Harvesting energy overview for sustainable wireless sensor networks
Abstract
Energy harvesting (EH) has emerged as a transformative research paradigm by converting ambient energy into electrical energy for natural and artificial applications. This paper explores the potential of EH in powering autonomous electronic devices facilitated by simplified processes to harness kinetic, solar, thermal, wind, and salinity gradients. Mainly, the focus lies on the applicability of these energy sources to small wireless automatic devices used in wireless sensor networks (WSNs). WSNs consist of cutting-edge sensors spatially distributed to monitor physical conditions and organize collected data at a central network location. Their pervasive existence enables efficient computing through sound resource management, interconnected via the internet and other high-tech innovations. This study evaluates EH developments to minimize resource utilization in WSNs, examining key features, proposed frameworks, and models. Furthermore, it reviews specific energy source productions utilized by WSNs. The feasibility of energy storage is also discussed, highlighting its potential for WSNs and paving the way for future directions in this field.
1.Introduction
Wireless Sensor Networks (WSNs) have proved to play a substantial role in the multi-hop wireless network testing market. This is because of its potential application in conservation and operational systems for safety and public fitness control. The architecture of communication protocols, localization, monitoring strategies, and power management are essential in this paradigm [52]. Internet of Things (IoT)-based hedge systems have demonstrated a positive effect on solar power generation. Existing algorithms, for example, localization [35], optimized clustering [59], and machine learning algorithms [25], focus on the edges for hedging, and solar radiation approaches to hedge the low radiation risk for solar investors are extensive. Prediction models based on edges availed a correlation coefficient of 0.917 and an R-squared value of 0.841. WSNs were inspired by technological emphases, such as conserving natural resources and changing them into energy. Characteristically, the WSN nodes are usually battery-powered. The system is considered “empty” when its power is exhausted; batteries can be replaced or recharged in minimal applications. The replacement or recharging process tends to be slow and costly and reduces network performance, but it is inevitable.
As a result, various methods have been suggested to delay battery capacity degradation, including power management and session-based activities in routing [9,69]. These approaches sometimes use low-current wireless transceiver techniques, the components of which can be turned off to save energy. In case the device reduces the power (like in power efficient) phase, the output of the device is significantly reduced versus when it is on [64]. Nevertheless, the device cannot relay or recognize the data packet while sleeping. The task loop completes in correlation to the time; the device is on and rests before it finally turns off. Using protocols running at a relatively low power level seems to be an acceptable explanation that accords with long-lasting WSNs.
Dependable IoT devices are required to generate correct data in several computations. The authors of this study presented an approach for characterizing IoT dependability measures. The reliability measurements are computed utilizing queuing theory. The theory entails response time, queue length, delay time or waiting time, and busy periods, among others. To account for the reliance of items in IoT contexts, the Markov Chain traits were used, and thus better reliability measures were obtained [71].
This approach leads to significant pitfalls; 1) there seems to be an apparent compromise between power consumption (reducing duty cycling) and information delay. 2) WSNs run with batteries, struggling to meet the demands of many new technologies requiring complex network computing and time-taking. 3) Leakage depletes the batteries even though they are rarely used. Recent studies on long-lasting WSNs take a vastly different approach, suggesting “constant” WSN performance through tandem energy harvesters utilizing battery packs and superconducting materials for power storage. WSN considering EH (WSNEH), based on energy harvesting, tends to result from imbuing WSN entities with the ability to collect power from numerous sources.
1.1.Research motivation
The utilization of ambient energy in smart cities serves as a means to power sustainable WSNs. The integration of technology and nature facilitates the operation of interconnected systems, hence facilitating the generation of data-driven insights for efficient resource management [36], minimizing environmental harm [31], and establishing a robust urban infrastructure. The adoption of energy harvesting technologies facilitates the advancement towards an environmentally sustainable, technologically advanced, and highly networked urban environment [10]. The EH uses various power sources, such as solar energy, air, mechanical stress, temperature fluctuations, and electromagnetic waves. The EH subsystems regularly supply and process power for future use, enabling the sensor node to last forever [1]. This study extends the dynamics of smart cities in depicting the current challenges and opportunities of the WSNEH besides the existing studies, assessing why it is necessary to rethink the architecture of the WSNs fundamentally. The review starts with the vital definitions, followed by the WSNEH node’s architecture, particularly its power system. The analysis of the different sources of energy available is also presented. Furthermore, a discussion on the classification of energy harvesting is examined.
The remainder of this study is structured as follows. In Section 2, a comprehensive overview of EH is presented. The third section illustrates the classification of EH techniques, including the EH based on radio frequency (RF), astrophysics-based EH, thermal-based EH, mechanical-based EH, and human-based EH. Meanwhile, the appropriation, inverse, and future directions are documented in Section 4. Regarding appropriation industrial conditions monitoring, operating isolated setups, wireless pressure sensors, and pipeline actuators are detailed. Inverse issues like alternative green energy options, collecting wasted energy, self-charging electronics, and drop-in battery replacement are demonstrated. Lastly, future research avenues are documented in the context of system integration, low power, wireless communications, smart cities, biological systems, and fluctuating prices. Finally, Section 5 concludes the article and describes future work.
2.Energy harvesting
Energy harvesting is widely described as converting ambient energy into electricity. We describe energy harvesting as “the on-demand, off-grid processing and storage of ambient energy.” The first concept deals with the energy transmission transducer system, which implies that energy needs to be transferred to electric power. This interpretation provides a detailed and comprehensive software-centric perspective. The transmitter is part of a complete power supply network for applications for which other energy sources are inaccessible or unacceptable [16].
Ambient energy comes in several forms: nuclear, biological, electrical, mechanical, and many more, as discussed [40]. Either of these power sources should be available for energy harvesting. Moreover, an opposite transducer must be in place to transform electricity. Power harvesting has been used for electricity generation without an external power source. Excavators require investment, and they are an excellent alternative when using conventional sources such as grid power or batteries becomes too expensive and impractical. On-demand electricity must be supplied by the energy harvester when required, not merely when it is available. Some form of power storage is usually the recommended ordering value for the requirement [34]. In principle, there are various forms of energy available for energy harvesting, such as radiation (light, electrical, cosmic, and electromagnetic), thermal, physical (potential, mechanical, dynamic, fluid), gravitational, chemical (capacitor, battery storage, coal power, phase transition), nuclear, gravitational (magnetization, movement), and electrical.
Sound cosmic radiation, atmospheric pressure variation, and solar atomic neutrinos are readily available but have almost no related energy. When other energy fields are accessible, the harvester relies exclusively on the location and the selection of feasible forms. The options are usually limited to only one or two appropriate for a particular area. Sunshine is a decent energy source with a high power density and correct consistency outdoors. Air can be suited to extensive facilities high enough to have direct air currents [34]. Optimal temperature and vibration differences are challenging to detect. Such lighting systems, like calculators, could provide adequate indoor electricity for low-power applications. Moreover, the device waves and temperature radiation can also be employed for industrial applications such as extreme temperatures and intense oscillations occurring at a predicted behavior.
Data security and information privacy remain key challenges in sensitive computations with IoT and sensors. The configuration state could be attacked or updated without privacy-preserving precautions. This often results in security issues and sensitive data loss. An ant colony optimization approach was suggested to tackle the security aspects. This was determined by the need to preserve secret and sensitive information on 6G IoT networks by implementing multiple objectives and utilizing transaction deletions. A set of deletion operations represents each ant in the population that may be used to conceal sensitive information [11]. Table 1 demonstrates the different energy source availability, illustrating its location and where it can be accessed.
