Implicit Motor Sequence Learning in People with Mild to Moderate Parkinson’s Disease: Behavior and Related Brain Function
Abstract
Background:
Deficits in motor learning could be an important explanation for the balance and gait impairments characteristic of people with Parkinson’s disease (PD). Empirical studies often report that so-called implicit motor sequence learning is impaired in people with PD, but the results are inconclusive. Altered brain activity during implicit motor sequence learning has also been reported for people with PD in comparison to healthy individuals.
Objective:
To investigate implicit motor sequence learning and associated neural correlates in individuals with mild to moderate PD.
Methods:
Fifty-seven participants with PD and 34 healthy participants, all ≥60 years of age, performed the serial reaction time task (SRTT) during the acquisition of functional magnetic resonance imaging (fMRI) data. We analyzed the SRTT as a measure of implicit motor sequence learning in two complementary ways. We analyzed the task-induced fMRI data within regions of interest (ROIs) as well as functional connectivity between ROIs.
Results:
We found a significant group difference in SRTT performance indicating that the participants with PD had a somewhat lower level of implicit motor sequence learning than the healthy participants. Exploratory analyses suggested that impairments in implicit motor sequence learning for people with PD might be due to a lower learning rate. We did not find any significant group differences in the fMRI data.
Conclusion:
Our exploratory finding of a lower implicit motor learning rate in PD could have important implications for how people with PD should practice new motor tasks and physical exercise. Future studies need to confirm this finding with hypothesis-driven analyses.
INTRODUCTION
Learning processes can be both explicit (with awareness) and implicit (less or no awareness) [1, 2]. Implicit sequence learning is the ability to learn a series of stimuli without being able to explicitly access the information learned while often also unaware that the learning took place. This type of learning is considered important for acquiring, performing, and adapting motor skills [1, 2]. Impairments in motor abilities are a core symptom in people with Parkinson’s disease (PD), and these could partly be the result of impairments in implicit motor sequence learning [3]. Empirical findings indicate an impairment in implicit motor sequence learning in people with PD but the results are still inconclusive [4]. Motor abilities including implicit motor sequence learning involve the striatum that is negatively affected in people with PD [4, 5].
A model for motor learning (including implicit motor sequence learning) and its neural correlates was proposed by Doyon and Benali [6]. The model describes implicit motor learning as dependent on a cortico-striatal system and a cortico-cerebellar system. The cortico-striatal system is suggested to become more active when the implicit motor sequence learning increases and approaches a consolidation phase. Because the striatum and thereby presumably the cortico-striatal system is negatively affected in people with PD, Doyon [7] suggested that the cerebellum and possibly other brain regions and systems would be more active in people with PD than in healthy individuals, during implicit motor sequence learning. Empirical studies of implicit motor sequence learning in people with PD have reported different activity patterns in the striatum, the cerebellum, the hippocampus, and the dorsolateral prefrontal cortex (DLPFC) as compared to healthy individuals, but the results are inconsistent over the studies [8–10].
As many studies of implicit motor sequence learning in PD [4], we choose to use the so-called serial reaction time task (SRTT). An important benefit of the SRTT is that it requires actual movement (finger movement) but is still unlikely to induce large head movements with detrimental effects for fMRI data. Previous studies of implicit motor sequence learning in PD have often used small samples and sometimes included people with PD with different medication states (ON/OFF). Studies have also included participants with a wide range of symptom severity even though implicit motor sequence learning might deteriorate over disease progression in people with PD [8–10]. These study characteristics could partly explain the inconclusive results for both the behavioral outcomes, i.e., whether people with PD have impaired implicit learning, and the neural correlates.
In the present study, we will compare implicit motor sequence learning in people with mild to moderate PD to healthy individuals of a similar age. That our sample is restricted to people with mild to moderate PD is a strength as it will reduce the effect of symptom severity as a potential confounder of our results. We will also take into account that any learning is a process over time and that people with PD might have a lower motor learning rate than healthy individuals [3]. To this end, we will test the hypothesis that people with mild to moderate PD have a lower level of implicit motor sequence learning than healthy participants. Additionally, we will investigate whether people with mild to moderate PD have a different brain activity than healthy individuals, as measured by functional MRI (fMRI) during implicit sequence learning. In line with the model by Doyon and Benali [6], we also want to test the hypotheses that people with mild to moderate PD have a different functional connectivity than healthy individuals, as measured by fMRI during implicit sequence learning. Finally, we will explore whether the learning process and the associated neural correlates differ for the two groups. Our analysis plan for this study was preregistered on the Open Science Framework (https://osf.io/abprn/).
MATERIALS AND METHODS
Participants
In this cross-sectional study, 57 individuals with mild to moderate PD and 34 healthy individuals, all ≥60 years old, were included from a subsample of the EXercise in PArkinson’s disease and Neuroplasticity project (EXPANd) [11–13]. All participants with PD had a documented diagnosis of idiopathic PD as assessed by their neurologist. The EXPANd project consisted of a randomized controlled trial (RCT) with pre- and post-assessments for people with PD as well as assessments of healthy controls. In the present study, only the pre-assessment data for the participants with PD was used and the healthy controls were assessed parallel to the pre-assessments of the RCT. This means that there were no differences in the exposure to either the situation or the task between the participants with PD and the healthy controls for the data used for the present analyses.
Inclusion criteria for the individuals with PD were: a diagnosis of idiopathic PD with mild to moderate severity defined as Stage 2 or 3 on the Hoehn & Yahr scale [14], age ≥60 years, a score ≥21 on the Montreal Cognitive Assessment (MoCA) [15], and no disease or symptom, over and above PD, that could significantly affect balance or gait. More details on the inclusion/exclusion criteria for individuals with PD can be found in Franzén et al. [12]. Inclusion criteria for the healthy controls were age ≥60 years, a MoCA score ≥23, and no disease or symptom that could significantly affect balance or gait. The cut-off of ≥23 on MoCA for the healthy controls was chosen as a previous study reported this cut-off value to show excellent sensitivity and specificity for mild cognitive impairment in a sample of healthy older adults [16]. The lower MoCA cut-off of ≥21 for the participants with PD was chosen to recruit a representative sample of the PD population where cognitive deficits are common in people with PD already in the mild to moderate stages [17]. The participants with PD were tested in the ON state of dopaminergic medication as we were interested in implicit motor learning in daily life when most people with PD are on medication. There was no fixed time for testing in relation to the last medication intake because as is common in the research field [10, 18–20], the participants differed in the type of dopaminergic medication used and thereby in the medications’ duration of action. The participants were reminded to take their medication according to their daily schedule to reduce the risk that they would perform the SRTT with suboptimal levels of medication. The groups did not statistically differ from each other for any of the baseline outcomes (tested with independent t-tests and chi-squared tests). See Table 1 for more baseline characteristics.
