Precision Medicine in Parkinson’s Disease – Exploring Patient-Initiated Self-Tracking
Abstract
Background:
Individually tailored healthcare, in the form of precision medicine, holds substantial potential for the future of medicine, especially for a complex disorder like Parkinson’s disease (PD). Patient self-tracking is an under-researched area in PD.
Objective:
This study aimed to explore patient-initiated self-tracking in PD and discuss it in the context of precision medicine.
Methods:
The first author used a smartphone app to capture finger-tapping data and also noted times for medication intakes.
Results:
Data were collected during four subsequent days. Only data from the first two days were complete enough to analyze, leading to the realization that the collection of data over a period of time can pose a significant burden to patients. From the first two days of data, a dip in finger function was observed around the time for the second medication dose of the day.
Conclusions:
Patient-initiated self-tracking enabled the first author to glean important insights about how her PD symptoms varied over the course of the day. Symptom tracking holds great potential in precision medicine and can, if shared in a clinical encounter, contribute to the learning of both patient and clinician. More work is needed to develop this field and extra focus needs to be given to balancing the burden of tracking for the patient against any expected benefit.
INTRODUCTION
Precision medicine (or personalized medicine) is achieved when “health care is individually tailored on the basis of a person’s genes, lifestyle and environment” [1]. A complex and multifaceted neurodegenerative disorder like Parkinson’s disease (PD), with its plethora of symptoms (motor and non-motor) and treatment side effects, is likely to benefit from a precision medicine approach. Such an approach would include consideration of for example genes, clinical subtypes, personality and preferences, lifestyle, pharmaco-economics, aging, and comorbidities [2, 3]. Technology-based objective measures are seen as a potential contributor to precision medicine in PD [4].
To achieve precision medicine, focus needs to shift from patient cohorts to what works for an individual patient [5, 6]. Historically, important insights have come from observations made by clinicians on individual patients or on themselves. The former is often reported as a case study and the latter is known as self-experimentation. Before the Helsinki Declaration, physicians conducting experiments on themselves prior to testing their ideas on patients was one way to ensure research ethics [7]. Self-experimentation has resulted in important progress including a number of Nobel prizes [8], for example for the discovery of Helicobacter pylori as a cause for gastric ulcers [9]. Observations by patients have also contributed to important insights. A few notable examples are the surprising side effects of Sildenafil or that smoking cessation is associated with depression, both of which has led to new effective treatments [10]. Case reports are frequently used in PD to report on clinical observations or findings and we have previously published a patient-driven study, in which the first author (SR) conducted an experiment on herself to improve self-management of her PD [11]. To the best of our knowledge, no other patient-driven case reports have been published in PD.
Technology enables patients to be more active in the management of their health by making use of technology for self-tracking of symptoms and selfcare [12]. Self-tracking can also significantly improve clinical measures; in an RCT of 766 patients undergoing chemotherapy, overall survival for patients tracking 12 symptoms using a web-based platform was compared to care as usual. Patients tracking symptoms had a median overall survival of 31.2 months compared with 26.0 months for the group receiving usual care. The main reasons for the difference are early responsiveness by nurses on potentially adverse events, and increased tolerability to chemotherapy for the patients through the process of tracking [13].
Patient self-tracking is an under-researched area in PD. A review of sensors for patient self-tracking found that technology-based objective measures have been demonstrated to have clinical relevance for assessing motor aspects of PD as well as the potential to improve individual outcomes [14]. Efforts have also been made into discussing the potential of using technology to quantify well-being and HRQoL in PD [15]. The latter is a viewpoint article and also includes the patient perspective in the form of three persons with PD (PwP) sharing what they hope technology can contribute to their PD management. We have however not been able to find any studies on patients’ practical experiences of applying technological self-tracking to aspects of PD.
The primary objective of this study was to explore patient-initiated self-tracking in PD by investigating the daily variations of the effects of the first author’s PD medication. The secondary objective was to discuss this example in the context of precision medicine.
