Configuration based on Industry 4.0 technologies as a step towards an affordable smart warehouse
Abstract
The rise of Industry 4.0, marked by its focus on digitalization and intelligent technologies, is redefining traditional warehouse operations within smart cities. This new era leverages tools like Artificial Intelligence (AI) and the Internet of Things (IoT) to create automated solutions that address the complex challenges faced by modern urban centers. While smart cities grapple with interconnected issues, traditional methods often fall short. Data-driven, technology-powered solutions offer a more efficient approach. In the field of logistics, innovative warehouse solutions are emerging to address the growing complexities of the supply chain. One major challenge faced by many small businesses is inaccurate inventory knowledge, leading to inefficient warehouse management. This research proposes a cost-effective solution that utilizes Industry 4.0 technologies, specifically AI and IoT, to optimize inventory control and reorder point forecasting. This approach aims to democratize warehouse efficiency in smart cities by making it accessible to businesses of all sizes. This paves the way for a “smarter” future, upgrading warehouses with intelligent yet affordable solutions.
1.Introduction
The digital revolution has been sweeping across various aspects of human life, and today’s electronic devices possess the capability for wireless identification and internet connectivity, facilitating the exchange of information and enable a variety of other functionalities [1] in different areas including industrial production systems. This convergence facilitates the realization of interconnected industrial manufacturing facilities, intelligent retail environments, next-generation healthcare systems, smart homes and cities, and wearable devices. Logistics and storage are the core of companies, therefore, its development and improvement intelligent are crucial for their survival and growth [13].
Automation, streamlines operations from picking and packing to inventory tracking, enabling logistics facilities to prepare to meet the demands of a fast-paced, interconnected world where data becomes the differentiating element. Warehouses serve as critical infrastructure within logistics networks, ensuring the secure storage and efficient distribution of goods, thereby facilitating a smooth and timely flow of products throughout the supply chain [8]. Many of them growing its function acting as fulfillment centers, consolidating, assembling and shipping goods.
Warehouses also are evolving beyond mere storage spaces into specialized data centers [21]. In this sense, inventory management is central, where the alignment of stock with demand, in addition to maintaining optimal and safe levels, are fundamental processes [19]. This transition emphasizes the paramount importance of precise inventory control. The absence of necessary products at the point of demand translates into economic losses due to both missed sales opportunities and disruptions in operational efficiency and productivity [4]. Ultimately, this has a deleterious effect on customer satisfaction levels.
So, this tracking of inventory from the time of its manufacture to distribution is one of the logistics activities where there are the most possibilities of reducing costs for companies [8]. Particularly in warehouses it is possible to increase efficiency in processes, monitoring key performance indicators (KPIs) to assess the impact of technology investments and identify areas for further optimization (Fig. 1). In this competitive context, the inventory management methodology as well as choosing the appropriate inventory management software is key in achieving optimal inventory [7].
Fig. 1.
Inventory management.
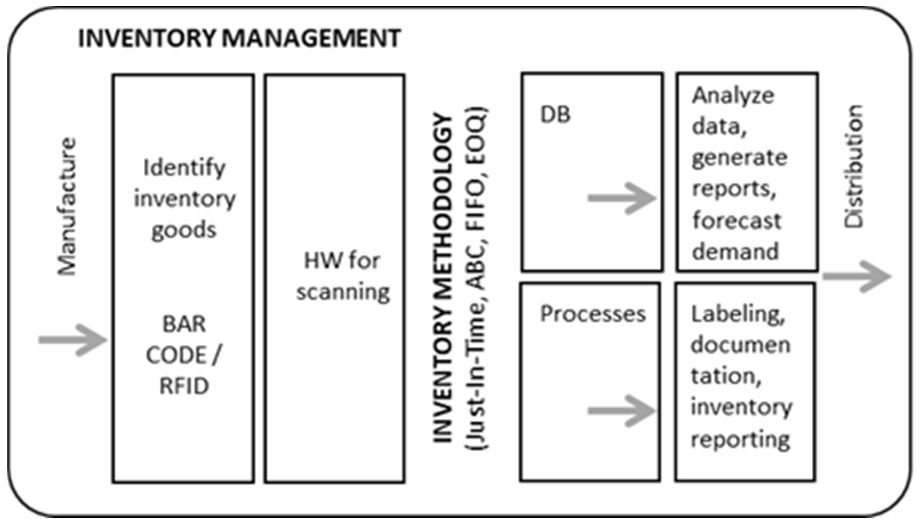
The IoT fosters a paradigm shift by enabling physical objects to communicate, exchange, and store information. This intricate network leverages communication channels, databases, and internet protocols collectively known as an IoT platform. AI serves as a critical driver for the IoT, empowering it to analyze and interpret the vast amount of data collected, ultimately unlocking its full potential [1].
1.1.Warehouse control and management systems
Inventory management is the process of ordering, handling, storing, and using a company’s non-capitalized assets, also known as its inventory. Inventory a complete list of items such as products, materials, or supplies that are stored in a warehouse before they are produced, shipped, or sold [23]. An inventory system is the set of policies and controls that monitors inventory levels and determines what levels should be maintained, when the inventory should be replenished, and what size the order should be, providing in turn the organizational and operational policies to maintain and track products in stock [11]. In an inventory system with continuous review, like Economic Order Quantity (EOQ) inventory methodology, the optimal inventory size is the one that minimizes its costs. EOQ, represents the ideal quantity of units a company should purchase to meet demand while minimizing inventory costs, in its formula, the specific point R, at which an order will be placed, is determined, as well as its size Q. The order point R is always a specific number of units. An order of size Q is placed when available inventory, currently in stock or on order, reaches point R [21].
