The Black Box of Technological Outcome Measures: An Example in Duchenne Muscular Dystrophy
Abstract
Background:
Outcome measures for non-ambulant Duchenne muscular dystrophy (DMD) patients are limited, with only the Performance of the Upper Limb (PUL) approved as endpoint for clinical trials.
Objective:
We assessed four outcome measures based on devices developed for the gaming industry, aiming to overcome disadvantages of observer-dependency and motivation.
Methods:
Twenty-two non-ambulant DMD patients (range 8.6–24.1 years) and 14 healthy controls (HC; range 9.5–25.4 years) were studied at baseline and 16 patients at 12 months using Leap Motion to quantify wrist/hand active range of motion (aROM) and a Kinect sensor for reached volume with Ability Captured Through Interactive Video Evaluation (ACTIVE), Functional Workspace (FWS) summed distance to seven upper extremity body points, and trunk compensation (KinectTC). PUL 2.0 was performed in patients only. A stepwise approach assessed quality control, construct validity, reliability, concurrent validity, longitudinal change and patient perception.
Results:
Leap Motion aROM distinguished patients and HCs for supination, radial deviation and wrist flexion (range p = 0.006 to <0.001). Reliability was low and the manufacturer’s hand model did not match the sensor’s depth images. ACTIVE differed between patients and HCs (p < 0.001), correlated with PUL (rho = 0.76), and decreased over time (p = 0.030) with a standardized response mean (SRM) of –0.61. It was appraised as fun on a 10-point numeric rating scale (median 9/10). PUL decreased over time (p < 0.001) with an SRM of –1.28, and was appraised as fun (median 7/10). FWS summed distance distinguished patients and HCs (p < 0.001), but reliability in patients was insufficient. KinectTC differed between patients and HCs (p < 0.01), but correlated insufficiently with PUL (rho = –0.69).
Conclusions:
Only ACTIVE qualified as potential outcome measure in non-ambulant DMD patients, although the SRM was below the commonly used threshold of 0.8. Lack of insight in technological constraints due to intellectual property and software updates made the technology behind these outcome measures a kind of black box that could jeopardize long-term use in clinical development.
INTRODUCTION
Duchenne muscular dystrophy (DMD) is typically characterized by progressive muscle weakness in a proximal to distal gradient [1]. Independent ambulation is generally lost years before upper arm function [2]. The progressive impairment of arm function causes difficulties in performing daily-life activities [3]. The first drugs for ambulant DMD patients have received conditional approval, but results on efficacy cannot be extrapolated to later disease stages due to progressive and irreversible reduction in targeted muscle tissue [4, 5]. Therefore, separate trials with dedicated outcome measures for non-ambulant patients need to be performed.
The Performance of the Upper Limb (PUL) 2.0 scale is currently the only outcome measure that is accepted as primary endpoint for non-ambulant DMD patients (e.g. NCT03406780 and NCT04371666) [6]. However, the PUL has its limitations: it requires a clinical assessment, is observer-dependent, and has a floor and ceiling effect [6, 7]. Commercial technology with motion-tracking capabilities developed for gaming are being explored as new outcome measures for clinical trials in non-ambulant DMD [8, 9]. They potentially enable measurements at home, are observer-independent, and may overcome disadvantages of ordinal scales, such as the use of non-linear statistics. Furthermore, if the assessment can be performed in the form of a game, this could overcome variability due to lack of motivation in patients and lead to quantification of motions that are closer to the activities of daily living.
To address the lack of outcome measures in non-ambulant DMD, we evaluated several assessment methods based on off-the-shelf motion tracking technologies that could provide easy-to-use and affordable assessments in clinic. We chose Leap Motion which features marker-less hand tracking and Microsoft Kinect v2 which includes full-body tracking capabilities. The Leap Motion was used previously to assess the active range of motion (aROM) of the wrist and hand in healthy controls (HCs). The study revealed high test-retest reliability, but also issues of occlusions with some of the finger joints [10, 11]. Leap Motion aROM has not yet been evaluated as an outcome measure in neuromuscular disorders. For the Kinect v2 sensor, we evaluated three assessment protocols: “Ability Captured Through Interactive Video Evaluation” (ACTIVE) [12], Functional Workspace (FWS) [13], and Kinect Trunk Compensation (KinectTC) [14]. ACTIVE was developed as an outcome measure for neuromuscular diseases through the assessment of the reaching ability of the arm, summarized as a volume of reach during performance of a game activity. ACTIVE was shown previously to be responsive to treatment with nusinersen in spinal muscular atrophy (SMA) patients, and demonstrated excellent test-retest reliability in DMD [9, 12]. However, change over time has not yet been evaluated in DMD. In the FWS protocol, the Kinect is used to assess the ability to touch seven upper extremity body points via a guided video. The methodology for the analysis of the motion has been validated with a standard marker-based motion capture in HCs [13]. The FWS assessment has not been studied previously in DMD patient population. Finally, the KinectTC protocol was applied to assess the participants’ trunk compensation during repeated task performance. Patients with neuromuscular weakness often use their trunk to compensate for the loss of muscle strength in the upper extremity. In this study, DMD participants performed ten hand-to-mouth movements while being tracked by Kinect to quantify trunk compensation. In summary, we assessed the feasibility of Leap Motion aROM of the wrist and hand, ACTIVE, FWS and KinectTC, as outcome measures in non-ambulant DMD patients.
MATERIALS AND METHODS
Participants
Participants were included between March 2018 and July 2019 in a longitudinal study conducted at Leiden University Medical Center (LUMC; ABR number NL63133.058.17, https://www.toetsingonline.nl). For patients, visits were scheduled at baseline, 12 and 18 months which lasted about four hours each as approved by the medical ethical board. One half day visit was scheduled at baseline for HCs. Due to unforeseen restrictions during the COVID-19 pandemic, the 12 months follow-up visit could only take place for 16 patients and the 18 months follow-up visit for 12. Therefore, we report results from baseline (DMD and HC) and 12 months follow-up (DMD). DMD patients were recruited from the Dutch Dystrophinopathy Database [15], and via outpatient clinics and patient organizations. Inclusion criteria were male, non-ambulant, genetically confirmed DMD, aged ≥8 years. Exclusion criteria were exposure to an investigational drug ≤6 months prior to participation and recent (≤6 months) upper extremity surgery or trauma. As the study protocol included muscle MRI, patients with MRI contra-indications (e.g. spinal fusion, daytime respiratory support, or the inability to lie still for 45 minutes) were also excluded. Healthy age-matched controls were recruited using posters and advertisements in local media. The study was approved by the local medical ethics committee in accordance with the ethical standards laid down in the 1975 Declaration of Helsinki and its later amendments. Written informed consent had been obtained from all patients and from legal representatives for patients under 16 years of age. Patient inclusion in this study has been reported previously [16].
Measurements and data analysis
All measurements were performed seated behind a height-adjustable table. DMD patients sat in their own wheelchair and HCs sat on a chair with a backrest and armrests. All unilateral assessments were performed only with the right hand or arm. Height was calculated from the ulna length for both DMD patients and HCs with 18 as maximum age to create scaled scores for some of the outcome measures [17]. Patients performed assessment in the following order at every visit: ACTIVE, FWS, KinectTC, Leap Motion aROM and PUL.
Leap motion active range of motion
A Leap Motion (Leap Motion Inc., San Francisco, USA) measurement setup was adjusted from Nizamis et al. [10], with the sensor oriented downward or from the side, instead of upward (Fig. 1A). This enabled un-occluded tracking of primarily the dorsal side of the hands, and allowed patients to rest their hands on the setup during movement recording. Maximum active range of motion (aROM) was assessed using the Leap Motion in two separate trials of at least three repetitions for the following five movements of the right arm: flexion/extension of the finger joints, thumb abduction/adduction, radial/ulnar deviation, pronation/supination, and wrist flexion/extension. Trials without at least one complete movement were excluded.
Fig. 1
Leap Motion active range of motion (aROM) setup, construct validity and reliability. In (A) the Leap Motion measurement setup is shown with the Leap Motion sensor oriented downward or from the side by using an aluminum frame with mat black wooden shielding. Construct validity and reliability of supination are shown in (B) and (C) respectively. Supination showed the largest difference between Duchenne muscular dystrophy (DMD) patients and healthy controls (HC) and thus the best construct validity. The Bland-Altman plot with mean bias (straight lines) and 95%-confidence intervals (dotted lines) shows that reliability is low for both HCs (round) and patients (square). Average of the two trials is plotted on the x-axis and difference between the trials on the y-axis.
