The collection, analysis and exploitation of footballer attributes: A systematic review
Abstract
There is growing on-going research into how footballer attributes, collected prior to, during and post-match, may address the demands of clubs, media pundits and gaming developers. Focusing upon individual player performance analysis and prediction, we examined the body of research which considers different player attributes. This resulted in the selection of 132 relevant papers published between 1999 and 2020. From these we have compiled a comprehensive list of player attributes, categorising them as static, such as age and height, or dynamic, such as pass completions and shots on target. To indicate their accuracy, we classified each attribute as objectively or subjectively derived, and finally by their implied accessibility and their likely personal and club sensitivity. We assigned these attributes to 25 logical groups such as passing, tackling and player demographics. We analysed the relative research focus on each group and noted the analytical methods deployed, identifying which statistical or machine learning techniques were used. We reviewed and considered the use of character trait attributes in the selected papers and discuss more formal approaches to their use. Based upon this we have made recommendations on how this work may be developed to support elite clubs in the consideration of transfer targets.
1Introduction and motivation for study
There has been significant progress in the development of techniques to deliver more effective automated and intelligent analysis of footballer and team performance (de Sousa, 2011). The demands of broadcasters, media pundits, gaming developers and the clubs themselves to gather accurate and timely player attributes have continued to grow. In all cases the financial rewards which may result from the interpretation of these data are a very significant driver. For example, the annual transfer fee investments in the five major European championships (English Premier League, Spanish La Liga, German Bundesliga, Italian Serie A and French Ligue 1) increased by 429% to Euro 6,622M between 2010 and 2019 (Poli et al., 2019). In the gaming industry, FIFA 19 generated $786M in 2019 (Saed, 2020). For the gaming developers, continuing to improve the realism of their products is a key business driver. For the increasing number of broadcasters and pundits, the ability to present and discuss player and team activities and performances better than the competition is a major component of their ability to attract audiences and therefore maximise their subscriptions and advertising revenues. For example, in 2018/19 Sky TV’s global football revenues were Euro 28.9 Bn (Delloite, 2020). For the clubs themselves, the pursuit of all opportunities to improve the performance of individual players and the team as a whole is vital to their businesses. The combined revenues of clubs in the five major European championships is projected to grow by over 42% from Euro 11.3Bn in season 2013/14 to 16.1Bn in 2020/21 (Deloitte, 2017; Deloitte, 2020). The pressures on clubs to identify successful transfer targets, at the right fee and consequent salary and bonus package, is a very significant issue for all clubs and particularly for the elite clubs facing seemingly unending price escalation.
There is considerable on-going research into how player and team attributes, both static and dynamic during matches, may be collected automatically, for example using automated video data collection and analysis (Filetti et al., 2017). This is often supplemented by experts, usually ex-players (PA Sport, 2020) and in the case of SoFIFA input from a community of 8,000 coaches, scouts and season ticketholders (SoFIFA, 2020).
In a variety of different player and match attributes and scenarios, statistical (Gelade & Hvattum, 2020) and increasingly artificial intelligence, in the main machine learning (Stanojevic, & Gyarmati, 2016), methods have been deployed to draw conclusions and make useful predictions of individual and team performances.
We have, however, found very few examples of analyses including player character traits such as motivation, cognitive functions, self-control, sustained attention etc. This is in stark contrast to other industry recruitment activities where the calibration of such traits is considered critical. We suggest that the inclusion of an appropriate selection of such attributes presents the opportunity for a game-changing step forward in footballer analytics, in particular, in the selection of potential transfer targets.
2Methods
2.1Data collection
A systematic review of papers relevant to sporting analytics, with a specific focus on those addressing football (soccer) was conducted. No historical time limit was placed upon the papers considered, with over 1,500 initially selected papers falling within a timeframe of January 1999 to January 2021. All papers identifying footballer attributes, such as passing, tackling, assists etc., for review, analysis or predictive purposes, were curated. A focus upon eleven-a-side competitive professional football was maintained and papers addressing the analyses of small sided games (such as five-a-side games, training/practice games and video game matches) were excluded unless novel footballer attributes were identified. This resulted in a collection of 132 directly relevant papers (Table 12). With the aim of achieving a comprehensive review of relevant research, the identification of these papers included the review of relevant papers referenced by each, as well as those citing them, and where appropriate these were included for curation. In each case the publishing journal, conference or organization was noted. Additionally, where analyses were conducted, the analytical methods (statistical analysis, machine learning, mixed) were recorded. In order to determine whether the analyses were statistical or machine learning methods we adopted the accepted definition that statistical models (e.g. ANOVA, Chi squared analysis, Spearman correlation test) are designed for inference and description of the relationships between variables, whereas machine learning models (e.g. decision tree, neural networks) are designed to make the most accurate predictions possible (Rajula et al., 2020).
Table 12
Selected papers
Paper (Citation) | Publisher | Year |
The foundations of tactics and strategy in team sports (Godbout & Bouthier, 1999) | Journal of teaching in physical education | 1999 |
Talent identification and development in soccer (Williams & Reilly, 2000) | Journal of sports sciences | 2000 |
The roles of talent, physical precocity and practice in the development of soccer expertise (Helsen et al., 2000) | Journal of sports sciences | 2000 |
Match performance of high-standard soccer players with special reference to development of fatigue (Mohr et al,. 2003) | Journal of sports sciences | 2002 |
An analysis of home advantage in the English Football Premiership (Thomas et al., 2004) | Perceptual and motor skills | 2004 |
Computerized Real-Time Analysis of Football Games (Beetz et al., 2005) | IEEE pervasive computing | 2005 |
An option pricing framework for valuation of football players (Tunaru et al., 2005) | Review of financial economics | 2005 |
Are winners different from losers? Performance and chance in the FIFA World Cup Germany 2006 (Lago, 2006) | International Journal of Performance Analysis in Sport | 2006 |
Predicting football results using bayesian nets and other machine learning techniques (Joseph et al., 2006) | Knowledge-Based Systems | 2006 |
Mathematical analysis of a soccer game. Part I: Individual and collective behaviors (Yue et al., 2008a) | Studies in applied mathematics | 2008 |
Mathematical analysis of a soccer game. Part II: Energy, spectral, and correlation analyses (Yue et al., 2008b) | Studies in Applied Mathematics | 2008 |
ASPOGAMO: Automated Sports Game Analysis Models (Beetz et al., 2009) | International Journal of Computer Science in Sport | 2009 |
Game creativity analysis using neural networks (Memmert & Perl, 2009) | Journal of sports sciences | 2009 |
Differentiating the top English premier league football clubs from the rest of the pack: Identifying the keys to success (Oberstone, 2009) | Journal of Quantitative Analysis in Sports | 2009 |
Technical performance during soccer matches of the Italian Serie A league: Effect of fatigue and competitive level (Rampinini et al., 2009) | Journal of science and medicine in sport | 2009 |
An overview of automatic event detection in soccer matches (de Sousa et al., 2011) | IEEE Workshop on Applications of Computer Vision | 2011 |
Analyzing Soccer Goalkeeper Performance Using a Metaphor-Based Visualization (Rusu et al., 2011) | 15th International Conference on Information Visualisation | 2011 |
On the Development of a Soccer Player Performance Rating System for the English Premier League (McHale et al., 2012) | Interfaces | 2012 |
Performance analysis in football A critical review and implications for future research (MacKenzie & Cushion, 2013) | Journal of sports sciences | 2012 |
Big 2’s and Big 3’s: Analyzing How a Team’s Best Players Complement Each Other (Ayer, 2012) | MIT Sloan Sports Analytics Conference | 2012 |
Inter-operator reliability of live football match statistics from OPTA Sportsdata (Lui et al., 2013) | International Journal of Performance Analysis in Sport | 2013 |
Competing together: Assessing the dynamics of team–team and player–team synchrony in professional association football (Duarte et al., 2013) | Human movement science | 2013 |
Match performance and physical capacity of players in the top three competitive standards of English professional soccer (Bradley et al., 2013) | Human movement science | 2013 |
Team play in football: How science supports FC Barcelona’s training strategy (Chassy, 2013) | Psychology | 2013 |
The hidden foundation of field vision in English Premier League (EPL) soccer players (Jordet et al., 2013) | Proceedings of the MIT sloan sports analytics conference | 2013 |
Science and football: Evaluating the influence of science on performance (Drust & Green, 2013) | Journal of sports sciences | 2013 |
Real-Time Crowdsourcing of Detailed Soccer Data (Perin et al., 2013) | HAL (hal.inria.fr) | 2013 |
SoccerStories: A Kick-off for Visual Soccer Analysis (Perin et al., 2013) | IEEE transactions on visualization and computer graphics | 2013 |
The possession game? A comparative analysis of ball retention and team success in European and international football (Collet, 2013) | Journal of sports sciences | 2013 |
A mixed effects model for identifying goal scoring ability of footballers (McHale & Szczepański, 2014) | Journal of the Royal Statistical Society: Series A (Statistics in Society) | 2013 |
Win at home and draw away’: Automatic formation analysis highlighting the differences in home and away team (Bialkowski et al., 2014) | Proceedings of 8th annual MIT sloan sports analytics conference | 2014 |
Identifying Team Style in Soccer Using Formations Learned from Spatiotemporal Tracking Data (Bialkowski et al., 2014) | IEEE international conference on data mining workshop | 2014 |
Intelligent systems for analyzing soccer games: The weighted centroid (Clemente et al., 2014) | Ingeniería e Investigación | 2014 |
Dynamical stability and predictability of football players: The study of one match (Couceiro et al., 2014) | Entropy | 2014 |
Match analysis in football: A systematic review (Sarmento et al., 2014) | Journal of sports sciences | 2014 |
Steven Gerrard and Frank Lampard in 2013/14: A Statistical Comparison (Oberstone J.L., 2014) | EPL Index | 2014 |
A novel way to soccer match prediction (Shin & Gasparyan, 2014) | Stanford University: Department of Computer Science | 2014 |
Ball recovery patterns as a performance indicator in elite soccer (Barriera et al., 2014) | Proceedings of the Institution of Mechanical Engineers, Part P: Journal of Sports Engineering and Technology | 2014 |
How important is it to score a goal? The influence of the scoreline on match performance in elite soccer (Lago-Peñas & Gómez-López, 2014) | Perceptual and motor skills | 2014 |
Evaluation of research using computerised tracking systems (Amisco and Prozone) to analyze physical performance in elite soccer: A systematic review (Castellano et al., 2014) | Sports medicine | 2014 |
Football Player’s Performance and Market Value (He et al., 2015) | MLSA@ PKDD/ECML | 2015 |
Performance profiles of football teams in the UEFA champions league considering situational efficiency (Liu et al., 2015) | International Journal of Performance Analysis in Sport | 2015 |
Why Soccer’s Most Popular Advanced Stat Kind Of Sucks (Bertin, 2015) | Deadspin | 2015 |
Association between playing tactics and creating scoring opportunities in counterattacks from United States Major League Soccer games (Gonzalez-Rodenas et al., 2016) | International Journal of Performance Analysis in Sport | 2016 |
Visual analysis of pressure in football (Andrienko et al., 2017) | Data Mining and Knowledge Discovery | 2016 |
Developing a Data-Driven Player Ranking in Soccer Using Predictive Model Weights (Brooks et al., 2016) | International Conference on Knowledge Discovery and Data Mining | 2016 |
Periodization Training Focused on Technical Tactical Ability in Young Soccer Players (Aquino et al., 2016) | Journal of Strength and Conditioning Research | 2016 |
The micro-macro link in understanding sport tactical behaviours: Integrating information and action at different levels of system analysis in sport (Araújo et al., 2015) | Movement & Sport Sciences-Science & Motricité | 2016 |
Age-related effects of practice experience on collective behaviours of football players in small-sided games (Barnabé et al., 2016) | Human movement science | 2016 |
Discovering Team Structures in Soccer from Spatiotemporal Data (Bialkowski et al., 2016) | Transactions on Knowledge and Data Engineering | 2016 |
Real time quantification of dangerousity in football using spatiotemporal tracking data (Link et al., 2016) | PloS one | 2016 |
Coordination analysis of players’ distribution in football using cross-correlation and vector coding techniques (Moura et al., 2016) | Journal of sports sciences | 2016 |
Big data and tactical analysis in elite soccer: Future challenges and opportunities for sports science (Rein & Memmert, 2016) | SpringerPlus | 2016 |
Identifying keys to win in the Chinese professional soccer league (Mao et al., 2016) | International Journal of Performance Analysis in Sport | 2016 |
Visual exploration of match performance based on football movement data using the continuous triangular model (Zhang et al., 2016) | Applied Geography | 2016 |
The Pressing Game: Optimal Defensive Disruption in Soccer (Bojinov & Bornn, 2016) | Procedings of MIT Sloan Sports Analytics. | 2016 |
Can Artificial Intelligence Modelling Approaches Assist Football Clubs In Identifying Transfer Targets, While Maintaining A Fair Transfer Market Using Player Performance Data? (Ahmed, 2016) | PhD diss., Cardiff Metropolitan University | 2016 |
When do soccer players peak? (Dendir, 2016) | Journal of Sports Analytics | 2016 |
Modelling the financial contribution of soccer players to their clubs (Sæbø & Hvattum, 2019) | Journal of Sports Analytics | 2016 |
Towards data-driven football player assessment (Stanojevic & Gyarmati, 2016) | EEE 16th International Conference on Data Mining Workshops | 2016 |
Quantifying the relation between performance and success in soccer (Pappalardo & Cintia, 2018) | Advances in Complex Systems | 2016 |
Beyond completion rate: Evaluating the passing ability of footballers (Szczepański & McHale, 2016) | Journal of the Royal Statistical Society: Series A (Statistics in Society) | 2016 |
Bring it to the Pitch: Combining Video and Movement Data to Enhance Team Sport Analysis (Stein et al., 2017) | IEEE transactions on visualization and computer graphics | 2017 |
A study of relationships among technical, tactical, physical parameters and final outcomes in elite soccer matches as analyzed by a semiautomatic video tracking system (Goldlücke & Keim, 2017) | Perceptual and Motor Skills | 2017 |
Not all passes are created equal (Power et al., 2017) | ACM SIGKDD international conference on knowledge discovery and data mining | 2017 |
Hypernetworks reveal compound variables that capture cooperative and competitive interactions in a soccer match (Ramos et al., 2017) | Frontiers in Psychology | 2017 |
Which pass is better? Novel approaches to assess passing effectiveness in elite soccer (rein et al., 2017) | Human movement science | 2017 |
The Leicester City Fairytale?”: Utilizing New Soccer Analytics Tools to Compare Performance in the 15/16 & 16/17 EPL Seasons (Ruiz et al., 2017) | International Conference on Knowledge Discovery and Data Mining | 2017 |
A Bayesian inference approach for determining player abilities in soccer (Whitaker et al., 2017) | arXiv preprint arXiv | 2017 |
What’s in a game? A systems approach to enhancing performance analysis in football (McLean et al., 2017) | PloS one | 2017 |
Beyond crowd judgments: Data-driven estimation of market value in association football (Müller et al., 2017) | European Journal of Operational Research | 2017 |
Pricing Football Players Using Neural Networks (Dey, 2017) | arXiv preprint arXiv: | 2017 |
Predicting the Potential of Professional Soccer Players (Vroonen et al., 2017) | Proceedings of the 4th Workshop on Machine Learning and Data Mining for Sports Analytics | 2017 |
Physics-based modeling of pass probabilities in soccer (Spearman et al., 2017) | Proceeding of the 11th MIT Sloan Sports Analytics Conference. | 2017 |
State of the Art of Sports Data Visualization (Perin et al., 2018) | Computer Graphics Forum | 2018 |
Player valuation in European football (Extended version) (Nsolo et al., 2018) | Linköping University, Sweden | 2018 |
A weighted plus minus metric for individual soccer player performance (Schultze & Wellbrock, 2018) | Journal of Sports Analytics | 2018 |
Exploring the effects of playing formations on tactical behaviour and external workload during football small-sided games (Baptista et al., 2020) | The Journal of Strength & Conditioning Research | 2018 |
Wide Open Spaces: A statistical technique for measuring space creation in professional soccer (Fernandez & Bornn, 2018) | Sloan Sports Analytics Conference | 2018 |
Football Match Prediction Using Players Attributes (Danisik et al., 2018) | World Symposium on Digital Intelligence for Systems and Machines (DISA) | 2018 |
Quantifying the Value of Transitions in Soccer via Spatiotemporal Trajectory Clustering (Hobbs et al., 2018) | MIT Sloan Sports Analytics Conference. | 2018 |
Identifying key players in soccer teams using network analysis and pass difficulty (McHale & Relton, 2018) | European Journal of Operational Research | 2018 |
Player Performance Prediction in Football Game (Pariath et al., 2018) | Second International Conference on Electronics, Communication and Aerospace Technology | 2018 |
Singing it for “us”: Team passion displayed during national anthems is associated with subsequent success (Slater et al., 2018) | European journal of sport science | 2018 |
Artificial neural networks and player recruitment in professional soccer (Barron et al., 2018) | PloS one | 2018 |
Not every pass Can Be An Assist: A Data-Driven Model to Measure Pass Effectiveness in Professional Football (Goes et al., 2019) | Big data, | 2018 |
Pitch actions that distinguish high scoring teams: Findings from five European football leagues in 2015-16 (Sarkat & Chakraborty, 2018) | Journal of Sports Analytics | 2018 |
Technical demands of different playing positions in the UEFA Champions League (Yi et al., 2018) | International Journal of Performance Analysis in Sport | 2018 |
Evaluating Passing Behaviour in Association Football (Håland & Wiig, 2018) | Norwegian University of Science and Technology | 2018 |
Goal scoring in elite male football A systematic review (Pratas et al., 2018) | CIPER, Faculdade de Motricidade Humana, SpertLab, Universidade de Lisboa, Portugal | 2018 |
PlayeRank: Data-driven Performance Evaluation and Player Ranking in Soccer via a Machine Learning Approach (Pappalardo et al., 2019) | ACM Transactions on Intelligent Systems and Technology | 2019 |
The Application of Machine Learning Techniques for Predicting Results in Team Sport: A Review (Bunker & Susnjak, 2019) | arXiv, Cornell University | 2019 |
Sports Analytics Algorithms for Performance Prediction (Apostolou & Tjortjis, 2019) | International Conference on Information, Intelligence, Systems and Applications (IISA) | 2019 |
Team spirit in football: an analysis of players symbolic communication in a match between Argentina and Iceland at the mens 2018 World Cup (Halldorssom, 2019) | Arctic & Antarctic: International Journal of Circumpolar Sociocultural Issues | 2019 |
A case study assessing possession regain patterns in English Premier League Football (Jamil, 2019) | International Journal of Performance Analysis in Sport | 2019 |
A study of Prediction models for football player valuations by quantifying statistical and economic attributes for the global transfer market (Patnaik et al., 2019) | IEEE International Conference on System, Computation, Automation and Networking (ICSCAN) | 2019 |
Machine learning in men’s professional football: Current applications and future directions for improving attacking play (Herold et al., 2019) | International Journal of Sports Science & Coaching | 2019 |
A new paradigm to understand success in professional football: analysis of match statistics in LaLiga for 8 complete seasons (Brito Souza et al.,2019) | International Journal of Performance Analysis in Sport | 2019 |
Actions speak louder than goals: Valuing player actions in soccer (Decroos et al., 2019) | ACM SIGKDD International Conference on Knowledge Discovery & Data Mining | 2019 |
A public data set of spatio-temporal match events in soccer competitions (Pappalardo et al., 2019) | Scientific data | 2019 |
Chinese soccer association super league, 2012–2017: key performance indicators in balance games (Zhou ey al., 2018) | Journal of Performance Analysis in Sport | 2019 |
Valuing On-the-Ball Actions in Soccer: A Critical Comparison of xT and VAEP (Decroos & Davis, 2020) | KU Leuven, Department of Computer Science | 2019 |
Sports Analytics for Football League Table and Player Performance Prediction (Pantzalis & Tjortjis, 2020) | International Hellenic University | 2019 |
Choke or Shine? Quantifying Soccer Players’ Abilities to Perform Under Mental Pressure (Bransen et al., 2019) | MIT Sloan Sports Analytics Conference | 2019 |
Maximizing performance with an eye on the finances a chance-constrained model for football transfer market decisions (Pantuso & Hvattum, 2020) | arXiv Cornell University | 2019 |
The Data Gap in Sports Analytics and How to Close It (Harell & Bajic, 2019) | School of Engineering Science, Simon Fraser University Burnaby, BC, Canada | 2019 |
