Challenges of Incorporating Digital Health Technology Outcomes in a Clinical Trial: Experiences from PD STAT
Abstract
Digital health technologies (DHTs) have great potential for use as clinical trial outcomes; however, practical issues need to be addressed in order to maximise their benefit. We describe our experience of incorporating two DHTs as secondary/exploratory outcome measures in PD STAT, a randomised clinical trial of simvastatin in people with Parkinson’s disease. We found much higher rates of missing data in the DHTs than the traditional outcome measures, in particular due to technical and software difficulties. We discuss methods to address these obstacles in terms of protocol design, workforce training and data management.
INTRODUCTION
Digital health technologies (DHTs) encompass a broad set of tools, such as wearable sensors, smartphone applications, and computer tasks, which generate digital data relevant to health. Compared to clinical rating scales, participant questionnaires and other traditional health data outcome measures, they have a number of potential advantages. These include objectivity, precision, scalability, continuous data collection, and ability to test remotely—a particular advantage in the current pandemic. DHTs are increasingly being used in clinical trials of neurodegenerative disease, especially Parkinson’s disease (PD), and the majority of pharmaceutical companies plan to incorporate them in future trials [1]. However, their use in clinical trial settings comes with a number of considerations and practical issues that are distinct from traditional clinical trial outcome measures, including unfamiliarity with platforms, connectivity difficulties and lack of data visibility. Here we describe our experience of using two digital measures (Bradykinesia-Akinesia Incoordination (BRAIN) Tap Test (BTT) [2]; PD Monitor (ClearSky Medical Diagnostics Ltd., York, UK) [3]) within a clinical trial in terms of data completeness achieved and the challenges experienced.
DIGITAL MEASURES
BRAIN (Bradykinesia-Akinesia Incoordination) Tap Test (BTT)
The BTT is an online keyboard finger-tapping task administered via a QWERTY keyboard. Participants are asked to alternately tap the ‘s’ and ‘;’ keys as fast and as accurately as possible for 30 s with each hand in turn. Software compatibility was an issue for implementation in previous studies, but there were no issues with data capture [2, 4].
PD Monitor (ClearSky Medical Diagnostics Ltd., York, UK)
The PD Monitor records finger and thumb movements in 3D space by using two small electromagnetic tracking sensors (Polhemus, VT, USA), one each attached to a participant’s thumb and forefinger, and an electromagnetic source connected to a nearby computer (Fig. 1). Each hand is recorded separately. The movements recorded are the same as the Movement Disorder Society Unified Parkinson’s Disease Rating Scale (MDS-UPDRS) upper limb bradykinesia items (finger tapping, hand opening, hand pronation-supination) and rest tremor items. PD Monitor has previously been evaluated in numerous clinical studies worldwide [3, 5–7]. In earlier studies, problems were encountered with the correct initialization of the equipment, rectified by improving the Instructions For Use and clearly labeling equipment to avoid misconfiguration; there were no issues with data capture.
Fig. 1
PD Monitor equipment showing electromagnetic sensors attached to participant’s thumb and forefinger that measure movements in 3D space, in relation to an electromagnetic source (box).
