Smartphone-Based Assessment of Mobility and Manual Dexterity in Adult People with Spinal Muscular Atrophy
Abstract
Background:
More responsive, reliable, and clinically valid endpoints of disability are essential to reduce size, duration, and burden of clinical trials in adult persons with spinal muscular atrophy (aPwSMA).
Objective:
The aim is to investigate the feasibility of smartphone-based assessments in aPwSMA and provide evidence on the reliability and construct validity of sensor-derived measures (SDMs) of mobility and manual dexterity collected remotely in aPwSMA.
Methods:
Data were collected from 59 aPwSMA (23 walkers, 20 sitters and 16 non-sitters) and 30 age-matched healthy controls (HC). SDMs were extracted from five smartphone-based tests capturing mobility and manual dexterity, which were administered in-clinic and remotely in daily life for four weeks. Reliability (Intraclass Correlation Coefficients, ICC) and construct validity (ability to discriminate between HC and aPwSMA and correlations with Revised Upper Limb Module, RULM and Hammersmith Functional Scale - Expanded HFMSE) were quantified for all SDMs.
Results:
The smartphone-based assessments proved feasible, with 92.1% average adherence in aPwSMA. The SDMs allowed to reliably assess both mobility and dexterity (ICC > 0.75 for 15/22 SDMs). Twenty-one out of 22 SDMs significantly discriminated between HC and aPwSMA. The highest correlations with the RULM were observed for SDMs from the manual dexterity tests in both non-sitters (Typing, ρ= 0.78) and sitters (Pinching, ρ= 0.75). In walkers, the highest correlation was between mobility tests and HFMSE (5 U-Turns, ρ= 0.79).
Conclusions:
This exploratory study provides preliminary evidence for the usability of smartphone-based assessments of mobility and manual dexterity in aPwSMA when deployed remotely in participants’ daily life. Reliability and construct validity of SDMs remotely collected in real-life was demonstrated, which is a pre-requisite for their use in longitudinal trials. Additionally, three novel smartphone-based performance outcome assessments were successfully established for aPwSMA. Upon further validation of responsiveness to interventions, this technology holds potential to increase the efficiency of clinical trials in aPwSMA.
INTRODUCTION
Spinal muscular atrophy (SMA) is an autosomal recessive neuromuscular disorder characterized by progressive loss of motor function due to the ongoing death of lower motor neurons in the anterior horn of the spinal cord [1]. SMA is a rare disease with a prevalence of 1 to 2 in 100,000 persons [1]. While the most prevalent SMA types have symptom onset occurring in infancy to childhood, adult onset can also occur. Further, the population of adult people with SMA (aPwSMA) is expected to grow over time due to rapid advances in disease-modifying therapies, which allows people with SMA to retain functional capacity until adulthood [2].
SMA displays a large heterogeneity of symptoms and disease trajectories [3, 4] and is typically categorized into three types based on disease onset and achieved motor milestones. SMA type I usually has an onset before 6 months of age and is characterized by severe disability of motor, eating, and breathing function. SMA type II has an onset within 6 to 18 months, and individuals typically can sit without support but are unable to stand or walk unaided, especially due to muscle weakness. SMA type III has an onset after 18 months of age and individuals reach, at least temporarily, the ability to walk without assistance, but they have difficulty walking and reduced proximal upper limb function. Irrespective of the type, aPwSMA are typically also classified according to their current motor capabilities as walkers, sitters and non-sitters. These classifications are instrumental to understand the clinical manifestation and progression under new disease-modifying therapies [5].
Design and implementation of successful clinical trials evaluating the effect of novel therapies in aPwSMA is challenging. Longitudinal disease progression is typically slow and outcome measures adopted in current trials are noisy, therefore resulting in low signal-to-noise ratio and demanding high sample size to detect treatment effect. Yet, SMA is a rare disease with a large phenotypic spectrum, thus limiting recruitment rates and sample sizes [6]. Highly responsive study endpoints with enhanced signal to noise ratio properties are essential to overcome this challenge and achieve sufficient statistical power for detecting treatment effects while keeping sample sizes small and study durationsshort.
Currently available endpoints are based on clinical outcome assessments, such as the Revised Upper Limb Module (RULM) or the Hammersmith Functional Motor Scale-Expanded (HFMSE) [7, 8]. These assessments are administered during sparse in-clinic study visits and rely on expert-based subjective rating of motor tasks. Such approaches can be affected by inter-rater variability, especially if assessors are not sufficiently trained [9]. This, paired with the infrequent collection of data that makes in-clinic assessment susceptible to day-to-day variations in disease symptomatology, leads to considerable measurement noise. Additionally, the visits to the clinic represent a sizeable burden for aPwSMA, given their severe muscle weakness and overall mobility impairment [10]. Hence, current clinical outcome assessments are burdensome and only have low to moderate responsiveness to measure longitudinal changes in disability of aPwSMA [11, 12]. It is expected that disease-modifying therapies in aPwSMA will delay disease onset, alter disease trajectories, and affect the time to transition in status between traditional SMA classifications. This creates the need to adapt the traditional assessments and identify new ways to track the heterogeneous SMA symptomatology in a feasible and ecological way within the daily life of aPwSMA [13]. Smartphone-based assessments have potential for augmenting outcomes collected during in-clinic visits by providing more frequent and objective assessment of disability through data collected remotely in the daily life of aPwSMA [11, 14]. This promises to reduce measurement noise, increase responsiveness and signal to noise ratio to ultimately lead to smaller and faster interventional clinical trials with a higher probability of technical success.
A few studies provided evidence for the usability of smartphone-based assessments performed in a variety of diseases, including Parkinson’s Disease [14–16]. Typically, data from a battery of upper and lower limb functional smartphone-based tests is collected remotely and transformed through dedicated signal processing pipelines into objective and numeric outcomes called sensor-derived measures (SDMs), which can allow to comprehensively quantify the loss of ability and the response to treatment. While smartphone-based assessments are promising to advance disease measurement in aPwSMA, further evidence on the usability, reliability and validity of SDMs is required to enable large-scale adoption in clinical trials.
The aim of this study is to investigate the feasibility of smartphone-based assessments of upper and lower limb function in aPwSMA and to assess the reliability and construct validity of remotely collected SDMs in aPwSMA. This will be achieved by fulfilling the following objectives: 1) patients’ adherence to the assessments and their satisfaction; 2) ability of SDMs to capture expected disease-related functional limitations when comparing able-bodied controls and aPwSMA; 3) differences between SDMs computed from multiple smartphone-based tests in daily life (unsupervised) versus their in-clinic (supervised) collection; 4) test-retest reliability of the selected SDMs; and 5) construct validity of the SDMs collected both in-clinic and remotely against standard in-clinic assessments of upper and lower limb function.
METHODS
Subjects and protocol
Data were collected as part of DigiNOA, an observational, cross-sectional, and multicentric study to assess the clinical validity of Konectom™ in aPwSMA (ClinicalTrials.gov identifier NCT05109637). aPwSMA and age and sex-matched healthy controls (HC) were recruited at five sites in Germany where local ethics approval was granted for the study (DE/EKNW32, Ethics Committee of the Medical Faculty of the University of Duisburg-Essen, DE/EKNI24, Ethics Committee of the Hannover Medical School, DE/EKBY08, Ethics Committee of the Faculty of Medicine of the LMU, Munich, DE/EKBW03, Ethics Committee of the Heidelberg Medical Faculty, DE/EKSN38, Ethics Committee at the TU Dresden). All participants were able to understand the purpose and risks of the study and provided informed written consent. The aPwSMA were recruited in each of the three functional categories (i.e., walkers, sitters and non-sitters), according to the following inclusion criteria: a chronological age between 18 and 64 years; genetic documentation of 5q SMA (homozygous gene deletion, mutation, or compound heterozygote); literacy in the use of mobile phones; willingness and capability to use a mobile phone during the study duration. Exclusion criteria included: severe depression (according to DSM-5 classification) or severe ongoing psychiatric condition, as per evaluation by the investigator; change of Disease Modifying Treatment (DMT) in the last 1 month; recent history of bacterial meningitis, viral encephalitis, or hydrocephalus; addiction (alcohol or another drug abuse); any clinically significant neurological disorders (e.g. mild cognitive impairment, dementia, etc.) other than SMA; presence of an implanted shunt for the drainage of CSF or of an implanted CNS catheter; hospitalization for surgery (i.e., scoliosis surgery or other surgery), pulmonary event, or nutritional support in the previous 2 months or planned within the study duration; currently participating in a Biogen-sponsored clinical study; known pregnancy. The study was powered evaluating the association between a single SDM and one standard clinical measure. Due to the exploratory nature of this study, multiple testing was not addressed. Using a type I error rate of 5% (two-sided) and hypothesized moderate correlation (r = 0.4) between a single SDM and one standard clinical measurement, it was estimated that the analysis will achieve an 90% power for evaluating 60 SMA patients.