Inventory control systems are technological solutions that integrate all aspects of an organization’s inventory tasks [20]. Modern warehouses use a warehouse management system (WMS) that can coexist with appropriate and functional associated systems for information exchange. It is important to highlight that the WMS is responsible for executing all the movements carried out in the warehouse, and must be compatible with other systems [16], which is why it is necessary for the implementation of technical automation solutions in warehouse operations, providing the technical framework for the connectivity of these technologies and supporting the day-to-day business in a warehouse [9], also facilitating its global management by allowing the accumulation of all operations derived from its processes, being the main source of concentration of information regarding its flows [5].
This paper addresses the challenges faced specially by small and medium-sized businesses (SMBs), which are the backbone of most economies, in managing their warehouses effectively through an automated solution that streamlines inventory control and demand forecasting, enabling real-time inventory accuracy and transforming the warehouse into a strategic asset that drives logistics efficiency and competitive advantage.
2.Background and related work
The fourth industrial revolution, known as Industry 4.0, basically consists of the symbiosis between production methods and Information and Communication Technologies (ICT), offering a range of possibilities in the automation of processes, especially those that, by their nature, present incremental information, and changes of adaptation [13]. It is the future of production, where innovation and advanced technology come together to create a more efficient, connected, and smart world [13]. The IoT represents a significant technological convergence, encompassing embedded systems, wireless sensor networks, control systems, and automation [1].
So, the increase in globally connected business activities raises complexity within industry networks; volatile demand and personalized products influence planning and production processes [2]. Therefore, efficient Supply Chain Management (SCM) is essential for any business. The objective of SCM is to guarantee adequate interactions of logistical elements so that the supply chain presents an optimal flow of products and information that allows for cost reduction and an increase in customer satisfaction [3], the implementation of technologically enhanced workflows fosters the optimized utilization of equipment, labor, and space within warehouses.
To maintain competitiveness in the modern business landscape, logistics systems must strive for interconnected information, optimized time and resource utilization, and continuous investment in innovation and development [4]. This aligns with the core principles of so-called Logistics 4.0, also known as smart logistics. Building upon the foundation of traditional logistics, Logistics 4.0 integrates advanced technologies to establish a highly automated, interconnected, and data-driven supply chain ecosystem. Within this ecosystem, ensuring product visibility is top [5].
Furthermore, logistics activities must be redesigned within the context of business architecture, fostering the development of intelligent logistics services focused on maximizing the aggregate output through the optimization of inter-component interactions. Seamless real-time data integration in IoT-based warehouses remains a main challenge, often out of reach due to a fundamental conflict between the fragmented nature of the current technological landscape and the holistic, unified vision of the future supply chain [20].
To ensure the optimal utilization of the warehouse component, a comprehensive analysis must be conducted for each enterprise attaining own resources and capabilities. This analysis will subsequently determine the most efficacious management and utilization methods [3]. By accurately tracking inventory levels, logistics providers can ensure they have the right amount of stock to fulfill customer orders promptly preventing stockouts [6].
Currently, two different types of inventory control systems are generally available: perpetual and periodic [21], which are mainly supported by the extended types of system inventory control: barcode and radio frequency identification (RFID) [18], these technologies enable tracking and recording actions [3], but RFID utilizes radio waves to read data from tags remotely, facilitating faster and more efficient registration and tracking compared to barcodes.
In a comparative analysis of their effectiveness for controlling logistical movements of goods, RFID technology offers a significant advantage. While both barcodes and RFID enable product tracking and history recording, RFID boasts a notably lower error rate of only 1%. This translates to enhanced data accuracy and reliability within the logistical workflow [24].
So, an affordable solution for a smart warehouse must allow obtaining, sharing, and analyzing information in real-time about the different activities in a warehouse, RFID is a valuable tool for this purpose enhancing supply chain logistics. Additionally, leveraging the power of the IoT technology allows objects to communicate and exchange data seamlessly through interconnected platforms, databases, and communication channels, further enhancing data flow and visibility [3].
By analyzing warehouse data, repetitive tasks suitable for automation can be identified and prioritized [13], which ultimately improves efficiency and contributes to a more agile and productive environment. That is, determining the maximum, minimum and reorder point appropriate to both the market and production needs and the investment of money in inventories to improve their performance [5], in addition to the data also serving to evaluate processes and find where the most value is added, especially in a low-cost technological implementation.
Thus, technologies for warehouse automation, due to the complexity of warehouse operations, must be focused on simplifying the response to one of the main challenges in inventory management, which is keeping product information updated in real time [12], by offering a greater degree of visibility and control, this approach goes beyond simply providing information. It empowers organizations to transform asset management into a dynamic and efficient process. This translates to optimized productivity and efficiency across the entire supply chain, leading to several key advantages like enhanced decision-making.
2.1.State of technique
The fiercely competitive landscape presents unique challenges for SMBs that arises to streamline warehouse performance given that demand is uncertain, and it can be difficult to predict demand accurately, especially for new products or products with historical data that is only available for a few months. Rapid growth, trends, and seasonality can also impact demand and lead times. This can translate into significant inventory management problems, such as excess or shortage of stock, high storage costs, and lost sales opportunities.
The efficacy of inventory management stands as a pivotal factor in meeting customer demands, curtailing costs, and preserving profitability [11]. Generally, the inventory system is carried out through a digital automation process, in most cases through a WMS, however, more specific systems are added to this to improve control over warehouse operations [16]. Where demand and budget allow, sophisticated software, robotics and automation are having a groundbreaking impact on logistics [21].
As previously stated, IoT refers to the interconnection of everyday objects through the Internet infrastructure [2]. In the context of inventory management, Industrial IoT (IIoT), can have a profound impact, facilitating better resource allocation and utilization. By seamlessly connecting RFID tracking devices, it empowers real-time inventory tracking and enhances product identification accuracy, ultimately leading to improved operational efficiency.
AI has demonstrated remarkable adaptability within the ever-evolving technological landscape. This is evidenced by the significant advancements in both technical complexity and the breadth of tasks AI can perform [19]. Moreover, its applicability has demonstrably expanded.
