26 May [online] https://cricketmastery.com/how-does-the-ipl-auction-work/(accessed 27 January 2022). |
20 | Malvania U. 2019, IPL franchises to have heavier salary purses in 2018’, Business Standard, 16 December [online] https://www.business-standard.com/article/current-affairs/ipl-franchises-to-have-heavier-salary-purses-in-2018-1171206008591.html (accessed 27 January 2022). |
21 | Mu E. (2016) , Who reallywon the FIFA2014 Golden BallAward?: What sports can learn from multi-criteria decision analysis, International Journal of Sport Management and Marketing 16: (3/4/5/6), pp. 239–258. |
22 | Navarro O. The numbers revolution: Rise of analytics in sports is breaking old traditions’, The California Aggie, August [online] https://theaggie.org/2020/03/18/the-numbers-revolution-rise-of-analytics-in-sports-is-breaking-old-traditions/(accessed January, 2022) |
23 | Pantzalis V.C. , Tjortjis C. 2020, Sports analytics for football league table and player performance prediction, 2020 11th International Conference on Information, Intel-ligence, Systems and Applications (IISA), available at: http://doi.org/10.1109/iisa50023.2020.9284352. |
24 | Plumley D. , Ramchandani G. , Wilson R. Mind the gap: an analysis of competitive balance in the English Football League system, International Journal of Sport Management and Marketing 18: (5) 357–375. |
25 | Rani P.J. , Kulkarni A. , Kamath A.V. , Menon A. , Dhatwalia P. , Rishabh D. 2020, Prediction of player price in IPL auction using machine learning regression algorithms, 2020 IEEE International Conference on Electronics, Computing and Communication Technologies (CONECCT), available at: http://doi.org/10.1109/conecct50063.2020.9198668. |
26 | Senevirathne, H.K.W. , Manage A.B. Predicting the winning percentage of limited-overs cricket using the Pythagorean formula, Journal of Sports Analytics 7: (3) pp. 169–183. |
27 | Sportskeeda 2021, IPL Auction Rules 2021 [online] https://www.sportskeeda.com/cricket/ipl-auction-rules (accessed 27 Jan 2022) |
28 | TNN 2013, ‘Indian Premier League: How it all started’, The Times of India, 2 April [online] https://timesofindia.indiatimes.com/ipl-history/indian-premier-league-how-it-all-started/articleshow/19337875.cms (accessed January 2022). |
29 | Wickramasinghe, I. Naive Bayes approach to predict the winner of an ODI cricket game, Journal of Sports Analytics 6: (2) pp. 75–84. |
Appendices
ANNEXURE-1
Exhibit 1: Proposed base, actual & auction prices and algorithm efficiency for Indian players auctioned in the 2022 IPL Mega Auction
S. no | Player | Country | Identified most similar player | Actual base price (in million Rupees) | Proposed base price (in million Rupees) | Actual auction price (in million Rupees) | Actual time taken for auction | Time taken with the proposed base price |
1 | Mohammed Shami | Indian | MM Sharma | 20000000 | 24000000 | 62500000 | 0 | 0 |
2 | WP Saha | Indian | SS Tiwary | 10000000 | 8000000 | 19000000 | 6 | 0 |
3 | J Yadav | Indian | STR Binny | 10000000 | 5000000 | 17000000 | 24 | 9 |
