Increased Diagnostic Yield by Reanalysis of Whole Exome Sequencing Data in Mitochondrial Disease
Abstract
Background:
The genetic diagnosis of mitochondrial disorders is complicated by its genetic and phenotypic complexity. Next generation sequencing techniques have much improved the diagnostic yield for these conditions. A cohort of individuals with multiple respiratory chain deficiencies, reported in the literature 10 years ago, had a diagnostic rate of 60% by whole exome sequencing (WES) but 40% remained undiagnosed.
Objective:
We aimed to identify a genetic diagnosis by reanalysis of the WES data for the undiagnosed arm of this 10-year-old cohort of patients with suspected mitochondrial disorders.
Methods:
The WES data was transferred and processed by the RD-Connect Genome-Phenome Analysis Platform (GPAP) using their standardized pipeline. Variant prioritisation was carried out on the RD-Connect GPAP.
Results:
Singleton WES data from 14 individuals was reanalysed. We identified a possible or likely genetic diagnosis in 8 patients (8/14, 57%). The variants identified were in a combination of mitochondrial DNA (n = 1, MT-TN), nuclear encoded mitochondrial genes (n = 2, PDHA1, and SUCLA2) and nuclear genes associated with nonmitochondrial disorders (n = 5, PNPLA2, CDC40, NBAS and SLC7A7). Variants in both the NBAS and CDC40 genes were established as disease causing after the original cohort was published. We increased the diagnostic yield for the original cohort by 15% without generating any further genomic data.
Conclusions:
In the era of multiomics we highlight that reanalysis of existing WES data is a valid tool for generating additional diagnosis in patients with suspected mitochondrial disease, particularly when more time has passed to allow for new bioinformatic pipelines to emerge, for the development of new tools in variant interpretation aiding in reclassification of variants and the expansion of scientific knowledge on additional genes.
INTRODUCTION
Mitochondrial disorders are highly heterogeneous and complex both in their phenotype and genotype [1]. They are individually rare but collectively affect 1 in 5000 individuals. Mitochondria are well known for their role in oxidative phosphorylation and ATP synthesis and both mitochondrial DNA (mtDNA) and nuclear DNA (nDNA) encoded genes contribute to this key mitochondrial function. Mitochondrial disorders can present with a broad range of symptoms affecting virtually every organ system. This complexity in genotype and phenotype is reflected in the difficulties and burden reported by patients, that often see multiple doctors over many years before a diagnosis is established [2]. It is also reflected in the difficulty in establishing a genetic diagnosis in patients with a suspected mitochondrial disorder.
Traditionally, mitochondrial disorders have been diagnosed primarily by a combination of clinical features and histochemical or biochemical analysis of an affected tissue, followed by targeted molecular genetic testing [3]. With Next Generation Sequencing (NGS), exome and genome sequencing became cost-effective, and the diagnosis of rare diseases, including mitochondrial disorders, was transformed. In recent years these technologies have been used exponentially in the diagnosis of mitochondrial diseases. In cohorts of patients with suspected mitochondrial disorders whole exome sequencing (WES) establishes a diagnosis in 40–60% of the patients [4–7]. Crucial to a high yield in genetic diagnoses is a well-established phenotype.
Several methods have been proposed to increase the diagnostic yield established by WES for mitochondrial disorders, including whole genome sequencing, suggested as the ideal first line genomic investigation [8], long read sequencing and Deep-WES as well as using other ‘omics’ such as RNA sequencing, epigenomics and metabolomics [9]. However, not identifying a causative variant from WES does not necessarily mean the disease-causing variant lies outside the data already produced and reanalysis of WES is a valuable approach to further increase diagnostic yield [10].
Here we proposed to reanalyse the WES data of a well phenotyped cohort of patients with a suspected mitochondrial disease, with combined respiratory chain deficiency, initially reported 10 years ago [4], using the improved WES diagnostic pipeline provided by the RD-Connect Genome-Phenome Analysis Platform (GPAP).
The RD-Connect GPAP (https://platform.rd-connect.eu) is a user-friendly resource of genomic and phenotypic data of patients with rare disease and family members, which facilitates diagnosis and gene discovery, and has been used as the primary analysis tool in a number of large European projects [11].
MATERIALS AND METHODS
Original cohort
Taylor and colleagues [4] compiled a cohort of 53 patients with suspected mitochondrial disease and histochemical and/or biochemical evidence of decreased activities of multiple respiratory chain complexes in a clinically affected tissue based on published criteria [12]. These patients were previously tested for and did not have large scale mtDNA rearrangements, mtDNA depletion, and mtDNA point mutations (with the exception of some patients, including patient 5 in our cohort, in whom decreased levels of mtDNA was confirmed in muscle).