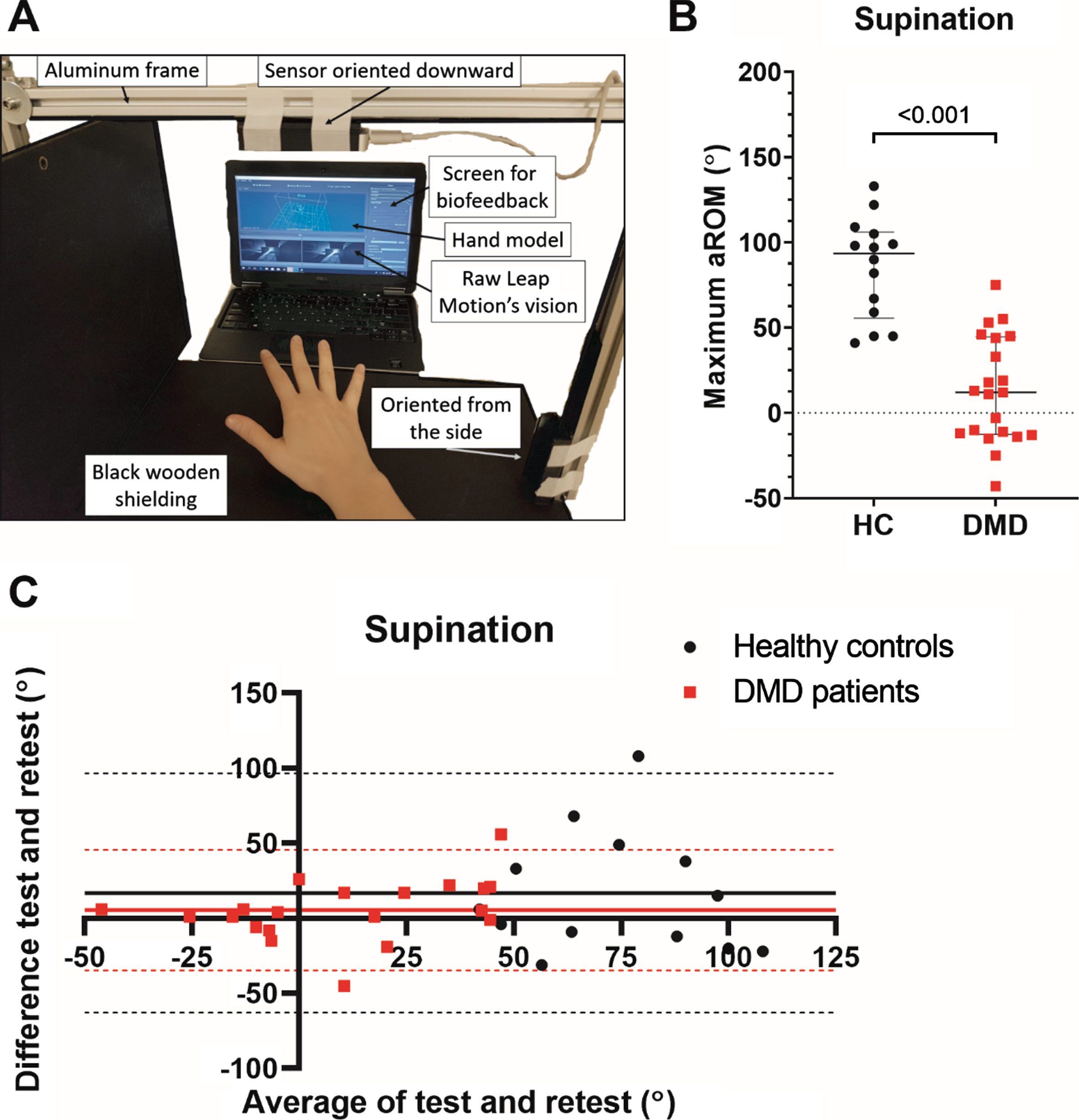
The Leap Motion operates based on infra-red stereoscopy, consisting of two infrared cameras that capture motion with 200 frames per second and three infra-red LEDs that provide the illumination. Based on the reconstructed depth image, the Leap Motion software development kit (SDK) provides a skeletal model of the hand with the lower arm. In this study, Orion Beta v3.2.1 SDK was used to extract the internal hand model. Movements of the elbow, wrist, finger and thumb points were recorded using Brekel Pro Hands software, version 1.35 (Brekel, Amsterdam, The Netherlands). The maximum aROM was determined for both extremes of the five movements by calculating the raw joint angles using custom-made software written in MATLAB (MATLAB R2016a, The MathWorks, Inc., Natick, USA). Screen recordings were captured to compare the raw Leap Motion’s depth images with the provided hand model.
ACTIVE scaled volume
The reached volume of the arms was determined using the ACTIVE game (software version 2017) and a Microsoft Kinect v2 sensor (Microsoft Corp., Redmond, Washington, USA) mounted at a height of 1.80m and 2.95m in front of the participant. In this game, participants are virtually situated in a cave where they are stimulated to gather as many diamonds as possible. More diamonds can be collected when participants reach further upwards, sideways and forward with their arms and trunk as described previously (Fig. 2A) [9]. The maximal volume out of three ACTIVE trials of 60 seconds was used. If the last trial yielded the largest volume, a fourth trial was added to the protocol, assuming that the patient had not yet reached his maximum potential. The volume was normalized to create a Scaled Volume score using the participant’s calculated height and the reached volume (Fig. 2B) [9, 12].
Fig. 2
Ability Captured Through Interactive Video Evaluation (ACTIVE) setup and construct validity. In (A) the ACTIVE avatar is shown while the participant is pushing away the walls on the left on the three levels: upper, middle, and lower level. In (B) the reached width and height for the three levels are shown for a healthy control (HC) and Duchenne muscular dystrophy (DMD) patient. In (C) the percentage of participants who reached the largest volume in that trial is presented. The highest result was reached in the third and fourth trials in seven HCs (50%) and ten DMD patients (45%). In (D) the Scaled Volume is shown to be higher for HCs compared to DMD patients (p < 0.001).
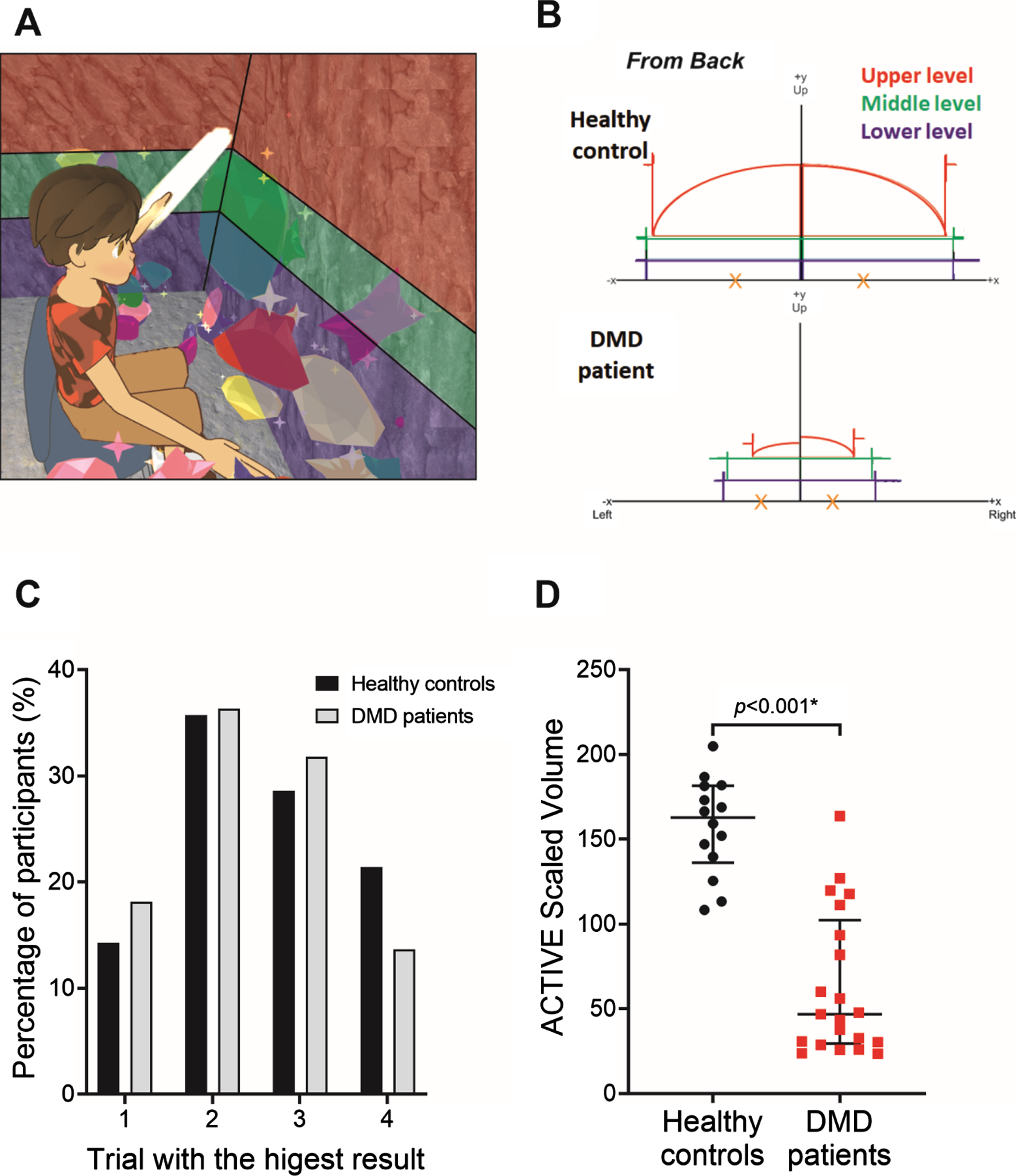
Functional workspace summed distance
The same Kinect sensor was used to assess the FWS. FWS determines the ability to reach different targets close to the body with the hand (simulating the motions of some common activities of daily living). Custom software was developed at University of California to collect the Kinect skeletal motion data [13]. During each FWS trial, participants were asked to reach with their right hand towards seven upper extremity targets: belt buckle or stomach, back pocket, ipsilateral shoulder, contralateral shoulder, mouth, top of head, and back of head (Fig. 3A). Patients were instructed not to use their trunk to assist with their motion. The rigid body model was utilized to define the position for each of the seven landmark targets as described previously [13]. A second order, 1 Hz low-pass Butterworth filter was used to smooth the estimated distance timeseries. Since the tracking of the fingertips by the Kinect is relatively unreliable, the wrist trajectory was used to calculate the Euclidean distance to the expected wrist position at each target location. Based on the target sequence provided by the video instructions and the duration of the hand at each landmark, the minimal distance was extracted for each landmark. The distance was normalized by the individual’s hand length to obtain the relative distance measure by using a ratio between hand length and ulna length that has been described for different ages [18]. All the data processing was performed in MATLAB (version R2016a). The summed FWS distance was calculated as the sum of the number of hand lengths distance to the seven targets, where higher scores indicated larger distances from the target.