Table 1
Availability of energy by location
Energy by location | Radiation | Wind | Sunlight | Artificial lighting | Human | Machine vibration | Gravitational | Thermal gradients |
Office or home | Yes | No | No | Yes | Yes | No | No | No |
Along the street | Yes | Yes | Yes | No | No | No | No | No |
Bridge | Yes | Yes | Yes | No | No | No | Yes | No |
Vehicles | Yes | Yes | Yes | No | No | Yes | No | Yes |
Station of the pumping | Yes | Yes | Yes | No | No | Yes | No | Yes |
Petrol rig | Yes | Yes | Yes | No | Yes | No | Yes | |
Battlefield | Yes | Yes | Yes | No | Yes | Yes | No | |
Sensing for remote locations | Yes | Yes | Yes | No | No | No | No | Yes |
Diverse types of systems have varying requirements for electricity. A pacemaker necessitates only around five joules to operate in one day. On the other hand, a desktop PC needs about five Mega Joules. Some systems operate continuously, and regular power consumption is much like the demand for immediate electricity. Contrarily, certain machines function occasionally. Anything that is on for 1 second and then off for 9 seconds has a ‘duty ratio’ (electronics) of 10%. Thus, the average requisite energy is only 10% of the total power demand. Whether or not a particular application uses EH depends on the knowledge of the energy consumption profile and its comparison with the available energy from extraction. Table 1 presents a description of the available energy for different locations. All areas in the table with “No” notation indicate that they remain subject to research since existing models have not explored them. Table 2 summarizes the different energy sources and their capacity [19].
Table 2
Available power sources and their vital summary
Source | Power capacity | Vital summary |
Cosmic raying | 0.1 μW (microwatt) | Negating transfer inefficiencies |
EM-ray radiation | 0.5 μW (microwatt) | 10 × 10 cm antenna 10 m under a 10 kV (kilowatt) transmission line |
The pressure of the blood | 15 μW (microwatt) | The estimate of the Southampton University Hospital |
The vibration of the computer | 05 mW (milliwatt) | Perpetuum PP27 |
Lighting indoor | 20 mW (microwatt) | Solar cell 5 × 5 cm |
Human electricity | 2 W (watt) | Winding a radio from Baygen |
Sensor node energy efficiency is critical to the long-term viability of IoT networks. When the number of live nodes is more significant, the converging rate compared to cost performance is faster, so there is more residual energy, the load is equally distributed, and the temperature produced by IoT nodes is lower. The energy consumption will be ideal. Within this research, we propose a hybrid WOA-MFO approach that optimizes the 500 criteria described above to find the best CH. In comparison with the existing algorithms, the proposed model is examined. The experiment’s findings indicate that the suggested model outperforms other models, such as load, temperature, energy, latency, cost performance, and total number of live nodes [29].
The primary goal of staying practical life estimation is to achieve maintenance at a cheap cost and resource conservation. In the field of prognostics, cutting-edge applications and the models’ findings have been outstanding. The proposed approach aims to improve recurrent neural network training to make better predictions. The results show that the improved design outperforms the current GRU and LSTM models [2]. The new model must demonstrate superior training efficiency, handling long-term dependencies, and capturing complex sequential patterns while minimizing vanishing gradient problems.
Throughout this work, the PCA-based random forest regression methodology is utilized to forecast the life of the battery of an IoT network. Missing values are filled in using the attribute mean during the preprocessing stage, normalization is done using the regular scalar method, and transformation is done using the technique of one-shot encoding. The PCA algorithm is then used for feature selection and dimensionality reduction. The data set’s reduced dimensions are then fed into the random forest regression algorithm. The obtained results support the superiority of the presented model. It is possible to assess the suggested model on a large-scale set of data in the future. Deep neural networks can also improve prediction [30].
2.1.Sensor node’s architecture with harvesting capabilities
In this subsection, an analysis of the nodes of the wireless sensor system is described. The significant components include (a) An energy harvester responsible for converting energy produced by the natural environment or a living organism into electric power. (b) The module extracts electric power from the harvester, saves it for immediate use, or supplies it to other components, (c) Housing power for the harvester, (d) microcontroller. (e) Data-transmitting radio transceiver, (f) sensor devices, (g) A/D (analog-to-digital) converter for digitizing the audio voltage produced by the sensor and making it accessible for further transmission to the microcontroller, and (i) memory for storing sensing data, application-related data, and code as demonstrated in Fig. 1.
Fig. 1.
Architectural design of a wireless node with harvesters of energy.
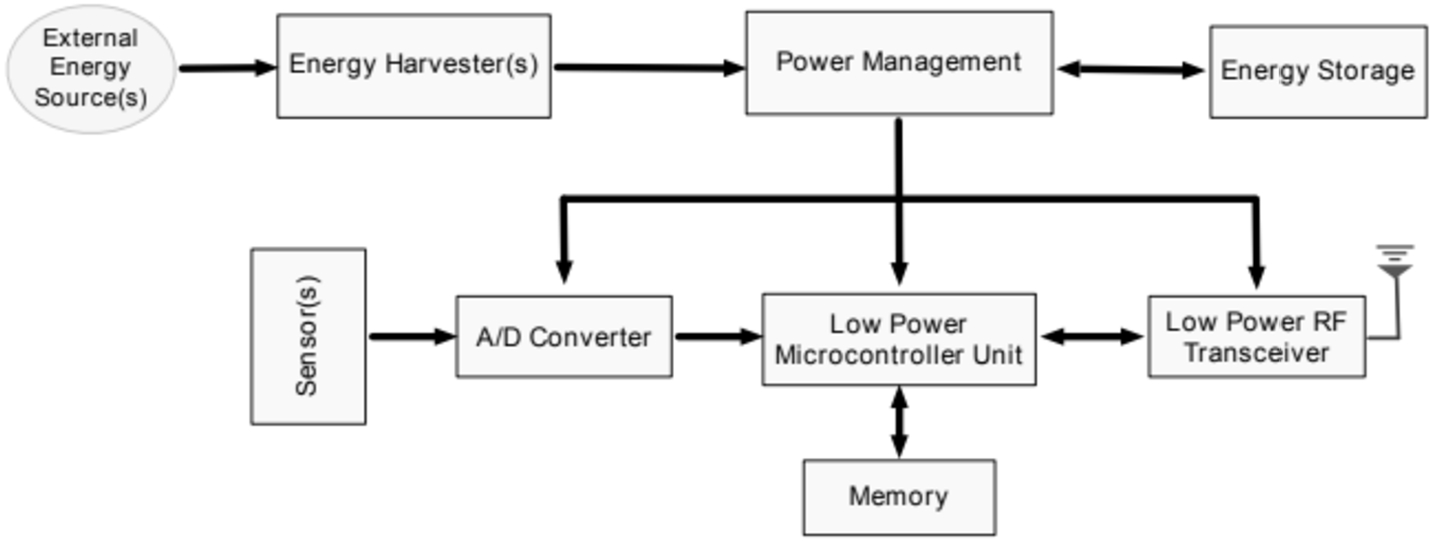
To convert the accessible energy from the atmosphere to electrical power, the power system needs one or more harvesters. The harvester gathers could transfer energy straight to the node or retain it for future usage. In specific applications, the sensor node may be directly powered by harvested electricity without storing energy (extract-use architecture) [51], although it is not a viable choice. A relatively more rational design allows the node to use the harvested energy directly and includes a portion of the storage that acts as a power source for storing and preserving the extracted energy. When the extraction exceeds the utilization, the buffer portion can preserve the surplus energy for future consumption. Figure 2 shows the general configuration of the wireless sensor node for the power module, including the power generation functionality.
The secondary battery packs and superconducting materials (ultra-capacitors) are two energy storage options. Supercapacitors are like condensers except those that generate exceptionally high power on a small scale. There are several advantages of powered batteries [7]; firstly, the supercapacitors can be powered and drained almost indefinitely, while the cumulative life of electrical and chemical batteries is less than 1000 cycles [63].
Fig. 2.
WSN energy subsystem design.
