Domotics, Smart Homes, and Parkinson’s Disease
Abstract
Technology has an increasing presence and role in the management of Parkinson’s disease. Whether embraced or rebuffed by patients and clinicians, this is an undoubtedly growing area. Wearable sensors have received most of the attention so far. This review will focus on technology integrated into the home setting; from fixed sensors to automated appliances, which are able to capture information and have the potential to respond in an unsupervised manner. Domotics also have the potential to provide ‘real world’ context to kinematic data and therapeutic opportunities to tackle challenging motor and non-motor symptoms. Together with wearable technology, domotics have the ability to gather long-term data and record discrete events, changing the model of the cross-sectional outpatient assessment. As clinicians, our ultimate goal is to maximise quality of life, promote autonomy, and personalise care. In these respects, domotics may play an essential role in the coming years.
BACKGROUND
Parkinson’s disease (PD) is a neurodegenerative condition with widespread social and economic im-plications [1]. As with all chronic diseases, effective, patient-centred, and equitable systems for monitoring and management are desirable [2].
Chronic neurological conditions have experienced a digital revolution over the last decade [3]. Several aspects make PD an excellent candidate for the integration of technology into routine clinical care [4]. First, there is a lack of validated diagnostic and disease progression biomarkers for PD, and hence there is a reliance on clinical assessment. Second, the heterogenous clinical manifestations of PD demand a personalised approach to care. Finally, although PD is a generally progressive disorder, daily variation of symptoms is a norm experienced by many patients. The timing of medication, dietary choices, and psychological factors can influence the clinical examination findings. Gross motor fluctuations, which occur in many patients, are a source of even greater variability during the disease course. As such, it is difficult to get an accurate picture of a patient’s current status from a single outpatient consultation [5]. For these reasons, unsupervised evaluation of patients over longer periods of time, ideally in their home environment, could help us to better understand the complexity, diversity, and true functional implications of PD [6].
Although there has been substantial progress with regards to digital technology in PD, the focus to date has been on wearable devices and smart phone apps, or sophisticated sensors in dedicated laboratories [7]. Better use of digital technology could be implemented at home to support day to day management, and this need has never been greater than it has been during the coronavirus pandemic [8]. Even when there are no restrictions on attendance to hospital, home assessment can be used to supplement traditional face-to-face visits, or provide information on vulnerable patients or those that have busy working lives [9].
A Movement Disorders Society (MDS) Task Force on Technology recently published a roadmap to facilitate the integration of digital technologies in healthcare systems [10]. Their strategy was based on four areas: target domains, means of assessment, open and integrated display platforms, and regulated commercialisation. A lot of progress has been made in the design and development of home integrated tools. Now is time to study their potential applications for the care of patients with PD. As has been the case for wearables and apps, technological evolution risks outpacing clinical testing and implementation. To our knowledge, apart from the guidance by the MDS Task Force on Technology, there are no validated standards of assessment for domestic technology. Creation of such guidance for regulation and clinical use is necessary [7]. This review will focus on domestic integrated devices connected to the internet, otherwise known as ‘domotics’. We will summarise the potential applications, current challenges, and future directions.
DEFINITIONS
The term domotics comes originally from the Latin ‘domus’ which means house and ‘tics’ which includes robotics, telematics, and computational science. Domotics are not new; the first ‘smart house’ was designed by the French engineer Pierre Sarda in 1974 (https://youtu.be/cqPsI1YBSgc).
Domotics, smart homes, and home automation are often used as interchangeable terms and describe the integration of technology and appliances to maximise well-being and function in the home environment [11]. From a healthcare perspective, they are not only designed for increasing comfort, security, and autonomy of patients, but can also be a rich source of continuous data [3, 4]. While domotics were originally created for automating tasks, the range of possibilities, alongside internet connectivity, could hugely improve understanding and management of PD, leading to optimised clinical decision making [3].
POTENTIAL APPLICATIONS
Motor symptoms
Technology can provide real-world information that is difficult to obtain from a brief clinical consultation [6, 7]. Most of the current research in motor symptoms, including the cardinal signs and motor complications, has been centred on using body-worn sensors (for use either in free-living settings or dedicated movement laboratories), smartphone apps or other domestic hardware, such as measuring typing patterns using computer keyboards [12–17].
There are potential advantages to be gained through combining wearable technology with fixed sensors integrated in the home (such as video cameras, or sensors of movement, temperature, and pre-ssure) to contextualise patterns of movement in the home environment. This helps capture the global clinical picture and provide feedback to users, caregivers, and clinicians about patient-relevant endpoints [16–18]. Additionally, voice-controlled lights, automated electrical appliances, and smart beds, may offer tangible benefits to patients with disabling symptoms [6] (see Fig. 1).
