Diffusion MRI Captures White Matter Microstructure Alterations in PRKN Disease
Abstract
Background:
Although pathological studies usually indicate pure dopaminergic neuronal degeneration in patients with parkin (PRKN) mutations, there is no evidence to date regarding white matter (WM) pathology. A previous diffusion MRI study has revealed WM microstructural alterations caused by systemic oxidative stress in idiopathic Parkinson’s disease (PD), and we found that PRKN patients have systemic oxidative stress in serum biomarker studies. Thus, we hypothesized that PRKN mutations might lead to WM abnormalities.
Objective:
To investigate whether there are WM microstructural abnormalities in early-onset PD patients with PRKN mutations using diffusion tensor imaging (DTI).
Methods:
Nine PRKN patients and 15 age- and sex-matched healthy controls were recruited. DTI measures were acquired on a 3T MR scanner using a b value of 1,000 s/mm2 along 32 isotropic diffusion gradients. The DTI measures were compared between groups using tract-based spatial statistics (TBSS) analysis. Correlation analysis was also performed between the DTI parameters and several serum oxidative stress markers obtained in a previously conducted metabolomic analysis.
Results:
Although the WM volumes were not significantly different, the TBSS analysis revealed a corresponding decrease in fractional anisotropy and an increase in mean diffusivity and radial diffusivity in WM areas, such as the anterior and superior corona radiata and uncinate fasciculus, in PRKN patients compared with controls. Furthermore, 9-hydroxystearate, an oxidative stress marker, and disease duration were positively correlated with several parameters in PRKN patients.
Conclusion:
This pilot study suggests that WM microstructural impairments occur in PRKN patients and are associated with disease duration and oxidative stress.
INTRODUCTION
Mutations in the gene encoding parkin (PRKN) are the most common genetic cause of autosomal recessive familial Parkinson’s disease (PD) [1]. Early-onset parkinsonism, a marked response to levodopa treatment, and dystonia and hyperreflexia are characteristic features of patients with PRKN mutation-associated autosomal recessive PD (hereafter referred to as PRKN patients). However, there are no specific clinical signs that distinguish PRKN patients from patients with idiopathic PD (iPD) [2]. Selective dopaminergic neuronal degeneration in the substantia nigra pars compacta (SNc) is considered a characteristic pathological finding of PRKN patients. Furthermore, several studies have reported that PRKN patients have a severe loss of dopaminergic neurons in the SNc, and a mild or moderate loss of locus coeruleus neurons with or without Lewy bodies, which are pathological hallmarks of iPD [3]. However, whereas iPD patients have cortical atrophy in the course of the disease, most PRKN patients do not have any cortical atrophy [4, 5]. It is therefore controversial whether the clinicopathological features of PRKN patients are in line with those of iPD.
Parkin protein functions as an E3 ubiquitin ligase in the ubiquitin–proteasome system (UPS), which monoubiquitinates and polyubiquitinates target proteins [6]. The UPS is a protein degradation system, and Lewy bodies stain strongly for anti-ubiquitin antibodies; therefore, parkin dysfunction may contribute to the pathomechanisms of dopaminergic neuronal degeneration. In addition, many groups, including our own, have revealed that parkin also participates in the quality control of damaged mitochondria, collaborating with PTEN-induced kinase 1 (PINK1) [7]. Impairment of the mitochondrial quality control system may induce higher oxidative stress. We have previously demonstrated elevated serum levels of several oxidative stress-related markers in PRKN patients, suggesting a systemic hyperoxidative state [8, 9]. Additionally, several studies have reported that oxidative stress might perturb white matter microstructures [10, 11]. Thus, we hypothesized that parkin dysfunction might be associated with brain and systemic oxidative stress, resulting in white matter alterations. In the present study, we investigated white matter microstructures in PRKN patients using diffusion tensor imaging (DTI) methods, which are useful for evaluating detailed microstructures when there are only subtle impairments [12, 13]. Furthermore, we analyzed the correlations between alterations in white matter microstructures and a range of serum oxidation-related markers, which were determined from a previously reported metabolomic analysis [8].
MATERIALS AND METHODS
Participants
The target populations were PRKN patients and healthy controls (HCs) without any neurodegenerative diseases. We enrolled 10 PRKN patients, but one female patient (age: 47, disease duration: 18 years, Unified PD Rating Scale [UPDRS] part III: 12 points, Hoehn & Yahr [H&Y]: 2, mutation: homozygous exon 3 deletion) was excluded because she had severe hypothyroidism. Thus, the participants consisted of nine PRKN patients (five females) and 15 age-matched HCs (six females). All of the PRKN patients had participated in the previous serum metabolomic study [8]. The characteristics (sex, age, disease duration, UPDRS part III, H&Y stage, levodopa equivalent daily dose, white matter volume, and PRKN mutations) of the patients and HCs are shown in Table 1. The levodopa equivalent daily dose was calculated on the basis of a previously reported systematic review [14]. All PRKN patients had been treated as outpatients at Juntendo University in Tokyo, Japan. The HCs were the patients’ spouses or volunteers who were free of neurological and psychiatric illnesses. Three movement disorder specialists (T.K., T.H., and N.H.) performed clinical assessments of all PRKN patients. Genetic analysis revealed that all PRKN patients carried homozygous or compound heterozygous mutations in PRKN. The detailed methods, materials, and results of the genetic and metabolomic analyses have been described previously [1, 15]. Data were collected between March 2014 and April 2016.
Table 1
Demographic and clinical characteristics of participants in the metabolomic study
HCs | PARK2 | p | ||
Numbers (Female:Male) | 15 (6 : 9) | 9 (5 : 4) | 0.46‡ | |
Age, Mean (SD) | 55.2 (±20.7) | 58.3 (±14.1) | 0.68‡‡ | |
Disease Duration (y), Mean (SD) | N.A. | 27.5 (±11.9) | ||
*UPDRS part III, Mean (SD) | N.A. | 13.7 (±5) | ||
Hoehn &Yahr | N.A. | 2.4 (±0.7) | ||
**LEDD (mg), Mean (SD) | N.A. | 982.7 (±362.2) | ||
Normalized white matter volume | 0.29 (0.041) | 0.30 (0.045) | 0.67‡‡ | |
Participants with Cerebrovascular | 7 | 3 | 0.52‡ | |
Risk Factors (n) | [Arterial hypertension (5), Dyslipidemia (3), Type2 diabetes mellitus (1), Internal carotid artery stenosis (1), Minor hemorrhagic stroke (1), White matter ischemia (2)] | [Arterial hypertension (2) Type2 diabetes mellitus (1), White matter ischemia (2)] | ||
Parkin mutations | N.A. | Exon 4 deletion (Homozygous) | ||
Exon 5 deletion (Homozygous) | ||||
Exon 2–4 deletion (Homozygous) (2 patients) | ||||
Exon 6–7 deletion (Homozygous) | ||||
Exon 6 duplication (Homozygous) | ||||
Exon 2,3 deletion / c.1358G>A (compound heterozygous) | ||||
Exon3–7 duplication/Exon 3–5 deletion (compound heterozygous) | ||||
Exon 3 deletion/c.535-3A>G (compound heterozygous) |
‡Chi-squared test, ‡‡ Student’s t-test HCs, healthy controls; LEDD, levodopa equivalent daily dose; N.A., not applicable; UPDRS, Unified Parkinson’s Disease Rating Scale.
Ethics statement
Written informed consent was acquired from all subjects participating in this study, in accordance with the Declaration of Helsinki. The study was approved by the Ethics Committee of the Juntendo University School of Medicine (14-011).