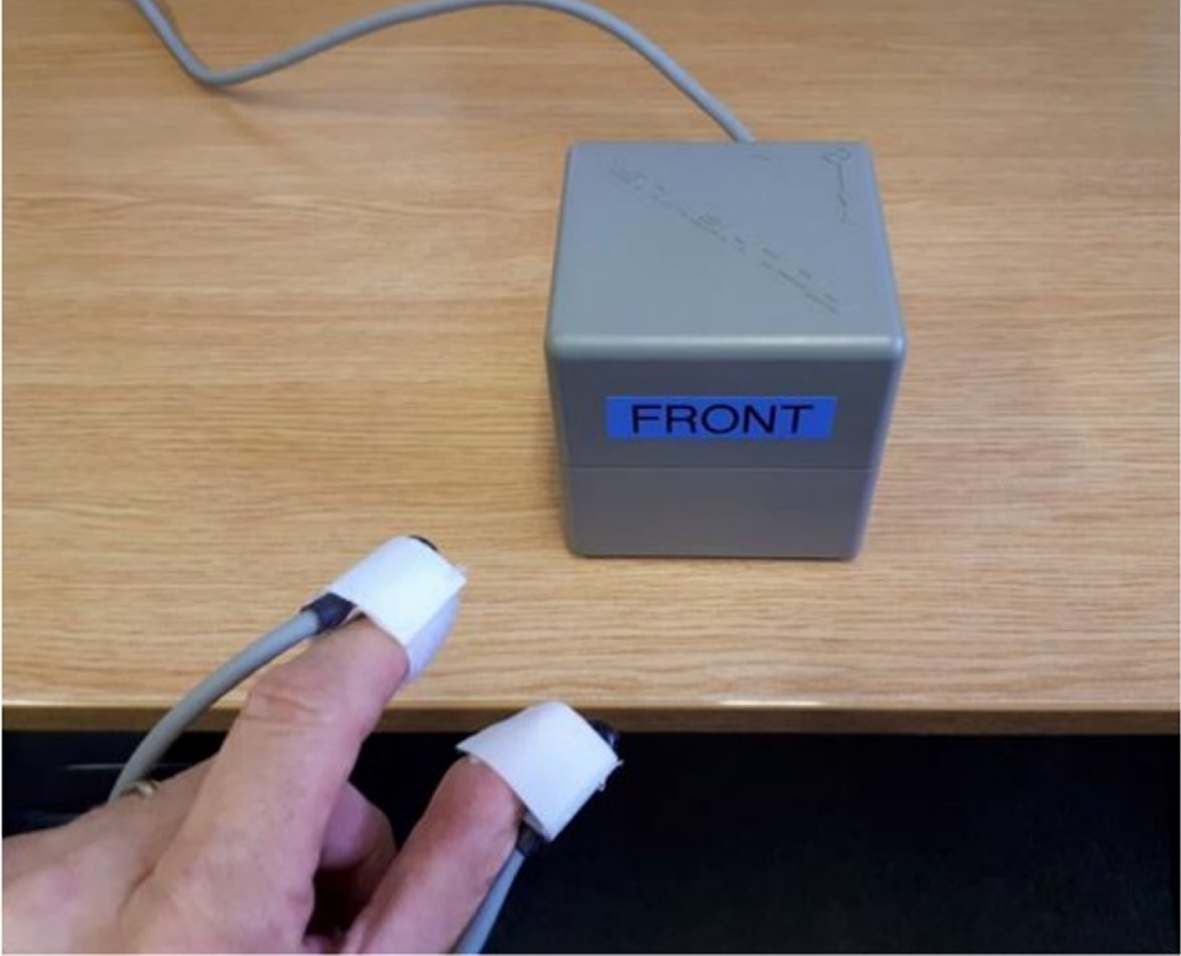
TRIAL SETTING
The PD STAT study was a UK-based multicentre randomised clinical trial that recruited 235 participants from 23 sites between March 2016 and May 2020 [8]. It assessed the neuroprotective potential of simvastatin versus placebo in people with mild-moderate PD. Participants were evaluated over 26 months. The primary outcome was change in the MDS-UPDRS part III motor subscale score [9] in the practically-defined off-medication (OFF) state, which at the time of conducting the trial was generally considered the gold standard measure of disease severity. Planned secondary motor outcomes included other elements of the MDS-UPDRS, a 10 m timed walk and BTT, the number of key taps being the reported outcome. PD Monitor was incorporated as an additional exploratory digital motor outcome of upper limb bradykinesia and tremor, added to the protocol after study initiation in the seven highest recruiting PD STAT study centres.
IMPLEMENTATION OF DIGITAL MEASURES
Both digital measures were supervised tasks administered by the research team during scheduled study visits.
A unique single-use passcode (‘token’) was generated for each participant and used by the research staff to log in to the BTT website portal. Training was provided to site staff by the Clinical Trials Unit (CTU) team at remote site initiation visits, at regular site retraining video conferences and by way of written instructions. BTT was administered to participants in the OFF state at the baseline, 12-, 24-, and 26-month visits alongside the 10 m walk test and the MDS-UPDRS part III. Data were downloaded at the coordinating Peninsula CTU, with data completeness monitored contemporaneously by CTU staff and reasons for missing data documented.