Fig. 1
Multi-sensor system integrated at home connecting people with PD with their health-care network. 1) Wired optical sensors able to detect patient interaction with home environment and request switchboard access to emergency contacts. 2) Bed alarm system connected to a pressure sensor able to detect vigorous movements during sleep (RBD), time spent in bed (apathy/depression), or wandering at night. 3) Wearable sensors interconnected with (1), (2), (5), and (6). 4) Voice control to home appliances. 5) Switchboard when fall is detected by (1) or voice operated (4). 6) Patient interaction with computer: typing (bradykinesia) and internet browsing or shopping (ICD).
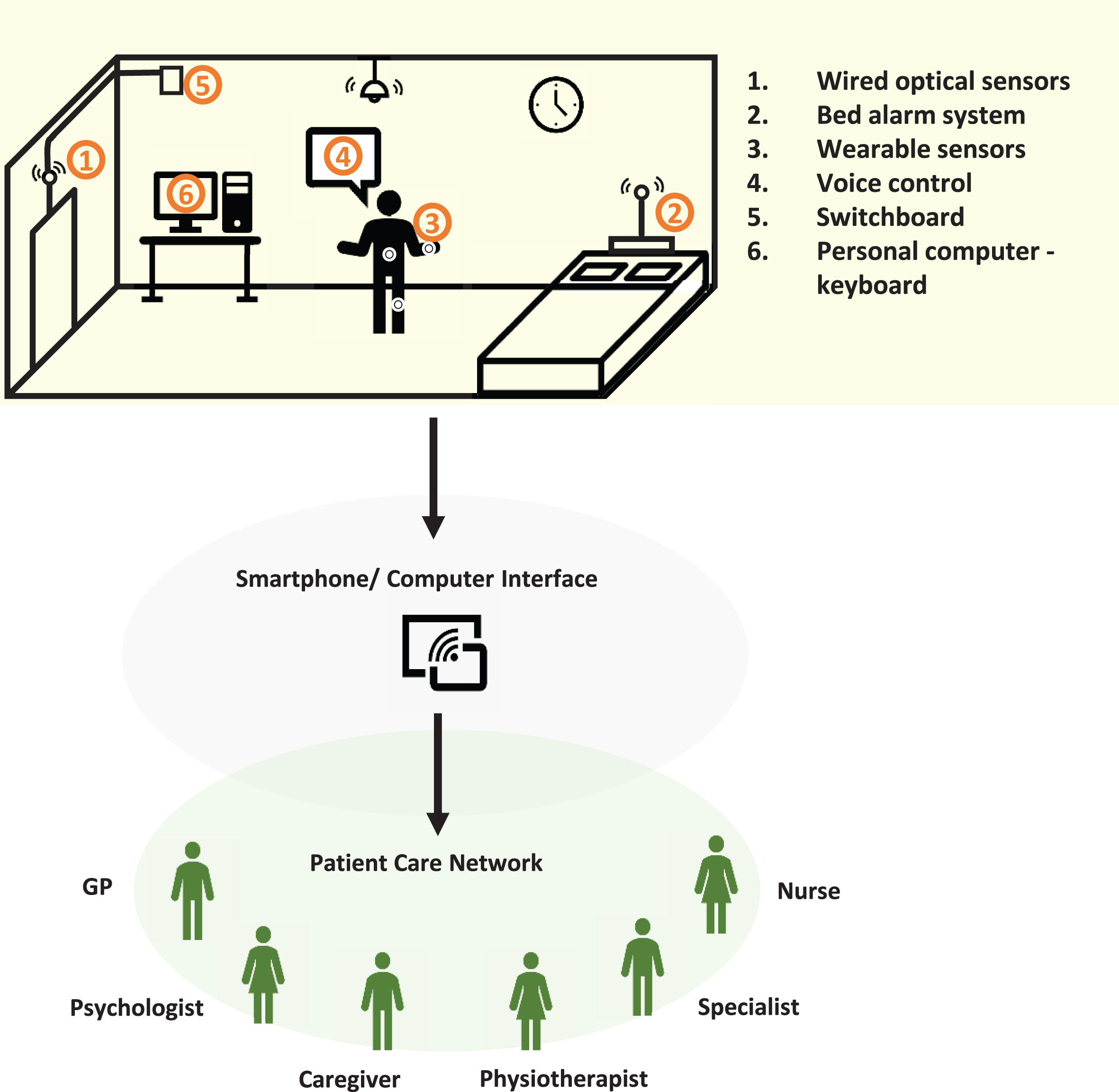
Falls detection along with the identification of precipitating factors such as sudden OFF periods and freezing of gait (FOG), are potential examples for how domotics may be used. Falls are one of the most challenging aspects of PD to treat, with limited responsiveness to medication. They are frequently encountered during the course of PD progression, and the cause of falls may be obscure; ranging from postural hypotension, gait impairment (including freezing) and postural instability [19]. Currently, falls that do not lead to hospital attendance, tend to be tracked by patients and caregivers in diaries. However, diaries are often not reliable, with a tendency for under-reporting, and a lack of clarity about fall mechanisms. Technology has gone some way to address these limitations mainly through wearable sensors and smartphone technology, but most research has been centred on describing patterns of movement in PD rather than exploring potential therapeutic interventions and preventive measures [16, 17, 20, 21]. As a detection system, domotics might help to interpret kinetic data from wearable sensors and ambulation monitoring devices to quantify and characterise falls or FOG in the home environment [16]. Imagine a smart home capable of tracking movement which could immediately assist patients during FOG and release an external cue when it occurred, such as shining a light on the floor or playing music at a given tempo [21, 22]. Similarly, for sudden OFF periods, when patients are alone, speech recognition systems designed for controlling household devices and automated connection to the internet could be reassuring [23]. Patients would be able to contact caregivers, clinicians or emergency support. The net effect of this increased connectivity is that patients could feel more secure at home, while simultaneously relieving caregiver burden.