Table 1
Baseline characteristics of the participants with Parkinson’s disease (PD) and the healthy controls (HC)
Outcomes | PD (n = 57) | HC (n = 34) | p |
Age (y)† | 70.6 (6) | 69.9 (4.8) | 0.57 |
Male∥ | 34 (59.6%) | 23 (67.6%) | 0.59 |
Years of education† | 15.2 (3.2) | 15.8 (4) | 0.44 |
Cohabiting† | 45 (78.9%) | 24 (70.6%) | 0.24 |
Montreal Cognitive Assessment score† | 26.4 (2.3) | 26.6 (1.9) | 0.56 |
Disease duration, years since diagnosis‡ | 3 (6) | n.a. | |
Movement Disorders Society-Unified Parkinson’s | 30.1 (9.6) | n.a. | |
Disease Rating Scale-III score† | |||
Movement Disorders Society-Unified Parkinson’s | 48.8 (15.5) | n.a. | |
Disease Rating Scale-Total score† | |||
Hoehn and Yahr 2∥ | 44 (77.2%) | n.a. | |
Hoehn and Yahr 3∥ | 13 (22.8%) | n.a. | |
On dopaminergic therapy∥ | 55 (96.5%) | n.a. | |
Levodopa equivalent daily dose (mg)‡ | 500 (360) | n.a. | |
Levodopa∥ | 49 (86%) | n.a. | |
Dopamine agonists∥ | 24 (42.1%) | n.a. | |
COMT inhibitors∥ | 8 (14%) | n.a. | |
MAO-B inhibitors∥ | 15 (26.3%) | n.a. |
†mean (sd), ∥n (%), ‡median (iqr), n.a., not applicable.
Ninety-five participants with PD were included in the EXPANd trial, of these 14 participants were not able to perform our task of implicit motor sequence learning inside the MRI scanner and had to be excluded from the present study and one participant was excluded due to technical issues. Based on predefined inclusion criteria specific to this study (see https://osf.io/abprn/), we excluded three participants with PD due to excessive movement during fMRI, 16 participants with PD and one healthy participant because they had less than 70% correct trials on the SRTT performed during fMRI, and lastly four of the participants with PD and four of the healthy participants because the showed signs of awareness of the sequence. This left 57 people with PD and 34 healthy individuals for the analyses.
Procedure
We used the SRTT as a measure of implicit motor sequence learning [21]. The SRTT was performed during fMRI. The task was presented on a computer screen behind the scanner and a mirror inside the scanner head coil enabled the participants to view the task. Two response pads were placed on the participant’s lap. The total time inside the scanner was approximately 40 min. The SRTT was 9 min long and presented as the first MR sequence after an initial brief sequence to set up the scanner. The participants practiced a simplified version of the SRTT before they entered the scanner. This training ended when the participant achieved 80% accuracy or after a maximum of three rounds consisting of 40 trials each.
The healthy participants received two cinema vouchers after participation while the participants with PD participated as part of the RCT where they attended interventions aimed to ameliorate PD-related symptoms. After providing oral and written study information, written consent was obtained from all participants. The study was approved by the Regional Research Ethics Board of Stockholm (2016/1264–31/4 with amendments).
Acquirement of the fMRI data
Indirect measures of brain activity were acquired by fMRI and the blood-oxygen-level-dependent (BOLD) signal. A 3T Phillips Ingenia scanner with a 15-channel head coil with the following parameters was used: repetition/echo time (TR/TE) = 2085/35 ms, flip angle = 75°, voxel-size: 3.5×3.5×3.5 mm, the field of view: 224×224×140, 265 slices in ascending order.
Task design
The SRTT was presented in PsychoPy (version 1.85.4). Four white circles on a horizontal line were shown on a black screen and each circle’s position corresponded to one of the four buttons of the two response pads (Fig. 1). Every 1.2 s, one of the circles turned grey and the participant was to press the corresponding button as quickly as possible. They used the index and middle fingers of both hands and two two-button response pads, one for each hand.
Fig. 1
The Serial Reaction Time Task. Showing the order of the random (R) and sequence (S) blocks and the partitioning of blocks into the three parts.