A summary of the study protocol is provided in Fig. 1. Participants were initially invited to a baseline visit (V1), where their age, sex and anthropometric and general clinical characteristics were recorded. The HFMSE, RULM, Nine-Hole Peg Test (9HPT) and six-minute walk test (6MWT) were then administered, as per standard recommended protocols [17, 18]. Participants underwent a battery of tests that were administered via the Konectom digital outcome assessments tool (https://konectom.com/), which is a smartphone application designed to assesses upper and lower limb motor functions. The battery included two walking ability tests (an instrumented 6MWT (i6MWT) and a 5 U-Turn Test (5UTT)) that were only administered to walkers, and three manual dexterity tests (Drawing, Pinching and Typing). During the i6MWT and the 5UTT tests, participants were instructed to place their smartphones in a running belt at the lower back level. At V1 and V2, the i6MWT data were recorded during the standard clinical test (6MWT), while the phone was placed on the lower back using a running belt. The same belt was used during the 5UTT, in which the participant was asked to perform five steps and a U-Turn, in a sequence repeated five times. The Drawing test, designed to measure movement speed and accuracy, involved drawing a spiral, square, and infinity shape on the smartphone screen with the index finger as quickly and accurately as possible. The Pinching test, designed to measure visuomotor coordination and inter-finger coordination, involved pinching as many balloon shapes on the smartphone screen with the thumb and index finger as possible within 30 seconds. Patients were asked to perform the Drawing and Pinching with both their dominant and non-dominant hand if possible, or only with their functional side if not. Finally, in the Typing the participant was asked to type a series of words presented on the screen as quickly and accurately as possible using the smartphone keyboard.
Fig. 1
(A) DigiNOA Study Design. (B) Design of Konectom™ Upper and Lower Limb Assessments. (C) Description of test content.
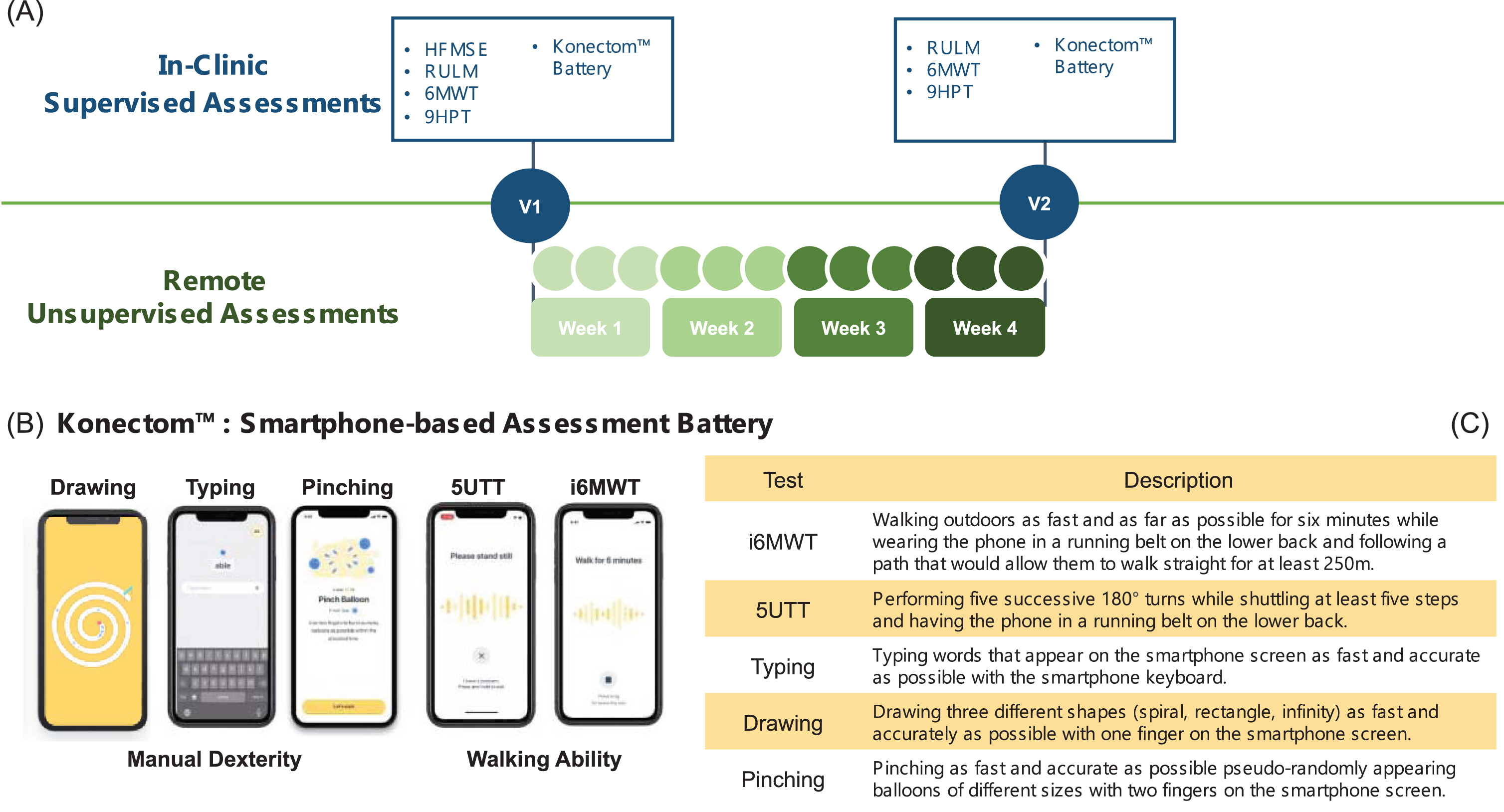
At the end of V1 participants were sent home with the smartphone (iPhone XR, Apple) and were asked to perform the same battery of tests in unsupervised conditions for four weeks, once a week for the i6MWT, and three times per week for the 5UTT and the dexterity tests. Differently from the V1 visit, in the remote i6MWT they were asked to walk outdoors as fast as they could for six minutes, following a path that would allow them to walk straight for at least 250 m. At the end of the four weeks, the participant’s satisfaction to the smartphone was assessed using a questionnaire administered via the same application. If participants were consistently not performing the smartphone-based assessments, the site would contact them and encourage them to adhere to the study schedule. The four-week observation period, which is in line with analogue studies in neurological diseases [14, 16, 19], was chosen as compromise between having enough data measured during a clinically stable period and minimising the burden to the patients.
A second in-clinic visit (V2) was performed twenty-eight days after V1 (with a 0–5 days window), which included an additional administration of the RULM, 9HPT, 6MWT and the supervised Konectom Battery.
Calculation of the sensor-derived measures (SDMs)
During each test, various sensors embedded in the pre-configured study smartphones were used to capture the necessary raw data. These sensors included screen input and coordinates (sampling frequency 60 Hz), and accelerometer, gyroscope, and magnetometer data (sampling frequency 50 Hz). Assessments’ metadata was also collected, including information such as the participant’s ID, the timestamp of each assessment, and the related session. Data security and integrity was ensured via a secure protocol for transmitting the recorded data from the smartphone to secure Microsoft Azure servers. To maximize data quality, data transmission would automatically stop for assessments where a major disruption occurred, and a specific exit reason would be recorded in the related metadata. After their transmission, the presence and consistency of expected data (e.g., accelerometer data being within a determined range) were verified. Finally, visual inspection of the recorded signals and purposely developed algorithms [20] were used to identify critical variations in the test executions, potentially occurring in remote unsupervised conditions. In particular, the following behaviors were identified as critical and used to identify signals or measures to be considered as non-valid and excluded from the analysis: 1) smartphone not worn in the belt during the 5UTT, and i6MWT; 2) no actual walking detected during the i6MWT; 3) no U-turns recorded during the 5UTT; 4) drawing path length not corresponding to the expected shape or unusable drawing segment greater than 10%; 5) no attempts detected in the pinching test; 6) use of the auto-complete suggestions during the typingtests.
Starting from the analysis of the smartphone signals, two SDMs were selected for each test (Fig. 1 and Table 1), according to the authors’ experience with similar data and the results from an interim analysis performed in data from twenty-three participants. For the sake of simplicity, for all dexterity tests, SDMs were only extracted from data collected with the dominant hand. Similarly, given that this is the most clinically adopted shape, the SDMs for the drawing test were only calculated for the spiralshape.
Table 1
List and description of the sensor derived measures (SDMs) selected for each smartphonebased test
Test | SDM name (unit) | Description | Aggregation level |
i6MWT | Step Power (ln (m2/s3)) | Natural logarithm of the integral of the mean-centered acceleration magnitude signal over a step. | Median over all detected straight walking bouts |
Stride Length (m) | Distance covered during one stride | Median over all detected straight walking bouts | |
5UTT | Duration (s) | Duration of a turn | Median over first 4 turns |
Mean Speed (rad/s) | Mean angular velocity recorded during the turn | Median over first 4 turns | |
Typing | Interval between correct letters (s) | Time interval between pairs of consecutive letters belonging to a correct series. A correct series ends when a user makes a mistake. | Median over the trial |
Reaction time (s) | The reaction time is the time elapsed between the appearance of a word and the time the user typed a letter. | Median over the trial | |
Drawing | Duration (s) | Time taken to draw a spiral shape with the dominant hand. | Median over all attempts |
Normalized Accuracy (1/(point*s)) | Accuracy (dissimilarity between reference and drawn shapes) in drawing a spiral shape with the dominant hand, normalized by the time spend between the first and last interaction of the subject with the screen. | Median over all attempts | |
Pinching | Successful Attempts (unitless) | The number of successful pinches for the dominant hand, where a successful pinch attempt is any screen interaction with at least two fingers down that leads to the target bubble bursting. | Median over all bubble sizes |
Speed (point/s) | Speed of the top finger during successful pinch attempts of the right hand. | Median over trial |
Data analysis
Patients’ adherence and satisfaction
Patients’ adherence was calculated considering the number of tests performed remotely by each participant as a percentage of the expected number according to the study protocol. An average adherence across different tests was calculated for each participant and the mean and standard deviations were then calculated across participants in each of the three groups. The analysis of the satisfaction questionnaire focused on the question ‘I would like to use Konectom regularly’, rated on an ordinal scale from 1 (absolutely disagree) to 5 (absolutely agree).