In the original Taylor et al cohort the variants were called by an in-house pipeline and classified into 4 groups: 1) presumptive pathogenic –homozygous or compound heterozygous mutations in genes previously shown to cause multiple respiratory chain deficiencies; 2) possible pathogenic –homozygous or compound heterozygous mutations in novel genes predicted to case a mitochondrial translation defect based on their proposed function and similarity to known disease genes; 3) variants of unknown significance –homozygous or compound heterozygous mutations in novel or known disease genes not known to be associated with mitochondrial pathology and; 4) unresolved cases in which a single plausible genetic cause could not be identified [4]. They established a probable or possible diagnosis in 32 of 53 patients (60%). Despite the high diagnostic yield, an additional 21 patients did not have a diagnosis established following careful biochemical phenotyping and whole exome analysis.
Our cohort
We collated the 21 patients with no established diagnosis in the original cohort including patients with variants of uncertain significance. For 5 of the 21 patients the consent forms did not allow the upload of data to the RD-Connect GPAP platform. Two of the 21 patients were since found to have disease causing variants, one in MIEF2 [13] and another one in TANGO2 [14]. Fourteen of the 21 patients had, to our knowledge, no genetic cause identified for their condition (Fig. 1).
Fig.1
Patient cohort and number of diagnosed and undiagnosed patients
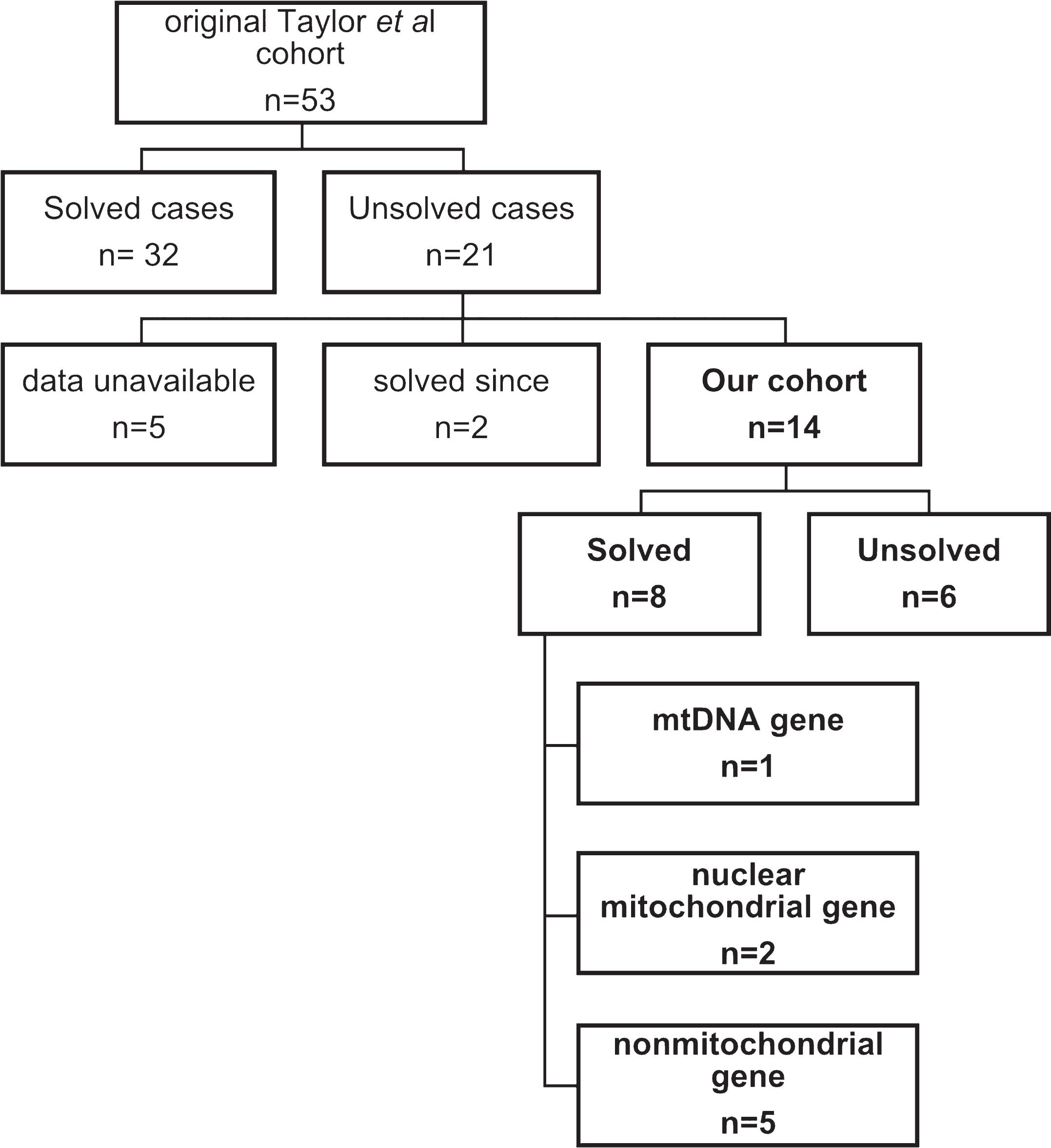
RD-Connect GPAP
The RD-Connect GPAP is a user-friendly online resource which facilitates the collation, analysis, interpretation and sharing of integrated genome-phenome datasets. Phenotypic and raw WES data from our cohort was submitted to RD-connect GPAP [11].
