Body-Worn Sensors for Remote Monitoring of Parkinson’s Disease Motor Symptoms: Vision, State of the Art, and Challenges Ahead
Abstract
The increasing prevalence of neurodegenerative conditions such as Parkinson’s disease (PD) and related mobility issues places a serious burden on healthcare systems. The COVID-19 pandemic has reinforced the urgent need for better tools to manage chronic conditions remotely, as regular access to clinics may be problematic. Digital health technology in the form of remote monitoring with body-worn sensors offers significant opportunities for transforming research and revolutionizing the clinical management of PD. Significant efforts are being invested in the development and validation of digital outcomes to support diagnosis and track motor and mobility impairments “off-line”. Imagine being able to remotely assess your patient, understand how well they are functioning, evaluate the impact of any recent medication/intervention, and identify the need for urgent follow-up before overt, irreparable change takes place? This could offer new pragmatic solutions for personalized care and clinical research. So the question remains: how close are we to achieving this? Here, we describe the state-of-the-art based on representative papers published between 2017 and 2020. We focus on remote (i.e., real-world, daily-living) monitoring of PD using body-worn sensors (e.g., accelerometers, inertial measurement units) for assessing motor symptoms and their complications. Despite the tremendous potential, existing challenges exist (e.g., validity, regulatory) that are preventing the widespread clinical adoption of body-worn sensors as a digital outcome. We propose a roadmap with clear recommendations for addressing these challenges and future directions to bring us closer to the implementation and widespread adoption of this important way of improving the clinical care, evaluation, and monitoring of PD.
INTRODUCTION: THE “VISION”—ARE WE THERE YET?
At the 2013 World Congress of the International Society of Posture and Gait Research, a keynote speaker predicted that digital health technology such as body-worn sensors (BWS) would soon become a routine, widely used tool to augment the clinical examination of patients with Parkinson’s disease (PD) and, more importantly, enhance patients’ quality of life [1]. Accumulating evidence at that time demonstrated that low-cost, easy-to-use BWS could be used in the clinic to provide new information and add needed objectivity to the assessment of PD motor symptoms, gait, and mobility. Use of BWS could, therefore, help patients by enhancing their therapeutic management, function and quality of life, and offer them a personalized approach to their symptoms. Moreover, an emerging, exciting set of studies demonstrated that continuous, “24/7” remote monitoring empowered by BWS had the potential to measure, characterize, and quantify both subtle and large changes in mobility and other critical motor symptoms of a patient with PD, providing a robust comprehensive map of the patient’s function and its changes over time. In this vision, a patient would receive a small package in the mail containing a BWS several weeks before a routine clinical exam. After following simple instructions and wearing the device continuously for one week, the information collected would be uploaded to a cloud, automatically analyzed, summarized, sent to the clinician for review and provide feedback to the patient. At the clinical exam, the healthcare professional would read an objective, detailed report on the patient’s motor function that could be compared and contrasted to the results of previous years, even before the patient took a single step into the clinic. In this way, the clinical visit would be transformed into a more productive and informed meeting, enhancing clinical care in a truly personalized manner.
This optimistic vision has not yet been realized [2, 3]. Nonetheless, at a time when COVID-19 is wreaking havoc throughout the globe, the need has become even greater. In this short review, we provide an overview of the current use of BWS (accelerometers, inertial measurement units (IMUs)) for the remote monitoring of PD motor symptoms, summarize the challenges that must be overcome to achieve that potential, and outline steps that should and are being taken, in the long road ahead [2] to address this important opportunity for improving the evaluation and monitoring of PD motor symptoms.
STATE OF THE ART: WHERE ARE WE AT?
The rapidly expanding field of remote monitoring has been the subject of several recent systematic reviews [4–7]. Here, we provide an overview of recent representative work (2017–2020) on remote monitoring of PD motor symptoms using BWS (Table 1). We report studies using BWS for remote monitoring (real-world, at-home and in the community) in both unsupervised or scripted conditions, in order to quantify digital outcomes, focusing on papers related to motor symptoms and motor complications: tremor, bradykinesia, dyskinesia, postural instability, gait disturbances and turning, falls risk, freezing of gait (FoG) and physical activity. Using a previously proposed framework [8], in Table 1 we report:
Table 1
Representative studies examining remote monitoring of Parkinson’s disease (PD) motor symptoms using body worn sensors (BWS), between 2017 and 2020. Validation of digital outcomes has been classified ‘yes’ for each of the following criteria: 1. criterion validity: if digital outcome has been validated against a gold standard reference in the study cited, or in previous studies; 2. construct validity: if digital outcome has been validated (e.g., correlated) against clinical scales (convergent validity) and/or it has shown significant differences between groups (discriminant validity) in the study cited, or in previous studies. Digital outcome regulated/qualified has been classified ‘yes’ if BWS and/or digital outcome has received FDA (510K1) or EMA2 positive decision/qualification
Study, Year | Dataset | Protocol | BWS Type/Position | Clinical Concept of Interest | Digital Outcome | Digital Outcome Validated (1. criterion validity, 2. construct validity) | BWS and/ or Digital Outcome Regulated/ Qualified |
Tremor, bradykinesia, dyskinesia, motor fluctuations | |||||||
Samà, et al., 2017 [13] | 12 PD | 1 day* (40 min), scripted tests ON and OFF state | IMUs (9 x 2)/Waist | Bradykinesia | Gait, frequency domain features for SVM model | 1. Yes, at home (SVM, against videos) | No |