Within an automated warehouse environment, AI algorithms perform in-depth analyses on data acquired by IIoT sensors embedded in items. This analysis enables the identification of recurring patterns and trends within the data to generate forecasts of future product requirements. This capability facilitates proactive inventory management, ultimately influencing production planning optimizations.
Through the adept identification of patterns and trends hidden within vast data sets, AI demonstrably enhances the accuracy of demand forecasting, since supply chains often suffer from excessive inventory and stockout costs due to inaccurate demand forecasting [22]. Inventory position is defined as the quantity on hand plus the quantity ordered minus backlogged orders [4]. The solution for a fixed order quantity model may stipulate when the demand for the product is constant and uniform throughout the period, and the delivery time and the price per unit of the product is constant, factors that are considered for meticulously adjust inventory levels.
The rise of smart warehouses, facilitated by a diverse array of warehouse automation technologies, directly addresses another critical challenge in inventory management: maintaining real-time access to accurate product information within the warehouse. This convergence of AI and Industrial Internet of Things (AIIoT) presents a potent solution, fostering a paradigm shift towards proficient and optimized inventory management practices. This sensor network gathers a comprehensive range of data crucial for informed decision-making. Leveraging AI, the system analyzes parameters like product location, environmental conditions, and equipment performance metrics, enabling intelligent optimization of warehouse operations [17].
Within smart cities, the strategic integration of advanced technologies and interconnected systems fosters a data-driven decision-making environment. This extends to the realm of warehouse operations, enabling them to achieve unprecedented efficiency and precision in inventory management and tasks, ultimately optimizing overall process flows as shown in Fig. 2, empowering businesses to leverage real-time data for enhanced efficiency.
Fig. 2.
The warehouse flows that feed systems.
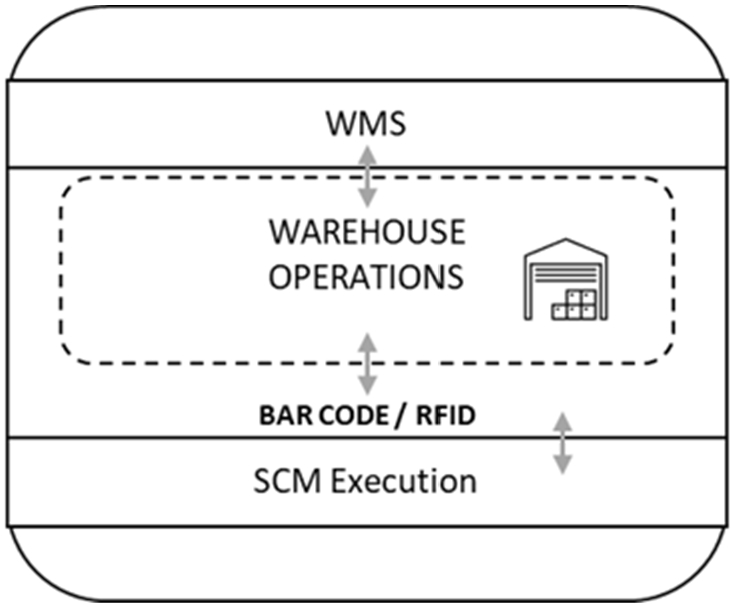
Warehouse automation encompasses the process of automating inventory movement with minimal human intervention. This automation can be achieved through various means, not necessarily limited to physical robots. In many cases, it simply involves leveraging software solutions to streamline and replace manual tasks associated with inventory management [9]. However, the high costs associated with the commercial systems limit its accessibility, motivating innovative low-cost solutions aimed primarily at automating the WMS, using software and data analysis to optimize operations [4].
The level of automation within a warehouse directly correlates with the standardization of goods movement [9]. Highly homogeneous goods with narrow tolerance levels are ideal for extensive automation. This maximum degree of automation is achieved when a central computer manages all movements through a network of fully automated equipment. This necessitates the implementation of such equipment alongside the programming of comprehensive loading/unloading plans for the storage system [4].
Effective automation initiatives necessitate the synergistic integration of a diverse technological landscape. In this context, an AIIoT solution facilitates the real-time acquisition of data through a network of interconnected sensors, this hybridized approach presents a transformative solution with the potential to revolutionize diverse sectors and unlock unprecedented opportunities for both businesses and individuals.
Therefore, this project assesses the implementation of a reference model based on free software platforms focused on demand forecasting, emphasizing the optimization of the trade-off between inventory management costs and the maintenance of sufficient stock levels to satisfy customer demand and avoid stockout scenarios.
3.Experimental development
As mentioned, this paper investigates the synergy between low-cost enabling technologies for the realization of a cost-optimized intelligent warehouse system. The studies reviewed emphasizes the potential for improved quality control through additional sensors controlling by IoT [15] also some findings give a clear picture that RFID can deliver more rapid scanning times than barcode scanning [24]. Consequently, this optimization translates into the effective minimization of superfluous costs associated with overstocking or understocking.
3.1.System design
The proposed solution leverages AIIoT functionalities. The AI component ingests both real-time and historical data from existing WMS. This data is then utilized to dynamically update inventory reports and KPIs. Furthermore, by incorporating a demand forecasting module, this predictive analytics framework expands its capabilities to project future sales requirements and optimal safety stock levels [25]. This approach facilitates the strategic deployment of inventory across designated storage locations within the warehouse facility.
Within the IoT, interoperability ensures seamless communication and data exchange between devices, irrespective of their manufacturer or brand [15]. This capability facilitates the aggregation of data and access to a wider range of functionalities from a unified platform. The underlying protocols significantly impact the overall functionality and efficacy of data transmission across the internet. These protocols, which are often dependent on the specific system architecture, can sometimes incorporate features like network interface level address resolution and auto-configuration [14].
