Selection of ergonomic risk assessment method with pythagorean fuzzy sets: practice in emergency medical services
Abstract
It is difficult to evaluate ergonomic risk factors in occupations with unpredictable tasks, random demands, and variable settings such as emergency medical services (EMS). This study deals with the problem of selecting an ergonomic risk-evaluation method with Pythagorean Fuzzy Sets (PFSs) based Pythagorean Fuzzy AHP (PF-AHP) and Pythagorean Fuzzy WASPAS (PF-WASPAS) methodology. The method selection criteria were obtained by consulting five different anonymous experts on the candidate criteria obtained from the literature review. The final four main criteria and ten sub-criteria were then decided. After the determination of the decision criteria, five experts were asked to evaluate the criteria and to express their opinions on criteria-alternative scoring by means of a questionnaire for method selection. A two-step method is suggested for the selection of the ergonomic risk-evaluation method. In the first step, PF-AHP is utilized in order to identify the weight of criteria used in the method selection. In the second step, the PF-WASPAS method is proposed in order to OWAS, RULA, and REBA methods. The accuracy and validity of the suggested hybrid model is tested with real data in İstanbul Ambulance Service stations. A sensitivity analysis is carried out to test the reliability of the model. Moreover a comparative analysis is carried out with AHP and Fuzzy AHP methods to identify criteria weights. Study results show that REBA is the most appropriate ergonomic risk-evaluation method in EMS.
1Introduction
Musculoskeletal system disorders (MSDs) involve damage and disorders that occur in joints and other tissues and affect the back, neck, shoulders, and extremities [1]. According to the World Health Organization, MSDs are the leading source of disability in the workplace in developed countries [2]. In the 2019 report of the European Occupational Health and Safety Agency, three of five employees on average report MSDs, while 60% of all employees who have an occupational health problem define MSDs as their most significant problem [3]. Kee [4] reveals in his study that 9440 occupational MSD cases were reported in Korea in 2019, and that these cases constituted around one-third of all occupational diseases (67.3%) of that year.
As in other sectors, emergency medical service (EMS) staff encounter complications due to occupational MSDs [5]. Back pain is the most prevalent problem among them and the risk of waist injury is approximately 13 times higher than among nurses [6]. Current studies emphasize that the most common MSD prevention policy is the implementation of intervention programs to reduce exposure to risk factors [4]. The observational method, one of the ergonomic risk assessment methods, is still the most common approach for assessing physical workload, identifying exposure risks, and monitoring ergonomic enhancement [7].
A review of observational method studies in the field of emergency medical service shows us three methods: the Ovako Working Posture Analyzing System (OWAS) [8], the Rapid Upper Limb Assessment (RULA) [9], and the Rapid Entire Body Assessment Method (REBA) [10]. OWAS was developed by 32 steel workers and ergonomists analyzing the postures exemplified in a steel factory [8]. OWAS divides possible postures into three categories: back, arms, and legs. RULA is a method developed to assess the load on the musculoskeletal system, and particularly to analyze upper-extremity postures. This method analyzes the neck, body, upper extremity (upper arm, lower arm, wrist), and leg postures with applied load-force and muscle activity, assigning a score [9]. REBA is a posture analysis method developed by Hignett and McAtamney to be sensitive to the type of unpredictable working postures. It analyzes body posture by adding coupling scores to the upper extremity (upper arm, lower arm, wrist), and load-force values to neck, body, and leg posture scores [10].
Assessing ergonomic risk factors is a difficult endeavor for jobs such as EMS, due to unpredictable tasks, random demands, changing medical equipment, and cases. Therefore, considering the limited time and emergency of patients, it is inevitable to choose the most appropriate method to assess the staff’s exposure and identify the cases that require ergonomic enhancement. Thus, the process of choosing the most appropriate ergonomic risk factor assessment method among the aforementioned three methods and their different variables breeds multi-criteria decision-making problems. The Analytical Hierarchy Process (AHP), one of the MCDM methods, is the most common method in literature used to analyze and order criteria by means of data analysis [11]. In AHP analysis, the input is likely to be uncertain to an extent, and the data are based on opinions [12].