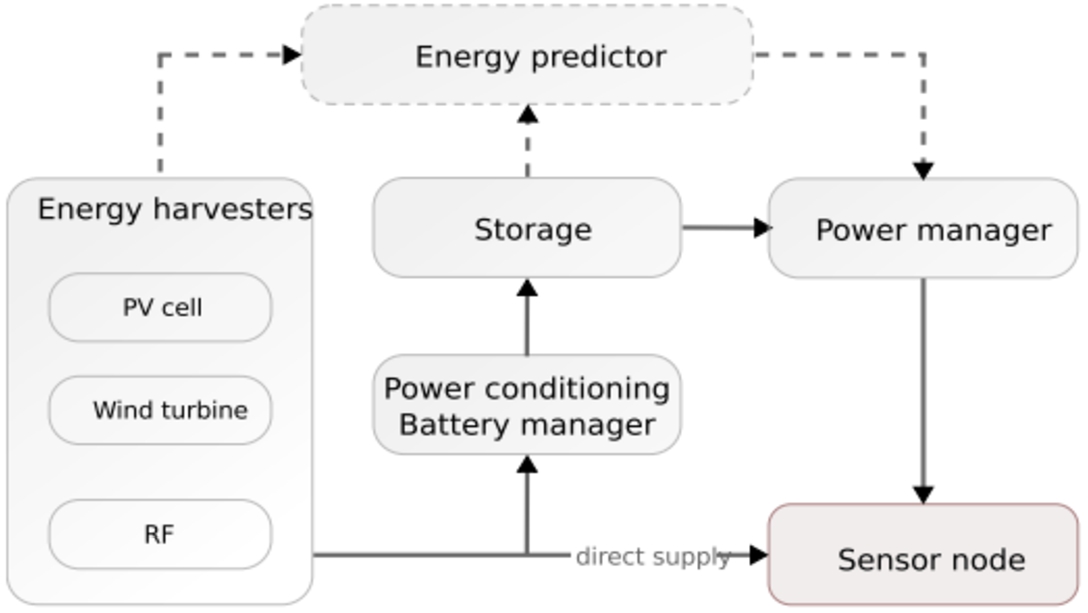
Besides, simple charging loops may power them efficiently, increasing the network’s complexity and not requiring deep discharge protection circuits. Secondly, there is more charge and additional unloading flexibility than rechargeable batteries. Moreover, decreasing the environmental issues associated with the disposal of batteries is an extra benefit. Figure 2 depicts the WSN energy subsystem design flow. Because of these characteristics, many mining systems use super condensers as energy storage on their own or in conjunction with batteries [65].
3.Classification of technique of energy harvesting
Various sources of energy have been discovered for the EH. Whether or not a particular source can provide enough power to the sensor node is one of the critical criteria for selecting an energy source. Usually, the voltage movement dissipates the energy, and the dissipation increases as the input-to-output ratio rises. Therefore, it is crucial to guarantee that the power is generated at the required current and voltage levels. The energy source is an energy storage device or a source adjusted to an optimum level of electricity. For instance, in radio frequency (RF), the required demand is met by increasing the source power.
Much electricity is produced in the photovoltaic (PV) cell area, resulting in a greater electricity rate. Nevertheless, in some situations, matching the sources or converting energy efficiently is impossible. For example, the energy derived from industrialized vibrations cannot be increased automatically without the effect of the vibrations on the system. Therefore, the harvesting system can be extended; many WSN applications allow nodes to be compact and thin. Researchers typically use the energy density metric to compare different strategies for extracting energy [37]. Therefore, designers should consider this exchange when selecting an appropriate energy-harvesting device for a specific application. The energy sources are divided into two broad classes, i.e., internal and external. Biological resources are readily available in the atmosphere at almost no cost. Nonetheless, secondary sources are intentionally used for energy storage purposes in environments.
Internal sources include those that are inherently generated within a system, such as the heat produced by the Earth’s core or the energy released during nuclear reactions. These sources are often long-lasting and stable but can be challenging to harness [56]. On the other hand, external sources encompass energy derived from external factors, like solar radiation, wind, or fossil fuels, and are more readily accessible and widely utilized. While external sources can be abundant, they may vary in availability and sustainability, posing challenges for energy production and management [42]. These categories are further subdivided, as described below.
3.1.Energy harvesting based on radio frequency
After conditioning, the received radio signals are transformed into DC voltage for RF-based energy processing. Depending on the specifications of the required application (e.g., weight, output, or voltage), RF signals can be converted to DC power through various methods, such as single-stage versus multistage. All factors affecting the amount of electricity produced are the source of electricity, including the antennas’ gain, the range between the target sources, and electrical efficiency. Usually, over an input power range of 100 m, the RF to DC exchange rate is between 50% and 75% [66]. Since rectifiers, which convert AC signals from RF waves into DC, are designed to maximize conversion efficiency, they are inherently limited by semiconductor characteristics and losses, which can account for the variation in efficiency within this range. There are two RF energy harvesting configurations: the sensor nodes can use two radios, one for RF harvesting and the other for communicating with other sensor nodes, or only one radio can be used for both. Providing a single WSN radio to reduce the program’s complexity and minimize the overall code size is better.
For the intentional sources of WSNs, a case point sinks to distribute energy, and the platform obligates the control to be terminated by the available power. The anticipated foundation can deliver power on a continuous, intended, or ultimatum basis and can supply power to stimulate the fully charged instrument nodes. The nodes can also predict other sensor nodes and provide the radio frequency and energy harvesting basis. The intermediate nodes operate regularly or intermittently as a power source; nevertheless, the nodes cannot usually be regulated because the continuous dissemination of records may not be significant for ideal wireless and sensor networks. Radiofrequency energy harvesting improves the performance of one-to-many wireless controls involving WSNs; diffusion starting at a given node will supply power(s) to communicate or eavesdrop on the communication during such transfer. It is vital to note that safety and health regulations restrict radiofrequency production power [4].
In various WSN systems, RF energy storage may be used indoors and outdoors. Their applications include environmental surveillance, smart homes, systemic safety monitoring, and supervision of locations. Rf energy harvesting has tremendous potential for indoor usage because peripheries often need additional low-light conditions or lack of lightning, while astrophysical energy harvesting assemblies are inefficient. Moreover, temperature variations and disruptions are unlikely indoors, providing excellent conditions for wireless sensor network applications [23].
3.2.An astrophysical-based energy harvesting
Astrophysical energy tends to emerge as an affordable and clean energy source due to global abundance, and it may potentially alleviate the impending issues of WSN energy. However, due to the night-time disadvantages of solar energy storage systems, engineers must achieve the best possible performance during the daytime to ensure efficiency. The photovoltaic effects may be observed when specific semiconductor resources are exposed to sunlight and cosmic rays are transmuted into electricity. A cosmic booth tends to be an electrical semiconductor confluence device, typically self-possessed of silicon.
As sunbeams impact an astrophysical device with adequate dynamics, the electrons are quarantined and continue to pass through an input and output regulator toward the attached charge. Cosmological harvesting employs an output stock of a consuming unit with numerous loading adoptions; these phenomenal capacitors, arrays, or a combination of both, immersed on the external surface of the astrophysical cell, yield excellent cosmological coordination that offers tremendous efficiency. The astrophysical energy, overall, is generally appropriate in an outdoor environment. Indoor atmospheres, such as infirmaries, grounds, and engineering construction, can also benefit; however, they usually obligate extra light than other indoor surroundings [33,57].
3.3.Thermal-based energy harvesting
Transforming the temperature and corresponding energy into electrical energy requires a container to be combined with the hot and cold surfaces of the thermal energy generator. Several large-scale machines have been developed since the production of passionate heaters. More significantly, the extreme apprehension triggers voltage from anthropological physique malaises. A thermos-electric regenerator obligates a long service cycle, inactive parts, and stable functionality. Nevertheless, thermal harvesting has a low efficiency of 5% to 6%, a significant impediment to widespread adoption. Recently, more than 10% of the output efficiency has been reached. The development of modern thermo-electric components and segments [17].