Fig. 3
Functional Workspace (FWS) summed distance setup, construct validity and reliability. In (A) the seven upper extremity targets of the FWS are shown and alongside this a typical movement pattern of the right wrist during the FWS is presented in red. Construct validity and reliability of the summed hand length distance to these seven targets are shown in (B) and (C) respectively. This summed distance differed significantly between Duchenne muscular dystrophy (DMD) patients and healthy controls (HC; p < 0.001). The Bland-Altman plot with mean bias (straight lines) and 95%-confidence intervals (dotted lines) shows that reliability is high for HCs (round), but much lower for patients (square). Average of the two trials is plotted on the x-axis and difference between the trials on the y-axis.
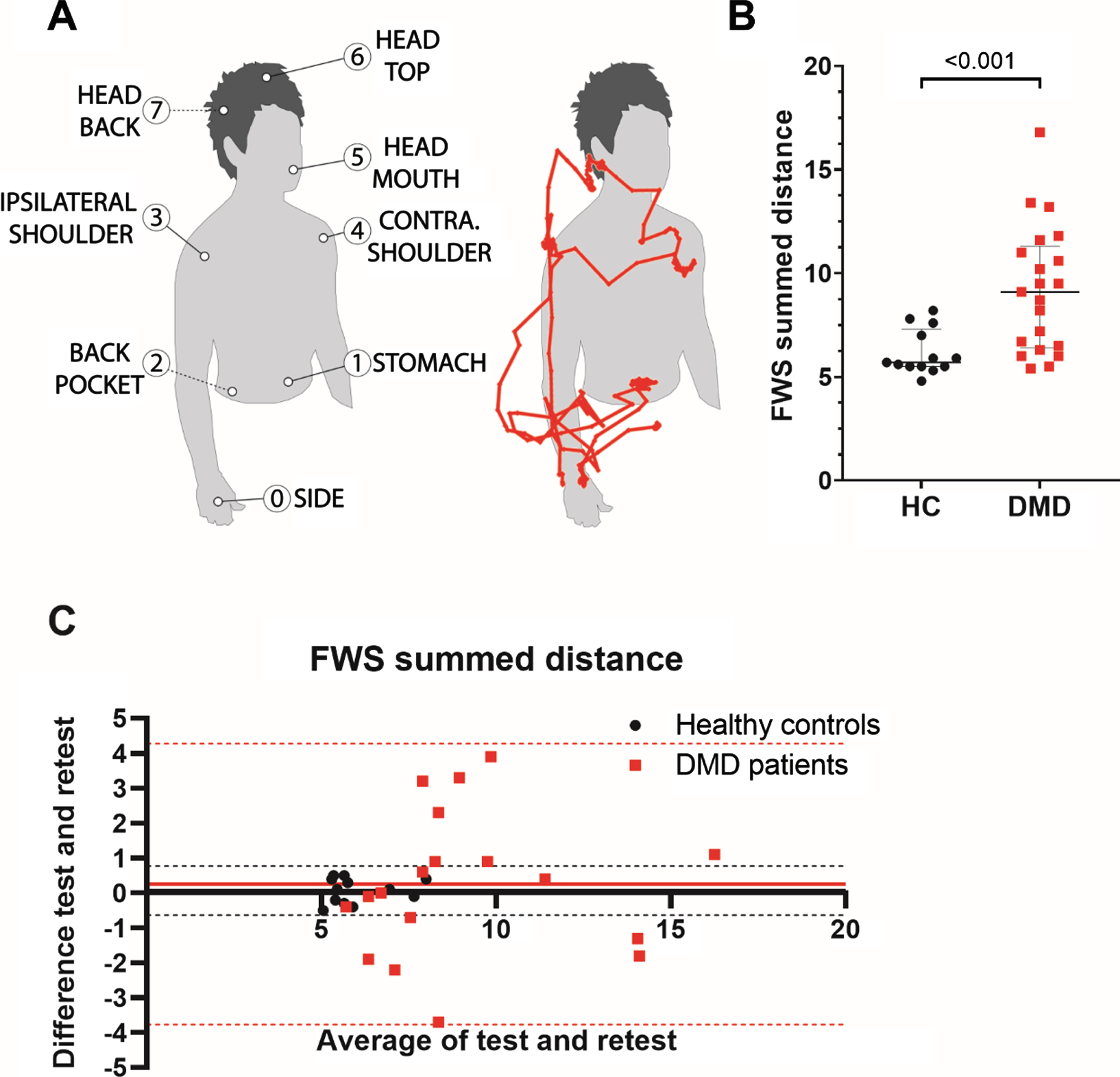
Kinect trunk compensation
KinectTC was assessed by quantifying the participants’ trunk compensation during repeated task performance with the upper extremity using the same Kinect sensor. Participants performed ten hand-to-mouth movements using only their right hand whilst holding a 200g cup, similar to the PUL 2.0 hand-to-mouth item. In accordance with this PUL item, participants were instructed to use as little compensation as possible: sitting straight, keeping trunk and head still and primarily using the elbow flexion muscles. As a gold standard, the trunk compensation (i.e. flexing, lateral flexing or extending) during the movement was visually assessed by a single observer (K.J.N.) and scored as present or absent. Kinect depth data was related to a model of body points by Microsoft Kinect SDK 2.0 software. Movements of wrist, elbow, shoulder, head, and spine body points were recorded using customized Unity3D software (version 5.6.0, Unity Technologies, San Francisco, USA). Trunk distance was defined as the total distance covered by the Kinect ‘spine shoulder’ point, which was quantified for all hand-to-mouth movement cycles using custom-made software in MATLAB (version R2016a; Fig. 4A). Data were resampled to a uniformly distributed time series (30Hz), a 5Hz filter (fourth-order bidirectional Butterworth) was applied, and hand-to-mouth movement cycles were detected based on the distance between the wrist and head point. Using a 3D model of the body points, onset and/or offset of detected cycles was corrected manually, if necessary. Cycles without a dip in head-wrist distance and those with artefacts in the movements of the spine shoulder point were excluded. Trunk distance per cycle in mm was divided by the participant’s calculated height in m to yield trunk compensation as a ratio to height. The KinectTC outcome was calculated as the average of at least five complete movement cycles without artefacts.
Fig. 4
Kinect Trunk Compensation (KinectTC) setup, construct validity and reliability. Recorded spine shoulder, head point, wrist point and other body points for the hand-to-mouth movement of a healthy control (HC) and a Duchenne muscular dystrophy (DMD) patient with trunk compensation are shown in (A). KinectTC (trunk compensation in mm as a ratio to height in m) is shown in (B) to be significantly higher for DMD patients compared to HCs (p < 0.01), and in (C) for DMD patients with visually scored trunk compensation compared to patients without visually scored compensation (p < 0.001). In (D) the within subject SD is shown for HCs and DMD patients. The average within subject SD is 36 for patients and 14 for HCs.