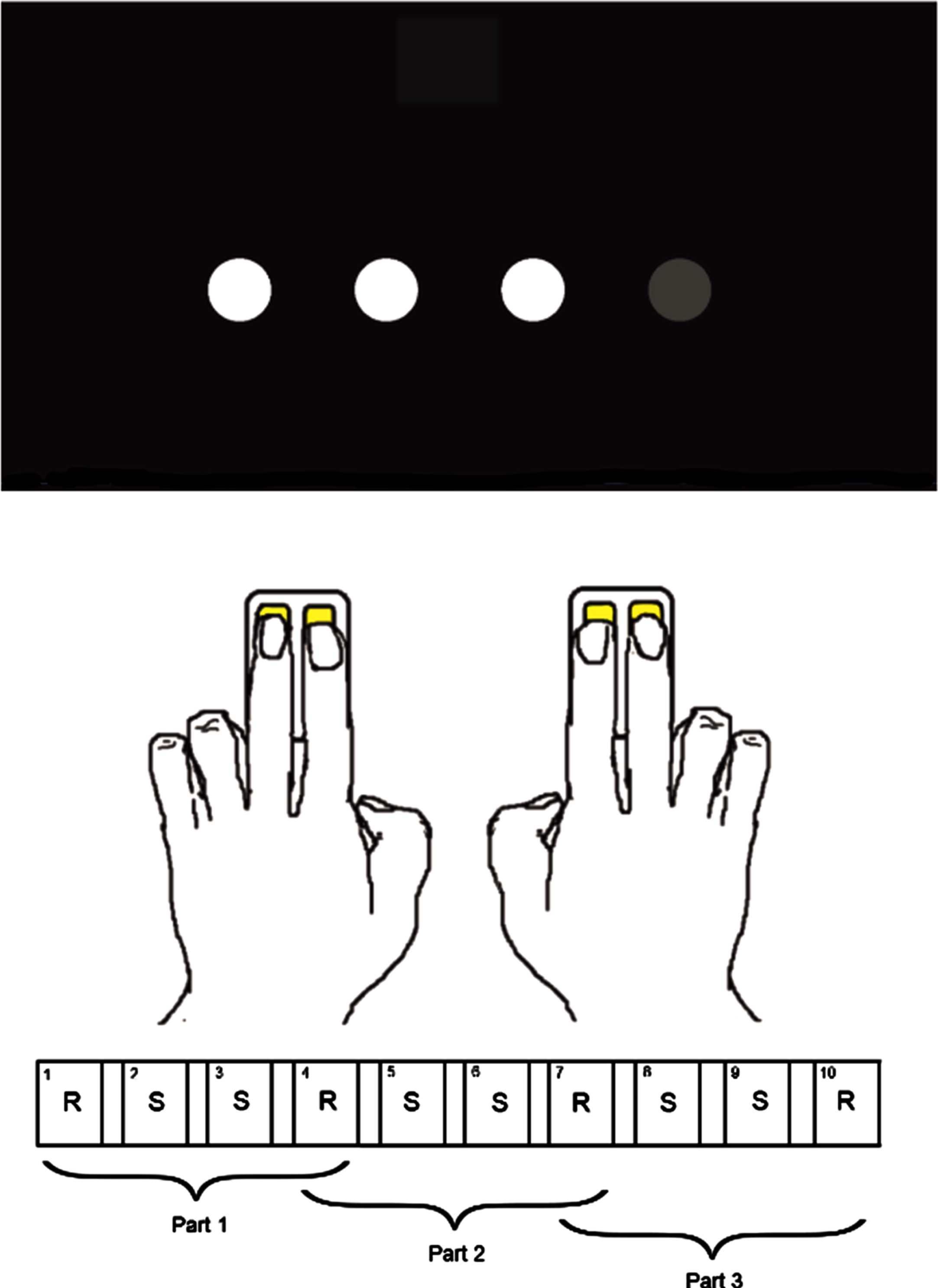
The SRTT consisted of 10 blocks of 40 trials each, and all blocks were followed by a 6-s break. Unbeknownst to the participants, in 6 of the 10 blocks, the trials followed a 10-item higher (second) order sequence (1 3 1 2 4 3 2 4 1 3), which from here on are referred to as sequence blocks. The participants were exposed to the sequence four times per sequence block and 24 times in total. The remaining four blocks consisted of trials presented in a random order with the constraint that a trial could not be the same as the directly preceding trial. These blocks will from here on be referred to as random blocks. The simplified SRTT practiced outside the scanner consisted solely of trials in random order.
Our hypotheses were investigated where all ten blocks, i.e., all trials of the SRTT were included in the analyses. However, because the SRTT measures a learning process, we also wanted to explore whether the behavioral outcomes and the neural correlates changed over the different phases of the learning process. To enable these analyses, we divided the SRTT into three parts. Part 1 consisted of the random blocks 1 and 4 and the sequence blocks 2 and 3, part 2 consisted of the random blocks 4 and 7 and the sequence blocks 5 and 6 and part 3 consisted of the random blocks 7 and 10 and the sequence blocks 8 and 9.
To assess whether any of the participants had achieved explicit knowledge of the sequence, which would lead to exclusion of their data, they were asked to fill out a questionnaire on whether they thought the trials in the SRTT followed a pattern. The healthy participants did this after their only scanning session while the participants with PD answered the questionnaire after their second scanning session. This difference in the procedure was necessary as the participants with PD could not be asked about patterns in the task at their pre-intervention assessment as that would have made them aware of the presence of a hidden sequence during their post-intervention assessment. It was also necessary to use a different sequence (with the same characteristics) at the post-intervention assessment to avoid measuring the participants’ memory of the hidden sequence rather than the ability to learn implicitly. If the participants reported any notion of a pattern, they were asked to reproduce this pattern on a sheet of paper where the first trial of the sequence was given. The participants who reported that they knew the sequence and the participants who correctly reproduced ≥4 consecutive trials of the sequence were excluded from the analyses. Additionally, we scored the responses on the questionnaire for a more detailed report on their awareness of the sequence (see the Supplementary Material).
Statistical analyses
The reaction time analyses of the SRTT
We used the differences in reaction time (RT) between the sequence and random trials as the indicator of implicit learning. Only RTs from correct responses were included in the analyses. Non-responses were coded as incorrect responses and were included in the analyses as missing values to keep the same number of trials for all participants. Responses with an RT <100 ms were excluded (and coded as missing values) as 100 ms has been reported to be the minimum time for physiological processes such as the perception of stimuli [22].
A multilevel model (MLM) was used to assess group differences in implicit learning using the predictors trial number, block type, group and the interaction of block type and group. The primary outcome of this analysis (i.e., of the SRTT outcome) was the interaction effect of group (PD/healthy) and block type (random/sequence block). A random intercept was used but the model did not converge with a random slope. A gamma distribution was assumed based on evaluations of the residuals. Because some of the participants could only perform the SRTT outside the scanner, we performed a sensitivity MLM analysis including all participants who had performed the SRTT either inside or outside the scanner (n = 102, accuracy ≥70% and no signs of awareness). We also calculated the median and IQR of RT per group and part of the SRTT. Additionally, we performed descriptive analyses investigating the correlation of the SRTT performance with the levodopa equivalent daily dose (mg) as well as the effect of Hoehn & Yahr stage on the SRTT performance. The software R 3.6.2 was used for the analyses.
Analyses of the fMRI data
Initial quality control of the MRI data was done using MRIQC and the preprocessing was done using fMRIPrep [23, 24]. Ninety-one participants had fMRI data of sufficient quality (see Supplementary Material for details). We did two main types of analyses of the fMRI data: 1) we investigated the activity within regions of interest (ROI) (from here on called within-regions analyses) and 2) we investigated the functional connectivity between ROIs (from here on referred to as analyses of functional connectivity).