Domestic entertainment appliances and virtual reality could be used in home physiotherapy programmes to improve balance and gait performance in people with PD. For example, in a study using a Nintendo® Wii the authors demonstrated that 20 sessions of balance training for 5 days a week improved balance and gait performance [24].The authors suggested that continuous visual feedback may facilitate movement execution and maintain focused attention. The fact that it was self-administered in the home facilitated long term compliance. Another clinical trial with a randomised, controlled design measured the feasibility of home-based training using a smartphone app (CuPiD-system) which provided real-time feedback to patients. The investigators studied the effects on gait in people with PD, finding that it was well tolerated and easy to use. Despite a limited follow-up period, patients experienced a positive effect on their balance and quality of life [21]. A separate double-blind randomised controlled trial found that ‘gamifying’ exercises using virtual reality had benefits on mobility [25].
Non-motor and neuropsychiatric symptoms
Cognitive impairment is a milestone of disease progression in PD [26]. It has a huge impact on the extent of disability and caregiver burden [27]. An important consequence of cognitive impairment in PD is that the therapeutic window narrows; most drugs used to treat neuropsychiatric symptoms of PD can worsen motor symptoms. To-date most remote technology tools for dementia have been studied in the Alzheimer’s disease field, but the potential benefits for PD are also clear [28]. Beyond tracking of movement, motion sensors in smart houses could be used to analyse behavioural patterns. Episodes of disorientation and confusion, patients wandering or leaving the house at unusual times of the day, and the amount of time spent in bed are all examples of information that could be extracted from combining domotics and wearable sensors [29]. Collateral information from relatives and caregivers is crucial for understanding the needs of patients with dementia, but at early stages and for patients living alone this information can be difficult to obtain. By monitoring domestic tasks, early detection of cognitive impairment or behaviour change could be possible, even before symptoms are noticed by others.
There are other neuropsychiatric symptoms which are under-reported by patients. Impulse control disorders (ICD) and apathy might be detectable based on abnormal day and night-time behavioural patterns such as spending long hours in front of the computer, performing repetitive tasks or staying in bed during the daytime. This could be used to detect ICD in patients or monitor treatment response for apathy and depression.
Sleep quality has mainly been studied using accel-erometres and gyroscopes worn at the wrists or on the trunk [30, 31]. Patients with REM sleep Behaviour Disorder (RBD) act out their dreams due to a lack of muscle atonia during REM sleep. Physical safeguards may be employed, but technology could support diagnosis or offer a therapeutic intervention for RBD. For example, Howell and colleagues designed a bed sensory-alarm system to prevent sleep related injuries in medically refractory RBD patients. They found their method to be an effective measure to prevent injuries in RBD as an alternative to medication or for those who did not tolerate medication [32].
Patient empowerment
We have provided some examples of how domotics might support patients as disease milestones loom. Overall, what technology, particularly domestic technology, may offer is reassurance and empowerment of patients. Mobile technologies including wearable sensors, smartphones, and domestic-integrated devices can work together to provide patients with feedback about their symptoms [5]. This digital health pathway could integrate patients, caregivers, and clinicians in a network model centred on personalised care in which patients have a proactive role in decision making and feel more confident with the management of their symptoms [33]. Having an integrated model also offers the possibility of connecting automatically or through voice command with caregivers and emergency services if an unexpected event occurs. This offers further reassurance to the caregivers of more vulnerable people and a greater sense of security [28].
The concept of health literacy is emerging and comprises patient education regarding their condition [34]. Internet and home-environment monitoring data can be an important source of information to enable effective self-management which will hopefully be demonstrable through improved quality of life [9].