To ensure the successful development and construction of the proposed system, a phased approach is recommended. This methodology entails the strategic subdivision of the project into distinct stages, each with clearly defined deliverables. These stages typically encompass automation configuration, solution delivery, and architectural design. This phased approach fosters improved project management, facilitates risk mitigation, and allows for iterative refinement throughout the development lifecycle.
This optimization prioritizes customer satisfaction by enabling the fulfillment of projected demand through the utilization of predictive analytics.
3.2.Development phases
Optimizing warehouse operational management necessitates a holistic analysis of its constituent sub-processes. These interdependent sub-processes form a critical chain, requiring a thorough evaluation to identify bottlenecks and inefficiencies. This analysis should delve into the specific needs and objectives of the target warehouse. By doing so, it facilitates the identification of improvement opportunities and the subsequent definition of relevant KPIs that directly align with the desired operational outcomes [16].
Different types of warehouses have their own storage allocation mechanisms based on their needs and requirements [10]. To adequately predict product needs and generate ideal inventory policies, the integration of different technologies that allow identification, registration of operations and traceability is required.
The system must allow parameterizing the relevant information for the correct management of the warehouse as a smart unit, presenting a series of reports considering the presenting an overview about specific items and storage units and their availability in inventory [16], an appreciation of inventory valuation that provide historical information on the value of inventory inputs and outputs over time, and the age composition of the selected products in the inventory in terms of quantity and value.
To develop the forecasting algorithm, the value of the coefficient of determination (
Finally, the inventory forecasting solution will be integrated into the WMS, or the system intended for inventory control. Real-time and remote data exchange will be enabled, empowering improved inventory management practices.
3.3.System architecture
Implementing an automatic inventory control system requires complementing the WMS with a product tracking system in the warehouse. In addition, the aim is to integrate the data into a centralized platform for processing and analysis, since large volumes of data are managed in this core, so an Artificial Neural Network (ANN) is applied for the analysis and generation of reports on the status of the data inventory, allowing, among other actions, optimize inventory levels to meet demand without overstocking setting the reorder point of certain items based on the estimate [22]. The application front end must be compatible with complementary applications that allow inventory to be always tracked as well as with mobile devices.
The proposed architecture for the prototype (Fig. 3) consists of tracking merchandise inventoried in a warehouse using a development platform with a Particle Photon microcontroller unit (MCU), in addition to RFID tags and sensors, mobile devices, with complementary cloud applications.
The demand forecasting algorithm leverages historical data encompassing sales figures, promotional events, and market trend indicators. This comprehensive data analysis enables the generation of accurate forecasts, empowering proactive inventory management decisions. These decisions include the determination of optimal order quantities and product types required to maintain minimum stock levels. Additionally, cloud-based technologies facilitate the accessibility and deployment of a wider range of technological solutions, further augmenting the capabilities of the forecasting algorithm.
Fig. 3.
Warehouse automation’s block diagram.
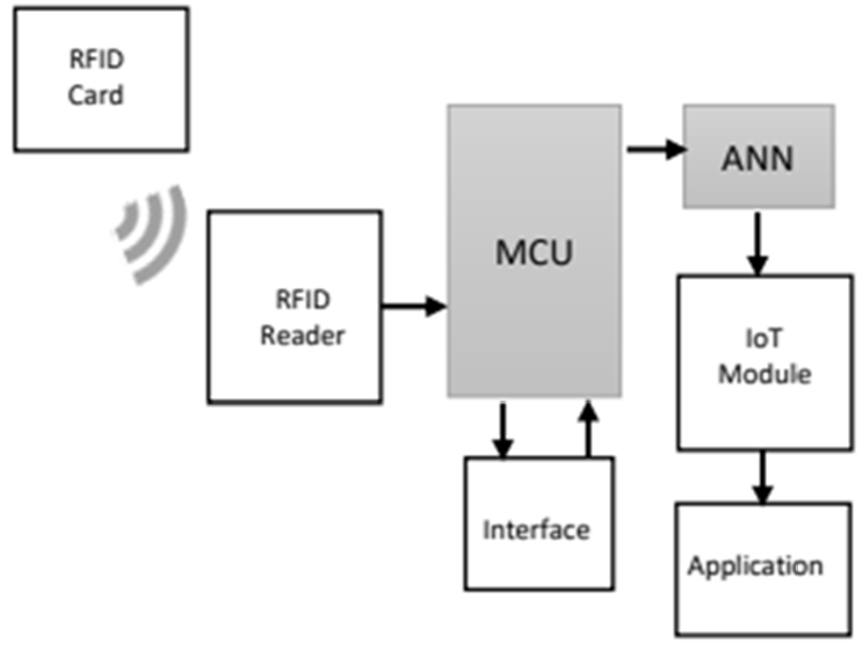
Inventory data patterns can be detected and provide valuable information on products that have a higher turnover, as well as those whose demand is seasonal, allowing the optimization of storage space and analyzing supply chain data in its entirety joint identifying bottlenecks and other issues that could affect the availability of products in inventory.
In this way, goods into, within, and out of warehouse are detected by the RFID sensor (Fig. 4) and all events are documented within a designated log. This log is then processed by the console, which stores the data in a database. The EOQ method is subsequently employed to analyze the information and generate calculations, obtaining data on the available inventory, which is monitored automatically using the MCU (Fig. 5), until the reorder point – demand during delivery time.
Fig. 4.
Prototype of the RFID sensor connected to the MCU.