Fuzzy sets presented by Zadeh (1965) have been integrated with AHP to cope with vagueness and impreciseness [13]. Intuitive fuzzy sets (IFs) proposed by Atanasov (1986) have considered the uncertainty deal with membership function, non-membership function, and hesitancy degrees [14]. The two membership functions and hesitation degree in the IFs have limitations in terms of their arithmetic addition particularly. The sum of the two membership functions of the IFs is limited to one. It puts forth that the IFs cannot address the circumstances that the sum of two-membership functions is higher than one. Yager [2013] presented a Pythagorean fuzzy set (PFs) to handle this limitation [15]. Pythagorean fuzzy sets provide more freedom to decision makers in expressing their opinions regarding vagueness and uncertainty (11). Because, for the PFs, the sum of the two-membership function can be more than one, but the sum of two membership functions cannot be more than one [16]. Therefore, PFSs are more powerful to handle uncertainty and vague information than IFs [17].
In this study, we utilized the Analytical Hierarchy Process (AHP), which is one of the most popular MCDM methods, to determine the weight of the main and sub-criteria. However, the AHP method is criticized for its incapability to handle uncertainty in human judgments [18]. The use of the fuzzy set theory in AHP helps in capturing the vagueness in preference [19]. That’s why we used Pythagorean fuzzy sets, which have the advantages mentioned in the previous paragraph, based on AHP methods due to eliminating the disadvantages of AHP.
Weighted Aggregated Sum Product Assessment (WASPAS) is the combination of the Weighted Sum Model (WSM) and the Weighted Product Model (WPM) [11]. The advantage of the WSM method is the easy evaluation of alternatives using the weighted Sum, while the WMP prevents obtaining solutions with low values. The WASPAS method combines the advantages of both the WSM and WPM methods [20]. Furthermore, the WASPAS method provides a higher level of accuracy than the WSM and WPM methods, accordingly [21]. The WASPAS method also presents the following advantages: the steps of the method are short and easy and it is useful for ranking alternatives completely and as a whole. In this study, PF-WASPAS is presented for the ranking of alternatives. Accordingly, an extended version of WASPAS is developed, while simultaneously considering the PFSs advantages.
In light of previous studies, the main motivations for this study are the following:
– This study aims to present a reliable and valuable model to select an ergonomic risk assessment method, in order to assess work-related musculoskeletal system loads of employees based on the criteria identified specifically for emergency medical services.
– There is no study in the literature related to the most appropriate ergonomic risk factor assessment method selection. Despite its importance, this topic has not been addressed.
– In previous studies on the ergonomic risk-assessment method selection, the fuzzy state set was ignored. They have introduced their model under the context that cannot handle the vague, and uncertain information effectively. One of the motivations of this study is to introduce a novel multi-criteria decision-making approach based on Pythagorean fuzzy numbers, in order to consider information uncertainty.
The goal of this study is to identify the most appropriate ergonomic risk assessment method among the three observation methods used in the field to assess work-related musculoskeletal system loads of employees, based on the criteria identified specifically for emergency medical services. A two-step method was suggested in this study for the selection of the ergonomic risk-evaluation method. In the first step, the Pythagorean Fuzzy AHP (PF-AHP) is applied to determine the weight of the criteria. In the second step, the Pythagorean Fuzzy WASPAS (PF-WASPAS) method is employed in order to rank the ergonomic risk-evaluation method.
The main contributions of this study are as follows:
– To the best of the authors’ knowledge, this is the first study that presents a comprehensive, reliable and valuable framework to select an ergonomic risk assessment method to evaluate work-related musculoskeletal system loads of emergency medical services employees.
– To the best of the authors’ knowledge, this is the first study that hybridizes the AHP and WASPAS methods based on PFs to select the most appropriate ergonomic risk assessment method for the study of work-related musculoskeletal system loads of employees for emergency medical services.
– The proposed PF-AHP & PF-WASPAS method copes with uncertainty by enabling decision-makers to express their opinions more flexibly.
– A sensitivity analysis is performed to reveal the robustness of the suggested method to the variation. And also to verify the validity of the introduced method, we compared the PF-AHP& PF-WASPAS approach with the proposed F-AHP& PF-WASPAS, and AHP&PF-WASPAS approach, respectively.
This study contains five sections after the introduction. Section 2 presents a literature review on observational methods in the field of emergency medical service, while Section 3 explains the methodology deployed in the study. Next, in Section 4.1, the suggested model is applied in a real-world case study. Then, Section 4.2 provides a sensitivity analysis. Lastly, Section 5 concludes the research and presents future research directions.
2Literature review
In this section, firstly, we investigated studies in the literature related to Ergonomic Risk Assessment Methods and studies that deploy PF-AHP and PF-WASPAS methods. Secondly, we discussed the literature gaps.