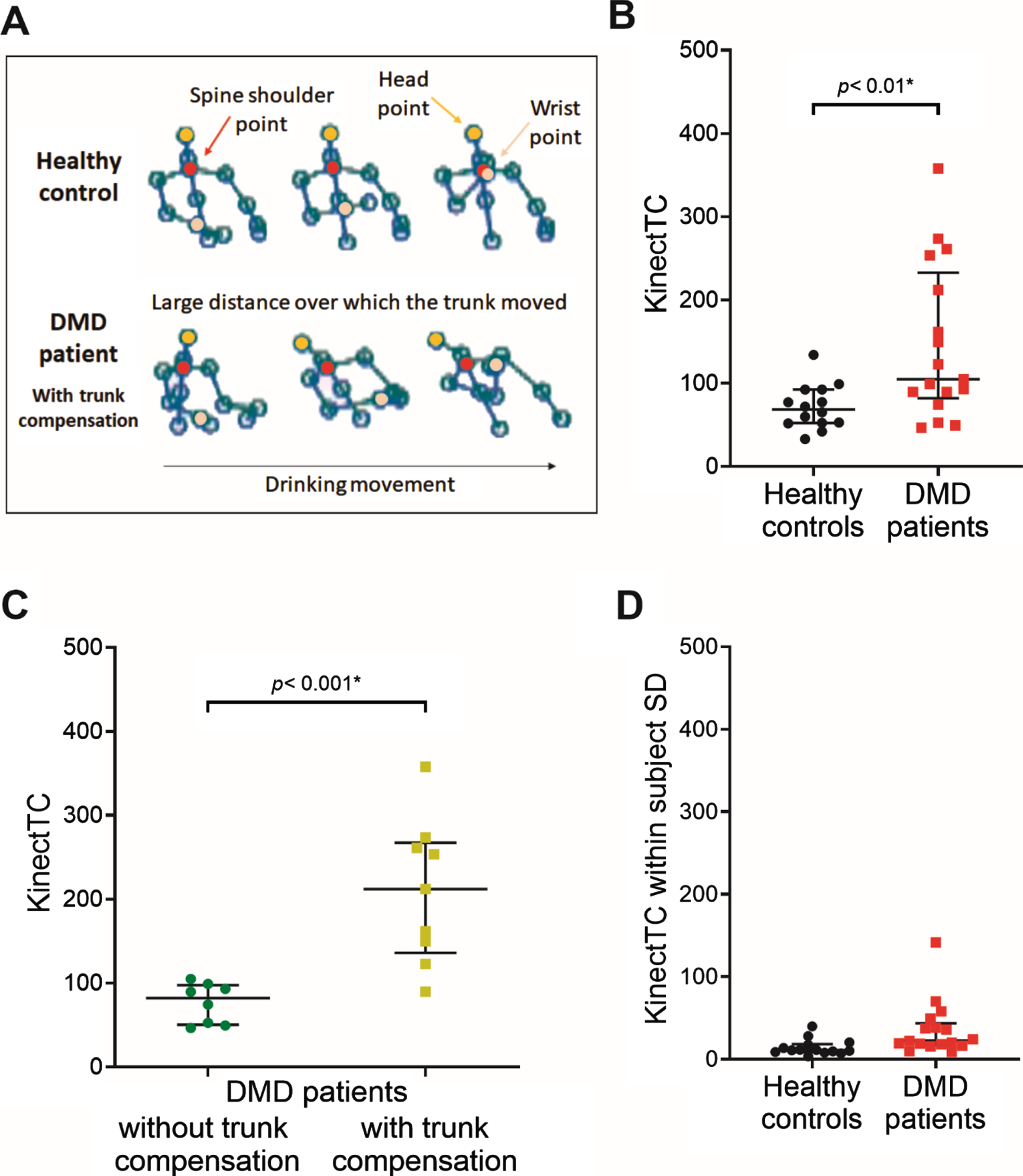
Performance of the upper limb
PUL 2.0 was assessed in DMD patients only. PUL 2.0 was performed for the right arm and consists of 22 items that are divided into a shoulder (12 points), elbow (17 points) and distal wrist/hand dimension (13 points), yielding a maximum total score of 42 points [19].
Patient perception
After the Leap Motion, ACTIVE, FWS and PUL, patients rated these assessments in the categories fun, annoying and tiring. This was done using 10-point numeric rating scales (NRS) ranging from ‘not at all fun/annoying/tiring’ (score 0) to ‘a lot of fun/very annoying/tiring’ (score 10) with matching facial cartoons based on the Wong-Baker Faces Rating Scale [20].
Statistical analysis and stepwise approach
Leap, ACTIVE, FWS and KinectTC were evaluated in a stepwise approach that first assessed critical requirements for any outcome measure: quality control, construct validity and reliability. If results for this first step were of sufficient quality, the next steps consisted of [2] concurrent validity, [3] longitudinal change, and [4] patient perception. A flowchart of the stepwise approach for all four outcome measures is shown in Fig. 5. Results are described as median (interquartile range (IQR) 1st quartile to 3rd quartile), unless otherwise stated.
Fig. 5
Flowchart of the stepwise approach for all four outcome measures. Leap, ACTIVE, FWS and KinectTC were assessed in a stepwise approach that first tested quality control, construct validity and reliability. If results for this step were of sufficient quality, the next steps consisted of: concurrent validity, longitudinal change and patient perception. Leap did not perform well enough on quality control and reliability and FWS on reliability, so these two measures did not continue after the first step. KinectTC did not have a strong relation with PUL and did not continue further. ACTIVE was analyzed according to the entire stepwise approach.
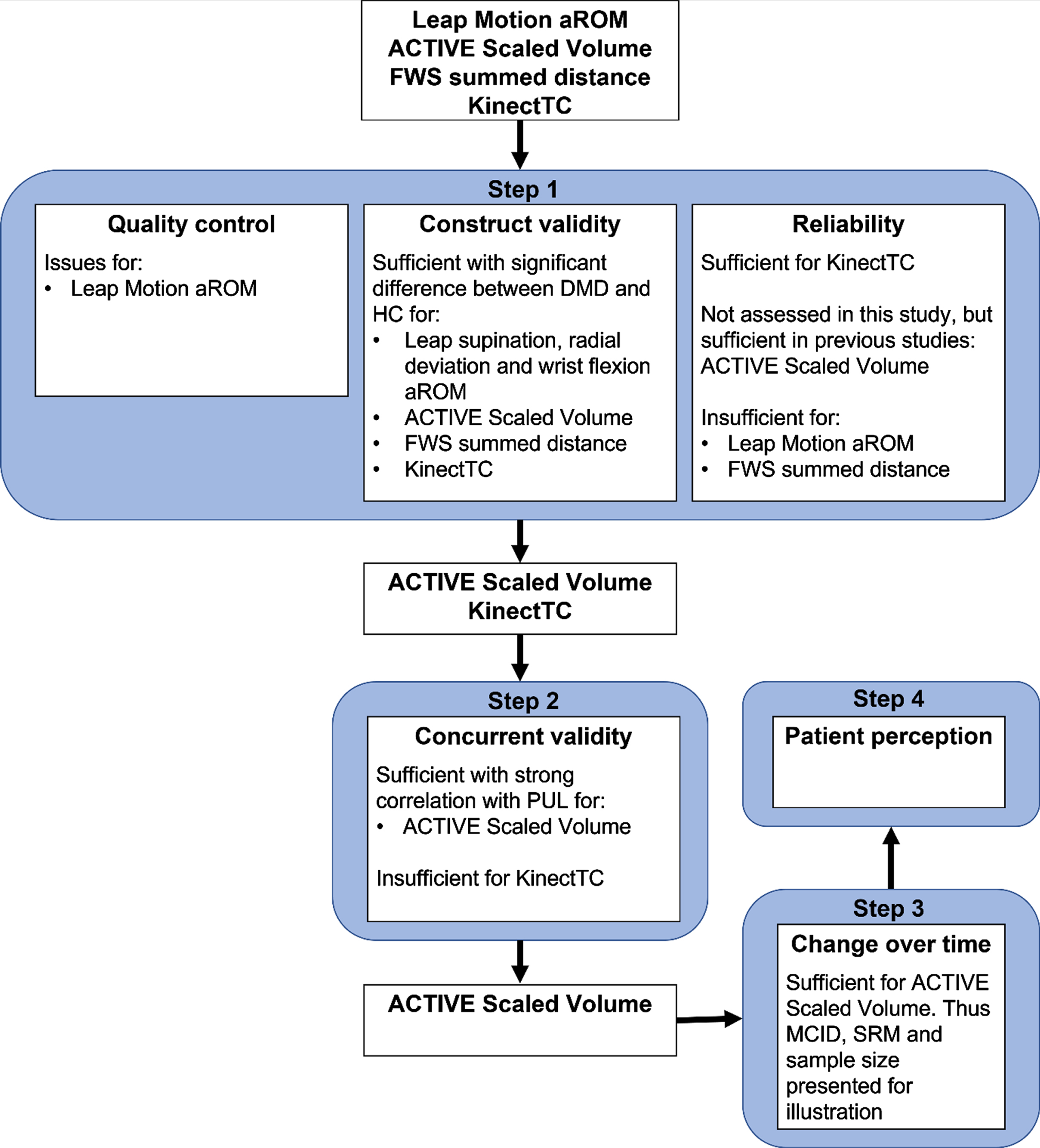
In the first step, quality control consisted of describing excluded data and evaluating screen recordings for Leap Motion aROM for anatomical inconsistencies by visually comparing the simultaneously recorded raw Leap Motion’s depth images and manufacturer’s hand model. In case serious quality issues were encountered in part of the assessment, it could be decided to continue the stepwise approach with a specific part of the assessment for which consistent data were available. For ACTIVE, FWS and KinectTC, a comparison with screen recordings was not possible, because the depth images of the Kinect could not be obtained. Next, the construct validity criterion was tested. This was passed if outcomes differed significantly between patients and HCs, and for KinectTC between patients with and without visible trunk compensation using Mann-Whitney U tests. Statistical significance was set at p < 0.05. Bonferroni-Holm correction was used to correct for multiple comparisons within the aROM assessments. Finally, reliability was assessed using a Bland-Altman analysis to determine mean bias and 95%-confidence interval (CI) of test-retest assessments. Test-retest data were available for Leap Motion aROM and FWS, which was deemed reliable if the 95%-CI in patients did not exceed the difference between patients and HCs. No test-retest data was available for ACTIVE and KinectTC, but reliability of ACTIVE has been determined previously [9]. KinectTC was deemed reliable, if the 95%-CI of the within subject standard deviation (SD) of movement cycles for patients was smaller than the difference between patients with and without trunk compensation.