The creation of goal scoring opportunities in professional soccer Tactical differences between Spanish La Liga English Premier League German Bundesliga and Italian Serie A (Mitrotasios et al., 2019) | International Journal of Performance Analysis in Sport | 2019 |
The open international soccer database for machine learning (Dubitzky et al., 2019) | Machine Learning | 2019 |
Methodological Issues in Soccer Talent Identification Research (Bergkamp et al., 2019) | Sports Medicine | 2019 |
Technical demands across playing positions of the Asian Cup in male football (Ermidis et al., 2019) | International Journal of Performance Analysis in Sport | 2019 |
Automated Machine Learning A Game Changer for Sports Analytics Executive Briefing v1.0 | DataRobot | 2019 |
Evaluating Passing Ability in Association Football Goal scoring in elite male football A systematic review (Håland et al., 2020) | IMA Journal of Management Mathematics | 2019 |
At what age are English Premier League players at their most productive A case study investigating the peak performance years of elite professional footballers (Jamil & Kerruish, 2020) | International Journal of Performance Analysis in Sport | 2020 |
Unlocking the potential of big data to support tactical performance analysis in professional soccer A systematic review (Goes et al., 2020) | European Journal of Sport Science | 2020 |
Exploring elite soccer teams’ performances during different match-status periods of close matches’ comebacks (Gomez et al., 2020) | Chaos, Solitons & Fractals | 2020 |
Identifying playing talent in professional football using artificial neural networks (Barron et al., 2020) | Journal of Sports Sciences | 2020 |
Investigating the impact of the mid-season winter break on technical performance levels across European football –Does a break in play affect team momentum? (Jamil et al., 2020) | International Journal of Performance Analysis in Sport | 2020 |
A Systematic Literature Review of Intelligent Data Analysis Methods for Smart Sport Training (Rajšp & Fister, 2020) | Applied Sciences | 2020 |
On the relationship between+/–ratings and event-level performance statistics (Gelade & Hvattum, 2020) | Journal of Sports Analytics | 2020 |
Constraints on visual exploration of youth football players during 11v11 match play: The influence of playing role pitch position and phase of play (McGuckian et al., 2020) | Journal of Sports Sciences | 2020 |
Behavioral, physiological, and neural signatures of surprise during naturalistic sports viewing (Anthony et al., 2020) | BioRxiv | 2020 |
Success factors in football: an analysis of the German Bundesliga (Lepschy et al., 2020) | International Journal of Performance Analysis in Sport, | 2020 |
Theory to Practice Performance Preparation Models in Contemporary High-Level Sport Guided by an Ecological Dynamics Framework (Woods et al., 2020) | Sports Medicine-Open | 2020 |
A Narrative Review in Sport Analytics (Singh, 2020) | International Journal of Management (IJM) | 2020 |
Applications of Artificial Intelligence in the Game of Football The Global Perspective (Rathi et al., 2020) | Researchers World | 2020 |
Development of Defence and Offence Play Items for Deep Learning Model of Offence Play Analysis in Soccer Game (Matsuoka et al.,2020) | DoctoralProgram in Graduate School of Comprehensive Human Sciences, University of Tsukuba, Japan | 2020 |
Comparison of the football specific tactical performance of women and men in Europe (Mammert et al., 2020) | German Sport University Cologne | 2020 |
Analysis System for Emotional Behavior in Football Professional football players emotional behavior in ghost games in the Austrian Bundesliga (Leitner & Richlan, 2020) | Humanities & Social Sciences Communications. | 2020 |
An Analysis on the Effectiveness of Cooperation in A Soccer Team (Ge et al.,2020) | 2020 15th International Conference on Computer Science & Education (ICCSE) | 2020 |
Where do the best technical football players in the world come from Analysing the association between technical proficiency and geographical origin in elite football (Jamil, 2020) | University of Sussex | 2020 |
Visualizing and Analyzing Disputed Areas in Soccer (Allegre & Vuillemot, 2020) | Conference Visualization in Data Science. 2020 | 2020 |
A Data Science Approach to Football Team Player Selection (Rajesh et al., 2020) | 2020 IEEE International Conference on Electro Information Technology (EIT) | 2020 |
For each paper their main findings and conclusions were summarized (Table 14).
Table 14
Selected papers’ main findings and conclusions
Paper (Citation) | Findings |
The foundations of tactics and strategy in team sports (Godbout & Bouthier, 1999) | Presents approach to be taken by teachers introducing pupils to team sports. Concludes on 4 key elements: the essence of a rapport of strength, or an opposition relationship, between two teams; understanding and appropriate management of its competency network; winning implies defeating the opponents and therefore selection of appropriate tactical and strategic manoeuvres. |
Talent identification and development in soccer (Williams & Reilly, 2000) | Detailed assessment of progress made in talent identification and development in football between 2000 and 2020. Presents some potential predictors of adult high performance footballers, grouped by physical, skill, sociological and psychological attributes and taking account of defined maturation, chance event, development environment and external environment attributes. |
The roles of talent, physical precocity and practice in the development of soccer expertise (Helsen et al., 2000) | Concludes that coaches’ determination of talent appears to be heavily weighted in terms of physical maturation and not technical skill or team play and while standards of competition in soccer is tied to birth-date-determined age categories, this bias is likely to persist. Proposes several potential solutions, including variation of age groups and an increase in individual vs team practice. |
Match performance of high-standard soccer players with special reference to development of fatigue (Mohr et al., 2003) | Results showed: (1) top class soccer players performed more high-intensity running during a game and were better at the Yo-Yo test than moderate players; (2) fatigue occurred towards the end of matches as well as temporarily during the game, independently of competitive standard and of team position; (3) defenders covered a shorter distance in high-intensity running than players in other playing positions; (4) defenders and attackers had a poorer Yo-Yo intermittent recovery test performance than midfielders. |
An analysis of home advantage in the English Football Premiership (Thomas et al., 2004) | Findings showed that mean home advantage was significantly lower for both the periods 1984- 1992 and 1992-2003 than in previous research. However, since there is no statistically significant difference in mean home advantage between these periods, there is no evidence to suggest a continuing reduction in home advantage. The introduction of the 3-points-for-a-win in 1981 may be a major factor in explaining this change. |
Computerized Real-Time Analysis of Football Games (Beetz et al., 2005). | Describes a position tracking system product and related benefit analysis which aims to recognise intentional activities based on position data and automate game interpretation and analysis. |
An option pricing framework for valuation of football players (Tunaru et al., 2005) | Presents a general theoretical framework to enable the financial worth of footballers. Worth is calculated through a combination of club turnover, the number of Opta Index points for the individual player and the sum of Opta Index points for all players playing for the club. Effects of injuries are included. |
Predicting football results using bayesian nets and other machine learning techniques (Joseph et al., 2006) | Compares the results of naive Bayes Network, K-nearest neighbour and Decision Tree machine learning techniques to predict foolball match outcomes using attributes: presence or absence of three key players; playing position of a key player; quality of the opposing team; venue. The Bayesian Network method was the most accurate. |
Mathematical analysis of a soccer game. Part I: Individual and collective behaviors (Yue et al., 2008a) | Time series analysis of a soccer match is given based on detailed data of the 2D motions of all 22 players and of the ball. Various results for individual and collective behaviors of the two teams during the entire first half and during different phases obtained. Relevant parameters, e.g., the possession time, the distance coverage, etc., were derived. |
Mathematical analysis of a soccer game. Part II: Energy, spectral, and correlation analyses (Yue et al., 2008b) | Time series analysis of a soccer match is given based on detailed data of the 2D motions of all 22 players and of the ball for the match. Various quantitative results regarding individual and collective behaviors, major ranges and group of players, including distance coverage, specific kinetic energy, power density, cross- and auto-correlations. |
ASPOGAMO: Automated Sports Game Analysis Models (Beetz et al., 2009) | Presentation of the sports game analysis modeling system. Results show that trajectories of ball and players extracted from video by a camera-based observation subsystem allow the system to classify situations and interpret game events. |
Game creativity analysis using neural networks (Memmert & Perl, 2009) | Defines framework for analysing types of individual development of creative performance based on neural networks. Findings that football and field hockey game creativity could be improved by a structured field-training programme. |
Differentiating the top English premier league football clubs from the rest of the pack: Identifying the keys to success (Oberstone, 2009) | Development of a robust, statistically significant, six independent variable multiple regression model that accounts for the relative success of English Premier League football clubs. Identifies pitch actions that statistically separate the top 4 clubs from the dozen clubs forming the middle of the pack and by a greater contrast, the bottom 4 clubs. |
Technical performance during soccer matches of the Italian Serie A league: Effect of fatigue and competitive level (Rampinini et al., 2009)An overview of automatic event detection in soccer matches (de Sousa et al., 2011) | Examination of the changes in technical and physical performance between the first and second half during Italian Serie A league matches. Concluded that players from the more successful teams covered greater total distance with the ball and high-intensity running distance with the ball and also had more involvements with the ball, completed more short passes, successful short passes, tackles, dribbling, shots and shots on target compared to the less successful teams. Also, showed a significant decline in technical and physical performance between the first and second halves. |
Analyzing Soccer Goalkeeper Performance Using a Metaphor-Based Visualization (Rusu et al., 2011) | Demonstrates a goalkeeper visualization technique, to provide team managers with the ability to evaluate goalkeeper performance qualities or deficiencies, |
On the Development of a Soccer Player Performance Rating System for the English Premier League (McHale et al., 2012) | Describes construction of the EA Sports Player Performance Index explaining how footballer ratings are generated from analytics data. |
Performance analysis in football A critical review and implications for future research (MacKenzie & Cushion, 2013) | Critically review of literature on performance analysis in football, arguing that an alternative approach is warranted given an overemphasis on researching predictive and performance controlling variables. Approach proposed that works with and from performance analysis information to develop research investigating athlete and coach learning. |
Big 2’s and Big 3’s: Analyzing How a Team’s Best Players Complement Each Other (Ayer, 2012) | Concludes that the composition of a National Basketball Association team’s top 2 and top 3 players is a strongly statistically significant factor in the success of a team, and shows which combinations yield over-performance, and which combinations yield underperformance, relative to the team’s talent and coaching quality. |
Competing together: Assessing the dynamics of team–team and player–team synchrony in professional association football (Duarte et al., 2013) | Investigates movement synchronization of players within and between teams during competitive football matches Concludes that stability of synchronisation and relative coordination tendencies was higher in the longitudinal than in lateral direction of the field, whilst the structure of variability was more irregular. |
Match performance and physical capacity of players in the top three competitive standards of English professional soccer (Bradley et al., 2013) | Compares match performance and physical capacity of players across the top three tiers of English football. Found that less distance covered in high-intensity running in the Premier League compared to the lower divisions. Players also covered more high-intensity running when moving down from the Premier League to the Championship but not when moving up a league. |
Team play in football: How science supports FC Barcelona’s training strategy (Chassy, 2013) | Concludes that team play constitutes the core of performance, based upon passing being the hallmark of team-play. Four hypotheses examined and statistically supported: passing density and passing precision predict possession; passing density and passing precision predict shooting opportunities; passing and shooting abilities predict performance; team play, formalised as a compound of self-organisation capability and offensive power. Found no significant relationship between possession and performance. |
Science and football: Evaluating the influence of science on performance (Drust & Green, 2013) | Suggests that the influence of the scientific information that is available has a relatively small influence on the day-to-day activities within the “real world” of football. |
SoccerStories: A Kick-off for Visual Soccer Analysis (Perin et al., 2013) | Presents a visualization interface to support analysts in exploring soccer data, focusing upon player positions and phases of player actions. The interface was validated as useful by two football journalists, an Opta data analyst and a trainer/coach. |
The possession game? A comparative analysis of ball retention and team success in European and international football (Collet, 2013) | Using data from five European leagues, UEFA and FIFA tournaments, the study concludes that both variables were poor predictors at match level once team quality and home advantage taken account of. In league play, effects of greater possession were consistently negative; in the Champions League, it had virtually no impact. |
A mixed effects model for identifying goal scoring ability of footballers (McHale & Szczepański, 2014) | Implementation of a model that can be used to identify the goal scoring ability of footballers. Findings that a player’s team attacking ability does not appear to be a predictor of the number of shots that a player has. |
Win at home and draw away’: Automatic formation analysis highlighting the differences in home and away team (Bialkowski et al., 2014) | Using automatic formation analysis, presents that teams tend to play the same formation at home as away, but with modified execution. In particular, that home team formation is significantly higher up the field compared to away. Concludes that coaches taking a conservative approach at away games suggests that they aim to win home games and draw away games. |
Identifying Team Style in Soccer Using Formations Learned from Spatiotemporal Tracking Data (Bialkowski et al., 2014) | Describes a completely unsupervised system to learn and identify spatial structure of a team directly from data, giving an indication of dominance and tactics. The formation descriptor was shown to represent the characteristic style of teams significantly better (3 times more) than other match descriptors typically used to describe team behaviour. |
Intelligent systems for analyzing soccer games: The weighted centroid (Clemente et al., 2014) | Proposes a modification of the centroid metric (positions of all team members and the position of the ball allows a greater understanding of team behaviors) used in the analysis of soccer games. Analyses using the revised definition of the centroid revealed strong correlations between team centroids in the lateral and longitudinal directions. Results also concluded that winning teams, when on the defensive, maintained a separation between their own centroid and that of the opposing team, making the defence more effective. |
Dynamical stability and predictability of football players: The study of one match (Couceiro et al., 2014) | Results suggest that the most predictable player is the goalkeeper while, conversely, the most unpredictable players are the midfielders. Also concludes that, despite his predictability, the goalkeeper is the most unstable player, while lateral defenders are the most stable during the match. |
Match analysis in football: A systematic review (Sarmento et al., 2014) | Reviews the available literature between 2001 and 2011 on match analysis in adult male football. Findings that the main limitations of the reviewed studies are related to a lack of operational definitions, conflicting classifications of activity or playing positions, and limited studies that consider interactional context in their analyses. |
Steven Gerrard and Frank Lampard in 2013/14: A Statistical Comparison (Oberstone J.L., 2014) | Conclusions from 34 player attributes over 28 matches were that in creativity and attacking there were no significant differences between the players, however, Gerard’s passing performance was three percentage points better than Lampard. |
A novel way to soccer match prediction (Shin & Gasparyan, 2014) | Presents a novel approach to soccer match prediction using only virtual data collected from a video game (FIFA 2015). Results were comparable and in some places better than results achieved by predictors that used real data. |
Ball recovery patterns as a performance indicator in elite soccer (Barriera et al., 2014) | This study presents that the type and the zone of ball recovering seem to affect attacking efficacy in elite soccer. Found that recovering directly the ball possession in mid-defensive central zones increases attacking efficacy |
How important is it to score a goal? The influence of the scoreline on match performance in elite soccer (Lago-Peñas & Gómez-López, 2014) | Concluded that players explored more extensively when they were in possession, and less extensively during transition phases. Further, players explored most extensively when in the back third of the pitch, and least when in the middle third of the pitch. |
Evaluation of research using computerised tracking systems (Amisco and Prozone) to analyze physical performance in elite soccer: A systematic review (Castellano et al., 2014) | Concludes that computerised video tracking systems are a valuable data collection tool to enable sports scientists to identify player physical demands, allowing personalised training and testing protocols. New global and local positioning system technology will allow further advances in tracking systems. |
Football Player’s Performance and Market Value (He et al., 2015) | Creation of La Liga individual player financial value using regression techniques with inputs player performance data and recent transfer price. Results were biased towards forwards and good players. |
Why Soccer’s Most Popular Advanced Stat Kind Of Sucks (Bertin, 2015) | Provides analyses of the Expected Goals statistic being presented in football analytics, casting doubt about its validity and usefulness. Examples of flaws in the underlying data and the calculation methods are given. |
Visual analysis of pressure in football (Andrienko et al., 2017) | Propose a computational approach to detecting and quantifying the relationships of pressure (exerted by defenders on the ball and opponents) emerging during a match. The extracted pressure relationships are then analysable through the use of static and dynamic visualisations and interactive query tools. |
Developing a Data-Driven Player Ranking in Soccer Using Predictive Model Weights (Brooks et al., 2016) | Describes a novel player ranking system based entirely on the value of passes completed (based on the relationship of pass locations in a possession and shot opportunities generated). Player rankings were largely consistent with general perceptions of offensive ability, e.g., Messi and Ronaldo are near the top. When used to rank midfielders, more offensively-minded players were identified. |
Periodization Training Focused on Technical Tactical Ability in Young Soccer Players (Aquino et al., 2016) | Over a period of 22 weeks, concluded that there was reduced activity in biochemical markers related to muscle damage, as well as increases in game high-intensity performance and the tactical performance of study participants. Furthermore, players who showed greater reduction in plasma activity of creatine kinase and lactate dehydrogenase also obtained greater increases in-game high-intensity performance along the periodization. |
The micro-macro link in understanding sport tactical behaviours: Integrating information and action at different levels of system analysis in sport (Araújo et al., 2015) | Discusses the link between individual decision-making (micro) vs team decision-making (macro) behaviours, using phase transitions as the explanatory mechanism, providing a common language for understanding order-order transitions in behaviours. Concludes that where sport performance is emergent under the influence of many interacting constraints, rather than reducing performance variability, learning designs should attempt to increase functional variability in practice conditions. |
Age-related effects of practice experience on collective behaviours of football players in small-sided games (Barnabé et al., 2016) | Findings suggested that the age-related experience of football players tend to influence their collective behaviours in offensive and defensive phases. The likely mechanisms for these age-related differences are differences in maturation and development (e.g., physical and psychological capacities), as well as greater levels of experience and learning. |
Discovering Team Structures in Soccer from Spatiotemporal Data (Bialkowski et al., 2016) | Describes a completely unsupervised system to learn and identify spatial structure of a team directly from data, giving an indication of dominance and tactics. The formation descriptor was shown to represent the characteristic style of teams significantly better (3 times more) than other match descriptors typically used to describe team behaviour. |