2.1Literature description
Studies that use observational methods in the field of EMS are presented below. Doormaal et al. [22] assess physical workload of ambulance assistants with OWAS method. Ferreira and Hignett [23] observed ambulance workers in 16 shifts (130 hours) and applied the OWAS method. Gentzler and Stader [24], analyzed the working postures of American firefighters and emergency medical technicians with REBA and RULA. Deros et al. [5] studied MSD risk factors of EMS staff in Malaysia with REBA method. Verjans et al. [25] used OWAS method to identify workload in EMS during patient transfer and associated it with a survey. Kahya and Sakarya [26] used Cornell survey and REBA methods to analyze physical strain during treatment and care.
There are also studies in literature based on the comparative analysis of methods used for measuring postural stress. For example, Genaidy et al. [27] classified observational methods used in postural stress analysis, macro postural, micro postural and postural work activity. Juul-Kristensen et al. [28] assessed eight observational methods according to the criteria used in the classification of body angles and motion intervals. Denis et al. [29] categorized the proposed observation procedures to characterize physical work with 7 observational variables. Takala et al. [7] developed a systematic assessment procedure to evaluate simultaneous and estimation-based validity, repeatability and utility of methods. Roman-Liu [30] grouped 10 methods according to their characteristics. Sukadarin et al. [31] displayed the strengths and weaknesses of the observational methods.
In the second section of the literature review, we investigated studies that employed PF-AHP and PF-WASPAS. We employed the PFSs-based AHP and WASPAS methods in this study. The Analytical Hierarchy Process (AHP) is one of the most popular MCDM methods to determine the weight of main and sub-criteria. The use of fuzzy set theory in AHP helps capture the vagueness in preference [19]. In the literature, the fuzzy set theory has been widely utilized to select problems that consider information uncertainty [32]. However, the AHP method is criticized for its incapability to handle uncertainty in human judgments [18]. That is why we used the PFSs-based AHP methods to eliminate the disadvantages of AHP.
We also used WASPAS, which is a combination of the Weighted Sum Model (WSM) and the Weighted Product Model (WPM) [11]. The advantage of the WSM method is the easy evaluation of alternatives using the weighted sum, while the WMP method prevents obtaining solutions with low values. The WASPAS method combines the advantages of both methods [20]. The WASPAS method provides an optimal level of accuracy rather than the WSM and WPM methods [21]. The WASPAS method’s advantages are as follow: The calculation steps of the method are short and easy, and it is useful for listing alternatives completely and as a whole. The method tries to achieve the highest level of accuracy compared to the WSM and WPM methods. The PF-WASPAS provides both advantages of the WASPAS and Pythagorean fuzzy sets. In this study, PF-WASPAS is presented for the ranking of alternatives. Accordingly, an extended version of WASPAS is developed, while simultaneously considering the PFSs advantages. Appendix 1 shows the prominent studies that utilize PFs-based PF-AHP and PF-WASPAS methods.
2.2Research gaps
When the literature studies are examined, OWAS, RULA, and REBA methods are frequently used for ergonomic risk assessment. However, there is no study on the most appropriate ergonomic risk assessment method and, no list of selection criteria. The gap in the literature in this area is evident. Furthermore, previous studies related to ergonomic risk assessment comprise no application for emergency medical services.
The current research attempts related to ergonomic risk assessment and fuzzy multi-criteria decision-making are dispersed in different sectors, such as transportation, renewable energy, occupational health and safety, refugee camp location selection, and supply chain. We addressed the ergonomic risk evaluation method selection for the emergency medical service. Despite its strategic importance, this topic has not been addressed.
Therefore, in this study, we propose a novel PFs -based method to determine the most appropriate observational ergonomic risk assessment method in the field of emergency medical services.
3Methodology
3.1Preliminaries of Pythagorean fuzzy sets
Since they were suggested by Atanassov [14] intuitive fuzzy sets have been used by researchers in order to deal with uncertainty [45]. Pythagorean fuzzy set (PFS) is a generalization of the intuitive fuzzy set and is used in modeling uncertain data at the decision-making stage [50]. These sets have more flexibility than intuitive fuzzy sets in expressing uncertainty and fuzziness during MCDM process [51]. As an extension of intuitive fuzzy sets, the goal of Pythagorean fuzzy sets is to help decision-makers bypass the membership and non-membership obligation forced upon them, the sum of which must be maximum 1 [11]. Contrary to intuitive fuzzy sets, the sum of membership and non-membership might exceed 1 in Pythagorean fuzzy sets while the sum of squares cannot [11, 16].
