Prefrontal BOLD Responses Coupled to Changing Emotional Faces in Adolescents with and without a History of Suicide Attempt
Abstract
Background:
Functional abnormalities in emotion processing neural circuitry in adolescents with a history of suicide attempt relative to depressed adolescents with no history of suicide and healthy controls have been identified, typically utilizing static face presentations.
Objective:
The objective of the present work was to characterize functional activations associated with emotional face processing in adolescents with and without a history of suicide attempt.
Methods:
64 adolescents including 19 with a history of depression and suicide attempt (ATT), 22 with a history of depression but no suicide attempt (NAT) and 23 healthy controls (HC) performed an implicit emotional-faces task during functional neuroimaging, in which they identified a color label superimposed on neutral faces that dynamically morphed into one of four emotional faces (angry, fearful, sad, and happy).
Results:
HC showed greater Blood Oxygenation Level Dependent (BOLD) responses compared with ATT in the Right Dorsolateral Prefrontal Cortex (rDLPFC) to all emotional faces compared to shapes. A similar pattern of group differences was seen when both ATT and NAT groups were compared with HC. Across all participants, an association between child trauma and rDLPFC activation was seen, although this was not corrected for multiple comparisons.
Conclusions:
Together, the findings are consistent with prior observations of emotion-related alterations in neural function in suicide attempters. However, they also suggest that adequate control groups are necessary to dissociate specific correlates of suicide risk from depression or trauma severity, which may contribute to prefrontal alterations in emotion processing.
INTRODUCTION
Prevention of suicide attempt, across all age groups, is identified as a mission of the US National Institute of Mental Health (NIMH: [1]) and associated public and private stakeholders. Suicidal behavior is a leading cause of morbidity and mortality in adolescence, and suicidal ideation and attempt are commonly reported. For example, [2] reports suicidal ideation and behaviors in around 7–17% of high school students. Despite progress in understanding risk factors for suicidal behavior [3], the pathogenesis is poorly understood, including possible alterations in neural circuitry that might predispose to suicidal behavior. Progress in the prediction and prevention of suicidal behavior would be facilitated by identification of biomarkers for suicide risk and an increased understanding of the underlying pathogenesis of suicidal behavior in order to target and reverse such processes.
Several laboratories have identified potential neural markers of suicidal risk using fMRI [4–7], particularly using emotion- and cognitive control-related paradigms. For example, we have previously identified functional differences in emotion processing and cognitive control-related neural circuitry in depressed adolescents with past suicide attempt relative to depressed adolescents with no history of suicide attempt and healthy controls [4, 8, 9]. Specifically, we have shown increased activity in anterior cingulate and dorsolateral prefrontal cortical attentional control networks, and decreased functional connectivity between the dorsal anterior cingulate cortex and the insula when viewing static, mild angry faces in adolescents with a history of depression and suicide attempt compared with healthy controls and depressed adolescent non-attempters [9]. In contrast, we observed no abnormalities in performance accuracy, dorsal anterior cingulate activity, or attentional control network activity on tasks of learning in the context of economic risk [10]. These findings suggest that abnormal functioning of ‘task-positive’ networks involved in salience and executive control, perhaps restricted to the setting of emotion processing or other specific contexts, may represent markers of past suicide attempt. Nevertheless, functional abnormalities in these regions have been identified using resting state functional connectivity in suicidal ideators [11], and further work is required to confirm that a unique endophenotype for suicidal behavior, that is distinct from the neuropathophysiology from MDD per se, can be observed. The use of emotional faces may offer potential to evaluate predispositions towards abnormal affective responses that have been proposed to be characteristic of suicide risk. In particular, one proposal is that individuals at risk of suicide show alterations in the negative valence system [12].
Other reports have implicated abnormalities in the functioning of emotional processing regions in suicide attempters (e.g. [13, 14], often in response to negative emotion. Specifically, adult male suicide attempters showed greater activity in the right lateral orbitofrontal cortex and decreased activity in the right superior frontal gyrus to intense angry versus neutral faces relative to healthy and depressed non-attempter controls [13]. More recently, machine learning techniques have been successful in decoding putative emotional states that may characterize suicidal ideation [15]. These findings, taken together with our findings of differences in the attention and salience networks in adolescent attempters described above suggest that it may be possible to identify consistent abnormalities in emotion processing that may be associated with past, or predictive of future suicide attempts. Measuring functional activity during a task that better captures the dynamic nature of real-world emotion processing is the logical next step in understanding how abnormalities of attention to emotion and processing the evolution of emotion may contribute to suicidal behavior. To date, no study has examined neural circuitry underlying dynamic emotional face processing in adolescents with a history of suicide attempt.
The aim of the present study was to measure neural activity during dynamic emotional face processing in adolescents with a history of depression and suicide attempt versus age-matched adolescents with a history of depression but no suicide attempt and age-matched healthy controls, using functional neuroimaging. First, previous studies used static images of facial displays that varied in intensity of emotion. In the present study, we employed stimuli in which a video of facial display dynamically morphed over a one-second interval from 0% emotion (neutral) to 100% emotional intensity. These stimuli are designed to mimic more closely the real-world experience of witnessing changes in facial displays of emotion during a social encounter. In addition, we examined neural activity in adolescents with a history of depression and suicide attempt (ATT), adolescents with a history of depression and no suicide attempt (NAT), and healthy control (HC) participants, to facial displays of anger, happiness, fear, and sadness. To our knowledge, this is the first such study to compare neural activity to dynamic emotional faces in adolescents with a history of suicide attempt. We performed two sets of hypothesis-led analyses: first, to examine an overall difference in emotion- versus shape-related activations with planned t-tests contrasting all three groups in whole brain activation; second, to identify a group by emotion-type interaction – in particular, a selective effect of group on a particular emotional category compared to the others. These analyses would address the question of whether neural activations to emotional faces were abnormal overall in suicide attempters, or whether they are biased specifically towards particular emotions rather than others (e.g. towards negative rather than positive emotions). Although our primary hypothesis was that suicide attempters would show selective alterations compared to the other two groups, we also examined the presence of non-specific illness-related factors which might differentiate the patient groups from controls.