4 | V Shankar | Indian | KV Sharma | 5000000 | 50000000 | 14000000 | 49 | 43 |
5 | VR Aaron | Indian | PJ Sangwan | 5000000 | 15000000 | 5000000 | 0 | 0 |
6 | Gurkeerat Singh | Indian | STR Binny | 5000000 | 5000000 | 5000000 | 66 | 60 |
7 | DL Chahar | Indian | Sandeep Sharma | 20000000 | 30000000 | 1.4E+08 | 11 | 26 |
8 | AT Rayudu | Indian | KV Sharma | 20000000 | 50000000 | 67500000 | 0 | 0 |
9 | S Dube | Indian | STR Binny | 5000000 | 5000000 | 40000000 | 31 | 6 |
10 | RV Uthappa | Indian | SV Samson | 20000000 | 80000000 | 20000000 | 29 | 11 |
11 | S Singh | Indian | R Vinay Kumar | 2000000 | 10000000 | 2000000 | 34 | 39 |
12 | Jagadeesan | Indian | Gurkeerat Singh | 2000000 | 7500000 | 2000000 | 48 | 43 |
13 | KM Asif | Indian | S Gopal | 2000000 | 2000000 | 2000000 | 0 | 0 |
14 | Deshpande | Indian | RA Tripathi | 2000000 | 34000000 | 2000000 | 0 | 0 |
15 | SN Thakur | Indian | UT Yadav | 20000000 | 42000000 | 1.08E+08 | 35 | 24 |
16 | K Ahmed | Indian | UT Yadav | 5000000 | 42000000 | 52500000 | 42 | 31 |
17 | C Sakariya | Indian | AS Rajpoot | 5000000 | 30000000 | 42000000 | 51 | 52 |
18 | KS Bharat | Indian | Gurkeerat Singh | 2000000 | 7500000 | 20000000 | 0 | 0 |
19 | Kuldeep Yadav | Indian | P Sahu | 10000000 | 2000000 | 20000000 | 7 | 17 |
20 | KL Nagarkoti | Indian | Basil Thampi | 4000000 | 9500000 | 11000000 | 8 | 0 |
21 | Mandeep Singh | Indian | A Choudhary | 5000000 | 3000000 | 11000000 | 0 | 0 |
22 | Lalit Yadav | Indian | Kuldeep Yadav | 2000000 | 58000000 | 6500000 | 37 | 6 |
23 | Praveen Dubey | Indian | Anureet Singh | 2000000 | 3000000 | 5000000 | 8 | 0 |
24 | SN Khan | Indian | Gurkeerat Singh | 2000000 | 7500000 | 2000000 | 0 | 0 |
25 | RV Patel | Indian | SN Thakur | 2000000 | 26000000 | 2000000 | 2 | 0 |
26 | SS Iyer | Indian | AM Rahane | 20000000 | 40000000 | 1.23E+08 | 18 | 19 |
27 | N Rana | Indian | Harbhajan Singh | 10000000 | 20000000 | 80000000 | 32 | 42 |
28 | UT Yadav | Indian | PJ Sangwan | 20000000 | 15000000 | 20000000 | 14 | 9 |
29 | AM Rahane | Indian | MK Tiwary | 10000000 | 10000000 | 10000000 | 13 | 2 |
30 | M Shahrukh Khan | Indian | N Rana | 4000000 | 34000000 | 90000000 | 0 | 0 |
31 | S Dhawan | Indian | AM Rahane | 20000000 | 40000000 | 82500000 | 26 | 15 |
32 | RD Chahar | Indian | Harbhajan Singh | 7500000 | 20000000 | 52500000 | 0 | 0 |
33 | H Brar | Indian | Gurkeerat Singh | 2000000 | 7500000 | 38000000 | 36 | 5 |
34 | Prabhsimran Singh | Indian | R Vinay Kumar | 2000000 | 10000000 | 6000000 | 10 | 26 |
35 | Sandeep Sharma | Indian | PP Chawla | 5000000 | 42000000 | 5000000 | 9 | 0 |
36 | Avesh Khan | Indian | STR Binny | 2000000 | 5000000 | 1E+08 | 22 | 0 |
37 | KH Pandya | Indian | P Negi | 20000000 | 10000000 | 82500000 | 11 | 4 |
38 | DJ Hooda | Indian | STR Binny | 7500000 | 5000000 | 57500000 | 3 | 0 |
39 | MK Pandey | Indian | MM Sharma | 10000000 | 24000000 | 46000000 | 57 | 28 |
40 | K Gowtham | Indian | Mohammed Shami | 5000000 | 30000000 | 9000000 | 0 | 0 |