Definition 1: Take X as a fixed set. A Pythagorean fuzzy set
(1)
Where the degree of membership is defined by the function
(2)
Here, also the degree of hesitancy condition is as follows:
(3)
Definition 2: Let =
(4)
(5)
(6)
(7)
Definition 3: Let
(8)
3.2The presented PF-AHP and PF-WASPAS methodology
This study proposes a two-stage method for selecting the most appropriate ergonomic risk-evaluation method. In the first stage, PF-AHP is used to determine the weight of the criteria. In the second stage, the PF-WASPAS method is employed to rank alternatives. The suggested PF-AHP&FP-WASPAS Model is presented in Fig. 1.
Fig. 1
The suggested PF-AHP&FP-WASPAS Model.
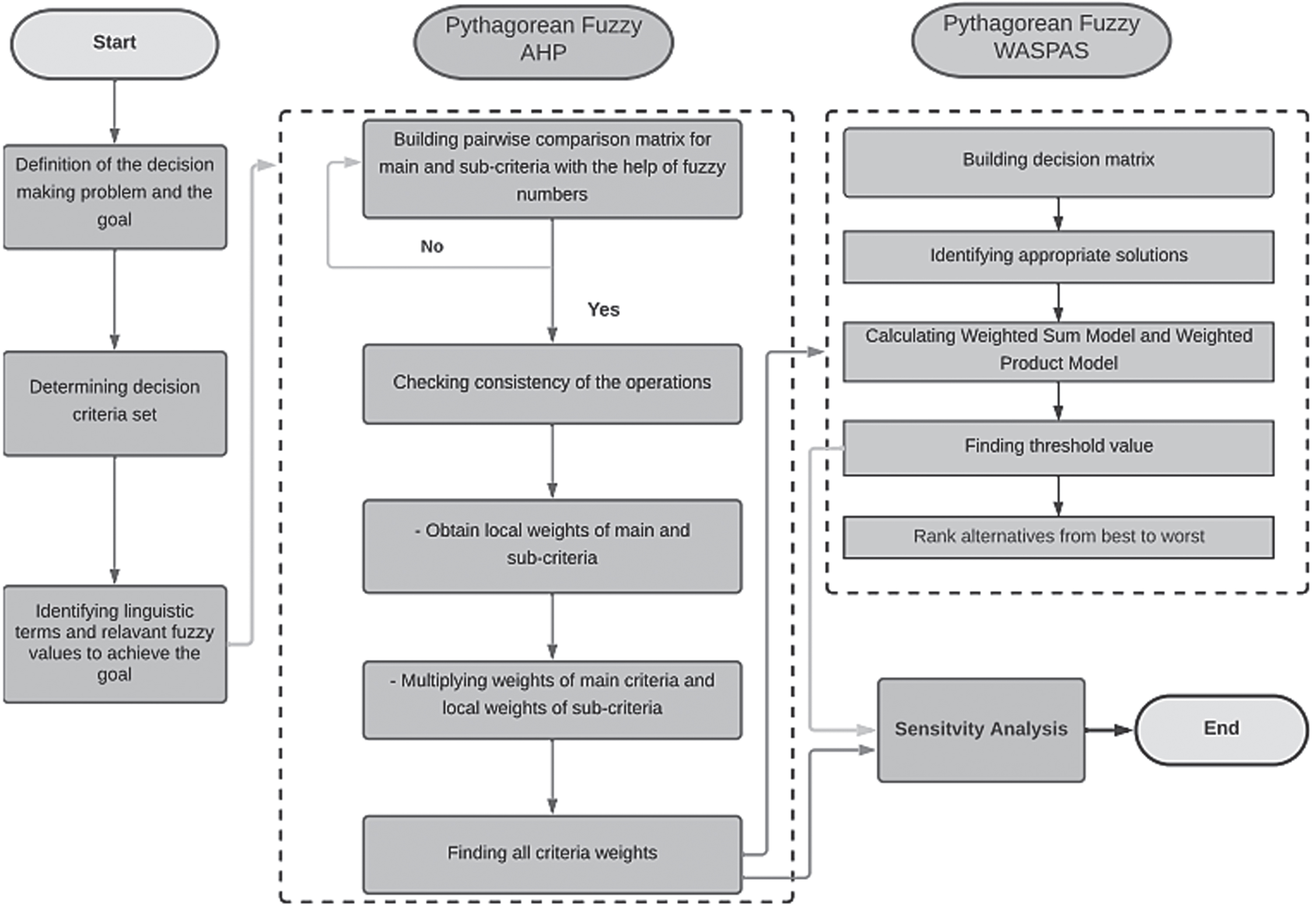
First Stage: Find criteria weight with PF-AHP
Steps of the presented PH-AHP to determine criteria weight as follows [12].