CHALLENGES
There are several limitations to consider when gathering and interpreting digital health data which we have summarised here [5, 6].
Data privacy and ownership
The nature of recording aspects of daily life brings legal and ethical issues [35]. Although domotics have a potential role in helping to understand the needs and functional status of the most vulnerable patients, the amount of data and the images that result from recording can threaten individual privacy [36]. Data sharing is necessary for the cross-validation and interpretation of data from technology-based tools. Whether gathered for research or clinical care, data about patients in their home environment must be treated in the same way as other confidential information and governed by data protection laws. Issues around consent and not infringing on autonomy, even when intentions are good, are important considerations and we must be vigilant about conflicts of interest [37].
There are several considerations which can be divided into privacy and confidentiality, threats to autonomy, safety issues (‘do not harm’ principle), and the boundaries of data ownership. Table 1 summaries the most relevant issues with examples and possible solutions based on two ethical guidelines designed for digital health research [35] and home environment technology for people with dementia [28]. The main principle is to focus on the interests of the patient above the interests of research and industry. In the research setting, IRB (Institutional Review Board) approval is mandatory for any clinical study involving patients and provides important safeguards. Whilst the guiding principles of data confidentiality are ubiquitous in many countries, the interpretation of such guidance varies and must be considered. Country-specific evaluation will be required for devices before regulatory approval is granted and this is an important aspect of implementing new technology [28].
Table 1
Ethical issues and possible solutions
Ethical concerns | Approach |
Privacy issues: intrusive surveillance sensors, unwanted image data, third parties involvement | •Data encryption (blur, pixelating, silhouettes, skeleton, 3D avatar) to protect identity [36] |
Loss of autonomy: feeling of lack of data control involving private life content | •Written consent after detailed information disclosure |
•To informe about rights: to view and delete unwanted images, temporarily pause image recording whenever they wish | |
•Cognitively impaired individuals: consent given by people with decision-making authority anticipating benefits and risks | |
•Participants to ask third parties for consent | |
Security issues: full reliance on technology, sensor failure to detect a dangerous situation, software hacking | •Technology demystification |
•Glitches detection | |
•Trained investigators | |
Data ownership: right of self-management of personal data | •Support regulatory bodies |
•Testable quality standards certification [10] |
Motor considerations
Hyperkinetic movements, such as tremor or dyskinesia, have characteristic patterns in accelerometer data, but other features such as bradykinesia can be misinterpreted through unsupervised assessments [5]. When motion sensors detect slowness or lack of movement it is not necessarily due to bradykinesia, but may also be seen with fatigue, pain, and apathy. Fixed sensors, as part of a domotic setup, could help to contextualise movement patterns suggested from accelerometery data.
Spontaneous physical activity captured by remote, unsupervised devices involves a great amount of background noise and high variability between individuals [38]. Coexisting factors such as performing multiple tasks simultaneously, interference from other people, and domestic obstacles can confound data interpretation. Again, this limitation could partially be addressed by combining domotic devices with wearable sensors.
Uptake and implementation
The technology era has not been embraced by all and a substantial proportion of patients are reluctant to adopt new technology. The coronavirus pandemic has helped to increase the acceptability of technology as an alternative means of providing clinical information. Further research and consideration of the utility of domotics has never been timelier.
Setting up domotics into a private environment like someone’s home could be considered intrusive for many and may be a limitation compared to wearable technology and apps. There is also more setup time involved given the need to take account of room layout, furniture configuration and individual requirements. One might expect that over time patients will be increasingly comfortable with technology compared with the current elderly population, and as such, acceptability will improve gradually.
Feasibility and usability studies are essential to understand compliance and comfort. The SENSE-PARK study assessed a quantitative assessment (we-arable sensor, app, balance board, and computer software) of PD symptoms. As a primary outcome the number of dropouts were quantified. Secondly, feedback from participants regarding usability was evaluated using a Post-Study System Usability Questionnaire (PSSUQ) [15]. All patients completed the 12-week study, provided good feedback and highlighted the user-friendly design. A study on long term feasibility of wearable sensors in PD suggested that having a ‘helpdesk’ improved adherence of participants which would be worth considering for designing further digital-health protocols [39].
Clinical validation and relevance
Unsupervised outcomes need to be validated against standard measures, such as disease severity rating scales or diaries. However, inter-rater variability in the case of disease rating scales and self-reporting biases related to diaries need to be considered when these are used as a ‘gold’ standard measures [40]. Thus, using test-retest repeatability and accuracy could be a better way for validating information from domotic setups. However, the validation of a home sensor system is challenging on its own and is subject to patient factors such as variation in symptoms and awareness of being constantly observed (known as ‘The Hawthorne’ effect [41]), and environment factors, such as the variability of home layouts. Distinct context (supervised vs unsupervised) and different raw data (accelerometery vs video images) will demand the creation of validation standards to be used across different studies. Increasing the number of participants and raters, including assessment battery with diaries, telephone calls and the use of other devices with data filters could be possible solutions to overcome these issues and improve the quality of validation studies and ensure results are not device dependent [42]. Another way to potentially improve the power of the study is expanding the amount of data collected through continuous monitoring [10]. It is important to bear in mind that large quantities of data or “big data”, does not necessarily mean “good data”. Although there is expansion in the use of sofisticated artificial intelligence and deep learning algorithms, these in themselves generate challenges and depend on the quality of the underlying data [43, 44].