41 | S Nadeem | Indian | P Sahu | 5000000 | 2000000 | 5000000 | 23 | 11 |
42 | AS Rajpoot | Indian | PJ Sangwan | 2000000 | 15000000 | 5000000 | 11 | 15 |
43 | M Vohra | Indian | MA Agarwal | 2000000 | 10000000 | 2000000 | 22 | 20 |
44 | Ishan Kishan | Indian | YK Pathan | 20000000 | 19000000 | 1.53E+08 | 40 | 30 |
45 | M Ashwin | Indian | A Mishra | 2000000 | 40000000 | 16000000 | 18 | 0 |
46 | JD Unadkat | Indian | YK Pathan | 7500000 | 19000000 | 13000000 | 50 | 29 |
47 | M Markande | Indian | Basil Thampi | 5000000 | 9500000 | 6500000 | 8 | 0 |
48 | Basil Thampi | Indian | PJ Sangwan | 3000000 | 15000000 | 3000000 | 6 | 4 |
49 | Anmolpreet Singh | Indian | MA Agarwal | 2000000 | 10000000 | 2000000 | 0 | 0 |
50 | HV Patel | Indian | PP Chawla | 20000000 | 42000000 | 1.08E+08 | 15 | 15 |
51 | KD Karthik | Indian | YK Pathan | 20000000 | 19000000 | 55000000 | 33 | 29 |
52 | M Lomror | Indian | Gurkeerat Singh | 4000000 | 7500000 | 9500000 | 55 | 6 |
53 | S Kaul | Indian | Mohammed Siraj | 7500000 | 26000000 | 7500000 | 32 | 17 |
54 | KV Sharma | Indian | Basil Thampi | 5000000 | 9500000 | 5000000 | 0 | 0 |
55 | P Krishna | Indian | UT Yadav | 10000000 | 42000000 | 1E+08 | 0 | 0 |
56 | D Padikkal | Indian | KK Nair | 20000000 | 56000000 | 77500000 | 32 | 22 |
57 | YS Chahal | Indian | Harbhajan Singh | 20000000 | 20000000 | 65000000 | 30 | 30 |
58 | R Ashwin | Indian | Harbhajan Singh | 20000000 | 20000000 | 50000000 | 11 | 0 |
59 | R Parag | Indian | STR Binny | 3000000 | 5000000 | 38000000 | 0 | 0 |
60 | N Saini | Indian | UT Yadav | 7500000 | 42000000 | 26000000 | 0 | 6 |
61 | KK Nair | Indian | Gurkeerat Singh | 5000000 | 7500000 | 14000000 | 0 | 0 |
62 | KC Cariappa | Indian | RA Tripathi | 2000000 | 34000000 | 3000000 | 0 | 0 |
63 | K Yadav | Indian | M Ashwin | 2000000 | 22000000 | 2000000 | 42 | 31 |
64 | Washington Sundar | Indian | R Ashwin | 15000000 | 76000000 | 87500000 | 45 | 35 |
65 | RA Tripathi | Indian | KD Karthik | 4000000 | 74000000 | 85000000 | 0 | 0 |
66 | Abhishek Sharma | Indian | STR Binny | 2000000 | 5000000 | 65000000 | 20 | 0 |
67 | B Kumar | Indian | DS Kulkarni | 20000000 | 7500000 | 42000000 | 0 | 5 |
68 | T Natarajan | Indian | Kuldeep Yadav | 10000000 | 58000000 | 40000000 | 14 | 0 |
69 | Kartik Tyagi | Indian | Mohammed Shami | 2000000 | 30000000 | 40000000 | 0 | 0 |
70 | S Gopal | Indian | KV Sharma | 2000000 | 50000000 | 7500000 | 9 | 13 |
71 | Priyam Garg | Indian | NV Ojha | 2000000 | 14000000 | 2000000 | 39 | 6 |
72 | J Suchith | Indian | Anureet Singh | 2000000 | 3000000 | 2000000 | 23 | 23 |
Exhibit 2: Proposed base, actual & auction prices and algorithm efficiency for Foreign players auctioned in the 2022 IPL Mega Auction
S. no | Player | Country | Identified most similar player | Actual base price (in million Rupees) | Proposed base price (in million Rupees) | Actual auction price (in million Rupees) | Actual time taken for auction | Time taken with the proposed base price |
1 | L Ferguson | Foreign | Shakib Al Hasan | 20000000 | 20000000 | 1E+08 | 17 | 0 |