Differences between patients and controls
The first step to establish validity of the proposed remote monitoring approach entailed assessing the effectiveness of SDMs in discriminating between aPwSMA and HC. Aggregated data from all available valid remote tests were used to this purpose. Tests to evaluate statistically significant differences between groups were performed using a Mann– Whitney U test, with the level of significance set at p = 0.05.
Comparison between in-clinic and remote assessments
To establish the differences observed between supervised and unsupervised administration of the Konectom tests, the median of the SDMs values obtained at V1 and V2 for each subject were compared to the median of the SDMs from the valid remote assessments for the same subject. The median value between V1 and V2 was chosen in order to compare changes that occurred across the entire study period. SDM distributions in the two conditions were calculated and their differences were established using a Mann-Whitney U test with a level of significance of p = 0.05. Additionally, Z scores were derived (i.e., average of all valid remote minus average of V1 and V2, divided by standard deviation of V1 and V2) to enable an intuitive visualization of the differences between in-clinic and remote assessments that is comparable across SDMs.
Test-retest reliability
Test-retest reliability of the SDMs was assessed using the intra-class correlation coefficient (ICC 3, k) [21]. The ICC was used to summarize the relative magnitude of the inter- and intra-participant variability of a measure and values less than 0.50 were considered indicative of poor reliability, values between 0.50 and 0.75 of moderate reliability, values between 0.75 and 0.90 of good reliability, and values greater than 0.90 of excellent reliability. Intra-participant variability was also specifically assessed using the interquartile range (IQR) calculated across the valid remotes assessments. Finally, the minimum detectable change at 95% confidence (MDC95, also referred to as smallest real difference) [22, 23] was used to establish the level of measurement noise of an SDM, which is expected to be closely linked to its longitudinal responsiveness. The MDC95 was also expressed relative to the range of a SDM over the full dataset to enable comparability across SDMs (MDC95%). All computations were performed using only data from aPwSMA or HC for whom SDMs could be calculated from at least two valid remote assessments.
Construct validity
Construct validity was established calculating the Spearman correlations (ρ) between the SDMs and suitable clinical assessment scores (HFMSE, RULM, 9HPT and 6MWT). The analysis was performed calculating the correlations (V1) between the clinical assessment scores and the SDMs at V1 and between the V1 clinical assessment scores and the median over the valid remotely collected SDMs (R-V1). Correlations were considered as significant if p < 0.05 and the correlation coefficients were interpreted as very high: ∣ρ∣≥0.9; high: 0.7≤∣ρ∣ < 0.9; moderate: 0.5≤∣ρ∣ < 0.7; low: 0.3≤∣ρ∣ < 0.5; very low: ∣ρ∣ < 0.3 [23].
RESULTS
The recruited sample (Table 1) included 59 aPwSMA (23 walkers, 20 sitters and 16 non-sitters) and 30 HC, with all groups being balanced in terms of sex and age and most participants having a right-hand dominance. In the aPwSMA cohort the HFMSE ranged between 0 and 63 and the RULM between 0 and 37, with a clear separation between the three groups, as expected.
Patients’ adherence
Participants from all three groups presented high adherence to the study protocol (Fig. 2), with an average adherence of 92.1% ±12.7% across aPwSMA. Lowest adherence values were observed for the walkers in the i6MWT (84.1% ±37.8%). This result was driven by two participants, who reported that they would not feel comfortable in remotely performing the i6MWT since they would normally only go outdoor using a wheelchair. The median rating of the question whether aPwSMA would like to use Konectom regularly was 3±1, representing the mid-point of the satisfaction scale.
Fig. 2
Mean adherence results shown at (a) group level (mean adherence per participant across all tests) and at (b) test level (mean adherence per test across all participants). The bars represent the corresponding standard deviations, which were clipped to the maximal possible adherence (100%).
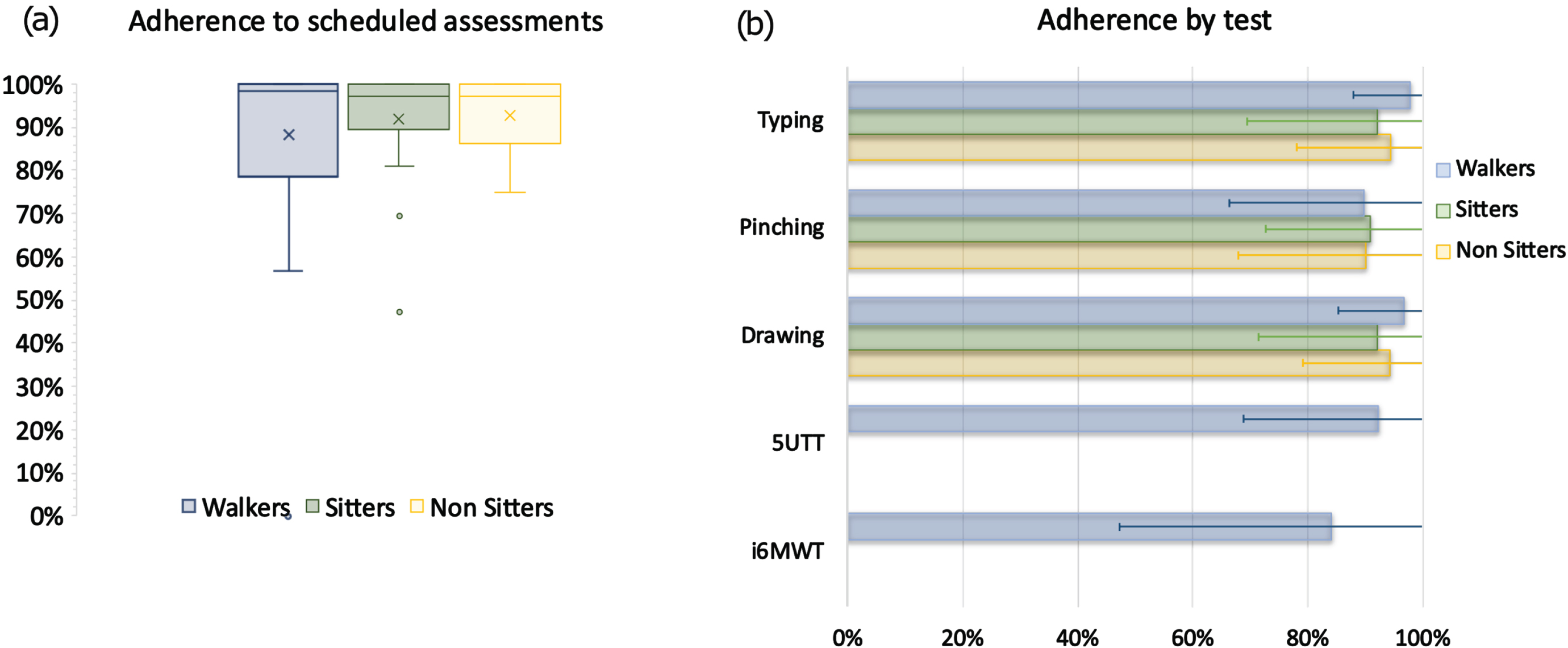
The detection of critical variations in test executions listed in the methods and additional technical checks causing the inability to calculate the SDMs of the Drawing test, led to discarding several recordings, as per details reported in Table 3. The behavior that led to discard most data for the current analysis was the use of the belt, which was not worn by a large part of the HC. No disease related patterns were observed in the occurrence of deviations from test instructions. Data from the following participants could not be used due to deviations from test instructions, technical checks, or because the test has not been performed at all: 3 walkers and 13 HC for the i6MWT; 4 walkers and 12 HC for the 5UTT; 1 sitter and 3 non-sitters for the drawing; and 1 sitter and 5 non-sitters for the pinching. Table S1 in the Supplementary Material provides the details about the final number of recordings remaining available per each participant, which were those effectively used for all following analyses.