Finally, we performed follow up analysis of individual differences with respect to clinical measures to identify dimensions of pathology which might account for variation in brain activation. Suicide attempters, when considered as a group, can be highly heterogeneous, and regression analysis may help to identify dimensions of pathology which can explain some of this variation. Moreover, while our design allowed some control over non-specific, illness related factors, it remains possible that any identified markers reflect inadequate matching between the attempter and non-attempter groups. Correlational analysis was conducted to address these possibilities.
MATERIALS AND METHODS
Participants (Table 1)
64 adolescents completed the study, including those with 1) lifetime history of suicide attempt and Major Depressive Disorder (MDD) (ATT; n = 19); 2) history of MDD, but no history of suicide attempt: non-attempters (NAT; n = 22); and 3) healthy control participants without personal or first-degree relative history of psychiatry disorder or suicide attempt (HC; n = 23). Given the age range of the participants, screening the healthy controls in this way increases the likelihood that they will not show trait-level risk factors for future mental illness.
Table 1
Demographic, clinical and behavioral measures for all three groups
HC | NAT | ATT | F/χ2 test | Post-Hoc Tests | ATT vs NAT | |
N | 19 (9M:14F) | 22 (6M:16F) | 23 (5M:14F) | χ2 = 1.042, p = 0.59 | N/A | X2 < 1 |
Age | 14.65 (1.75) | 16.00 (1.38) | 15.63 (1.54) | Fw(2,39.86) = 4.15, p = 0.023 | NAT > HC (p = 0.017) | T < 1 |
IQ | 117.78 (10.34) | 121.91 (8.46) | 123.32 (6.95) | F(2,61) = 2.30, p = 0.11 | N/A | T < 1 |
Petersen | 3.17 (0.72) | 3.64 (0.49) | 3.47 (0.69) | F(2,61) = 3.01, p = 0.057 | N/A | T < 1 |
SIQ | 3.44 (2.79) | 23.32 (2.86) | 22.21 (3.075) | Fw(2,33.50) = 28.02, p < 0.001 | NAT&ATT > HC (p’s < 0.001) | T < 1 |
BDI | 3.70 (2.015) | 16.86 (2.060) | 15.26 (2.22) | Fw(2,37.35) = 15.38, p < 0.001 | NAT&ATT > HC (p’s < 0.001) | T < 1 |
SCARD-P | 6.22 (2.78) | 28.77 (2.84) | 17.58 (3.054) | Fw(2,33.85) = 20.31, p < 0.001 | NAT&ATT > HC (p’s < 0.024) | T(39) = –2.29, p = 0.028 |
SCARD-C | 11.83 (2.99) | 35.14 (3.052) | 26.74 (3.28) | Fw(2,38.36) = 16.34, p < 0.001 | NAT&ATT > HC (p’s < 0.002) | T(39) = –1.70, p = 0.098 |
CTQ | 26.83 (1.71) | 33.23 (1.75) | 39.32 (1.88) | Fw(2,27.84) = 15.66, p < 0.001 | NAT&ATT > HC (p’s < 0.011) | T(39) = 1.92, p = 0.062 |
BIS-11 Total | 58.043 (2.35) | 66.00 (2.41) | 68.47 (2.59) | F(2.61) = 5.039, p = 0.009 | ATT > HC (p = 0.009) | T < 1 |
BIS-11 NP | 22.39 (1.081) | 24.27 (1.11) | 24.079 (1.19) | F < 1 | N/A | T < 1 |
BIS-11 Motor | 14.83 (0.70) | 15.77 (0.71) | 18.079 (0.76) | Fw(2,39.34) = 4.52, p = 0.017 | ATT > HC (p = 0.013) | T(39) = 2.20, p = 0.034 |
BIS-11 Attention | 9.22 (0.64) | 13.091 (0.65) | 12.71 (0.70) | F(2,61) = 10.95, p < 0.001 | NAT&ATT > HC (p’s < 0.002) | T < 1 |
Mean RT (ms) | 828.06 (81.68) | 945.06 (173.46) | 922.60 (187.45) | Fw(2,32.98) = 5.33, p = 0.010 | HC < NAT (p = 0.020) | T < 1 |
Mean accuracy (%) | 89.1 (0.089) | 95.2 (0.034) | 89.7 (0.075) | F(2,61) = 6.17, p = 0.004 | NAT > HC&ATT (p’s < 0.014) | T(39) = –3.047, p = 0.004 |
Means and standard deviations are reported in the columns 2–4, F, Welch (Fw) or χ2 tests in the 5th, Games-Howell post hoc tests in the 6th if the F or X2 test was significant, and the 7th reports a simple t-test between the ATT and NAT groups.
ATT and NAT were recruited from existing studies and a clinic for depressed youth. HC were recruited from advertisement in pediatric practices. Inclusion criteria for NAT and ATT included a lifetime history of MDD according to DSM-IV criteria [16]. ATT had a history of at least one suicide attempt as defined by the Columbia Classification Algorithm of Suicide Assessment (C-CASA) [17]. All participants were right-handed.
Exclusion criteria included neurological disorders, head injury, Verbal Subtest of the Wechsler Intelligence Test (Wechsler, 1999) score < 80, use of sedative medication, drug or alcohol disorder or positive urine drug screen, pregnancy, MRI ineligibility, bipolar disorder or psychosis and left-handedness. Any ATT with suicide attempt causing neurological damage or long term physiologic effects was excluded.
DSM-IV criteria were assessed using the K-SADS interview [18]. Suicide attempt was assessed using the Columbia Suicide Intent Scale (SIS: [19]) and Suicide History Form (SHF: [20]). Depression, anxiety, suicidal ideation, and pubertal status were assessed with the Beck Depression Inventory (BDI: [21], Screen for Childhood Anxiety Related Emotional Disorders (SCARED: [22] – both the parent- and self-report version were included as is conventional), Suicidal Ideation Questionnaire (SIQ) [23], and Petersen Development Scale [24], respectively. In addition, the Child Trauma Questionnaire (CTQ: [25] and the Barratt Impulsiveness Scale (BIS-11: [26]) were also administered. After complete description of the study to all subjects, parental written informed consent and participant written informed assent was obtained.