METHODS
SR displayed her first symptoms of PD in her early teens, around 1984, and was formally diagnosed with juvenile onset PD in 2003. Her engineering background and interest in technology has led to exploration of self-tracking for personal benefit. Her most affected side is the left and main symptoms are bradykinesia and rigidity. She does not have tremor and was on a stable dose of PD medication during the study period (see Table 1).
Table 1
Medication regimen for the study period (names given as name of active substance(-s) with Swedish brand names in brackets)
Medication timings | 6AM | 11:30AM | 3PM | 6:30PM | 9PM | 10:30PM |
Levodopa/Carbidopa, 100/25 mg (Madopark) |
|
|
|
|
| |
Ropinerol controlled release, 2 mg (Requip depot) | 2 | 1 | 1 | |||
Entacapone, 200 mg (Comtess) | 1 | 1 | 1 | |||
Rasagiline, 1 mg (Azilect) | 1 | |||||
Levothyroxine, 100 microg* (Levaxin) | 1 |
* Levothyroxine is taken as a consequence of a thyroidectomy in 2000.
Tapping tests are frequently used in neurological clinical examinations to assess different aspects of motor function. They have been shown to be a useful, reliable and valid tool for clinicians to assess symptoms of advanced PD [16]. At home touch screen assessments of finger tapping have been shown to correlate to conventional scales for evaluating PD [17]. Our study was inspired by a Swedish study [18] using a personal digital assistant (PDA) for capturing finger tapping during 20 seconds of alternate tapping, where the user taps with the same finger but alternates between two boxes, side by side, to tap in. In that study, patients were expected to perform the test in a home environment several times per day for a period of about one week. The system has demonstrated the ability to capture individual daily variability of PD [19].
At the time for data collection (March 2012), the subject/first author (SR) owned an iPhone 4 to use for capturing finger tapping data. Therefore a search was made of the Apple app store for a free iOS app that was able to capture alternating finger tapping for at least 20 seconds. The app ‘FastFingers’ was found to record the number of taps on the screen for 30 seconds from the first tap and present the total number on the screen. We could not find any apps designed for alternating tapping so we decided to use FastFingers with non-alternating tapping.
The intention was to conduct tapping tests frequently, at least 10 times per day with each hand during a study period of 5 days. During the study period, times for medication intakes were noted manually and finger tapping was captured using the iPhone app FastFingers. Data was collated and visualized using Microsoft Excel.
Ethical considerations are important in all research and although an ethical review board has not reviewed our study, ethical issues have been considered. The subject, who is also the first author, conceived the study. She was well aware of the potential risks and benefits and the usual power imbalance between researcher and research subject does not apply. This experiment was originally conducted without the intent to publish the results. However, we consider it ethical to share our experiences and lessons learned in order to further the field of patient-initiated self-tracking by publishing our findings in a scientific journal.
RESULTS
Data collection was initiated on 12 March 2012 and continued for four days. Finger tapping was conducted 13 times with each hand on the first day, 9 times on the second, 7 times on the third, and 3 times on the fourth. On the fifth day no data was collected. SR realized that to add the collection of data to your everyday life, even if it is self-imposed, takes time and effort and can be a challenge to combine with work, family life and other obligations.
Only data from the first two days were complete enough to analyze. Data from finger tapping tests performed on 12 March and 13 March are presented in Fig. 1. The graph was produced in Microsoft Excel and shows tapping test results (lines) and medication intakes (bars).
Fig.1
Lines show finger tapping results (right and left hand) from 12th (day 1) and 13th (day 2) of March 2012. Bars show medication intake times.