Table 2
Summary of the characteristic of the study groups. IQR = interquartile range; HFMSE = Hammersmith Functional Motor Scale Expanded; RULM = Revised Upper Limb Module; 9HPT=Nine-Hole Peg Test; 6MWT=6-minute walk test
Variable | Walkers (N = 23) | Sitters (N = 20) | Non-sitters (N = 16) | Healthy Controls (N = 30) |
Sex, n (%) | Females = 12 (52.2) Males = 11 (47.8) | Females = 11 (55) Males = 9 (45) | Females = 9 (56.3) Males = 7 (43.8) | Females = 17 (56.7) Males = 13 (43.3) |
Males = 11 (47.8) | Males = 9 (45) | Males = 7 (43.8) | Males = 13 (43.3) | |
Age, median, y (IQR) | 40 (33–54) | 34 (29–40) | 40 (33–45) | 38 (30–42) |
Height, median, cm (IQR) | 172 (168.5–180.5) | 155 (146.5–166) | 160 (147–165.75) | 173.5 (168–178) |
Mass, median, kg (IQR) | 72 (64.5–81) | 48 (38.8–76) | 51 (45–76.5) | 70.75 (67.25–78) |
SMA type | Type 2 = 0; Type3 = 22; Type4 = 1 | Type 2 = 11; Type3 = 9; Type4 = 0 | Type 2 = 11; Type3 = 5; Type4 = 0 | – |
Type4 = 1 | Type4 = 0 | Type4 = 0 | ||
Time since diagnoses median, y (IQR) | 18 (13–33) | 31 (28–38) | 36 (31–44) | – |
Walking device, n (%) | – | |||
None | 10 (43.5) | 0 (0) | 0 (0) | 30 (100) |
Wheelchair | 4 (17.4) | 3 (15) | 0 (0) | 0 (0) |
Motorized wheelchair | 1 (4.3) | 17 (85) | 16 (100) | 0 (0) |
Others (crane, crutch, rollator) | 8 (34.8) | 0 (0) | 0 (0) | 0 (0) |
Right-hand dominance,n (%) | 21 (91.3) | 18 (90) | 14 (87.5) | 25 (83.3) |
HFMSE, median (IQR) | 44 (35–49) | 6 (4–8) | 1 (0–2) | – |
RULM, median (IQR) | ||||
dominant hand | 37 (20–37) | 16 (14–18) | 10 (7–14) | – |
non dominant hand | 36 (34–37) | 14 (11–17) | 10 (3–14) | – |
6MWT distance median, m (IQR) | 319.3 (170.6–396.6) | – | – | 556.8 (452.9–577.8) |
9HPT median, s (IQR) | ||||
dominant side | 20.1 (19.1–21.4) | 28.4 (22.7–30.9) | 57.2 (35.1–128.6) | 17.3 (16.3–18.9) |
non dominant side | 21.6 (20.3–24.1) | 36.5 (32.7–45.7) | 61.3 (59.3–78.4) | 17.3 (16.3–18.7) |
Table 3
Details of the trials that were discarded due to the presence of critical variations in test executions or technical checks as detected separately for each test and for the aPwSMA and the HC
Test | Reason for Invalidating Test recordings | aPwSMA | Controls | ||||
Number of occurrences | Available Recordings | Invalidated recordings (%) | Number of occurrences | Available Recordings | Invalidated recordings (%) | ||
i6MWT | No walking detected in the signals | 10 | 133 | 10.4 | 0 | 195 | 0 |
Phone not in the belt | 31 | 133 | 32.3 | 136 | 195 | 69.74 | |
5UTT | No turns detected | 1 | 295 | 0.4 | 0 | 411 | 0 |
Technical issues with the signals | 1 | 295 | 0.4 | 0 | 411 | 0 | |
Phone not in the belt | 132 | 295 | 47.5 | 297 | 411 | 72.26 | |
Typing | Use of Auto-Correction | 12 | 806 | 1.5 | 2 | 415 | 0.48 |
Pinching | No pinching detected | 103 | 787 | 13.8 | 2 | 414 | 0.48 |
Drawing | Drawing path length outside expected range | 1 | 817 | 0.1 | 2 | 427 | 0.47 |
Unusable drawing segment greater than 10% | 4 | 817 | 0.5 | 2 | 427 | 0.47 |
Ability to discriminate between patients and controls
All SDMs were able to discriminate between the two groups (p≤0.01), except for the typing reaction time (1.21±0.33 in aPwSMA vs 1.11±0.15 in HC, p = 0.111), with all differences being in the expected directions (Fig. 3). Stride length was 19.6% higher in HC who also had 48% larger step power and turned 27% faster in the 5UTT. aPwSMA were 48% slower at typing correct letters and 28% slower at pinching, and they took longer and had worse speed-normalized accuracy in their drawing (27% and 26%, respectively).
Fig. 3
Values of the SDMs for each of the tests for the aPwSMA (orange) and the HC (blue). All valid remote data points before aggregation are visualized.
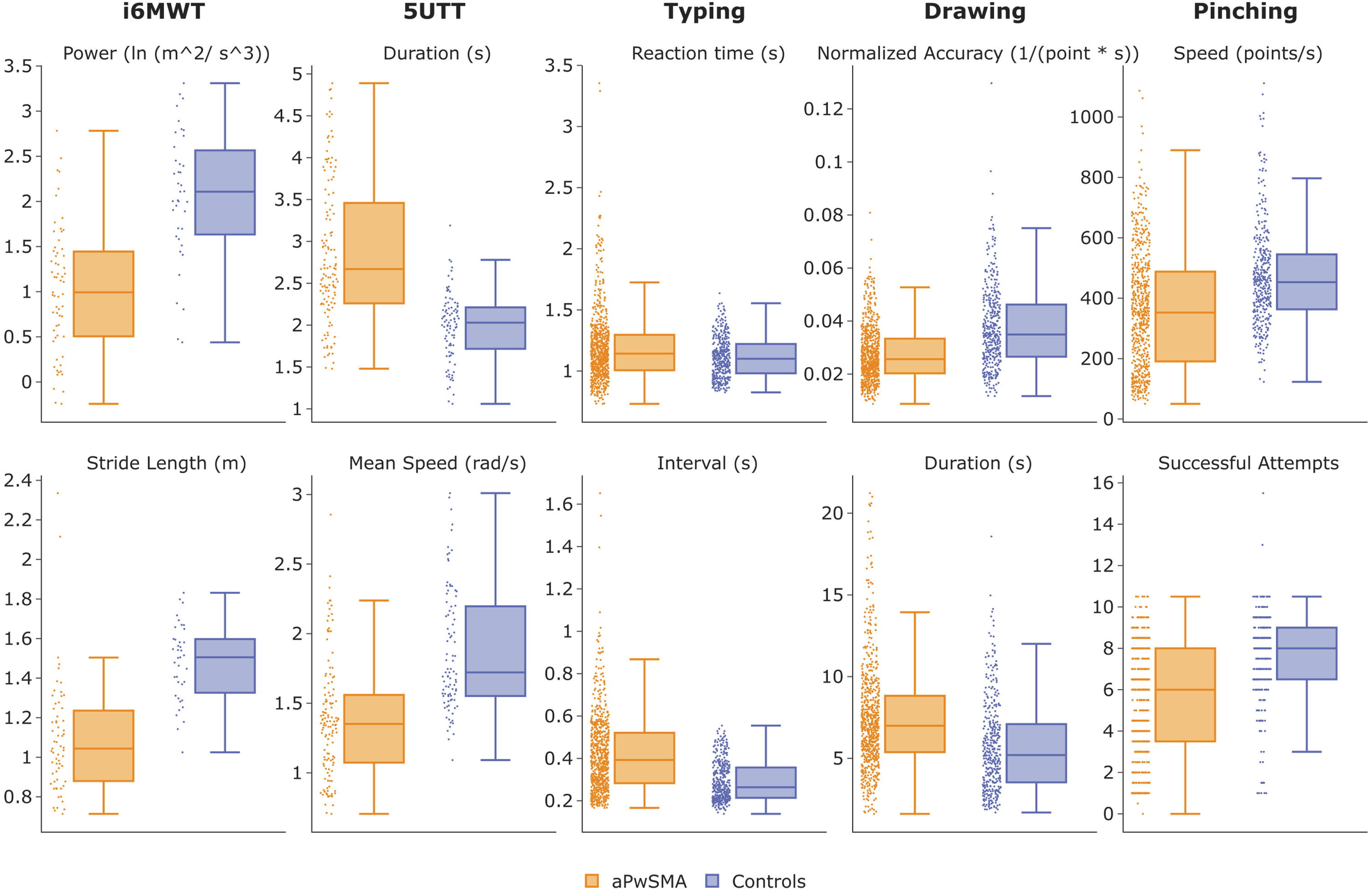
Comparison between in-clinic and remote assessments
The group analysis showed that none of the SDMs, on average, significantly differed when comparing data collected in-clinic and remotely (p > 0.05 in all comparisons). At individual level, considerable changes were observed in the SDM between the conditions (Fig. 4). These changes, however were highly variable across both individuals and functional groups and as such the average z-score values were all close to 0. The i6MWT step power was the most variable among these, while the 5UTT SDMs seemed to be quite stable. Similar pattern of stability was observed for the Typing, while slightly bigger variations were observed for the Drawing and even bigger in the Pinching, especially for the sitters.
Fig. 4
Changes in the SDMs values when comparing data collected in-clinic (median between V1 and V2) and the remote environment (median across all valid remotes). The horizontal boxes represent the distribution of the values calculated for each subject in each of the three groups.