Real time quantification of dangerousity in football using spatiotemporal tracking data (Link et al., 2016) | Presents a procedure for determining dangerousity in football in real-time using an optical tracking system. Results indicate that the performance and dominance metrics derived are more robust in the context of the effects of chance, and map the match performance of a team more reliably than the traditional performance indicators of possession of the ball, shots on goal, tackle, and pass rates. |
Coordination analysis of players’ distribution in football using cross-correlation and vector coding techniques (Moura et al., 2016) | Study using a video-based tracking system investigating how players change their distribution across the pitch for attacking and defending purposes. Trajectories of 257 players over 10 matches suggest that team organisation during matches can induce the behaviour of the opponent. |
Big data and tactical analysis in elite soccer: Future challenges and opportunities for sports science (Rein & Memmert, 2016) | Discusses handling very large player and match datasets created from game logs, player tracking systems and training ground data collection with modern machine learning technologies to analyse tactics. Concludes that performance analysts, exercise scientists, biomechanics as well as practitioners will have to work together to make sense of these complex data sets. |
Visual exploration of match performance based on football movement data using the continuous triangular model (Zhang et al., 2016) | Exploration of footballer match performance utilising the Continuous Triangular Model, based on sports-oriented movement data. The motion attributes used are speed, ball possession and territorial advantage, combined to calculate a dominance index. |
The Pressing Game: Optimal Defensive Disruption in Soccer (Bojinov & Bornn, 2016) | Creates a team-specific cartography that maps out strengths and weaknesses of a team’s attack and defence to explore a team’s disruptive ability. Describes how this information can be used to understand the tactics employed by managers across different teams. |
Can Artificial Intelligence Modelling Approaches Assist Football Clubs In Identifying Transfer Targets, While Maintaining A Fair Transfer Market Using Player Performance Data? (Ahmed, 2016) | Evaluation of Artificial Intelligence approaches to identify football transfer targets. Concluded on a Case-Based Reasoning Expert System approach with a k- Nearest neighbour algorithm. |
When do soccer players peak? (Dendir, 2016) | Results showed that the average professional footballer peaks between the ages of 25 and 27, the average forward peaks at 25, the typical defender peaks at 27 and midfielders between 25–27. Results also indicated that peak age may vary directly with ability. |
Modelling the financial contribution of soccer players to their clubs (Sæbø & Hvattum, 2019) | Presents a framework consisting of three methods: evaluate the quality of each player; translate the quality of players in the starting line-ups to probabilities for match outcomes; simulate the relevant soccer competitions with the help of calculated match outcome probabilities. Monte Carlo simulation is used to predict the final league standings and the financial gains obtained as a function of sporting success. Results were validated using the 2014-2015 English Premier League season. |
Towards data-driven football player assessment (Stanojevic & Gyarmati, 2016) | Describes the drawbacks of human-based scouting including high cost, inability to scale and inevitable subjective biases and presents a statistical methodology for data-driven player market value estimation as a stronger predictor. |
Quantifying the relation between performance and success in soccer (Pappalardo & Cintia, 2018) | Findings that a team’s position in a competition’s final ranking is significantly related to its typical performance, and that, while victory and defeats can be explained by the team’s performance during a game, it is difficult to detect draws by using a machine learning approach. |
Beyond completion rate: Evaluating the passing ability of footballers (Szczepański & McHale, 2016) | Presents a statistical model where passing success depends on the skill of the executing player as well as other factors including the origin and destination of the pass, the skill of teammates and opponents, and proxies for the defensive pressure put on the executing player as well as random chance. Resulting predictions considerably outperform a naive method of simply using the previous season’s completion rate as a predictor of the following season’s completion rate. |
Bring it to the Pitch: Combining Video and Movement Data to Enhance Team Sport Analysis | Proposes a visual analytics system integrating team sport video recordings with abstract visualization of underlying trajectory data. Applies computer vision techniques to extract trajectory data from video input. Applies advanced trajectory and movement analysis techniques to derive relevant team sport analytic measures for region, event and player analysis. |
A study of relationships among technical, tactical, physical parameters and final outcomes in elite soccer matches as analyzed by a semiautomatic video tracking system (Goldlücke & Keim, 2017) | Analysis of mean physical (physical efficiency index; PEI) and technical–tactical (technical efficiency index; TEI) performance of 360 players in 70 Italian Serie A matches. Findings that technical performance appears to be a better predictor of winning games, alongside player decision making ability. |
Not all passes are created equal (Power et al., 2017) | Presents an objective method of estimating the risk (likelihood of executing a pass in a given situation, and reward (likelihood of a pass creating a chance) of all passes using a supervised learning approach. |
The Leicester City Fairytale?”: Utilizing New Soccer Analytics Tools to Compare Performance in the 15/16 & 16/17 EPL Seasons (Ruiz et al.,2017) | Machine learning analyses concluded Leicester’s unique strategy, e.g., organised defence allowing them to reduce the quality of their opponents’ chances; their disruptive game, embodied by N’Golo Kante, which made them one of the most difficult teams to attack against; and focusing their shot production on the most dangerous strategies. |
A Bayesian inference approach for determining player abilities in soccer (Whitaker et al., 2017) | Determination of a footballer’s ability for a given event type, e.g., scoring a goal. Method applied to the English Premier League, over the 2013/2014 season, to predict whether over or under 2.5 goals will be scored in a given fixture or not in the 2014/2015 season. |
What’s in a game? A systems approach to enhancing performance analysis in football (McLean et al., 2017) | Presents results of two workshops comprising eight elite level football Subject Method Experts to develop a systems football match model. Results enabled identification of several unutilised performance analysis measures, including communication between team members, team adaptability, appropriate tempo play, and attacking and defending related measures. |
Beyond crowd judgments: Data-driven estimation of market value in association football (Müller et al., 2017) | Results across 146 teams from the top 5 European leagues and a 6 playing seasons, using multilevel regression models produced comparatively accurate estimates compared to crowdsourcing estimates. |
Pricing Football Players Using Neural Networks (Dey, 2017) | Using a multilayer perceptron neural network, modeling results achieved a top-5 accuracy of 87.2%, and places any footballer on average within 6.32% of his actual price. |
Predicting the Potential of Professional Soccer Players (Vroonen et al., 2017) | Presents a system (APROPOS) to predict footballer potential by searching a historical database to identify similar players of the same age, based upon its prediction for the target player’s progression on how the similar previous players actually evolved. |
Physics-based modelling of pass probabilities in soccer (Spearman et al., 2017) | Presents a model for ball control based on the concepts of how long it takes a player to reach and control the ball. Likelihood that a given pass will succeed is quantified and correctly predicts the receiving team with an accuracy of 81% and the specific receiving player with an accuracy of 68%. correlating strongly with league standing at the end of the season. |
State of the Art of Sports Data Visualization (Perin et al., 2018) | Detailed review of sports data visualization work, from both academics and practitioners, in particular presenting strong evidence that it all relies on three main data categories: box-score data, tracking data, and meta-data. |
Player valuation in European football (Extended version) (Nsolo et al., 2018) | Evaluates which attributes and skills best predict the success of footballers in the 5 European leagues, and positions (defenders, midfielders, forwards, and goal keepers). Results included: Prediction success was highest for forwards, followed by midfielders, then defenders, then goalkeepers; Bayes Net and Random Forest machine learning methods were the most successful. |
A weighted plus minus metric for individual soccer player performance (Schultze & Wellbrock, 2018) | Proposes a weighted plus/minus metric to evaluate player performance. Concludes soccer is years behind other sports such as baseball and basketball in terms of advanced statistical analytics. |
Quantifying the Value of Transitions in Soccer via Spatiotemporal Trajectory Clustering (Hobbs et al., 2018) | Uses player and ball tracking data to automatically identify counterattacks and counter-pressing without requiring unreliable human annotations. The “defensive disorder” of a team as they transition from offense to defence is quantified and sub-clusters of plays which were likely to produce goal-scoring opportunities through a measure of “offensive threat” identified. |
Identifying key players in soccer teams using network analysis and pass difficulty (McHale & Relton, 2018) | Presents methodology for identifying key players in a football team using the locations of all players on the pitch at a frequency of ten times per sec. Results suggest that running more than the opposition isn’t necessarily positively related to success. Key players identified statistically model to determine probability of a pass being successful. |
Player Performance Prediction in Football Game (Pariath et al., 2018) | Model presented of relationship between footballer performance and overall value with between 84.34 % and 91% accuracy. Second model predicts future market value of players on basis of the overall performance predicted by the first model. |
Singing it for “us”: Team passion displayed during national anthems is associated with subsequent success (Slater et al., 2018) | Examines link between passion of team members during singing of national anthems and team performance in the tournament. Findings that teams that sang with greater passion conceded fewer goals and that the impact of passion on the likelihood of winning a game depended on the stage of the competition. For example, in the knockout stage (but not the group stage) greater passion was associated with a greater likelihood of victory. |
Artificial neural networks and player recruitment in professional soccer (Barron et al., 2018) | Findings that using ProZone data it is possible to identify performance indicators that influence a players’ league status and accurately predict their career trajectory. Results correctly predicted between 61.5% and 78.8% of the players’ league status. |
Not every pass Can Be An Assist: A Data-Driven Model to Measure Pass Effectiveness in Professional Football (Goes et al., 2019) | Presents a new approach to quantify pass effectiveness by means of live tracking data. The measures quantify the effectiveness of a pass in terms of how well it disrupts the opposing defence, allowing differentiation between effective and less effective passes, as well as between the effective and less effective players. |
Pitch actions that distinguish high scoring teams: Findings from five European football leagues in 2015-16 (Sarkat & Chakraborty, 2018) | Presents model estimating the number of non-penalty goals per game with error of less than 0.33 for 93 teams out of 98, and less than 0.1 for 52 teams the margin of error was less than 0.1. Shots from penalty box per game, share of shots from goal box in total shots and long pass accuracy have statistically significant positive impact on non-penalty goals scored per game. Share of long passes in total passes and crosses per game have significant negative impact. |
Evaluating Passing Behaviour in Association Football (Håland & Wiig, 2018) | The developed pass effectiveness model drew attention to the value of counter attacking, indicating that teams can benefit from putting low pressure on opponents and looking for counter-attack opportunities. Also indicating the importance of pass type selection based upon ground/pitch type. |
Goal scoring in elite male football A systematic review (Pratas et al., 2018) | Review of available literature on goal scoring in elite male football leagues. Concludes significant performance indicators (that is goal difference, shots on goal, disciplinary sanctions and substitutions) associated with goal scoring are match dependent. |
PlayeRank: Data-driven Performance Evaluation and Player Ranking in Soccer via a Machine Learning Approach (Pappalardo et al., 2019) | Presents framework to evaluate footballer performance, outperforming existing approaches in being significantly more aligned with professional scouts. Results showed excellent performances are rare and unevenly distributed, since a few top players produce most of the observed excellent performances. Also, top players do not always play excellently, they just achieve excellent performances more frequently than others. |
The Application of Machine Learning Techniques for Predicting Results in Team Sport: A Review (Bunker & Susnjak, 2019) | Systematic review of studies between 1996 and 2019 that have used ML for predicting results in team sport. Findings suggest that a wide set of candidate algorithms and ensembles should be used, and applied to different subsets of features to compare their performance against full feature supersets. |
Sports Analytics Algorithms for Performance Prediction (Apostolou & Tjortjis, 2019) | Analysis of English Premier League, Italian Serie A, Spanish La Liga and French Ligue 1, to classify teams that would perform better (more points) or worse. Results using machine learning techniques achieved 70% accuracy. Defining which attributes and match actions are mainly influencing a central defender’s match rating also gave statistically significant positive results. |
Team spirit in football: an analysis of players’ symbolic communication in a match between Argentina and Iceland at the men’s 2018 World Cup (Halldorssom, 2019). | Uses micro-sociological theory and perspective to account for players' use of symbolic communication and gestures in regard to team spirit. The framework suggested that a key factor in Iceland’s better result was that their team consisted of more productive and emergent team spirit during the match than Argentina, exemplified in their players' shared use of positive on-the-field symbolic gestures and communication providing the players with support and encouragement and creating recurrent momentum. |
A study of Prediction models for football player valuations by quantifying statistical and economic attributes for the global transfer market (Patnaik et al., 2019) | Concludes in addition to player performance, transfer pricing depends upon contract length, popularity, job mobility, amount of games played and goal scoring opportunities. Top clubs generally pay more than market estimate for attracting top talent; whereas, a club lacking a player in a particular position may pay more, to fill the void. |
Machine learning in men’s professional football: Current applications and future directions for improving attacking play (Herold et al., 2019) | Provides critical appraisal of the application of machine learning related to attacking play, discussing current challenges and future directions that may provide deeper insight. Concludes that machine learning techniques require improvement, but the representation of knowledge in a way that can be understood and utilised in practice is essential. This implies use of multi-disciplinary approaches including computer science research groups and football experts to interpret the data. |
Actions speak louder than goals: Valuing player actions in soccer (Decroos et al., 2019) | Presents a language for representing event stream data with the goal of facilitating data analysis and a framework for assigning a value to each footballer action during a match. Action types (e.g., passes, crosses, dribbles, and shots) are valued on game context, and reasons about an action’s possible effects on subsequent actions. Concludes that by aggregating soccer players’ action values, their offensive and defensive contributions to their team can be quantified. |
A public data set of spatio-temporal match events in soccer competitions (Pappalardo et al., 2019) | Describes largest available open collection of soccer-logs, containing all the spatio-temporal events (passes, shots, fouls, etc.) that occurred during each match for an entire season of seven prominent soccer competitions (La Liga, Serie A, Bundesliga, Premier League, Ligue 1, FIFA World Cup 2018, UEFA Euro Cup 2016). |
Valuing On-the-Ball Actions in Soccer: A Critical Comparison of xT and VAEP (Decroos & Davis, 2020). | Identifies limitations of footballer contributions by measuring the quality of shots and assists only, which represent less than 1% of all on-the-ball actions. Presents the comparison of two footballer match contribution models: expected threat; and valuing actions by estimating probabilities. |
Sports Analytics for Football League Table and Player Performance Prediction (Pantzalis & Tjortjis, 2020) | Analysis of English Premier League, Italian Serie A, Spanish La Liga and French Ligue 1, to classify teams that would perform better (more points) or worse. Results using machine learning techniques achieved 70% accuracy. Defining which attributes and match actions are mainly influencing a central defender’s match rating also gave statistically significant positive results. |
Choke or Shine? Quantifying Soccer Players’ Abilities to Perform Under Mental Pressure (Bransen et al., 2019) | Considers how to objectively understand how high-mental pressure situations affect performances of soccer players. Illustrates concrete use cases about how it could inform acquiring players, coaching individual players, making tactical decisions, and deciding on line-ups or substitutions. |
Maximizing performance with an eye on the finances a chance-constrained model for football transfer market decisions (Pantuso & Hvattum, 2020) | The model seeks a top-performing team while adapting to different budgets and financial risk profiles. A new rating system that is able to numerically reflect the on-field performance of football players and thus contribute to an objective assessment of football players is presented Then tested on a case study based on real market data and results illustrate that the model mimics the reasoning of a club’s decision maker when dealing with transfers of professional players. |
The Data Gap in Sports Analytics and How to Close It (Harell & Bajic, 2019) | Discusses the significant gap in data availability that exists in the sports analytics community - between sports, leagues (especially between pros and amateurs), genders and between private and public data. Describes the consequential people-related and model-related negative impacts and how they may be mitigated. |
The open international soccer database for machine learning (Dubitzky et al., 2019) | Presents the development of the Open International Soccer Database (216,743 league matches, 52 leagues in 35 countries) and the results of the nine submissions to the 2017 Soccer Prediction Challenge on the use of machine learning to predict match outcomes. |
Methodological Issues in Soccer Talent Identification Research (Bergkamp et al., 2019) | Identifies four methodological issues relevant for talent identification research: Operationalization of criterion variables (the performance to be predicted) as performance levels; Focus on isolated performance indicators as predictors of soccer performance; Effects of range restriction on the predictive validity of predictors used in talent identification; Effect of base rate on the utility of talent identification procedures. |
Automated Machine Learning A Game Changer for Sports Analytics Executive Briefing v1.0 | Describes how the DataRobot automated machine learning platform makes advanced predictive analytics more accessible to sports organizations by reducing barriers to accurate predictions. |
Evaluating Passing Ability in Association Football Goal scoring in elite male football A systematic review (Håland et al., 2020) | Determination of footballer passing ability in terms of difficulty, risk and potential. provide insight into the factors affecting the success of a pass including location of the pass, relationship to previous passes and to situations such as throw-ins, corners, free kicks, or tackles, as well as conditions such as the time of season and the ground surface type. |
Unlocking the potential of big data to support tactical performance analysis in professional soccer A systematic review (Goes et al., 2020) | Systematic literature search for studies employing football position tracking data to study tactical behaviour (2338 studies and 73 papers). Presents a multidisciplinary framework where each domain’s contributions to feature construction, modelling and interpretation can be situated. |
Exploring elite soccer teams’ performances during different match-status periods of close matches’ comebacks (Gomez et al., 2020) | Found statistically significant differences between winning and losing teams in Period 3 for ball possession and passing effectiveness. Also, significant differences for winning teams in ball possession with period 4 compared with other periods. Also, winning teams showed significant differences in passing effectiveness (period 4 vs 3), and in shots (period 3 vs periods 1, 2 and 4). Ball possession showed significant differences for losing teams with periods 3 and 4 compared to periods 1 and 2. |