FUTURE PERSPECTIVE: PD CARE AND RESEARCH
In contrast to wearable sensors and smartphone applications, clinical trials of home-based technology, especially for people with PD, are limited. More feasibility and acceptability studies are needed to identify patient-relevant endpoints which will guide the design of clinical trials. Home-based sensors offer the opportunity to analyse a wide range of outcomes: disease progression markers, therapeutic interventions for a specific symptom (freezing, falls), and monitoring of treatment response and side effects which initially could only be used as a surrogate markers, but in the future might be even used as primary outcomes [10].
Take–home messages
• Domestic integrated devices connected to the internet (domotics) go beyond portable sensors, providing context to real-time and highly granular information.
• Integrated multisensory systems at home can be used to assist and prevent falls. They can also be used as a source of automate cueing delivery to treat FOG.
• The study of behavioural patterns in a home environment is a promising area of research with potential applications in early detection of dementia and monitoring ICDs.
• Digital medicine in combination with traditional medical care can help to empower patients and relieve caregiver burden.
• There are several limitations to tackle in the future:privacy implications, heavy and complex data (unsupervised, heterogenous, subject to external interferences), and restricted applicability in non-technology literate users.
We can learn from similar studies done in dementia and aging. The Oregon Centre for Aging & Technology (ORCATECH) is a multi-disciplinary organization focused on developing cutting-edge technologies to measure real-life data (https://www.ohsu.edu/oregon-center-for-aging-and-technology). The Collaborative Aging (in Place) Research Using Technology (CART) is an initiative which is part of ORCATECH platform and has a decade of experience in technology for aging and Alzheimer’s disease [45]. Data was gathered from multiple sources of information such as sensors in the home, in the car, and worn on the person. This system was iteratively tested and embedded in to 232 homes across the USA for 3.5 years. Cognitive performance, physical mobility, sleep duration, and social interaction were used as outcome measures. Another example is The HomeAssist project which developed an assisted living platform at the home of the elderly. A multi-disciplinary approach (geriatrics, psycho-logists, caregivers, and users) was essential to identify user needs from a variety of perspectives. Thirty-two dyads were monitored for 6 months: half of them were equipped with the HomeAsssit platform and the other half did not. Overall, their findings showed potential applications in home support and reducing burden on caregivers [46].
CONCLUSIONS
Future directions will be centred on developing multi-disciplinary digital platforms, connecting patients, carers, and clinicians [18]. More research is necessary and there is a need to share and combine data on a large scale to train recognition systems and classification methods to identify a wide range of movement signatures [47].
Domotics have the ability to increase autonomy, self-management, and provide security, whilst providing data about functional status over time. These are crucial aspects of the shift towards precision and personalised care for PD patients.
ACKNOWLEDGMENTS
We would like to thank Brook Frances Rose Huxford for her contribution reproducing the illustrative figure presented in the current article.
CONFLICT OF INTEREST
Dr Simonet has no conflict of interest to report.
Dr Noyce has the following disclosures. Grants from the Barts Charity, Parkinson’s UK, Aligning Science Across Parkinson’s and Michael J Fox Foundation. Personal fees/honoraria from Britannia, BIAL, AbbVie, Profile, Biogen, Roche and UCB, outside of the submitted work.