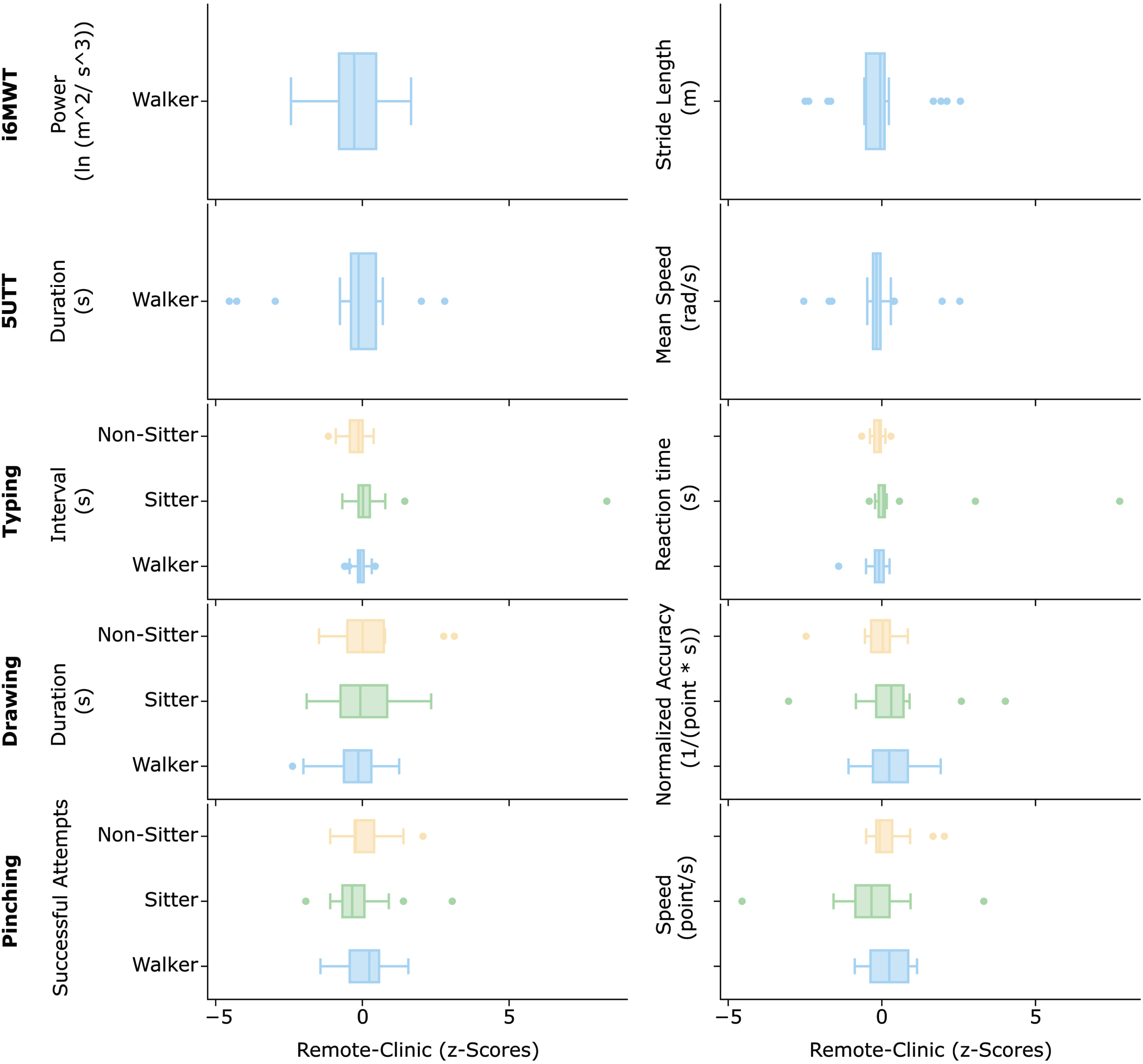
Test-retest reliability
Figure 5 illustrates the changes over times of the SDMs, as observed in the three groups. Most SDMs proved to be highly reliable across repeated remote measurements for all groups, with good to excellent ICC values (Table 4). The average ICC was 0.78±0.16 across SDMs. The highest ICC values and the lowest MDC values were observed for the sitters in all three dexterity tasks. While high ICC values were found for the walkers in the 5UTT and in the i6MWT, these groups tended to perform less reliably in the drawing and pinching tests. The average MDC95% was 31.3% ±12.5%, was lowest for the SDMs from the Typing in sitters (10.1% and 12.0%) and highest for SDMs from the Pinching in walkers (59.2% and 47.3%).
Fig. 5
Variation of the SDMs across repeated remote measurements for the three groups of aPwSMA for (a) mobility-related and (b) dexterity-related smartphone tests. The colored lines represent the data from each individual, while the black line represents the mean values, and the shaded bands represent the confidence intervals calculated at each visit.
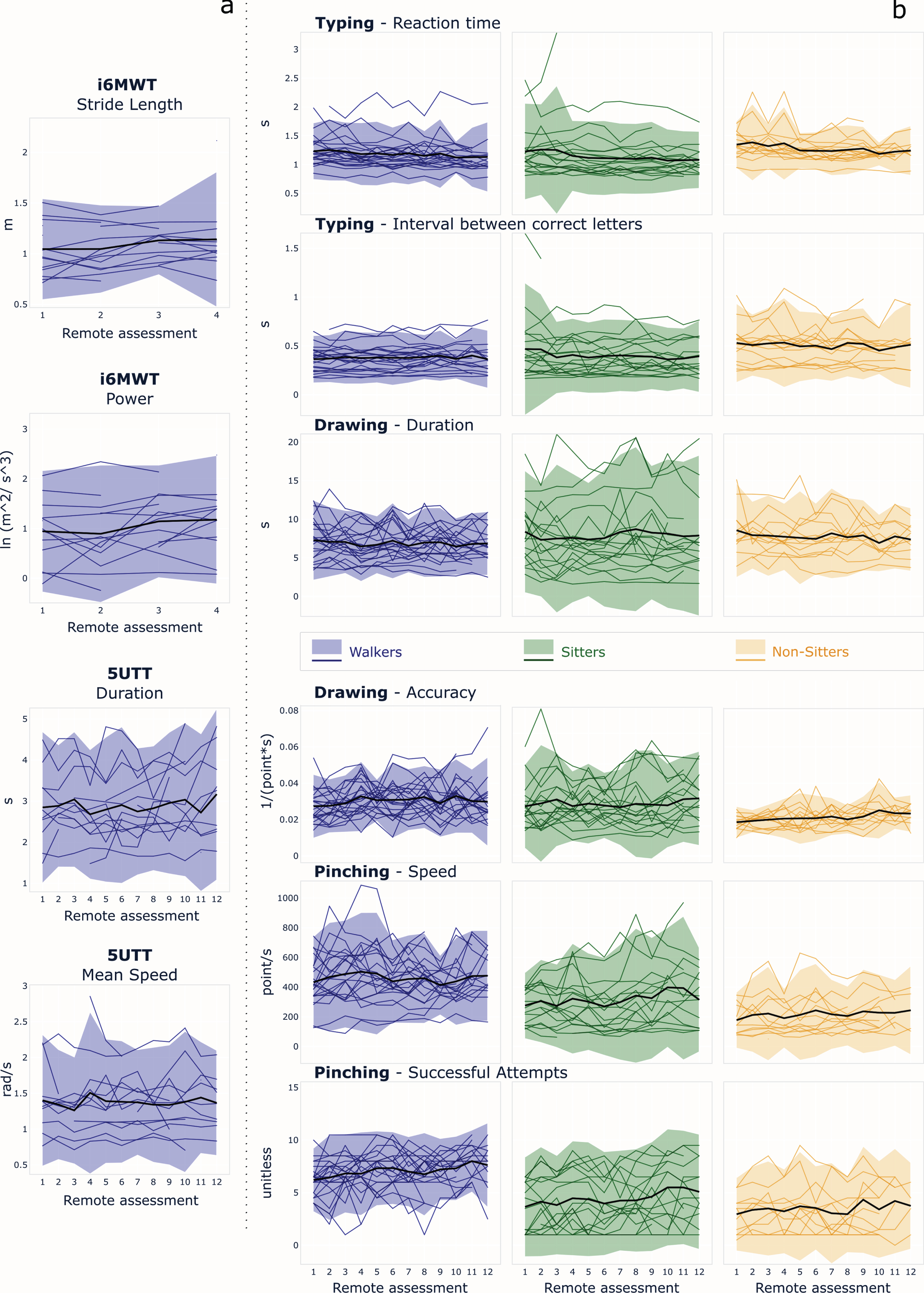
Table 4
Summary of the test-retest reliability of the SDMs across remote sessions for the three groups and the five tests. IQR = interquartile range; ICC = intraclass correlation coefficient; MDC95 = minimum detectable change. MDC95% =MDC normalized with respect to range of SDM. ICC values above 0.75 (good reliability) are in bold font
Test | SDM | Median | IQR | ICC | MDC95 | MDC95% |
Walkers | ||||||
i6MWT | Step power (ln (m2/s3)) | 1.12 | 0.21 | 0.81 | 0.87 | 36.4 |
Stride length (m) | 1.09 | 0.06 | 0.93 | 0.25 | 34.4 | |
5UTT | Turn Duration (s) | 2.58 | 0.32 | 0.80 | 1.08 | 38.4 |
Mean Speed (rad/s) | 1.40 | 0.12 | 0.88 | 0.44 | 32.3 | |
TYPING | Interval between correct letters (s) | 0.38 | 0.04 | 0.90 | 0.12 | 25.6 |
Reaction time (s) | 1.14 | 0.07 | 0.89 | 0.25 | 29.1 | |
DRAWING | Duration (s) | 0.28 | 0.08 | 0.88 | 4.43 | 22.6 |
Normalized Accuracy (1/(points*s)) | 0.03 | 0.01 | 0.48 | 0.02 | 59.3 | |
PINCHING | Successful Pinches (unitless) | 7.25 | 1.25 | 0.56 | 3.26 | 59.2 |
Speed (point/s) | 0.48 | 0.12 | 0.68 | 0.27 | 47.3 | |
Sitters | ||||||
TYPING | Interval between correct letters (s) | 0.35 | 0.04 | 0.97 | 0.15 | 10.1 |
Reaction time (s) | 1.06 | 0.09 | 0.94 | 0.31 | 12.0 | |
DRAWING | Duration (s) | 0.28 | 0.08 | 0.88 | 4.43 | 22.6 |
Normalized Accuracy (1/(points*s)) | 0.03 | 0.01 | 0.77 | 0.02 | 23.2 | |
PINCHING | Successful Pinches (unitless) | 0.25 | 0.08 | 0.81 | 3.22 | 37.9 |
Speed (point/s) | 3.50 | 1.13 | 0.80 | 0.24 | 26.0 | |
Non-Sitters | ||||||
TYPING | Interval between correct letters (s) | 0.52 | 0.06 | 0.88 | 0.18 | 21.0 |
Reaction time (s) | 1.26 | 0.13 | 0.73 | 0.32 | 23.0 | |
DRAWING | Duration (s) | 7.45 | 1.10 | 0.68 | 3.88 | 30.3 |
Normalized Accuracy (1/(points*s)) | 0.02 | 0.00 | 0.30 | 0.01 | 39.5 | |
PINCHING | Successful Pinches (unitless) | 3.00 | 1.38 | 0.72 | 3.30 | 34.7 |
Speed (point/s) | 0.14 | 0.06 | 0.77 | 0.17 | 29.7 |
Construct validity
Spearman correlation coefficients between the clinical scales and the SDMs are shown in Table 5. The correlations were all in the expected directions and magnitude ranges (i.e., low to high correlations dependent on SDMs and chosen clinical outcomes). Stronger correlations with HFMSE and 6MWT were found for the walkers for SDMs from the 5UTT than from the i6MWT. Pinching led to the strongest correlations for the sitters, while in the non-sitters, the highest values were found for SDMs from the Typing test. Correlations between the Drawing test and the RULM were low for the sitters and moderate for the non-sitters. The Drawing and Pinching tests showed moderate and high correlations for at least one SDM. The Walking SDMs from the remote sessions showed stronger convergent validity with the HFMSE and the 6MWT than those from the in-clinic visits, both for i6MWT and the 5UTT. Pinching SDMs from remote sessions, as compared to in-clinic visits, had overall stronger correlations with the RULM and 9HPT for both sitters and non-sitters.