PD Monitor data were collected in both ON and OFF states. Staff training was provided in person by the PD Monitor team when the equipment was delivered to the sites and telephone/video support was offered at regular intervals. Data were collected at the 12-month and either the 24- or 26-month visits. Data were uploaded to a secure cloud-storage database and analysed by the data research team separate to the CTU team.
DATA COMPLETENESS
Data completeness for the motor outcomes (primary, timed walk and digital) are presented in Table 1, with reasons for missing digital data detailed in Table 2. BTT data were available for 69–85% of participants across the different visits, compared with 79–100% and 79–98% for the MDS-UPDRS part III and 10m timed walk test respectively. The most common reason for missing BTT data was blocking of the BTT website by firewalls within study centres. Data were also lost due to an unavoidable change in the software provider.
Table 1
Data completeness of motor assessments conducted at various time points in PD STAT. Figures provided are absolute numbers of participant data collected/participants available (%)
Outcome measure | Clinic visits | |||||
Baseline | 12-month | 24-month | 26-month | |||
Traditional | UPDRS III –OFF | 228/228 (100) | 198/205 (97) | 178/193 (92) | 146/185 (79) | |
10m walk | 223/228 (98) | 196/205 (96) | 168/193 (87) | 146/185 (79) | ||
Digital | BTT | 193/228 (85) | 172/205 (84) | 154/193 (80) | 128/185 (69) | |
PD Monitor | OFF | ND | 17/80 (21) | 21/56* (38) | ||
ON | ND | 56/80 (70) | 34/56* (61) |
UPDRS III, Movement Disorder Society Unified Parkinson’s Disease Rating Scale part III motor subscale score; OFF, off-medication state; ON, on-medication state; BTT, BRAIN (Bradykinesia-Akinesia Incoordination) Tap Test; ND, not done; *PD Monitor data were scheduled to be collected at either the 24- or 26-month visit.
Table 2
Reasons for digital motor measures data unavailability in PD STAT
Category | Reason | Number assessments impacted | |
BTT | Accessing DHT portal | Technical issues/security access | 36 |
Change of licence | 28 | ||
No access token | 11 | ||
Data collection | Virtual visit due to COVID-19 | 29 | |
Home visit – no keyboard/internet | 15 | ||
Data management | Data not downloaded | 27 | |
Participant-related | Participant in ON state | 1 | |
Participant declined | 1 | ||
Other | 16 | ||
Total missing/total available (%) | 164/811 (20.2%) | ||
PD Monitor | Data collection | Home visit – equipment not available | 36 |
Virtual visit due to COVID-19 | 26 | ||
Staff failed to use device in clinic | 20 | ||
Data management | OFF state assessment data overwritten by ON state assessment data | 50 | |
Other | 10 | ||
Total missing/total available (%) | 142/272 (52.2%) |
BTT, BRAIN tap test; OFF, off-medication state; ON, on-medication.
PD Monitor measurements were scheduled to be taken from 80 participants with 56/80 (70%) completing the task at the 12-month visit. OFF measurements at the 12-month visit were lost in 39/56 (70%) participants due to a failed software update which meant that the OFF state measures were inadvertently overwritten rather than retained. Measurements at the 24/26-month visits were missed mainly due to home visits, visits undertaken remotely due to the COVID-19 pandemic and failure of staff to use the equipment in the clinic.
DISCUSSION
DHTs hold much promise in terms of enriched trial data and more inclusive research. However, our experience of incorporating DHTs into our clinical trial, PD STAT, has identified simple, practical challenges to digital data collection that impacted data completeness.
In PD STAT we incorporated two digital measures which were deployed in the in-clinic supervised environment, with less risk in terms of external sources of variability (e.g., undertaking tasks unsupervised in the home environment) and concerns related to data attribution. Nevertheless, despite the apparently lower risk locality and method of deployment, we still experienced significant impact on data capture.
One of our digital measures, the BTT, has been widely used in other studies, primarily to facilitate data capture from participants unsupervised in their own homes, for example in a longitudinal study identifying people at risk of PD [4]. The other DHT, PD Monitor, previously validated in PD as a supervised test of upper limb bradykinesia and tremor [3], was incorporated within the protocol as an additional substudy to facilitate its further evaluation as a motor measure, as well as to assess its feasibility as an outcome measure in a randomised clinical trial. Our experience with these measures has highlighted learnings for DHT deployment in relation to protocol design, workforce training and data management.