2. Yes (convergent validity (UPDRS, UPDRS-III)) | |||||||
Tsiouris et al., 2017 [14] | 20 PD | Scripted tests | PD manager: IMUs (Microsoft Band), Sensor insoles (Moticon), Smart Pillbox (SimpleMed+, Vaica), Smartphone/Wrist, Feet | Tremor, dyskinesia, bradykinesia, gait, FoG | Amplitude and constancy of tremor/not detailed features for ML techniques | 1. No 2. Yes (convergent validity (UPDRS)) | No |
Wagner et al., 2017 [17] | 19 PD | 2 days | Accelerometer (GENEActiv)/Wrist | Tremor, bradykinesia, dyskinesia | Wavelet features (contribution and relative energy of each scale) | 1. Yes, in the lab (SVM, against clinician scores) | No |
2. No | |||||||
Farzanehfar et al., 2018 [12] | 103 PD | 6-7 days, unsupervised | Accelerometer (PKG, Global Kinetic Corporation)/Wrist | Bradykinesia, dyskinesia | Bradykinesia score classified as movements with lower acceleration and amplitude. Dyskinesia classified as movements of normal amplitude and acceleration, but shorter periods without movement | 1. Yes, previous work [49] 2. Yes, (convergent validity (UPDRS III)) | Yes |
Rodríguez-Molinero et al., 2018 [15] | 23 PD | 1–3 days | IMUs (9×2)/Waist | Bradykinetic gait, dyskinesia, ON-OFF state | Bradykinesia fluidity measure (frequency domain measure, power spectra 1–10Hz for each stride), dyskinesia (power spectra 1–4Hz for each stride) | 1. Yes (SVM, against diaries) 2. No | No |
Rodríguez- Molinero, et al., 2019 [16] | 13 PD | 30 min of scripted activities | IMUs (9×2)/Waist | Dyskinesia | Power spectrum density in the frequency band comprised of harmonics of 1–4Hz | 1. Yes, in home environment using video data | No |
2. Yes, concurrent validity against clinical scales | |||||||
Coates, et al., 2020 [46] | 5 PD, 5 OA | 7 days, unsupervised | Axivity AX3/Lower back | Motor symptom severity (MDS-UPDRS III) | Sample entropy (SampEnt) | 1. No | No |
2. Yes (convergent validity (against UPDRS III &levodopa equivalent daily dose (LEDD)) and discriminant validity (PD vs. CL)) | |||||||
Evers et al., 2020 [11] | 25 PD, 25 CL | 1 day, scripted tests at home | IMUs (Gait Up Physilog 4, Android Wear smartwatch), contextual (smartphone) and physiological (Empatica E4) sensors/Lower back, wrists, ankles, pocket | ON-OFF state, FoG | Gait: Frequency domain measures (power spectral density (PSD), total power in the 0.5-10 Hz band, frequency, height and width of PSD dominant frequency) | 1. No 2. Yes (convergent validity (ON vs. OFF state) and discriminant validity (PD vs. CL)) | No |
Postural instability, gait disturbances, and turning | |||||||
Rodríguez-Molinero et al., 2017 [26] | 75 PD | 1 day*, clinical assessment and scripted tests at home in ON and OFF state | IMUs (9×3)/Waist | UPDRS-III (axial function, balance, and gait) | Scalar value for ON-OFF state based on frequency domain features (power spectra 1–10Hz for each stride). | 1. Yes, in the lab, previous work, SVM against videos [50] 2. Yes (convergent validity (UPDRS-III, UPDRS-III factor 1: “axial function, balance, and gait.”)) | No |
Haertner et al., 2018 [27] | 55 PD | 12 days (median) | IMUs (RehaGait®, Hasomed)/Lower back | Turning, falls risk | Duration, angle, average angular velocity, starting, middle and ending angular velocity and maximum angular velocity | 1. Yes, in home-like environment, previous work [51] | No |
2. Yes (discriminant validity (various PD fallers types)) | |||||||
Mancini et al., 2018 [25] | 94 PD (25 freezers) | 3 days, unsupervised, clinical assessment and scripted test at home | IMUs (Dynaport Hybrid, McRoberts)/Lower back | Turning, FoG | Mean and coefficient of variation (CV) of: number of turns per 30 min, turn angle amplitude, turn duration, mean and peak turn velocity, turn jerkiness, turn medio-lateral range of acceleration. | 1. Yes, in the lab for turning, previous work [52] 2. Yes (convergent validity (NFOG-Q) and discriminant validity (freezers vs. non-freezers)). | No |
Shah et al., 2020 [22] | 29 PD, 20 OA | 7 days, unsupervised* | IMUs (Opal, APDM)/Lower back, Feet | Gait | Gait speed, stride length, cadence, double-support, swing duration, pitch of feet at initial ground contact, frequency of bout length (number of strides) over a week | 1. Yes, in the lab, previous work [53] | No |
2. Yes (convergent validity (UPDRS-III, PIGD, previous work) and discriminant validity (PD vs. OA)) | |||||||
Shah et al., 2020 [24] | 29 PD, 27 CL | 7 days, unsupervised* | IMUs (Opal, APDM)/Lower back, Feet | Gait, turning | 43 digital mobility characteristics (lower body, upper body, turning, activity, variability) | 1. Yes, in the lab, previous work [52, 53] 2. Yes (discriminant validity (PD vs. CL)) | No |
Shah et al., 2020 [23] | 29 PD, 20 CL, 13 MS, 21 CL | 7 days, unsupervised* | IMUs (Opal, APDM)/Lower back, Feet | Gait, turning | 46 digital mobility characteristics (lower body, upper body, turning, activity, variability) | 1. Yes, in the lab, previous work [52, 53] | No |
2. Yes (convergent validity (UPDRS-III, PIGD) and discriminant validity (PD vs. CL)) | |||||||
Falls risk, freezing of gait (FoG) | |||||||
Rodríguez-Martín et al., 2017 [36] | 21 PD | 1 day* (40 mins), scripted tests ON and OFF state | IMUs (9×2)/Waist | FoG | 55 features for real-time SVM model | 1. Yes, at home (against videos) 2. No | No |
Rodríguez-Martín et al., 2017 [37] | 12 PD | 3 days*, unsupervised, and in-lab scripted tests | IMUs (9×3)/Waist | FoG, bradykinetic gait | 55 features for real-time SVM model, frequency domain measures (strides) | 1. Yes, at home (against videos) 2. No | No |
Mancini et al., 2018 [54] | 24 PD | 7 days*, unsupervised, clinical assessment and scripted test at home | IMUs (Opal, APDM)/Lower back, Ankles | FoG | Average of time spent freezing per hour (Total % time with Freezing ratio > 1 normalised on recording time), variability of % time spent freezing, turning and walking features | 1. Yes, in the lab, for turning, previous work [52] No for FoG and walking | No |
2. Yes for FoG, turning, and walking (convergent validity (NFOG-Q and ABC) and known group differences (freezers vs. non-freezers)). | |||||||
Del Din et al., 2019 [29] | 155 PD F, 122 OA F, 15 PD NF, 50 OA NF | 7 days, unsupervised | Accelerometer, (AX3, Axivity)/Lower back | Falls risk, ambulatory activity | 14 Micro gait characteristics (pace, rhythm, variability, asymmetry, postural control), 7 Macro gait characteristics (volume, pattern, variability) | 1. Yes, in the laboratory for Micro gait characteristics, previous work [55]. In real-world for Macro gait characteristics in YA, previous work [56] | No |