The first and second-level analyses of the within-region analyses were performed in SPM 12 (version 7765) [25] while the first and second-level analyses of functional connectivity were performed in the CONN 20b toolbox [26]. For the analyses of functional connectivity, the pre-processed data was denoised before the first-level analyses. We used the default denoise method in the CONN 20b toolbox for data pre-processed with fmriPrep and applied a high pass filter of 0.008 Hz but no low pass filter, as recommended for task data. For both the within-region analyses and the analyses of functional connectivity, the independent variables for the first-level analyses were the experimental timeline convoluted with the canonical hemodynamic function, 24 motion-derived regressors as well as the first five aCompCor regressors and the cosine regressors.
The masks of our predefined ROIs were based on atlases, see the Supplementary Material for details. The cortical motor area used for the analyses of functional connectivity was the primary motor cortex. This choice was made as the primary motor cortex was previously used in a study of effective connectivity during the SRTT [27].
We investigated whether the data from the healthy participants 1) implied more striatal activity during the sequence blocks than the random blocks and 2) implied greater functional connectivity between the striatum and the primary motor cortex during the sequence blocks than during the random blocks of the SRTT. The analysis of within striatum activity was done in SPM 12 using a one-sample t-test on the contrast images (sequence –random) created in the first-level analyses and the analyses of functional connectivity were done in CONN using dependent t-tests to compare connectivity during the sequence and random blocks.
We also performed analyses to compare the activity during the SRTT between the healthy participants and the participants with PD. For the within-region analyses, independent two-sample t-tests using the contrast images (sequence –random) from the first-level analyses were used to compare the two groups’ task-induced activity in the striatum, the motor parts of the cerebellum, the DPLFC, and the hippocampus. A cluster-defining threshold with a cluster-creating threshold of uncorrected p < 0.001 (voxel-level) and cluster extent threshold of p < 0.05, family-wise error corrected (FWE), was used.
For the group comparisons of functional connectivity, two-way repeated-measures ANOVAs were performed in the CONN toolbox to test for the interaction effects of condition (sequence –random blocks) and group (PD –HC). We investigated the functional connectivity between the primary motor cortex and the following regions: the striatum, the cerebellum, the DLPFC, and the hippocampus. We used the method available in CONN for inferences on the ROI-to-ROI connection-level, i.e., a false discovery rate (FDR)-corrected p-value with p < 0.05 as the threshold for significant connections.
Exploratory analyses of the fMRI data
In addition to the analyses within the healthy group described above, we investigated whether any clusters of voxels in the brain were statistically more active during the sequence blocks than the random blocks. We also investigated whether there were functional connectivity measures over and above between the primary cortex and the striatum, that were significantly larger during the sequence blocks than the random blocks within the healthy group. The exploratory analyses of functional connectivity within the healthy group were first done between the primary motor cortex and the cerebellum, the hippocampus and the DLPFC respectively. For this, we used the connection-level FDR-corrected p-value with p < 0.05 as a threshold for significant connections. Secondly, we also investigated the functional connectivity between the primary motor cortex and all other ROIs defined by CONN 20b’s default anatomical atlas within the healthy group. CONN’s default atlas consists of 132 ROIs and is a combination of cortical & subcortical areas from the Harvard-Oxford atlas and cerebellar areas from the Automated anatomical labelling atlas. As for exploratory group comparisons, we investigated whether there were any significant interaction effects of group and condition in functional connectivity between the primary motor cortex and all the ROIs part of the CONN default atlas. For the ROI-to-ROI analyses where the entire CONN atlas was used, we used the Network-Based-Statistics approach to investigate the presence of significant clusters of connectivity as this method is suggested to be suitable for testing large networks of ROIs [28]. For this, we used the default criterion in CONN with a combination of an uncorrected p < 0.001 height threshold to initially define networks of interest, followed by a network-level FDR-corrected p-value using p < 0.05 as the threshold for significance. The analyses described in this paragraph included all ten blocks of the SRTT.
As planned, we also performed exploratory analyses where the functional connectivity for each of the three parts of the SRTT was investigated separately within the two groups using dependent t-tests. We investigated the functional connectivity between the primary cortex and the striatum, the cerebellum, the DLPFC and the hippocampus respectively. We used the connection-level FDR-corrected p-value with p < 0.05 as the threshold for significant connections.
Reproducibility statement
The analysis plan, the R and Matlab scripts, the.mat files used for the CONN analyses, the brain masks, and all files needed to run the SRTT can be downloaded at https://osf.io/abprn/.
Deviations from the preregistration
Because the MLM models for the RT outcome of the SRTT did not converge with a random slope, the random slope was excluded from the MLM models. All statistical analyses of functional connectivity were performed using CONN instead of R, but there was no difference in the methods described in the analysis plan.
RESULTS
Analyses of the reaction time outcomes
For the primary statistical analyses of the RT outcome of the SRTT, i.e., including all trials of the SRTT, there was a statistically significant main effect of group showing that the participants with PD were slower than the healthy participants independent of block type. There was also a statistically significant effect of block type where the sequence blocks rendered lower RTs independent of the group. Lastly, the block by group interaction effect was also statistically significant (b = –9.88, SE = 3.07, p = 0.001). The interaction was in the direction that the PD group showed a lower level of implicit motor sequence learning than the healthy group, as measured by RT. The results for the sensitivity analysis where we included all participants who had performed the SRTT either inside or outside the scanner, were in the same direction as the primary MLM analysis. For more details on the statistical analyses, see the Supplementary Material.
Exploratory analyses of the reaction time outcomes
There were no indices of a correlation between the SRTT performance and the levodopa equivalent dose or that the SRTT performance differed between the participants at Hoehn & Yahr stage II and III (Supplementary Figures 1 and 2).
For the exploratory analyses where the three parts of the SRTT were investigated separately, a group-dependent pattern in the RT outcome was observed. The healthy participants, but not the participants with PD, descriptively had a somewhat lower RT during the sequence trials than the random trials in part 2 of the SRTT. In part 3, both groups descriptively had a lower RT during the sequence trials than the random trials with a difference of approximately the same size for the two groups. See Supplementary Figure 2.