Protocol design
When selecting DHTs for use in trials, it is important to ensure the DHT is valid for assessment of the outcome of interest and can be feasibly deployed in the intended trial environment [10], including any costs for technology support or further development. Given the successful prior largescale use of BTT, we did not anticipate the problems we encountered with organisational firewalls and connectivity in study centres (which were mostly in NHS hospitals)—issues that were not found to be relevant to use of the measure in the home. Had we undertaken feasibility assessments across a few pilot sites, we may have identified these issues and built mitigation into the protocol. Implementing the PD Monitor within a study protocol that had already started meant that opportunities for robust feasibility testing were limited and some of the risk mitigation strategies (such as incorporating DHT reminders in the data-capture documentation) were not in place.
Our protocol was amended during the PD STAT study to allow for home visits as a means of reducing study burden for participants; however, the impact of this amendment on the DHT outcomes was a further reduction in data capture due to additional hardware requirements (e.g., a QWERTY keyboard for BTT, transporting the PD Monitor to participants’ homes) and connectivity issues. These difficulties were compounded when visits were conducted remotely as a result of the COVID-19 pandemic. Home and remote visits are increasingly utilised to support retention, particularly for frail participants and those more remotely located from the study site. However, those in rural or economically deprived localities are more likely to experience connectivity challenges. Failure to anticipate these challenges could therefore bias data collected utilising DHTs.
Workforce training
The FDA requires all those responsible for data capture using mobile technologies to have adequate training, education and experience [11]. Training was provided at site initiation and/or deployment of each of the technologies. Additional training was available on request for new staff and as a means of providing updates. However, despite this, some data losses were due to misunderstandings relating to the method of DHT deployment (in the case of BTT, the use of tokens for participant identification and the means by which these were requested), or due to lack of engagement with the DHT (for example, forgetting to take the PD Monitor device to clinic rooms for the study visits). It is important to ensure that site staff are well trained in technologies to be deployed, with easy access to relevant training and technical support. This is particularly important for longer duration studies such as ours where staff turnover at sites is likely to be encountered. Utilisation of training devices and practice runs prior to study initiation would be useful. Co-design of DHTs with study staff and patients to ensure maximum usability would help mitigate this risk.
Data management
The use of DHTs opens the possibility of centralised data capture and monitoring, with provision of technical support in real time, as well as potential for data quality and completeness monitoring to be automated with programmed alerts. Clinical Trials Transformation Initiative recommendations include presentation of DHT-captured data to investigators at sites, in order to support discharge of their oversight responsibilities with regard to data integrity [12]. In our study, data from neither measure was visible to study site investigators. However, the BTT data were visible to the central trial co-ordinating team, which allowed for reasons for missing data to be explored and mitigated, such as by provision of additional staff training. The PD Monitor data were not visible to the investigators or the central trial team, as data were uploaded directly to the DHT development team. The DHT data analysts had insufficient understanding of the study protocol to appreciate that data capture errors had occurred due to a software malfunction. Ensuring clear communication with a shared understanding of the data management plan would have prevented this data loss with the PD Monitor in our study.
CONCLUSION
DHTs hold significant promise as outcome measures in clinical trials. We have identified challenges with their deployment that limit data completeness. Ensuring appropriate workforce training, pilot evaluation in study sites and data visibility at sites and the central co-ordinating team are mitigations that could be considered in order for the benefits of DHTs to be fully realised.
CONFLICT OF INTEREST
SS is a director and shareholder of ClearSky Medical Diagnostics Ltd. JA is a shareholder of ClearSky Medical Diagnostics Ltd. AJN developed the current version of the BRAIN test.