Identifying playing talent in professional football using artificial neural networks (Barron et al., 2020) | Presents the results of using an artificial neural network to create fifteen position-specific models to predict out-field player’s league status, with over 75% results accuracy of the player’s league status for fourteen different position comparisons. |
A Systematic Literature Review of Intelligent Data Analysis Methods for Smart Sport Training (Rajšp & Fister, 2020) | Systematic literature review of smart sport training, presenting intelligent data analysis methods. Computational intelligence algorithms have risen in popularity in recent years, while the most used intelligent data analysis methods remain support vector machine, artificial neural networks, k-nearest neighbours, and random forest. |
On the relationship between+/–ratings and event-level performance statistics (Gelade & Hvattum, 2020) | Identification and assessment of contribution of a footballer towards performance of the team. Uses advanced plus-minus ratings for individual players. Findings include marginal improvements in the prediction of match results can be achieved by combining information from player top-down and bottom-up ratings. |
Constraints on visual exploration of youth football players during 11v11 match play: The influence of playing role pitch position and phase of play (McGuckian et al., 2020) | Study investigating how a player’s on-pitch position, playing role and phase of play influenced their visual exploratory head movements. Findings that players explored more extensively when they were in possession, and less extensively during transition phases. Further, players explored most extensively when in the back third of the pitch, and least when in the middle third of the pitch. |
Behavioral, physiological, and neural signatures of surprise during naturalistic sports viewing (Anthony et al., 2020) | Using automatic formation analysis, presents that teams tend to play the same formation at home as away, but with modified execution. In particular, that home team formation is significantly higher up the field compared to away. Concludes that coaches taking a conservative approach at away games suggests that they aim to win home games and draw away games. |
Theory to Practice Performance Preparation Models in Contemporary High-Level Sport Guided by an Ecological Dynamics Framework (Woods et al., 2020) | Describes how high-level organisations have attempted to integrate ecological dynamics (views movement as emerging from a self-organising relationship formed between an individual, the task being performed, and the environment in which it occurs for performance preparation). Describes two case examples of high-level sports organisations utilising ecological dynamics for performance preparation in each of Australian football and Association Football. |
A Narrative Review in Sport Analytics (Singh, 2020) | Sports analytics literature review, including analysis of crowd opinions on social media, player performance indicators, match strategy variations, and trends in the betting market. Objective of applying data analytics to player bidding, fan base marketing, sport promotion, consumer sentiment analysis, player performance, sport injury, hosting games and events such as the Olympics. |
Applications of Artificial Intelligence in the Game of Football The Global Perspective (Rathi et al., 2020) | Study on applications of AI in football and it’s limitations. Findings are that with the help of AI and other technologies, teams are able to discover new potential and achieve goals which were thought to be impossible before, especially in enhancing team competitiveness, decision making and better customer experience. The technology is still immature and needs significant improvement. |
Comparison of the football specific tactical performance of women and men in Europe (Mammert et al., 2020) | Comparison of the tactical behaviour of women’s and men’s teams No differences in football specific tactical performance between women and men identified. Specifically, analysis of event-based KPIs (number of passes, dribbles etc.,) showed that individual tactical events in women’s and men’s games occur with similar frequency. Women and men have comparable pass quality and switching behaviour after ball loss. Only video-based analysis of team tactical KPIs (Counter attacking “play and go” etc.) revealed isolated differences between women’s and men’s football. This underlines the importance of objective analysis methods to avoid subjective (gender) bias. |
Development of Defence and Offence Play Items for Deep Learning Model of Offence Play Analysis in Soccer Game (Matsuoka et al., 2020) | Study developing offence and defence tactical play items from ball touch and tracking data for analysis using deep learning. Concluding that such tracking data may be used as features for deep learning tactical play analysis. |
Analysis System for Emotional Behaviour in Football Professional football players emotional behaviour in ghost games in the Austrian Bundesliga (Leitner & Richlan, 2020) | Findings that during Covid the absence of supporters has a substantial influence on the experience and behaviour of players, staff and officials alike. |
An Analysis on the Effectiveness of Cooperation in A Soccer Team (Ge et al.,2020) | Measurement of the effectiveness of teamwork to provide advice to coaches. Establishment of a passing network through a season, to find core players and closely matched player combinations. Results allowed us to measure effectiveness of teamwork in order to provide advice to coaches. |
Where do the best technical football players in the world come from Analysing the association between technical proficiency and geographical origin in elite football (Jamil, 2020) | Compares the performance of South American, African, European, Asian and North American footballers. Concludes that a footballer’s geographical origin can impact their technical proficiency. For example, South American players were significantly better at scoring the first goal, scoring penalties and attempting shots than their European counterparts. European and South American players were more adept at passing than African, Asian or North American players. |
Visualizing and Analyzing Disputed Areas in Soccer (Allegre & Vuillemot, 2020) | Presents a process to visualise and analyse disputed areas (cases where two or more footballers can reach a given location simultaneously) providing insights to understand assists and the ultimate pass that is critical for a team to score. |
A Data Science Approach to Football Team Player Selection (Rajesh et al., 2020) | Considers the cost effective selection of players based upon player skills, performance, positions, ratings, market value and costs. Presents results showing that it leads to improved business profits through a systematic enhancement to football data sets. |
A case study assessing possession regain patterns in English Premier League Football (2019 | Concludes that ball recovery patterns likely vary between teams and leagues due to factors such as the manager’s philosophy and coaching ability, strategies and tactics employed by each team and team skill and quality level. Also, the number of successful ball recoveries in the opponent’s half had a significant positive impact upon attacking performance. Opponent quality has an impact on the number of recoveries completed. |
A new paradigm to understand success in professional football: analysis of match statistics in LaLiga for 8 complete seasons (2019) | Concludes shooting accuracy while attacking along with the avoidance of clear shots from the opposing team are the indicators most associated with points tally. Although number of passes and passing accuracy had a statistically significant association to points-total, their contribution to the variance of the number of obtained points at the end of the season was minor. Intensity of defensive actions in zones where the opposing team might be inclined to shoot should be the focus of the defensive team. These outcomes are as useful to teams avoiding relegation as to higher ranked teams. |
Are winners different from losers? Performance and chance in the FIFA World Cup Germany 2006 (2006) | Concludes that performance relevant to points obtained in World Cup Germany group stages, with increasing impact as more games are played. While there are statistically significant differences in performance in round one this wasn’t the case in round two, where chance was more important. |
Association between playing tactics and creating scoring opportunities in counterattacks from United States Major League Soccer games (2016) | Findings that counterattacks starting in pre-offensive zones were more effective in creating scoring opportunities than those starting in defensive zones, and those without initial penetration, only when the defensive team did not exert initial defensive pressure. Counterattacks with four or more passes were more effective than shorter ones, regardless of the initial defensive pressure. In defending, not exerting initial defensive pressure after losing ball possession increased the probability of conceding counterattacking scoring opportunities threefold. The effectiveness in counterattacks were associated with regaining ball possession in offensive zones, performing initial penetration, making four or more passes and playing against no initial defensive pressure. |
At what age are English Premier League players at their most productive A case study investigating the peak performance years of elite professional footballers (2020) | Concludes that forwards and wingers reach their peak performance age prior to the age of 25. However, contrary to previous studies, evidence was discovered confirming that age has no bearing upon the technical performances of goalkeepers, defenders or midfielders. |
Chinese soccer association super league, 2012–2017: key performance indicators in balance games (2018) | Concluded that winning teams had increased shots, shots on target, 50–50 challenges won, offsides, sprinting distance, sprinting effort, sprinting distance in ball possession and high-speed-running distance in ball possession. Losing teams had significantly higher averages in the variable crosses, passes, forward passes, sprinting distance out of ball possession and high-speed-running distance out of ball possession. The variables that discriminate between winning, drawing and losing teams were shots on target, sprinting distance in ball possession, quality of opposition, passes and forward passes. |
Identifying keys to win in the Chinese professional soccer league (2016) | Findings were that Shot on Target (positive), Shot Accuracy (positive), Cross Accuracy (trivial), Tackle (trivial) and Yellow Card (trivial) were the five variables that showed consistent effects matches. |
Inter-operator reliability of live football match statistics from OPTA Sportsdata (2013) | Results suggest the OPTA Client System is reliable to be used to collect live football match statistics by well-trained operators. Team events coded by independent operators reached a very good agreement. The reliability of goalkeeper actions and outfield players were also at high level. |
Investigating the impact of the mid-season winter break on technical performance levels across European football –Does a break in play affect team momentum? (2020) | Concludes that a mid-season winter break of less than 13 days will not affect technical performance levels but breaks that last longer can halt momentum and cause performances to deteriorate. Shooting performance declined significantly post winter break in the German Bundesliga which had an average break of 32 days. Passing performance deteriorated significantly in the French Ligue 1 which had an average break of 19 days. The Spanish La Liga had a 13-day break on average and remained unaffected as did the English Premier League which had no mid-season break. |
Performance profiles of football teams in the UEFA champions league considering situational efficiency (2015) | Results suggest that scouting upcoming opposition should be done under circumstances that are reflective of the conditions under which the future match will occur. Time and opportunity constraints prevent this, so establishing appropriate profiles was a potential solution. Similarly, post-match assessments of performance on the own team can be made more objectively and directly by profiling performance-related match variables in effects of situational variables. Variation of teams’ performance associated with specific situational variables could be identified by the profiles, hence, possible causes can be examined and match preparation focusing on reducing such effects can be made. |
Technical demands across playing positions of the Asian Cup in male football (2019) | Concluded that wide midfielders scored more goals than fullbacks, and that full backs had less goal attempts than central midfielders, wide midfielders and forwards, whereas central defenders had less attempts than forwards. Central midfielders passed more than central defenders, wide midfielders and forwards, while forwards passed less than central defenders, full backs and central midfielders. Central defenders and central midfielders and CM passed more successfully than full backs and forwards, and central midfielders also had more passes than wide midfielders. Moreover, forwards had more aerial duels than central midfielders, full backs and wide midfielders. Similar numbers of aerial duels occurred for central defenders and forwards. Ground duels occurred less frequently for central defenders compared to full backs, midfielders and forwards. |
Success factors in football: an analysis of the German Bundesliga (2020) | Results showed that defensive errors, market value, goal efficiency, shots from counter attacks, shots on target and total shots have the greatest impact on team success in the German Bundesliga. Crosses showed a negative relationship with success. Opponent and home advantage are important contextual effects. Overall, 11 and 12 variables are significant, respectively. Duel success is only significant for away teams and a higher market value seems to have a more positive impact for them. |
Technical demands of different playing positions in the UEFA Champions League (2018) | Identification of the technical demands of different playing positions in the UEFA Champions League. Results showed the differences between central defenders and forwards were biggest while central defenders and full backs the least. Midfielder performance in variables related to passing and organising were worse than expected and wide midfielders showed relative better performances than central midfielders in passing and organising. Defenders, especially, central defenders, achieved good performance in variables related to passing and organising. Forwards played an important role in aspects of goal scoring and organising, as well as the initial defending process. |
The creation of goal scoring opportunities in professional soccer Tactical differences between Spanish La Liga English Premier League German Bundesliga and Italian Serie A (2019) | Comparison of how goal scoring opportunities emerge in the top four European soccer leagues in 2017/18. Spanish La Liga showed a greater proportion of long and combinative attacks. English Premier League had a higher tendency of progressing by means of fast and directs attacks. German Bundesliga had the greatest number of counterattacks, and Italian Serie A had the shortest offensive sequences and more proportion of counter-attacks and direct attacks than combinative and fast attacks. |
A comprehensive list of attributes was then compiled from all papers selected, resulting in 2537 attributes used in total across all selected papers, including duplicates. Analyses were made to establish the frequency and predominance of types of individual attributes addressed in the papers selected.
Where these papers exploited footballer attributes extracted from available football datasets such as SoFIFA (SoFIFA, 2020), Stats Perform (Stats Perform, 2020) etc., this was noted in order to develop a full list of available datasets (Table 1). In most cases these are freely available: however, where not the case this is noted.
Table 1
Sources of player data
Name | No. of attributes 1 | Freely Available (Y/N) | Notes |
SoFIFA (SoFIFA, 2020) | 98 | Y | TheSoFIFA.com website provides the player ratings included in FIFA video games since 2007. |
Open International Soccer Database (Dubitzkyet al., 2017) | 37 | Y | Sourced from SoFifa, containing the outcomes of > 200,000 international soccer matches. |
WhoScored.com (WhoScored, 2020) | 65 | Y | Includes data from 500 tournaments, 15,000 teams, and 250,000 players, from top 5 leagues in Europe and more using Opta. |
European Soccer Database (Mathien, 2016) | 37 | Y | Includes data from +25,000 matches, +10,000 players, 11 European Countries with their lead championship Seasons 2008 to 2016 and SoFIFA data. |
Football Database EU (2020) | 65 | Y | Data on +417,000 players across all nations presenting all transfer news in tabular form. |
StatsBomb (2020) | 19 | N | Data analytics organisation providing football data and analytics. |
WyScout (2020) | 9 | N | Includes data from all nations, from +470,00 players across +43,000 teams. Now part of Kudl.. |
EA Sports Player Performance Index (PA Sport, 2020) | 17 | N | Includes global data from 250+ football competitions. |
Opta Index (Stats Perform, 2020) | 30 | N | Now part of Stats Perform. Includes data from a variety of football leagues. |
Stats Perform (Stats Perform, 2020) | 30 | N | Data analytics organisation providing football data and analytics. Acquired ProZone and Opta Index. |
Amisco (Stats Perform, 2020) | 30 | N | Acquired by ProZone, now part of Stats Perform. |
StatDNA (2020) | – | N | Video analysis services. Information requested. |
Sportec Solutions (2020) | 16 | N | Data collection and analysis organization. Accesses the Bundesliga database. |
Bundesliga database (2020) | – | Y | Contains 10 seasons of German Bundesliga including match (not player) data extracted from Football-data.co.uk. |
Gracenote Sports Data (2020) | 11 | N | Contains statistics of historical results, squad information and detailed player data. |
1Number of player attributes extracted from selected papers.
These datasets assign values to the selected attributes and often apply their own formulae to create an overall score for each player as a measure of their rank compared to other players. For example, the SoFIFA dataset comprises 80 attributes for each of 18,944 international players. The SoFIFA overall score is calculated as the sum of each attribute value multiplied by a coefficient specific to the position of the individual player, added to a value representing the player’s international reputation (SoFIFA, 2020). As an example, the SoFIFA attributes, including calculated overall value are shown in Table 13, which lists the actual attribute values for each of Robert Lewandowski and Kevin DeBruyne. This table illuminates the diversity of the player attributes collected ranging from age, weight, height and other demographic data to measures of technical skills such as shooting and passing as well as mentality measures.
Table 13
SoFIFA player attributes illustrated by Robert Lewandowski and Kevin De Bruyne values
SoFIFA Player Attribute | Robert Lewandowski | Kevin De Bruyne |
sofifa_id | 188545 | 192985 |
player_url | https://sofifa.com/player/188545/robert-lewandowski/210002 | https://sofifa.com/player/192985/kevin-de-bruyne/210002 |
short_name | R. Lewandowski | K. De Bruyne |
long_name | Robert Lewandowski | Kevin De Bruyne |
age | 31 | 29 |
dob | 1988-08-21 | 1991-06-28 |
height_cm | 184 | 181 |
weight_kg | 80 | 70 |
nationality | Poland | Belgium |
club_name | FC Bayern München | Manchester City |
league_name | German 1. Bundesliga | English Premier League |
league_rank | 1 | 1 |
overall | 91 | 91 |
potential | 91 | 91 |
value_eur | 80000000 | 87000000 |
wage_eur | 240000 | 370000 |
player_positions | ST | CAM, CM |
preferred_foot | Right | Right |
international_reputation | 4 | 4 |
weak_foot | 4 | 5 |
skill_moves | 4 | 4 |
work_rate | High/Medium | High/High |
body_type | PLAYER_BODY_TYPE_276 | PLAYER_BODY_TYPE_321 |
real_face | Yes | Yes |
release_clause_eur | 132000000 | 161000000 |
player_tags | #Distance Shooter, #Clinical Finisher | #Dribbler, #Playmaker, #Engine, #Distance Shooter, #Crosser, #Complete Midfielder |
team_position | ST | RCM |
team_jersey_number | 9 | 17 |
loaned_from | ||
joined | 2014-07-01 | 2015-08-30 |
contract_valid_until | 2023 | 2023 |
nation_position | RCM | |
nation_jersey_number | 7 | |
pace | 78 | 76 |
shooting | 91 | 86 |
passing | 78 | 93 |
dribbling | 85 | 88 |
defending | 43 | 64 |
physic | 82 | 78 |
gk_diving | ||
Robert Lewandowski | Kevin De Bruyne | |
gk_handling | ||
gk_kicking | ||
gk_reflexes | ||
gk_speed | ||
gk_positioning | ||
player_traits | Solid Player, Finesse Shot, Outside Foot Shot, Chip Shot (AI) | Injury Prone, Leadership, Early Crosser, Long Passer (AI), Long Shot Taker (AI), Playmaker (AI), Outside Foot Shot |
attacking_crossing | 71 | 94 |
attacking_finishing | 94 | 82 |
attacking_heading_accuracy | 85 | 55 |
attacking_short_passing | 84 | 94 |
attacking_volleys | 89 | 82 |
skill_dribbling | 85 | 88 |
skill_curve | 79 | 85 |
skill_fk_accuracy | 85 | 83 |
skill_long_passing | 70 | 93 |
skill_ball_control | 88 | 92 |
movement_acceleration | 77 | 77 |
movement_sprint_speed | 78 | 76 |
movement_agility | 77 | 78 |
movement_reactions | 93 | 91 |
movement_balance | 82 | 76 |
power_shot_power | 89 | 91 |
power_jumping | 84 | 63 |
power_stamina | 76 | 89 |
power_strength | 86 | 74 |
power_long_shots | 85 | 91 |
mentality_aggression | 81 | 76 |
mentality_interceptions | 49 | 66 |
mentality_positioning | 94 | 88 |
mentality_vision | 79 | 94 |
mentality_penalties | 88 | 84 |
mentality_composure | 88 | 91 |
defending_marking | ||
defending_standing_tackle | 42 | 65 |
defending_sliding_tackle | 19 | 53 |
goalkeeping_diving | 15 | 15 |
goalkeeping_handling | 6 | 13 |
goalkeeping_kicking | 12 | 5 |
goalkeeping_positioning | 8 | 10 |
goalkeeping_reflexes | 10 | 13 |
ls | 89 + 2 | 83 + 3 |
st | 89 + 2 | 83 + 3 |
Robert Lewandowski | Kevin De Bruyne | |
rs | 89 + 2 | 83 + 3 |
lw | 85 + 0 | 88 + 0 |
lf | 87 + 0 | 88 + 0 |
cf | 87 + 0 | 88 + 0 |
rf | 87 + 0 | 88 + 0 |
rw | 85 + 0 | 88 + 0 |
lam | 85 + 3 | 89 + 2 |
cam | 85 + 3 | 89 + 2 |
ram | 85 + 3 | 89 + 2 |
lm | 83 + 3 | 89 + 2 |
lcm | 79 + 3 | 89 + 2 |
cm | 79 + 3 | 89 + 2 |
rcm | 79 + 3 | 89 + 2 |
rm | 83 + 3 | 89 + 2 |
lwb | 64 + 3 | 79 + 3 |
ldm | 65 + 3 | 80 + 3 |
cdm | 65 + 3 | 80 + 3 |
rdm | 65 + 3 | 80 + 3 |
rwb | 64 + 3 | 79 + 3 |
lb | 61 + 3 | 75 + 3 |
lcb | 60 + 3 | 69 + 3 |
cb | 60 + 3 | 69 + 3 |
rcb | 60 + 3 | 69 + 3 |
rb | 61 + 3 | 75 + 3 |
2.2Data classification
Each attribute was classified by data type (Wakelam et al., 2016), integrity, temporality, accessibility and sensitivity (Table 2).