Table 5
Spearman correlation coefficients between the clinical scales and the SDMs at V1 and between SDMs from remotes sessions and clinical scales at V1 (R-V1). The coloring indicates the strength of the absolute correlation values (white = absent or very low; lightest green = low; medium green = moderate; darkest green = high)
![]() |
DISCUSSION
Novel outcome measures of SMA progression, more feasible in daily practice and more responsive than current clinical outcome assessments, are urgently needed to improve the efficiency of clinical trials developing disease-modifying therapies [6, 11]. To this end, this study investigated the usability, construct validity, and reliability of a novel smartphone-based remote assessment of upper and lower limb functions in adult people with SMA with different disease phenotypes.
The battery of here proposed tests entails some novel elements compared to previous similar studies in aPwSMA, which focused on respiratory and upper limb tests [14]. Specifically, the addition of the i6MWT and 5UTT allowed to also assess mobility, which is especially relevant in aPwSMA that are still walkers and do not yet exhibit severe upper limb and respiratory symptoms [25]. Additionally, the inclusion of the Typing test allowed to measure aspects of fine manual dexterity, which are expected to be especially relevant in aPwSMA who are sitters and non-sitters. Feasibility (Objective 1) of the proposed assessment was confirmed by very high adherence of the aPwSMA group to the scheduled smartphone-based remote assessments across all tests (92.1%). This was achieved by tailoring the battery of tests to the functional status of the participants and by the possibility for the study team to access real time adherence data and use this information to re-engage non-compliant participants. This provides further evidence that smartphone-based assessments might have high usability in neurological and neuromuscular disorders if administered correctly and their integration is supported by study sites [14, 26, 27]. Nonetheless, this was a relatively short study and further investigation is needed to confirm maintenance of these observations in a longer interventional trial. The satisfaction questionnaire revealed that aPwSMA would be only moderately willing to use Konectom outside a study setting. This highlights that further research is required to add gamification elements to the application [14] and reduce the number of smartphone-based tests to a core set that is clinically meaningful, highly responsive, and further reduces assessment duration.
Performing a structured test in unsupervised remote conditions can lead to different behaviors than those adopted in the clinic (Objective 3). This is an important aspect that is often underestimated and rarely reported in studies evaluating smartphone-based assessments. The automated detection of some of these behaviors allowed us to isolate and exclude unreliable measures. Nonetheless, further effort should be devoted to these aspects, especially in the manual dexterity-related tests (Typing, Drawing, Pinching), where variability in phone handling (e.g., phone static on the table instead of handheld) and execution criteria (e.g. preferring speed over accuracy) might become a critical confounder. The biggest differences between in-clinic versus remote unsupervised test administrations were observed for the i6MWT, which can be explained by the differences in the test protocols: in the clinic, this test is administered in the form of a shuttle walk along a relatively short path, while in remote settings it is performed outdoors along long rectilinear stretches. Expectedly, the differences between in-clinic and remote were strongest for the step power SDM of the i6MWT, which is highly dependent on the walking speed and hence likely to differ between the two conditions. The smallest differences between in-clinic and daily life for manual dexterity-related tests were observed for Typing, thus further underlining its robustness, ecological nature and relevance for assessing manual dexterity in aPwSMA. Other possible causes for the differences in the Drawing and Pinching SDMs might be practice effects and motivation. Further studies are warranted to test these hypotheses.
Evidence for the reliability of the remote assessments (Objective 4) was provided by the ICC, which is an overall indicator accounting for the ability of a SDM to discriminate both within a participant across repeated measurements (intra-participant variability) and between participants (inter-participant variability). The ICCs were good to excellent (>0.75) for 15 out of 22 SDMs across functional groups. Moderate values were found for the Drawing test in sitters and walkers (only for normalized accuracy, ICC = 0.30) and for the Pinching test in walkers. Overall, these values indicate low intra-participant variability compared to inter-participant variability, suggesting that the SDMs obtained from smartphone-based assessments are good measures to sensitively discriminate between aPwSMA and repeated measures. Lower ICCs likely stemmed from low inter-participant variability in subgroups with high level of functioning in a given concept of interest (e.g., manual dexterity in walkers) due to the progressive course of SMA, but further studies would be needed to fully corroborate this hypothesis. In the specific case of the normalized accuracy SDM from the Drawing test, the low ICC results were likely because of the chosen algorithmic implementation, which uses dynamic time warping to match the drawn to the reference shape. Alternative implementations should be explored in the future to address this shortcoming [28]. Nevertheless, the results are overall in line with previous work that also reported good to excellent test-retest reliability in smartphone-based assessments in aPwSMA [14].
The analysis of test-retest reliability also showed the potential of the selected SDMs as sensitive measures of disease progression, which was investigated using the MDC95% as a quantification of their measurement noise. The MDC95% establishes the range of values (relative to a measures’ expected range of variation) below which it would not be possible to distinguish between an actual change in the measured concept of interest (e.g., because of an intervention) and the measurement noise (e.g., random noise in repeated measures). This is closely linked to the responsiveness of a measure when longitudinally monitoring an individual in a clinical trial [29]. Average MDC95% was 31.3% across SDMs and functional groups, while being lowest (10.1%) for the Typing in sitters and highest (59.2%) for the Pinching in walkers. While previous research suggested that MDC95% of SDMs should be below 10% or 30% [30, 31], these thresholds do not directly apply to the specific case of identifying SDMs for drug development purposes and further studies based on interventional data would be needed to establish the correct ones. Nonetheless, reported results can be used to concurrently compare tests and SDMs. The SDMs from Typing and Drawing had lowest measurement noise across all functional subgroups and as such are ultimately most promising to exhibit high responsiveness in longitudinal studies. Conversely, the Pinching test exhibited highest MDC95% values and highest measurement noise, likely due to the unusual and complex two-finger interaction with the smartphone. Measurement noise might also have been influenced by practice effects leading to systematic improvement in test performance, typically observed in manual dexterity tests having a cognitive component susceptible to practice [32]. Further studies are needed to investigate these aspects and identify a strategy to further reduce measurement noise.
The construct validity (Objective 5) of the proposed assessments was proven by the ability of SDMs to measure the desired concept of interest and capture disability consistent with the clinical scales. This study showed for the first time that measures from smartphone-based tests significantly discriminated between HC and aPwSMA (Objective 2), capturing the expected impairments in aPwSMA. The only exception was the Typing reaction time, likely due to this capturing cognitive function rather than dexterity, with the former unlikely to be affected in aPwSMA [33]. The letter interval, more related to dexterity, was indeed able to discriminate between the two groups, confirming the potential of the Typing. This test might also be usable in a continuous passive monitoring scenario [34], further reducing the burden to perform standardized assessments.