REFERENCES
[1] | Artusi CA , Mishra M , Latimer P , Vizcarra JA , Lopiano L , Maetzler W , Merola A , Espay AJ ((2018) ) Integration of technology-based outcome measures in clinical trials of Parkinson and other neurodegenerative diseases. Parkinsonism Relat Disord 46: Suppl 1, S53–S56. |
[2] | Noyce AJ , Nagy A , Acharya S , Hadavi S , Bestwick JP , Fearnley J , Lees AJ , Giovannoni G ((2014) ) Bradykinesia-akinesia incoordination test: Validating an online keyboard test of upper limb function. PLoS One 9: , e96260. |
[3] | Gao C , Smith S , Lones M , Jamieson S , Alty J , Cosgrove J , Zhang P , Liu J , Chen Y , Du J , Cui S , Zhou H , Chen S ((2018) ) Objective assessment of bradykinesia in Parkinson’s disease using evolutionary algorithms: Clinical validation. Transl Neurodegener 7: , 18. |
[4] | Noyce AJ , Bestwick JP , Silveira-Moriyama L , Hawkes CH , Knowles CH , Hardy J , Giovannoni G , Nageshwaran S , Osborne C , Lees AJ , Schrag A ((2014) ) PREDICT-PD: Identifying risk of Parkinson’s disease in the community: Methods and baseline results. J Neurol Neurosurg Psychiatry 85: , 31–37. |
[5] | Lones MA , Smith SL , Tyrrell AM , Alty JE , Jamieson DRS ((2013) ) Characterising neurological time series data using biologically motivated networks of coupled discrete maps. BioSystems 112: , 94–101. |
[6] | Lones MA , Smith SL , Alty JE , Lacy SE , Possin KL , Jamieson DRS , Tyrrell AM ((2013) ) Evolving classifiers to recognize the movement characteristics of Parkinson’s disease patients. IEEE Trans Evol Comput 18: , 559–576. |
[7] | Lones MA , Alty JE , Lacy SE , Jamieson DRS , Possin KL , Schuff N , Smith SL (2013) Evolving classifiers to inform clinical assessment of Parkinson’s disease. 2013 IEEE Symposium on Computational Intelligence in Healthcare and e-health (CICARE), pp. 76-82. |
[8] | Carroll CB , Webb D , Stevens KN , Vickery J , Eyre V , Ball S , Wyse R , Webber M , Foggo A , Zajicek J , Whone A , Creanor S ((2019) ) Simvastatin as a neuroprotective treatment for Parkinson’s disease (PD STAT): Protocol for a double-blind, randomised, placebo-controlled futility study. BMJ Open 9: , e029740. |
[9] | Goetz CG , Tilley BC , Shaftman SR , Stebbins GT , Fahn S , Martinez-Martin P , Poewe W , Sampaio C , Stern MB , Dodel R , Dubois B , Holloway R , Jankovic J , Kulisevsky J , Lang AE , Lees A , Leurgans S , LeWit PA , Nyenhuis D , Warren Olanow C , Rascol O , Schrag A , Teresi JA , van Hilten JJ , LaPelle N , Movement Disorder Society UPDRS Revision Task Force ((2008) ) Movement Disorder Society-sponsored revision of the Unified Parkinson’s Disease Rating Scale (MDS-UPDRS): Scale presentation and clinimetric testing results. Mov Disord 23: , 2129–2170. |
[10] | Coran P , Goldsack JC , Grandinetti CA , Bakker JP , Bolognese M , Dorsey ER , Vasisht K , Amdur A , Dell C , Helfgott J , Kirchoff M , Miller CJ , Narayan A , Patel D , Peterson B , Ramirez E , Schiller D , Switzer T , Wing L , Forrest A , Doherty A ((2019) ) Advancing the use of mobile technologies in clinical trials: Recommendations from the Clinical Trials Transformation Initiative. Digit Biomark 3: , 145–154. |
[11] | US Food and Drug Administration (2021); Title 21—Food and Drugs; Chapter 1 –Food and Drug Administration, Department of Health and Human Services, Subchapter A –General; Part 11 –Electronic records; electronic signatures; Subpart B –Electronic records; Sec 11.10 Controls for closed systems., CFR - Code of Federal Regulations Title 21, https://www.accessdata.fda.gov/scripts/cdrh/cfdocs/cfcfr/cfrsearch.cfm?fr=11.10, Current as of 1 October 2021, Accessed 3 January 2022. |
[12] | Clinical Trials Transformation Initiative (2021) Recommendations for Managing Data, Digital Health Trials, https://ctti-clinicaltrials.org/wp-content/uploads/2021/07/CTTIManagingDataRecommendations.pdf, Posted July 2021, Accessed 8 December 2021. |