REFERENCES
[1] | GBD 2016 Parkinson’s Disease Collaborators ((2018) ) Global, regional, and national burden of Parkinson’s disease, 1990–2016: A systematic analysis for the Global Burden of Disease Study 2016. Lancet Neurol 17: , 939–953. |
[2] | Dorsey ER , Vlaanderen FP , Engelen LJ , Kieburtz K , Zhu W , Biglan KM , Faber MJ , Bloem BR ((2016) ) Moving Parkinson care to the home. Mov Disord 31: , 1258–1262. |
[3] | Bloem BR , Henderson EJ , Dorsey ER , Okun MS , Okubadejo N , Chan P , Andrejack J , Darweesh SKL , Munneke M ((2020) ) Integrated and patient-centred management of Parkinson’s disease: A network model for reshaping chronic neurological care. Lancet Neurol 19: , 623–634. |
[4] | Klucken J , Krüger R , Schmidt P , Bloem BR ((2018) ) Management of Parkinson’s disease 20 years from now: Towards digital health pathways. J Parkinsons Dis 8: , S85–S94. |
[5] | Warmerdam E , Hausdorff JM , Atrsaei A , Zhou Y , Mirelman A , Aminian K , Espay AJ , Hansen C , Evers LJW , Keller A , Lamoth C , Pilotto A , Rochester L , Schmidt G , Bloem BR ((2020) ) Long-term unsupervised mobility assessment in movement disorders. Lancet Neurol 19: , 462–470. |
[6] | Espay AJ , Bonato P , Nahab FB , Maetzler W , Dean JM , Klucken J , Eskofier BM , Merola A , Horak F , Lang AE , Reilmann R , Giuffrida J , Nieuwboer A , Horne M , Little MA , Litvan I , Simuni T , Ray Dorsey E , Burack MA , Kubota K , Kamondi A , Godinho C , Daneault J-F , Mitsi G , Krinke L , Hausdorff JM , Bloem BR , Papapetropoulos S ((2016) ) Technology in Parkinson disease: Challenges and opportunities. Mov Disord 31: , 1272–1282. |
[7] | Morgan C , Rolinski M , McNaney R , Jones B , Rochester L , Maetzler W , Craddock I , Whone AL ((2020) ) Systematic review looking at the use of technology to measure free-living symptom and activity outcomes in Parkinson’s disease in the home or a home-like environment. J Parkinsons Dis 10: , 429–454. |
[8] | Helmich RC , Bloem BR ((2020) ) The impact of the COVID-19 pandemic on Parkinson’s disease: Hidden sorrows and emerging opportunities. J Parkinsons Dis 10: , 351–354. |
[9] | Riggare S , Höglund PJ , Hvitfeldt Forsberg H , Eftimovska E , Svenningsson P , Hägglund M ((2019) ) Patients are doing it for themselves: A survey on disease-specific knowledge acquisition among people with Parkinson’s disease in Sweden. Health Informatics J 25: , 91–105. |
[10] | Espay AJ , Hausdorff JM , Sánchez-Ferro Á , Klucken J , Merola A , Bonato P , Paul SS , Horak FB , Vizcarra JA , Mestre TA , Reilmann R , Nieuwboer A , Dorsey ER , Rochester L , Bloem BR , Maetzler W , on behalf of the Movement Disorder Society Task Force on Technology ((2019) ) A roadmap for implementation of patient-centered digital outcome measures in Parkinson’s disease obtained using mobile health technologies. Mov Disord 34: , 657–663. |
[11] | Stamford JA , Schmidt PN , Friedl KE ((2015) ) What engineering technology could do for quality of life in Parkinson’s disease: A review of current needs and opportunities. IEEE J Biomed Health Inform 19: , 1862–1872. |
[12] | Heijmans M , Habets JGV , Herff C , Aarts J , Stevens A , Kuijf ML , Kubben PL ((2019) ) Monitoring Parkinson’s disease symptoms during daily life: A feasibility study. NPJ Parkinsons Dis 5: , 21. P1-6 |
[13] | Evers LJW , Krijthe JH , Meinders MJ , Bloem BR , Heskes TM ((2019) ) Measuring Parkinson’s disease over time: The real-world within-subject reliability of the MDS-UPDRS. Mov Disord 34: , 1480–1487. |
[14] | Farzanehfar P , Woodrow H , Braybrook M , McGregor S , Evans A , Nicklason F , Horne M ((2018) ) Objective measurement in routine care of people with Parkinson’s disease improves outcomes. NPJ Parkinsons Dis 4: , 10. P1-8 |
[15] | Ferreira JJ , Godinho C , Santos AT , Domingos J , Abreu D , Lobo R , Gonçalves N , Barra M , Larsen F , Fagerbakke Ø , Akeren I , Wangen H , Serrano JA , Weber P , Thoms A , Meckler S , Sollinger S , van Uem J , Hobert MA , Maier KS , Matthew H , Isaacs T , Duffen J , Graessner H , Maetzler W ((2015) ) Quantitative home-based assessment of Parkinson’s symptoms: The SENSE-PARK feasibility and usability study. BMC Neurol 15: , 89. P1-7 |