DTI acquisition and pre-processing
DTI acquisition was performed using a 3 T MR scanner (Achieva; Philips Healthcare, Best, Netherlands) with an eight-channel phased array head coil for sensitivity encoding parallel imaging. Whole-brain diffusion-weighted imaging was obtained using spin-echo echo-planar imaging (EPI) in the anterior-to-posterior phase-encoding direction, using a b value of 1,000 s/mm2 along 32 uniformly distributed directions. Acquisition of each diffusion-weighted image was completed with a gradient-free image (b = 0). Standard and reverse phase-encoded blipped images with no diffusion weighting (blip up and blip down) were also acquired to correct for magnetic susceptibility-induced distortions related to the EPI acquisitions [16]. The scanning parameters were as follows: repetition time = 9,810 ms; echo time = 100 ms; field of view = 256×256 mm; matrix size; 128×128; slice thickness = 2 mm, number of slices = 75; orientation = axial; and scanning time = 6.50 min.
The diffusion-weighted data were corrected for susceptibility-induced geometric distortions, eddy current distortions, and inter-volume subject motion using the EDDY and TOPUP toolboxes [16]. All datasets were then checked visually in the axial, sagittal, and coronal views. No datasets had severe artifacts related to gross geometric distortions, bulk motion, or signal dropout. The diffusion tensors were computed at each voxel by fitting a tensor model to the diffusion-weighted images with b = 0 and 1,000 s/mm2, based on standard formulae [17]. Next, fractional anisotropy (FA), mean diffusivity (MD), axial diffusivity (AD), and radial diffusivity (RD) maps were generated using the DTIFIT tool of the FMRIB Software Library 5.0.9 (FSL, Oxford Center for Functional MRI of the Brain, UK; www.fmrib.ox.ac.uk/fsl).
Tract-based spatial statistics (TBSS) analysis
Whole-brain white matter skeleton-wise analysis was performed using TBSS[18] implemented with the FSL to regionally map significant differences in each of the DTI measures between the groups with the use of a skeleton projection step. This analysis was also performed to evaluate the relationship between each index and the disease duration or serum oxidative stress markers.
Briefly, the FA images of all subjects were registered into 1×1×1×mm3 Montreal Neurological Institute (MNI 152) common space using the FMRIB nonlinear registration tool, followed by visual inspection to ensure registration quality. A mean FA image was then created by averaging the registered FA images. Next, the mean FA images were thinned to generate a mean FA skeleton representing the centers of all tracts of the groups. The aligned FA images of each subject were projected onto the mean FA skeleton using a threshold of FA > 0.2, to include major white matter pathways and exclude peripheral tracts and grey matter. The aligned FA map of each subject was then projected onto the skeleton. Finally, MD, AD, and RD maps were projected onto the mean FA skeleton after applying the warping registration field of each subject to the standard space. The anatomical locations of regions with significant correlations on the white matter skeleton were identified using the John Hopkins University DTI white matter atlas within FSL [19].
Region of interest analysis
The mean value of DTI metrics was extracted from the whole cluster showing significance on TBSS between-group differences or correlation analyses.
White matter volumetry
FreeSurfer (version 5.3.0; http://surfer.nmr.mgh.harvard.edu, Harvard University, Boston, MA, USA) was used for the automated whole white matter volumetric measures. For normalization purposes, the white matter volume was divided by the total intracranial volume.
Serum collection procedure
Venous blood samples for laboratory analysis were collected between 9:00 and 11:00 AM, following overnight fasting. Serum samples were collected and stored at –80°C until processing at Metabolon, Inc (Durham, NC, USA). The detailed methods, materials, and results of the metabolomic analyses have been described previously [8, 20].
Statistical analysis
Statistical analyses of the demographic and clinical data of participants were performed using JMP11 (SAS Institute Inc., Cary, NC, USA). All demographic and clinical variables were normally distributed, and were evaluated using the Kolmogorov–Smirnov test. The Student’s t-test and the chi-squared test were used to analyze continuous and categorical variables, respectively. Statistical significance was set at p < 0.05.
For the TBSS analyses, a skeleton-wise general linear model (GLM) framework with unpaired t-test, which included age and sex as covariates, was applied to compare all DTI measures between the HC and PRKN groups using the FSL Randomize tool. The skeleton-wise analysis was also performed using the permutation analysis of linear models (PALM) package [21] implemented with FSL to control for family-wise errors (FWEs) and multiple testing errors across the DTI measures.
The threshold-free cluster enhancement (TFCE) option was used in Randomize and PALM to avoid the selection of an arbitrary cluster-forming threshold, and 5,000 permutations were generated to provide an empirical null distribution of maximal cluster size [22]. An FWE-corrected p-value (pFWE) of < 0.05 was considered significant for the TBSS analyses. The multiple testing errors across the DTI metrices were also controlled using PALM. The same method was applied for the voxel-wise correlation analysis of all diffusion metrics with disease duration or serum oxidative stress markers in the PRKN group.
For the ROI analyses, the Student’s t-test was used to assess between-group differences in mean FA, MD, and RD (Bonferroni-corrected p < 0.017 was considered as significant) of the whole cluster that was significant in the TBSS analysis. The effect size was then calculated using Cohen’s d to evaluate the statistical power of the relationship that was determined during inter-group comparisons. Effect sizes of 0.2, 0.5, and 0.8 were classified as small, medium, and large, respectively [23]. Next, post-hoc statistical power was calculated based on the sample size and observed effect size. Furthermore, the Pearson correlation test was used to evaluate the correlations between the mean values of DTI metrics of the whole cluster showing significant correlation with 9-hydroxystearate (FA, MD, AD, and RD) or disease duration (MD, AD, and RD), respectively, in the TBSS analyses. Considering the exploratory nature of this analysis, the Bonferroni correction was not applied. Correlation coefficients (r) were classified as follows: 0.0–0.2, weak-to-low correlation; 0.2–0.4, low-to-moderate correlation; 0.4–0.6, moderate correlation; 0.6–0.8, strong correlation; and 0.8–1.0, very strong correlation.
For white matter volumetry, the normalized white matter volume was compared between the HCs and PRKN groups using the Student’s t-test, with p < 0.05 considered as significant.
RESULTS
MRI demographic and clinical features
Table 1 shows the characteristics of the participants in our cohort. There were no differences in sex, age, or cerebrovascular risk factors between PRKN patients and HCs. In the PRKN patients, disease duration and H&Y stage were 27.5±11.9 years and 2.4±0.70, respectively. Serum oxidative stress markers, including 2-hydroxydecanoate, 2-hydroxypalmitate, 9-hydroxystearate, α-tocopherol, and γ-CEHC, were analyzed using metabolomic analysis.
White matter alterations in PRKN patients compared with healthy controls
Using TBSS, we performed a DTI comparison (FA, MD, AD, and RD) between the HCs and PRKN patients (Fig. 1). Details regarding the anatomical regions, peak t-values, and peak MNI coordinates of significant clusters are shown in Table 2. DTI analyses revealed several changes in the cerebral white matter (Fig. 1). Specifically, there was a significant decrease in FA and an increase in MD and RD in the PRKN patients compared with HCs (pFWE < 0.05). TBSS with PALM also demonstrated that there was a significant increase in RD in the PRKN patients compared with the HCs (pFWE < 0.05). The altered area in PALM was similar to the results in TBSS using Randomize (Supplementary Figure 1A).
Fig. 1
Comparison of diffusion tensor imaging between healthy controls and patients with Parkinson’s disease caused by PRKN mutations. The tract-based spatial statistics analyses show that PRKN patients have significantly lower FA (blue/light-blue voxels; p < 0.05) and significantly higher MD and RD (red voxels; p < 0.05) compared with HCs. FA, fractional anisotropy; HCs, healthy controls; MD, mean diffusivity; RD, radial diffusivity.