2. Yes (convergent validity (FES-I), and discriminant validity (PD vs. OA, F vs. NF)) | |||||||
Del Din et al., 2020 [30] | 128 PD F,109 OA F,38 MCI F | 7 days, unsupervised | Accelerometer, (AX3, Axivity)/Lower back | Falls risk, ambulatory activity | 7 Macro gait characteristics (volume, pattern, variability), fall rates relative to activity exposure (FRA) index | 1. Yes, in real-world for Macro gait characteristics in YA, previous work [56] | No |
2. Yes (convergent validity (FES-I), previous work, and discriminant validity (PD vs. OA)) | |||||||
Reches et al., 2020 [38] | 71 PD | FoG provoking test in the lab in ON and OFF states | IMUs (Opal, APDM)/Lower back, Feet | FoG | 86 features from previous work for SVM model. | 1. Yes, in the lab against labelled video | No |
2. Yes (convergent validity (NFOG, UPDRS-III and TUG time), discriminant validity (OFF vs. ON state)) | |||||||
Sigcha et al., 2020 [57] | 21 PD | 20 minutes at home, scripted ADLs in ON and OFF states | IMUs (9×2)/Waist | FoG | Mean, standard deviation, variance, frequency, entropy, energy, freeze index, sum of freeze index, locomotion band and variables related to FFT. ML and DL models from previous work. | 1. Yes, at-home against labelled video 2. No | No |
Physical activity | |||||||
Cai et al., 2017 [42] | 21 PD, 20 CL | 5 days, unsupervised | Bong Smart Sports bracelet/Wrist | Physical activity | Average daily physical activity amount and calories | 1. Yes, using self-report diaries 2. Yes (convergent validity (UPDRS –III, H&Y, Levodopa) and discriminant validity (PD vs. CL)) | No |
Silva de Lima et al., 2018 [41] | 304 PD | 13 weeks | Pebble watch/Wrist | Physical activity/motor fluctuations (ON/OFF state) | Mean time spent walking | 1. No | No |
2. Yes (convergent validity (UPDRS item 4.4)) | |||||||
Galperin, et al., 2019 [43] | 125 PD | 7 days, unsupervised | Accelerometer, Axivity (AX3)/Lower back | Physical activity | Acceleration derived features: Number of steps, number of walking bouts, step length, step regularity, amplitude of dominant frequency, SD of the peaks amplitude CV) and signal vector magnitude (SVM) | 1. Yes, in the laboratory against another BWS (GENEActiv which has been validated in previous work [58] 2. Yes (convergent validity (UPDRS-III)) | No |
Pradhan et al., 2019 [40] | 30 PD, 30 OA | 14 days, unsupervised | Fitbit Charge HR/Wrist | Physical activity | Daily step count and METs | 1. Yes, in the laboratory and outdoor, previous work [59] | Yes (only ECG App) |
2. No | |||||||
Ito et al., 2020 [44] | 13 PD | 1–7 days* | Accelerometer (Active Style Pro HJA 750C, OMRON)/Waist | Physical activity and motor symptoms (ON state, dyskinesia) | MET, PAL | 1. Yes in the laboratory, previous work [60] | No |
2. Yes (convergent validity (UPDRS-III, ON state, dyskinesia)) |
*Night excluded. ABC, Activity specific Balance Confidence scale; ADLs, Activities of Daily Living; CL, Controls; DL, Deep Learning; ECG, Electrocardiogram; F, Fallers; FES, Falls Efficacy Scale; H&Y, Hoehn and Yahr; MET, Metabolic Equivalent; ML, Machine Learning; MS, People with Multiple Sclerosis; NF, Non-fallers; NFOG-Q, New freezing of gait questionnaire; MDS-UPDRS, Movement Disorder Society Unified Parkinson’s disease Rating Scale; OA, Older Adults; PAL, Physical Activity Level; PD, People with Parkinson’s disease; PKG, Parkinson’s KinetiGraph; SVM, Super Vector Machine; TUG, Timed Up and Go; UPDRS-III, Unified Parkinson’s disease Rating Scale, Part III. 1https://www.accessdata.fda.gov/scripts/cdrh/cfdocs/cfpmn/pmn.cfm. 2https://www.ema.europa.eu/en/human-regulatory/research-development/scientific-advice-protocol-assistance/novel-methodologies-biomarkers/opinions-letters-support-qualification-novel-methodologies-medicine-development
(a) the clinical concept of interest;
(b) quantified digital outcomes;
(c) validity:
i. criterion validity: digital outcome validated against a reference system,
ii. construct validity: digital outcome validated against clinical scales (convergent validity) and/or showed known groups differences (discriminant validity));
(d) digital outcome regulatory/qualification status assessed by regulatory bodies (e.g., EMA, FDA) [9, 10].
Tremor, bradykinesia, dyskinesia, motor fluctuations
BWS have been used to automatically detect and evaluate tremor, bradykinesia, dyskinesia and on/off medication state [11–17]. The main techniques for identification of these symptoms are based on machine learning (ML) models (e.g., support vector machines (SVM)). Models are usually fed with digital outcomes that are signal-based features (e.g., frequency domain) extracted from the BWS.
In terms of criterion validity, studies tend to validate the digital outcome against a reference system (e.g., videos, self-report), using mainly ML techniques, showing good accuracy (>90%) [13, 15, 17]. Construct validity is generally tested utilizing clinical scales (e.g., Unified Parkinson’s disease Rating Scale (UPDRS)), but is less explored.
Although preliminary results are promising for some digital outcomes, excluding Farzanehfar et al. [12], these studies include a limited number of subjects (≤25) and, therefore, the generalizability of ML models and related validity for clinical adoption is problematic. We note using BWS to study rigidity, one of the cardinal symptoms of PD, is especially challenging [18].
Postural instability, gait disturbances, and turning
The importance of postural instability, gait, and turning as diagnostic, prognostic, and progression markers in PD is well recognized [19–21]. Nonetheless, static balance tests are usually confined to laboratory environments. Difficulty in identifying and discriminating periods of static, “quiet” standing balance from sedentary behavior during everyday activities (especially using single BWS on the trunk) and achieving a “totally unsupervised” postural instability assessment is challenging.
Evaluations of digital outcomes are based on: first identification in the BWS signal of the clinical concept of interest (e.g., gait, turning) using either ML methods or previously validated signal-based methods (e.g., methods developed from lab-based validation against gold standards); and second on the quantification of digital outcomes in the identified segments of the signals. Digital outcomes often include signal-based features (e.g., extracted from the BWS signal – frequency domain) or clinically relevant and “translatable” features (e.g., walking speed).