Whole exome sequencing and data analysis
Exome sequencing was performed on whole blood tissue samples in Newcastle upon Tyne as previously described [4]. The raw WES data, excluding the 5 cases without the appropriate consent forms, was since transferred and processed by the RD-connect GPAP using their standardized pipeline (as described in Laurie et al. [11]).
Variant prioritisation was carried out on the RD-Connect GPAP. Likely pathogenic variants were identified by applying standard filtering criteria including moderate to high variant effect predictor (VEP) score (i.e. nonsense, splice site, frame-shift, in-frame and non-synonymous variants), gnomAD allele frequency of <0.01 and a GPAP internal frequency <0.02. WES data was sequentially analysed on GPAP using available gene lists on the platform (such as Mitocarta 2.0, n = 1158; European Reference Network on Neurological Diseases (ERN-RDN) n = 1820 and on Neuromuscular Diseases (ERN-NMD), Muscle Gene Table May 2021, n = 611, Medically Interpretable Genome, n = 5419, Genomics England Mitochondrial disorders panel v.4.157, n = 487; and Genomics England Inborn Errors of Metabolism panel v.4.129, n = 934). If no candidate variant was identified, we created patient specific phenotype-based gene lists using Human Phenotype Ontology (HPO) as embedded on GPAP and also searched for variants across the whole exome dataset.
Candidate variants were selected based on their predicted in silico deleteriousness, previous known association with human disease and phenotype fit (evaluated using OMIM and reviewing the literature in PubMed). Possible causative variants were individually classified according to the American College of Medical Genetics (ACMG) standards and guidelines for interpretation of variants [15] and ACGS Best Practice Guidelines for Variant Classification in Rare Disease [16]. For mtDNA variants the ACMG/AMP standards and guidelines for mitochondrial DNA variant interpretation [17] was used.
Due to the historical nature of the data set we were not able to do segregation studies or confirm phase for the presumed compound heterozygous variants.
RESULTS
Our cohort
The demographics and phenotype of the 14 patients included in our cohort is described in Table 1. Of note all patients in our cohort were children or young adults (<18 years of age) at the time of recruitment. Five were female (36%) and nine were male (64%).
Table 1
Patient cohort: phenotype and demographics
Gender | Age at onset | Consanguinity | Affected organs | Histochemistry | Deficient complexes | Other | |
P1 | F | 2 yr | yes | Muscle, CNS | Normal | cI, cIV | – |
P2 | M | 17 yr | no | CNS | COX deficiency | cI, cIV | Optic atrophy, axonal neuropathy |
P3 | F | 7 yr | yes | Muscle, CNS | RRF, COX mosaic | – | Multiple mitochondrial deletions, PEO, raised CK |
P4 | M | 4 yr | Yes | Muscle, CNS, Liver | COX mosaic | cI, cIV | Lactic acidosis, growth retardation |
P5 | M | 2 wk | Yes | Muscle | COX mosaic | cI, cIV | Multiorgan failure and lactic acidosis |
P6 | M | Birth | No | Muscle, CNS, Heart | COX mosaic | cI, cIV | Raised CK |
P7 | M | 6 yr | No | Muscle | COX mosaic, RRF and lipid | cI, cIII and cIV | Raised CK |
P8 | M | 18 mo | unknown | Muscle, Liver | COX normal, SDH positive | cI, cIII | – |
P9 | F | 1 mo | No | Muscle, CNS, Liver | COX mosaic | cI, cIV | mtDNA depletion, immune defect, deafness, renal failure |
P10 | M | 6 yr | No | Muscle | RRF, COX mosaic | cI, cIV | PEO, lactic acidosis, raised CK |
P11 | M | 2 mo | Yes | Muscle, CNS, Liver | COX mosaic | cI, cIV | Alpers’ phenotype |
P12 | F | 1 yr | No | Muscle, CNS | COX normal, lipid storage | cI, cIII | Lactic acidosis |
P13 | M | 2 yr | No | Muscle, CNS, Liver | COX normal, RRF | cIII, cIV | Lactic acidosis |
P14 | F | Birth | No | Muscle, CNS | Normal | cI, cIV | Lactic acidosis |
F: female, M: male, Yr: years; wk: weeks; mo: months; CNS: central nervous system; RRF: raggered red fibres; cI: complex I; cII: complex II; cIII: complex III; cVI: complex VI; PEO: progressive external ophthalmoplegia; CK: creatinine kinase.