In the second step, concurrent validity was determined via the correlation of the outcome measures with PUL 2.0 total score using Spearman correlation coefficient. The correlation with PUL should be strong (rho ≥0.7) [21].
In the third step, change over time was satisfactory if outcomes showed significant change over 12 months as assessed by the Wilcoxon signed-ranks test. If the change was significant, the size of the change was illustrated using the minimally clinically important difference (MCID), standardized response mean (SRM) and corresponding sample size. MCID was determined via a distribution-based method using one-third of the SD of the baseline values. The SRM was calculated as the mean change over 12 months/SD of that change and should exceed 0.8 [22]. Corresponding sample sizes for a potential clinical trial with the measurement as primary outcome measure were calculated using Lehr’s formula [23]. In this calculation, we assumed a treatment effect of 50%reduction in disease progression over 12 months with a power of 80%and α< 0.05 in a 1:1 randomization. For comparison, all change over time values were also determined for PUL.
For the fourth and final step, patient perception was determined. Patient perception was assessed using NRS fun, annoying, and tiring scores of the three outcomes. These were compared to those of the PUL using Wilcoxon signed-ranks test.
RESULTS
Participants
Twenty-two DMD patients and 14 HCs were included in the study. Baseline characteristics and results from all outcome measures are presented in Table 1. One patient with autism spectrum disorder was only able to perform the ACTIVE and PUL assessments. Four patients were unable to perform the described hand-to-mouth movement at baseline, having lost the ability at a median age of 14.5 years (range 8.9–18.2 years). Baseline median PUL total score was 21 points (IQR 19 to 34; Table 1). All patients used glucocorticoids in an intermittent schedule, except one patient who used daily deflazacort. One patient had ceased glucocorticoid treatment six weeks prior to baseline for a total of six months due to weight gain. Ambulation was lost median 2.5 years before baseline visit (range 0.6–5.8 years), at a median age of 11.5 years (range 8.0–18.9 years). Median age at start steroid use was 5.6 years (range 2.5–9.6 years).
Table 1
Baseline characteristics and construct validity results from all outcome measures for HCs and DMD patients
Healthy controls n = 14 | DMD patients n = 22 | p-value | |||
Age, years | 15.2 (11.5;20.6) | 13.4 (12.3;16.2) | 0.413 | ||
Height, m | 1.74 (1.49;1.76) | 1.52 (1.45;1.66) | 0.016* | ||
Body mass index | 18.7 (16.6;22.2) | 27.4 (23.6;30.8) | <0.001* | ||
Leap Motion aROM | |||||
Pronation, ° | 92 (84;112) | 89 (82;96) | n = 21 | 0.400 | |
Supination, ° | 94 (56;106) | 12 (–13;45) | n = 21 | <0.001* | |
Radial deviation, ° | 20 (14;28) | 10 (6;19) | n = 21 | 0.002* | |
Ulnar deviation, ° | 42 (36;48) | 46 (39;49) | n = 21 | 0.576 | |
Wrist flexion, ° | 60 (49;64) | 48 (31;53) | n = 21 | 0.006* | |
Wrist extension, ° | 55 (45;64) | 38 (22;53) | n = 21 | 0.043 | |
ACTIVE Scaled Volume, points | 163 (136;182) | 47 (30;102) | n = 21 | <0.001* | |
FWS summed distance, hand lengths | 5.7 (5.5;7.3) | n = 13 | 9.1 (6.4;11.3) | n = 21 | <0.001* |
KinectTC | 68 (52;92) | 105 (82;233) | n = 17 | <0.01* | |
PUL 2.0 total score, points | 21 (19;34) |
Median (1st quartile; 3rd quartile). Differences between patients and HCs were assessed using Mann-Whitney U tests. Statistical significance was set at p < 0.05 and is shown by *, for Leap Motion aROMs this is after Bonferroni-Holm correction and for clarity uncorrected p-values are reported. If a certain value was not available for all patients, the number of patients for whom the data was available was presented after the result with n = number. Abbreviations: HC = healthy control, DMD = Duchenne muscular dystrophy, aROM = active range of motion, ACTIVE = Ability Captured Through Interactive Video Evaluation, FWS = Functional Workspace, KinectTC = Kinect Trunk Compensation (trunk compensation in mm as a ratio to height in m), PUL = Performance of the Upper Limb.
Leap motion active range of motion
Regarding quality control, thumb movements in only the abduction/adduction plane should have led to recorded movements in only one axis, but instead showed unexplainable movements in three axis in all participants. Therefore, these were excluded from further analysis. All Leap Motion test trials, and 98%(123/126) of retest trials in patients and 99%(83/84) in HCs contained a complete movement and were thus included in the analyses. No screen recordings had been captured for the first patient and five HCs. The metacarpophalangeal joints should show maximum flexion angles of about 90 degrees for all fingers [10], but in our data the maximum flexion angles decreased from about 90 degrees for the index finger to about 70 degrees for the little finger in both patients and HCs (Fig. S1). Due to these structural problems that could be caused by occlusion of the finger joints [10], we continued the stepwise approach only for the wrist aROMs. Screen recordings revealed that in some patients the forearm position as recorded by the Leap did not match the position as seen in the screen recordings, which led to incorrect wrist flexion and extension values (Fig. S2A). For supination, some patients moved similarly on the screen recordings in both trials, while test and retest values differed as much as 68 degrees due to different estimation of the elbow position (Fig. S2B). These examples suggest that aROM values can be inconsistent while the data recordings show three complete movements that visually appear normal. Unfortunately, there was no quantitative method to filter out these incorrect recordings.
Regarding construct validity, significant differences between patients and HCs were found for supination, radial deviation and wrist flexion (Table 1 and Fig. 1B), but not for pronation, ulnar deviation and wrist extension.
Regarding reliability, radial deviation showed the smallest mean bias (0 degrees) and 95%-CI (–12 to 12 degrees) in patients, followed by wrist flexion (bias –5 degrees; 95%-CI –30 to 20 degrees) and supination (bias 5 degrees; 95%-CI –35 to 46 degrees). The 95%-CI of radial deviation and wrist flexion exceeded the difference between patients and HCs, while supination had only a slightly smaller 95%-CI. In HCs, radial deviation also showed the smallest mean bias (1 degrees) and 95%-CI (–15 to 16). The Bland-Altman plot of supination is presented in Fig. 1C, because this aROM showed the largest difference between patients and HCs. The stepwise approach for Leap Motion aROM was not continued after the first step (Fig. 5), because quality control showed unresolvable measurement problems and reliability was insufficient with a 95%-CIs that exceeded the differences between patients and HCs.