Recent studies on the construct validity of turning and gait corroborated that real-world gait and turning performances of PD were impaired (e.g., slower, more variable, and with lower cadence), compared with older adults [22–24] and reported moderate correlations with clinical scales (e.g., UPDRS) [25, 26]. Only a few studies have reported criterion validity for digital outcomes, and this was limited to in laboratory or home-like environments [25, 27, 28]. Real-world validation remains challenging and relies mainly on videos as a reference.
Falls risk, freezing of gait
BWS can help advance our understanding of fall risk. For these concepts of interest, the main techniques are again use of ML models to identify relevant segments of the BWS signals, and then quantification of digital outcomes by using signal-based features or validated clinically relevant digital outcomes (e.g., walking speed, variability).
Results show that quality (micro) and quantity (macro) digital outcomes describing gait and turning are associated not only with falls status (fallers vs. non-fallers) but also with PD specific characteristics (e.g., PD fallers showing higher variability than older adult fallers) [27, 29]. Real-world digital outcomes show promising results to quantify novel composite indexes (e.g., combining information on falls rate with walking activity) sensitive to change in fall risk in intervention studies [30–32]. Despite the availability of real-world falls repositories [33], methodologies for real-world automatic fall detection remain challenging, prone to the detection of false positives [34], and not thoroughly addressed in PD [35].
FoG is also notoriously difficult to fully replicate and detect, increasing the potential value of remote monitoring. To elicit FoG episodes, studies have tested participants in both ON and OFF conditions during scripted tests in the lab and home and used ML models [36–38]. The sensitivity and specificity for FoG detection both increase (88.09% and 88.01% respectively) when personalized (BWS data labeled by the participant, so user-dependent) rather than generic (automatic, user-independent) models are used [36, 37]. Comparisons between freezers and non-freezers indicate that the “quality of turning” digital outcome (e.g., turning angle smaller), rather than quantity of mobility, was impaired in PD freezers [25].
While construct validity is often reported (in terms of moderate relationship with clinical scales and discriminant validity) for fall risk and FoG, criterion validity is often limited to testing in the laboratory, rather than real-world environments for falls risk. Videos and ML techniques are mainly devoted to FoG.
Physical activity
Daily-living physical activity is one of the more mature applications of BWS. Outcomes such as the intensity of movement (e.g., energy expenditure, METs, step count) and temporal periods (bouts) of physical activity can be quantified [39]. Quantification of physical activity DMOs are based on features that describe the “magnitude” of BWS signals (e.g., counts, METs) or walking related features (based on identification of events—e.g., steps in the BWS signal for step count).
Commercial devices are widely utilized in PD for quantifying physical activity [40–42]. Although people with PD have lower levels of physical activity compared to older adults (discriminant validity), construct validity provides contrasting results with either no [42], moderate [43] or strong [44] relationships with clinical scales (e.g., UPDRS, Hoehn and Yahr staging) [40]. Criterion validity was again limited to laboratory-based tests (e.g., MET) rather than real-world environments [44], where the use of self-report diaries limit validity assessment due to subjectivity and recall issues [42].
Current limitations
Across the clinical concepts of interests presented, the vast majority of studies use a single or combination of BWS for data logging and off-line analysis with developed analytics. Only a few examples developed connected systems (e.g., multiple sensors systems, smartphones) and online/m-health (“cloud”) platforms to achieve true remote monitoring in real-time (e.g., REMPARK, PD_Monitoring) [14, 45]. These m-health platforms have been used in small studies [45], focusing on selective aspects (e.g., ON-OFF state). Although good usability and user satisfaction results were reported, feasibility aspects (e.g., limited sensor battery time of 20 hours) for clinical adoption were not thoroughly investigated.
Generally, studies using BWS are cross-sectional, with only a few examples assessing the ability to detect change and responsiveness in longitudinal or interventional studies [30, 46]. This aspect needs to be better explored. Only when digital outcomes derived from BWS demonstrate robust criterion and construct validity and equal or superior clinimetric properties (e.g., sensitivity to change, prediction of outcomes) compared to conventional clinical outcomes will their application become widespread.
Importantly, except for the Parkinson’s Kinetigraph for motor symptoms (tremor, bradykinesia and dyskinesia) [12] and Fitbit for electrocardiogram App [40], another common characteristic across clinical concepts of interest and BWS is the lack of qualification reports accepted for a PD context of use by regulatory bodies (e.g., EMA, FDA). This absence precludes the widespread clinical adoption of BWS and their related digital outcomes [2]. Therefore, despite the promise of BWS for remote monitoring, technical, validity, and regulatory limitations remain significant barriers to their uptake.
FUTURE PERSPECTIVES: HOW CAN WE MOVE FORWARD?
This brief review of the state-of-the-art shows that, although promising, widespread adoption of BWS in clinical settings is yet to transpire, likely because of several factors. To date, there has been no comprehensive demonstration of criterion (“technical”) and construct (“clinical”) validity, with differences in BWS and measurement techniques accounting for differences in reporting of results of the same mobility variables [7]. As highlighted earlier, the majority of algorithms that have been developed have not been validated in real-world conditions, which may be due in part to a lack of gold-standard references against which to test. Establishing technical and clinical validity, in addition to the demonstration of feasibility and usability of BWS in patients is essential to obtain qualification approval by regulatory bodies, and, as a consequence, more widespread use of BWS by clinicians [3].
Moreover, to truly transform clinical and research conventions, there needs to be sufficient evidence to show that remote monitoring is clearly “better” in some way (e.g., cost, discriminative and predictive value, clinimetric properties, healthcare economics) than traditional scales and methods. Last year, the Movement Disorder Society (MDS) Task Force on technology published concrete steps to facilitate adoption of BWS in clinical practice [3]. Nonetheless, although there are many examples and attempts in the growing literature to address selective aspects of the Task Force recommendations (e.g., validity and utility), definitive prospective and comprehensive studies are lacking. To move this field forward significantly, previous suggestions included the need to improve cross-discipline communication and larger collaborative efforts. Recent Innovative Medicines Initiative projects (e.g., Mobilise-D (https://www.mobilise-d.eu/), IDEA-FAST (https://idea-fast.eu/)) are paving the way to achieve this.
To address this need, we tried to summarize previous recommendations [2, 3, 47, 48] by proposing a roadmap with clear milestones to guide the practical clinical adoption of BWS and digital outcomes (Fig. 1):
Fig. 1
Roadmap for adoption of body worn sensors (BWS) and digital outcomes in clinical practice.