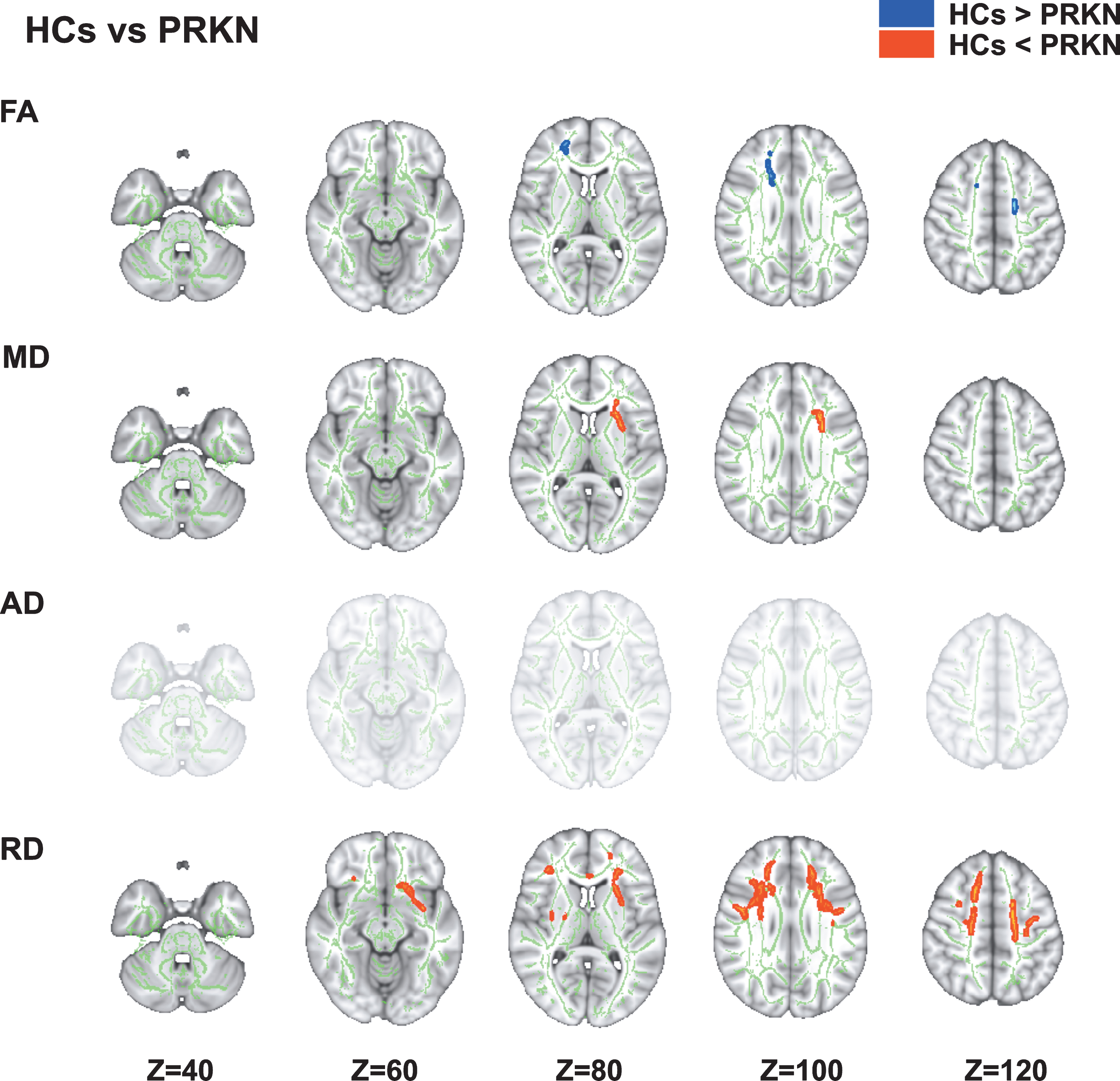
Table 2
Tract-based spatial statistics and region of interest analysis for PARK2 patients and healthy controls
Modality | Contrast | Cluster size | Anatomical region | Peak T-value | Peak MNI coordinates (X, Y, Z) | HCs | PARK2 | |||
Mean±SD | Mean±SD | p | Cohen’s d | Statistical power | ||||||
FA | HCs >PARK2 | 488 | Right ATR, ACR, SCR; | 3.67 | (74, 106, 128) | 0.46 ±0.03 | 0.41 ±0.02 | 0.0001* | 2.03 | 0.91 |
forceps minor, body of CC | ||||||||||
MD | HCs <PARK2 | 605 | Left ATR, IFOF, UF, ALIC, | 5.22 | (121, 136, 70) | 0.75 ±0.02 | 0.80 ±0.03 | 0.00005* | 2.00 | 0.93 |
ACR, SCR, external capsule | ||||||||||
RD | HCs <PARK2 | 7742 | Bilateral CST, IFOF, SLF, ALIC, | 5.25 | (58, 158, 91) | 0.54±0.02 | 0.60±0.04 | 0.0002* | 1.67 | 0.89 |
ACR, SCR, external capsule; | ||||||||||
Left ATR, ILF, UF; Right | ||||||||||
PLIC, SFOF; forceps minor, | ||||||||||
genu and body of CC |
ACR, anterior corona radiata; AD, axial diffusivity; ALIC, anterior limb of internal capsule; ATR, anterior thalamic radiation; CC, corpus callosum; CST, cerebrospinal tract; FA, fractional anisotropy; HCs, healthy controls; IFOF, inferior fronto-occipital fasciculus; ILF, inferior longitudinal fasciculus; MD, mean diffusivity; PLIC, posterior limb of internal capsule; RD, radial diffusivity; SCR, superior corona radiata; SFOF, superior fronto-occipital fasciculus; SLF, superior longitudinal fasciculus; UF, uncinate fasciculus. Mean values of FA, MD, and RD were extracted from the whole cluster showing significant group differences in tract-based spatial statistics analysis. *Bonferroni-corrected p < 0.017.
In the ROI analysis, the PRKN patients had a significant decrease in FA (p = 0.0001, Cohen’s d = 2.03, statistical power = 0.91) and significant increases in MD (p = 0.00005, Cohen’s d = 2.00, statistical power = 0.93) and RD (p = 0.0002, Cohen’s d = 1.67, statistical power = 0.89) compared with HCs (Table 2).
Associations between white matter alterations and disease duration
In the TBSS analysis of the PRKN group, MD, AD, and RD values were positively correlated with disease duration (Fig. 2). Details regarding the anatomical regions, peak t-values, and peak MNI coordinates of the significant clusters are shown in Table 3.
Fig. 2
Correlations between diffusion tensor imaging measures and disease duration and 9-hydroxystearate levels in patients with Parkinson’s disease caused by PRKN mutations. Tract-based spatial statistics analyses in PRKN patients revealed a significant positive correlation between disease duration and MD, AD, and RD (A). Additionally, there was a significant negative correlation between the serum levels of 9-hydroxystearate and FA and AD, and a significant positive correlation between the serum levels of 9-hydroxystearate and MD and RD (B). AD, axial diffusivity; FA, fractional anisotropy; MD, mean diffusivity; RD, radial diffusivity.