3.4.Mechanical-based energy harvesting
A Mechanic-Electrical Energy Generator (MEEG) extracts energy from the atmosphere and roughness. An electromagnetic harvester, for example, MEEG, uses a piezoelectric system to extract energy. Pressure variations can be rehabilitated to vigor using piezoelectrics that provide optimum and concrete impact. Vibrations are essential in processing the electromagnetic spectrum to shift a magnet around a coil and produce current. On the other hand, electrostatic converters use impulses to force the charged capacitor’s plates toward the electrostatic resistance, resulting in electrical energy in arrears to the capacitance proliferation. The piezoelectric materials can transform electric perspective when stimulated by electrical energy vibrations [55]. The mechanical electrical energy generator, which generates energy reduction in the system, produces 10 mW (milliwatt) per 100 cm3 on a realistic scale. Table 3 depicts EH approaches, related technologies, energy harvesting methodologies, and applications.
Table 3
Energy harvesting techniques and applications
EH Technique | Harvesting Method | Application | Technologies | Reference |
Frequency-based | High-performance thermoelectric materials | Prediction of electron-doped kzrcuse3 | Rectifying antenna | Wang et al. [55], 2020 |
Rectenna | ||||
Solar based | Acquaintance to dainty photovoltaic cells | Outdoor, indoor systems | Photovoltaic (PV) cells | Satyala et al. [43], 2014 |
Thermal based | Temperature difference, heating, or cooling pyroelectric | Wearable TEG | Thermoelectric, pyroelectric, TEG | Li et al. [27], 2016 |
Flex TEG, automatic-powered wireless temp sensor | ||||
Flow-based | Converting airflow | Outdoor | Wind turbines, miniature wind turbines | Zhu et al. [70], 2020 |
Mechanical based | Ambiances power-driven stress and compression, tension from the superficial of the device | The self-powered WSN node, driving vehicles (MEEG) | Piezoelectric, electrostatic, and electromagnetic | Elahi et al. [13], 2018 |
Human-based | Vicissitudes in extremity situations, the body heats, and the blood-flows | Micro TEG | Electroactive polymers, piezoelectric, shoe-mounted rotary harvesters | Khalid et al. [22], 2019 |
3.5.Human-based energy harvesting
The healthcare sector is of prime significance among other WSN applications due to the need to continuously track patients and ensure accurate, significant, and timely action by medical practitioners. Consequently, the wireless bodied-area networks need considerable attention; sensor nodes are mounted on or inside the human body in such networks to track biological constraints continuously [43]. These systems must be stable for long periods because of their human installation, preferably for their lifespan. Therefore, harvesting human energy for this purpose carries paramount importance.
4.Appropriation, inverse, and future directions
Usages of energy harvesting, when a balance exists between the available energy and the energy required for power storage, offer a value that cannot be accomplished with batteries or energy from the grid. Table 4 illustrates the proposed EH models and used technology-based device applications [27]. This section outlines the appropriate approach, inverse, and future motivations.
Table 4
Proposed harvesting techniques and their applications
Harvesting technique | Application(s) | Proposed model | Technologies | Pros | Cons | Reference |
Frequency-based | Movement speed of a monitored asset | RF energy through Wireless Power Transfer (WPT) | Battery-free tags | Wide applications like automation industry, agriculture, IoT, health | Equipment dependency | Zhu et al. [70], 2017 |
Solar based | Transmission range extension and energy consumption reduction in wireless sensor networks | software-defined architecture for CB communications | Collaborative beamforming (CB) | simple, cheap, efficient | Limited Solar energy supply | Elahi et al. [13], 2018 |
Thermal based | Autonomous WSN nodes | The DA + O&SO method | Novel maximum power point tracking (MPPT) | Static deployments, thus expenditure | Poor energy, high cost, and low output power | Khalid et al. [22], 2019 |
Flow-based | Data collection using a mobile sink | Two efficient algorithms to improve the data-gathering process | Maximizing Data Gathering with Minimum Energy Consumption (MDGMEC) | Predictable energy output | Not available for commercial usage | Rosa et al. [38], 2020 |
Mechanical based | Portable and wearable electric devices | Half-wave mechanical rectification mechanism | Bidirectional vertical oscillation into unidirectional rotation of the generator with nonlinear inertia | Environmentally enclosed, protected, reliable | Some piezoelectric materials used in devices deteriorate with time. | Bao et al. [5], 2019 |
Human-based | Utilizing the converted electricity to power the related electronic device | Off-the-shelf commercial chips, LTC3108, and BQ25504 circuit | Thermoelectric energy generator | Implantable applications for human being | Limitation on lifetime and reliability of biological components | Hou and Chen [18], 2020 |
4.1.Appropriation
Within this section, we present the identified appropriation of WSNs.
4.1.1.Industrial condition monitoring
Control and automation delve into the explosive growth in the use of wireless sensor networks in several applications over the last few years. Since wireless technology can reduce costs, increase efficiency, and make maintenance more accessible, it is becoming increasingly popular. Self-organization, fast deployment, versatility, and inherent intelligent processing are all advantages of industrial wireless sensor networks over conventional wired industrial monitoring and control systems. In this regard, industrial wireless sensor networks are critical in establishing more dependable, effective, and profitable industrial structures, enhancing companies’ market competitiveness. Many of the vibrating machinery are usually in a large oil pumping station. Understanding the operation conditions avoids errors and downtime, improves performance, and lowers costs. Wireless sensor networks can be expensive, with miles of cabling and standardized battery-operated spare parts. A wireless-controlled independent pulsation device network offers an incredible system for delinquent data collection [26].
4.1.2.Operating isolated setups
Energy harvesting systems can use both artificial and natural sources to generate electricity. The piezoelectric and thermoelectric (See beck) effects can also be used to generate electrical energy from movement. Environmental fuel, such as solar, wind, or water power, is an excellent outdoor source. Using ambient heat as an energy source for remote sensors inside a building may be beneficial. Thermoelectric devices can produce electrical energy from temperature variations through a thermocouple, while pyroelectric devices can generate electrical energy from changes in material temperature, detailed [46]. A transit stop with an interactive bus information board is beneficial. The technology is precious in rural areas; however, grid power is expensive to install due to its size, and single-use sequences would not continue for long. The astrophysical panels or small airstream turbines, self-propelled with a rechargeable battery and equipped with power controls, are the cheapest way to control the projector and additional electronics [61].
4.1.3.Wireless sensor networks
Due to their ubiquitous existence and broad deployment in IoT, cyber-physical systems, and other emerging areas. The WSNs have recently attracted much attention. WSN technologies face a significant bottleneck due to the limited energy associated with them. The design and development of reliable and high-performance energy harvesting systems for WSN environments are being investigated to address this significant limitation. Sensors seldom need much energy, meaning flow rates, temperature, humidity, and pressure measurements can be attained with a small amount of ingathered energy. The exchange rate, such as the latest readings of necessary wireless communication protocols, allows the use of information to save money through timely detection of complications and planned maintenance [21].
4.1.4.Wireless pressure sensors
Car manufacturers are integrating wireless communication systems into automobiles to improve the safety and performance of the automotive transportation system. The first wireless network installed in every new vehicle is an in-vehicle sensor network: the tire pressure monitoring system. While vehicle-to-vehicle and vehicle-to-infrastructure networks have gained much coverage, the first wireless network installed in every new vehicle is an in-vehicle sensor network, the tire pressure monitoring system. Tire pressure monitoring systems can track real-time tire pressure in vehicles and warn drivers and passengers when a tire is in an abnormal condition, ensuring driver and passenger safety. The pressure control of tires decreases fuel consumption and enhances driving efficiency, but there is no connection from the battery to the tires for power cables. The Battery-powered sensors via wireless data transmission do not last long to provide ample electricity, enhancing maintenance costs for changing batteries. Performing friction energy from everyday factors as the helm rotates is the idyllic way of undertaking the sensors explained; see [32].