Genetic results
Singleton whole exome sequencing (WES) data from the 14 individuals with suspected mitochondrial disease and multiple respiratory chain defects was reanalysed using the improved WES diagnostic pipeline provided by the RD-Connect GPAP.
Our reanalysis identified a possible or likely genetic diagnosis in a further 8 patients (8/14, 57%). The variants identified were a combination of mitochondrial DNA point mutations (n = 1, MT-TN), variants in nuclear encoded mitochondrial genes (n = 2, PDHA1, and SUCLA2) and variants in nuclear genes associated with other inborn errors of metabolism (n = 5, PNPLA2, CDC40, NBAS and SLC7A7). The details of each variant as well as their ACMG classification is described in Table 2. With the exception of MT-TN that follows mitochondrial inheritance and PDHA1 which is X-linked, all show autosomal recessive inheritance (Table 3). In 6 patients, a possible or probable genetic diagnosis could not be established, including one patient who subsequently had trio whole genome sequencing and this data added to and analysed by our team on the RD-connect GPAP.
Table 2
Likely or possible genetic diagnoses identified in our cohort
Gene | Genotype | Variant | ACMG classification | ACMG criteria | Variant previously reported | |
P3 | MT-TN | Heteroplasmic (49%) | m.5698 G > A | VUS | PS3 supPM2 mod | Spinazzola et al., 2004 |
P4 | SLC7A7 | Homozygous | NM_003982.4:c.1A>Cp.Met1? | LP | PVS1 modPS1 modPS3 supPM2 mod | Sperandeo et al., 2008[30] |
P7 | PNPLA2 | Presumedcompound heterozygous | NM_020376.4:c.24 G > Ap.Trp8Ter | P | PVS1 very strongPM2 mod | Pennisi et al., 2017[31] |
NM_020376.4:c.798dup p.Ala267ArgfsTer40 | P | PVS1 very strongM2 modPM3 sup | Jousserand et al., 2016[32] | |||
P8 | NBAS | Presumedcompound heterozygous | NM_015909.4:c.2330C > Ap.Pro777His | VUS | PM2 modPP3 sup | Haack et al., 2015 [18] |
NM_015909.4:c.1187G > Ap.Trp396Ter | P | PVS1 strongPM2 mod | Haack et al., 2015 [18] | |||
P11 | SUCLA2 | Homozygous | NM_003850.3:c.370T > C p.Ser124Pro | VUS | PM2 modPP3 mod | Issa et al., 2020[33] |
P12 | NBAS | Presumedcompound heterozygous | NM_015909.4:c.2330 C > Ap.Pro777His | VUS | PM2 modPP3 sup | Haack et al., 2015 [18] |
NM_015909.4:c.1187 G > Ap.Trp396Ter | P | PVS1 strongPM2 mod | Haack et al., 2015 [18] | |||
P13 | PDHA1 | Hemizygous | NM_000284.4:c.905 G > Ap.Arg302His | P | PM1 modPM2 modPM5 modPP3 strong | Otero et al., 1998[34] |
P14 | CDC40 | Homozygous | NM_015891.3:c.1505T > G p.Phe502Cys | LP | PS3 modPM2 modPM3 supPP2 strong | Chai et al., 2021[35] |
P: pathogenic; LP: likely pathogenic; VUS: variant of uncertain significance.
Table 3
Causative genes in our cohort and associated phenotypes
Genes | OMIM # | Associated OMIM Phenotype |
Mitochondrial disease | ||
MT-TN | * 590010 | Mitochondrial Complex I deficiency; Isolated opthalmoplegia |
SUCLA2 | * 603921 | AR Mitochondrial DNA depletion syndrome 5 |
PDHA1 | * 300502 | XLD Pyruvate dehydrogenase E1-alpha deficiency |
Other inborn errors of metabolism | ||
PNPLA2 | * 609059 | AR Neutral lipid storage disease with myopathy |
NBAS | * 608025 | AR Infantile liver failure syndrome 2AR Short stature, optic nerve atrophy, and Pelger-Huet anomaly |
SLC7A7 | * 603593 | AR Lysinuric protein intolerance |
CDC40 | * 605585 | AR ?Pontocerebellar hypoplasia, type 15 |
Curiously, both patients with NBAS variants were heterozygous for the same variants despite them not being known to be related. The disease phenotype on both patients fitted the condition well. We were not able to confirm that they were compound heterozygous but there is a patient described in the literature who is reported to be compound heterozygous for the same variants [18].
Whole exome sequencing is not traditionally used to identify mitochondrial DNA variants despite mtDNA reads being generated. This is because standard pipelines do not report mtDNA variants. However, a number of studies have shown WES to be a valid tool for mtDNA analysis when the appropriate pipeline is used [19, 20]. The RD-Connect GPAP pipeline does allows, with some limitations, for the calling of mtDNA variants [10, 21]. As such, we were able to identify a variant in the mt-tRNAAsn (MT-TN). This was classified by us as a variant of uncertain significance but was previously reported to be disease causing, with a phenotype similar to that of our patient, and so it remains a possible diagnosis.