Table 2
Data classifications
Identifier | Description |
Data type2 | Num = Numeric (measurable/ quantitative data is defined as being in the form of counts or numbers where each data-set has a unique numerical value associated with it. E.g. Number of assists). |
Ord = Ordinal (nominal data in which order is important. E.g., Player total contribution (low, average, high). | |
Nom = Nominal (data where the values are labels where no order may be attributed, such as male/female or yes/no. E.g. preferred foot (Right, Left). | |
Data integrity3 | O = Objective (Unambiguously measureable data. E.g, number of assists or length of pass). |
S = Subjective (Data based upon expert opinion. E.g., player composure or contribution to team spirit). | |
M = Mixed (Data typically based upon subjective judgement, however experimental research has proposed techniques for mechanistic calculations to assign a measured value based upon related measurable data. For example, player influence). | |
Temporality | S = Static (Data which does not change. For example, date of birth or preferred foot). |
E = Evolving Static (Data which would be considered static for analysis during a match, however, has the potential for change through coaching/practice, e.g. skills or strength. Or data which naturally changes over time, for example age and contract expiration date. | |
D = Dynamic (Data which changes during the course of a match. E.g. number of shots or passing accuracy). | |
Accessibility | Y = Yes (The data is collectible independently of the player’s direct input. For example, age or number of yellow cards)4. |
N = No (The data ideally obtainable by direct interaction with the player themselves. E.g. cognitive abilities, measurable through psychometric testing or creativity)3 | |
Sensitivity5 | R = Readily and publicly available data having no privacy or ethical issues with their collection or use in analyses. For example, nationality, age. |
S = Sensitive personal data which would either require the player’s permission for collection and would not be made publicly available, and/or would require specific ethical approval for its use in analytics in addition to being subject to strict limitations on its availability. E.g. life events or family support. | |
P = Potentially sensitive data which a player or club would be required to follow pre-agreed data collection and usage rules and only with explicit player permission. E.g. socio-economic background or cognitive ability. |
2,3Where the source paper(s) are unclear or conflicting in data type specification or data attribute, the authors have done their best to select the most appropriate. 4Alternatively, such data may be given a subjective measure by club scouts/coaches/psychologists. 5Where in any doubt in the identification of sensitivity of data items the authors have selected the more sensitive definition.
Attributes were then allocated to 25 logical groups: Player data & history; Speed & movement; Pass; Goals, shots & shooting; Tackles; Aerial & header; Possession; Fouls & cards; Dribble; Free kick; Cross; Interception; Block; Duel; Clearance; Error, mistake, fail; Ball; Ball recovery; Assist; Offside; Injury; Outfielder position specific; Goalkeeper; Data applicable to any player; Character traits. Given the very wide variety of player attributes, it is possible to select these groups in a variety of different ways, and for the purposes of this paper we have tried to align our selection to reflect some of what appear from our research to be groups of interest to clubs and researchers, whilst at the same time keeping the groups as logical as possible. For example, while Free kicks may be considered as a component of Goals, shots and shooting, free kicks tend to be taken by so called “free kick specialists” in teams and we therefore chose to allocate them to a group of their own. In the case of the Player data and history group we have included the data that describes player demographics such as age and nation origin, physical attributes such as height and BMI, statistical attributes such as games played and international caps and those attributes which attempt to define the player such as their specific skills and strengths.
Where an attribute was allocatable to more than one group this was done. For example, ball recovery by tackle is relevant to each of the Tackles and the Recoveries groups and running while in possession to both the Possession and Speed and Movement groups.
3Results
3.1Papers
The complete list of the 132 papers selected is provided in Table 12 and the main findings and conclusions of each paper are summarized in Table 14.
The papers are sourced from a wide range of publishers, in total 78. We find that each of the International Journal of Performance Analysis in Sport with 14 of the selected papers, the Journal of Sports Sciences, with 11, the MIT Sloan Sports Analytics Conference proceedings, with 8, and the Journal of Sports Analytics with 5, together, account for 29% of the total. The next highest sources are Human Movement Science (4) and Cornell University Library’s arXiv (4), although we must note that arXiv is classed as a pre-publication distribution service and open-access archive for scholarly articles and publications are not peer-reviewed. Publishers Sports Medicine, Perceptual and Motor Skills and PLOS ONE, each with 3 papers follow and the remainder are ones and twos.
An analysis of the publication dates of the 132 relevant papers compiled shows how the growth of research interest in the field of footballer analytics has accelerated between 1999 and 2020 (Fig. 1). Nineteen of the selected papers were published between 1999 and 2012, an average of less than 1.5 per year, whereas 113 of the selected papers were published in the 8 years from 2013 to 2020, an average of almost 14 papers per year.
Fig. 1
Number of relevant papers published between 1999 and 2020.
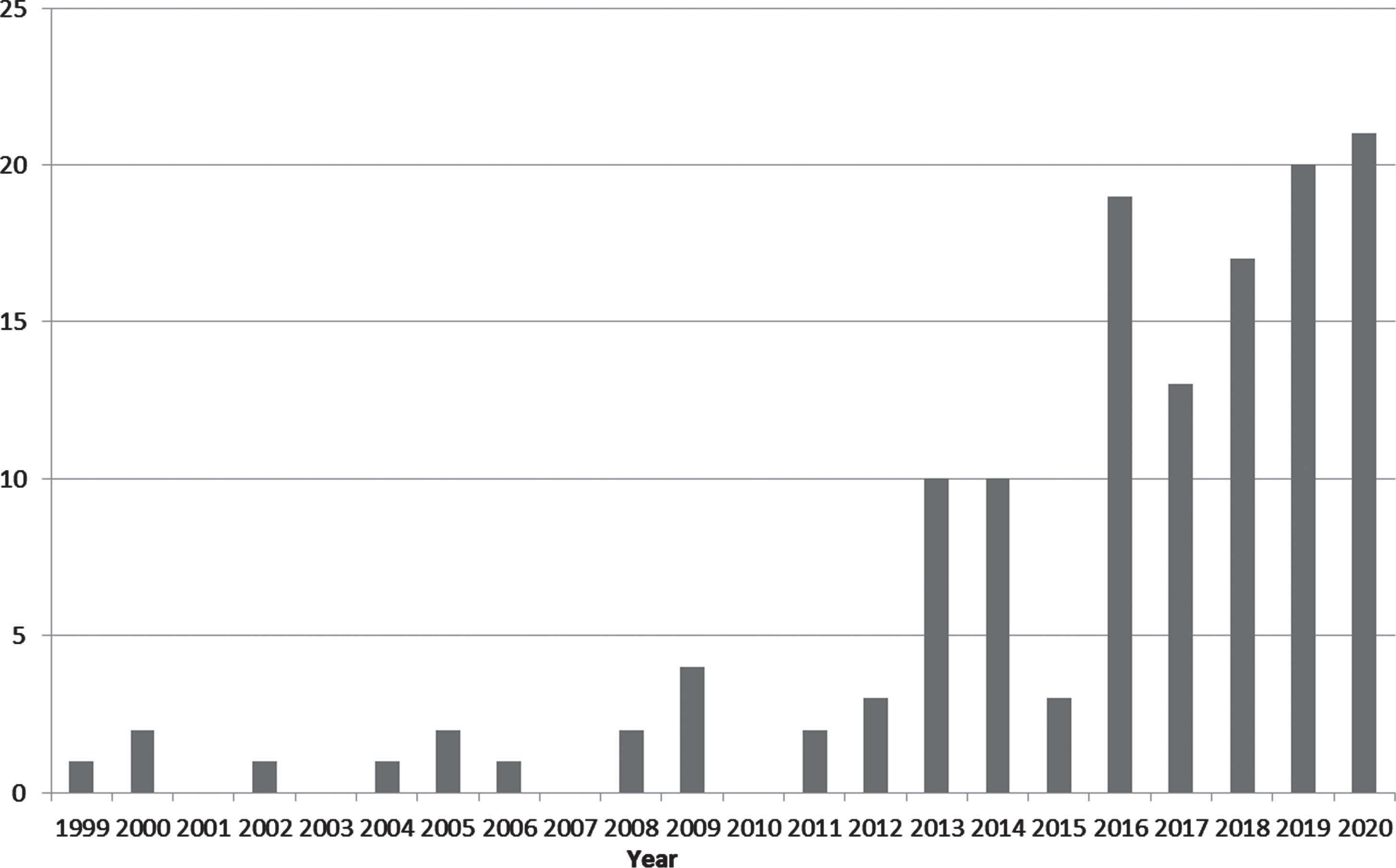
Where player attributes were analysed, either statistical, machine learning or a mixture of both techniques were applied (Table 3), with 117 of the 132 papers conducting some form of analysis, and over two thirds of these solely applying descriptive statistical techniques. The remaining 15 used combinations of machine learning and statistical techniques. Where machine learning was deployed, linear regression techniques were the most deployed, however, as we might expect, a variety of other commonly used ML techniques were also used (Table 4). It should be noted that the number of papers analysed in Table 4 is consistent with some papers deploying more than one technique, for example, the deployment of a combination of artificial neural networks, case based reasoning systems and k- Nearest neighbor algorithms is noteworthy in the paper A study of Prediction models for football player valuations by quantifying statistical and economic attributes for the global transfer market (Patnaik et al., 2019). Table 14 illustrates the very wide variety of research topics both statistical and machine learning techniques are applied to.
Table 3
Data analysis methods
Method of Analysis | No. of papers | % of Papers (excluding those N/A) |
Statistical Analyses | 81 | 69% |
Machine Learning | 28 | 24% |
Mixture | 8 | 7% |
Total | 117 |
Table 4
Analysis of machine learning techniques
Machine Learning Technique | No. of Papers | % |
Linear regression | 18 | 50% |
Neural network | 10 | 28% |
Clustering | 7 | 19% |
Random Forest | 7 | 19% |
Decision tree | 6 | 17% |
K Nearest Neighbour | 6 | 17% |
Support Vector Machine | 5 | 14% |
Feature weighting | 1 | 3% |
Gradient boosting trees regression | 1 | 3% |
3.2Player attributes
The resulting database comprised 2,537 extracted attributes, including those attributes duplicated across papers (noted to permit analyses of their frequency of use). Following the removal of duplicates, a master list of 1,518 attributes was produced for future analysis.
After allocation of attributes to each of the 25 selected groups, comparisons between the predominance of attributes in the different groups were calculated (Table 5).
Table 5
Attribute groups
Attribute Group | No. of Attributes6 | % |
Pass | 355 | 13% |
Goals, shots & shooting | 343 | 12% |
Player data & history | 342 | 12% |
Outfielder position specific | 318 | 12% |
Speed & movement | 308 | 11% |
Applicable to any player | 171 | 6% |
Goalkeeper | 140 | 5% |
Tackles | 89 | 3% |
Character traits | 83 | 3% |
Aerial & header | 75 | 3% |
Fouls & cards | 64 | 2% |
Possession | 62 | 2% |
Cross | 58 | 2% |
Dribble | 52 | 2% |
Duel | 45 | 2% |
Free kick | 42 | 2% |
Interception | 38 | 1% |
Clearance | 30 | 1% |
Block | 28 | 1% |
Ball recovery | 26 | 1% |
Error, mistake, fail | 26 | 1% |
Ball | 22 | 1% |
Assist | 20 | 1% |
Offside | 16 | 1% |
Injury | 3 | 0% |
Total | 2756 | 100% |
6Where an attribute appropriate to more than one group it has been included in each.
Perhaps unsurprisingly, the groups pass and Goals, shots & shooting comprised the two with the highest proportion of attributes analysed by researchers. These were very closely followed by Player data & history. This attribute group includes player demographic (data and history) and attributes such as age, international caps, playing position and assessments of their motivation, potential and specialties such as free kicks, playmaking etc.
Similarly the group Outfield player specific which directly addresses attributes for each of defenders, attackers, midfielders etc. followed closely in terms of proportion of attributes collected, including attributes such as wide midfielder interceptions, forward successful aerial duels, central midfielder shots.
The next most analysed attributes are those measuring player speed and movement such as locations of play, speeds and percentages of times spent jogging/walking or running.
These first 5 of the 25 groups accounted for 60% of the attributes selected by researchers for collection and analysis.
Despite each being a critical part of success in matches, it is a little surprising that related attributes such as possession, dribbling, ball recovery, interceptions and blocking are not more highly placed in analyses; none of these were higher than 2% of the attributes analysed.
As football fans will recognize, while pundits, coaches and fans spend a great deal of time discussing players skills such as speed, passing vision, shooting, free kick taking, a great deal of emphasis appears to be placed upon their character traits such as attitude, composure, influence, motivation. Given this it is somewhat surprising that only 3% of such attributes have been considered for analysis in our research findings.
3.3Player attribute data types
An analysis of attribute data types is presented in Table 6 below. More than four fifths (81%) of all player attributes are numeric, allowing analysis by a wide range of statistical and machine learning techniques and a further 7% are ordinal.
Table 6
Attribute data types
Attribute | Number of Group | Numeric Attributes7 | Ordinal | Nominal | |||
Number7 | % | Number7 | % | Number7 | % | ||
Pass | 355 | 309 | 87% | 4 | 1% | 42 | 12% |
Goals, shots & shooting | 343 | 259 | 76% | 10 | 3% | 74 | 22% |
Player data & history | 342 | 173 | 51% | 78 | 23% | 91 | 27% |
Outfielder position specific | 318 | 304 | 96% | 3 | 1% | 11 | 3% |
Speed & movement | 308 | 286 | 93% | 16 | 5% | 6 | 2% |
Applicable to any player | 171 | 118 | 69% | 26 | 15% | 27 | 16% |
Goalkeeper | 140 | 126 | 90% | 7 | 5% | 7 | 5% |
Tackles | 89 | 79 | 89% | 3 | 3% | 7 | 8% |
Character traits | 83 | 36 | 43% | 41 | 49% | 6 | 7% |
Aerial & header | 75 | 64 | 85% | 0 | 0% | 11 | 15% |
Fouls & cards | 64 | 62 | 97% | 0 | 0% | 2 | 3% |
Possession | 62 | 58 | 94% | 3 | 5% | 1 | 2% |
Cross | 58 | 52 | 90% | 0 | 0% | 6 | 10% |
Dribble | 52 | 45 | 87% | 2 | 4% | 5 | 10% |
Duel | 45 | 43 | 96% | 0 | 0% | 2 | 4% |
Free kick | 42 | 28 | 67% | 4 | 10% | 10 | 24% |
Interception | 38 | 37 | 97% | 0 | 0% | 1 | 3% |
Clearance | 30 | 29 | 97% | 0 | 0% | 1 | 3% |
Block | 28 | 25 | 89% | 0 | 0% | 3 | 11% |
Ball recovery | 26 | 23 | 88% | 3 | 12% | 0 | 0% |
Error, mistake, fail | 26 | 19 | 73% | 0 | 0% | 7 | 27% |
Ball | 22 | 19 | 86% | 0 | 0% | 3 | 14% |
Assist | 20 | 20 | 100% | 0 | 0% | 0 | 0% |
Offside | 16 | 15 | 94% | 0 | 0% | 1 | 6% |
Pass | 3 | 2 | 67% | 0 | 0% | 1 | 33% |
Total | 2756 | 2231 | 81% | 200 | 7% | 325 | 12% |
7Where an attribute appropriate to more than one group it has been included in each.
Of the remaining 12% nominal attributes, almost 30% (91 of 325) are player demographic attributes, such as name, team, position, dominant foot, in the Player data and history group. This is followed by 23% (74 of 325) and 13% (42 of 325) in the Goals, shots and shooting and Pass groups respectively.
Noting the data types present in the data set is essential as not all machine learning techniques are suitable to be applied to combined numeric and nominal data, and while it is possible to encode the nominal data as numeric, this does not exploit the strengths of the technique. For example, in the cases of K-nearest neighbours, the distance measurement needs to be adjusted to cope with a data set involving both continuous values and nominal values. Decision trees, random forest and naïve Bayes techniques, however are suitable for the analysis of mixed data.
For most attributes their measurement may be either quantitative or qualitative. For example, passing could be measured as the number of passes during a specified period or as the quality of passing (where quality could be defined on a Likert scale - poor, average, good, very good) or as a nominal value such as passing back (yes/no).
With the exception of the Player data and history and the Character traits attribute groups, all other groups are comprised of 67% and above numeric attributes and in total numeric and ordinal attribute counts comprise almost 90% of total attributes.
3.4Player attribute data accuracy
An analysis of attribute data accuracy is presented in Table 7 below. The majority (84%) of player attributes are objectively measured, i.e. are capable of unambiguous measurement, for example, the number of goals scored, the percentage of time running or jogging, the position of a player on the pitch at any given time. It is important to identify which attributes fall into this category as analyses based upon objective data are fundamentally more reliable.
Table 7
Attribute accuracy
Attribute Group | Number of | Objective (Measurable) | Subjective | Mixture | |||
Group | Attributes8 | Number8 | % | Number8 | % | Number8 | % |
Pass | 355 | 337 | 95% | 18 | 5% | 0 | 0% |
Goals, shots & shooting | 343 | 317 | 92% | 26 | 8% | 0 | 0% |
Player data & history | 342 | 163 | 48% | 178 | 52% | 1 | 0% |
Outfielder position specific | 318 | 302 | 95% | 16 | 5% | 0 | 0% |
Speed & movement | 308 | 284 | 92% | 23 | 7% | 1 | 0% |
Applicable to any player | 171 | 121 | 71% | 49 | 29% | 1 | 1% |
Goalkeeper | 140 | 126 | 90% | 14 | 10% | 0 | 0% |
Tackles | 89 | 78 | 88% | 11 | 12% | 0 | 0% |
Character traits | 83 | 18 | 22% | 65 | 78% | 0 | 0% |
Aerial & header | 75 | 71 | 95% | 4 | 5% | 0 | 0% |
Fouls & cards | 64 | 64 | 100% | 0 | 0% | 0 | 0% |
Possession | 62 | 59 | 95% | 3 | 5% | 0 | 0% |
Cross | 58 | 52 | 90% | 6 | 10% | 0 | 0% |
Dribble | 52 | 47 | 90% | 5 | 10% | 0 | 0% |
Duel | 45 | 45 | 100% | 0 | 0% | 0 | 0% |
Free kick | 42 | 28 | 67% | 14 | 33% | 0 | 0% |
Interception | 38 | 36 | 95% | 2 | 5% | 0 | 0% |
Clearance | 30 | 30 | 100% | 0 | 0% | 0 | 0% |
Block | 28 | 28 | 100% | 0 | 0% | 0 | 0% |
Ball recovery | 26 | 23 | 88% | 3 | 12% | 0 | 0% |
Error, mistake, fail | 26 | 26 | 100% | 0 | 0% | 0 | 0% |
Ball | 22 | 22 | 100% | 0 | 0% | 0 | 0% |
Assist | 20 | 20 | 100% | 0 | 0% | 0 | 0% |
Offside | 16 | 16 | 100% | 0 | 0% | 0 | 0% |
Injury | 3 | 1 | 33% | 2 | 67% | 0 | 0% |
Total | 2756 | 2314 | 84% | 439 | 16% | 3 | 0% |
8Where an attribute appropriate to more than one group it has been included in each.