74 total participants were assessed. 10 Participants were excluded (3 HC/4 NAT/3 ATT): eight with poor movement only (total movement > 3 mm), and two who fell asleep during the scan and > 3 mm movement.
Paradigm
Participants completed a 12.5-minute emotional dynamic faces task during fMRI. Stimuli comprised faces from the NimStim set [27] that were morphed in 5% increments, from neutral (0% emotion) to 100% emotion for four emotions: happy, sad, angry, and fear (Fig. 1). Morphed faces were collated into one-sec movies progressing from 0% to 100% emotional display. In control trials, movies comprised a simple shape (dark oval) superimposed on a light-grey oval, with similar structural characteristics to the face stimuli, which subsequently morphed into a larger shape, approximating the movement of the morphed faces. An additional set of experimental trials, not-examined below, included neutral faces that morphed from one identity to another. Neutral faces may be associated with a distinct set of psychological and neural responses (e.g. [28]), perhaps related to decoding ambiguous expressions, which were not relevant to the present work. There were three blocks for each of the four emotional conditions with 12 stimuli per block, and six control blocks with six stimuli per block. Emotional and control blocks were presented in a pseudo-randomized order so that no two blocks of any condition were presented sequentially. Participants were asked to use a button press, selecting one of three buttons, to indicate the color of a semi-transparent foreground color flash (orange, blue, or yellow) that appeared during the mid-200–650msec of the one-sec presentation of the dynamically changing face. The emotional faces were task irrelevant and, thus, were processed implicitly.
Fig.1
The top panel presents a fear trial of the dynamic emotional faces task. Over a one-sec duration, the participants viewed a movie of a face that changed in 5% increments from neutral (0% emotion) to a happy, sad, angry, or fearful (100% emotion) face. The bottom panel represents the changing shape condition, which was used as the baseline condition. In both cases, participants were asked to identify the color flash presented in the middle of the dynamic change.
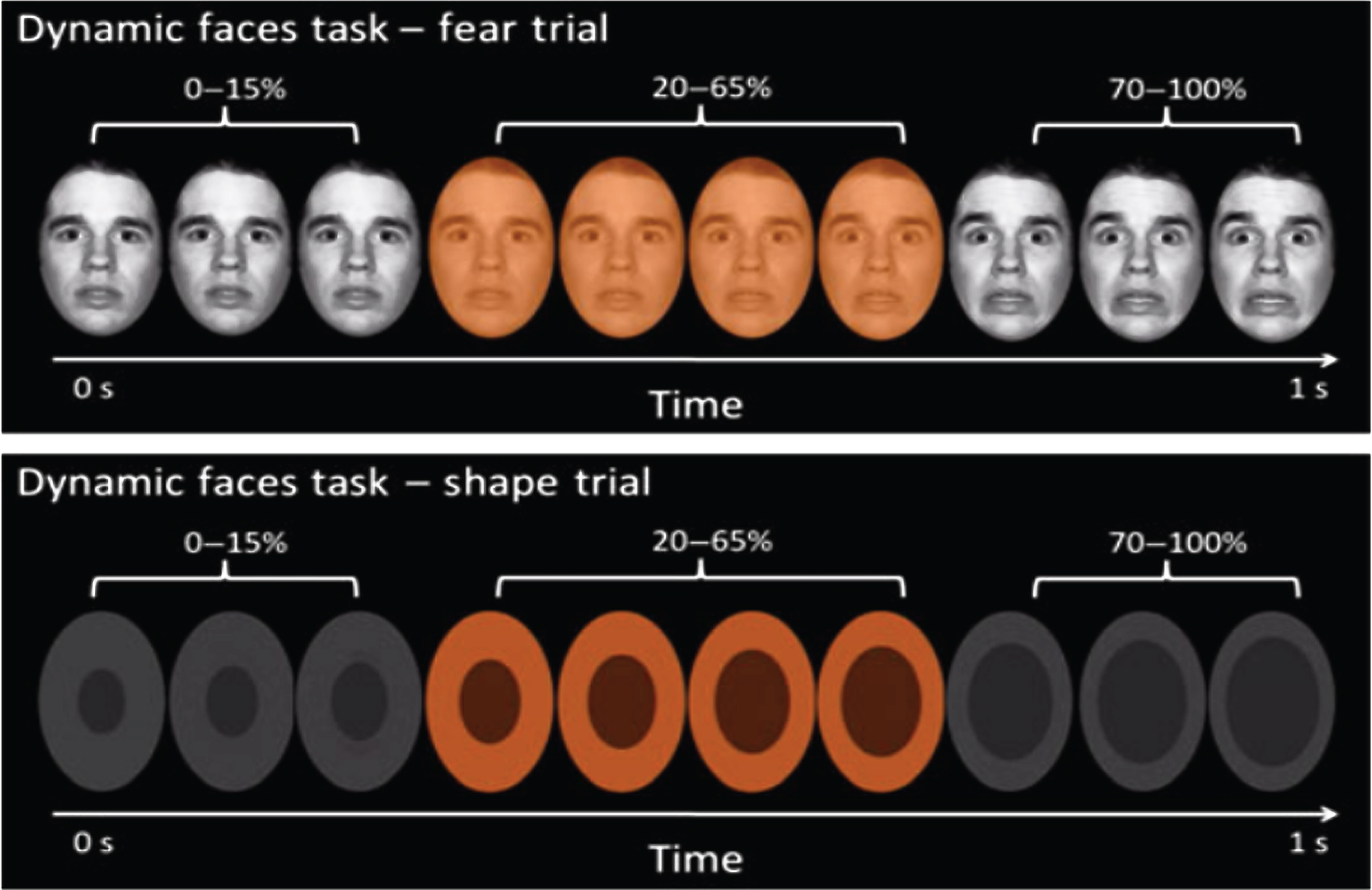
Fig.2
Display of contrast of all emotional faces versus shapes (red), and shapes versus all emotional faces (blue), across all individuals. Contrast map thresholded at p < 0.001 uncorrected.