Additional support to the claim that the investigated tests provide valid measures of manual dexterity and mobility in aPwSMA was provided by the correlations between SDMs and clinical outcome assessment scores that were in the expected moderate to high ranges. The highest correlations were observed between the Typing test (letter interval) and the RULM and 9HPT in non-sitters, between the Pinching test (speed) and the RULM in sitters, and between the i6MWT (stride length) and 5UTT (mean speed) with the 6MWT distance in walkers, respectively. These results also support the effectiveness of the envisioned strategy of tailoring the battery of smartphone-based tests to each functional subgroup to capture different levels of disease progression. Interestingly, the correlations between traditional clinical outcome assessments and SDMs differed when considering SDMs from data collected in-clinic or remotely in daily life. For the i6MWT in walkers, considerably stronger correlations with the 6MWT were observed when considering SDM data collected in daily life as compared to in-clinic. The chosen gait measures, step power and stride length, are expected to be more representative of overall gait function when estimated for steady state gait. Hence, it is not surprising that they more strongly correlated with the distance walked in 6 minutes when they were calculated from remote assessments, performed along straight outdoor path in daily life. The distance walked during a standard clinical 6MWT is also highly affected by turning ability, which is a component well captured by the 5UTT test both in the clinics and remotely. For sitters and non-sitters, the SDMs extracted from remote Pinching tests performed in daily life were more strongly correlated to the RULM and 9HPT than were SDMs obtained from in-clinic Pinching tests. This may be explained by an initial practice effect that must overcome to be able to adequately capture manual dexterity in aPwSMA. For the other smartphone-based tests, the change in correlation between in-clinic and remote conditions was less strong or inconsistent across SDMs. This indicates that the smartphone-based assessment of mobility and manual dexterity is robust across data collection conditions, despite the variability in the SDMs when comparing data collected in-clinic and remotely in daily life (Fig. 4). This further speaks for the validity of SDMs that were collected remotely in daily life with smartphone-based assessments.
This study had several limitations. The sample size was relatively small and the design was cross-sectional. Future studies are warranted with longitudinal data collection to evaluate the responsiveness of the SDMs and their potential to make clinical trials faster and smaller. Also, the participants enrolled in this study were adults. Additional validation work is required to make such SDMs accessible to pediatric SMA populations [14]. While a large variety of SDMs can be extracted from smartphone-based assessments, only two SDMs per test were pre-selected in a hypothesis- and data-driven manner. In the future, a comprehensive SDM selection process should be implemented that uses separate training and validation datasets and transparently reports all SDMs that were initially considered [14, 35].
This study has a number of strengths, too. Specifically, the design enabled a rigorous evaluation of the differences between in-clinic and remote test administration, test-retest reliability of SDMs, and a thorough construct validity analysis, including an analysis of differences in SDMs between aPwSMA and healthy controls. This contrasts with other studies that directly integrated smartphone-based assessments into longitudinal drug development trials, which makes the latter suboptimal for specific validation of smartphone-based assessments. Another strength is that the study was able to obtain SDMs with high test-retest reliability being multi-centric with five sites. This suggests that any instructions potentially provided by clinical personnel, in addition to the standardized instructions in our smartphone application, have minor influence on test performance and paves the way for integration in multi-center and multi-country clinical trials. Novelties of this work are the deployment of the previously developed i6MWT and 5UTT and the introduction of the Typing test, which showed excellent measurement properties to assess mobility and manual dexterity in aPwSMA. Taking all available evidence into consideration, further developments should focus especially on the Typing test in sitters and non-sitters, and the i6MWT and 5UTT in walkers.
CONCLUSIONS
This exploratory work provided important additional evidence of the usability of smartphone-based assessments of upper and lower limb function in aPwSMA and of the reliability and construct validity of SDMs extracted from data collected remotely in daily life. Upon further validation of the SDMs’ responsiveness in longitudinal studies, this technology promises to increase the efficiency of interventional clinical trials by enabling the use of endpoints with higher signal-to-noise ratio, leading to potentially smaller sample size and shorter duration, while reducing the burden of sites and study participants through remote and more ecological assessments performed in daily life.
ACKNOWLEDGMENTS
We wish to thank all the patients, family members and staff from all the units that participated in the study. We are particularly thankful to Imen Alioua, Louise Marais, N’Deye Seck, Houria Chekroun, and Nicholas Levitt at Biogen Digital Health for their support.
CONFLICTS OF INTEREST
EAB, GC, CK, AK, CM, JPA, CZ, CS, KME, and SB were Biogen employees at the time of writing and might hold stock of the Company. HSL has received advisory fees from Biogen outside of the submitted work. SW has received research grant by the DGM— Deutsche Gesellschaft für Muskelkranke e.V. He has served on advisory boards for Alexion Pharma, UCB Pharma GmbH, AMICUS Therapeutics GmbH, and Sanofi Genzyme GmbH. He received funding for travel or speaker Honoraria from Sanofi-Aventis Germany GmbH; SH Glykogenose Gesellschaft; AbbVie Germany GmbH; Recordati Pharma GmbH; CSLBehring GmbH; Alexion Pharma GmbH; Desitin Germany; Akcea GmbH. SP received honoraria as speaker/consultant from Biogen GmbH, Roche, Novartis, Teva, Cytokinetics Inc., Desitin, Italfarmaco, Amylyx, and Zambon; and grants from DGM e.V, Federal Ministry of Education and Research, German Israeli Foundation for Scientific Research and Development, EU Joint Program for Neurodegenerative Disease Research and Neurodegenerative Research, Inc., outside of the submitted work. MW has received advisory board and consultant honoraria from Biogen and Hoffmann-La Roche, speaker honoraria and travel support for conference attendance from Biogen, outside of the submitted work, and is a member of the
European Reference Network for Neuromuscular Diseases (ERN EURO-NMD). WMC attended advisory boards of AveXis Pharma, Biogen Pharma GmbH, Novartis Pharma GmbH, Pfizer Inc., PharNEXT, PTC Therapeutics, Roche Pharma AG, RTI HS, Santhera Pharmaceuticals, Sarepta Therapeutics Inc, received funding for travel and speaker honoraria from Biogen Pharma GmbH, Colloquium Neurologicum, Novartis Pharma GmbH, PTC Therapeutics, Santhera Pharmaceuticals, and consulted for Affinia, Audentes Therapeutics, Avexis, Biogen Pharma GmbH, BridgeBio, Edgewise, Fulcrum Therapeutics, Grünenthal PharmaML Bio, Novartis Pharma GmbH, Pfizer Inc., PharNEXT, PTC therapeutics, Roche Pharma AG. RG has received personal fees from Biogen, Hofmann-La Roche, Zambon and ITF Pharma and research support from Biogen, all support was received outside the scope of the submitted work. TH received compensation for adboard consultancy, speaker fees and research support from Biogen and Roche and research support from Novartis.
DATA AVAILABILITY STATEMENT
The data supporting the findings of this study are available within the article and/or its supplementary material.
SUPPLEMENTARY MATERIAL
[1] The supplementary material is available in the electronic version of this article: https://dx.doi.org/10.3233/JND-240004.