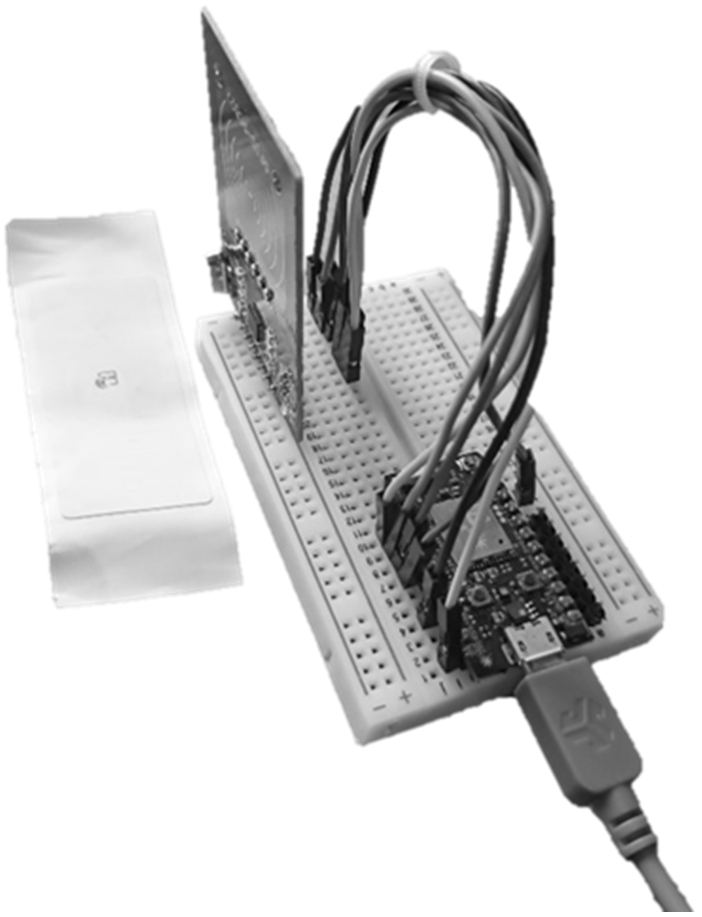
Fig. 5.
MCU and RFID reader connection electrical diagram.
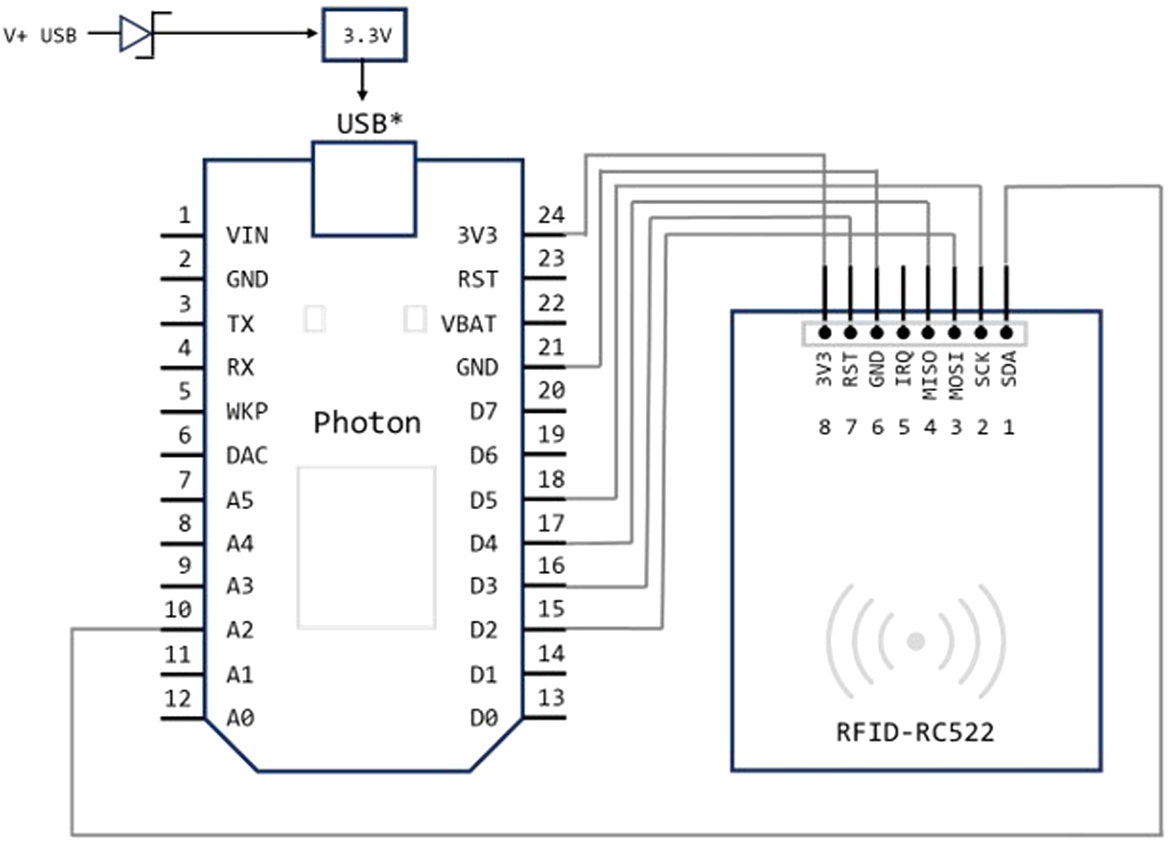
The model undergoes a normalization process to establish a consistent demand baseline. Leveraging this normalized data, the AI then predicts future demand with the objective of minimizing total costs across the supply chain [18]. This cost minimization incorporates both inventories holding costs and order preparation/placement costs. Automation within this specific segment of the supply chain can be initiated through the implementation or enhancement of a WMS. By placing a strategic focus on data collection and meticulous inventory control, such a WMS improved is expected to generate significant economic benefits.
When planning to implement such a solution for asset tracking and management in the warehouse, it is essential to consider data security and privacy concerns. Therefore, it is important to take these concerns into account and choose the best security measures; the platforms used allow you to configure firewalls, user authentication and data encryption, to protect the IoT infrastructure.
4.Results
As previously mentioned, an automation process in the warehouse must contemplate automating loading and unloading processes, which represent inputs and outputs of the warehouse, in addition to the storage process itself. Sensors and RFID tags connected to the centralized network can automatically detect when a product has entered or left, updating inventory levels in real-time and predict when inventory need to be replenished.