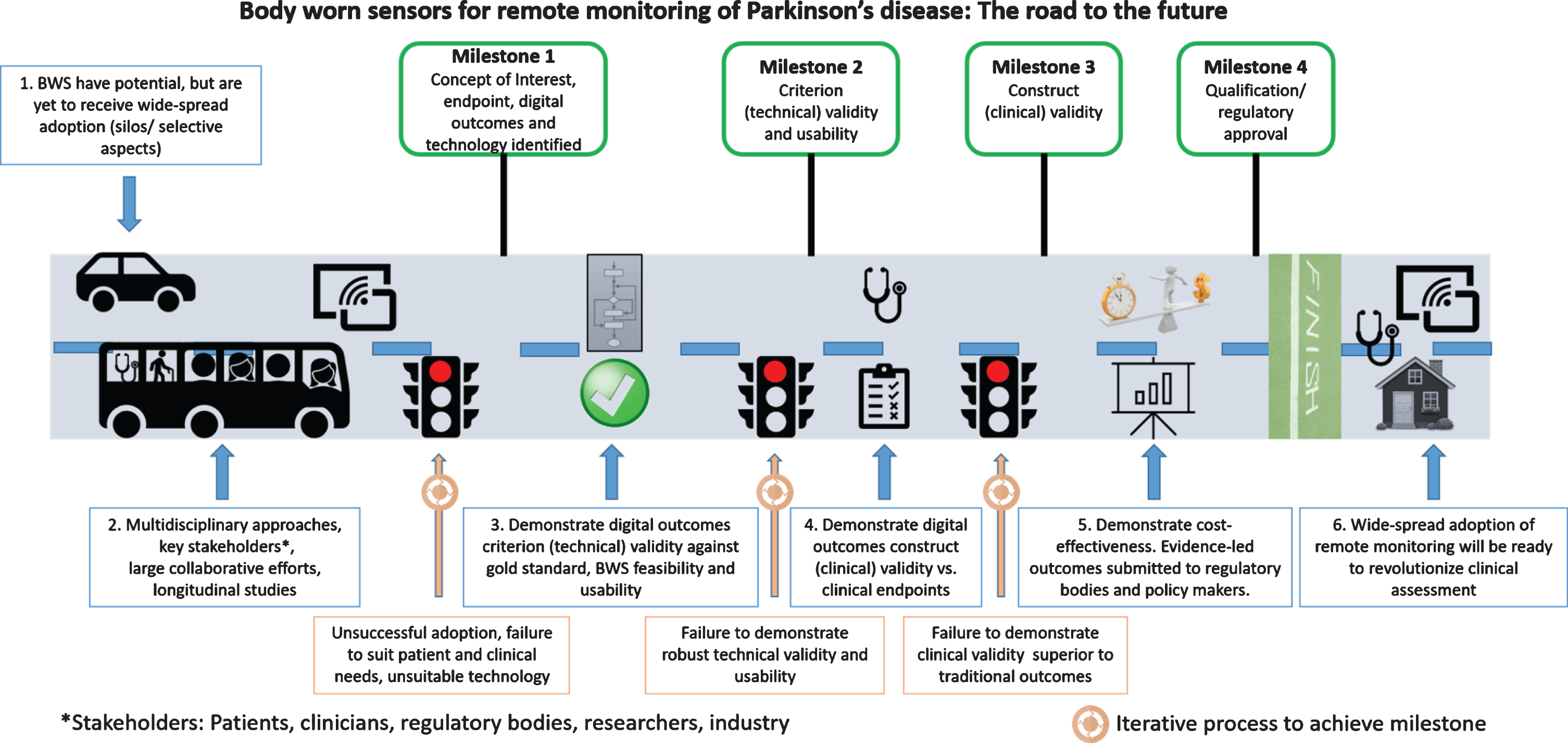
1a. A joint effort between clinicians and end-users (e.g., patient groups [47]) should identify critical relevant clinical concepts of interest (e.g., gait), related digital outcomes (e.g., real-world walking speed), the context of use, and clinical endpoints (e.g., falls risk).
1b. Select or develop appropriate technology (BWS) with key stakeholders (industry, res-earchers, clinicians, end-users) for quantification of identified digital outcomes.
2. If not already established, demonstrate criterion (technical, e.g., cross-sectional studies) validity of the digital outcome against a gold-standard reference, in real-world conditions, feasibility, and usability of the BWS for the end-users. This could be achieved, for example, by selecting a digital outcome (e.g., walking speed) and collecting data under controlled conditions with BWS and a gold standard that can quantify the same digital outcome and that can then be used also in real-world conditions.
3. Demonstrate at least equivalent, but preferably superior construct (“clinical”, e.g., longitudinal studies) validity of the digital outcome with respect to traditional measures. This could be done, for example, by demonstrating, in a longitudinal study, that the selected digital outcome at baseline (e.g., real walking speed) has stronger correlation (or predictive power) with the clinical endpoint of interest (e.g., perspective number of falls) than clinical scales or questionnaires (e.g., UPDRS III).
4. Describe the context of use and validation work for submission to qualification/regulatory bodies for approval (EMA, FDA).
5. Demonstrate cost-effectiveness (e.g., saving time of the clinician, improving the quality of life of patients). The barrier for adoption of low-cost solutions is lower than that of solutions that require large monetary investments. This could be demonstrated by carrying out a cost-effectiveness analysis, for example, by quantifying the cost of the BWS (both in monetary and time/effort terms) versus that of a gold standard or clinical assessment and showing evidence of a lower healthcare expenditures and better outcomes achieved with BWS (e.g., as described in recommendation 3).
CONCLUSIONS
Although BWS and digital outcomes have shown potential for clinical management, they have not yet achieved widespread clinical adoption. We can imagine a future where true remote monitoring of digital outcomes is used to enhance PD diagnosis, monitor progression, and facilitate clinical management. We hope that the recommendations and practical roadmap that are outlined in Fig. 1 will help to move the field forward toward that vision and to better care and monitoring of people with PD.
CONFLICT OF INTEREST
JH reports having submitted a patent for assessment of mobility using wearable sensors in Parkinson’s disease. The intellectual property rights are held by the Tel Aviv Medical Center. All of the authors declare that they have no conflicts of interest.
ACKNOWLEDGMENTS
The work was supported by Innovative Medicines Initiative 2 Joint Undertaking (JU) under Grant agreement 820820 (Mobilise-D). The JU receives support from the European Union’s Horizon 2020 research and innovation programme and the European Federation of Pharmaceutical Industries and Associations (EFPIA). SDD, AJY and LR are also supported by the Newcastle Biomedical Research Centre (BRC) based at Newcastle upon Tyne and Newcastle University. The work was also supported by the NIHR/Wellcome Trust Clinical Research Facility (CRF) infrastructure at Newcastle upon Tyne Hospitals NHS Foundation Trust. The views expressed are those of the author(s) and not necessarily those of the NIHR or the Department of Health and Social Care or the funders.