4.1.5.Pipeline actuators
The advancement of embedded systems and communication technology has resulted in a need in the industry to automate tasks and provide more power. Rapid advances in data processing technology, wireless networking, the evolution of micro-electromechanical systems, business intelligence, and process planning and management have provided opportunities for change in all aspects of oil and gas processing industry practices. Because of their duration, reliable monitoring and timely reporting to remote control rooms for oil and gas-carrying pipelines is a more significant challenge. Plenty of electricity is available from a natural gas tank or a steam pipe delivery system. Maintenance-free solid-state thermoelectric transducers can capture thermal energy. The energy can be used to open and shut down valves without needing the actuator’s cables [47].
4.2.The inverse of energy harvesting
Free energy also comes at a cost, and going about the entire system is critical in deciding how energy harvesting can satisfy the power needs.
4.2.1.Green energy
A fallacy that continues to spread is that energy harvesting is a moderate example of how the population can capture naturally available energy and that such systems can be industrialized and recycled in the future to power our hospices and industries. The systems are incompatible, except for the photovoltaic cells capturing sunlight [62].
4.2.2.Collecting wasted energy
Calculations for demonstrating how much electricity is ‘consumed’ by a thousand people’s feet are easy to do. There is little economic sense in running permitted types of machinery from passengers’ pathways at subway locations. The harvesters would be costly; besides, the amount of generated energy is insignificant compared to the electricity consumed in illumination, reheating, and aeration. If the charge drop was the goal, it would pay off even faster to improve the operation of current construction facilities and replace old equipment [20].
4.2.3.Self-charging consumer electronics
It would be wonderful to have a mobile phone without charging. Despite increasing print and electronic media announcements, we are uncertain of seeing one soon. Modern palm-sized astrophysical devices can yield energy for this; however, the phone cannot be carried in the pocket when charging. The vibration energy harvesters that use movement energy usually offer sufficient power, nevertheless, merely under specific circumstances. Combine these negatives with sophisticated mobile influence conventions comparatively with mature headphones, and the alteration between the energy required and the energy obtainable is only widening; see [12].
4.2.4.Drop-in battery replacement
A battery-operated system runs wherever it is. Harvesters can only produce electricity where there is sufficient energy. Batteries are standard products available from various manufacturers at a low cost. As new, high-cost, mostly bespoke technology solutions, energy harvesters are available from a limited range of manufacturers [44].
4.3.The challenges in energy harvesting/optimization in WSN/IoT networks
The increasing development of the IoT has heightened the need to develop wireless sensors with low power consumption. Wireless sensors have now been incorporated into IoT systems to provide data in a precise and reliable way for monitoring and controlling the flow of activities in various sectors. The EH technology is a green method for extending the sensor’s life and, in certain circumstances, eliminates the need for batteries. The EH also has economic and practical advantages, for instance, increased energy efficiency and cheaper grid maintenance costs [45]. We outlined some of the upcoming research problems that must be solved on a wide scale to realize the potential for EH solutions in the IoT fully. Although significant progress has been made during the last decades in the development and design of energy-harvesting devices, major scientific difficulties remain before IoT device production becomes self-sustaining:
4.3.1.Harvested energy modeling
A balance between the quantity of energy generated and the quantity of energy used is essential. This necessitates IoT device power profiling and customization of their functioning to the amount of gathered vigor. The accessibility of collected energy fluctuates with time in a non-deterministic way. As a result, prediction techniques are used to estimate the amount of energy scavenged and to govern the power coming from the energy sources, classical power management methods (such as PLL (software Phase Locked Loops) and MPPT (Maximum Power Point Tracking)) are utilized. Models for energy forecasting that have recently been offered should be improved to produce more accurate findings, and management of energy decisions must be taken to reduce energy damage. Furthermore, the power supply must offer sufficient energy for processing processes of data, activities of transmission and/or reception, and intervals of sleep [48]. Most of the time, data processing does not use the most energy. As a result, to lower the cost of energy during wireless data transmission, future researchers should develop optimum consumption models and examine creative approaches to tailor wireless transmission protocols to the energy harvesting process’s features.
4.3.2.Harvested energy storage
Because the technique that was employed to store the energy gathered influences the size, price, and working life of the IoT devices. This entails the advancement of rechargeable batteries and supercapacitors as acceptable storage components. Due to the cycling deterioration phenomenon, batteries with high power densities are not ideal for IoT devices that must last long. Furthermore, they are harmed by both hot and low temperatures [53]. Although the energy density of supercapacitors is lower than that of batteries, they do not suffer from cyclic deterioration. In addition, supercapacitors experience higher current leakage, which consumes a significant portion of the energy collected. As a result, future researchers should look at new methodologies for identifying the best contender for storing collected energy, as determined by the following criteria: minimal cycle degradation, minimal energy leakage, High-density energy, and continuous functioning even at extreme temperatures.
4.3.3.Energy harvesting from multiple sources
In certain circumstances, a single power harvesting source is inadequate to provide electricity to IoT devices. The dependability of IoT devices may be improved by integrating energy from several sources and developing designs and methodologies for circuits for low-power management that collect energy from numerous heterogeneous energy sources. The findings show that the suggested designs are appropriate for specified utilization. For example, the power ring topology or the complementary usage of harvesters is an acceptable scheme when all input energy sources are not anticipated to supply a considerable amount of power simultaneously. Energy is combined from heterogeneous sources using Switched-inductor and switched capacitor converter topologies with multiple inputs [42]. Developers should consider creating customizable matching impedance techniques for MPPT control regardless of the architecture used. Additionally, scientists must concentrate their efforts on the creation of sophisticated algorithms with the ability to pick up energy sources of information based on their accessibility, obviating the requirement for the storage of energy.
4.3.4.Size and cost efficiency
IoT device dimensions and weight are sometimes important (implantable and Wearable IoT devices, for example). However, these gadgets only create a small amount of power, which is insufficient to accomplish their primary purpose (For example, powering the gadget and any associated sensors, as well as data transfer). Micro and nanoscale harvesting systems that can power IoT devices while supporting additional tasks (for example, monitoring patient health status and functioning as stimulators for tissue regeneration) should be created with little manufacturing costs in mind. According to scientific literature, PEHs can power tiny and miniscule IoT devices successfully. Future researchers must discover new eco-friendly materials to allow PEH mini and nanofabrication with increased versatility and output energy density [50]. Recent breakthrough microelectronics hold much promise and may be leveraged to create energy harvesters that are resilient, compact, low-cost, and low-power.
4.3.5.Impact of renewable energy sources on the environment
Sources of renewable energy assist in reducing contamination, and as a result, they are being utilized to produce current IoT gadgets as the sector has grown significantly in recent years. In IoT devices, batteries that do not include energy-collecting technology gradually deteriorate and are discarded after a few weeks or months. The ecology suffers if there are no battery recycling methods in place. In this scenario, the task is to design IoT devices that capture energy with a substantially longer lifespan than batteries. It’s also worth mentioning that specific IoT devices that capture energy use harmful or infrequent minerals (for example, TEEHs use bismuth telluride, PEHs use lead zirconate titanate, and PVs use cadmium.). As a result, another difficulty must be addressed: using environmentally benign things, electroactive polymers, for example, and carbon nanowire semiconductors, to create electrical components for energy harvesting IoT devices. For a sustainable future, makers of IoT devices must consider biodegradable and biocompatible technologies [6].
4.4.Future directions
The energy dispensation work has concentrated on the transducer to resolve how to extract as much energy as possible from an assumed dynamic pitch. As our knowledge progresses, the emphasis will turn to new applications recognizing the potential of energy harvesters.
4.4.1.System integration and smart cities
The idea of smart cities is now a reality; cities are becoming increasingly connected and intelligent, with rapid advancements in areas such as transportation, infrastructure, and public services. These will help deliver services more effectively and safely, enriching the lives of residents and tourists, and the data produced can be used for new and creative applications. Although energy harvesting can power many of the sensors that allow these applications, there is a growing demand for autonomous, distributed, or wearable sensing devices that can also perform edge analytics. These systems are now integrated and usually operated by energy harvesting systems, allowing for fast and low-cost deployment. The EH plug-and-play would become the standard. We recognized the vendors for procuring and installing various harvester components on demand. The conglomerate’s community is developing systems to allow a variety of individual harvesters to be linked to mutual energy acclimatization and storage units. Portable and mechanical wireless devices have generated power from these systems, adding helpful knowledge to make travel, safety, and infrastructure changes possible.