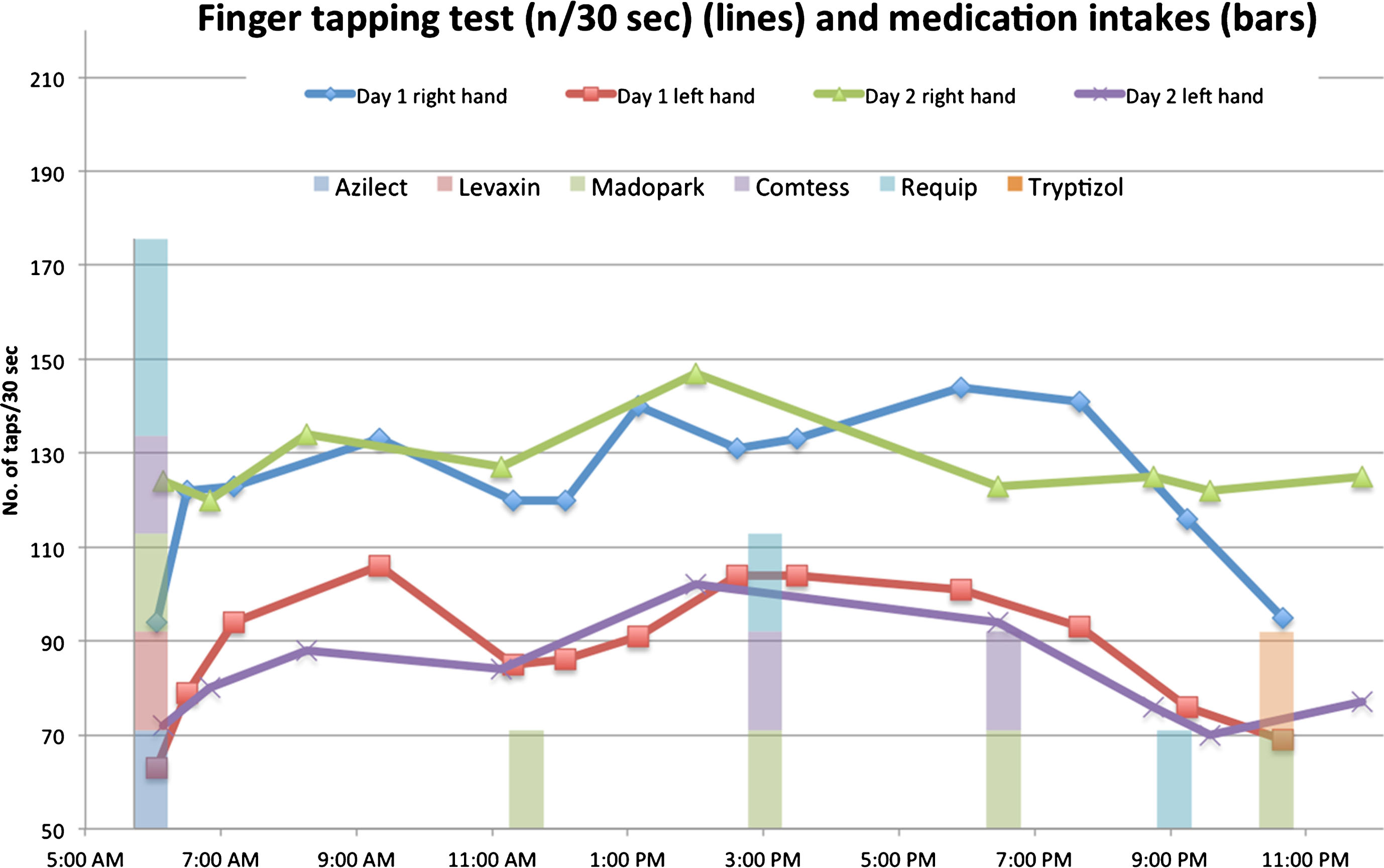
Reflecting on the results of the two days lead to the observation that there seemed to be a dip in finger function, especially on the more affected, left side, around the time for the second dose of the day (11:30 am), see Fig. 1. The results are 85 taps with the left hand in 30 seconds at 11:19AM on the first day, to be compared to the maximum value that day, which was 106 taps at 9:21AM.
Based on the results of the test, SR initiated a dialogue with her neurologist about adjusting the timing and/or dosage of medications to reduce the dip seen in Fig. 1.
DISCUSSION
The future of medicine lies in precision medicine and its potential for contributing to personalized treatments. The individualized manifestations and fluctuating nature of PD should make it particularly suitable for a precision medicine approach, including patients’ self-tracking efforts with the aim of enabling better understanding of individual variations.