Analyses of the fMRI data
As for the healthy group, we found no statistically significant difference in striatal activity between the sequence and the random blocks (no significant clusters of voxels after correcting for the multiple statistical testing). Neither was there a statistically significant greater functional connectivity between the primary motor cortex and the striatum during the sequence blocks compared to the random blocks for the healthy group (t = 0.20, pFDR = 0.86).
Comparing the two groups, the PD group did not have statistically significantly less task-induced striatal activity than the healthy group. Neither did the PD group have statistically significantly more task-induced activity in the DLPFC, the motor parts of the cerebellum, or the hippocampus than the healthy group. The task-induced functional connectivity between the primary motor cortex and the striatum was not statistically significantly lower for the PD group than the healthy group. Neither was the task-induced functional connectivity between the primary motor cortex and the cerebellum, the hippocampus and the DLPFC respectively, statistically significantly larger for the PD group than the healthy group (Table 2).
Table 2
Between-group estimates of task-induced functional connectivity during the SRTT
Prim. motor cortex - | Prim. motor cortex – | Prim. motor cortex - | Prim. motor cortex - | |
Striatum | Cerebellum, motor parts | Hippocampus | DLPFC | |
F | –0.68 | –3.09 | 0.49 | –0.83 |
pFDR | 0.77 | 0.05 | 0.78 | 0.77 |
Contrasts: The interaction effects of group (HC –PD) and condition (sequence –random blocks). All blocks of the SRTT were included. Prim. motor cortex, primary motor cortex; pFDR, connection-level FDR-corrected p-value.
Exploratory analyses of the fMRI data
We found no clusters of voxels that significantly differed between the sequence and the random blocks for the healthy group. Neither did we find any significant outcome of functional connectivity between the primary motor cortex and our predefined ROIs (the cerebellum, the DLPFC or the hippocampus, Supplementary Table 5) or between the primary motor cortex and all other ROIs in CONN’s default atlas, when contrasting the sequence blocks and the random blocks within the healthy group. Also, we did not find any significant outcome for the interaction analyses of group and condition of functional connectivity between the primary motor cortex and all ROIs of the CONN default atlas.
For the exploratory analyses of functional connectivity conducted within the two groups and for each of the three parts of the SRTT separately, the t-values differed to some extent between the parts of the SRTT and between the groups, but all p-values were non-significant (Table 3).
Table 3
Within-group estimates of task-dependent functional connectivity over time
Prim. motor cortex - | Prim. motor cortex - | Prim. motor cortex - | Prim. motor cortex - | ||||||
striatum | cerebellum | hippocampus | DPLFC | ||||||
PD | HC | PD | HC | PD | HC | PD | HC | ||
part 1 | t | 0.84 | –0.63 | 1.09 | –1.86 | –0.11 | 0.93 | –1.01 | 1.33 |
pFDR | 0.92 | 0.89 | 0.92 | 0.48 | 0.92 | 0.86 | 0.92 | 0.86 | |
part 2 | t | 0.84 | –0.34 | 1.47 | –1.7 | –0.07 | 0.78 | –0.73 | 1.17 |
pFDR | 0.91 | 0.84 | 0.91 | 0.65 | 0.98 | 0.84 | 0.95 | 0.84 | |
part 3 | t | 0.98 | 0.17 | 1.74 | –2.05 | 0.02 | 0.65 | –1.56 | 1.54 |
pFDR | 0.66 | 0.97 | 0.42 | 0.49 | 0.98 | 0.97 | 0.42 | 0.67 |
Contrast: condition (sequence - random blocks). Part 1: random trials from blocks 1 and 4 and sequence trials from blocks 2 and 3, part 2: random trials from blocks 4 and 7 and sequence trials from blocks 5 and 6, part 3: random trials from blocks 7 and 10 and sequence trials from block 8 and 9. Prim. motor cortex, primary motor cortex; pFDR, connection-level FDR-corrected p-value.
DISCUSSION
There was a statistically significant group by block type interaction effect in the RT analysis where all trials of the SRTT were included. The effect was in the direction that the PD group showed less implicit learning than the healthy group. For the exploratory analyses of RT, where the three parts of the SRTT were investigated separately, indications of implicit learning were observed at an earlier phase for the healthy individuals than for the participants with PD. There were no statistically significant group differences in either the within-region analyses or the functional connectivity analyses of the task-induced fMRI data.
The PD group showed statistically significantly less implicit motor sequence learning than the healthy group, which is in line with a meta-analysis of SRTT in people with PD [4], even though the difference found in the present study is considered small. The meta-analysis did however report a wide range of effect sizes in different studies and showed that differences in the experimental set-up, as well as symptom severity, may explain the different effect sizes. It should also be remembered that the PD participants in the present study were tested in the ON state of medication which could affect the results and effect sizes.
Because we also investigated Parts 1 to 3 of the SRTT separately, an exploratory discussion of the different phases of the learning process is possible. As can be observed in Fig. 2, the group difference in implicit motor sequence learning is negligible in part 3 of the SRTT (difference random-sequence PD: 33.5 ms, HC: 32.7 ms) but descriptively larger though still small, in part 2 (difference random-sequence PD: 0.02 ms, HC: 16.55 ms). It has previously been suggested that motor learning in general i.e., undefined if explicit or implicit, is slower in people with PD [3, 29]. Our results are in line with this, i.e., the results suggest that people with mild to moderate PD can reach an equal level of implicit motor sequence learning as healthy individuals, but that they need more time and/or repetitions to do so than healthy individuals in the same age range. Further empirical findings of a lower implicit learning rate would support the common assumption that people with PD need more practice than healthy individuals to learn and adapt motor tasks [29]. A lower implicit learning rate could also partly be a cause of the often-reported impairment of dual-tasking in people with PD [30]. If people with PD need to allocate a lot of time to learn and adapt a motor task, it is plausible that they have less time or resources to perform a second task than healthy individuals, which would manifest as an impairment in dual-tasking [31].
Fig. 2
Implicit motor sequence learning for both groups presented for each part of the SRTT. The point values represent the observed median RTs of the trials in the two blocks included for each point, and the error bars represent the semi-interquartile ranges. PD, participants with Parkinson’s disease; HC, healthy controls. Part 1: random trials from blocks 1 and 4 and sequence trials from blocks 2 and 3, part 2: random trials from blocks 4 and 7 and sequence trials from blocks 5 and 6, part 3: random trials from blocks 7 and 10 and sequence trials from blocks 8 and 9.