4.4.2.Low power wireless communications
In the last decade, rapid advances in energy harvesting have greatly improved the performance of devices in transforming ambient free energy into usable electrical energy, allowing for the design of energy-autonomous systems today. The design of low-power systems is highly motivated by a clear understanding of the harvesting capability from the source side to achieve such energy-autonomous systems. Communication protocols are being developed and are unambiguously planned to diminish the complex use of data communication. Their implementation is delayed by a rational fear of obligatory technologies that may slip down the way in the superlative protocol markets.
4.4.3.Biological systems and falling costs
While EH and power generation are still looming challenges hindering their widespread use, wireless biomedical implantable devices on the millimeter scale allow a broad range of human health protection and identification applications. Thermal, kinetic, radio frequency, and radiative sources have all supported biomedical implants as energy scavengers. However, achieving effective energy scavenging for millimeter-scale biomedical implants will be tough. The regulation of breathing or blood pressure may operate medical devices with ultra-low electricity. Scientists ought to build an advanced pacemaker capable of pulling a third of the required energy from the blood supply. The cost of EH would reduce as it makes its way into products for global customers. Therefore, business projects should allow greater use of energy harvesting-powered technologies supporting the developmental work, all summarized in Table 5.
Table 5
A summarised appropriation, inverse, and future directions
Harvesting technique | Application | Proposed model | Reference |
Appropriation | Industrial condition monitoring | A pulse-based condition monitoring approach | Ferg et al. [14], 2019 |
Operating isolated setups | Wireless power transfer to eventually allow battery-free sensor nodes | Khazaee et al. [24], 2020 | |
Wireless sensor networks | Two different vibration-based harvesters: piezoelectric and electromagnetic | Rosa et al. [39], 2019 | |
Pipeline actuators | The micro-electro-mechanical system using a vertical multi-junction photovoltaic cell | Chamanian et al. [8], 2019 | |
Green energy | The integration of organic polymers with inorganic nanoparticles | Sejaan et al. [41], 2020 | |
The inverse | Collecting wasted energy | Energy consumption and data delivery | Zhang & Park [67], 2019 |
Self-charging consumer electronics | Battery-free short-range self-powered wireless sensor network | Allam et al. [3], 2019 | |
Drop-in battery replacement | Two piezoelectric vibration energy harvesters via COMSOL | Wen et al. [58], 2020 | |
System integration | Integrating a nonlinear vibration absorber | Wang et al. [56], 2019 | |
Future directions | Smart cities | Dependable control schemes for microgrid management | Zhang et al. [68], 2019 |
Biological systems | Track and monitor the pink iguana of the Galápagos | Zungeru et al. [72], 2019 | |
Falling costs | A self-powered human motion monitoring | Ha & Phung [15], 2019 | |
Artificial intelligence (AI) | Technology fusion of wearable systems and AI is reviewed | Loreti et al. [28], 2019 |
5.Lessons learned and the conclusion
Firstly, embracing renewable energy sources, such as solar and wind power, is crucial for sustainability and reducing environmental impact. Secondly, advancements in energy storage technologies, such as batteries and supercapacitors, are pivotal for managing intermittent renewable energy and ensuring a stable power supply. Thirdly, optimizing energy efficiency in various sectors, including transportation, manufacturing, and buildings, can significantly reduce energy waste. Moreover, fostering a culture of energy conservation and awareness among individuals and organizations is essential for long-term sustainability. Lastly, government policies and incentives play a vital role in driving innovation and promoting the adoption of clean energy solutions, underscoring the importance of public and private sector collaboration in shaping a more energy-efficient future. This paper presents a clear trend of energy harvesting strategies in fulfilling the energy demands for prospective wireless sensor network installations. Due to their design and implementation, the WSNs sojourn to attract much interest from different shareholders. This study investigated the energy harvesting node architecture, harvester hardware configurations, the amount of energy harvested, and the harvester output. The prestige, potential, and anticipated essential uses in the future were discussed. Some open research issues that must be tackled have also been explored. Overall, energy harvesting and WSNs have witnessed an increased research paradigm recently. These approaches can find numerous innovative applications and offer novel solutions to otherwise intricate problems, and thus deserve significant attention.
The future direction in the energy field lies in continued advancements toward decarbonization, resilience, and sustainability. This involves a significant expansion of renewable energy sources like solar, wind, and hydroelectric power, combined with cutting-edge energy storage solutions to ensure a reliable and continuous energy supply. The electrification of transportation, industry, and heating sectors and the integration of smart grid technologies will be critical for achieving a low-carbon future. Additionally, focusing on distributed energy systems energy efficiency measures and exploring emerging technologies such as advanced nuclear reactors and green hydrogen production will play pivotal roles in shaping a more sustainable and resilient global energy landscape.
Conflict of interest
None to report.
References
[1] | K.S. Adu-Manu, N. Adam, C. Tapparello, H. Ayatollahi and W. Heinzelman, Energy-harvesting wireless sensor networks: A review, ACM Transactions on Sensor Networks 14: (2) ((2020) ), 1–5. doi:10.1145/3183338. |
[2] | S. Agrawal, S. Sarkar, G. Srivastava, P.K. Maddikunta and T.R. Gadekallu, Genetically optimized prediction of remaining useful life, (2021) , arXiv preprint arXiv:2102.08845. |
[3] | A.H. Allam, M. Taha and H.H. Zayed, Enhanced zone-based energy aware data collection protocol for wsns, Journal of King Saud University – Computer and Information Sciences 31: (4) ((2019) ), 415–560. doi:10.1016/j.jksuci.2017.12.007. |
[4] | M. Amirinasab, S. Shamshirband, A.T. Chronopoulos, A. Mosavi and N. Nabipour, Energy-efficient method for wireless sensor networks low-power radio operation in Internet of things, Electronics 9: (2) ((2020) ), 3–20. |
[5] | X. Bao, H. Liang, Y. Liu and F. Zhang, A stochastic game approach for collaborative beam forming in sdn-based energy harvesting wireless sensor networks, IEEE Internet of Things Journal 6: (6) ((2019) ), 9583–9595. doi:10.1109/JIOT.2019.2930073. |
[6] | D. Cavalcanti, J. Perez-Ramirez, M.M. Rashid, J. Fang and M. Galeev, Extending accurate time distribution and timeliness capabilities over the air to enable future wireless industrial automation systems, Proc. IEEE 107: (6) ((2019) ), 1132–1152. doi:10.1109/JPROC.2019.2903414. |