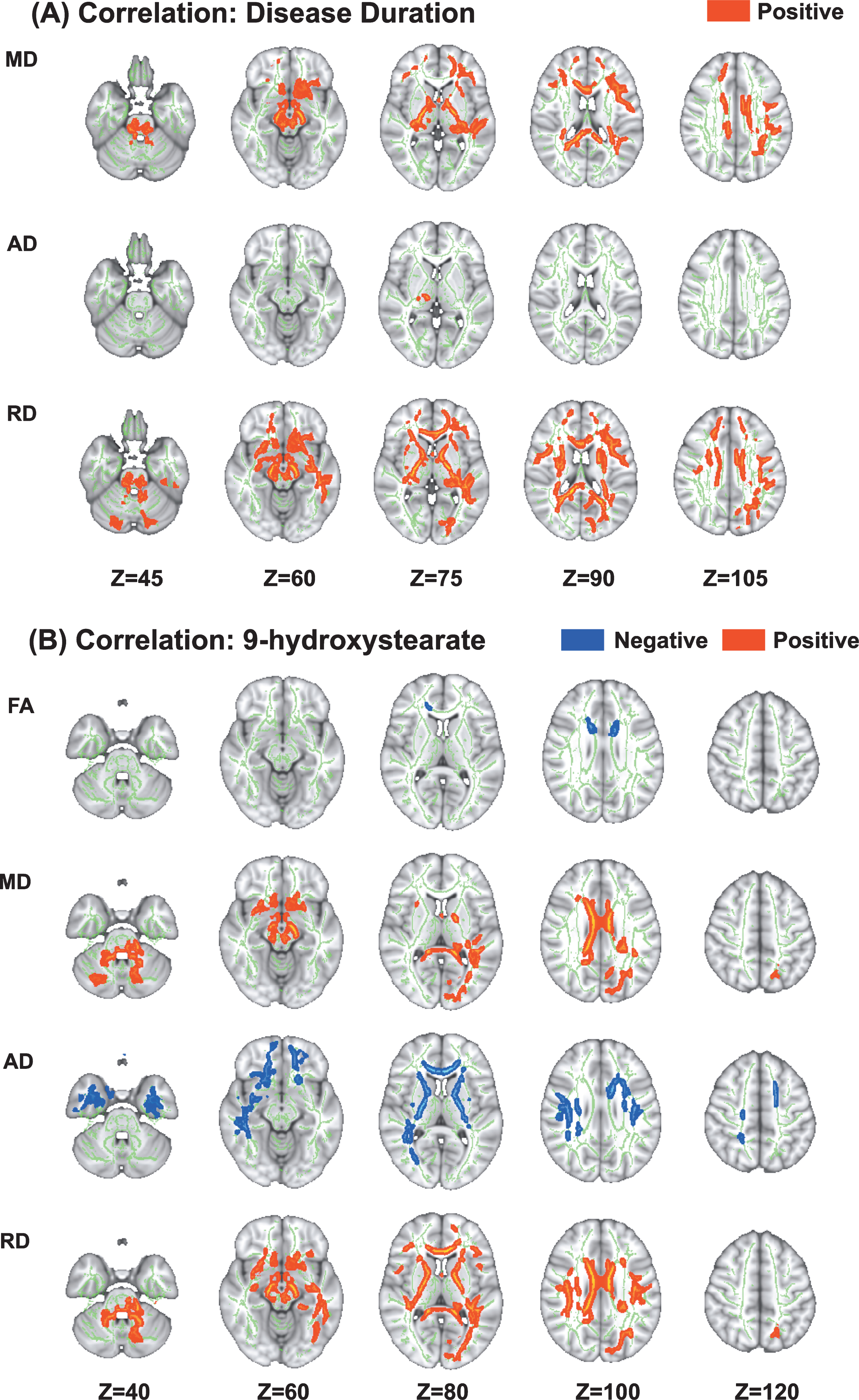
Table 3
Correlations between disease duration and diffusion tensor imaging in tract-based spatial statistics and region of interest analysis
Modality | Contrast | Cluster size | Anatomical region | Peak T-value | Peak MNI coordinates (X, Y, Z) | Mean±SD | r | p |
MD | DD (+) | 24229 | Bilateral ATR, CST, CCG, IFOF, medial lemniscus, ICP, SCP, CP, ALIC, PLIC, RIC, ACR, SCR, PCR, PTR, external capsule, fornix stria terminalis; Left ILF, SLF, UF; Right sagittal stratum; forceps major, forceps minor, MCP, pontine crossing tract, genu, body and splenium of CC, fornix | 13.1 | (116, 156, 87) | 0.84±0.23 | 0.65 | 0.06 |
AD | DD (+) | 72 | Right cerebral peduncle, PLIC | 6.87 | (78, 106, 75) | 1.04±0.13 | 0.83 | 0.006* |
RD | DD (+) | 39981 | Bilateral ATR, CST, CCG, IFOF, SLF, UF, medial lemniscus, ICP, SCP, cerebral peduncle, ALIC, PLIC, RIC, ACR, SCR, PCR, PTR, sagittal stratum, external capsule, fornix stria terminalis, SFOF; Left ILF, SLF temporal part; forceps major, forceps minor, MCP, pontine crossing tract, genu, body and splenium of CC, fornix | 9.86 | (120, 151, 87) | 0.60±0.22 | 0.67 | 0.05* |
ACR, anterior corona radiata; AD, axial diffusivity; ALIC, anterior limb of internal capsule; ATR, anterior thalamic radiation; CC, corpus callosum; CCG, cingulum cingulate gyrus; CP, cerebellar peduncle; CST, cerebrospinal tract; DD, disease duration; FA, fractional anisotropy; ICP, inferior cerebellar peduncle; IFOF, inferior fronto-occipital fasciculus; ILF, inferior longitudinal fasciculus; MCP, middle cerebellar peduncle; MD, mean diffusivity; PCR, posterior corona radiata; PLIC, posterior limb of internal capsule; PTR, posterior thalamic radiation; RD, radial diffusivity; RIC, retrolenticular part of internal capsule; SCR, superior corona radiata; SCP, superior cerebellar peduncle; SFOF, superior fronto-occipital fasciculus; SLF, superior longitudinal fasciculus; UF, uncinate fasciculus. Mean values of MD, AD, and RD were extracted from the whole cluster showing significant correlations with disease duration in tract-based spatial statistics analysis. *p < 0.05
In the ROI analysis (Fig. 3, Table 3), there were significant very strong and strong positive correlations between disease duration and AD (r = 0.83, p = 0.006) or RD (r = 0.67, p = 0.05), respectively.
Associations between white matter alterations and oxidative stress markers
Several parameters of DTI were correlated with the serum levels of 9-hydroxystearate. In the PRKN group, the FA and AD values were negatively correlated with the serum levels of 9-hydroxystearate, while the MD and RD values were positively correlated with these levels (Fig. 3). Details regarding the anatomical regions, peak t-values, and peak MNI coordinates of significant clusters are shown in Table 4. There were no correlations between DTI measures and any other oxidative stress markers, including 2-hydroxypalmitate, α-tocopherol, or γ-CEHC.
Fig. 3
Scatter plots showing the correlation between the mean values of diffusion tensor imaging measures of the whole cluster in ROI analysis and disease duration (A) or 9-hydroxystearate (B). (A) There were significant positive correlations between AD or RD and disease duration. (B) There were significant negative correlations between FA or AD and 9-hydroxystearate, and significant positive correlations between MD or RD and 9-hydroxystearate. AD, axial diffusivity; FA, fractional anisotropy; MD, mean diffusivity; RD, radial diffusivity; ROI, region of interest.