The subject of our study realized with the help of a finger tapping test that her motor function was not the same throughout the day and specifically that she had a dip in function around lunchtime. The question arises if such a dip is clinically relevant. The study was patient-initiated and no clinicians were involved in collecting, analyzing, or interpreting the results. We do however consider it reasonable to assume that a difference in finger agility of 21 taps per 30 seconds is likely to have an effect on for example the subject’s typing speed, which is of relevance to the subject in question.
Another relevant question would be: Is two days of data enough to find patterns in disease and medication effect? Would not a longer period of data collection be able to provide more valuable insights for both patient and physician? Of course more data is always desirable and we cannot be certain that the patterns identified would be seen also on the subsequent days. From a patient perspective, identifying a problem or seeing an effect may often be considered enough, and weighing the added benefit of gathering more data against the burden of continuing the data collection may result in patients terminating data collection earlier than what would be the case in a conventional clinical study. The primary objective of this study was to explore patient-initiated self-tracking in PD and all findings will need further work to confirm or dismiss their importance.
There also seems to be other potential benefits to self-tracking. SR found, similar to what Pantzar and Ruckenstein [20] reported from their participants, that when she interacted with the collected data during analysis and visualization, she was able to reflect on what they meant in her specific context. The feedback loops that were created led to SR learning about both the specifics of her different medications as well as what the combination of them meant for her motor function over the course of the day. When data from self-tracking is shared in a clinical encounter, it has the double benefit of potentially contributing to the learning of both patient and clinician.
Since March 2012, when the data for this study were collected, there has been a lot of development in the area of smartphone aided self-tracking for PD. A few notable examples are the smartphone apps mPower and uMotif, and the SENSE-PARK system. The mPower app was launched as part of Apple Research Kit in March 2015, aiming towards a better understanding of the variations of PD and potential modulators as well as providing real-time feedback to the participants [21]. Publications from research using mPower show interesting results and great potential but as far as we can understand, real-time feedback has not yet been achieved [22–24]. The self-management app uMotif (available on iPhone and Android) was evaluated in a 16 week trial of 158 PwP where using the app was compared to treatment as usual. A small but statistically significant improvement was seen in self-reported medication adherence and PwP perceived increased quality of consultation (other outcome measures were QoL and symptom control). The SENSE-PARK system was developed in an EU project for home based evaluation of PD and consists of software, a smartphone app, a Wii balance board and a set of sensors. The feasibility and usability of the system tested positively in a 12 week study of 22 PwP [25]. The funding period of the project has ended and we have not been able to ascertain the current status of the system. Evaluations of the patient perspective seem to not have been the primary focus in these systems. The only example we have been able to find exploring the user perspective is a formative evaluation of the consent process of mPower [26], which is of little relevance to our study, since consent is given a limited number of times.
Limitations
Our study is not without limitations. The test we used does not give any information regarding the non-motor symptoms of PD. In the case of our user, SR, this may not be a major issue, since she does not experience a lot of non-motor symptoms. Complementary tests and/or devices can be added if other symptoms need to be investigated. Furthermore, we have only explored self-tracking for PwP in the context of medication effect. Other potential uses, for example tracking the effects of exercise or diet could be investigated in future studies.
There are also advantages and limitations associated with our chosen study design. A case study is able to describe an observed effect or phenomenon in one or a small number of subjects in a timely manner in order to offer the scientific community the opportunity to discuss and critique the findings. The results from SR’s self-tracking can however not be extrapolated beyond the subject in this study without further research. One can also question whether SR is representative for the larger population of people with PD. Not everyone will have the skills, knowledge and attitude to do this type of self-tracking, however we hypothesize that with improved tools and awareness of the benefits of self-tracking an increasing number of people with PD would be able to apply such approaches. Another important limitation for broader application of approaches of this kind is that they require patients with a certain level of autonomy that have the necessary abilities and skills to handle technology.
Conclusions
In conclusion, this study shows that a smartphone finger-tapping test enabled the subject in the study, also the first author, to glean important insights about how the effects of her PD medications varied over the course of the day. This suggests that symptom self-tracking may be useful to PwP. These variations in medication effect could not have been discovered easily in another way and observations like these can be used as a basis for medication adjustments in collaboration with a physician. If shared in a clinical encounter, it has the double benefit of potentially contributing to the learning of both patient and clinician. The patient perspective can also be valuable when developing and implementing new healthcare technologies.