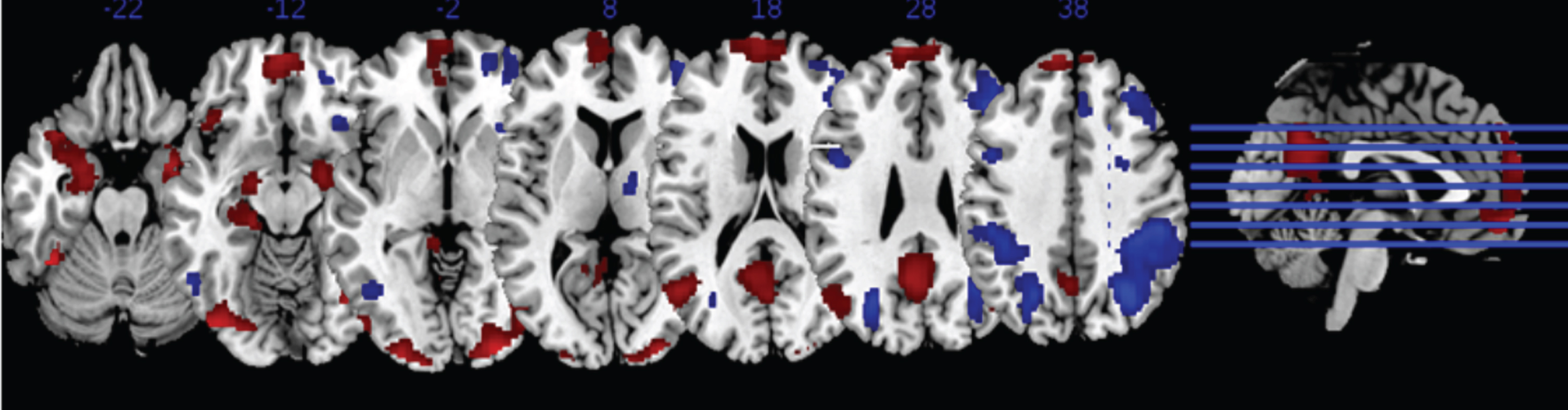
Fig.3
Left - Display of the contrast of ATT vs controls (blue) and both patient groups vs controls (red). Overlap is signified in purple. Contrast thresholded at p < 0.001 uncorrected for display purposes. Right – extracted parameter estimates from the right dorsolateral prefrontal cortex (rDLPFC), plotted for each group. Error bars reflect standard error of the mean.
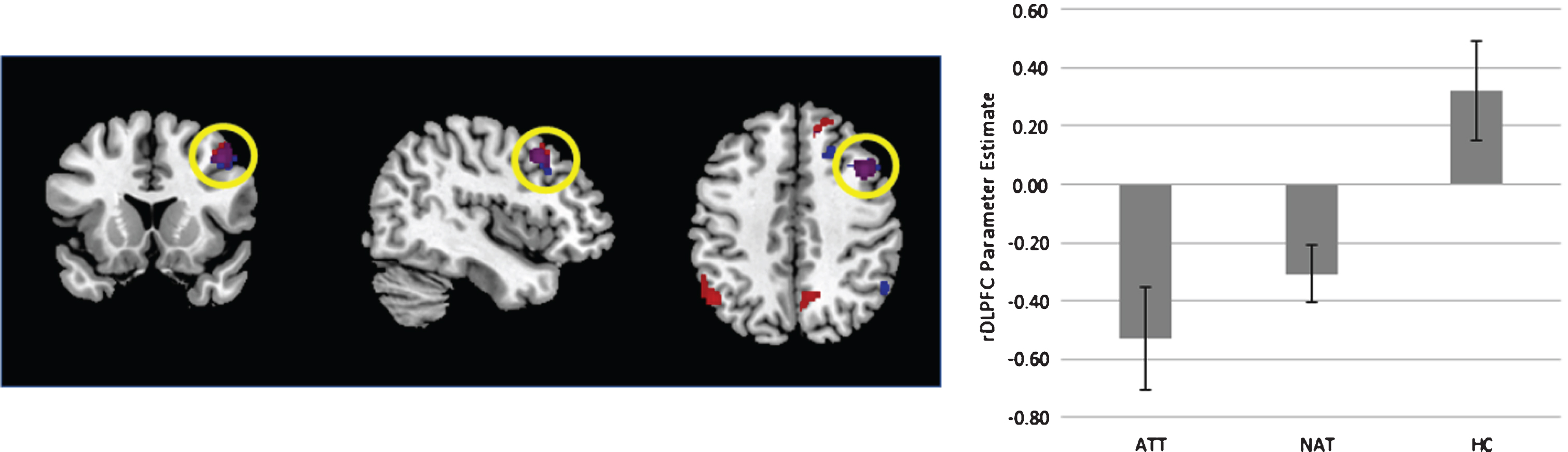
Data acquisition
Neuroimaging data were collected using a 3.0 Tesla Siemens Trio MRI scanner at the Magnetic Resonance Research Center in the University of Pittsburgh Medical Center. Structural three-dimensional axial magnetization prepared rapid acquisition gradient echo (MPRAGE) images were acquired in the same session [repetition time (TR)/echo time (TE) = 2200/3.29msec, flip angle = 9°, field of view (FOV) = 256×192mm2, slice thickness = 1 mm, matrix = 256×256, 192 continuous slices]. BOLD images were then acquired with a gradient echo planar imaging (EPI) sequence during approximately 13 minutes (378 successive brain volumes) covering 39 axial slices (3.2 mm thick, TR/TE = 2000/28msec, FOV = 205×205 mm2, matrix = 64×64, flip angle = 90°).