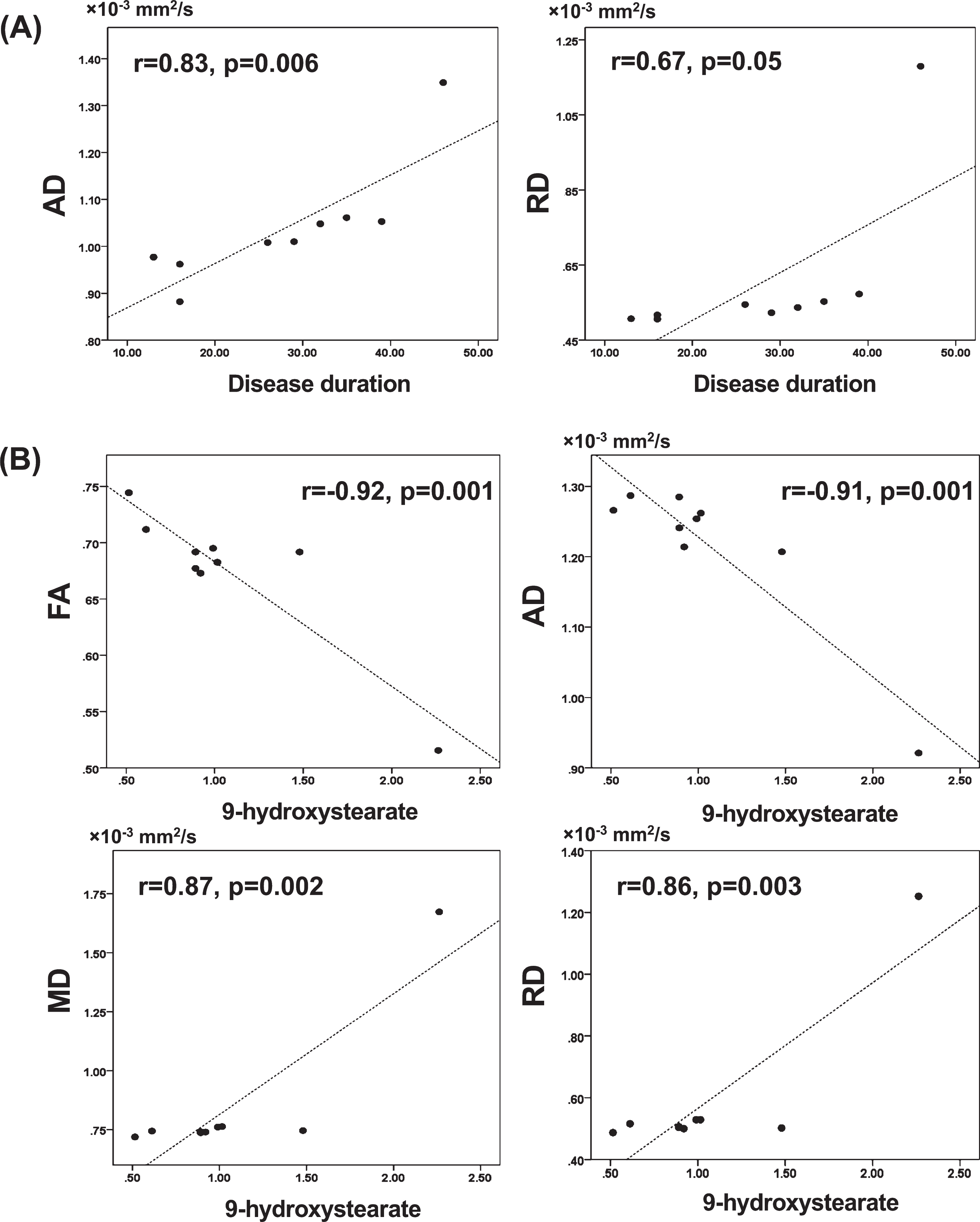
In the ROI analysis (Fig. 3, Table 4), the serum levels of 9-hydroxystearate were also very strongly negatively correlated with FA (r = –0.92, p = 0.001) and AD (r = –0.91, p = 0.001), and positively correlated with MD (r = 0.87, p = 0.002) and RD (r = 0.86, p = 0.003).
Table 4
Correlations between 9-hydroxystearate levels and diffusion tensor imaging measures in tract-based spatial statistics and region of interest analysis
Modality | Contrast | Cluster size | Anatomical region | Peak T-value | Peak MNI coordinates (X, Y, Z) | Mean±SD | r | p |
FA | 9-hydroxystearate (–) | 963 | Left SCR; Right ACR; forceps minor, genu and body of CC | 14.7 | (81, 139, 95) | 0.68±0.06 | –0.92 | 0.001* |
MD | 9-hydroxystearate (+) | 18436 | Bilateral ATR, CST, CCG, IFOF, UF, medial lemniscus, ICP, SCP, CP, ALIC, PLIC, ACR, PCR, PTR, external capsule; Left CHp, ILF, SLF, RIC, SCR, fornix stria terminalis; forceps major, forceps minor, MCP, pontine crossing tract, genu, body and splenium of CC, fornix | 46.2 | (102, 130, 65) | 0.85±0.31 | 0.87 | 0.002* |
AD | 9-hydroxystearate (–) | 19774 | Bilateral ATR, CST, IFOF, ILF, SLF, UF, ALIC, PLIC, RIC, ACR, SCR, PTR, external capsule, SFOF; Left CCG; Right CHp, SLF temporal part, PCR, sagittal stratum, fornix stria terminalis; forceps major, forceps minor, genu and body of CC | 33.7 | (108, 161, 54) | 1.22±0.11 | –0.91 | 0.001* |
RD | 9-hydroxystearate (+) | 32905 | Bilateral ATR, CST, CCG, IFOF, SLF, UF, medial lemniscus, ICP, SCP, CP, ALIC, PLIC, RIC, ACR, SCR, PCR, PTR, sagittal stratum EC, fornix stria terminalis, SFOF; Left CHp, ILF, SLF temporal part; forceps major, forceps minor, MCP, pontine crossing tract, genu, body and splenium of CC, fornix | 37.6 | (97, 152, 71) | 0.59±0.25 | 0.86 | 0.003* |
ACR, anterior corona radiata; AD, axial diffusivity; ALIC, anterior limb of internal capsule; ATR, anterior thalamic radiation; CC, corpus callosum; CCG, cingulum cingulate gyrus; CHp, cingulum hippocampus; CP, cerebral peduncle; CST, cerebrospinal tract; FA, fractional anisotropy; ICP, inferior cerebellar peduncle; IFOF, inferior fronto-occipital fasciculus; ILF, inferior longitudinal fasciculus; MCP, middle cerebellar peduncle; MD, mean diffusivity; PCR, posterior corona radiata; PLIC, posterior limb of internal capsule; PTR, posterior thalamic radiation; RD, radial diffusivity; RIC, retrolenticular part of internal capsule; SCR, superior corona radiata; SCP, superior cerebellar peduncle; SFOF, superior fronto-occipital fasciculus; SLF, superior longitudinal fasciculus; UF, uncinate fasciculus. Mean values of FA, MD, AD, and RD were extracted from the whole cluster showing significant correlations with 9-hydroxystearate in tract-based spatial statistics analysis. *p < 0.05.
White matter volumetry
The normalized white matter volumes (Table 1) of HCs and PRKN patients were not significantly different (p = 0.93).
TBSS and TBSS with PALM in patients with PRKN excluding the outlier
One patient with PRKN, who was the oldest patient (78 years, female) and had the longest disease duration (46 years), showed marked alterations of several markers. Thus, the data were re-analyzed excluding the data from the patient considered as an outlier. The TBSS with Randomize (Supplementary Figure 1C) and PALM (Supplementary Figure 1B) analyses revealed significant alterations in RD in the PRKN patients compared with HCs (pFWE < 0.05). The white matter MD alterations were slightly but significantly correlated with both disease duration and serum levels of 9-hydroxystearate in TBSS (Supplementary Figure 1D). Furthermore, the RD alterations in the significantly increased RD areas (Supplementary Figure 1C) in patients with PRKN excluding one outlier compared with HCs were significantly correlated with disease duration (r = 0.715, p = 0.046). (Supplementary Figure 1E).
DISCUSSION
We revealed an apparent alteration in white matter microstructures marked by a corresponding decrease in FA and an increase in MD and RD in PRKN patients compared with age- and sex-matched HCs. White matter microstructural alterations were observed in extensive white matter areas, including the anterior and superior corona radiata, anterior limb of the internal capsule, anterior thalamic radiation, splenium of the corpus callosum, corticospinal tract, inferior fronto-occipital fasciculus, superior and inferior longitudinal fasciculus, posterior corona radiata, posterior limb of the internal capsule, retrolenticular part of the internal capsule, and uncinate fasciculus. No differences in white matter volume were observed between the HC and PRKN groups. This finding suggests that diffusion MRI indices are potentially more sensitive as biomarkers for detecting microstructural changes in PRKN patients, prior to tissue loss being detected during volumetric MRI. Furthermore, in our previous metabolomic analysis [8], the serum levels of oxidative lipids, such as 2-hydroxypalmitate and 9-hydroxystearate, which are known oxidative stress markers, were higher in PRKN patients than in control subjects. Of these, 9-hydroxystearate was the most significantly elevated in PRKN patients. In contrast, the serum levels of α-tocopherol and γ-CEHC, which are known anti-oxidative stress markers, were lower in PRKN patients than in control subjects. In the present study, we thus investigated the correlations between alterations in DTI measures and the serum levels of these markers in PRKN patients using TBSS. The alterations in DTI parameters were closely associated with both disease duration and serum levels of 9-hydroxystearate, which is an oxidized lipid that can be used as a marker of oxidative stress. Thus, the white matter microstructural changes in PRKN patients might be caused by disease duration and oxidative stress.