However, that is not to say that subjective data are not valuable. For example, the assessment of a player’s potential is likely to remain most accurately assessed by the subject matter experts, in this case managers and coaches. Other subjective attributes include ball control skill and composure.
It is also important to note that in some of the collections of freely available attribute data (Table 1) elements of the data collection are delegated to selected fans attending matches who provide their data. These data also have value but must be clearly identified as subjective, compared to subject matter experts and treated with care in any scientific analysis.
As we identified in the analysis of attribute data types we can see that it is the Player data and history and the Character traits attribute groups that depend upon the highest numbers of subjective assessments, for example, self-confidence, motivation, playing style, degree of ball control. In the case of data accuracy we can add to this the attribute group Applicable to any player. This group includes attributes such as ball control skill, effective/balanced defensive play, performance rating at a given position, all measurable subjectively. However, upon close inspection of individual attributes in all the Player data and history and the performance rating at a given position groups, although they were treated as subjective in the source research papers, it is clear that many may be collected objectively. For example, pass accuracy can also be measured as the percentage of successful pass completions.
In the case of Character traits, although the majority (78%) have been identified as subjective, there is a significant body of scientific evidence supporting how a number of these may be more rigorously measured using cognitive psychometric testing. We discuss this later under the section Potential for exploitation of character trait attributes.
Minimal player attributes which were derived from a mixture of objective and subjective data were identified. An example is Number of man of the match awards where although the number of awards is an objective value, the award itself is in each case a subjective selection by a human being or group of human beings.
3.5Player attribute data temporality
An analysis of attribute data temporality is presented in Table 8 below.
Table 8
Attribute temporality
Attribute | Number of Group | Static | Evolving Static | Dynamic | |||
Attributes9 | Number 9 | % | Number9 | % | Number9 | % | |
Player data & history | 355 | 0 | 0% | 9 | 3% | 346 | 97% |
Outfielder position specific | 343 | 0 | 0% | 26 | 8% | 317 | 92% |
Speed & movement | 342 | 49 | 14% | 189 | 55% | 104 | 30% |
Pass | 318 | 0 | 0% | 18 | 6% | 300 | 94% |
Goals, shots & shooting | 308 | 4 | 1% | 23 | 7% | 281 | 91% |
Applicable to any player | 171 | 7 | 4% | 35 | 20% | 129 | 75% |
Goalkeeper | 140 | 0 | 0% | 12 | 9% | 128 | 91% |
Character traits | 89 | 0 | 0% | 10 | 11% | 79 | 89% |
Tackles | 83 | 5 | 6% | 57 | 69% | 21 | 25% |
Aerial & header | 75 | 0 | 0% | 4 | 5% | 71 | 95% |
Possession | 64 | 0 | 0% | 0 | 0% | 64 | 100% |
Fouls & cards | 62 | 0 | 0% | 3 | 5% | 59 | 95% |
Dribble | 58 | 0 | 0% | 4 | 7% | 54 | 93% |
Free kick | 52 | 0 | 0% | 4 | 8% | 48 | 92% |
Cross | 45 | 0 | 0% | 0 | 0% | 45 | 100% |
Interception | 42 | 0 | 0% | 17 | 40% | 25 | 60% |
Block | 38 | 0 | 0% | 2 | 5% | 36 | 95% |
Duel | 30 | 0 | 0% | 0 | 0% | 30 | 100% |
Clearance | 28 | 0 | 0% | 0 | 0% | 28 | 100% |
Error, mistake, fail | 26 | 0 | 0% | 3 | 12% | 23 | 88% |
Ball | 26 | 0 | 0% | 0 | 0% | 26 | 100% |
Ball recovery | 22 | 0 | 0% | 0 | 0% | 22 | 100% |
Assist | 20 | 0 | 0% | 0 | 0% | 20 | 100% |
Offside | 16 | 0 | 0% | 0 | 0% | 16 | 100% |
Injury | 3 | 0 | 0% | 1 | 33% | 2 | 67% |
Total | 2756 | 65 | 2% | 417 | 15% | 2274 | 83% |
9Where an attribute appropriate to more than one group it has been included in each.
The majority of published research activity into footballer analytics focuses upon their performance during matches and this is reflected in the high proportion (83%) of player attributes categorised as dynamic. As we would therefore expect, these focus upon player activities such as assists, pass, and duels. As with our data type and accuracy metrics, it is the attribute groups Character traits and Player data and history that have the least dynamic measurements.
It is important to note, however, that in a number of attribute groups we can see player attributes which although they may be viewed as a static statement of a player’s ability or performance, are also capable of change: these are therefore categorised as evolving static. For example, the quality of free kick taking or shooting accuracy are examples of capabilities which may be improved through practice and coaching on the training ground and match experience. Similarly in the group Player data and history, a player’s strength and fitness levels may be developed as part of their inter match training routines. Also, in the group Character traits, a player’s self-confidence and a selection of mentality traits are good examples of player attributes which may be developed.
3.6Player attribute data accessibility and sensitivity
An analysis of attribute data accessibility and sensitivity is presented in Table 9 below.
Table 9
Attribute Accessibility and Sensitivity
Attribute | Player data & history | Character traits |
Accessibility | ||
Readily accessible | 277 | 29 |
Player input required | 65 | 54 |
Sensitivity | ||
Readily available | 246 | 0 |
Sensitive | 61 | 83 |
Potentially Sensitive | 35 | 0 |
Accessibility of player attributes alongside sensitivity (privacy/ethical) issues is critically important in all analysis activities.
In terms of accessibility, there is a considerable difference between those attributes which are readily accessible and measurable, such as the number of passes or shots and data which may only be collected through direct interaction and cooperation with the player, such as the level of family support.
A great deal of activity is being invested into the development of automated vision systems to recognise and count such metrics in real time, both for during match punditry and for post-match analysis by clubs too (Castellano et al., 2014). These systems rely upon accurate tracking of momentary position, speed and acceleration measures of players using stereo camera technology (Linke et al., 2020). For example, the application of appropriate computer vision techniques to extract trajectory data from match video input (Stein et al., 2017) allows the automatic collection of metrics such as pass distance, player movement and dominant regions of the pitch.
Of the 25 attribute groups, 23 comprise of attributes which are readily available to anyone for collection and analysis. It is only the group Player data and history and the group Character traits where we find attributes where player input/cooperation is required. Examples in the former group include such attributes as sleep patterns and parental/social support which in total represent fewer than 20% of the attributes in this group. However, in the latter group, Character traits, the proportion of attributes where player input/cooperation is required is almost two thirds (65%). This high proportion is consistent with the potentially intrusive nature of character trait assessments, with its predisposition to psychometric testing.
We see a similar pattern in the assessment of attribute sensitivity in terms of privacy and ethical issues. It is only the Player data and history and Character traits groups where this is an issue. In respect of character traits, by their very nature it is appropriate to categorise all (100%) of these attributes as sensitive. Even where an individual player may be happy for publication of attributes such as game influence or decision making, where these have been rigorously measured as opposed to pundit opinions in the media, the club would likely consider these data commercially sensitive.
In respect of the group Player data and history, we see a clear split between sensitive (18%) and readily available attributes (72%), however we have also categorised a modest number (10%) as potentially sensitive. These include attributes such as body type, provocation, hours of practice and market value. In each case these tend to be attributes where some assessments external to the player and club may be made. Nevertheless, ethical and privacy decisions made by the player and the club will take precedence in these and all cases of attribute accessibility and sensitivity.
4Potential for exploitation of character trait attributes
4.1Inclusion of character traits in the reviewed papers
As described above, very few occurrences of player character traits were identified (proportionally 3% of the total attributes collected). Of the 2,537 attributes identified from the selected papers, only 83 may be categorized as character traits, reducing to 72 after the removal of duplicates. In fact, only 3 of the 132 papers (2%) included a significant number (between 8 and 15) of such attributes in their analyses (Table 10).
Table 10
Papers including character trait attributes
Title | Citation | No. of Character Trait Attributes |
Talent identification and development in soccer | (Williams & Reilly, 2000) | 15 |
Methodological Issues in Soccer Talent Identification Research | (Bergkamp et al., 2019) | 11 |
The foundations of tactics and strategy in team sports | (Godbout & Bouthier, 1999) | 9 |
Choke or Shine? Quantifying Soccer Players’ Abilities to Perform Under Mental Pressure | (Bransen et al., 2019) | 4 |
Game creativity analysis using neural networks | (Memmert & Perl, 2009) | 4 |
Sports Analytics for Football League Table and Player Performance Prediction | (Pantzalis & Tjortjis, 2020) | 4 |
Football Player’s Performance and Market Value. | (He et al., 2015) | 3 |
What’s in a game? A systems approach to enhancing performance analysis in football | (McLean et al., 2017) | 3 |
A novel way to soccer match prediction | (Shin & Gasparyan, 2014) | 2 |
Analysis System for Emotional Behavior in Football Professional football players emotional behavior in ghost games in the Austrian Bundesliga | (Leitner & Richlan, 2020) | 2 |
Behavioral, physiological, and neural signatures of surprise during naturalistic sports viewing | (Anthony et al., 2020) | 2 |
Football Match Prediction Using Players Attributes | (Danisik et al., 2018) | 2 |
Singing it for “us”: Team passion displayed during national anthems is associated with subsequent success | (Slater et al., 2018) | 2 |
A Data Science Approach to Football Team Player Selection | (Rajesh et al., 2020) | 1 |
An option pricing framework for valuation of football players | (Tunaru et al., 2005) | 1 |
Hypernetworks reveal compound variables that capture cooperative and competitive interactions in a soccer match. | (Ramos et al., 2017) | 1 |
The lack of such attributes in the identified body of research is likely to be related to the perceived and actual difficulty of measuring them.
This is surprising given the importance assigned to such characteristics in other businesses. Furthermore, it is evident that football fans seem to regard attributes such as tenacity, composure, determination very highly. Indeed, managers and coaches often refer to these characteristics when discussing individual players in media situations, as do commentators during matches and media pundits in their post-match analyses. Most important, however, is their potential role in the identification of suitable transfer targets.
It is worth noting that in other industries interviewing and psychometric testing is permissible prior to making recruitment decisions. This is not the case in professional football where in transfer considerations no approach to a player is permissible before clubs have agreed terms. Typically, club staff may only meet the player when the subsequent medical and personal terms negotiation is taking place.
4.2Potential approaches to character trait attributes
It would appear that the development of in-roads into the inclusion of selected character traits in footballer analytics could provide a step change in the improvement of successful transfer selection for elite clubs.
In order for the use of a player’s attributes such as self-control, aggression or self-confidence to be useful for analytical or predictive purposes it is critical that some authenticity is given to their measurement.
There would appear to be two alternatives: either, the use of formal psychological testing methods based upon established research-based character trait theory; or, expert-based subjective scoring.
For the latter we may consider a scoring (for example, on a scale of 1 to 10) against each selected attribute, made by each of a psychologist and a club appointed football expert, for example the team coach. The combined, perhaps averaged, score would provide an ordinal value for the attribute. Over time, the measured feedback of results versus prediction scores may allow improvement of the efficacy of the process, however these would remain subjective data.
For the former method, in order to take advantage of the established body of psychological research, a suitable and more objective starting point may be to consider those categorisations already in use in the field of psychology. In particular it may then be feasible to exploit proven methods of character trait measurement. Previous research in this area includes several different categorisations of character/personality traits. For the purposes of this paper, we have included four respected categorisations for illustrative purposes.
Many personality psychologists believe that there are five basic dimensions of personality, often referred to as the “Big 5” personality traits (Digman, 1990). These are openness, conscientiousness, extraversion, agreeableness, and neuroticism, sometimes described by the acronym OCEAN, each of which is sub-dividable into on average five sub-traits.
Another approach is the “Alternative five model of personality” (Zuckerman, 1992) which focusses upon Neurotism, Aggression, Impulsiveness, Sociability and Activity, each of which sub-divide into on average eight sub-traits
The Eysenck Personality Questionnaire (Eysenck, 1975) focuses upon temperament, measuring Extraversion, Neuroticism, Psychoticism and Dissimulation (lying) tendencies. Each of these is further sub-divided into nine further sub-traits.
Lastly, Cattell’s 16 Personality Factors (Cattell, 2008) includes Abstractedness, Apprehension, Dominance, Emotional stability, Liveliness, Openness to change, Perfectionism, Privateness, Reasoning, Rule-consciousness, Self-reliance, Sensitivity, Social boldness, Tension, Vigilance and Warmth.
An examination of the character trait attributes included for analysis in the selected papers (Table 11) indicates that many of these are potentially alignable with one or another of the above formal categorisations, in some cases with appropriate football specific interpretation.
Table 11
Character traits used in selected papers
Character Trait | Source Paper |
Achievement motive | Methodological Issues in Soccer Talent Identification Research |
Aggression | A novel way to soccer match prediction |
Ambition | Choke or Shine? Quantifying Soccer Players’ Abilities to Perform Under Mental Pressure |
Anticipation | Talent identification and development in soccer |
Anxiety intention and direction | Methodological Issues in Soccer Talent Identification Research |
Attitude | Talent identification and development in soccer |
Belief consistent surprise | Behavioral, physiological, and neural signatures of surprise during naturalistic sports viewing |
Belief inconsistent surprise | Behavioral, physiological, and neural signatures of surprise during naturalistic sports viewing |
Cognitive ability - working memory | Game creativity analysis using neural networks |
Cognitive flexibility | Game creativity analysis using neural networks |
Cognitive functions | Methodological Issues in Soccer Talent Identification Research |
Cohesion Principle | The foundations of tactics and strategy in team sports |
Communicate | What’s in a game? A systems approach to enhancing performance analysis in football |
Competency Principle | The foundations of tactics and strategy in team sports |
Composure | A Data Science Approach to Football Team Player Selection |
Contribution to team spirit | Team spirit in football: an analysis of players symbolic communication in a match between Argentina and Iceland at the men’s 2018 World Cup |
Coping | Talent identification and development in soccer |
Dangerousity | Real time quantification of dangerousity in football using spatiotemporal tracking data |
Deception Principle | The foundations of tactics and strategy in team sports |
Decision making | Talent identification and development in soccer |
Defenders’ dilemma | Hypernetworks reveal compound variables that capture cooperative and competitive interactions in a soccer match. |
Determination | Sports Analytics for Football League Table and Player Performance Prediction |
Discipline | What’s in a game? A systems approach to enhancing performance analysis in football |
Drive to improve | Talent identification and development in soccer |
Economy Principle | The foundations of tactics and strategy in team sports |
Efficiency | Football Player’s Performance and Market Value. |
Ego orientation | Methodological Issues in Soccer Talent Identification Research |
Endurance | Talent identification and development in soccer |
Execution weighted score or mark for ingenious executions | An option pricing framework for valuation of football players |
Executive functions | Methodological Issues in Soccer Talent Identification Research |
Flexibility | Talent identification and development in soccer |
Game intelligence | Talent identification and development in soccer |
Government | Talent identification and development in soccer |
Growth | Player Performance Prediction in Football Game |
Harmonious passion | Singing it for “us”: Team passion displayed during national anthems is associated with subsequent success |
Improvement Principle | The foundations of tactics and strategy in team sports |
Influence | Wide Open Spaces: A statistical technique for measuring space creation in professional soccer |
In-game mental pressure | Choke or Shine? Quantifying Soccer Players’ Abilities to Perform Under Mental Pressure |
Judgement | Game creativity analysis using neural networks |
Leadership | What’s in a game? A systems approach to enhancing performance analysis in football |
Lower and higher cognitive functions | Methodological Issues in Soccer Talent Identification Research |
Maturity | Methodological Issues in Soccer Talent Identification Research |
Mental rating | Football Player’s Performance and Market Value. |
Mental toughness | Talent identification and development in soccer |
Mobility Principle | The foundations of tactics and strategy in team sports |
Motivation | Talent identification and development in soccer |
Net hope | Methodological Issues in Soccer Talent Identification Research |
Obsessive passion | Singing it for “us”: Team passion displayed during national anthems is associated with subsequent success |
Opportunity Principle | The foundations of tactics and strategy in team sports |
Overall mental pressure | Choke or Shine? Quantifying Soccer Players’ Abilities to Perform Under Mental Pressure |
Physical precocity | The roles of talent, physical precocity and practice in the development of soccer expertise |
Pre-game mental pressure (no, low, normal, high) | Choke or Shine? Quantifying Soccer Players’ Abilities to Perform Under Mental Pressure |
Presence | Football Player’s Performance and Market Value. |
Professionalism and ability to perform well in important matches | Sports Analytics for Football League Table and Player Performance Prediction |
Provocation | Analysis System for Emotional Behavior in Football Professional football players emotional behavior in ghost games in the Austrian Bundesliga |
Reserve Principle | The foundations of tactics and strategy in team sports |
Self-confidence | Talent identification and development in soccer |
Self-Adaptor (undirected nonverbal behavior (e.g. self-reproaches after missed chance)) | Analysis System for Emotional Behavior in Football Professional football players emotional behavior in ghost games in the Austrian Bundesliga |
Self-concept | Methodological Issues in Soccer Talent Identification Research |
Self-control | Talent identification and development in soccer |
Self-determination | Methodological Issues in Soccer Talent Identification Research |
Self-efficacy | Methodological Issues in Soccer Talent Identification Research |
Self-regulation | Talent identification and development in soccer |
Sport orientation | Methodological Issues in Soccer Talent Identification Research |
Surprise Principle | The foundations of tactics and strategy in team sports |
Sustained attention | Game creativity analysis using neural networks |
Task and ego orientation | Methodological Issues in Soccer Talent Identification Research |
Versatility | Sports Analytics for Football League Table and Player Performance Prediction |
Vision | A novel way to soccer match prediction |
Visual search or scanning | Talent identification and development in soccer |
Volition | Methodological Issues in Soccer Talent Identification Research |
Work rate | Performance analysis in football A critical review and implications for future research |
Note: The 72 character trait attributes tabulated correspond to a total of 83 identified from the selected papers, less duplicates.