2 | DA Miller | Foreign | KS Williamson | 10000000 | 30000000 | 30000000 | 4 | 9 |
3 | A Joseph | Foreign | K Rabada | 7500000 | 42000000 | 24000000 | 13 | 4 |
4 | JJ Roy | Foreign | SW Billings | 20000000 | 10000000 | 20000000 | 8 | 0 |
5 | DJ Bravo | Foreign | GJ Maxwell | 20000000 | 90000000 | 44000000 | 15 | 0 |
6 | CJ Jordan | Foreign | CR Woakes | 20000000 | 74000000 | 36000000 | 22 | 0 |
7 | M Santner | Foreign | AJ Tye | 10000000 | 72000000 | 19000000 | 12 | 0 |
8 | AF Milne | Foreign | CJ Jordan | 15000000 | 10000000 | 19000000 | 13 | 0 |
9 | MR Marsh | Foreign | CR Brathwaite | 20000000 | 20000000 | 65000000 | 0 | 5 |
10 | DA Warner | Foreign | DJ Bravo | 20000000 | 64000000 | 62500000 | 0 | 0 |
11 | Mustafizur Rahman | Foreign | PJ Cummins | 20000000 | 54000000 | 20000000 | 1 | 0 |
12 | TL Seifert | Foreign | CJ Jordan | 5000000 | 10000000 | 5000000 | 30 | 40 |
13 | L Ngidi | Foreign | CJ Jordan | 5000000 | 10000000 | 5000000 | 29 | 3 |
14 | PJ Cummins | Foreign | GJ Maxwell | 20000000 | 90000000 | 72500000 | 0 | 5 |
15 | SW Billings | Foreign | Mohammad Nabi | 20000000 | 10000000 | 20000000 | 0 | 5 |
16 | TG Southee | Foreign | CJ Jordan | 15000000 | 10000000 | 15000000 | 0 | 10 |
17 | Mohammad Nabi | Foreign | DT Christian | 10000000 | 15000000 | 10000000 | 39 | 44 |
18 | L Livingstone | Foreign | JJ Roy | 10000000 | 15000000 | 1.15E+08 | 29 | 3 |
19 | K Rabada | Foreign | SR Watson | 20000000 | 40000000 | 92500000 | 37 | 27 |
20 | J Bairstow | Foreign | AJ Finch | 15000000 | 62000000 | 67500000 | 40 | 40 |
21 | NT Ellis | Foreign | C Munro | 7500000 | 19000000 | 7500000 | 53 | 48 |
22 | IC Porel | Foreign | CJ Jordan | 2000000 | 10000000 | 2500000 | 0 | 0 |
23 | JO Holder | Foreign | JP Duminy | 15000000 | 10000000 | 87500000 | 31 | 0 |
24 | E Lewis | Foreign | JJ Roy | 20000000 | 15000000 | 20000000 | 9 | 0 |
25 | TH David | Foreign | CJ Jordan | 4000000 | 10000000 | 82500000 | 23 | 23 |
26 | J Archer | Foreign | TG Southee | 20000000 | 10000000 | 80000000 | 0 | 0 |
27 | DR Sams | Foreign | AJ Tye | 10000000 | 72000000 | 26000000 | 0 | 0 |
28 | RP Meredith | Foreign | CJ Jordan | 10000000 | 10000000 | 10000000 | 47 | 47 |
29 | FA Allen | Foreign | CJ Jordan | 7500000 | 10000000 | 7500000 | 0 | 0 |
30 | JR Hazlewood | Foreign | AJ Tye | 20000000 | 72000000 | 77500000 | 27 | 0 |
31 | S Rutherford | Foreign | CR Brathwaite | 10000000 | 20000000 | 10000000 | 0 | 0 |
32 | J Behrendorff | Foreign | B Stanlake | 7500000 | 5000000 | 7500000 | 38 | 43 |
33 | S Hetmyer | Foreign | SW Billings | 15000000 | 10000000 | 85000000 | 0 | 0 |
34 | TA Boult | Foreign | TG Southee | 20000000 | 10000000 | 80000000 | 0 | 10 |
35 | JDS Neesham | Foreign | CJ Jordan | 15000000 | 10000000 | 15000000 | 30 | 40 |
36 | N Pooran | Foreign | DT Christian | 15000000 | 15000000 | 1.08E+08 | 0 | 5 |
37 | M Jansen | Foreign | AJ Tye | 5000000 | 72000000 | 42000000 | 54 | 42 |
38 | AK Markram | Foreign | C Munro | 10000000 | 19000000 | 26000000 | 0 | 0 |