A limitation of our study is that, because of the historic nature of the cohort, we were not able to confirm the variants using alternative methods, and we were not able to do segregation studies and confirm phase. Due to constraints of the GPAP and of WES we were not able to systematically look for structural variants or copy number variants.
DISCUSSION
Reanalysis of exomes from 14 individuals with a suspected mitochondrial disorder, based on clinical, as well as histochemical and/or biochemical phenotype, detected a possible or likely diagnosis in 8 (57%). This included variants in mitochondrial DNA (n = 1, 12.5%), variants in nuclear encoded mitochondrial genes (n = 2, 25%) as well as variants in genes that cause other inborn errors of metabolism (n = 5, 62.5%). This highlights that systematic reanalysis of existing WES data is an important route for increasing the diagnostic yield in cohorts of patients with suspected mitochondrial disorders. WES reanalysis has been a valid method to increase diagnostic yield in a number of studies [10,22], and although there are challenges associated with data reanalysis (including time, manpower and cost), it should be considered before generating further genomic and other ‘omics’ data [10].
Additionally, taking an agnostic approach, rather than a gene panel approach, to the WES (and WGS) data analysis increases diagnostic yield in mitochondrial disorders [4, 8]. This is partly because of the heterogenicity of mitochondrial disorders themselves and the increasing role of ‘phenocopies’ in the diagnosis of patients with suspected mitochondrial disorders. Defects in mitochondrial respiratory chain complexes are typically an attribute of mitochondrial diseases and combined respiratory chain defects are common finding in disorders of mitochondrial energy metabolism [23]. None the less, in line with other published literature [24], 5 of our patients with combined respiratory chain defects had likely disease-causing variants in nonmitochondrial genes. Similarly, in a cohort study of patients with suspected mitochondrial disorders who underwent WGS, 63% of newly diagnosed patients had a nonmitochondrial diagnosis [8]. In line with this finding, in our cohort only 3 (38%) of the patients with a likely diagnosis found had a mitochondrial disease and in 5 cases (63%) we identified a nonmitochondrial diagnosis (PNPLA2, CDC40, NBAS and SLC7A7 genes). Pathogenic variants in these genes all cause metabolic disorders with some clinical features that overlap with mitochondrial disease, and it is likely that biochemical evidence of respiratory chain deficiency is a secondary cellular process in other disorders [24]. Our study further highlights the advantages of ‘untargeted’ analysis.
Reanalysis of our cohort has lifted the diagnostic yield of the original cohort by 15%. Of note this cohort was extremely well characterized from a biochemical, histological, and clinical point of view and therefore the diagnostic yield is high both in the original paper (60%) [4] and our reanalysis (15%). In a more heterogeneous cohort of patients with suspected mitochondrial disorders the diagnostic yield following WGS was 31% [8]. Additionally, all individuals in our cohort (14/14) where children or young adults at the time of recruitment and or disease onset and it was previously noted that the diagnostic yield of children with suspected mitochondrial disorder by WGS was higher than that of adults [8].
Other important factors that are likely to have contributed to the relatively high diagnostic yield are: i) improved WES diagnostic pipeline provided by the RD-Connect GPAP [11]; ii) a number of variant interpretation resources have become available since the original cohort was published in 2014 including the ACMG guidelines for variant interpretation [15] as well as a number of additional tools for variant classification such as improved computational algorithms for predicting pathogenicity; iii) novel genes, with variants in two of the genes likely to be the disease causing in our cohort (NBAS and CDC40) being established as disease causing after the original cohort was published; iv) agnostic approach with manual curation of candidate variants using disease databases such as OMIM, and literature search.
The variants in MT-TN, PDHA1 and SLC7A7 in our cohort, as well as variants in SUCLA2 and PNPLA2 different to the ones identified in our cohort, were reported in the literature prior to the original Taylor et al cohort was published [4] but these were not identified in the paper as possible disease-causing variants. The MT-TN m.5698G > A variant is classified by us as a variant of uncertain significance and therefore, even if the variant was identified, the team may have wished to seek an alternate diagnosis by WES. With the regards to the PDHA1, SLC7A7, SUCLA2 and PNPLA2 variants, although we cannot be sure as we have no access to the original Taylor et al. pipeline and the raw data, it is possible that the variants were not called by the original pipeline and therefore not reported. In our cohort we did not identify any novel genes.
Despite careful WES data reanalysis and detailed phenotyping, in 6 of the 14 patients a diagnosis or likely diagnosis was not established. This included a patient who subsequently had trio WGS with a diagnosis or possible diagnosis still not identified. WES has limitations in the identification of structural variants, copy number variants, rare intronic variants and mitochondrial variants and it may also be that the causative genes have not yet been ‘discovered’.