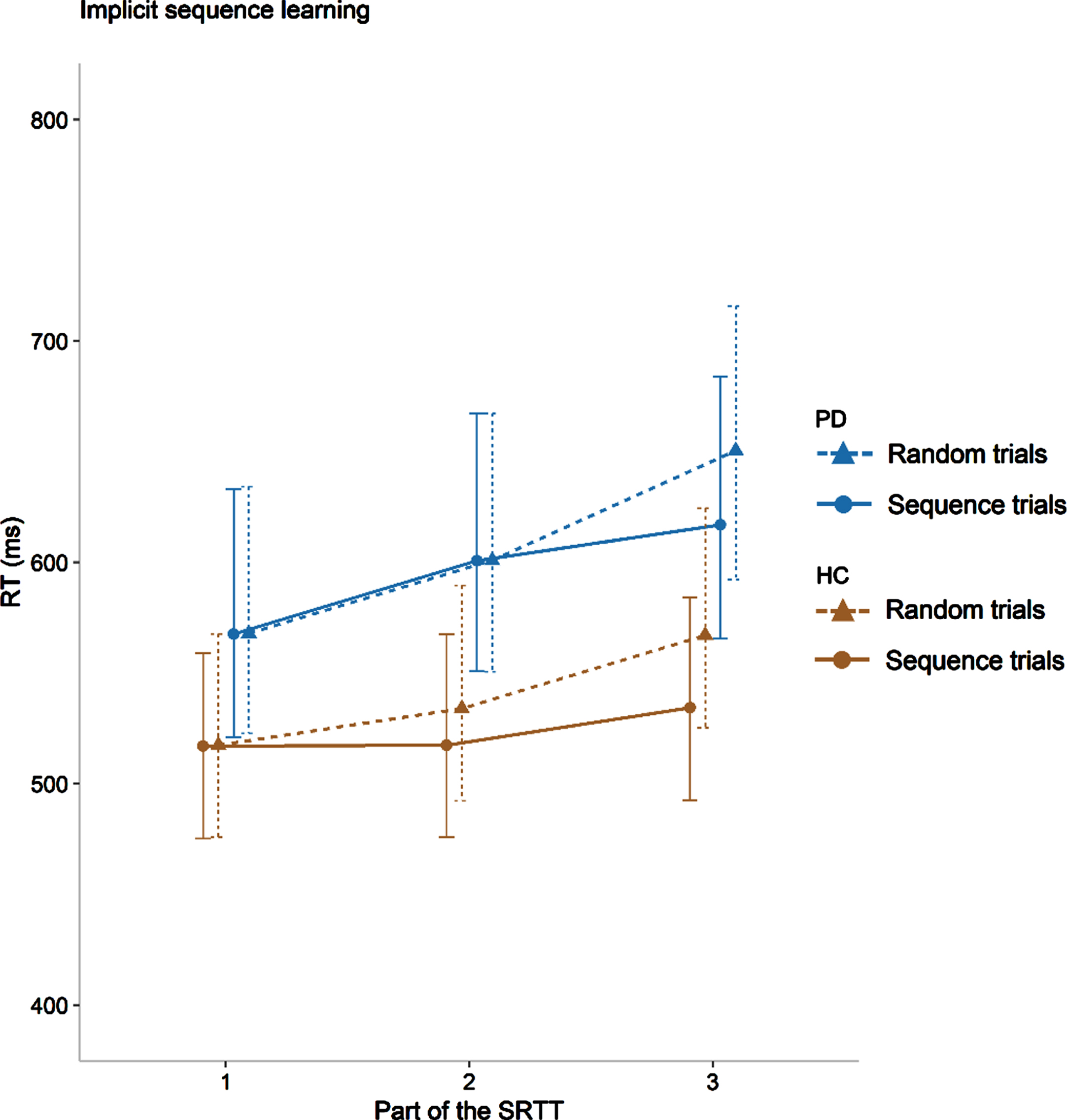
If people with PD have a lower learning rate in implicit learning than healthy individuals, it would also imply that the non-convergent results of previous studies of implicit motor sequence learning in PD, could partly be due to differences in the experimental designs and the analysis methods used. In other words, it is possible that studies using a shorter version of the SRTT, where the participants with PD do not get enough exposure to the sequence, are likely to find that people with PD are impaired at implicit motor sequence learning in comparison to healthy individuals. On the contrary, studies using longer versions of the SRTT might more often find that people with PD show an equal level of implicit learning as healthy individuals. For these reasons, we believe that longer versions of the SRTT should be used and that the analyses should focus not only on the endpoint of the SRTT but also on the learning process.
The absence of statistically significant group differences in the task-induced fMRI data, both for the within-region analyses and the measures of functional connectivity, do not lend support to any of our hypotheses for the fMRI data. However, in a purely exploratory and humble way, we will discuss the results of the analyses of functional connectivity in relation to the model by Doyon and Benali [6] that we based several hypotheses on. A main idea in the model by Doyon and Benali [6] is that the cortico-striatal system increases its activity as the implicit motor sequence learning progresses, at least for healthy individuals. In our study, the healthy group showed a tendency for increased functional connectivity between the primary motor cortex and striatum as the SRTT progressed while this pattern was very small or negligible for the PD group (Table 3). Doyon [7] also suggested that the cerebellum will be more active in people with PD than in healthy individuals, as a form of compensation for the impaired striatal function. In line with this claim, our group by block interaction effect for the functional connectivity between the primary cortex and the cerebellum was the largest estimate of our analyses and had a p-value very close to the significance level (Table 2). The estimate was in the direction of stronger functional connectivity between the primary motor cortex and the cerebellum for the PD group than for the healthy group. Additionally, the estimates of functional connectivity between the primary motor cortex and the cerebellum increased throughout the SRTT for the PD group while the opposite pattern was observed for the healthy group (Table 3). It should again be emphasized that none of these estimates can give robust support to Doyon’s model or our hypotheses as they are not statistically significant. To our knowledge, the present study is the first to test the implications of the model and ideas by Doyon and Benali [6] and Doyon [7] in people with PD, and we think that the results encourage future similar investigations with directed hypotheses and greater statistical power. We also want to briefly discuss that we did not find the expected differences between the random and the sequence blocks in the fMRI data within the healthy group. Possible explanations include a lack of sufficient statistical power to detect these differences or of course that there is no true block-dependent difference in brain activity for older healthy adults during the SRTT.