Functional neuroimaging analysis
Functional imaging data were preprocessed and analyzed with statistical parametric mapping software (SPM8; http://www.fil.ion.ucl.ac.uk/spm). During preprocessing, data were corrected for differences in acquisition time between slices, co-registered, realigned, resampled to 2×2×2 mm3 voxels, spatially normalized into standard stereotactic space [Montreal Neurologic Institute (MNI)], and spatially smoothed using a 6-mm full width at half maximum (FWHM) Gaussian kernel. A first-level fixed-effect model was constructed in which each of the four emotion conditions (anger, fear, sad, happy) were entered as separate conditions, while the shape condition, which served as the baseline in the design matrix, was subtracted from each. Trials were modeled using a boxcar function that lasted the duration of the trial, which were then convolved with the canonical hemodynamic response function. To account for irrelevant variability in the hemodynamic response function, temporal derivatives of each were also included for each of the modelled conditions [29]. Movement parameters from the reprocessing procedure were entered as covariates of no interest to control for subject movement, as was a timeseries reflecting fluctuations within white matter and cerebrospinal fluid (CSF: see also [29]).
Two sets of second level analyses were performed. First, a contrast which reflected general responses to emotional stimuli was generated by contrasting all four emotion conditions with the shape contrast, after weighting to correct for the one control condition and four manipulation conditions. This contrast was analyzed using T-tests to examine the effect of emotional faces versus shapes across all groups, as well as whole-brain differences between the groups. In general, these analyses employed a cluster forming threshold of p < 0.001, followed by a cluster threshold of family wise error (FWE)-corrected p < 0.05. For the analysis of group differences, as there were six potential tests for the 3 groups, we used a more conservative FWE-cluster threshold of p < 0.0083 rather than p < 0.05. To assess the effect of patient (ATT/NAT) status versus HC, we performed a follow-up analysis test with a FWE-corrected p < 0.05 to evaluate the potential of general illness effects.
The second analysis involved an ANOVA model implemented in SPM. The four emotion contrasts (i.e., anger–minus–shape, fear–minus–shape, sad–minus–shape, and happy–minus–shape) were entered into second-level analyses. This analysis allowed us to test the presence of a main effect of emotion, or an emotion by group interaction. In all whole brain analyses, age, gender, and IQ were included as covariates of no interest.
Although our main analytical strategy focused on evaluating group differences, an alternative approach would be to focus on dimensions of pathology. Thus, we also investigated whether any differences in neural activations identified by between-group contrasts were related to dimensions of illness severity of relevance to suicide. We examined CTQ, BDI, SIQ, BIS-11 total score, SCARED-P and SCARED-C. We used Pearsons’s correlations, and corrected for six potential comparisons (p = 0.0083).
Behavioral and Clinical data analyses
Task performance data were analyzed using one-way Analysis of Variance ANOVAs to examine the main effect of group upon response time and accuracy for all face and shape trials. Accuracy data were transformed using an arcsin transform before analysis. Clinical and demographic data were analyzed using Pearson Chi-Square’s (gender) and ANOVAs (all other variables) to examine the main effect of group. In the case of significant variance inhomogeneity or non-normality, Welch’s ANOVA was computed instead of conventional ANOVA. All ANOVA analyses were followed by Games-Howell post-hoc tests if the main effect of group was significant. Planned t-tests were also conducted to compare the ATT and NAT groups on all variables.
RESULTS
Demographics and clinical measures
Demographic information for the ATT, NAT, and HC groups are reported in Table 1. As expected, HC reported significantly fewer symptoms of depression, suicidal ideation, and anxiety than did the two patient groups (Table 1). ATT and NAT were intentionally matched for depression severity, and did not differ on SIQ or BDI. HC were younger than NAT and ATT, but groups did not differ significantly in pubertal status, IQ, or gender ratio. 17 NAT and 18 ATT were taking medication (see Table 2). All patients taking medication were taking an antidepressant. Two ATT had augmentation with atypical antipsychotics/benzodiazepines, and 3 ATT and 2 NAT had augmentation with the stimulant medication methylphenidate. Mean time since last attempt for ATT was 13 months 325 days (SD) and mean lethality of attempt from the SHF was 2.12 (2 = requiring medical intervention, Table 2).
Table 2
Medication Status for the patient (NAT/ATT) groups: 18 out of 19 Depressed Suicide Attempters and 17 out of 22 Depressed Non-Attempters were taking an antidepressant
Attempters (n = 18) | Non-Attempters (n = 17) | |
Antidepressants (n = 41) | Escitalopram (n = 2) | Escitalopram (n = 1) |
Citalopram (n = 2) | Citalopram (n = 2) | |
Bupropion (n = 3) | Bupropion (n = 3) | |
Fluoxetine (n = 5) | Fluoxetine (n = 7) | |
Trazodone (n = 1) | Amitriptyline (n = 1) | |
Sertraline (n = 7) | Sertraline (n = 7) | |
Stimulants (n = 5) | Methylphenidate (n = 3) | Methylphenidate (n = 2) |
Antipsychotics (n = 1) | Aripiprazole (n = 1) | |
Benzodiazepine (n = 1) | Clonazepam (n = 1) | |
Mean time since last attempt: | 12 months and 325 days (range 4–30 months) | |
Mean lethality of attempt (Suicide History Form): | 2.12 (requiring medical intervention) |
*All participants taking medication were taking an antidepressant.
Task performance
Mean color-labeling accuracy and mean reaction time were calculated for each participant across all conditions. Overall, task accuracy was high (see Table 1), and performance was broadly comparable among groups. Repeated-measures ANOVA did not reveal strong group by emotion interactions (RT: group by emotion F(8, 244) = 2.14, p = 0.077; accuracy: group by emotion F(8, 244) = 1.12, p = 0.35). On the accuracy scores, a main effect of emotion was seen (F(4,244) = 4.21, p = 0.003), which mostly reflected more accurate performance on the sad faces compared to the other expressions across all subjects (all T’s > 2.66, p’s < 0.011). Due to the lack of significant emotion by group interactions, we collapsed across emotions to clarify the main effect of group in both RT and accuracy (see Table 1). We observed faster responding in HC compared to NAT, and more accurate performance in NAT compared to the other two groups. Thus, the NAT group were generally somewhat slower but more accurate than the other groups. Including age, IQ and gender as covariates in these analyses did not alter the overall pattern of findings.