Establishing a diagnosis is important for patients and their families and can potentially change clinical management [2]. Reanalysis of WES data has consistently shown to increase diagnostic yield in cohorts of undiagnosed patients with rare disease and periodic reanalysis of genomic data is important particularly when enough time has lapsed when technology is likely to have evolved and new genes possibly discovered [25–27]. With novel mitochondrial genes being discovered at a rate of about 20 per year since the advent of NGS [36], we suggest reanalysis every 2 years as suggested by the ACMG [25] could be an appropriate timeline. In our cohort all but one patient could have had a diagnosis after 2 years, if the GPAP pipeline was used. To overcome some of the issues associated with reanalysis, including cost, expertise and time required, some automation and machine learning algorithms may have an important role [10, 28]. Additional to WES and WGS, which further increases the diagnostic yield in patients with suspected mitochondrial disorders [8], new omic technologies such as long read sequencing, transcriptomics, proteomics and metabolomics are likely to be needed to improve diagnostic yield in similar cohorts. Deep phenotyping will continue to be key in all the additional techniques and for automation of analysis.
ACKNOWLEDGMENTS
The authors have no acknowledgments to report.
FUNDING
C.O. is supported by the Evelyn Trust (G117372). R.H. is supported by the Wellcome Trust (G118015), Muscular Dystrophy UK, the Medical Research Council (UK) (MR/V009346/1), the Addenbrookes Charitable Trust (G100142), the Evelyn Trust, the Stoneygate Trust, the Lily Foundation, Action for AT and an MRC strategic award to establish an International Centre for Genomic Medicine in Neuromuscular Diseases (ICGNMD) (MR/S005021/1). This research was supported by the NIHR Cambridge Biomedical Research Centre (BRC-1215-20014). The views expressed are those of the authors and not necessarily those of the NIHR or the Department of Health and Social Care.
CONFLICT OF INTEREST
R.H, H.L, P.F.C, J.K and U.S-S are Editorial Board Members of this journal, but were not involved in the peer-review process nor had access to any information regarding its peer-review.
DATASETS/DATA AVAILABILITY STATEMENT
Genetic and phenotypic data of the study participants has been uploaded to RD-Connect GPAP. The data supporting the findings of this study are available within the article and/or its supplementary material.
REFERENCES
[1] | Gorman GS , Chinnery PF , DiMauro S , Hirano M , Koga Y , McFarland R , et al. Mitochondrial diseases. Nat Rev Dis Primers. (2016) ;2: :16080. doi: 10.1038/nrd2016.80. |
[2] | Thompson JLP , Karaa A , Pham H , Yeske P , Krischer J , Xiao Y , et al. The evolution of the mitochondrial disease diagnostic odyssey [published correction appears in Orphanet J Rare Dis. 2023;18(1):194]. Orphanet J Rare Dis. (2023) ;18: (1):157. doi: 10.1186/s13023-023-02754-x. |
[3] | McFarland R , Taylor RW , Turnbull DM . A neurological perspective on mitochondrial disease. Lancet Neurol. (2010) ;9: (8):829–40. doi: 10.1016/S1474-4422(10)70116-2. |
[4] | Taylor RW , Pyle A , Griffin H , et al. Use of whole-exome sequencing to determine the genetic basis of multiple mitochondrial respiratory chain complex deficiencies. JAMA. (2014) ;312: (1):68–77. doi: 10.1001/jama.2014.7184. |
[5] | Puusepp S , Reinson K , Pajusalu S , et al. Effectiveness of whole exome sequencing in unsolved patients with a clinical suspicion of a mitochondrial disorder in Estonia. Mol Genet Metab Rep. (2018) ;15: :80–9. doi :10.1016/j.ymgmr.2018.03.004. |
[6] | Wortmann SB , Mayr JA , Nuoffer JM , Prokisch H , Sperl W . A Guideline for the Diagnosis of Pediatric Mitochondrial Disease: The Value of Muscle and Skin Biopsies in the Genetics Era. Neuropediatrics. (2017) ;48: (4):309–14. doi: 10.1055/s-0037-1603776. |
[7] | Theunissen TEJ , Nguyen M , Kamps R , Hendrickx AT , Sallevelt SCEH , Gottschalk RWH , et al. Whole Exome Sequencing Is the Preferred Strategy to Identify the Genetic Defect in Patients With a Probable or Possible Mitochondrial Cause. Front Genet. (2018) ;9: :400. doi: 10.3389/fgene.2018.00400. |