[7] | R. Chai, H. Ying and Y. Zhang, Super capacitor charge redistribution analysis for power management of wireless sensor networks, IET Power Electronics 10: (2) ((2017) ), 169–177. doi:10.1049/iet-pel.2015.1029. |
[8] | S. Chamanian, S. Baghaee, H. Uluşan, Ö. Zorlu and E. Uysal-Biyikoglu, Implementation of energy-neutral operation on vibration energy harvesting WSN, IEEE Sensors Journal 19: (8) ((2019) ), 3092–3099. doi:10.1109/JSEN.2019.2890902. |
[9] | C. Chien, I. Elgorriaga and C. McConaghy, Low-power direct-sequence spread-spectrum modem architecture for distributed wireless sensor networks, in: Proceedings of the 2001 International Symposium on Low Power Electronics and Design, (2001) , pp. 251–254. doi:10.1145/383082.383151. |
[10] | S. Choenni et al., Data governance in smart cities: Challenges and solution directions, (2022) , 31–51. |
[11] | J.L. Chun-Wei, G. Srivastava, Y. Zhang, Y. Djenouri and M. Aloqaily, Privacy-preserving multi-objective sanitization model in 6G IoT environments, IEEE Internet of Things Journal 8: (7) ((2020) ), 5340–5349. |
[12] | O.A. Egaji, S. Chakhar and D. Brown, An innovative decision rule approach to tyre pressure monitoring, Expert System Application 124: ((2019) ), 252–270. doi:10.1016/j.eswa.2019.01.051. |
[13] | H. Elahi, M. Eugeni and P. Gaudenzi, A review on mechanisms for piezoelectric-based energy harvesters, Energies 11: (7) ((2018) ), 18–50. |
[14] | E.E. Ferg, F. Schuldt and J. Schmidt, The challenges of a Li-ion starter lighting and ignition battery: A review from cradle to grave, Journal of Power Sources 423: ((2019) ), 380–403. doi:10.1016/j.jpowsour.2019.03.063. |
[15] | Q. Ha and M.D. Phung, Iot-enabled dependable control for solar energy harvesting in smart buildings, IET Smart Cities 1: (2) ((2019) ), 61–70. doi:10.1049/iet-smc.2019.0052. |
[16] | Z. Hadas, L. Janak and J. Smilek, Virtual prototypes of energy harvesting systems for industrial applications, Mechanical System Signal Process 110: ((2018) ), 152–164. doi:10.1016/j.ymssp.2018.03.036. |
[17] | S. Hao, L. Ward, Z. Luo and V. Ozolins, Design strategy for high-performance thermoelectric materials: The prediction of electron-doped kzrcuse3, Chemistry of Materials 31: (8) ((2019) ), 3018–3024. doi:10.1021/acs.chemmater.9b00840. |
[18] | L. Hou and W. Chen, A novel mppt method for autonomous wireless sensor networks node with thermal energy harvesting, Engineering Research Express 2: (1) ((2020) ), 01–005. |
[19] | J. Huang, Y. Zhou, Z. Ning and H. Gharavi, Wireless power transfer and energy harvesting: Current status and future prospects, IEEE Wireless Communication 26: (4) ((2019) ), 163–169. doi:10.1109/MWC.2019.1800378. |
[20] | A. Iqbal, Y. Kim, J. Ahn and T. Lee, Energy level-based efficient wireless power and information transfer in sensor networks, in: Proc. 11th International Conference on Ubiquitous Information Management and Communication, (2017) , pp. 1–5. |
[21] | A.Z. Kausar, A.W. Reza, M.U. Saleh and H. Ramiah, Energizing wireless sensor networks by energy harvesting systems: Scopes, challenges and approaches, Renewable Sustainable Energy Revelation 38: (2) ((2014) ), 973–989. doi:10.1016/j.rser.2014.07.035. |
[22] | S. Khalid, I. Raouf, A. Khan, N. Kim and H.S. Kim, A review of human-powered energy harvesting for smart electronics: Recent progress and challenges, International Journal of Precise Engineering Manufacture Green Technology 6: (4) ((2019) ), 821–851. doi:10.1007/s40684-019-00144-y. |
[23] | A. Khalifeh, M. Saadeh, K.A. Darabkh and P. Nagaradjane, Radio-frequency based energy charging- an experimental study, in: 2nd IEEE Middle East and North Africa Communications Conference, Manama, (2019) , pp. 1–4. |
[24] | M. Khazaee, A. Rezaniakolaie, A. Moosavian and L. Rosendahl, A novel method for autonomous remote condition monitoring of rotating machines using piezoelectric energy harvesting approach, Sensors and Actuators 295: ((2020) ), 37–50. doi:10.1016/j.sna.2019.05.016. |
[25] | D.P. Kumar, T. Amgoth and C.S. Annavarapu, Machine learning algorithms for wireless sensor networks: A survey, Information Fusion 49: ((2019) ), 1–25. doi:10.1016/j.inffus.2018.09.013. |
[26] | N. Kumar and D. Dash, Flow based efficient data gathering in wireless sensor network using path-constrained mobile sink, Journal of Ambient Intelligence and Humanized Computing 11: (3) ((2020) ), 1163–1175. doi:10.1007/s12652-019-01245-x. |
[27] | D. Li, Y. Wu, A. Da Ronch and J. Xiang, Energy harvesting by means of flow-induced vibrations on aerospace vehicles, Progress in Aerospace Sciences 86: ((2016) ), 28–62. doi:10.1016/j.paerosci.2016.08.001. |
[28] | P. Loreti, A. Catini, M.D. Luca, L. Bracciale and G. Gentile, The design of an energy harvesting wireless sensor node for tracking pink guanas, Sensors 19: (5) ((2019) ), 9–85. |
[29] | P.K. Maddikunta, T.R. Gadekallu, R. Kaluri, G. Srivastava, R.M. Parizi et al., Green communication in IoT networks using a hybrid optimization algorithm, Computer Communications 159: ((2020) ), 97–107. doi:10.1016/j.comcom.2020.05.020. |
[30] | P.K. Maddikunta, G. Srivastava, T.R. Gadekallu, N. Deepa and P. Boopathy, Predictive model for battery life in IoT networks, IET Intelligent Transport Systems 14: (11) ((2020) ), 1388–13895. doi:10.1049/iet-its.2020.0009. |
[31] | D. Marie-Andree and D. Sylvie, Geomatics and smart city: A transversal contribution to the smart city development, Journal of Smart Cities and Society ((2014) ), 57–72. |
[32] | S.M. Matinkhah and W. Shafik, Unmanned aerial vehicles analysis to social networks performance, The CSI Journal on Computer Science and Engineering 18: (2) ((2023) ), 24–31. |
[33] | K. Mausam, S. Kumar, S. Kumar Ghosh, A.K. Tiwari, M. Sehgal et al., Solicitation of nanoparticles/fluids in solar thermal energy harvesting: A review, Material Today Processing 26: (2) ((2020) ), 2289–2295. doi:10.1016/j.matpr.2020.02.495. |
[34] | C.V. Nguyen, T.V. Quyen, A.M. Le, L.H. Truong, M.T. Nguyen et al., Advanced hybrid energy harvesting systems for unmanned ariel vehicles, Advances in Science, Technology and Engineering Systems Journal 5: (1) ((2020) ), 34–39. doi:10.25046/aj050105. |
[35] | A. Pal, Localization algorithms in wireless sensor networks: Current approaches and future challenges, Netw. Protoc. Algorithms 2: (1) ((2010) ), 45–73. |
[36] | C. Periñán-Pascual, From smart city to smart society: A quality-of-life ontological model for problem detection from user-generated content, Journal of Smart Cities and Society ((2023) ), 263–306. |
[37] | J. Raja and N. Mookhambika, A novel energy harvesting with middle-order weighted probability (EHMoWP) for performance improvement in wireless sensor network (WSN), Journal of Ambient Intelligence and Humanized Computing ((2021) ), 1–12. |
[38] | R.L. Rosa, C. Dehollain, F. Pellitteri, R. Miceli and P. Livreri, A battery-free asset monitoring system based on rf wireless power transfer, in: IEEE 20th Mediterranean Electrotechnical Conference, Palermo, (2020) , pp. 181–186. |
[39] | R.L. Rosa, P. Livreri, C. Trigona, L.D. Donato and G. Sorbello, Strategies and techniques for powering wireless sensor nodes through energy harvesting and wireless power transfer, Sensors 19: (12) ((2019) ), 26–60. |