ACTIVE scaled volume
Regarding quality control, we included 96%(70/73) of ACTIVE trials, because all three trials of one patient’s baseline visit had to be excluded due to a measurement error. The largest Scaled Volume was achieved in the third or fourth trial in 45%of DMD patients and 50%of HCs (Fig. 2C). One patient did not want to perform more than one trial.
Regarding construct validity, ACTIVE Scaled Volume differed significantly between patients and HCs (Table 1 and Fig. 2D).
Regarding concurrent validity, the correlation of ACTIVE Scaled Volume with PUL total was strong (rho = 0.76; Table 2, Fig. 6A).
Table 2
Concurrent validity, change over time and patient perception results
DMD patients, n = 22 | ||||||||
Correlation | 12-months | MCID | SRM | Sample | NRS fun | NRS tiring | NRS annoying | |
with PUL | change | size per | score | score | score | |||
n = 16 | study arm | n = 21 | n = 21 | n = 21 | ||||
ACTIVE Scaled | 0.76 (0.47 to 0.90) | –5.6 (–23.4 to 1.3) n = 15 | 14.1 | –0.61 | 169 | 9 (7–10) | 6 (4–7) | 2 (0–5) |
Volume, points | ||||||||
KinectTC | –0.69 (–0.88 to –0.29) | – | – | – | – | – | – | – |
PUL 2.0 total | – | –3.0 (–3.8 to –2.0) | 2.9 | –1.28 | 39 | 7 (5–10) | 3 (2–4) | 1 (0–2) |
score, points |
Correlation values are shown as rho (95%-confidence interval), and 12-months change and NRS scores as median (first-third quartiles). Abbreviations: DMD = Duchenne muscular dystrophy, PUL = Performance of the Upper Limb, MCID = minimally clinically important difference, SRM = standardized response mean, NRS = Numeric Rating Scale, ACTIVE = Ability Captured Through Interactive Video Evaluation, KinectTC = Kinect Trunk Compensation (trunk compensation in mm as a ratio to height in m).
Fig. 6
ACTIVE and KinectTC change over time and relation with function tests. ACTIVE Scaled Volume plotted for baseline and 12 months follow-up of Duchenne muscular dystrophy (DMD) patients (red) against Performance of the Upper Limb (PUL) 2.0 total score in (A). Correlation was strong with PUL 2.0 (rho = 0.76). ACTIVE Scaled Volume did decrease significantly over 12 months. KinectTC scaled trunk distance (trunk compensation in mm as a ratio to height in m) is plotted for baseline and 12 months follow-up of DMD patients against PUL 2.0 total score in (B). Correlation was moderate with PUL 2.0 (rho = –0.69).
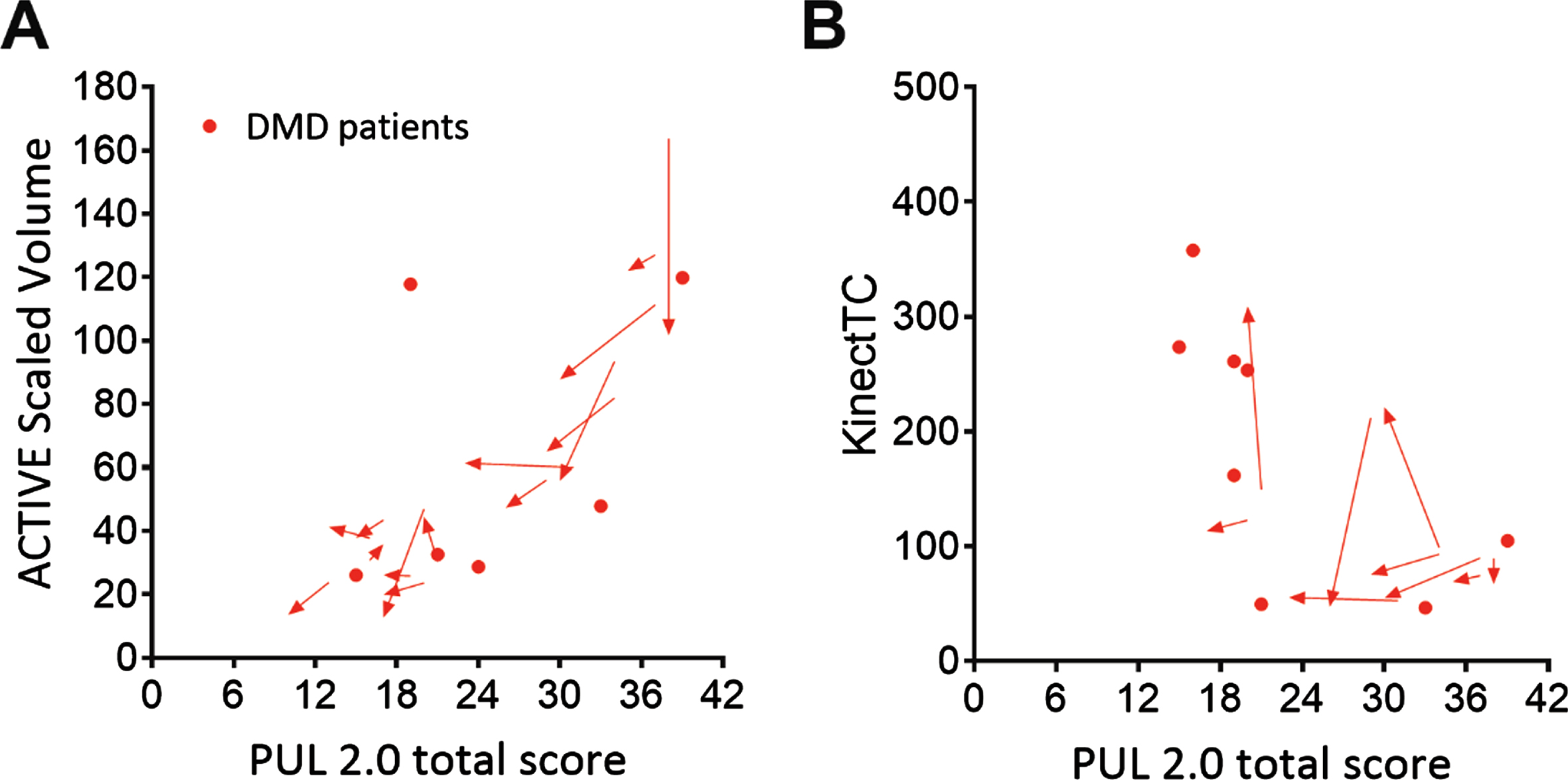
Regarding change over time, ACTIVE Scaled Volume showed a decrease of median 5.6 points over 12 months (IQR –23.4 to 1.3; p = 0.030; n = 15), from median 47 (IQR 30 to 102) to median 44 (IQR 29 to 64). MCID for patients was 14.1 points for Scaled Volume (Table 2). The change in Scaled Volume exceeded the MCID in five out of 15 patients and the resulting SRM was –0.61, with a sample size of 169. PUL total changed from median 21 points (IQR 19 to 34) at baseline to median 19 (IQR 17 to 30) at 12 months, and this decrease was significant (–3.0 (IQR –3.8 to –2.0), p < 0.001). The MCID for PUL total was 2.9 points, and the change exceeded the MCID in nine out of 16 patients. The SRM was –1.28 and corresponding sample size 39 (Table 2).
Regarding patient perception, ACTIVE was reported to be a lot of fun (median 9) and a little tiring (median 6), but not annoying (median 2). In comparison, patients appraised PUL 2.0 to be fun (median 7), not really tiring (median 3) and not at all annoying (median 1). Only NRS tiring scores differed significantly between ACTIVE and PUL (p = 0.002), where ACTIVE was more tiring. All NRS results can be found in Table 2.
Functional workspace summed distance
Regarding quality control, FWS test and retest trials were captured for 93%(14/15) of HCs. For patients, a test trial was available for 100%(21/21) and retest trial for 86%(18/21). For HCs, 87%(13/15) of retest trials were included, due to the exclusion of a retest trial of one participant who was able to reach all targets in both trials, while the wrist point did not move in the skeletal data in the retest trial. Primarily in patients, some trials differed substantially between test and retest, but because no reference video was available, it was unclear whether this was caused by the differences in movements or body tracking issues of the Kinect.
Regarding construct validity, FWS summed distance differed significantly between patients and HCs (Table 1 and Fig. 3B).
Regarding reliability in patients, Bland-Altman mean bias was 0.3 with 95%limits of agreement of –3.8 to 4.3 hand lengths (n = 18; Fig. 3C). FWS summed distance was more reliable in HCs with a mean bias of 0.1 and 95%limits of agreement of –0.68 to 0.8 hand lengths (n = 12). Stepwise approach for FWS summed distance was not continued after the first step (Fig. 5), because of insufficient reliability where the 95%-CI exceeded the difference between patients and HCs.