REFERENCES
[1] | Hausdorff JM ((2013) ) Keynote address: What happens when body-fixed sensors meet parkinsonian gait? A look back to the future. World Congress of the International Society of Posture and Gait Research, Akita, Japan. |
[2] | Fasano A , Mancini M ((2020) ) Wearable-based mobility monitoring: The long road ahead. Lancet Neurol 19: , 378–379. |
[3] | Espay AJ , Hausdorff JM , Sánchez-Ferro Á , Klucken J , Merola A , Bonato P , Paul SS , Horak FB , Vizcarra JA , Mestre TA , Reilmann R , Nieuwboer A , Dorsey ER , Rochester L , Bloem BR , Maetzler W ((2019) ) A roadmap for implementation of patient-centered digital outcome measures in Parkinson’s disease obtained using mobile health technologies. Mov Disord 34: , 657–663. |
[4] | Monje MHG , Foffani G , Obeso J , Sánchez-Ferro Á ((2019) ) New sensor and wearable technologies to aid in the diagnosis and treatment monitoring of Parkinson’s disease. Annu Rev Biomed Eng 21: , 111–143. |
[5] | Rovini E , Maremmani C , Cavallo F ((2019) ) Automated systems based on wearable sensors for the management of Parkinson’s disease at home: A systematic review. Telemed J E Health 25: , 167–183. |
[6] | Thorp JE , Adamczyk PG , Ploeg HL , Pickett KA ((2018) ) Monitoring motor symptoms during activities of daily living in individuals with Parkinson’s disease. Front Neurol 9: , 1036. |
[7] | Warmerdam E , Hausdorff JM , Atrsaei A , Zhou Y , Mirelman A , Aminian K , Espay AJ , Hansen C , Evers LJW , Keller A , Lamoth C , Pilotto A , Rochester L , Schmidt G , Bloem BR , Maetzler W ((2020) ) Long-term unsupervised mobility assessment in movement disorders. Lancet Neurol 19: , 462–470. |
[8] | Manta C , Patrick-Lake B , Goldsack JC ((2020) ) Digital measures that matter to patients: A framework to guide the selection and development of digital measures of health. Digit Biomark 4: , 69–77. |
[9] | Cerreta F , Ritzhaupt A , Metcalfe T , Askin S , Duarte J , Berntgen M , Vamvakas S ((2020) ) Digital technologies for medicines: Shaping a framework for success. Nat Rev Drug Discov 19: , 573–574. |
[10] | Izmailova ES , Wagner JA , Perakslis ED ((2018) ) Wearable devices in clinical trials: Hype and hypothesis. Clin Pharmacol Ther 104: , 42–52. |
[11] | Evers LJ , Raykov YP , Krijthe JH , Silva de Lima AL , Badawy R , Claes K , Heskes TM , Little MA , Meinders MJ , Bloem BR ((2020) ) Real-life gait performance as a digital biomarker for motor fluctuations: The Parkinson@Home Validation Study. J Med Internet Res 22: , e19068. |
[12] | Farzanehfar P , Woodrow H , Braybrook M , McGregor S , Evans A , Nicklason F , Horne M ((2018) ) Objective measurement in routine care of people with Parkinson’s disease improves outcomes. NPJ Parkinsons Dis 4: , 10. |
[13] | Samà A , Pérez-López C , Rodríguez-Martín D , Català A , Moreno-Aróstegui JM , Cabestany J , de Mingo E , Rodríguez-Molinero A ((2017) ) Estimating bradykinesia severity in Parkinson’s disease by analysing gait through a waist-worn sensor. Comput Biol Med 84: , 114–123. |
[14] | Tsiouris KM , Gatsios D , Rigas G , Miljkovic D , Koroušić Seljak B , Bohanec M , Arredondo MT , Antonini A , Konitsiotis S , Koutsouris DD , Fotiadis DI ((2017) ) PD_Manager: An mHealth platform for Parkinson’s disease patient management. Healthc Technol Lett 4: , 102–108. |
[15] | Rodríguez-Molinero A , Pérez-López C , Samà A , de Mingo E , Rodríguez-Martín D , Hernández-Vara J , Bayés À , Moral A , Álvarez R , Pérez-Martínez DA , Català A ((2018) ) A kinematic sensor and algorithm to detect motor fluctuations in Parkinson disease: Validation study under real conditions of use. JMIR Rehabil Assist Technol 5: , e8. |
[16] | Rodríguez-Molinero A , Pérez-López C , Samà A , Rodríguez-Martín D , Alcaine S , Mestre B , Quispe P , Giuliani B , Vainstein G , Browne P , Sweeney D , Quinlan LR , Arostegui JMM , Bayes À , Lewy H , Costa A , Annicchiarico R , Counihan T , Laighin G , Cabestany J ((2019) ) Estimating dyskinesia severity in Parkinson’s disease by using a waist-worn sensor: Concurrent validity study. Sci Rep 9: , 13434. |
[17] | Wagner A , Fixler N , Resheff YS ((2017) ) A wavelet-based approach to monitoring Parkinson’s disease symptoms. 2017 IEEE International Conference on Acoustics, Speech and Signal Processing (ICASSP), IEEE, pp. 5980–5984. |
[18] | Teshuva I , Hillel I , Gazit E , Giladi N , Mirelman A , Hausdorff JM ((2019) ) Using wearables to assess bradykinesia and rigidity in patients with Parkinson’s disease: A focused, narrative review of the literature. J Neural Transm (Vienna) 126: , 699–710. |
[19] | Horak FB , Mancini M ((2013) ) Objective biomarkers of balance and gait for Parkinson’s disease using body-worn sensors. Mov Disord 28: , 1544–1551. |
[20] | Wilson J , Alcock L , Yarnall AJ , Lord S , Lawson RA , Morris R , Taylor J-P , Burn DJ , Rochester L , Galna B ((2020) ) Gait progression over 6 years in Parkinson’s disease: Effects of age, medication, and pathology. Front Aging Neurosci 12: , 577435. |
[21] | Mirelman A , Bonato P , Camicioli R , Ellis TD , Giladi N , Hamilton JL , Hass CJ , Hausdorff JM , Pelosin E , Almeida QJ ((2019) ) Gait impairments in Parkinson’s disease. Lancet Neurol 18: , 697–708. |
[22] | Shah VV , McNames J , Harker G , Mancini M , Carlson-Kuhta P , Nutt JG , El-Gohary M , Curtze C , Horak FB ((2020) ) Effect of bout length on gait measures in people with and without Parkinson’s disease during daily life. Sensors 20: , 5769. |