Neuroimaging
Group differences in across all emotional faces versus shapes
A positive effect of all emotion minus shape was observed in Bilateral Amygdala (R:MNI: x = 18 y = –6 z=–18; T = 9.46, k = 637, p_FWE = 0.001; L:MNI: x = –18 y=–8 z = –18;T=6.79, k = 1059, p_FWE = 0.001), Left Superior Frontal Gyrus (MNI: x = –6 y = 64 z = 24; T = 6.27, k = 1831, p_FWE = 0.001), Left Middle Temporal Gyrus (MNI: x = –48 y=–64 z = 18; T = 6.00, k = 580, p_FWE = 0.001), Left Parietal Gyrus (MNI: x = –4 y=–60 z = 30; T = 6.29, k = 1333, p_FWE = 0.001), and the Right Occipital Gyrus (MNI: x = 42 y = –78 z = –10; T = 9.92, k = 1296, p_FWE = 0.001).
A group difference emerged when comparing the HC to ATT groups, which revealed greater activity among HC in the Right Dorsolateral Prefrontal Cortex (MNI: x = 42 y = 16 z = 42; T = 4.99, k = 233, cluster p_FWE = 0.008). A secondary finding in the Right Superior Frontal Gyrus did not reach corrected significance after correcting for multiple whole-brain contrasts (MNI: x = 8 y = 38 z = 54; T = 5.40, k = 181, cluster p_FWE = 0.015).
A follow-up analysis, collapsing both patient cohorts (NAT&ATT) and comparing with healthy controls, yielded a similar map - showing greater activation among controls compared to patients in the Right Superior Frontal Gyrus (MNI: x = 8 y = 38 z = 54; T = 5.24, k = 287, cluster p_FWE = 0.001), the Right Dorsolateral Prefrontal Cortex (MNI: x = 42 y = 14 z = 44; T = 4.92, k = 188, cluster p_FWE = 0.013). No significant differences between NAT and ATT were observed.
ANOVA: Main effect of emotion and Group×Emotion Interaction
Analysis of the main effect of emotion revealed no significant differences. We also conducted a 3×4 (group×condition) repeated measures ANOVA to determine if the different emotional faces showed different activation patterns across groups. Again, no significant main effect of group nor group by emotion interactions were observed.
Exploratory analyses
Exploratory analyses were performed to examine relationships between clinical variables (BDI, SCARED (parent and child), SIQ) and emotion-related activation in the right DLPFC region across all individuals. A relationship with total CTQ was observed which reached uncorrected significance (r = –0.29, p = 0.02), but no other corrected or uncorrected associations were observed. Given that this correlation was obtained from a non-independent region of interest, we calculated a cross-validated estimate of the coefficient of determination using k-folds cross-validation. A value of R2 = 0.042 was obtained, corresponding to a moderate/small effect size. Since almost all of the patient groups received medication, an analysis was performed within the patient groups only of each class of medication (antidepressant, methylphenidate, antipsychotic, benzodiazepine), which did not yield significant differences.
DISCUSSION
The specific aim of the study was to determine the extent to which ATT showed abnormal activity during dynamic emotion processing compared with NAT and HC. The use of this task was relevant for its dynamic nature and more diverse assessment of emotions. A robust difference between the ATT and HC groups was seen in the right dorsolateral prefrontal cortex (DLPFC), with ATT showing less activity for emotional faces compared to shapes in this region than HC. No significant differences were seen between ATT and NAT groups, but both patient groups together showed significantly less activity in a network of regions including the DLPFC compared to HC. No significant group by emotion interactions were observed. Overall, a simple account of the findings would be that illness/depression severity, in which the ATT group, and to a lesser extent, the NAT group, is associated with a generalized alteration in neural activations to emotional stimuli in regions such as the right DLPFC and the Superior Frontal Gyrus. Broadly, these regions play a role in diverse task demands [e.g. 30, 31] and can show complex patterns of functional co-activation [32]. Nevertheless, this explanation remains to be confirmed in more detail, and in particular, the selectivity of the deficit for emotion-processing over and above other cognitive domains remains to be established.
The findings therefore stand in contrast to previous literature in which specific neural abnormalities have been identified which can distinguish individuals at heightened risk of suicide (including suicidal ideation [33]) and/or with a suicide history [e.g. 7, 13, 34, 35], but are consistent with studies which have reported null differences between the groups [14]. The whole-brain analysis approach which we adopted risks the potential of a false negative – a failure to identify a true, if moderate sized, underlying effect. Our findings suggest however that the non-specific effect of illness severity may be substantial, and thus important to correct for when trying to identify trait markers of suicide risk. In addition, our findings are somewhat consistent with a recent study of emotion regulation in suicidal adolescents [36] in which heightened DLPFC activity was observed in suicidal ideators compared to controls during explicit emotion regulation, but less DLPFC activity was observed during passive viewing. They are also consistent with our previous work [9] insofar as they also highlight deficits in cognitive control-relevant networks in suicidal individuals, but suggest that these deficits may be less selective for this suicide risk than previously thought.
One variable that explained variability in neural activation was a history of trauma: specifically, a weak association between scores on the child trauma questionnaire and right DLPFC emotion-related activation was seen across all individuals. In future, stronger evidence may be obtained by a more specific measure of trauma and its impact. At present, we conclude the trauma history may be one of a number of illness severity-related factors that influence DLPFC function during emotion processing.
We found significant differences between groups in task performance with regard to accuracy and reaction time (RT), though this was primarily driven by higher accuracy in NAT relative to ATT/HC and slower RT in NAT relative to HC. Generally, this pattern is consistent with a more conservative response threshold in NAT i.e. favoring accuracy over speed in RTs. This suggests that the neural abnormalities in cognitive control networks are not directly associated with behavioral deficits. The dynamic faces paradigm does require attentional control as the faces are irrelevant to the color discrimination task, so more sensitive tests of cognitive control are needed to identify the predicted behavioral abnormalities in the patient groups.