It is crucial to acknowledge the pivotal role of inventory analysis in providing critical data as input for product management. This data directly impacts cost reduction and operational improvements throughout the supply chain. Leveraging the insights gleaned from inventory analysis, a comprehensive analysis of demand-related data can now be conducted. This in-depth demand analysis facilitates the optimization of inventory levels and the subsequent refinement of planning strategies across the entire supply chain. The core objective of robust forecasting models is to mitigate uncertainty and empower data-driven decision-making, moving beyond reliance solely on intuition. Comparative evaluations between implemented ANN forecasts and traditional manual forecasting methods indicate that ANNs can potentially improve prediction accuracy [24]. Furthermore, by aligning with the EOQ method, these forecasts can effectively prevent stockouts.
The widespread adoption of smart warehouses represents an inevitable future trajectory. While technological advancements have rendered the necessary technology readily attainable, the successful implementation hinges upon the establishment of standardized protocols, universal compatibility, and a robust trust infrastructure. While individual enterprises may possess the capacity to implement smart solutions within their own isolated environments, broader realization necessitates a comprehensive integration with the surrounding economic ecosystem [25].
Considering that smart logistics and smart cities do not serve themselves but rather stakeholders, it is necessary to create solutions like the modelled that allows turning the warehouse into an intelligent unit, with reduced investment and no hidden costs, giving above all visibility over inventory at any time for decision-making.
4.1.Results analysis
To implement the proposed architecture in the WMS, in the first instance modelling was carried out as an information system connected to several internal and external systems, developing a prototype where the different hardware modules interact with the specific software, which allows the integration of an IIoT system.
In the warehouse case study, the data is integrated by detecting RFID tags generating the receipt of goods (Fig. 6), which are added to a test database. The data normalized is stored in a separate repository where it is available and ready for exchange.
Fig. 6.
RFID card reading sequence using a Particle Photon MCU.
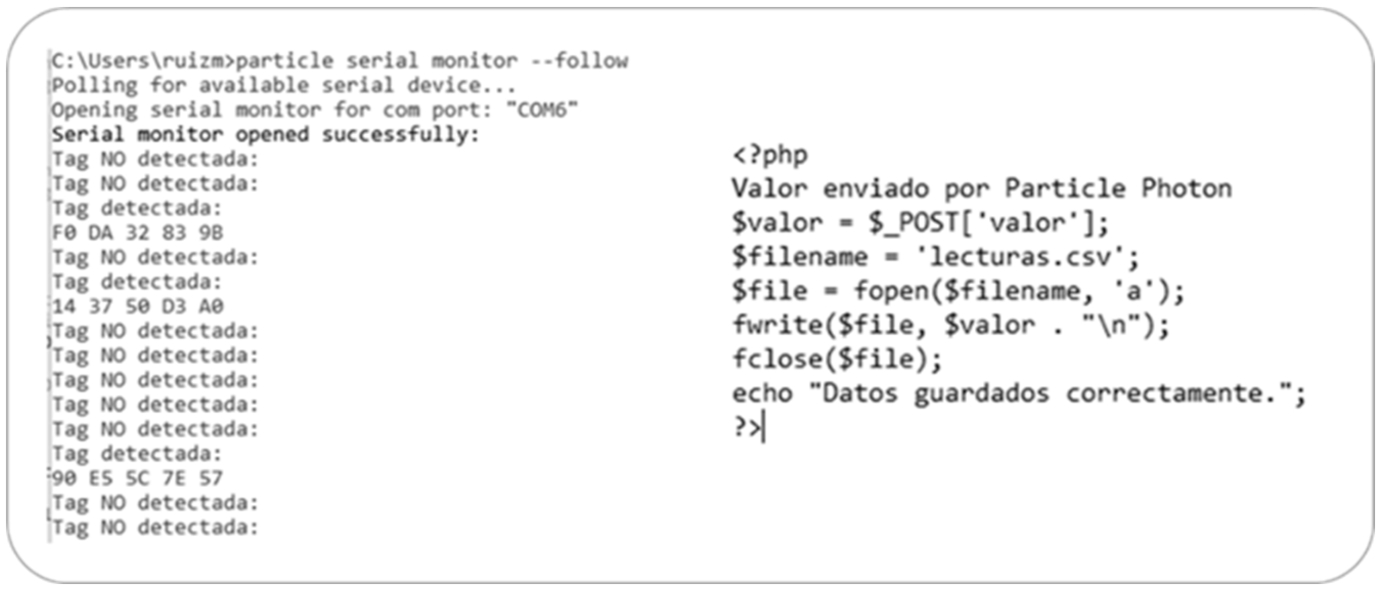
A process was implemented to migrate the data from the visualization console through a connection with the database for registration, validating its integrity and consistency. It is necessary to ensure that the transfer and storage process complies with the best security and access control practices in accordance with institutional policies.
The historical data is based on the classification of data from different time ranges that constitutes the ingest for the forecast model. In this way the data stored in the database also can be displayed raw to allow visibility of the data and movement of goods in the warehouse. Subsequently, an algorithm was designed for the forecasting of inventory needs (Fig. 7), which performs preprocessing on the data by dividing it into a training set and a test set, which depends on the size, complexity, and availability of the inventory data.
Fig. 7.
Example of an ANN algorithm for inventory forecasting in Python.