Symptom tracking holds great promise in the field of precision medicine and more work is needed to develop this field. If done right, technology has the potential to improve the understanding of PD from the perspective of all stakeholders. A major challenge when it comes to addressing the needs and wishes of all stakeholders is that the views of clinicians and researchers regarding what is important in order to help PwP are often different from what PwP consider most important [27–29]. This means that extra focus has to be given, especially in the area of self-tracking, to ensuring that new technological applications are developed to address the issues that patients find important rather than the ones clinicians prioritize. Furthermore, the burden of tracking for the patient needs to be considered in relation to the potential benefit. This will be a key factor in ensuring long-term adoption and use of self-tracking as a sustainable part of precision medicine.
CONFLICT OF INTEREST
The authors have no conflict of interest to report.
ACKNOWLEDGMENTS
This work was financially supported by FORTE, the Swedish Research Council for Health, Working Life and Welfare [grant number 2014-4238] and Vinnova, the Swedish Governmental Agency for Innovation Systems.
REFERENCES
[1] | Hodson R ((2016) ) Outlooks: Precision medicine. Nature 537: , S49. |
[2] | Titova N , Chaudhuri KR ((2017) ) Personalized medicine in Parkinson’sdisease: Time to be precise. Mov Disord 32: , 1147–1154. |
[3] | Espay AJ , Brundin P , Lang AE ((2017) ) Precision medicine for diseaodification in Parkinson disease. Nat Publ Gr 13: , 119–126. |
[4] | Espay AJ , Bonato P , Nahab FB , Maetzler W , Dean JM , Klucken J , Eskofier BM , Merola A , Horak F , Lang AE , Reilmann R , Giuffrida J , Nieuwboer A , Horne M , Little MA , Litvan I , Simuni T , Dorsey ER , Burack MA , Kubota K , Kamondi A , Godinho C , Daneault J-F , Mitsi G , Krinke L , Hausdorff JM , Bloem BR , Papapetropoulos S ((2016) ) Technology in Parkinson’s disease: Challenges and opportunities. Mov Disord 31: , 1272–1282. |
[5] | Schork NJ ((2015) ) Personalized medicine: Time for one-person trials. Nature 520: , 609–611. |
[6] | Lillie EO , Patay B , Diamant J , Issell B , Topol EJ , Schork NJ ((2011) ) The N-of-1 clinical trial: The ultimate strategy for individualizing medicine? Per Med 8: , 1–19. |
[7] | Dresser R ((2014) ) Personal knowledge and study participation. J Med Ethics 40: , 471–474. |
[8] | Weisse AB ((2012) ) Self-experimentation and its role in medical research. Texas Hear Inst J 39: , 51–54. |
[9] | Marshall BJ , Armstrong JA , McGechie DB , Glancy RJ ((1985) ) Attempt tofulfil Koch’s postulates for pyloric campylobacter. Med J Aust 142: , 436–439. |
[10] | Vandenbroucke JP ((2001) ) In defense of case reports and case series. Ann Intern Med 134: , 330–334. |
[11] | Riggare S , Unruh KT , Sturr J , Domingos J ((2017) ) Patient-drivenN-of-1 in parkinson’s disease: Lessons learned from aplacebo-controlled study of the effect of nicotine on dyskinesia. Methods Inf Med 56: , 123–128. |
[12] | Sharon T ((2017) ) Self-tracking for health and the quantified self:Re-articulating autonomy, solidarity, and authenticity in an age of personalized healthcare. Philos Technol 30: , 93–121. |
[13] | Basch E , Deal AM , Dueck AC , Scher HI , Kris MG , Hudis C , Schrag D ((2017) ) Overall survival results of a randomized trial assessing patient-reported outcomes for symptom monitoring during routinecancer treatment. JAMA 318: , 197–198. |