[23] | Shah VV , McNames J , Mancini M , Carlson-Kuhta P , Spain RI , Nutt JG , El-Gohary M , Curtze C , Horak FB ((2020) ) Quantity and quality of gait and turning in people with multiple sclerosis, Parkinson’s disease and matched controls during daily living. J Neurol 267: , 1188–1196. |
[24] | Shah VV , McNames J , Mancini M , Carlson-Kuhta P , Nutt JG , El-Gohary M , Lapidus JA , Horak FB , Curtze C ((2020) ) Digital biomarkers of mobility in Parkinson’s disease during daily living. J Parkinsons Dis 10: , 1099–1111. |
[25] | Mancini M , Weiss A , Herman T , Hausdorff JM ((2018) ) Turn around freezing: Community-living turning behavior in people with Parkinson’s disease. Front Neurol 9: , 18. |
[26] | Rodríguez-Molinero A , Samà A , Pérez-López C , Rodríguez-Martín D , Quinlan LR , Alcaine S , Mestre B , Quispe P , Giuliani B , Vainstein G , Browne P , Sweeney D , Moreno Arostegui JM , Bayes À , Lewy H , Costa A , Annicchiarico R , Counihan T , Laighin G , Cabestany J ((2017) ) Analysis of correlation between an accelerometer-based algorithm for detecting parkinsonian gait and UPDRS subscales. Front Neurol 8: , 431. |
[27] | Haertner L , Elshehabi M , Zaunbrecher L , Pham MH , Maetzler C , van Uem JMT , Hobert MA , Hucker S , Nussbaum S , Berg D , Liepelt-Scarfone I , Maetzler W ((2018) ) Effect of fear of falling on turning performance in Parkinson’s disease in the lab and at home. Front Aging Neurosci 10: , 78. |
[28] | Pham MH , Elshehabi M , Haertner L , Del Din S , Srulijes K , Heger T , Synofzik M , Hobert MA , Faber GS , Hansen C , Salkovic D , Ferreira JJ , Berg D , Sanchez-Ferro Á , van Dieën JH , Becker C , Rochester L , Schmidt G , Maetzler W ((2017) ) Validation of a step detection algorithm during straight walking and turning in patients with Parkinson’s disease and older adults using an inertial measurement unit at the lower back. Front Neurol 8: , 457. |
[29] | Del Din S , Galna B , Godfrey A , Bekkers EMJ , Pelosin E , Nieuwhof F , Mirelman A , Hausdorff JM , Rochester L ((2019) ) Analysis of free-living gait in older adults with and without Parkinson’s disease and with and without a history of falls: Identifying generic and disease-specific characteristics. J Gerontol A Biol Sci Med Sci 74: , 500–506. |
[30] | Del Din S , Galna B , Lord S , Nieuwboer A , Bekkers EMJ , Pelosin E , Avanzino L , Bloem BR , Olde Rikkert MGM , Nieuwhof F , Cereatti A , Della Croce U , Mirelman A , Hausdorff JM , Rochester L ((2020) ) Falls risk in relation to activity exposure in high-risk older adults. J Gerontol A Biol Sci Med Sci 75: , 1198–1205. |
[31] | Klenk J , Becker C , Palumbo P , Schwickert L , Rapp K , Helbostad JL , Todd C , Lord SR , Kerse N ((2017) ) Conceptualizing a dynamic fall risk model including intrinsic risks and exposures. J Am Med Dir Assoc 18: , 921–927. |
[32] | Klenk J , Kerse N , Rapp K , Nikolaus T , Becker C , Rothenbacher D , Peter R , Denkinger MD ((2015) ) Physical activity and different concepts of fall risk estimation in older people–results of the ActiFE-Ulm Study. PLoS One 10: , e0129098. |
[33] | Klenk J , Schwickert L , Palmerini L , Mellone S , Bourke A , Ihlen EA , Kerse N , Hauer K , Pijnappels M , Synofzik M , Srulijes K , Maetzler W , Helbostad JL , Zijlstra W , Aminian K , Todd C , Chiari L , Becker C ((2016) ) The FARSEEING real-world fall repository: A large-scale collaborative database to collect and share sensor signals from real-world falls. Eur Rev Aging Phys Act 13: , 8. |
[34] | Broadley RW , Klenk J , Thies SB , Kenney LPJ , Granat MH ((2018) ) Methods for the real-world evaluation of fall detection technology: A scoping review. Sensors (Basel) 18: , 2060. |
[35] | Godfrey A , Bourke A , Del Din S , Morris R , Hickey A , Helbostad JL , Rochester L ((2016) ) Towards holistic free-living assessment in Parkinson’s disease: Unification of gait and fall algorithms with a single accelerometer. Annu Int Conf IEEE Eng Med Biol Soc 2016: , 651–654. |
[36] | Rodríguez-Martín D , Samà A , Pérez-López C , Català A , Moreno Arostegui JM , Cabestany J , Bayés À , Alcaine S , Mestre B , Prats A , Crespo MC , Counihan TJ , Browne P , Quinlan LR , ÓLaighin G , Sweeney D , Lewy H , Azuri J , Vainstein G , Annicchiarico R , Costa A , Rodríguez-Molinero A ((2017) ) Home detection of freezing of gait using support vector machines through a single waist-worn triaxial accelerometer. PLoS One 12: , e0171764. |
[37] | Rodríguez-Martín D , Pérez-López C , Samà A , Català A , Moreno Arostegui JM , Cabestany J , Mestre B , Alcaine S , Prats A , Cruz Crespo M , Bayés À ((2017) ) A waist-worn inertial measurement unit for long-term monitoring of Parkinson’s disease patients. Sensors (Basel) 17: , 827. |
[38] | Reches T , Dagan M , Herman T , Gazit E , Gouskova NA , Giladi N , Manor B , Hausdorff JM ((2020) ) Using wearable sensors and machine learning to automatically detect freezing of gait during a FOG-provoking test. Sensors (Basel) 20: , 4474. |
[39] | Brown LA , Cooper SA , Doan JB , Clark Dickin D , Whishaw IQ , Pellis SM , Suchowersky O ((2006) ) Parkinsonian deficits in sensory integration for postural control: Temporal response to changes in visual input. Parkinsonism Relat Disord 12: , 376–381. |
[40] | Pradhan S , Kelly VE ((2019) ) Quantifying physical activity in early Parkinson disease using a commercial activity monitor. Parkinsonism Relat Disord 66: , 171–175. |