There were limitations to the current study. The sample size was modest, even in light of the difficulty in recruiting participants with a history of suicide attempt. Further work might explore larger cohorts, and employ parametric designs which can account for potential methodological differences in prior work (e.g. static/dynamic faces). HC controls were younger than NAT and ATT, though their pubertal status did not differ significantly due to the limited age range. In all neuroimaging analyses, age was included as a covariate. Furthermore, it was necessary to recruit ATT and NAT who were taking medication. Exploratory analyses did not, however, show any significant relationships in NAT and ATT between medication and activity in the dynamic emotional faces task in the DLPFC. Medication use could have contributed to the overall effect of illness severity (i.e. ATT&NAT vs HC), however, particularly in medial, rather than lateral, prefrontal regions [37]. In general, ATT and NAT were fairly well matched for medication status, as well as depression and anxiety symptom severity. Future studies should aim to recruit unmedicated ATT and NAT, given clinical and practical constraints. The cross-sectional nature of the study was a further limitation, and future studies might seek to examine the capacity of neuroimaging biomarkers to predict future clinical outcomes.
Summary
To our knowledge, this study is the first to examine the functional integrity of neural circuitry in adolescents with a history of suicide attempt during a dynamic emotional faces task. Together, our findings demonstrate the importance of considering dimensions of illness severity, and potentially highlight history of trauma as a relevant dimension of pathology. Thus, tight control of disease severity is essential for future studies attempting to identify specific markers of heightened suicide risk. More complex interactions between suicidality with depression severity or other co-morbidities may also need to be considered by futures studies. A goal for such studies should be to determine the extent to which functional abnormalities in neural circuitry supporting emotion processing may yield potential biomarkers of suicide risk in adolescence.
CONFLICTS OF INTEREST
Dr. Brent receives royalties from Guilford Press, has or will receive royalties from the electronic self-rated version of the C-SSRS from ERT, Inc., is on the Editorial board of UpToDate, is a reviewer for Healthwise, is on the board of the Klingenstein Third Generation Foundation, and receives honoraria for presenting at Continuing Medical Education events. None of the other authors declare any financial or other conflicts of interest that might have biased the work. The work was supported by NIMH award number 1K23MH082884-01 (to LP).
REFERENCES
[1] | Claassen CA , Pearson JL , Khodyakov D , Satow PM , Gebbia R , Berman AL , et al. Reducing the burden of suicide in the U.S.: the aspirational research goals of the National Action Alliance for Suicide Prevention Research Prioritization Task Force. Am J Prev Med. (2014) ;47: (3):309–14. |
[2] | Kann L , McManus T , Harris WA , Shanklin SL , Flint KH , Queen B , et al. Youth Risk Behavior Surveillance - United States, 2017. MMWR Surveill Summ. (2018) ;67: (No. SS-8):1–114. |
[3] | Cha CB , Franz PJ , E MG , Glenn CR , Kleiman EM , Nock MK . Annual Research Review: Suicide among youth - epidemiology, (potential) etiology, and treatment. J Child Psychol Psychiatry. (2018) ;59: (4):460–82. |
[4] | Pan LA , Phillips ML . Toward identification of neural markers of suicide risk in adolescents. Neuropsychopharmacology. (2014) ;39: (1):236–7. |
[5] | van Heeringen K , Bijttebier S , Desmyter S , Vervaet M , Baeken C . Is there a neuroanatomical basis of the vulnerability to suicidal behavior? A coordinate-based meta-analysis of structural and functional MRI studies. Front Hum Neurosci. (2014) ;8: :824. |
[6] | Bani-Fatemi A , Tasmim S , Graff-Guerrero A , Gerretsen P , Strauss J , Kolla N , et al. Structural and functional alterations of the suicidal brain: An updated review of neuroimaging studies. Psychiatry Res Neuroimaging. (2018) . |
[7] | Olie E , Ding Y , Le Bars E , de Champfleur NM , Mura T , Bonafe A , et al. Processing of decision-making and social threat in patients with history of suicidal attempt: A neuroimaging replication study. Psychiatry Res. (2015) ;234: (3):369–77. |
[8] | Pan LA , Batezati-Alves SC , Almeida JR , Segreti A , Akkal D , Hassel S , et al. Dissociable patterns of neural activity during response inhibition in depressed adolescents with and without suicidal behavior. J Am Acad Child Adolesc Psychiatry. (2011) ;50: (6):602–11 e3. |
[9] | Pan LA , Hassel S , Segreti AM , Nau SA , Brent DA , Phillips ML . Differential patterns of activity and functional connectivity in emotion processing neural circuitry to angry and happy faces in adolescents with and without suicide attempt. Psychol Med. (2013) ;43: (10):2129–42. |
[10] | Pan L , Segreti A , Almeida J , Jollant F , Lawrence N , Brent D , et al. Preserved hippocampal function during learning in the context of risk in adolescent suicide attempt. Psychiatry Res. (2013) ;211: (2):112–8. |
[11] | Chase HW , Segreti AM , Keller TA , Cherkassky VL , Just MA , Pan LA , et al. Alterations of functional connectivity and intrinsic activity within the cingulate cortex of suicidal ideators. J Affect Disord. (2017) ;212: :78–85. |