[14] | Appelboom G , Camacho E , Abraham ME , Bruce SS , Dumont ELP , Zacharia BE , D’Amico R , Slomian J , Reginster JY , Bruyère O , Connolly ES ((2014) ) Smart wearable body sensors for patient self-assessment and monitoring. Arch Public Heal 72: , 1–9. |
[15] | van Uem J , Isaacs T , Lewin A , Bresolin E , Salkovic D , Espay AJ , Matthews H , Maetzler W ((2016) ) A viewpoint on wearabletechnology-enabled measurement of wellbeing and health-related quality of life in Parkinson’s disease. J Parkinsons Dis 6: , 279–287. |
[16] | Westin J ((2010) ) Decision support for treatment of patients with advanced Parkinson’s disease (Thesis). Uppsala University. |
[17] | Memedi M , Nyholm D , Johansson A , Palhagen S , Willows T , Widner H , Linder J , Westin J ((2015) ) Validity and responsiveness of at-hometouch screen assessments in advanced Parkinson’s disease. IEEEJ Biomed Health Inform 19: , 1829–1834. |
[18] | Westin J , Dougherty M , Nyholm D , Groth T ((2009) ) A home environment test battery for status assessment in patients with advanced Parkinson’s disease. Comput Methods Programs Biomed 98: , 27–35. |
[19] | Westin J , Schiavella M , Memedi M , Nyholm D , Dougherty M , Antonini A ((2012) ) Validation of a home environment test battery for supporting assessments in advanced Parkinson’s disease. Neurol Sci 33: , 831–838. |
[20] | Pantzar M , Ruckenstein M ((2017) ) Living the metrics: Self-trackingand situated objectivity. Digit Heal 3: , 1–10. |
[21] | Dorsey ER , Yvonne Chan YF , McConnell MV , Shaw SY , Trister AD , Friend SH ((2016) ) The use of smartphones for health research. Acad Med 92: , 157–160. |
[22] | Bot BM , Suver C , Neto EC , Kellen M , Klein A , Bare C , Doerr M , Pratap A , Wilbanks J , Dorsey ER , Friend SH , Trister AD ((2016) ) The mPowerstudy, Parkinson disease mobile data collected using ResearchKit. Sci Data 3: , 160011. |
[23] | Chaibub Neto E , Bot BM , Perumal T , Omberg L , Guinney J , Kellen M , Klein A , Friend SH , Trister AD ((2016) ) Personalized hypothesis tests for detecting medication response in Parkinson disease patientsusing iPhone sensor data. Pac Symp Biocomput 21: , 273–284. |
[24] | Trister AD , Dorsey ER , Friend SH ((2016) ) Smartphones as new tools inthe management and understanding of Parkinson’s disease. Parkinsons Dis 2: , 1–2. |
[25] | Ferreira JJ , Godinho C , Santos AT , Domingos J , Abreu D , Lobo R , Gonçalves N , Barra M , Larsen F , Fagerbakke Ø , Akeren I , Wangen H , Serrano JA , Weber P , Thoms A , Meckler S , Sollinger S , vanUem J , Hobert MA , Maier KS , Matthew H , Isaacs T , Duffen J , Graessner H , Maetzler W ((2015) ) Quantitative home-based assessment of Parkinson’s symptoms: The SENSE-PARK feasibility and usability study. BMC Neurol 15: , 89. |
[26] | Doerr M , Truong AM , Bot BM , Wilbanks J , Suver C , Mangravite LM ((2017) ) Formative evaluation of participant experience with mobile eConsent in the app-mediated Parkinson mPower Study: A mixed methodsstudy. JMIR Mhealth Uhealth 5: , e14. |
[27] | Nisenzon AN , Robinson ME , Bowers D , Banou E , Malaty I , Okun MS ((2011) ) Measurement of patient-centered outcomes in Parkinson’s disease: What do patients really want from their treatment?. Parkinson Relat Disord 17: , 89–94. |
[28] | Sjödahl Hammarlund C , Nilsson MH , Hagell P ((2012) ) Measuring outcomes in Parkinson’s disease: A multi-perspective concept mapping study. Qual Life Res 21: , 453–63. |
[29] | Mathur S , Mursaleen L , Stamford J , Dewitte S , Robledo I , Isaacs T ((2017) ) Challenges of improving patient-centred care in Parkinson’s disease. J Parkinsons Dis 7: , 163–174. |