We discuss potential next steps under recommendations for future research.
5Conclusions
A systematic review of the literature shows a steep increase in the number of studies involving football analytics research in the past seven years.
There appears to be scope for increasing and intensifying the application of machine learning analyses given that of the 103 papers conducting some form of analysis, 65% solely applied statistical techniques and only 21% applied ML techniques with the remaining 6% applying a mixture of both. Where machine learning was used, Linear regression techniques were the most deployed, however, as we might expect a variety of other commonly used ML techniques were also used, for example neural networks, clustering, random forest, decision tree, k nearest neighbour and support vector machines.
The sport of football allows the identification and measurement of a very large number of attributes. Over 1,500 different footballer attributes were curated from the selected papers.
However, of the 1,518, only 70 could be categorised as character traits. Experience from all other industries indicates that analyses of footballers’ potential may benefit from consideration of these traits (Tett, 1991).
A significant majority of all attributes (81%) are numeric (measurable) and a further 7% ordinal, therefore lending them to rigorous analysis and predictive techniques. The remaining 12% nominal attributes were mainly in the character trait and player base data groups and may be analysed separately in the first instance by proven statistical and machine learning techniques.
The majority (84%) of all attributes were categorised as objective, similarly supporting more scientifically credible analyses.
As with the remaining subjective data, attribute accessibility and sensitivity issues were also entirely focused on the player data and history and the character trait groups.
Because of this it would be appropriate to treat these two groups with more care in future analyses.
In respect of attribute subjectivity, where analyses include attributes which are collected by fans it is important that the results of subsequent analysis and predictions are noted as such.
Clearly, the very large number of over 1500 different attributes warrants examination in terms of their independence and usefulness. Although some papers have applied principle component analysis (PCA) methods to reduce dimensionality there does not appear to be a comprehensive study available. Such a study may be able to reduce the attributes list for analysis and prediction purposes.
6Recommendations for future work
It would be interesting to apply dimensionality reduction methods, for example principal component analysis, to the comprehensive attribute set, populated from freely available data. This research may allow the identification of a useful but reduced attribute set.
The comparative predictive accuracy of appropriately selected machine learning techniques, e.g. decision trees, neural networks, k nearest neighbors, random forest, etc. may be analysed, applied to the reduced attribute set.
The allocation of attributes to the selected groups would benefit from the input of club subject matter experts in order to better align groups. For example, Player data and history, and Outfielder position specific attribute groups. Similarly, club expert input into the selection of those character traits deemed critical to player selection would be beneficial.
The identification of an appropriate mapping of those character trait attributes identified in this paper to the traits defined within proven methods of character trait measurement may be of benefit, as may be the exploration of methods that involve using such data in the analysis of football transfer targets.
References
1 | Ahmed, M. , 2016, Can Artificial Intelligence Modelling Approaches Assist Football Clubs In Identifying Transfer Targets, While Maintaining A Fair Transfer Market Using Player Performance Data? (Doctoral dissertation, Cardiff Metropolitan University). |
2 | Allegre, J. , & Vuillemot, R. , 2020, October. Visualizing and Analyzing Disputed Areas in Soccer. In Visualization in Data Science. |
3 | Andrienko, G. , Andrienko, N. , Budziak, G. , Dykes, J. , Fuchs, G. , von Landesberger, T. , & Weber, H. , (2017) , Visual analysis of pressure in football, Data Mining and Knowledge Discovery 31: (6), 1793–1839. |
4 | Antony, J. W. , Hartshorne, T. H. , Pomeroy, K. , Gureckis, T. M. , Hasson, U. , McDougle, S. D. , & Norman, K. A. , 2020, Behavioral, physiological, and neural signatures of surprise during naturalistic sports viewing. Neuron. |
5 | Apostolou, K. , & Tjortjis, C. , (2019) , July. Sports Analytics algorithms for performance prediction. In 2019 10th International Conference on Information, Intelligence, Systems and Applications (IISA) (pp. 1–4). IEEE. |
6 | Aquino, R. L.Q. T. , Cruz Goncalves, L. G. , Palucci Vieira, L. H. , Oliveira, L. P. , Alves, G. F. , Pereira Santiago, P. R. , & Puggina, E. F. , (2016) , Periodization training focused on technical-tactical ability in young soccer players positively affects biochemical markers and game performance, Journal of Strength and Conditioning Research 30: (10), 2723–2732. |
7 | Araújo, D. , Passos, P. , Esteves, P. , Duarte, R. , Lopes, J. , Hristovski, R. , & Davids, K. , (2015) , The micro-macro link inunderstanding sport tactical behaviours: Integrating information andaction at different levels of system analysis in sport, Movement & Sport Sciences-Science & Motricité (89), pp. 53–63. |
8 | Ayer, R. , 2012, Big 2’s and Big 3’s: Analyzing how a team’s best players complement each other. MIT Sloan Sports Analytics Conference. |
9 | Baptista, J. , Travassos, B. , Gonçalves, B. , Mourão, P. , Viana, J. L. , & Sampaio, J. , (2020) , Exploring the Effects of Playing Formations on Tactical Behavior and External Workload During Football Small-Sided Games, The Journal of Strength & Conditioning Research 34: (7), 2024–2030. |
10 | Barnabé, L. , Volossovitch, A. , Duarte, R. , Ferreira, A. P. , & Davids, K. , (2016) , Age-related effects of practice experience oncollective behaviours of football players in small-sided games, Human Movement Science 48: , 74–81. |
11 | Barreira, J. , Garganta, D. , Guimaraes, P. , Machado, J. , & Anguera, M. T. , (2014) , Ball recovery patterns as a performance indicator in elite soccer, Proceedings of the Institution of Mechanical Engineers, Part P: Journal of Sports Engineering and Technology 228: (1), 61–72. |
12 | Barron, D. , Ball, G. , Robins, M. , & Sunderland, C. , (2018) , Artificial neural networks and player recruitment in professional soccer, PloS one 13: (10), e0205818. |
13 | Barron, D. , Ball, G. , Robins, M. , & Sunderland, C. , (2020) , Identifying playing talent in professional football using artificialneural networks, Journal of Sports Sciences 38: (11-12), 1211–1220. |
14 | Beetz, M. , Kirchlechner, B. , & Lames, M. , (2005) , Computerized real-time analysis of football games, IEEE Pervasive Computing 4: (3), 33–39. |
15 | Beetz, M. , von Hoyningen-Huene, N. , Kirchlechner, B. , Gedikli, S. , Siles, F. , Durus, M. , & Lames, M. , (2009) , Aspogamo: Automated sports game analysis models, International Journal of Computer Science in Sport 8: (1), 1–21. |
16 | Bergkamp, T. L. , Niessen, A. S. M. , Den Hartigh, R. J. , Frencken, W. G. , & Meijer, R. R. , (2019) , Methodological issues in soccer talent identification research, Sports Medicine 49: (9), 1317–1335. |
17 | Bertin, M. , 2015. Why soccer’s most popular advanced stat kind of sucks. |
18 | Bialkowski, A. , Lucey, P. , Carr, P. , Yue, Y. , & Matthews, I. , 2014, February.Win at home and draw away: Automatic formation analysis highlighting the differences in home and away team behaviors, In Proceedings of 8th annual MIT Sloan sports analytics conference (pp. 1–7). |
19 | Bialkowski, A. , Lucey, P. , Carr, P. , Yue, Y. , Sridhara, S. , & Matthews, I. , (2014) , December. Identifying team style in soccer using formations learned from spatiotemporal tracking data. In 2014 IEEE International Conference on Data Mining Workshop (pp. 9–14). IEEE. |
20 | Bialkowski, A. , Lucey, P. , Carr, P. , Matthews, I. , Sridharan, S. , & Fookes, C. , (2016) , Discovering team structures in soccer from spatiotemporal data, IEEE Transactions on Knowledge and Data Engineering 28: (10), 2596–2605. |
21 | Bojinov, I. , & Bornn, L. , 2016, The pressing game: Optimal defensive disruption in soccer. In 10th MIT Sloan Sports Analytics Conference. |
22 | Bradley, P. S. , Carling, C. , Diaz, A. G. , Hood, P. , Barnes, C. , Ade, J. , Boddy, M. , Krustrup, P. , & Mohr, M. , (2013) , Match performance and physical capacity of players in the top three competitive standards of English professional soccer, Human Movement Science 32: (4), pp. 808–821. |
23 | Bransen, L. , Robberechts, P. , Van Haaren, J. , & Davis, J. , 2019. Choke or Shine? Quantifying Soccer Players’ Abilities to Perform Under Mental Pressure. In Proceedings of the 13th MIT Sloan Sports Analytics Conference (pp. 1–25). MIT SLOAN; http://www.sloansportsconference.com/wp-content/uploads/2019/02/Choke-or-Shine-Quantifying-Soccer-Players-Abilities-to-Perform-Under-Mental-Pressure.pdf. |
24 | Brito Souza, D. , López-Del Campo, R. , Blanco-Pita, H. , Resta, R. , & Del Coso, J. , (2019) , A new paradigm to understand success in professional football: analysis of match statistics in LaLiga for complete seasons, International Journal of Performance Analysis in Sport 19: (4), 543–555. |
25 | Brooks, J. , Kerr, M. , & Guttag, J. , 2016, August. Developing a data-driven player ranking in soccer using predictive model weights. In Proceedings of the 22nd ACM SIGKDD International Conference on Knowledge Discovery and Data Mining (pp. 49-55). |
26 | Bundesliga Database. (2020). Data Hub. Available at: https://datahub.io/sports-data/german-bundesliga |
27 | Bunker, R. , & Susnjak, T. , 2019. The application of machine learning techniques for predicting results in team sport: a review. arXiv preprint arXiv:1912.11762. |
28 | Castellano, J. , Alvarez-Pastor, D. , & Bradley, P. S. , (2014) , Evaluation of research using computerised tracking systems(Amisco® and Prozone®) to analyse physicalperformance in elite soccer: A systematic review, SportsMedicine 44: (5), 701–712. |
29 | Cattell, H. E. , & Mead, A. D. , 2008, The Sixteen Personality Factor Questionnaire (16PF). |
30 | Chassy, P. , (2013) , Team play in football: How science supports FC Barcelona’s training strategy, Psychology 4: (09), 7. |
31 | Clemente, F. M. , Couceiro, M. S. , Martins, F. M. L. , Mendes, R. S. , & Figueiredo, A. J. , (2014) , Intelligent systems for analyzing soccergames: The weighted centroid, Ingeniería eInvestigación 34: (3), 70–75. |
32 | Collet, C. , (2013) , The possession game? A comparative analysis of ball retention and team success in European and international football, 2007–2010, Journal of Sports Sciences 31: (2), 123–136. |
33 | Couceiro, M. S. , Clemente, F. M. , Martins, F. M. , & Machado, J. A. T. , (2014) , Dynamical stability and predictability of football players: the study of one match, Entropy 16: (2), 645–674. |
34 | Danisik, N. , Lacko, P. , & Farkas, M. , (2018) , August. Football match prediction using players attributes. In 2018 World Symposium on Digital Intelligence for Systems and Machines (DISA) (pp. 201–206). IEEE. |
35 | de Sousa, S. F. , Araújo, A. D. A. , & Menotti, D. , (2011) , January. An overview of automatic event detection in soccer matches. In 2011 IEEE Workshop on Applications of Computer Vision (WACV) (pp. 31–38). IEEE. |
36 | Decroos, T. , Bransen, L. , Van Haaren, J. , & Davis, J. , 2019, July. Actions speak louder than goals: Valuing player actions in soccer. In Proceedings of the 25th ACM SIGKDD International Conference on Knowledge Discovery & Data Mining (pp. 1851–1861). |
37 | Decroos, T. , & Davis, J. , 2020, Valuing on-the-ball actions in soccer: a critical comparison of XT and VAEP. In Proceedings of the AAAI-20 Workshop on Artifical Intelligence in Team Sports. AI in Team Sports Organising Committee. |
38 | Deloitte, 2017, Annual Review of Football Finance: Ahead of the Curve. |
39 | Deloitte, 2020, Annual Review of Football Finance: Home Truths. |
40 | Dendir, S. , (2016) , When do soccer players peak? A note, Journal of Sports Analytics 2: (2), 89–105. |
41 | Dey, S. , 2017, Pricing Football Players using Neural Networks. arXiv preprint arXiv:1711.05865. |
42 | Digman, J. M. , (1990) , Personality structure: Emergence of thefive-factor model, Annual Review of Psychology 41: (1), 417–440. |
43 | Drust, B. , & Green, M. , (2013) , Science and football: evaluating the influence of science on performance, Journal of Sports Sciences 31: (13), pp. 1377–1382. |
44 | Duarte, R. , Araújo, D. , Correia, V. , Davids, K. , Marques, P. , & Richardson, M. J. , (2013) , Competing together: Assessing thedynamics of team–team and player–team synchrony inprofessional association football, Human Movement Science 32: (4), pp. 555–566. |
45 | Dubitzky, W. , Lopes, P. , Davis, J. , & Berrar, D. (2017), OSF Home. The Open International Soccer Database. Available at: https://doi.org/10.17605/OSF.IO/KQCYE. |
46 | Dubitzky, W. , Lopes, P. , Davis, J. , & Berrar, D. , (2019) , The open international soccer database for machine learning, Machine Learning 108: (1), 9–28. |
47 | Ermidis, G. , Randers, M. B. , Krustrup, P. , & Mohr, M. , (2019) , Technical demands across playing positions of the Asian Cup in male football, International Journal of Performance Analysis in Sport 19: (4), 530–542. |
48 | Eysenck, H. J. , (1975) , Manual of the Eysenck Personality Questionnaire. San Edacational and Industrial Testing Service, San Diego CA. |
49 | Fernandez, J. , & Bornn, L. , 2018, February. Wide Open Spaces: A statistical technique for measuring space creation in professional soccer. In Sloan Sports Analytics Conference (Vol. 2018). |
50 | Filetti, C. , Ruscello, B. , D’Ottavio, S. , & Fanelli, V. , (2017) , A study of relationships among technical, tactical, physical parameters and final outcomes in elite soccer matches as analyzed by a semiautomatic video tracking system, Perceptual and Motor Skills 124: (3), 601–620. |
51 | Football Database EU. 2020, Home page. Available at: https://www.footballdatabase.eu/en/ |
52 | Ge, T. , An, Z. , Cai, H. , & Wang, Y. , (2020) , August. An analysis on the effectiveness of cooperation in a soccer team. In 2020 15th International Conference on Computer Science & Education (ICCSE) (pp. 787–794). IEEE. |
53 | Gelade, G. A. , & Hvattum, L. M. , 2020, On the relationship between+/–ratings and event-level performance statistics, Journal of Sports Analytics, (Preprint), pp. 1–13. |
54 | Gréhaigne, J. F. , Godbout, P. , & Bouthier, D. , (1999) , The foundations of tactics and strategy in team sports, Journal of Teaching in Physical Education 18: (2), 159–174. |
55 | Goes, F. R. , Kempe, M. , Meerhoff, L. A. , & Lemmink, K. A. , (2019) , Not every pass can be an assist: a data-driven model to measure pass effectiveness in professional soccer matches, Big Data 7: (1), 57–70. |
56 | Goes, F. R. , Meerhoff, L. A. , Bueno, M. J. O. , Rodrigues, D. M. , Moura, F. A. , Brink, M. S. , Elferink-Gemser, M. T. , Knobbe, A. J. , Cunha, S. A. , Torres, R. S. , & Lemmink, K. A. P. M. , 2020, Unlocking the potential of big data to support tactical performance analysis in professional soccer: A systematic review, European Journal of Sport Science, pp. 1–16. |
57 | Gomez, M. A. , Reus, M. , Parmar, N. , & Travassos, B. , (2020) , Exploring elite soccer teams’ performances during different match-status periods of close matches’ comebacks, Chaos, Solitons & Fractals 132: , pp. 109566. |
58 | Gonzalez-Rodenas, J. , Lopez-Bondia, I. , Calabuig, F. , Pérez-Turpin, J. A. , & Aranda, R. , (2016) , Association betweenplaying tactics and creating scoring opportunities in counterattacksfrom United States Major League Soccer games, InternationalJournal of Performance Analysis in Sport 16: (2), 737–752. |
59 | Gracenote Sports Data. 2020, Global Sports Data. Available at: https://www.gracenote.com/sports/global-sports-data/ |
60 | Håland, E. M. , & Wiig, A. S. , 2018, Evaluating Passing Behaviour in Association Football (Master’s thesis, NTNU). |
61 | Håland, E. M. , Wiig, A. S. , Stålhane, M. , & Hvattum, L. M. , (2020) , Evaluating passing ability in association football, IMA Journal of Management Mathematics 31: (1), 91–116. |
62 | Halldorsson, V. , (2019) , Team spirit in football: an analysis of players symbolic communication in a match between Argentina and Iceland at the mens 2018 World Cup, Arctic & Antarctic: International Journal of Circumpolar Sociocultural Issues 12: (12), pp. 45–68. |
63 | Harell, A. , & Bajic, I. V. , 2019, The Data Gap in Sports Analytics and How to Close It. Artificial Intelligence in Team Sports Workshop at The Thirty Fourth AAAI Conference on Artificial Intelligence. |
64 | He, M. , Cachucho, R. , & Knobbe, A. J. , 2015, September. Football Player’s Performance and MarketValue. In Mlsa@ pkdd/ecml (pp. 87–95). |
65 | Helsen, W. , Hodges, N. J. , Winckel, J. V. , & Starkes, J. L. , (2000) , The roles of talent, physical precocity and practice in the development of soccer expertise, Journal of Sports Sciences 18: (9), pp. 727–736. |
66 | Herold, M. , Goes, F. , Nopp, S. , Bauer, P. , Thompson, C. , & Meyer, T. , (2019) , Machine learning in men’s professional football: Current applications and future directions for improving attacking play, International Journal of Sports Science & Coaching 14: (6), 798–817. |
67 | Hobbs, J. , Power, P. , Sha, L. , & Lucey, P. , 2018, February. Quantifying the value of transitions in soccer via spatiotemporal trajectory clustering. In MIT Sloan Sports Analytics Conference. |