REFERENCES
[1] | Verhaart IE , Robertson A , Wilson IJ , Aartsma-Rus A , Cameron S , Jones CC , Cook SF , Lochmüller H . Prevalence, incidence and carrier frequency of 5q– linked spinal muscular atrophy– a literature review. Orphanet Journal of Rare Diseases. (2017) ;12: (1):1–5. |
[2] | Hagenacker T , Wurster CD , Günther R , Schreiber-Katz O , Osmanovic A , Petri S , Weiler M , Ziegler A , Kuttler J , Koch JC , Schneider I , Wunderlich G , Schloss N , Lehmann HC , Cordts I , Deschauer M , Lingor P , Kamm C , Stolte B , Pietruck L , Totzeck A , Kizina K , Mönninghoff C , von Velsen O , Ose C , Reichmann H , Forsting M , Pechmann A , Kirschner J , Ludolph AC , Hermann A , Kleinschnitz C . Nusinersen in adults with 5q spinal muscular atrophy: a non-interventional, multicentre, observational cohort study. Lancet Neurol. (2020) ;19: (4):317–25. |
[3] | Wirth B , Karakaya M , Kye MJ , Mendoza-Ferreira N . Twenty-Five Years of Spinal Muscular Atrophy Research: From Phenotype to Genotype to Therapy, and What Comes Next. Annu Rev Genomics Hum Genet. (2020) ;21: , 231–61. |
[4] | Coratti G , Messina S , Lucibello S , Pera MC , Montes J , Pasternak A , Bovis F , Exposito Escudero J , Mazzone ES , Mayhew A , Glanzman AM , Young SD , Salazar R , Duong T , Muni Lofra R , De Sanctis R , Carnicella S , Milev E , Civitello M , Pane M , Scoto M , Bettolo CM , Antonaci L , Frongia A , Sframeli M , Vita GL , D’Amico A , Van Den Hauwe M , Albamonte E , Goemans N , Darras BT , Bertini E , Sansone V , Day J , Nascimento Osorio A , Bruno C , Muntoni F , De Vivo DC , Finkel RS , Mercuri E . Clinical Variability in Spinal Muscular Atrophy Type III. Ann Neurol. (2020) ;88: (6):1109–17. |
[5] | Maggi L , Bello L , Bonanno S , Govoni A , Caponnetto C , Passamano L , Grandis M , Trojsi F , Cerri F , Ferraro M , Bozzoni V , Caumo L , Piras R , Tanel R , Saccani E , Meneri M , Vacchiano V , Ricci G , Soraru’ G , D’Errico E , Tramacere I , Bortolani S , Pavesi G , Zanin R , Silvestrini M , Politano L , Schenone A , Previtali SC , Berardinelli A , Turri M , Verriello L , Coccia M , Mantegazza R , Liguori R , Filosto M , Marrosu G , Siciliano G , Simone IL , Mongini T , Comi G , Pegoraro E . Nusinersen safety and effects on motor function in adult spinal muscular atrophy type 2 and 3. J Neurol Neurosurg Psychiatry. (2020) ;91: (11):1166–74. |
[6] | Bonati U , Holiga Š , Hellbach N , Risterucci C , Bergauer T , Tang W , Hafner P , Thoeni A , Bieri O , Gerlach I , Marquet A . Longitudinal characterization of biomarkers for spinal muscular atrophy. Annals of Clinical and Translational Neurology. (2017) ;4: (5):292–304. |
[7] | Pera MC , Coratti G , Forcina N , Mazzone ES , Scoto M , Montes J , Pasternak A , Mayhew A , Messina S , Sframeli M , Main M . Content validity and clinical meaningfulness of the HFMSE in spinal muscular atrophy. BMC Neurology. (2017) ;17: (1):1–0. |
[8] | Mazzone ES , Mayhew A , Montes J , Ramsey D , Fanelli L , Young SD , Salazar R , De Sanctis R , Pasternak A , Glanzman A , Coratti G . Revised upper limb module for spinal muscular atrophy: Development of a new module. Muscle & Nerve. (2017) ;55: (6):869–74. |
[9] | Glanzman AM , Mazzone ES , Young SD , Gee R , Rose K , Mayhew A , Nelson L , Yun C , Alexander K , Darras BT , Zolkipli-Cunningham Z . Evaluator training and reliability for SMA global Nusinersen trials. Journal of Neuromuscular Diseases. (2018) ;5: (2):159–66. |
[10] | Youn BY , Ko Y , Moon S , Lee J , Ko SG , Kim JY . Digital biomarkers for neuromuscular disorders: A systematic scoping review. Diagnostics. (2021) ;11: (7):1275. |
[11] | Ray Dorsey E , Spyros Papapetropoulos , Mulin Xiong , Karl Kieburtz The First Frontier: Digital Biomarkers for Neurodegenerative Disorders. Digit Biomark 11; 1: (1):6–13. https://doi.org/10.1159/000477383. |
[12] | Vázquez-Costa JF , Povedano M , Nascimiento-Osorio AE , Moreno Escribano A , Kapetanovic Garcia S , Dominguez R , Exposito JM , González L , Marco C , Medina Castillo J , Muelas N . Validation of motor and functional scales for the evaluation of adult patients with 5q spinal muscular atrophy. European Journal of Neurology. (2022) ;29: (12):3666–75. |
[13] | Cano SJ , Mayhew A , Glanzman AM , Krosschell KJ , Swoboda KJ , Main M , Steffensen BF , Bérard C , Girardot F , Payan CA , Mercuri E . Rasch analysis of clinical outcome measures in spinal muscular atrophy. Muscle & Nerve. (2014) ;49: (3):422–30. |
[14] | Perumal TM , Wolf D , Berchtold D , Pointeau G , Zhang YP , Cheng WY , Lipsmeier F , Sprengel J , Czech C , Chiriboga CA , Lindemann M . Digital measures of respiratory and upper limb function in spinal muscular atrophy: Design, feasibility, reliability, and preliminary validity of a smartphone sensor-based assessment suite. Neuromuscular Disorders. (2023) ;3: (11):845–55. |
[15] | Montalban X , Graves J , Midaglia L , Mulero P , Julian L , Baker M , Schadrack J , Gossens C , Ganzetti M , Scotland A , Lipsmeier F . A smartphone sensor-based digital outcome assessment of multiple sclerosis. Multiple Sclerosis Journal. (2021) ;28: (4):654–64. |
[16] | Lipsmeier F , Taylor KI , Postuma RB , Volkova-Volkmar E , Kilchenmann T , Mollenhauer B , Bamdadian A , Popp WL , Cheng WY , Zhang YP , Wolf D . Reliability and validity of the Roche PD Mobile Application for remote monitoring of early Parkinson’s disease. Scientific Reports. (2022) ;12: (1):12081. |
[17] | Mathiowetz V , Weber K , Kashman N , Volland G . Adult norms for the nine hole peg test of finger dexterity. The Occupational Therapy Journal of Research. (1985) ;5: (1):24–38. |
[18] | Dunaway Young S , Montes J , Kramer SS , Marra J , Salazar R , Cruz R , Chiriboga CA , Garber CE , De Vivo DC . Six-minute walk test is reliable and valid in spinal muscular atrophy. Muscle & Nerve. (2016) ;54: (5):836–42. |
[19] | Woelfle T , Pless S , Reyes O et al. Reliability and acceptance of dreaMS, a software application for people with multiple sclerosis: A feasibility study. Journal of Neurology. (2023) ;270: , 262–71. |
[20] | Scotland A , Cosne G , Juraver A , Karatsidis A , Penalver de Andres J , Bartholomé E , Kanzler C.M , Mazzà C , Roggen D , Hincliffe C , Del Din S , Belachew S DISPEL: A Python Digital Signal Processing Library for Calculation of Sensor Derived Measures from Wearables and Smartphones. IEEE Open Journal of Engineering in Medicine and Biology. (2024) ;494–97. |
[21] | Shrout PE , Fleiss JL . Intraclass correlations: Uses in assessing rater reliability. Psychological Bulletin. (1979) ;86: (2):420. |
[22] | Furlan L , Sterr A . The applicability of standard error of measurement and minimal detectable change to motor learning research— a behavioral study. Frontiers in Human Neuroscience. (2018) ;12: , 95. |
[23] | Magnin E , Sagawa Y , Moulin T , Decavel P . What Are the Minimal Detectable Changes in SDMT and Verbal Fluency Tests for Assessing Changes in Cognitive Performance in Persons with Multiple Sclerosis and Non-Multiple Sclerosis Controls? European Neurology (2020) ;83: (3):263–70. |
[24] | Hinkle DE , Wiersma W , Jurs SG Applied Statistics for the Behavioral Sciences. Houghton Mifflin, Boston 1988. |
[25] | Salort-Campana E , Quijano-Roy S . Clinical features of spinal muscular atrophy (SMA) type 3 (Kugelberg-Welander disease). Archives de Pédiatrie. (2020) ;27: (7):7S23–8. |
[26] | Lipsmeier F , Taylor KI , Kilchenmann T , Wolf D , Scotland A , Schjodt-Eriksen J , Cheng WY , Fernandez-Garcia I , Siebourg-Polster J , Jin L , Soto J . Evaluation of smartphone-based testing to generate exploratory outcome measures in a phase 1 Parkinson’s disease clinical trial. Movement Disorders. (2018) ;33: (8):1287–97. |
[27] | Midaglia L , Mulero P , Montalban X , Graves J , Hauser SL , Julian L , Baker M , Schadrack J , Gossens C , Scotland A , Lipsmeier F . Adherence and satisfaction of smartphone-and smartwatch-based remote active testing and passive monitoring in people with multiple sclerosis: Nonrandomized interventional feasibility study. Journal of Medical Internet Research. (2019) ;21: (8):e14863. |
[28] | Creagh AP , Simillion C , Scotland A , Lipsmeier F , Bernasconi C , Belachew S , Van Beek J , Baker M , Gossens C , Lindemann M , De Vos M . Smartphone-based remote assessment of upper extremity function for multiple sclerosis using the Draw a Shape Test. Physiological Measurement. (2020) ;41: (5):054002. |
[29] | Schuck P , Zwingmann C . The ‘smallest real difference’as a measure of sensitivity to change: A critical analysis. International Journal of Rehabilitation Research. (2003) ;26: (2):85–91. |
[30] | Lu W-S , Chen CC , Huang S-L , Hsieh C-L . Smallest real difference of 2 instrumental activities of daily living measures in patients with chronic stroke. Archives of Physical Medicine and Rehabilitation. (2012) ;93: (6):1097–100. |
[31] | Kanzler CM , Rinderknecht MD , Schwarz A , Lamers I , Gagnon C , Held JP , Feys P , Luft AR , Gassert R , Lambercy O . A data-driven framework for selecting and validating digital health metrics: Use-case in neurological sensorimotor impairments. NPJ Digital Medicine. (2020) ;3: (1):80. |
[32] | Holm SP , Wolfer AM , Pointeau GH , Lipsmeier F , Lindemann M . Practice effects in performance outcome measures in patients living with neurologic disorders– A systematic review. Heliyon. (2022) ;8: (8):e10259. |
[33] | Mix L , Schreiber-Katz O , Wurster CD , Uzelac Z , Platen S , Gipperich C , Ranxha G , Wieselmann G , Osmanovic A , Ludolph AC , Petri S . Executive function is inversely correlated with physical function: The cognitive profile of adult Spinal Muscular Atrophy (SMA). Orphanet Journal of Rare Diseases. (2021) ;16: , 1–9. |
[34] | Lam KH , Twose J , Lissenberg-Witte B , Licitra G , Meijer K , Uitdehaag B , De Groot V , Killestein J . The use of smartphone keystroke dynamics to passively monitor upper limb and cognitive function in multiple sclerosis: Longitudinal analysis. Journal of Medical Internet Research. (2022) ;24: (11):e37614. |