Previous diffusion MRI studies revealed alterations in the water diffusivity of white matter in iPD [24, 25]. Moreover, a recent meta-analysis demonstrated decreased FA and increased MD in patients with iPD compared with HCs [26]. The alterations of MD in white matter microstructures of iPD patients were correlated with disease duration, similar to our results in the present study. In iPD, Lewy pathology and neuronal degeneration spread not only to dopaminergic neurons in the nigrostriatal pathway, but also to noradrenergic, serotonergic, and cholinergic neurons [27]. Thus, it has been suggested that white matter abnormalities in iPD might be associated with widespread neuronal degeneration [28]. In contrast, pathology in PRKN patients is known to be relatively restricted to dopaminergic neurons in the nigrostriatal pathway. We were unable to compare the white matter microstructure of PRKN patients with iPD patients because the onset age of PRKN patients is generally much younger than that of iPD patients. However, our current results indicate that dopaminergic neuronal degeneration in the nigrostriatal pathway might be also associated with abnormalities in white matter microstructures.
Interestingly, several tracts that were impaired in PRKN patients have also been reported in iPD. The inferior longitudinal fasciculus and uncinate fasciculus might be associated with several motor and non-motor symptoms in iPD, such as cognitive decline [25], freezing of gait [29], depression [30], and impulse control disorders [31]. Wu et al. [32] reported that iPD patients with depression have reduced FA in the left anterior corona radiata, left posterior thalamic radiation, left cingulum, left superior longitudinal fasciculus, left inferior longitudinal fasciculus, left inferior fronto-occipital fasciculus, and left uncinate fasciculus, similar to the results from our study. Although PRKN patients have relatively pure dopaminergic neuronal degeneration in the SNc, without cortical neuronal degeneration, some PRKN patients exhibit variable neuropsychiatric symptoms, such as paranoia, panic attacks, and depression [33]. Furthermore, Song et al. [34] reported that PRKN patients have a higher depression index on the Beck Depression Inventory, which is an assessment battery of depression status, compared with non-PRKN-linked young-onset PD patients. Alterations in white matter microstructures may therefore contribute to non-motor symptoms in PRKN patients.
A systematic review and meta-analysis of previous DTI studies revealed that patients with iPD have consistently decreased FA and increased MD in several regions, including the SN, corpus callosum, frontal lobe, and cingulate and temporal cortices [26, 35]. Interestingly, similar to iPD patients, PRKN patients also exhibit decreased FA and increased MD and RD in the corticospinal tract, anterior corona radiata, posterior corona radiata, and corpus callosum. FA values are a robust parameter of the degree of anisotropic diffusion within a region. MD is a parameter of the total diffusion within a voxel and indicates the magnitude of isotropic diffusion within brain tissue, and RD indicates the diffusion of water perpendicular to the white matter fibers. Decreased FA with increased MD is reportedly associated with an alleviation of neuronal injury or demyelination [35]. Previous DTI studies in PD patients have mostly evaluated only FA and MD [26]; however, RD can be used to demonstrate a more specific relationship to white matter pathology compared with FA and MD [36]. In keeping with this finding, our study revealed broader changes in RD compared with FA and MD. Furthermore, TBSS with PALM also showed a marked elevation of RD in PRKN patients compared with HCs. Considering the increased RD in multiple tests, this may be the most important pathological finding caused by parkin dysfunction. Song et al. [37] analyzed the RD and AD of white matter in shiverer mice, which lack CNS myelin without prominent axonal injury nor inflammation. They revealed that the absence of myelin appeared to increase RD, but did not significantly affect AD. Considering our results of diffusely increased RD, PRKN patients might have widespread demyelination in the white matter.
Selective dopaminergic neuronal degeneration in the SNc without Lewy body formation is a characteristic pathological finding of PRKN patients, although Lewy body-positive cases have also been reported. To date, however, there is no published evidence of cortical neuronal degeneration or white matter changes [4]. Thus, water diffusivity alterations in white matter might not be caused by neuronal degeneration, but may instead be associated with secondary impairment of axon and myelin sheath. Recent studies have revealed that the parkin/PINK1 pathway plays important roles in the mitochondrial quality control system. Mitochondria produce reactive oxygen species; therefore, mitochondrial dysfunction brings a risk of hyperoxidative stress [38]. Parkin expression is widely distributed in systemic tissues [1]; therefore, PRKN patients may be exposed to systemic hyperoxidative stress caused by impaired mitochondrial quality control. Indeed, we have previously revealed alterations in serum anti- and pro-oxidative markers in analyses of PRKN patients [8, 9].
A previous report has described the associations between white matter microstructural injuries and serum oxidative stress biomarkers, such as plasma nuclear and mitochondrial DNA, in iPD [39]. In addition, a rat model systemically injected with the mitochondrial inhibitor 3-nitropropionic acid displayed cytoskeletal breakdown in axons and oligodendrocytes in the white matter [40]. Thus, systemic oxidative stress might induce alterations in white matter microstructures. Neurodegenerative disorders associated with genetic risk polymorphisms of superoxide dismutase 2, an antioxidant enzyme, might be correlated with increased RD values [10]. Furthermore, overweight participants in an allostatic load model state, which is linked to systemic oxidative stress and inflammatory responses, have decreased FA values [11]. These findings are similar to the alterations in white matter microstructures observed in the PRKN patients in the present study. Thus, we hypothesize that the white matter microstructural damage may be caused by systemic oxidative stress in PRKN patients. In support of this hypothesis, we revealed a correlation between the serum levels of 9-hydroxystearate and MD and RD values in some regions of white matter tracts. In addition, a longer disease duration, which is likely to reflect a longer exposure to systemic oxidative stress, was associated with alterations in MD and RD values. Furthermore, Ashrafi et al. reported that mitophagy via the parkin/PINK1 pathway might occur in neuronal axons [41]. Thus, systemic oxidative stress and the impairment of mitophagy in axons might also be associated with alterations in white matter microstructures.
We failed to reveal any associations between the other redox markers, including 2-hydroxydecanoate, 2-hydroxypalmitate, α-tocopherol, and γ-CEHC, and alterations in white matter microstructures. In our previous report, serum 9-hydroxystearate levels were more altered in PRKN patients than 2-hydroxydecanoate or 2-hydroxypalmitate levels. Thus, 9-hydroxystearate might be the best marker of oxidative stress in PRKN patients. Furthermore, α-tocopherol and γ-CEHC were not associated with alterations in white matter microstructures. These compounds are known to have roles as reducers, but not sensors, of oxidative stress. They may therefore not be useful as markers of CNS oxidative stress, although they might reflect systemic oxidation.
Limitations
There are several limitations in this study. The first limitation was the small sample size for a case–control study, which was caused by the low prevalence of PRKN mutations in the general population. However, we found that the power of this study was adequate to detect any differences in the analysis of the significant cluster derived from TBSS results. In the future, we should confirm these findings in a second, larger cohort. Additionally, the white matter abnormalities were associated with the serum levels of 9-hydroxystearate and disease duration. Considering the function of parkin protein, the correlation between abnormal white matter microstructures and hyperoxidative stress might indicate the pathomechanisms of neurodegeneration in PRKN patients. Second, although the white matter microstructure alterations in PRKN patients were quite similar to those previously reported in iPD, we were unable to use these data to directly compare between both disorders because of the much younger average age of the PRKN patients compared with the iPD patients. In addition, we did not evaluate the non-motor symptoms of the PRKN patients. To explore which clinical symptoms are associated with white matter alterations in PRKN patients, further studies should investigate several clinical parameters to compare with iPD, including non-motor symptoms. Third, we did not conduct a metabolomic analysis of the HCs. White matter alterations in HCs might also be associated with oxidative stress as a result of aging and environmental factors. For example, oxidative stress might play an important role in the pathomechanisms of cellular senescence [38, 42]. However, in the current study, we focused on white matter abnormalities of PRKN patients alone. Thus, the intra-PRKN analysis shed light on our clinical question. Fourth, pathological alterations of the white matter should be confirmed by postmortem study. However, white matter is vulnerable to postmortem effects, and pathological studies mainly evaluate patients with end-stage disease. Fifth, the cohort included one PRKN patient with marked alterations in several parameters. We should consider this patient (78 years female) as an outlier. When the data from this patient were removed, the results failed to show a strong correlation between white matter microstructures and disease duration or 9-hydroxystearate. However, she was the oldest and had the longest disease duration. It is well known that oxidative stress is significantly correlated with aging. Thus, parkin dysfunction may impact strongly on white matter microstructural alterations caused by aging. Despite these limitations, we believe that parkin dysfunction might be associated with white matter abnormalities caused by brain and systemic oxidative stress.