[41] | Silva de Lima AL , Evers LJW , Hahn T , de Vries NM , Daeschler M , Boroojerdi B , Terricabras D , Little MA , Bloem BR , Faber MJ ((2018) ) Impact of motor fluctuations on real-life gait in Parkinson’s patients. Gait Posture 62: , 388–394. |
[42] | Cai G , Huang Y , Luo S , Lin Z , Dai H , Ye Q ((2017) ) Continuous quantitative monitoring of physical activity in Parkinson’s disease patients by using wearable devices: A case-control study. Neurol Sci 38: , 1657–1663. |
[43] | Galperin I , Hillel I , Del Din S , Bekkers EMJ , Nieuwboer A , Abbruzzese G , Avanzino L , Nieuwhof F , Bloem BR , Rochester L , Della Croce U , Cereatti A , Giladi N , Mirelman A , Hausdorff JM ((2019) ) Associations between daily-living physical activity and laboratory-based assessments of motor severity in patients with falls and Parkinson’s disease.. Parkinsonism Relat Disord 62: , 85–90. |
[44] | Ito H , Yokoi D , Kobayashi R , Okada H , Kajita Y , Okuda S ((2020) ) The relationships between three-axis accelerometer measures of physical activity and motor symptoms in patients with Parkinson’s disease: A single-center pilot study. BMC Neurol 20: , 340. |
[45] | Bayés À , Samá A , Prats A , Pérez-López C , Crespo-Maraver M , Moreno JM , Alcaine S , Rodriguez-Molinero A , Mestre B , Quispe P , de Barros AC , Castro R , Costa A , Annicchiarico R , Browne P , Counihan T , Lewy H , Vainstein G , Quinlan LR , Sweeney D , ÓLaighin G , Rovira J , Rodrigue ZMD , Cabestany J ((2018) ) A “HOLTER” for Parkinson’s disease: Validation of the ability to detect on-off states using the REMPARK system. Gait Posture 59: , 1–6. |
[46] | Coates L , Shi J , Rochester L , Del Din S , Pantall A ((2020) ) Entropy of real-world gait in Parkinson’s disease determined from wearable sensors as a digital marker of altered ambulatory behavior. Sensors (Basel) 20: , 2631. |
[47] | Bloem BR , Henderson EJ , Dorsey ER , Okun MS , Okubadejo N , Chan P , Andrejack J , Darweesh SKL , Munneke M ((2020) ) Integrated and patient-centred management of Parkinson’s disease: A network model for reshaping chronic neurological care. Lancet Neurol 19: , 623–634. |
[48] | Maetzler W , Klucken J , Horne M ((2016) ) A clinical view on the development of technology-based tools in managing Parkinson’s disease. Mov Disord 31: , 1263–1271. |
[49] | Griffiths RI , Kotschet K , Arfon S , Xu ZM , Johnson W , Drago J , Evans A , Kempster P , Raghav S , Horne MK ((2012) ) Automated assessment of bradykinesia and dyskinesia in Parkinson’s disease. J Parkinsons Dis 2: , 47–55. |
[50] | Samà A , Pérez-Lopez C , Romagosa J , Rodríguez-Martín D , Català A , Cabestany J , Pérez-Martínez DA , Rodríguez-Molinero A ((2012) ) Dyskinesia and motor state detection in Parkinson’s disease patients with a single movement sensor. Annu Int Conf IEEE Eng Med Biol Soc 2012: , 1194–1197. |
[51] | Pham MH , Elshehabi M , Haertner L , Heger T , Hobert MA , Faber GS , Salkovic D , Ferreira JJ , Berg D , Sanchez-Ferro Á , van Dieën JH , Maetzler W ((2017) ) Algorithm for turning detection and analysis validated under home-like conditions in patients with Parkinson’s disease and older adults using a 6 degree-of-freedom inertial measurement unit at the lower back. Front Neurol 8: , 135. |
[52] | El-Gohary M , Pearson S , McNames J , Mancini M , Horak F , Mellone S , Chiari L ((2013) ) Continuous monitoring of turning in patients with movement disability. Sensors (Basel) 14: , 356–369. |
[53] | Morris R , Stuart S , McBarron G , Fino PC , Mancini M , Curtze C ((2019) ) Validity of Mobility Lab (version 2) for gait assessment in young adults, older adults and Parkinson’s disease. Physiol Meas 40: , 095003. |
[54] | Mancini M , Curtze C , Stuart S , El-Gohary M , James McNames , Nutt JG , Horak FB ((2018) ) The impact of freezing of gait on balance perception and mobility in community-living With Parkinson’s disease. Annu Int Conf IEEE Eng Med Biol Soc 2018: , 3040–3043. |
[55] | Del Din S , Godfrey A , Rochester L ((2016) ) Validation of an accelerometer to quantify a comprehensive battery of gait characteristics in healthy older adults and Parkinson’s disease: Toward clinical and at home use. IEEE J Biomed Health Inform 20: , 838–847. |
[56] | Hickey A , Del Din S , Rochester L , Godfrey A ((2017) ) Detecting free-living steps and walking bouts: Validating an algorithm for macro gait analysis. Physiol Meas 38: , N1–n15. |
[57] | Sigcha L , Costa N , Pavón I , Costa S , Arezes P , López JM , De Arcas G ((2020) ) Deep learning approaches for detecting freezing of gait in Parkinson’s disease patients through on-body acceleration sensors. Sensors (Basel) 20: , 1895. |
[58] | Doherty A , Jackson D , Hammerla N , Plotz T , Olivier P , Granat MH , White T , van Hees VT , Trenell MI , Owen CG , Preece SJ , Gillions R , Sheard S , Peakman T , Brage S , Wareham NJ ((2017) ) Large scale population assessment of physical activity using wrist worn accelerometers: The UK Biobank Study. PLoS One 12: , e0169649. |
[59] | Lamont RM , Daniel HL , Payne CL , Brauer SG ((2018) ) Accuracy of wearable physical activity trackers in people with Parkinson’s disease. Gait Posture 63: , 104–108. |
[60] | Ohkawara K , Oshima Y , Hikihara Y , Ishikawa-Takata K , Tabata I , Tanaka S ((2011) ) Real-time estimation of daily physical activity intensity by a triaxial accelerometer and a gravity-removal classification algorithm. Br J Nutr 105: , 1681–1691. |