Kinect trunk compensation
Regarding quality control, movements of all participants contained at least five cycles which enabled calculation of the KinectTC value. We included 82%(139/170) of movement cycles for patients and 94%(132/140) for HCs. Some of the cycles that were excluded due to artefacts showed jumps in the spine shoulder point that occurred when the hand and arm occluded this point during the hand-to-mouth movement.
Regarding construct validity, KinectTC differed significantly between patients and HCs and between patients with and without visually scored trunk compensation (Table 1 and Fig. 4B and C).
Regarding reliability, the average within subject SD was 36 for DMD patients and 14 for HCs (Fig. 4D). The 95%CI (19–52) for patients was smaller than the difference between patients with and without trunk compensation.
Regarding concurrent validity, KinectTC showed a moderate correlation with PUL total (rho = –0.69, Fig. 6B). Therefore the stepwise approach was not continued (Fig. 5).
DISCUSSION
We studied the feasibility of Leap Motion aROM, ACTIVE, FWS and a new measure, KinectTC, as outcome measures in non-ambulant DMD patients. At this time, current versions of Leap Motion aROM and FWS summed distance were shown to be unreliable as outcome measure for clinical trials in DMD. KinectTC correlated insufficiently with functional measures. Only ACTIVE showed promise as outcome measure in non-ambulant DMD due to its correlation with a functional outcome scale, decline over 12 months, and patient appraisal. However, the SRM was lower than for PUL.
Low reliability of Leap Motion aROM in both DMD patients and HCs can at least partly be explained by the incorrect estimation of the forearm and elbow motion. It was observed that the elbow was not in view during the wrist flexion/extension motion. This was a consequence of our choice to position the sensor on the side to allow patients to rest their hands. In the Leap Motion developer archive it is stated that the elbow position is estimated in case the elbow is not in view [24]. While this might not cause problems when playing games, this caused the Leap Motion to not provide sufficiently reliable results on wrist aROM to be used as outcome measure.
FWS summed distance was reliable in HCs, but not in DMD patients. This may be due to the fact that Kinect had more difficulty to reliably recognize participants and their motion in a wheelchair, and to recognize the more subtle movements of severely affected patients. Finally, we also observed that patients responded differently to the instruction not to use trunk compensation. A potential solution could be to instruct patients to move the hand to the seven targets as they would in daily life, and thus use as much compensation as they choose. On the other hand this could also introduce more difficulties for the Kinect to follow extreme movement of patients due to occlusion. The custom software with the rigid model and analysis in MATLAB are still under development and further adjustments could improve the reliability in patients [13]. The concept to track different movements of the upper extremities close to the body that simulate functional movements used frequently in daily-life seems worthwhile to explore further whilst trying to improve the software and analysis.
KinectTC was applied as the first outcome to quantify compensation strategies in DMD. In our study, it fell short as outcome measure, because it did not show sufficient correlation with functional measures.
Occlusion is one of the challenges in using camera-based marker-less tracking devices, such as Leap Motion and Kinect. In KinectTC, small and sometimes larger jumps in the spine shoulder point were observed when the hand and arm occluded this point during the hand-to-mouth movement. The Kinect was also better positioned to register lateral flexion than flexion or extension of the trunk, although Kinect was shown to be comparable to 3D motion analysis in assessing both lateral and anteroposterior movements [25]. KinectTC did seem to give insight into use of trunk compensation in patients with a PUL total score of about 18–36 points. Since a measure similar to KinectTC was able to show response to the use of an arm support in three DMD patients [14], KinectTC could provide useful additional data in clinical care to support decisions of therapies and supportive devices. However, our data do not support its use as outcome measure in clinical trials.
ACTIVE showed the most promise as an outcome measure in non-ambulant DMD. Our study supports that patients should perform at least three or four ACTIVE trials, since 45%of patients did not achieve the highest Scaled Volume in the first or second attempt. In a previous study in SMA patients, only two trials were performed per participant [12]. In our study we could not determine test-retest reliability, but this was previously demonstrated to be excellent in a small population of eight DMD patients [9]. ACTIVE was responsive to disease progression, but the SRM was lower than the commonly used threshold of 0.8, and also lower when compared to the PUL. As a consequence, the sample size when using ACTIVE (169) was also much larger than that for PUL (39) [22]. In the study in SMA patients using ACTIVE, the MCID was 4.5–10.9 and the predicted sample size was 28 patients [12]. This MCID was smaller than our value of 14.1, which is potentially caused by our diverse patient population leading to large baseline SD. Their predicted required sample size of 28 patients was also much smaller than ours of 169 patients, most likely because our calculation was based on a 50%reduction in disease progression, while the SMA calculation was based on an improvement of median 15.9 points caused by nusinersen. In terms of enjoyability, patients showed no clear preference for ACTIVE or PUL, but PUL was reported less tiring than ACTIVE. The order of assessments is unlikely to have influenced these results, since PUL was performed later than ACTIVE. Also ACTIVE and PUL were both suited for our patient with autism spectrum disorder. ACTIVE showed promise as outcome measure in non-ambulant DMD, but sensitivity to change was lower than the commonly used threshold.
Hardware from the gaming industry has a limited production time, and the production of the Kinect v2 sensor by Microsoft that was applied in this study was discontinued in 2015 [26]. There are other sensors available that could also be used to determine the same outcomes. Some sensors require the use of markers, which is perhaps less time-efficient than using marker-less sensors. While switching to a different sensor is possible, a validation process or separate study should be conducted to determine characteristics of the outcome measure when using the new sensor, such as construct validity, reliability, concurrent validity and change over time.
Use of software from the gaming industry has limitations. The provided SDK software is not adjusted to correctly track persons with particular limitations, which poses challenges when using these devices in patient populations. For instance, DMD patients were sometimes not recognized by the SDK for ACTIVE, FWS and KinectTC software, because they sat in a wheelchair with armrests and a headrest from which they were hard to distinguish by the software. The presence of intellectual property poses an additional limitation. Constraints have been found in the Leap Motion software for the hand model that we used, Orion Beta v3.2.1 [10]. We were not allowed to get details about these constraints, and the terms and conditions for use of the software did not allow modification of the SDK to analyze the raw data differently, unless a Development License was procured. The current supplied version, v4.1.0, is different from the one we used, but a clear list of changes is not provided.
The ACTIVE software was developed by researchers from Research Institute at Nationwide Children’s Hospital, Ohio, USA. The used software (version 2017) is still operational, but was updated in 2019, after the start of this study. In this 2019 software version a new avatar was added, which provides real-time feedback to patients. This potentially leads to similar problems of comparability between results obtained with different software versions. When the analysis algorithm is not transparent, the same software version should be used continuously in clinical trials, or a new study should be conducted each time a new software version is used, to obtain data on the properties of this adjusted outcome measure. The ACTIVE yields a total volume and volume for the different levels, but data cannot be checked afterwards by replaying the movement of the wrist or hand points that are used to acquire these volumes. Transparency of analysis algorithms would enable updates, for instance to deal with bugs, if a standard validation process is in place to ensure that the changes made do not affect the outcomes.
The data presented illustrate the black box of commercial software or software otherwise protected by intellectual property, and the obstacles when using this software for sustainable scientific applications, such as use for outcome measures in future clinical trials. The term black box is used increasingly in this era of big data and medical algorithms [27]. For researchers, to develop a new device and get approval to use it as primary outcome measure in clinical trials is a lengthy process, as shown by the stride velocity 95th centile [28]. Commercial parties are able to develop and improve devices and software fast and the research field and patient organizations are looking to profit from this speed by collaborating. Examples are the recent World Duchenne Organization meeting about the use of wearables and a study using Apple watches to collect activity data, potentially to use as outcome measure [29]. The presented obstacles for use of hardware from commercial entities or software protected by intellectual property should incite the debate on future directions for the outcome measure research field and possible solutions for this black box, such as transparency of analysis algorithms.
Limitations of this study are the small study cohort for which not all follow-up visits could take place due to the COVID-19 pandemic. The change over time results were therefore based on small numbers. Although some trials and movement cycles for the different outcomes had to be excluded, this led only to exclusion of <5%of all data gathered.
In summary, of the evaluated technological outcome measures in their current iteration of development or version, ACTIVE showed the most promise due to a strong correlation with functional measures, change over 12 months and appraisal of being fun. However, the SRM was lower than commonly used thresholds. PUL met all criteria satisfactorily and had a SRM above this threshold. Outcome measures based on hardware and software from the gaming industry can indeed overcome problems such as observer dependence and lack of motivation. However, lack of insight in detailed operations of the software and hardware compounded by intellectual property constraints, and possible software updates and hardware discontinuation, make these outcome measures a black box and could jeopardize their long-term applicability in clinical trials.
ACKNOWLEDGMENTS
This study was supported by Stichting Spieren for Spieren (grant number SvS15). Several authors of this publication are members of the Netherlands Neuromuscular Center (NL-NMD) and the European Reference Network for rare neuromuscular diseases EURO-NMD.