Strengths of the present study are our unusually large sample size as well as the sample restriction to people with mild to moderate PD which limited disease degree as a confounder of the results. However, a related limitation of the study is the unknown statistical power for both the reaction time and fMRI analyses and that it is plausibly quite low for several outcomes, limiting the reliability of the results. It is difficult to both estimate and achieve a high statistical power, not the least for fMRI outcomes and when studying participants with a heterogenous disease such as PD where participants differ in both symptom expression and type and dosage of medication even with a sample restriction to mild to moderate PD. Future studies could either restrict their samples even further in relation to PD characteristics or aim for very large samples and include disease progression and medication as explanatory variables.
Other strengths of the study are that we investigated the SRTT data both by using all data in one statistical model for increased statistical power in comparison to traditional analyses of the SRTT and, by separating the data into three parts to study the learning process, something not previously done in people with PD. The two analyses complement each other and give a fuller picture than comparing the last random and sequence blocks, which is the most common analysis of the SRTT.
A limitation was that we could not evaluate the awareness of the sequence at the pre-intervention assessment for the PD participants. Lastly, we excluded participants with ≤70% correct trials on the SRTT. It could be tempting to include participants with even less correct answers to try to improve the statistical power but we argue that the quality of the included data is of equal importance as the sample size and that ≤70% correct trials is a reasonable cut-off to maintain data quality and reliability of the analyses. It should also be noted that the cut-off of ≤70% was defined in our preregistration and that other studies have used an even stricter threshold of ≥80% correct trials [32].
In conclusion, we found support for the hypothesis that implicit motor sequence learning is impaired in people with PD compared to healthy individuals even though the impairment was quite small. We saw that a possible explanation of the finding is that people with PD need more time to reach a similar level of implicit motor sequence learning as healthy individuals. If future studies would corroborate that people with PD need more time and repetition for motor learning, it would indicate that this should be an important aspect of the design of exercise and rehabilitation programs for people with PD. Slower motor learning could also be a part-explanation of the commonly reported impairment in dual-task ability for people with PD. We found no statistically significant group differences in the task-induced fMRI data indicating that there either are no group differences or that increased power or different methods than those used here are needed to study the underlying neural correlates.
ACKNOWLEDGMENTS
We would like to thank all our participants for their time. We are grateful to the staff at the MR unit and the Jonasson centre for medical imaging. We would also like to acknowledge Petra Koski for her great work on the logistics of the study including the recruitment, screening and support in assessments. We thank Hanna Johansson, Elvira Granqvist and Sanna Asp for assessments of motor abilities and inclusion criteria and Alexander V. Lebedev and Martin Lövdén for help in developing the study design. We also thank Heather Martin for her invaluable help with the MR safety decisions.
The trial was made possible by funds from the Swedish Research Council (2016–01965), the Parkinson’s Research Foundation, the Swedish Parkinson Foundation as well as the Regional Agreement on Medical Training and Clinical Research (ALF) and the Center for Innovative Medicine (CIMED) - Karolinska Institutet and Region Stockholm. The Research School in Health Care Science and the Strategic Research Area Health Care Science at Karolinska Institutet also provided funding. A draft of this article has been published as part of the PhD thesis of the first author M. F. [33].
CONFLICT OF INTEREST
The authors have no conflict of interest to report.
SUPPLEMENTARY MATERIAL
[1] The supplementary material is available in the electronic version of this article: https://dx.doi.org/10.3233/JPD-223480.
REFERENCES
[1] | Diedrichsen J , Kornysheva K ((2015) ) Motor skill learning between selection and execution, Trends Cogn Sci 19: , 227–233. |
[2] | Marinelli L , Quartarone A , Hallett M , Frazzitta G , Ghilardi MF ((2017) ) The many facets of motor learning and their relevance for Parkinson’s disease, Clin Neurophysiol 128: , 1127–1141. |
[3] | Olson M , Lockhart TE , Lieberman A ((2019) ) Motor learning deficits in Parkinson’s disease (PD) and their effect on training response in gait and balance: A narrative review, Front Neurol 10: , 62. |
[4] | Clark GM , Lum JA , Ullman MT ((2014) ) A meta-analysis and meta-regression of serial reaction time task performance in Parkinson’s disease, Neuropsychology 28: , 945–958. |
[5] | Kalia LV , Lang AE ((2015) ) Parkinson’s disease, Lancet 386: , 896–912. |
[6] | Doyon J , Benali H ((2005) ) Reorganization and plasticity in the adult brain during learning of motor skills, Curr Opin Neurobiol 15: , 161–167. |
[7] | Doyon J ((2008) ) Motor sequence learning and movement disorders, Curr Opin Neurol 21: , 478–483. |
[8] | Brown RG , Jahanshahi M , Limousin-Dowsey P , Thomas D , Quinn NP , Rothwell JC ((2003) ) Pallidotomy and incidental sequence learning in Parkinson’s disease, Neuroreport 14: , 21–24. |
[9] | Schendan HE , Tinaz S , Maher SM , Stern CE ((2013) ) Frontostriatal and mediotemporal lobe contributions to implicit higher-order spatial sequence learning declines in aging and Parkinson’s disease, Behav Neurosci 127: , 204–221. |