[16] | Rodríguez-Silva DA , Gil-Castiñeira F , González-Castaño FJ , Duro RJ , López-Peña F , Vales-Alonso J ((2008) ) Human motion tracking and gait analysis: A brief review of current sensing systems and integration with intelligent environments. 2008 IEEE Conference on Virtual Environments, Human-Computer Interfaces and Measurement Systems, Istanbul, pp. 166–171. |
[17] | Pasluosta CF , Eskofier BM , Gassner H , Winkler J , Klucken J (2015) Parkinson’s disease as a working model for global healthcare restructuration: The internet of things and wearables technologies. MOBIHEALTH 2015, October 14-16, London, Great Britain, pp. 162-165. |
[18] | Pasluosta CF , Gassner H , Winkler J , Klucken J , Eskofier BM ((2015) ) An emerging era in the management of Parkinson’s disease: Wearable technologies and the internet of things. IEEE J Biomed Health Informatics 19: , 1873–1881. |
[19] | De Pablo-Fernández E , Lees AJ , Holton JL , Warner TT ((2019) ) Prognosis and neuropathologic correlation of clinical subtypes of Parkinson disease. JAMA Neurol 76: , 470–479. |
[20] | Silva de Lima AL , Smits T , Darweesh SKL , Valenti G , Milosevic M , Pijl M , Baldus H , de Vries NM , Meinders MJ , Bloem BR ((2020) ) Home-based monitoring of falls using wearable sensors in Parkinson’s disease. Mov Disord 35: , 109–115. |
[21] | Ginis P , Nieuwboer A , Dorfman M , Ferrari A , Gazit E , Canning CG , Rocchi L , Chiari L , Hausdorff JM , Mirelman A ((2016) ) Feasibility and effects of home-based smartphone-delivered automated feedback training for gait in people with Parkinson’s disease: A pilot randomized controlled trial. Parkinsonism Relat Disord 22: , 28–34. |
[22] | Pepa L , Verdini F , Capecci M , Ceravolo MG , Leo T ((2014) ) Can the current mobile technology help for medical assistance? The case of freezing of gait in Parkinson disease. In Ambient Assisted Living, Longhi S, Siciliano P, Germani M, Monteriu` A, eds. Springer International Publishing, Cham, pp. 177–185. |
[23] | Alessandrini M , Biagetti G , Curzi A , Turchetti C ((2014) ) A speech interaction system for an ambient assisted living scenario. In Ambient Assisted Living, Longhi S, Siciliano P, Germani M, Monteriù A, eds. Springer International Publishing, Switzerland, pp. 233–239. |
[24] | Di Biagio L , Ferretti M , Cingolani D , Buzzatti L , Capecci M , Ceravolo MG ((2014) ) Virtual reality: A new rehabilitative approach in neurological disorders. In Ambient Assisted Living, Longhi S, Siciliano P, Germani M, Monteriù A, eds. Springer International Publishing, Switzerland, pp. 167–176. |
[25] | van der Kolk NM , de Vries NM , Kessels RPC , Joosten H , Zwinderman AH , Post B , Bloem BR ((2019) ) Effectiveness of home-based and remotely supervised aerobic exercise in Parkinson’s disease: A double-blind, randomised controlled trial. Lancet Neurol 18: , 998–1008. |
[26] | Schrag A , Hommel ALAJ , Lorenzl S , Meissner WG , Odin P , Coelho M , Bloem BR , Dodel R , CLaSP consortium. ((2020) ) The late stage of Parkinson’s -results of a large multinational study on motor and non-motor complications. Parkinsonism Relat Disord 75: , 91–96. |
[27] | Schrag A , Hovris A , Morley D , Quinn N , Jahanshahi M ((2006) ) Caregiver-burden in parkinson’s disease is closely associated with psychiatric symptoms, falls, and disability. Parkinsonism Relat Disord 12: , 35–41. |
[28] | Mahoney DF , Purtilo RB , Webbe FM , Alwan M , Bharucha AJ , Adlam TD , Jimison HB , Turner B , Becker SA ((2007) ) In-home monitoring of persons with dementia: Ethical guidelines for technology research and development. Alzheimers Dement 3: , 217–226. |
[29] | Alvarez F , Popa M , Solachidis V , Hernandez-Penaloza G , Belmonte-Hernandez A , Asteriadis S , Vretos N , Quintana M , Theodoridis T , Dotti D , Daras P ((2018) ) Behavior analysis through multimodal sensing for care of Parkinson’s and Alzheimer’s patients. IEEE Multimed 25: , 14–25. |
[30] | Madrid-Navarro CJ , Escamilla-Sevilla F , Mínguez-Castellanos A , Campos M , Ruiz-Abellán F , Madrid JA , Rol MA ((2018) ) Multidimensional circadian monitoring by wearable biosensors in Parkinson’s disease. Front Neurol 9: , 157. |