CONFLICT OF INTEREST
K.J. Naarding, M.M.H.P. Janssen, R.D. Boon, P.J.M. van Schaik-Bank, R.P. Matthew, J.J.G.M. Verschuuren, I.J.M. de Groot, H.E. Kan and E.H. Niks report no relevant disclosures in regard to this study.
G. Kurillo is a paid consultant for Bioniks, a medical device software company.
J.J. Han is a consultant for Bioniks, Sanofi/Genzyme, and Spark Therapeutics.
M. van der Holst reports paid consultancy for ATOM international ltd. outside the submitted work.
SUPPLEMENTARY MATERIAL
The supplementary material is available in the electronic version of this article: https://dx.doi.org/10.3233/JND-210767.
REFERENCES
[1] | Birnkrant DJ , Bushby K , Bann CM , Apkon SD , Blackwell A , Brumbaugh D , et al. Diagnosis and management of Duchenne muscular dystrophy, part 1: Diagnosis, and neuromuscular, rehabilitation, endocrine, and gastrointestinal and nutritional management. Lancet Neurol. (2018) ;17: (3):251–67. doi:10.1016/S1474-4422(18)30024-3. |
[2] | McDonald CM , Henricson EK , Abresch RT , Duong T , Joyce NC , Hu F , et al. Long-term effects of glucocorticoids on function, quality of life, and survival in patients with Duchenne muscular dystrophy: A prospective cohort study. Lancet. (2018) ;391: (10119):451–61. doi:10.1016/S0140-6736(17)32160-8. |
[3] | Brooke MH , Fenichel GM , Griggs RC , Mendell JR , Moxley R , Florence J , et al. Duchenne muscular dystrophy: Patterns of clinical progression and effects of supportive therapy. Neurology. (1989) ;39: (4):475–81. doi:10.1212/wnl.39.4.475. |
[4] | CHMP. Guideline on the clinical investigation of medicinal products for the treatment of Duchenne and Becker muscular dystrophy. In European Medicines Agency (EMA). Available at: http://www.ema.europa.eu/docs/en_GB/document_library/Scientific_guideline/2015/12/WC500199239.pdf. Accessed December 18, 2020. |
[5] | Verhaart IEC , Aartsma-Rus A . Therapeutic developments for Duchenne muscular dystrophy. Nature reviews Neurology. (2019) ;15: (7):373–86. doi:10.1038/s41582-019-0203-3. |
[6] | Pane M , Mazzone ES , Fanelli L , De Sanctis R , Bianco F , Sivo S , et al. Reliability of the Performance of Upper Limb assessment in Duchenne muscular dystrophy. Neuromuscul Disord. (2014) ;24: (3):201–6. doi:10.1016/j.nmd.2013.11.014. |
[7] | Pane M , Coratti G , Brogna C , Mazzone ES , Mayhew A , Fanelli L , et al. Upper limb function in Duchenne muscular dystrophy: 24 month longitudinal data. PLoS One. (2018) ;13: (6):e0199223. doi:10.1371/journal.pone.0199223. |
[8] | Han JJ , de Bie E , Nicorici A , Abresch RT , Anthonisen C , Bajcsy R , et al. Reachable workspace and performance of upper limb (PUL) in duchenne muscular dystrophy. Muscle Nerve. (2016) ;53: (4):545–54. doi:10.1002/mus.24894. |
[9] | Lowes LP , Alfano LN , Crawfis R , Berry K , Yin H , Dvorchik I , et al. Reliability and validity of active-seated: An outcome in dystrophinopathy. Muscle Nerve. (2015) ;52: (3):356–62. doi:10.1002/mus.24557. |
[10] | Nizamis K , Rijken NHM , Mendes A , Janssen M , Bergsma A , Koopman B . A novel setup and protocol to measure the range of motion of the wrist and the hand. Sensors (Basel). (2018) ;18: (10). doi:10.3390/s18103230. |
[11] | Gamboa E , Serrato A , Castro J , Toro D , Trujillo M . Advantages and limitations of leap motion from a developers’, physical therapists’, and patients’ perspective. Methods Inf Med. (2020) ;59: (2–03):110–6. doi:10.1055/s-0040-1715127. |
[12] | Alfano LN , Miller NF , Iammarino MA , Moore Clingenpeel M , Lowes SL , Dugan ME , et al. ACTIVE (Ability Captured Through Interactive Video Evaluation) workspace volume video game to quantify meaningful change in spinal muscular atrophy. Dev Med Child Neurol. (2020) ;62: (3):303–9. doi:10.1111/dmcn.14230. |
[13] | Matthew RP , Seko S , Kurillo G , Bajcsy R , Cheng L , Han JJ , et al. Reachable workspace and proximal function measures for quantifying upper limb motion. IEEE J Biomed Health Inform. (2020) ;24: (11):3285–94. doi:10.1109/JBHI.2020.2989722. |
[14] | Kooren PN , Dunning AG , Janssen MM , Lobo-Prat J , Koopman BF , Paalman MI , et al. Design and pilot validation of A-gear:Anovel wearable dynamic arm support. J Neuroeng Rehabil. (2015) ;12: :83. doi:10.1186/s12984-015-0072-y. |
[15] | van den Bergen JC , Ginjaar HB , van Essen AJ , Pangalila R , de Groot IJ , Wijkstra PJ , et al. Forty-five years of duchenne muscular dystrophy in The Netherlands. J Neuromuscul Dis. (2014) ;1: (1):99–109. . |
[16] | Naarding KJ , Doorenweerd N , Koeks Z , Hendriksen RGF , Chotkan KA , Krom YD , et al. Decision-making and selection bias in four observational studies on duchenne and becker muscular dystrophy. J Neuromuscul Dis. (2020) ;7: (4):433–42. doi:10.3233/JND-200541. |
[17] | Gauld LM , Kappers J , Carlin JB , Robertson CF . Height prediction from ulna length. Dev Med Child Neurol. (2004) ;46: (7):475–80. doi:10.1017/s0012162204000787. |
[18] | Aldegheri R , Agostini S . A chart of anthropometric values. J Bone Joint Surg Br. (1993) ;75: (1):86–8. doi:10.1302/0301-620X.75B1.8421044. |
[19] | Mayhew AG , Coratti G , Mazzone ES , Klingels K , James M , Pane M , et al. Performance of Upper Limb module for Duchenne muscular dystrophy. Dev Med Child Neurol. (2019) . doi:10.1111/dmcn.14361. |
[20] | Wong DL , Baker CM . Pain in children: Comparison of assessment scales. Pediatr Nurs. (1988) ;14: (1):9–17. |
[21] | Mukaka MM . Pain in children: Comparison of assessment scales. Pediatr Nurs. (1988) ;14: (1):9–17. |
[22] | Cohen J . Statistical power analysis for the behavioral sciences. Lawrence Erlbaum Associates; 1988. |
[23] | Morrow JM , Sinclair CD , Fischmann A , Machado PM , Reilly MM , Yousry TA , et al. MRI biomarker assessment of neuromuscular disease progression: A prospective observational cohort study. Lancet Neurol. (2016) ;15: (1):65–77. doi:10.1016/S1474-4422(15)00242-2. |
[24] | Arms https://developer-archive.leapmotion.com/documentation/csharp/devguide/Leap_Overview.html. Accessed on May 28, 2020, Leap Motion Inc. |
[25] | Clark RA , Pua YH , Fortin K , Ritchie C , Webster KE , Denehy L , et al. Validity of the Microsoft Kinect for assessment of postural control. Gait Posture. (2012) ;36: (3):372–7. doi:10.1016/j.gaitpost.2012.03.033. |
[26] | Microsoft Microsoft to consolidate the Kinect for Windows experience around a single sensor. Accessed on May 5, 2020 at: https://docs.microsoft.com/en-us/archive/blogs/kinectforwindows/microsoft-to-consolidate-the-kinect-for-windows-experience-around-a-single-sensor: Kinect for Windows, Microsoft. |
[27] | Price WN . Big data and black-box medical algorithms. Sci Transl Med. (2018) ;10: (471). doi:10.1126/scitranslmed.aao5333. |
[28] | CHMP. Qualification opinion on stride velocity 95th centile as a secondary endpoint in Duchenne Muscular Dystrophy measured by a valid and suitable wearable device*. In European Medicines Agency (EMA). Available at: https://www.ema.europa.eu/en/documents/scientific-guideline/qualification-opinion-stride-velocity-95th-centile-secondary-endpoint-duchenne-muscular-dystrophy_en.pdf. Accessed June 16, 2021. |
[29] | UK D KINEDMD is a study developing an activity monitoring biomarker. Available at: https://www.duchenneuk.org/outcome-measures/. Accessed July 30, 2021. |