68 | Jamil, M. , (2019) , A case study assessing possession regain patterns in English Premier League Football, International Journal of Performance Analysis in Sport 19: (6), 1011–1025. |
69 | Jamil, M. , 2020, Where do the best technical football players in the world come from? Analysing the association between technical proficiency and geographical origin in elite football, Journal of Human Sport and Exercise. |
70 | Jamil, M. , McErlain-Naylor, S. A. , & Beato, M. , (2020) , Investigating the impact of the mid-season winter break on technical performance levels across European football–Does a break in play affect team momentum? International Journal of Performance Analysis in Sport 20: (3), 406–419. |
71 | Jamil, M. , & Kerruish, S. , (2020) , At what age are English Premier League players at their most productive? A case study investigating the peak performance years of elite professional footballers, International Journal of Performance Analysis in Sport 20: (6), 1120–1133. |
72 | Jordet, G. , Bloomfield, J. , & Heijmerikx, J. , 2013, March. The hidden foundation of field vision in English Premier League (EPL) soccer players. In Proceedings of the MIT sloan sports analytics conference. |
73 | Joseph, A. , Fenton, N. E. , & Neil, M. , (2006) , Predicting football results using Bayesian nets and other machine learning techniques, Knowledge-Based Systems 19: (7), 544–553. |
74 | Lago, C. , (2006) , Are winners different from losers? Performance and chance in the FIFA World Cup Germany, International Journal of Performance Analysis in Sport 7: (2), 36–47. |
75 | Lago-Peñas, C. , & Gómez-López, M. , (2014) , How important is it to score a goal? The influence of the scoreline on match performance in elite soccer, Perceptual and Motor Skills 119: (3), 774–784. |
76 | Leitner, M. C. , & Richlan, F. , (2020) , Analysis System for Emotional Behavior in Football (ASEB-F): Professional football players’ emotional behavior in ghost games in the Austrian Bundesliga. Draft version 1 05-08-2020. University of Salzburg, Austria. |
77 | Lepschy, H. , Wäsche, H. , & Woll, A. , (2020) , Success factors in football: an analysis of the German Bundesliga, International Journal of Performance Analysis in Sport 20: (2), 150–164. |
78 | Link, D. , Lang, S. , & Seidenschwarz, P. , (2016) , Real time quantification of dangerousity in football using spatiotemporal tracking data, PloS one 11: (12), e0168768. |
79 | Linke, D. , Link, D. , & Lames, M. , (2020) , Football-specific validity of TRACAB’s optical video tracking systems, PloS one 15: (3), e0230179. |
80 | Liu, H. , Hopkins, W. , Gómez, A. M. , & Molinuevo, S. J. , (2013) , Inter-operator reliability of live football match statistics from OPTA Sportsdata, International Journal of Performance Analysis in Sport 13: (3), 803–821. |
81 | Liu, H. , Yi, Q. , Giménez, J. V. , Gómez, M. A. , & Lago-Peñas, C. , (2015) , Performance profiles of football teams inthe UEFA Champions League considering situational efficiency, International Journal of Performance Analysis in Sport 15: (1), 371–390. |
82 | Mackenzie, R. , & Cushion, C. , (2013) , Performance analysis in football: A critical review and implications for future research, Journal of Sports Sciences 31: (6), 639–676. |
83 | Mao, L. , Peng, Z. , Liu, H. , & Gómez, M. A. , (2016) , Identifying keys to win in the Chinese professional soccer league, International Journal of Performance Analysis in Sport 16: (3), 935–947. |
84 | Mathien, H. , 2016, Dataset. European Soccer Database. Available at: www.kaggle.com/hugomathien/soccer |
85 | Matsuoka, H. , Tahara, Y. , Ando, K. , & Nishijima, T. , Development of Defence and Offence Play Items for Deep Learning Model of Offence Play Analysis in Soccer Game. |
86 | McGuckian, T. B. , Cole, M. H. , Chalkley, D. , Jordet, G. , & Pepping, G. J. , (2020) , Constraints on visual exploration of youth football players during 11v11 match-play: The influence of playing role, pitch position and phase of play, Journal of Sports Sciences 38: (6), 658–668. |
87 | McHale, I. G. , Scarf, P. A. , & Folker, D. E. , (2012) , On the development of a soccer player performance rating system for the English Premier League, Interfaces 42: (4), 339–351. |
88 | McHale, I.G. , & Szczepański, Ł. , (2014) , A mixed effects modelfor identifying goal scoring ability of footballers, Journal ofthe Royal Statistical Society: Series A (Statistics in Society) 177: (2), 397–417. |
89 | McHale, I.G. , & Relton, S. D. , (2018) , Identifying key players in soccer teams using network analysis and pass difficulty, European Journal of Operational Research 268: (1), 339–347. |
90 | McLean, S. , Salmon, P. M. , Gorman, A. D. , Read, G. J. , & Solomon, C. , (2017) , What’s in a game? A systems approach to enhancing performance analysis in football, PloS one 12: (2), e0172565. |
91 | Memmert, D. , & Perl, J. , (2009) , Game creativity analysis using neural networks, Journal of Sports Sciences 27: (2), 139–149. |
92 | Memmert, D. , Klemp, M. , Caparrós, M. G. , & Imkamp, J. , (2020) , Comparison of the football specific tactical performance of women and men in Europe. German Sport University Cologne. |
93 | Mitrotasios, M. , Gonzalez-Rodenas, J. , Armatas, V. , & Aranda, R. , (2019) , The creation of goal scoring opportunities in professionalsoccer. tactical differences between spanish la liga, englishpremier league, german bundesliga and italian serie, A,International Journal of Performance Analysis in Sport 19: (3), 452–465. |
94 | Mohr, M. , Krustrup, P. , & Bangsbo, J. , (2003) , Match performance of high-standard soccer players with special reference to development of fatigue, Journal of Sports Sciences 21: (7), 519–528. |
95 | Moura, F. A. , van Emmerik, R. E. , Santana, J. E. , Martins, L. E. B. , Barros, R. M. L. D. , & Cunha, S. A. , (2016) , Coordination analysis of players’ distribution in football using cross-correlation and vector coding techniques, Journal of Sports Sciences 34: (24), 2224–2232. |
96 | Müller, O. , Simons, A. , & Weinmann, M. , (2017) , Beyond crowd judgments: Data-driven estimation of market value in association football, European Journal of Operational Research 263: (2), 611–624. |
97 | Nsolo, E. , Lambrix, P. , & Carlsson, N. , (2018) , Player valuation in European football (Extended version). Linköping University. |
98 | Oberstone, J. , (2009) , Differentiating the top English premier league football clubs from the rest of the pack: Identifying the keys to success, Journal of Quantitative Analysis in Sports 5: (3). |
99 | Oberstone, J. L. , 2014, Stephen Gerrard vs Frank Lampard 2013-14 Statistical Comparison. Available at: http://eplindex.com/50755/steven-gerrard-frank-lampard-13-14-stats-comparison.html |
100 | PA Sport. 2020. About Actim. Available at: https://web.archive.org/web/20060912203857/http://www.pa-sport.com:80/en/products/actim.html |
101 | Pantuso, G. , & Hvattum, L. M. , (2020) , Maximizing performance with an eye on the finances: a chance-constrained model for football transfer market decisions, TOP 1–29. |
102 | Pantzalis, V. C. , & Tjortjis, C. , (2020) , July. Sports Analytics for Football League Table and Player Performance Prediction. In 2020 11th International Conference on Information, Intelligence, Systems and Applications (IISA) (pp. 1–8). IEEE. |
103 | Pappalardo, L. , & Cintia, P. , (2018) , Quantifying the relation between performance and success in soccer, Advances in Complex Systems 21: (03n04), pp. 1750014. |
104 | Pappalardo, L. , Cintia, P. , Ferragina, P. , Massucco, E. , Pedreschi, D. , & Giannotti, F. , (2019) , PlayeRank: data-driven performance evaluation and player ranking in soccer via a machine learning approach, ACM Transactions on Intelligent Systems and Technology (TIST) 10: (5), pp. 1–27. |
105 | Pappalardo, L. , Cintia, P. , Rossi, A. , Massucco, E. , Ferragina, P. , Pedreschi, D. , & Giannotti, F. , (2019) , A public data set of spatio-temporal match events in soccer competitions, Scientific Data 6: (1), 1–15. |
106 | Pariath, R. , Shah, S. , Surve, A. , & Mittal, J. , (2018) , March. Player Performance Prediction in Football Game. In 2018 Second International Conference on Electronics, Communication and Aerospace Technology (ICECA) (pp. 1148–1153). IEEE. |
107 | Patnaik, D. , Praharaj, H. , Prakash, K. , & Samdani, K. , (2019) , March. Astudy of Prediction models for football player valuations by quantifying statistical and economic attributes for the global transfer market. In 2019 IEEE International Conference on System, Computation,Automation and Networking (ICSCAN) (pp. 1–7). IEEE. |
108 | Perin, C. , Vuillemot, R. , & Fekete, J. D. , 2013, October. Real-Time Crowdsourcing of Detailed Soccer Data. In What’s the score? The 1st Workshop on Sports Data Visualization. |
109 | Perin, C. , Vuillemot, R. , & Fekete, J. D. , (2013) , SoccerStories: Akick-off for visual soccer analysis, IEEE Transactions onVisualization and Computer Graphics 19: (12), 2506–2515. |
110 | Perin, C. , Vuillemot, R. , Stolper, C. D. , Stasko, J. T. , Wood, J. , S. , & Carpendale, . , (2018) , June. State of the art of sports data visualization. In Computer Graphics Forum (Vol. 37: , No. 3, pp. 663–686). |
111 | Power, P. , Ruiz, H. , Wei, X. , & Lucey, P. , 2017, August. Not all passes are created equal: Objectively measuring the risk and reward of passes in soccer from tracking data. In Proceedings of the 23rd ACM SIGKDD international conference on knowledge discovery and data mining (pp. 1605–1613). |
112 | Pratas, J. M. , Volossovitch, A. , & Carita, A. I. , (2018) , Goal scoring in elite male football:Asystematic review, CIPER, Faculdade de Motricidade Humana, SpertLab, Universidade de Lisboa, Portugal. |
113 | Rajesh, P. , Alam, M. , & Tahernezhadi, M. , (2020) , July. A Data Science Approach to Football Team Player Selection. In 2020 IEEE International Conference on Electro Information Technology (EIT) (pp. 175–183). IEEE. |
114 | Rajšp, A. , & Fister, I. , (2020) , A systematic literature review of intelligent data analysis methods for smart sport training, Applied Sciences 10: (9), pp. 3013. |
115 | Rajula, H. S. R. , Verlato, G. , Manchia, M. , Antonucci, N. , & Fanos, V. , (2020) , Comparison of conventional statistical methods with machine learning in medicine: diagnosis, drug development, and treatment, Medicina 56: (9), 455. |
116 | Ramos, J. , Lopes, R. J. , Marques, P. , & Araújo, D. , (2017) , Hypernetworks reveal compound variables that capture cooperative andcompetitive interactions in a soccer match, Frontiers inPsychology 8: , 1379. |
117 | Rampinini, E. , Impellizzeri, F. M. , Castagna, C. , Coutts, A. J. , & Wisløff, U. , (2009) , Technical performance during soccer matches of the Italian Serie A league: Effect of fatigue and competitive level, Journal of Science and Medicine in Sport 12: (1), 227–233. |
118 | Rathi, K. , Somani, P. , Koul, A. V. , & Manu, K. S. , (2020) , Applications of Artificial Intelligence in the Game of Football: The Global Perspective, Researchers World 11: (2), 18–29. |
119 | Rein, R. , & Memmert, D. , (2016) , Big data and tactical analysis in elite soccer: future challenges and opportunities for sports science, SpringerPlus 5: (1), 1–13. |
120 | Rein, R. , Raabe, D. , & Memmert, D. , (2017) , Which pass is better?” Novel approaches to assess passing effectiveness in elite soccer, Human Movement Science 55: , 172–181. |
121 | Ruiz, H. , Power, P. , Wei, X. and Lucey, P. , 2017, August. “The Leicester City Fairytale?” Utilizing New Soccer Analytics Tools to Compare Performance in the 15/16 & 16/17 EPL Seasons. In Proceedings of the 23rd ACM SIGKDD International Conference on Knowledge Discovery and Data Mining (pp. 1991–2000). |
122 | Rusu, A. , Stoica, D. , & Burns, E. , (2011) , July. Analyzing soccer goalkeeper performance using a metaphor-based visualization. In 2011 15th International Conference on Information Visualisation (pp. 194–199). IEEE. |
123 | Saed, S. , 2020, Fortnite and FIFA 19 were 2019’s top digital earners –report. Available at: https://www.vg247.com/2020/01/03/fortnite-fifa-19-top-digital-revenue-2019-report/#: :text=Free%2Dto%2Dplay%20games%20dominated,on%20top%2C%20with%20%24786%20million. |
124 | Sarmento, H. , Marcelino, R. , Anguera, M.T. , Campaniço, J. , Matos, N. , & Leitão, J. C. , (2014) , Match analysis in football: a systematic review, Journal of Sports Sciences 32: (20), 1831–1843. |
125 | SoFIFA, 2020, Players. Available at: http://sofifa.com/players/. |
126 | StatDNA. 2020, Available at: https://www.statdna.com/ |
127 | StatsBomb. 2020, Available at: https://statsbomb.com/ |
128 | Stats Perform. 2020, Available at: https://www.statsperform.com/. |
129 | Poli, R. , Ravenel, L. , & Besson, R. , 2019, Financial analysis of the transfer market in the big-5 European leagues (2010.2019), CIES Football Observatory Monthly Report n°47 - September 2019. |
130 | Sæbø, O. D. , & Hvattum, L. M. , (2019) , Modelling the financialcontribution of soccer players to their clubs, Journal of Sports Analytics 5: (1), 23–34. |
131 | Sarkar, S. , & Chakraborty, S. , (2018) , Pitch actions that distinguish high scoring teams: Findings from five European football leagues in, Journal of Sports Analytics 4: (1), 1–14. |
132 | Schultze, S. R. , & Wellbrock, C. M. , (2018) , A weighted plus/minus metric for individual soccer player performance, Journal of Sports Analytics 4: (2), 121–131. |
133 | Shin, J. , & Gasparyan, R. , (2014) , A novel way to soccer match prediction. Stanford University: Department of Computer Science. |
134 | Singh, N. , (2020) , A narrative review in sport analytics, International Journal of Management (IJM) 11: (6). |
135 | Slater, M. J. , Haslam, S. A. , & Steffens, N. K. , (2018) , Singing it for “us”: Team passion displayed during national anthems is associated with subsequent success, European Journal of Sport Science 18: (4), 541–549. |
136 | Spearman, W. , Basye, A. , Dick, G. , Hotovy, R. , & Pop, P. , 2017, March. Physics-based modeling of pass probabilities in soccer. In Proceeding of the 11th MIT Sloan Sports Analytics Conference. |
137 | Sportec Solutions. 2020, Available at: https://www.sportec-solutions.de/en/index.html |
138 | Stanojevic, R. , & Gyarmati, L. , (2016) , December. Towards data-driven football player assessment. In 2016 IEEE 16th International Conference on Data Mining Workshops (ICDMW) (pp. 167–172). IEEE. |
139 | Stein, M. , Janetzko, H. , Lamprecht, A. , Breitkreutz, T. , Zimmermann, P. , Goldlücke, B. , Schreck, T. , Andrienko, G. , Grossniklaus, M. , & Keim, D. A. , (2017) , Bring it to the pitch: Combining video and movement data to enhance team sport analysis, IEEE transactions on visualization and computer graphics 24: (1), pp. 13–22. |
140 | Szczepański, Ł. , & McHale, I. , 2016, Beyond completion rate: evaluating the passing ability of footballers, Journal of the Royal Statistical Society. Series A (Statistics in Society), pp. 513–533. |
141 | Tett, R. P. , Jackson, D. N. and Rothstein, M. , (1991) , Personality measures as predictors of job performance: A meta-analytic review, Personnel psychology 44: (4), pp. 703–742. |
142 | Thomas, S. , Reeves, C. , & Davies, S. , (2004) , An analysis of home advantage in the English Football Premiership, Perceptual and Motor skills 99: (3_suppl), pp. 1212–1216. |
143 | Tunaru, R. , Clark, E. , & Viney, H. , (2005) , An option pricingframework for valuation of football players, Review offinancial economics 14: (3-4), pp. 281–295. |
144 | Vroonen, R. , Decroos, T. , Van Haaren, J. , & Davis, J. , (2017) , Predicting the potential of professional soccer players. In Proceedings of the 4thWorkshop on Machine Learning and Data Mining for Sports Analytics (Vol. 1971, pp. 1–10). Springer. |
145 | Wakelam, E. , Davey, N. , Sun, Y. , Jefferies, A. , Alva, P. , & Hocking, A. , 2016, May. The Mining and Analysis of Data with Mixed Attribute Types. In Proceedings: IMMM 2016: Sixth International Conference on Advances in Information Mining and Management. IARIA. |
146 | Whitaker, G.A. , Silva, R. , & Edwards, D. , 2017, A Bayesian inference approach for determining player abilities in soccer. arXiv preprint arXiv:1710.00001. |
147 | WhoScored. 2020. http://www.whoscored.com/AboutUs |
148 | Williams, A. M. , & Reilly, T. , (2000) , Talent identification and development in soccer, Journal of Sports Sciences 18: (9), pp. 657–667. |
149 | Woods, C. T. , McKeown, I. , O’Sullivan, M. , Robertson, S. , & Davids, K. , (2020) , Theory to practice: performance preparation models in contemporary high-level sport guided by an ecological dynamics framework, Sports Medicine-Open 6: (1), 1–11. |
150 | Wyscout. 2020. Available at: https://wyscout.com/ |
151 | Yi, Q. , Jia, H. , Liu, H. , & Gómez, M. Á. , (2018) , Technicaldemands of different playing positions in the UEFA Champions League, International Journal of Performance Analysis in Sport 18: (6), 926–937. |
152 | Yue, Z. , Broich, H. , Seifriz, F. , & Mester, J. , (2008) , Mathematical analysis of a soccer game. Part I: Individual and collective behaviors, Studies in Applied Mathematics 121: (3), pp. 223–243. |
153 | Yue, Z. , Broich, H. , Seifriz, F. , J. , & Mester, . , (2008) , Mathematical analysis of a soccer game. Part II: Energy, spectral, and correlation analyses, Studies in Applied Mathematics 121: (3), pp. 245–261. |
154 | Zhang, P. , Beernaerts, J. , Zhang, L. , & Van de Weghe, N. , (2016) , Visual exploration of match performance based on football movement data using the continuous triangular model, Applied Geography 76: , pp. 1–13. |
155 | Zhou, C. , Zhang, S. , Lorenzo Calvo, A. , & Cui, Y. , (2018) , Chinese soccer association super league, 2012–2017: key performance indicators in balance games, International Journal of Performance Analysis in Sport 18: (4), 645–656. |
156 | Zuckerman, M. , (1992) , What is a factor and which factors are basic? Turtles all the way down, Personality and Individual Differences 13: (6), pp. 675–681. |