[8] | Schon KR , Horvath R , Wei W , Calabrese C , Tucci A , Ibañez K , et al. Use of whole genome sequencing to determine genetic basis of suspected mitochondrial disorders: Cohort study. BMJ. (2021) ;375: :e066288 doi: 10.1136/bmj-2021-066288. |
[9] | Zurek B , Ellwanger K , Vissers LELM , Schüle R , Synofzik M , Töpf A et al. Solve-RD: Systematic pan-European data sharing and collaborative analysis to solve rare diseases [published correction appears in Eur J Hum Genet. 2021 Aug 13]. Eur J Hum Genet. (2021) ;29: (9):1325–31. doi: 10.1038/s41431-021-00859-0. |
[10] | Matalonga L , Hernández-Ferrer C , Piscia D ;Solve-RD SNV-indel working group;, Schüle R , Synofzik M , et al. Solving patients with rare diseases through programmatic reanalysis of genome-phenome data [published correction appears in Eur J Hum Genet. 2021 Aug 16;:]. Eur J Hum Genet. (2021) ;29: (9):1337–47. doi: 10.1038/s41431-021-00852-7. |
[11] | Laurie S , Piscia D , Matalonga L , Corvó A , Fernández-Callejo M , Garcia-Linares C , et al. The RD-Connect Genome-Phenome Analysis Platform: Accelerating diagnosis, research, and gene discovery for rare diseases. Hum Mutat. (2022) ;43: (6):717–33. doi: 10.1002/humu.24353. |
[12] | Kirby DM , Thorburn DR , Turnbull DM , Taylor RW . Biochemical assays of respiratory chain complex activity. Methods Cell Biol. (2007) ;80: :93–119. doi: 10.1016/S0091-679X(06)80004-X. |
[13] | Bartsakoulia M , Pyle A , Troncoso-Chandía D , Vial-Brizzi J , Paz-Fiblas MV , Duff J , et al. A novel mechanism causing imbalance of mitochondrial fusion and fission in human myopathies. Hum Mol Genet. (2018) ;27: (7):1186–95. doi: 10.1093/hmg/ddy033. |
[14] | Mingirulli N , Pyle A , Hathazi D , Alston CL , Kohlschmidt N , O’Grady G , et al. Clinical presentation and proteomic signature of patients with TANGO2 mutations. J Inherit Metab Dis. (2020) ;43: (2):297–308. doi: 10.1002/jimd.12156. |
[15] | Richards S , Aziz N , Bale S , Bick D , Das S , Gastier-Foster J , et al. Standards and guidelines for the interpretation of sequence variants: A joint consensus recommendation of the American College of Medical Genetics and Genomics and the Association for Molecular Pathology. Genet Med. (2015) ;17: (5):405–24. doi: 10.1038/gim.2015.30. |
[16] | Ellard S , Baple EL , Berry I , Forrester N , Turnbull C , Owens M , et al. ACGS best practice guidelines for variant classification in rare disease 2020: Association for Clinical Genomic Science (ACGS), 2020. [cited 2024 Jan 22] Available from: https://www.acgs.uk.com/quality/bestpractice-guidelines/#VariantGuidelines |
[17] | McCormick EM , Lott MT , Dulik MC , Shen L , Attimonelli M , Vitale O , et al. Specifications of the ACMG/AMP standards and guidelines for mitochondrial DNA variant interpretation. Hum Mutat. (2020) ;41: (12):2028–57. doi: 10.1002/humu.24107. |
[18] | Haack TB , Staufner C , Köpke MG , Straub BK , Kölker S , Thiel C , et al. Biallelic Mutations in NBAS Cause Recurrent Acute Liver Failure with Onset in Infancy. Am J Hum Genet. (2015) ;97: (1):163–9. doi: 10.1016/j.ajhg.2015.05.009. |
[19] | Garret P , Bris C , Procaccio V , Amati-Bonneau P , Vabres P , Houcinat N , et al. Deciphering exome sequencing data: Bringing mitochondrial DNA variants to light. Hum Mutat. (2019) ;40: (12):2430–43. doi: 10.1002/humu.23885. |
[20] | Poole OV , Pizzamiglio C , Murphy D , Falabella M , Macken WL , Bugiardini E , et al. Mitochondrial DNA Analysis from Exome Sequencing Data Improves Diagnostic Yield in Neurological Diseases. Ann Neurol. (2021) ;89: (6):1240–7. doi: 10.1002/ana.26063. |
[21] | de Boer E , Ockeloen CW , Matalonga L , Horvath R ; Solve-RD SNV-indel working group; Rodenburg RJ , et al. A MT-TL1 variant identified by whole exome sequencing in an individual with intellectual disability, epilepsy, and spastic tetraparesis [published correction appears in Eur J Hum Genet .2021 Jul 15;:]. Eur J Hum Genet. (2021) ;29: (9):1359–68. doi: 10.1038/s41431-021-00900-2. |