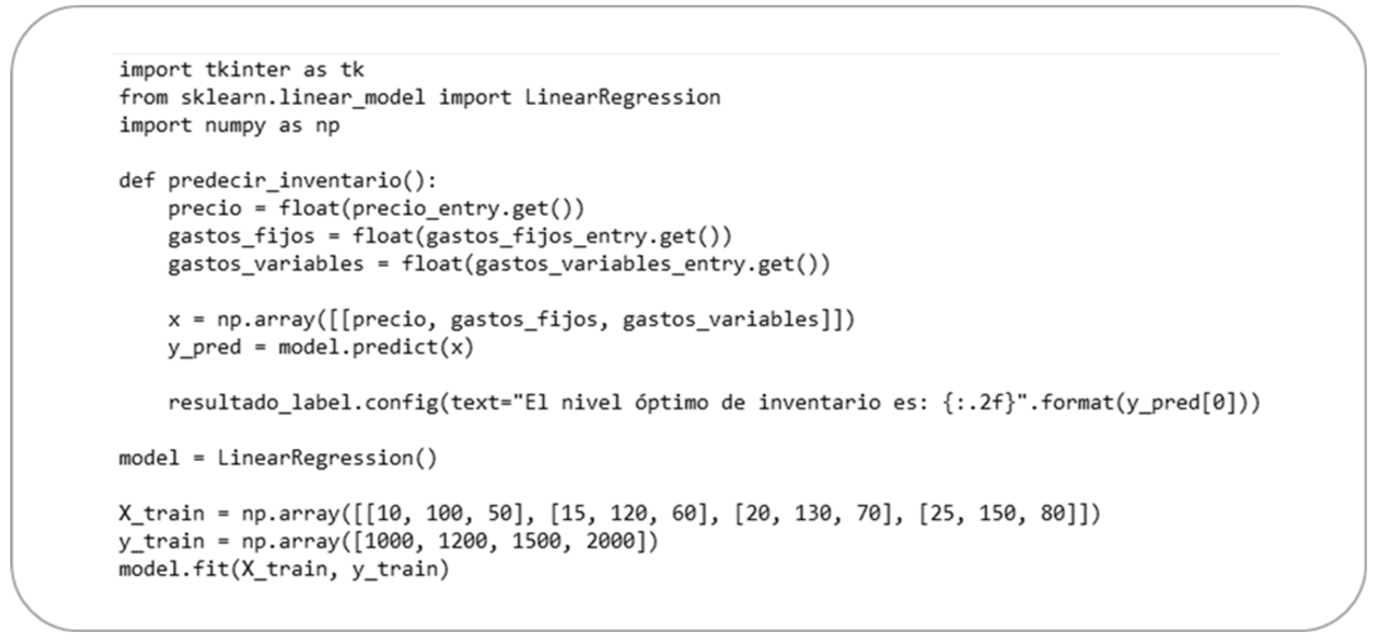
It is recommended to have a training set large enough to capture the variability and important characteristics of the data. In addition, it is also important to have a suitable test set to evaluate the generalization ability of the model after training.
The efficiency of the solution is measured in terms of time and accuracy with the prototype tests in the proposed architecture, it is important to note that a manual forecast of inventory reorder through an EOQ method can be efficient and relatively simple to implement in situations where the demand and costs are constant and predictable, helping minimize the total ordering cost incurred throughout the year. However, in more dynamic and complex environments, they could use other predictive models, in this sense, AI has the advantage of being able to better handle uncertainty and changing patterns to analyze data and predict what will happen to inventory in the immediate future.
5.Conclusions
Inventory management within the warehouse is a basic activity that often goes unnoticed in terms of its importance and can also be complicated if the company does not have adequate tools to monitor, maintain and use the assets, having the specific product organized and at the right time can be the difference in customer preference.
As supply chains expand, the SCM tasks become even more difficult, as companies need to organize manufacturing, distribution, and retail. Regardless of a warehouse’s unique setup, consistent measurement of KPIs and inventory levels empowers informed decision-making through comprehensive historical data, real-time insights, and even future predictions.
This research leverages automation to offer an affordable solution for inventory tracking. By optimizing the reorder point through data-driven insights from various sources, it empowers better management. Introducing a new system presents an opportunity to streamline operations and enhance efficiency. To capitalize on these advantages, organizations must demonstrate a commitment to transformation by strategically aligning their operational frameworks and investing in the modernization of their asset base.
Smart warehouses rely on real-time data collection and analysis to inform decision-making across various aspects, including inventory management, order fulfillment, and resource allocation. Without a comprehensive smart system, this data wouldn’t be readily available or actionable, hindering the ability to make informed choices, associated with customer retention, but also search of their satisfaction.
Leveraging AIIoT ecosystem for inventory control and demand prediction, coupled with optimized inventory management techniques, minimizes warehouse costs. Automating the process of obtaining data from different sources to be collected, measured, and detailed in real-time, as well as providing the possibility of aggregating them to present timely information in different formats to make predictions, is an innovative and effective strategy to optimize reordering. Also, the solution presented allows for greater automation and accuracy in tracking inventory levels, due to RFID tags can continuously transmit data across the warehouse infrastructure, resulting in generating solutions for traceability and monitoring, in addition to greater efficiency and cost reduction in the warehouse, and therefore across the supply chain.
This research delves into the hybridization of the physical and digital realms, targeting a reduction in both manual workload and human error within asset management. Furthermore, it facilitates the configuration of novel business automation models and potentially lays the groundwork for bridging the technological divide. This approach aligns with the evolving industrial landscape characterized by rapid shifts in consumer behavior, necessitating a corporate focus on high-impact initiatives that deliver cost optimization, efficiency gains, and ultimately, a secure and sustainable competitive advantage.
Implementing the proposed automatic warehouse inventory control system led to a new paradigm in warehouse management and task automation for inventory control, it is a step towards low-cost automation that can significantly improve efficiency and accuracy in inventory management for SMBs, this breakthrough mitigates economic barriers [17], granting them access to affordable technologies that empower autonomous asset management within smart warehouses fostering their participation in the digital revolution, and as a smart city participant, unlocking a world of opportunity and growth.
Evolving market demands and ever-shifting business needs necessitate a paradigm shift in warehouse management. This paper explores the concept of the “smart warehouse,” a system characterized by its adaptability and scalability in any size of business. Such a system seamlessly integrates with emerging technologies, ensuring a warehouse’s ability to meet dynamic market demands and maintain a competitive edge. This holistic approach translates into significant cost savings and the maximization of warehouse capacity. In today’s landscape, efficiency and productivity reign supreme.