CONCLUSION
We revealed white matter abnormalities in PRKN patients, although the pathological analysis was unable to elucidate the alterations in the white matter and cortex. The abnormalities in white matter microstructures were correlated with disease duration and serum oxidative stress markers. Previous studies have reported that the progression of motor symptoms in PRKN patients is milder than that in iPD patients [43]. In addition, it has been reported that PRKN patients show relatively pure nigral dopaminergic neurodegeneration, and the prevalence of non-motor symptoms in PRKN patients is less than that in iPD patients [44]. However, in the present study, we revealed alterations in white matter microstructures of PRKN patients that were similar to those of iPD patients, thus shedding light on the motor and non-motor symptoms that cannot be explained by pure nigral dopaminergic neuronal degeneration. To elucidate the pathomechanisms of white matter abnormalities caused by parkin dysfunction, intensive pathological analyses are needed in the future.
ACKNOWLEDGMENTS
We thank Drs. Kei-Ichi Ishikawa, Motoki Fujimaki, and Hiroyo Yoshino, and Ms. Yoko Imamichi (Juntendo University, Faculty of Medicine). Furthermore, we thank Drs. Sivapriya Ramamoorthy and Robert P. Mohney (Metabolon Inc.), and all of the participants in this study. We also thank Bronwen Gardner, PhD, from Edanz Group (https://en-author-services.edanz.com/ac) for editing a draft of this manuscript. This study was supported by a Strategic Research Foundation Grant-in-Aid for Private Universities, and Grants-in-Aid for Scientific Research on Priority Areas 24390224 (to N.H.); the program for Brain Mapping by Integrated Neurotechnologies for Disease Studies (Brain/MINDS) from AMED under grant number 19dm0207070 (to N.H.), AMED under grant number 19dm0107156 (to T.H.), JP19dm0307024 (to K.K.), 19ek0109358 (to N.H.), 19dm0307101 (to N.H.), and 19ek0109393 (to N.H.); and Grants-in Aid from the Research Committee of CNS Degenerative Disease, Research on Policy Planning and Evaluation for Rare and Intractable Diseases, Health, Labour, and Welfare Sciences Research Grants, the Ministry of Health, Labour, and Welfare, Japan (to N.H.), the Setsuro Fujii Memorial Osaka Foundation for Promotion of Fundamental Medical Research (to T.H.), and JSPS KAKENHI, under grant number JP19K17244 (to K.K.).
CONFLICT OF INTEREST
T. Koinuma, T. Hatano, K. Kamagata, C. Andica, A, Mori, T. Ogawa, H. Takeshige-Amano, W. Uchida, S. Saiki, A. Okuzumi, S-I. Ueno, Y. Oji, Y. Saito, M. Hori, and S. Aoki have no conflict of interest to report.
N. Hattori is an associate editor/member of the editorial board for Journal of Parkinson’s Disease.
SUPPLEMENTARY MATERIAL
[1] The supplementary material is available in the electronic version of this article: https://dx.doi.org/10.3233/JPD-202495.
REFERENCES
[1] | Kitada T , Asakawa S , Hattori N , Matsumine H , Yamamura Y , Minoshima S , Yokochi M , Mizuno Y , Shimizu N ((1998) ) Mutations in the parkin gene cause autosomal recessive juvenile parkinsonism, Nature 392: , 605–608. |
[2] | Hattori N , Mizuno Y ((2017) ) Twenty years since the discovery of the parkin gene, J Neural Transm (Vienna) 124: , 1037–1054. |
[3] | Mori H , Kondo T , Yokochi M , Matsumine H , Nakagawa-Hattori Y , Miyake T , Suda K , Mizuno Y ((1998) ) Pathologic and biochemical studies of juvenile parkinsonism linked to chromosome 6q, Neurology 51: , 890–892. |
[4] | Doherty KM , Silveira-Moriyama L , Parkkinen L , Healy DG , Farrell M , Mencacci NE , Ahmed Z , Brett FM , Hardy J , Quinn N , Counihan TJ , Lynch T , Fox ZV , Revesz T , Lees AJ , Holton JL ((2013) ) Parkin disease: A clinicopathologic entity? JAMA Neurol 70: , 571–579. |
[5] | Miyakawa S , Ogino M , Funabe S , Uchino A , Shimo Y , Hattori N , Ichinoe M , Mikami T , Saegusa M , Nishiyama K , Mori H , Mizuno Y , Murayama S , Mochizuki H ((2013) ) Lewy body pathology in a patient with a homozygous parkin deletion, Mov Disord 28: , 388–391. |
[6] | Shimura H , Hattori N , Kubo S , Mizuno Y , Asakawa S , Minoshima S , Shimizu N , Iwai K , Chiba T , Tanaka K , Suzuki T ((2000) ) Familial Parkinson disease gene product, parkin, is a ubiquitin-protein ligase, Nat Genet 25: , 302–305. |
[7] | Narendra D , Tanaka A , Suen DF , Youle RJ ((2009) ) Parkin-induced mitophagy in the pathogenesis of Parkinson disease, Autophagy 5: , 706–708. |
[8] | Okuzumi A , Hatano T , Ueno SI , Ogawa T , Saiki S , Mori A , Koinuma T , Oji Y , Ishikawa KI , Fujimaki M , Sato S , Ramamoorthy S , Mohney RP , Hattori N ((2019) ) Metabolomics-based identification of metabolic alterations in PARK2, Ann Clin Transl Neurol 6: , 525–536. |
[9] | Ueno SI , Hatano T , Okuzumi A , Saiki S , Oji Y , Mori A , Koinuma T , Fujimaki M , Takeshige-Amano H , Kondo A , Yoshikawa N , Nojiri T , Kurano M , Yasukawa K , Yatomi Y , Ikeda H , Hattori N ((2020) ) Nonmercaptalbumin as an oxidative stress marker in Parkinson’s and PARK2 disease, Ann Clin Transl Neurol 7: , 307–317. |
[10] | Salminen LE , Schofield PR , Pierce KD , Bruce SE , Griffin MG , Tate DF , Cabeen RP , Laidlaw DH , Conturo TE , Bolzenius JD , Paul RH ((2017) ) Vulnerability of white matter tracts and cognition to the SOD2 polymorphism: A preliminary study of antioxidant defense genes in brain aging, Behav Brain Res 329: , 111–119. |
[11] | Ottino-Gonzalez J , Jurado MA , Garcia-Garcia I , Segura B , Marques-Iturria I , Sender-Palacios MJ , Tor E , Prats-Soteras X , Caldu X , Junque C , Pasternak O , Garolera M ((2018) ) Allostatic load and disordered white matter microstructure in overweight adults, Sci Rep 8: , 15898. |
[12] | Le Bihan D , Johansen-Berg H ((2012) ) Diffusion MRI at 25: Exploring brain tissue structure and function, Neuroimage 61: , 324–341. |
[13] | Wassenaar TM , Yaffe K , van der Werf YD , Sexton CE ((2019) ) Associations between modifiable risk factors and white matter of the aging brain: Insights from diffusion tensor imaging studies. Neurobiol Aging 80: , 56–70. |
[14] | Tomlinson CL , Stowe R , Patel S , Rick C , Gray R , Clarke CE ((2010) ) Systematic review of levodopa dose equivalency reporting in Parkinson’s disease, Mov Disord 25: , 2649–2653. |
[15] | Hatano T , Okuzumi A , Kamagata K , Daida K , Taniguchi D , Hori M , Yoshino H , Aoki S , Hattori N ((2017) ) Neuromelanin MRI is useful for monitoring motor complications in Parkinson’s and PARK2 disease, J Neural Transm (Vienna) 124: , 407–415. |