[10] | Werheid K , Zysset S , Müller A , Reuter M , von Cramon DY ((2003) ) Rule learning in a serial reaction time task: An fMRI study on patients with early Parkinson’s disease, Cogn Brain Res 16: , 273–284. |
[11] | Albrecht F , Pereira JB , Mijalkov M , Freidle M , Johansson H , Ekman U , Westman E , Franzén E ((2021) ) Effects of a highly challenging balance training program on motor function and brain structure in Parkinson’s disease, J Parkinsons Dis 11: , 2057–2071. |
[12] | Franzen E , Johansson H , Freidle M , Ekman U , Wallen MB , Schalling E , Lebedev A , Lovden M , Holmin S , Svenningsson P , Hagstromer M ((2019) ) The EXPANd trial: Effects of exercise and exploring neuroplastic changes in people with Parkinson’s disease: A study protocol for a double-blinded randomized controlled trial, BMC Neurol 19: , 280. |
[13] | Freidle M , Johansson H , Ekman U , Lebedev AV , Schalling E , Thompson WH , Svenningsson P , Lövdén M , Abney A , Albrecht F , Steurer H , Leavy B , Holmin S , Hagströmer M , Franzén E ((2022) ) Behavioural and neuroplastic effects of a double-blind randomised controlled balance exercise trial in people with Parkinson’s disease, NPJ Parkinsons Dis 8: , 12. |
[14] | Hoehn MM , Yahr MD ((1967) ) Parkinsonism: Onset, progression, and mortality, Neurology 17: , 427–427. |
[15] | Nasreddine ZS , Phillips NA , Bédirian V , Charbonneau S , Whitehead V , Collin I , Cummings JL , Chertkow H ((2005) ) The Montreal Cognitive Assessment, MoCA: A brief screening tool for mild cognitive impairment, J Am Geriatr Soc 53: , 695–699. |
[16] | Luis CA , Keegan AP , Mullan M ((2009) ) Cross validation of the Montreal Cognitive Assessment in community dwelling older adults residing in the Southeastern US, Int J Geriatr Psychiatry 24: , 197–201. |
[17] | Svenningsson P , Westman E , Ballard C , Aarsland D ((2012) ) Cognitive impairment in patients with Parkinson’s disease: Diagnosis, biomarkers, and treatment, Lancet Neurol 11: , 697–707. |
[18] | Vandenbossche J , Deroost N , Soetens E , Coomans D , Spildooren J , Vercruysse S , Nieuwboer A , Kerckhofs E ((2013) ) Impaired implicit sequence learning in Parkinson’s disease patients with freezing of gait, Neuropsychology 27: , 28–36. |
[19] | Muslimovic D , Post B , Speelman JD , Schmand B ((2007) ) Motor procedural learning in Parkinson’s disease, Brain 130: , 2887–2897. |
[20] | Sarazin M , Deweer B , Merkl A , Von Poser N , Pillon B , Dubois B ((2002) ) Procedural learning and striatofrontal dysfunction in Parkinson’s disease, Mov Disord 17: , 265–273. |
[21] | Nissen MJ , Bullemer P ((1987) ) Attentional requirements of learning - evidence from performance-measures, Cognitive Psychol 19: , 1–32. |
[22] | Luce RD (1986) Response Times: Their Role in Inferring Elementary Mental Organization, Oxford University Press, Oxford, UK. |
[23] | Esteban O , Ciric R , Finc K , Blair R , Markiewicz CJ , Moodie CA , Kent JD , Goncalves M , DuPre E , Gomez DEP , Ye Z , Salo T , Valabregue R , Amlien IK , Liem F , Jacoby N , Stojić H , Cieslak M , Urchs S , Halchenko YO , Ghosh SS , De La Vega A , Yarkoni T , Wright J , Thompson WH , Poldrack RA , Gorgolewski KJ ((2020) ) Analysis of task-based functional MRI data preprocessed with fMRIPrep, Nat Protoc 15: , 2186–2202. |
[24] | Esteban O , Birman D , Schaer M , Koyejo OO , Poldrack RA , Gorgolewski KJ ((2017) ) MRIQC: Advancing the automatic prediction of image quality in MRI from unseen sites, PLoS One 12: , e0184661. |
[25] | Ashburner J , Barnes J , Chen C , Daunizeau J , Flandin G , Friston K , Jafarian A , Kiebel S , Kilner J , Litvak V , Moran R , Penny W , Razi A , Stephan K , Tak S , Zeidman P , Gitelman D , Henson R , Hutton C , Glauche V , Mattout J , Phillips C (2014), SPM Manual. |
[26] | Whitfield-Gabrieli S , Nieto-Castanon A ((2012) ) : A functional connectivity toolbox for correlated and anticorrelated brain networks, Brain Connect 2: , 125–141. |
[27] | Tzvi E , Munte TF , Kramer UM ((2014) ) Delineating the cortico-striatal-cerebellar network in implicit motor sequence learning, Neuroimage 94: , 222–230. |
[28] | Zalesky A , Fornito A , Bullmore ET ((2010) ) Network-based statistic: Identifying differences in brain networks, Neuroimage 53: , 1197–1207. |
[29] | Nieuwboer A , Rochester L , Müncks L , Swinnen SP ((2009) ) Motor learning in Parkinson’s disease: Limitations and potential for rehabilitation, Parkinsonism Relat Disord 15: , S53–S58. |
[30] | Raffegeau TE , Krehbiel LM , Kang N , Thijs FJ , Altmann LJP , Cauraugh JH , Hass CJ ((2019) ) A meta-analysis: Parkinson’s disease and dual-task walking, Parkinsonism Relat Disord 62: , 28–35. |
[31] | Kelly VE , Eusterbrock AJ , Shumway-Cook A ((2012) ) A review of dual-task walking deficits in people with Parkinson’s disease: Motor and cognitive contributions, mechanisms, and clinical implications, Parkinsons Dis 2012: , 918719. |
[32] | Rieckmann A , Fischer H , Backman L ((2010) ) Activation in striatum and medial temporal lobe during sequence learning in younger and older adults: Relations to performance, Neuroimage 50: , 1303–1312. |
[33] | Freidle M (2022) Motor and cognitive abilities in Parkinson’s disease with a brain activity perspective: Performance at baseline and the effects of a balance training program (Thesis). Department of Neurobiology, Care Sciences and Society, Karolinska Institutet, Stockholm, Sweden. |
[34] | Rolls ET , Huang CC , Lin CP , Feng J , Joliot M ((2020) ) Automated anatomical labelling atlas 3, Neuroimage 206: , 116189. |
[35] | Mayka MA , Corcos DM , Leurgans SE , Vaillancourt DE ((2006) ) Three-dimensional locations and boundaries of motor and premotor cortices as defined by functional brain imaging: A meta-analysis, Neuroimage 31: , 1453–1474. |
[36] | Shirer WR , Ryali S , Rykhlevskaia E , Menon V , Greicius MD ((2012) ) Decoding subject-driven cognitive states with whole-brain connectivity patterns, Cereb Cortex 22: , 158–165. |