[22] | Töpf A , Pyle A , Griffin H , Matalonga L , Schon K; Solve-RD SNV-indel working group; et al.Exome reanalysis and proteomic profiling identified TRIP4 as a novel cause of cerebellar hypoplasia and spinal muscular atrophy (PCH1). Eur J Hum Genet. (2021) ;29: (9):1348–53. doi: 10.1038/s41431-021-00851-8. |
[23] | Mayr JA , Haack TB , Freisinger P , Karall D , Makowski C , Koch J , et al. Spectrum of combined respiratory chain defects. J Inherit Metab Dis. (2015) ;38: (4):629–40. doi: 10.1007/s10545-015-9831-y. |
[24] | Pyle A , Nightingale HJ , Griffin H , Abicht A , Kirschner J , Baric I , et al. Respiratory chain deficiency in nonmitochondrial disease. Neurol Genet. (2015) ;1: (1):e6. Published 2015 Apr 27. doi: 10.1212/NXG.0000000000000006 |
[25] | Deignan JL , Chung WK , Kearney HM , Monaghan KG , Rehder CW , Chao EC , et al. Points to consider in the reevaluation and reanalysis of genomic test results: A statement of the American College of Medical Genetics and Genomics (ACMG). Genet Med. (2019) ;21: (6):1267–70. doi: 10.1038/s41436-019-0478-1. |
[26] | Sanchis-Juan A , Megy K , Stephens J , Armirola Ricaurte C , Dewhurst E , Low K , et al. Genome sequencing and comprehensive rare-variant analysis of 465 families with neurodevelopmental disorders. Am J Hum Genet. (2023) ;110: (8):1343–55. doi: 10.1016/j.ajhg.2023.07.007. |
[27] | Wright CF , Campbell P , Eberhardt RY , Aitken S , Perrett D , Brent S , et al. Genomic Diagnosis of Rare Pediatric Disease in the United Kingdom and Ireland. N Engl J Med. (2023) ;388: (17):1559–71. doi: 10.1056/NEJMoa2209046. |
[28] | O’Brien TD , Campbell NE , Potter AB , Letaw JH , Kulkarni A , Richards CS . Artificial intelligence (AI)-assisted exome reanalysis greatly aids in the identification of new positive cases and reduces analysis time in a clinical diagnostic laboratory. Genet Med. (2022) ;24: (1):192–200. doi: 10.1016/j.gim.2021.09.007. |
[29] | Spinazzola A , Carrara F , Mora M , Zeviani M . Mitochondrial myopathy and ophthalmoplegia in a sporadic patient with the 5698G–>A mitochondrial DNA mutation. Neuromuscul Disord. (2004) ;14: (12):815–7. doi: 10.1016/j.nmd.2004.09.002. |
[30] | Sperandeo MP , Andria G , Sebastio G . Lysinuric protein intolerance: Update and extended mutation analysis of the SLC7A7 gene. Hum Mutat. (2008) ;29: (1):14–21. doi: 10.1002/humu.20589. |
[31] | Pennisi EM , Arca M , Bertini E , Bruno C , Cassandrini D , D’amico A , et al. Neutral Lipid Storage Diseases: Clinical/genetic features and natural history in a large cohort of Italian patients. Orphanet J Rare Dis. (2017) ;12: (1):90.Published May 12. doi: 10.1186/s13023-017-0646-9. |
[32] | Jousserand G , Streichenberger N , Petiot P . Myopathie à surcharge lipidique secondaire à une nouvelle mutation du gène PNPLA2 [Unusual phenotype of myopathy associated with a new PNPLA2 mutation]. Med Sci (Paris). (2016) ;32 Hors série n°2: :10–11. doi: 10.1051/medsci/201632s203. |
[33] | Issa MY , Chechlacz Z , Stanley V , George RD , McEvoy-Venneri J , Belandres D , et al. Molecular diagnosis in recessive pediatric neurogenetic disease can help reduce disease recurrence in families. BMC Med Genomics. (2020) ;13: (1):68.68. Published May 13. doi: 10.1186/s12920-020-0714-1. |
[34] | Otero LJ , Brown RM , Brown GK . Arginine 302 mutations in the pyruvate dehydrogenase E1alpha subunit gene: Identification of further patients and in vitro demonstration of pathogenicity. Hum Mutat. (1998) ;12: (2):114–21. doi: 10.1002/(SICI)1098-1004(1998)12:2<114::AID-HUMU6>3.0.CO;2-#. |
[35] | Chai G , Webb A , Li C , Antaki D , Lee S , Breuss MW , et al. Mutations in Spliceosomal Genes PPIL1 and PRP17 Cause Neurodegenerative Pontocerebellar Hypoplasia with Microcephaly. Neuron. (2021) ;109: (2):241–56.e9. doi: 10.1016/j.neuron.2020.10.035. |
[36] | Frazier AE , Thorburn DR , Compton AG . Mitochondrial energy generation disorders: Genes, mechanisms, and clues to pathology. J Biol Chem. (2019) ;294: (14):5386–95. doi: 10.1074/jbc.R117.809194. |