[12] | Glenn CR , Kleiman EM , Cha CB , Deming CA , Franklin JC , Nock MK . Understanding suicide risk within the Research Domain Criteria (RDoC) framework: A meta-analytic review. Depression and anxiety. (2018) ;35: (1):65–88. |
[13] | Jollant F , Lawrence NS , Giampietro V , Brammer MJ , Fullana MA , Drapier D , et al. Orbitofrontal cortex response to angry faces in men with histories of suicide attempts. Am J Psychiatry. (2008) ;165: (6):740–8. |
[14] | Vanyukov PM , Szanto K , Siegle GJ , Hallquist MN , Reynolds CF 3rd , Aizenstein HJ , et al. Impulsive traits and unplanned suicide attempts predict exaggerated prefrontal response to angry faces in the elderly. Am J Geriatr Psychiatry. (2015) ;23: (8):829–39. |
[15] | Just MA , Pan L , Cherkassky VL , McMakin D , Cha C , Nock MK , et al. Machine learning of neural representations of suicide and emotion concepts identifies suicidal youth. Nat Hum Behav. (2017) ;1: :911–9. |
[16] | First MB , Spitzer RL , Gibbon M , Williams JB . Structured Clinical Interview for DSM-IV Axis I Disorders-Patient Edition (SCID-I/P, Version 2.0). New York: Biometrics Research Department, New York State Psychiatric Institute; (1995) . |
[17] | Posner K , Oquendo MA , Gould M , Stanley B , Davies M . Columbia Classification Algorithm of Suicide Assessment (C-CASA): classification of suicidal events in the FDA’s pediatric suicidal risk analysis of antidepressants. Am J Psychiatry. (2007) ;164: (7):1035–43. |
[18] | Kaufman J , Birmaher B , Brent D , Rao U , Flynn C , Moreci P , et al. Schedule for Affective Disorders and Schizophrenia for School-Age Children-Present and Lifetime Version (K-SADS-PL): Initial reliability and validity data. J Am Acad Child Adolesc Psychiatry. (1997) ;36: (7):980–8. |
[19] | Beck AT , Schuyler D , Herman I . Development of suicidal intent scales. In: Beck AT, Resnik HLP, Lettieri DJ, editors. The prediction of suicide. Bowie, MD: Charles Press; (1974) . pp. 45–56. |
[20] | Oquendo MA , Halberstam B , Mann JJ . Risk factors for suicidal behavior: Utility and limitations of research instruments. In: First MB, editor. Standardized Evaluation in Clinical Practice. Washington, DC.: American Psychiatric Press; (2003) . pp. 103–30. |
[21] | Beck AT , Ward C , Mendelson M . Beck Depression Inventory (BDI). Arch Gen Psychiatry. (1961) ;4: :561–71. |
[22] | Birmaher B , Khetarpal S , Brent D , Cully M , Balach L , Kaufman J , et al. The Screen for Child Anxiety Related Emotional Disorders (SCARED): Scale construction and psychometric characteristics. J Am Acad Child Adolesc Psychiatry. (1997) ;36: (4):545–53. |
[23] | Reynolds W . Suicidal ideation questionnaire (SIQ). Odessa, FL, (1987) . |
[24] | Petersen AC , Crockett L , Richards M , Boxer A . A self-report measure of pubertal status: Reliability, validity, and initial norms. J Youth Adolesc. (1988) ;17: (2):117–33. |
[25] | Bernstein DP , Stein JA , Newcomb MD , Walker E , Pogge D , Ahluvalia T , et al. Development and validation of a brief screening version of the Childhood Trauma Questionnaire. Child Abuse Negl. (2003) ;27: (2):169–90. |
[26] | Patton JH , Stanford MS , Barratt ES . Factor structure of the Barratt impulsiveness scale. J Clin Psychol. (1995) ;51: (6):768–74. |
[27] | Tottenham N , Tanaka JW , Leon AC , McCarry T , Nurse M , Hare TA , et al. The NimStim set of facial expressions: Judgments from untrained research participants. Psychiatry Res. (2009) ;168: (3):242–9. |
[28] | Bertocci MA , Bebko GM , Mullin BC , Langenecker SA , Ladouceur CD , Almeida JR , et al. Abnormal anterior cingulate cortical activity during emotional n-back task performance distinguishes bipolar from unipolar depressed females. Psychol Med. (2012) ;42: (7):1417–28. |
[29] | Fournier JC , Chase HW , Almeida J , Phillips ML . Model Specification and the Reliability of fMRI Results: Implications for Longitudinal Neuroimaging Studies in Psychiatry. Plos One. (2014) ;9: (8). |
[30] | Duncan J , Owen AM . Common regions of the human frontal lobe recruited by diverse cognitive demands. Trends Neurosci. (2000) ;23: (10):475–83. |
[31] | Dosenbach NU , Fair DA , Miezin FM , Cohen AL , Wenger KK , Dosenbach RA , et al. Distinct brain networks for adaptive and stable task control in humans. Proc Natl Acad Sci U S A. (2007) ;104: (26):11073–8. |
[32] | Laird AR , Riedel MC , Okoe M , Jianu R , Ray KL , Eickhoff SB , et al. Heterogeneous fractionation profiles of meta-analytic coactivation networks. Neuroimage. (2017) ;149: :424–35. |
[33] | Quevedo K , Ng R , Scott H , Martin J , Smyda G , Keener M , et al. The neurobiology of self-face recognition in depressed adolescents with low or high suicidality. J Abnorm Psychol. (2016) ;125: (8):1185–200. |
[34] | Ai H , van Tol MJ , Marsman JC , Veltman DJ , Ruhe HG , van der Wee NJA , et al. Differential relations of suicidality in depression to brain activation during emotional and executive processing. J Psychiatr Res. (2018) ;105: :78–85. |
[35] | Malhi GS , Das P , Outhred T , Gessler D , John Mann J , Bryant R . Cognitive and emotional impairments underpinning suicidal activity in patients with mood disorders: An fMRI study. Acta Psychiatr Scand. (2019) ;139: (5):454–63. |
[36] | Miller AB , McLaughlin KA , Busso DS , Brueck S , Peverill M , Sheridan MA . Neural Correlates of Emotion Regulation and Adolescent Suicidal Ideation. Biol Psychiatry Cogn Neurosci Neuroimaging. (2018) ;3: (2):125–32. |
[37] | Raab K , Kirsch P , Mier D . Understanding the impact of 5-HTTLPR, antidepressants, and acute tryptophan depletion on brain activation during facial emotion processing: A review of the imaging literature. Neurosci Biobehav Rev. (2016) ;71: :176–97. |