[31] | Klingelhoefer L , Rizos A , Sauerbier A , McGregor S , Martinez-Martin P , Reichmann H , Horne M , Chaudhuri KR ((2016) ) Night-time sleep in Parkinson’s disease - the potential use of Parkinson’s KinetiGraph: A prospective comparative study. Eur J Neurol 23: , 1275–1288. |
[32] | Howell MJ , Arneson PA , Schenck CH ((2011) ) A novel therapy for REM sleep behavior disorder (RBD). J Clin Sleep Med 7: , 639–644. |
[33] | Riggare S , Scott Duncan T , Hvitfeldt H , Hägglund M ((2019) ) “You have to know why you’re doing this”: A mixed methods study of the benefits and burdens of self-tracking in Parkinson’s disease. BMC Med Inform Decis Mak 19: , 1–16. |
[34] | Ratzan SC , Parker RM ((2006) ) Health literacy - identification and response. J Health Commun 11: , 713–715. |
[35] | Kelly P , Marshall SJ , Badland H , Kerr J , Oliver M , Doherty AR , Foster C ((2013) ) An ethical framework for automated, wearable cameras in health behavior research. Am J Prev Med 44: , 314–319. |
[36] | Padilla-López JR , Chaaraoui AA , Flórez-Revuelta F ((2015) ) Visual privacy protection methods: A survey. Expert Syst Appl 42: , 4177–4195. |
[37] | Mashhadi A , Kawsar F , Acer UG (2014) Human Data Interaction in IoT: The ownership aspect. 2014 IEEE World Forum on Internet of Things (WF-IoT), pp. 159-162. |
[38] | Toosizadeh N , Mohler J , Lei H , Parvaneh S , Sherman S , Najafi B ((2015) ) Motor performance assessment in Parkinson’s disease: Association between objective in-clinic, objective in-home, and subjective/semi-objective measures. PLoS One 10: , e0124763. |
[39] | Silva de Lima AL , Hahn T , Evers LJW , De Vries NM , Cohen E , Afek M , Bataille L , Daeschler M , Claes K , Boroojerdi B , Terricabras D , Little MA , Baldus H , Bloem BR , Faber MJ ((2017) ) Feasibility of large-scale deployment of multiple wearable sensors in Parkinson’s disease. PLoS One 12: , e0189161. |
[40] | Goetz CG , Leurgans S , Hinson VK , Blasucci LM , Zimmerman J , Fan W NT , Hsu A ((2008) ) Evaluating Parkinson’s disease patients at home: Utility of self-videotaping for objective motor, dyskinesia, and ON–OFF assessments. Mov Disord 23: , 1479–1482. |
[41] | Robles-García V , Corral-Bergantiños Y , Espinosa N , Jácome MA , García-Sancho C , Cudeiro J , Arias P ((2015) ) Spatiotemporal gait patterns during overt and covert evaluation in patients with Parkinson’s disease and healthy subjects: Is there a Hawthorne effect? J Appl Biomech 31: , 189–194. |
[42] | Lonini L , Dai A , Shawen N , Simuni T , Poon C , Shimanovich L , Daeschler M , Ghaffari R , Rogers JA , Jayaraman A ((2018) ) Wearable sensors for Parkinson’s disease: Which data are worth collecting for training symptom detection models. NPJ Digit Med 1: , 64. P1-8 |
[43] | Fröhlich H , Balling R , Beerenwinkel N , Kohlbacher O , Kumar S , Lengauer T , Maathuis MH , Moreau Y , Murphy SA , Przytycka TM , Rebhan M , Röst H , Schuppert A , Schwab M , Spang R , Stekhoven D , Sun J , Weber A , Ziemek D , Zupan B ((2018) ) From hype to reality: Data science enabling personalized medicine. BMC Med 16: , 150. P1-15 |
[44] | Auger SD , Jacobs BM , Dobson R , Marshall CR , Noyce AJ ((2020) ) Big data, machine learning and artificial intelligence: A neurologist’s guide. Pract Neurol 21: , 4–11. |
[45] | Beattie Z , Miller LM , Almirola C , Au-Yeung W-TM , Bernard H , Cosgrove KE , Dodge HH , Gamboa CJ , Golonka O , Gothard S , Harbison S , Irish S , Kornfeld J , Lee J , Marcoe J , Mattek NC , Quinn C , Reynolds C , Riley T , Rodrigues N , Sharma N , Siqueland MA , Thomas NW , Truty T , Wall R , Wild K , Wu C-Y , Karlawish J , Silverberg NB , Barnes LL , Czaja S , Silbert LC , Kaye J ((2020) ) The Collaborative Aging Research Using Technology Initiative: An open, sharable, technology-agnostic platform for the research community. Digit Biomarkers 4: , 100–118. |
[46] | Dupuy L , Froger C , Consel C , Sauzéon H ((2017) ) Everyday functioning benefits from an assisted living platform amongst frail older adults and their caregivers. Front Aging Neurosci 9: , 302. |
[47] | Nef T , Urwyler P , Büchler M , Tarnanas I , Stucki R , Cazzoli D , Müri R , Mosimann U ((2015) ) Evaluation of three state-of-the-art classifiers for recognition of activities of daily living from smart home ambient data. Sensors (Switzerland) 15: , 11725–11740. |