[40] | W.K. Seah, Z.A. Eu and H.P. Tan, Wireless sensor networks powered by ambient energy harvesting (WSN-HEAP)-survey and challenges, in: 2009 1st International Conference on Wireless Communication, Vehicular Technology, Information Theory and Aerospace & Electronic Systems Technology, IEEE, (2009) , pp. 1–5. |
[41] | G.A. Sejaan, F. Lamarque, N.E. Hajj, J.A. Assad and H.A. Hajjar, Optical energy harvesting in a smart materials-based micro-actuator using a vertical multi-junction PV cell, in: Energy Harvesting and Storage Materials, Devices, and Applications X, Vol. 11387: (2020) , pp. 113–870. |
[42] | E. Setyawan, A. Kurniawan, F. Rahmadianto, R.A.M. Napitupulu and P. Siagian, Flat plate type solar collector performance using double thermal insulation, Jakarta, IOP Conference Service Material Science Engineering 852: ((2020) ), 012–044. |
[43] | W. Shafik, Cyber security perspectives in public spaces: Drone case study, in: Handbook of Research on Cybersecurity Risk in Contemporary Business Systems IGI Global, (2023) , pp. 79–97. doi:10.4018/978-1-6684-7207-1.ch004. |
[44] | W. Shafik, Making cities smarter: IoT and SDN applications, challenges, and future trends, in: Opportunities and Challenges of Industrial IoT in 5G and 6G Networks, IGI Global, (2023) , pp. 73–94. doi:10.4018/978-1-7998-9266-3.ch004. |
[45] | W. Shafik, S.M. Matinkhah and M. Ghasemzadeh, A mobile fuzzy sink scheme for wireless sensor network period improvement, in: 2020 8th Iranian Joint Congress on Fuzzy and Intelligent Systems (CFIS), IEEE, (2020) , pp. 211–216. doi:10.1109/CFIS49607.2020.9238684. |
[46] | W. Shafik, S.M. Matinkhah and F. Shokoor, Cybersecurity in unmanned aerial vehicles: A review, International Journal on Smart Sensing and Intelligent Systems 16: (1) ((2023) ). doi:10.2478/ijssis-2023-0012. |
[47] | F.K. Shaikh and S. Zeadally, Energy harvesting in wireless sensor networks: A comprehensive review, Renewable and Sustainable Energy Reviews 55: ((2016) ), 1041–1054. doi:10.1016/j.rser.2015.11.010. |
[48] | H.H.R. Sherazi, L.A. Grieco and G. Boggia, A comprehensive review on energy harvesting MAC protocols in WSNs: Challenges and tradeoffs, Ad Hoc Network 71: ((2018) ), 117–134. doi:10.1016/j.adhoc.2018.01.004. |
[49] | Q. Shi, B. Dong, T. He, Z. Sun and J. Zhu, Progress in wearable electronics/photonics-moving toward the era of artificial intelligence and Internet of things, InfoMat 2: (6) ((2020) ), 1131–1162. doi:10.1002/inf2.12122. |
[50] | J. Singh, R. Kaur and D. Singh, Energy harvesting in wireless sensor networks: A taxonomic survey, International Journal of Energy Research 45: (1) ((2021) ), 118–140. doi:10.1002/er.5816. |
[51] | S. Sudevalayam and P. Kulkarni, Energy harvesting sensor nodes: Survey and implications, IEEE Communication Survey Tutorial 13: (3) ((2010) ), 443–461. doi:10.1109/SURV.2011.060710.00094. |
[52] | J.H. Syu, M.E. Wu, G. Srivastava, C.F. Chao and J.C. Lin, An iot-based hedge system for solar power generation, IEEE Internet of Things Journal 5: ((2021) ), 156–162. |
[53] | R.R. Tan, K.B. Aviso and D.K.S. Ng, Optimization models for financing innovations in green energy technologies, Renewable Sustainable Energy Revelation 113: ((2019) ), 109–258. |
[54] | A. Vrachimis, S. Gkegka and K. Kolomvatsos, Resilient edge machine learning in smart city environments, Journal of Smart Cities and Society ((2023) ), 3–24. doi:10.3233/SCS-230005. |
[55] | J. Wang, D. Liu, Q. Li, C. Chen and Z. Chen, Lightweight, superplastic yet thermo conductive boron nitride nan composite aerogel for thermal energy regulation, ACS Nano 13: (7) ((2020) ), 7860–7870. doi:10.1021/acsnano.9b02182. |
[56] | L. Wang, G. Luo, Z. Jiang, F. Zhang and L. Zhao, Broadband vibration energy harvesting for wireless sensor node power supply in train container, Review of Scientific Instruments 90: (12) ((2019) ), 12–50. |
[57] | Y. Wang et al., Service delay and optimization of the energy efficiency of a system in fog-enabled smart cities, Alexandria Engineering Journal 84: ((2023) ), 112–125. doi:10.1016/j.aej.2023.10.034. |
[58] | F. Wen, H. Wang, T. He, Q. Shi and Z. Sun, Battery-free short-range self-powered wireless sensor network (SS-WSN) using TENG based direct sensory transmission mechanism, Nano Energy 67: ((2020) ), 104–266. |
[59] | D. Wohwe Sambo, B.O. Yenke, A. Förster and P. Dayang, Optimized clustering algorithms for large wireless sensor networks: A review, Sensors 19: (2) ((2019) ), 322. |
[60] | Y. Xi, J. Hua and Y. Shi, Noncontact triboelectric nanogenerator for human motion monitoring and energy harvesting, Nano Energy 69: ((2019) ), 104–390. |
[61] | C. Xia, D. Zhang, W. Pedrycz, K. Fan and Y. Guo, Human body heat based thermoelectric harvester with ultra-low input power management system for wireless sensors powering, Energies 12: (20) ((2020) ), 39–42. |
[62] | Z. Yang et al., Green Internet of things and big data application in smart cities development, Complexity ((2021) ), 1–15. |
[63] | Z. Yang, Y. Yang, F. Liu, Z. Wang, Y. Li et al., Power backpack for energy harvesting and reduced load impact, ACS nano 15: (2) ((2021) ), 2611–26123. doi:10.1021/acsnano.0c07498. |
[64] | H. Yetgin, K.T.K. Cheung, M. El-Hajjar and L.H. Hanzo, A survey of network lifetime maximization techniques in wireless sensor networks, IEEE Communication Survey Tutorial 19: (2) ((2017) ), 828–854. doi:10.1109/COMST.2017.2650979. |
[65] | D. Zhang, Z. Chen, H. Zhou, L. Chen and X.S. Sherman, Energy-balanced cooperative transmission based on relay selection and power control in energy harvesting wireless sensor network, Computer Networks 104: ((2016) ), 189–197. doi:10.1016/j.comnet.2016.05.013. |
[66] | J. Zhang, X. Bai, W. Han, B. Zhao and L. Xu, The design of radio frequency energy harvesting and radio frequency-based wireless power transfer system for battery-less self-sustaining applications, International Journal of RF and Microwave Computing-Aided Engineering 29: (1) ((2019) ), 777–780. |
[67] | Y. Zhang and S.J. Park, Flexible organic thermoelectric materials and devices for wearable green energy harvesting, Polymers 11: (5) ((2019) ), 9–19. |
[68] | Y.W. Zhang, S. Wang, Z.Y. Ni, Z.W. Fang, J. Zang et al., Integration of a nonlinear vibration absorber and levitation magnetoelectric energy harvester for whole-spacecraft systems, ACTA Mechanica Solida Sinica 32: (3) ((2019) ), 298–309. doi:10.1007/s10338-019-00081-y. |
[69] | T. Zhao, L. Wang, K.W. Chin and C. Yang, Routing in energy harvesting wireless sensor networks with dual alternative batteries, IEEE Systems Journal 15: (3) ((2020) ), 3970–3979. doi:10.1109/JSYST.2020.3007166. |
[70] | Z. Zhu, L. Xu, M.A.A. Abdelkareem, J. Zou and J. Mi, Design and simulation analysis of vibration energy harvesting system based on Adams, in: International Design Engineering Technical Conferences and Computers and Information in Engineering Conference, California, Vol. 8: , (2020) , pp. 97–273. |
[71] | T.T. Zin, P. Tin and H. Hama, Characterizing reliability measure for Internet of things by Markov queue, Data Science and Pattern Recognition 2: (2) ((2018) ), 1–10. |
[72] | A.M. Zungeru, J. Gaboitaolelwe, B. Diarra, J.M. Chuma, L.M. Ang et al., A secured smart home switching system based on wireless communications and self-energy harvesting, IEEE Access 7: ((2019) ), 25063–25085. doi:10.1109/ACCESS.2019.2900305. |