[16] | Andersson JLR , Sotiropoulos SN ((2016) ) An integrated approach to correction for off-resonance effects and subject movement in diffusion MR imaging, Neuroimage 125: , 1063–1078. |
[17] | Basser PJ , Mattiello J , LeBihan D ((1994) ) MR diffusion tensor spectroscopy and imaging, Biophys J 66: , 259–267. |
[18] | Smith SM , Jenkinson M , Johansen-Berg H , Rueckert D , Nichols TE , Mackay CE , Watkins KE , Ciccarelli O , Cader MZ , Matthews PM , Behrens TE ((2006) ) Tract-based spatial statistics: Voxelwise analysis of multi-subject diffusion data, Neuroimage 31: , 1487–1505. |
[19] | Oishi K , Zilles K , Amunts K , Faria A , Jiang H , Li X , Akhter K , Hua K , Woods R , Toga AW , Pike GB , Rosa-Neto P , Evans A , Zhang J , Huang H , Miller MI , van Zijl PC , Mazziotta J , Mori S ((2008) ) Human brain white matter atlas: Identification and assignment of common anatomical structures in superficial white matter, Neuroimage 43: , 447–457. |
[20] | Hatano T , Saiki S , Okuzumi A , Mohney RP , Hattori N ((2016) ) Identification of novel biomarkers for Parkinson’s disease by metabolomic technologies, J Neurol Neurosurg Psychiatry 87: , 295–301. |
[21] | Winkler AM , Ridgway GR , Webster MA , Smith SM , Nichols TE ((2014) ) Permutation inference for the general linear model, Neuroimage 92: , 381–397. |
[22] | Smith SM , Nichols TE ((2009) ) Threshold-free cluster enhancement: Addressing problems of smoothing, threshold dependence and localisation in cluster inference, Neuroimage 44: , 83–98. |
[23] | Cohen J ((1992) ) A power primer, Psychol Bull 112: , 155–159. |
[24] | Kamagata K , Motoi Y , Abe O , Shimoji K , Hori M , Nakanishi A , Sano T , Kuwatsuru R , Aoki S , Hattori N ((2012) ) White matter alteration of the cingulum in Parkinson disease with and without dementia: Evaluation by diffusion tensor tract-specific analysis, AJNR Am J Neuroradiol 33: , 890–895. |
[25] | Kamagata K , Motoi Y , Tomiyama H , Abe O , Ito K , Shimoji K , Suzuki M , Hori M , Nakanishi A , Sano T , Kuwatsuru R , Sasai K , Aoki S , Hattori N ((2013) ) Relationship between cognitive impairment and white-matter alteration in Parkinson’s disease with dementia: Tract-based spatial statistics and tract-specific analysis, Eur Radiol 23: , 1946–1955. |
[26] | Atkinson-Clement C , Pinto S , Eusebio A , Coulon O ((2017) ) Diffusion tensor imaging in Parkinson’s disease: Review and meta-analysis, Neuroimage Clin 16: , 98–110. |
[27] | Poewe W , Seppi K , Tanner CM , Halliday GM , Brundin P , Volkmann J , Schrag AE , Lang AE ((2017) ) Parkinson disease, Nat Rev Dis Primers 3: , 17013. |
[28] | Kamagata K , Tomiyama H , Hatano T , Motoi Y , Abe O , Shimoji K , Kamiya K , Suzuki M , Hori M , Yoshida M , Hattori N , Aoki S ((2014) ) A preliminary diffusional kurtosis imaging study of Parkinson disease: Comparison with conventional diffusion tensor imaging, Neuroradiology 56: , 251–258. |
[29] | Pietracupa S , Suppa A , Upadhyay N , Gianni C , Grillea G , Leodori G , Modugno N , Di Biasio F , Zampogna A , Colonnese C , Berardelli A , Pantano P ((2018) ) Freezing of gait in Parkinson’s disease: Gray and white matter abnormalities, J Neurol 265: , 52–62. |
[30] | Huang P , Xu X , Gu Q , Xuan M , Yu X , Luo W , Zhang M ((2014) ) Disrupted white matter integrity in depressed versus non-depressed Parkinson’s disease patients: A tract-based spatial statistics study, J Neurol Sci 346: , 145–148. |
[31] | Imperiale F , Agosta F , Canu E , Markovic V , Inuggi A , Jecmenica-Lukic M , Tomic A , Copetti M , Basaia S , Kostic VS , Filippi M ((2018) ) Brain structural and functional signatures of impulsive-compulsive behaviours in Parkinson’s disease, Mol Psychiatry 23: , 459–466. |
[32] | Wu JY , Zhang Y , Wu WB , Hu G , Xu Y ((2018) ) Impaired long contact white matter fibers integrity is related to depression in Parkinson’s disease, CNS Neurosci Ther 24: , 108–114. |
[33] | Khan NL , Graham E , Critchley P , Schrag AE , Wood NW , Lees AJ , Bhatia KP , Quinn N ((2003) ) Parkin disease: A phenotypic study of a large case series, Brain 126: , 1279–1292. |
[34] | Song J , Shen B , Yang YJ , Liu FT , Zhao J , Tang YL , Chen C , Ding ZT , An Y , Wu JJ , Sun YM , Wang J ((2020) ) Non-motor symptoms in Parkinson’s disease patients with parkin mutations: More depression and less executive dysfunction, J Mol Neurosci 70: , 246–253. |
[35] | Andica C , Kamagata K , Hatano T , Saito Y , Ogaki K , Hattori N , Aoki S ((2020) ) MR biomarkers of degenerative brain disorders derived from diffusion imaging, J Magn Reson Imaging 52: , 1620–1636. |
[36] | Alexander AL , Lee JE , Lazar M , Field AS ((2007) ) Diffusion tensor imaging of the brain, Neurotherapeutics 4: , 316–329. |
[37] | Song SK , Sun SW , Ramsbottom MJ , Chang C , Russell J , Cross AH ((2002) ) Dysmyelination revealed through MRI as increased radial (but unchanged axial) diffusion of water, Neuroimage 17: , 1429–1436. |
[38] | Kauppila TES , Kauppila JHK , Larsson NG ((2017) ) Mammalian mitochondria and aging: An update, Cell Metab 25: , 57–71. |
[39] | Chen MH , Chen PC , Lu CH , Chen HL , Chao YP , Li SH , Chen YW , Lin WC ((2017) ) Plasma DNA mediate autonomic dysfunctions and white matter injuries in patients with Parkinson’s disease, Oxid Med Cell Longev 2017: , 7371403. |
[40] | McCracken E , Dewar D , Hunter AJ ((2001) ) White matter damage following systemic injection of the mitochondrial inhibitor 3-nitropropionic acid in rat, Brain Res 892: , 329–335. |
[41] | Ashrafi G , Schlehe JS , LaVoie MJ , Schwarz TL ((2014) ) Mitophagy of damaged mitochondria occurs locally in distal neuronal axons and requires PINK1 and Parkin, J Cell Biol 206: , 655–670. |
[42] | Campisi J , Kapahi P , Lithgow GJ , Melov S , Newman JC , Verdin E ((2019) ) From discoveries in ageing research to therapeutics for healthy ageing, Nature 571: , 183–192. |
[43] | Marsili L , Vizcarra JA , Sturchio A , Dwivedi AK , Keeling EG , Patel D , Mishra M , Farooqi A , Merola A , Fasano A , Mata IF , Kauffman MA , Espay AJ ((2020) ) When does postural instability appear in monogenic parkinsonisms? An individual-patient meta-analysis. J Neurol, doi: 10.1007/s00415-020-09892-3 |
[44] | Puschmann A ((2013) ) Monogenic Parkinson’s disease and parkinsonism: Clinical phenotypes and frequencies of known mutations, Parkinsonism Relat Disord 19: , 407–415. |