Acknowledgements
José E. Ruiz is a doctoral student from Programa de Doctorado en Ciencias de la Computación, Universidad Autónoma del Estado de México (UAEMéx) and received fellowship from CONAHCYT, Mexico (CVU. 619005).
Conflict of interest
The authors have no conflict of interest to report.
References
[1] | K. Almassar and M. Khasawneh, Using IoT and AI to replenish household food supplies: A systematic review, Journal of Smart Cities and Society 3: (1) ((2024) ), 23–62. doi:10.3233/SCS-230022. |
[2] | C. Bartodziej, The Concept Industry 4.0, in: The Concept Industry 4.0, Springer, Wiesbaden, (2017) , pp. 27–50. doi:10.1007/978-3-658-16502-4_3. |
[3] | A. Correa, R. Gómez and J. Cano, Gestión de Almacenes y Tecnologías de la Información y Comunicación (TIC) [Warehouse Management and Information and Communication Technologies (ICT)]. Estudios Gerenciales. Universidad ICESI. Vol. 26 No. 117, (2010) . |
[4] | M. Escudero, Logística de almacenamiento [Storage logistics]. Almacenaje de mercancías. Madrid, España: Ediciones Paraninfo, (2014) . |
[5] | M. Ezzat, S. Kassem and E. Abd, Logistics 4.0: Definition and historical background, (2019) . doi:10.1109/NILES.2019.8909314. |
[6] | IBM, What is inventory management? IBM. [Online] Available from: https://www.ibm.com/topics/inventory-management#:~:text=Inventory%20management%2C%20a%20critical%20element,place%20at%20the%20right%20time. |
[7] | ILSMD, Inventory management methodologies. Integrated logistics services. (2021) . [Online] Available from: https://ilsmd.com/2021/08/04/inventory-management-methodologies/. |
[8] | R. Jacobs and R. Chase, Operations and Supply Chain Management: The Core, 4th edn, McGraw Hill, (2016) , pp. 353–399. |
[9] | A. Jenkins, Warehouse automation explained: Types, benefits & best practices. Oracle Netsuite, (2020) . [Online] Available from: https://www.netsuite.com/portal/resource/articles/inventory-management/warehouse-automation.shtml. |
[10] | M. Khan, N. Huda and U. Zaman, Smart warehouse management system: Architecture, real-time implementation and prototype design, Machines 10: (2) ((2022) ), 150. doi:10.3390/machines10020150. |
[11] | A. Kittisak, Challenges and strategies for inventory management in small and medium-sized cosmetic enterprises: A review, International Journal of Information Technology and Computer Science Applications 1: (2) ((2023) ), 71–77. doi:10.58776/ijitcsa.v1i2.30. |
[12] | Mecalux, Almacenes inteligentes: de la automatización al big data. Mecalux, (2020) . [Online] Available from: https://www.mecalux.com.mx/blog/almacenes-inteligentes. |
[13] | R. Medina and D. Toapanta, Sistematización teórica de la Industria 4.0 en el área de logística [theoretical systematization of Industry 4.0 in the logistics area], Revista Dilemas Contemporáneos: Educación, Política y Valores XI: (1) ((2023) ), 78. |
[14] | Microsoft Azure, IoT technologies and protocols. Microsoft Azure, (2023) . [Online] Available from: https://azure.microsoft.com/en-us/solutions/iot/iot-technology-protocols. |
[15] | N. Nagrale, V. Nagrale and A. Deshmukh, Iot based smart food grain warehouse, in: 2023 2nd International Conference on Paradigm Shifts in Communications Embedded Systems, Machine Learning and Signal Processing (PCEMS) 2nd International Conference on 1-5 Apr, (2023) . |
[16] | A. Nilsson and D. Merkle, Technical Solutions for Automation of Warehouse Operations and Their Implementation Challenges, Linnaeus University, Sweden, (2018) . |
[17] | G. Pérez and R. Sánchez, Logistics for production, distribution and trade, FAL Bulletin ((2019) ) 369, Santiago. Economic Commission for Latin America and the Caribbean (ECLAC). |
[18] | Red Hat, What is IIoT?, Red Hat. (2021) . Available from: https://www.redhat.com/en/topics/internet-of-things/what-is-iiot. |
[19] | G. Richards, Warehouse Management. Complete Guide to Improving Efficiency and Minimizing Costs in the Modern Warehouse. Editorial Rating, (2011) . |
[20] | C. Sahara and A. Aamer, Real-time data integration of an Internet-of-things-based smart warehouse: A case study, International Journal of Pervasive Computing and Communications 18: (5) ((2022) ), 622–644. doi:10.1108/IJPCC-08-2020-0113. |
[21] | B. Sani and B. Kingsman, Selecting the best periodic inventory control and demand forecasting methods for low demand items, The Journal of the Operational Research Society 48: (7) ((1997) ), 700–713. doi:10.1057/palgrave.jors.2600418. |
[22] | P. Umamaheswaran, F. Ganjeizadeh and H. Ghasib, Inventory management and cost reduction of supply chain processes using AI based time-series forecasting and ANN modeling, in: 29th International Conference on Flexible Automation and Intelligent Manufacturing (FAIM2019), Limerick, Ireland, June 24–28, (2019) . |
[23] | Veeqo, The complete guide for inventory management, Veeqo, (2020) . [Online] Available from: https://www.veeqo.com/inventory-management. |
[24] | G. White, G. Gardiner, G. Prabhakar and A. Abd, A comparison of barcoding and RFID technologies in practice, Journal of Information, Information Technology, and Organizations 2: ((2007) ). |
[25] | W. Zuchowski, The smart warehouse trend: Actual level of technology availability, LogForum. doi:10.17270/J.LOG.2022.702. |