Step 1: This step includes defining the problem and the hierarchy construction of the goals, criteria, sub-criteria, and alternatives.
Step 2: Construct Based on the inputs received from the experts, build a pairwise comparison matrix means of linguistic terms as displayed in Table 1 [11].
(9)
Table 1
Weighing scale for the Pythagorean fuzzy AHP [38]
Linguistic terms | PFN equivalents IVP numbers | |||
μL | μU | vL | vU | |
Certainly Low Importance –CLI | 0 | 0 | 0.9 | 1 |
Very Low Importance –VLI | 0.1 | 0.2 | 0.8 | 0.9 |
Low Importance –LI | 0.2 | 0.35 | 0.65 | 0.8 |
Below Average Importance –BAI | 0.35 | 0.45 | 0.55 | 0.65 |
Average Importance –AI | 0.45 | 0.55 | 0.45 | 0.55 |
Above Average Importance –AAI | 0.55 | 0.65 | 0.35 | 0.45 |
High Importance –HI | 0.65 | 0.8 | 0.2 | 0.35 |
Very High Importance –VHI | 0.8 | 0.9 | 0.1 | 0.2 |
Certainly High Importance –CHI | 0.9 | 1 | 0 | 0 |
Equal importance (EI) | 0.5 | 0.5 | 0.5 | 0.5 |
Step 3: Using the lower and upper values of the membership and non-membership functions, compute the differences matrix D = (dik) m×n using Equations 10 and 11:
(10)
(11)
Step 4: Compute the interval multiplicative matrix S = (sik) m×n using Equations 12 and 13:
(12)
(13)
Step 5: Compute the determinacy value τ = (τik) m×n of the xik using Equation 14:
(14)
Step 6: Determine the matrix of weights, T = (tik) m×m, before normalization by multiplying the determinacy degrees with S = (sik) m×m with matrix using Equation 15:
(15)
Step 7: Calculate the normalized priority weights, wi using Equation 16:
(16)
Second stage: Rank each alternative with the PF- WASPAS method
The WASPAS (Weighted Aggregated Sum Product Assessment) was introduced in literature by Zavadskas et al. [55]. The Interval-Valued Pythagorean Fuzzy WASPAS (IVPF-WASPAS) is the integration of the Pythagorean fuzzy sets and the WASPAS method [33]. With the help of a linguistic scale, decision-makers assess certain alternatives in terms of several criteria. Decision-making often requires aggregating the judgment of decision-makers in a single matrix [56] before carrying out the relevant calculations required by the suggested method.
Step 8. Decision makers are asked for their judgments in linguistic form and transformed into interval valued Pythagorean fuzzy numbers (IVPFNs) with the scale presented in Table 2. Equation 17 presents these IVPFNs aggregated with the interval-valued Pythagorean fuzzy weighted average (IVPFWA) operator [57]. Where n is the number of decision-makers.
Table 2
An IVPFN scale to rate alternatives with respect to criteria [49]
Linguistic terms | μL | μU | vL | vU |
Very very bad— VVB | 0.03 | 0.18 | 0.75 | 0.90 |
Very bad— VB | 0.12 | 0.27 | 0.66 | 0.81 |
Bad— B | 0.21 | 0.36 | 0.57 | 0.72 |
Medium bad— MB | 0.3 | 0.45 | 0.48 | 0.63 |
Fair— F | 0.39 | 0.54 | 0.39 | 0.54 |
Medium good— MG | 0.48 | 0.63 | 0.30 | 0.45 |
Good— G | 0.57 | 0.72 | 0.21 | 0.35 |
Very good— VG | 0.66 | 0.81 | 0.12 | 0.27 |
Very very good— VVG | 0.75 | 0.90 | 0.03 | 0.18 |
(17)
Step 9. To normalize the values in the aggregated decision matrix as in Equations 19–20, maxi pij must be obtained using the defuzzification formula presented in Equation 18. This defuzzification formula is developed based on the formula used in [58] for the defuzzification of interval valued intuitionistic fuzzy numbers. After obtaining 1/(maxi pij), Equation 21 is used to calculate the multiplication of a crisp number and an IVPFN.
(18)
(19)
(20)
(21)
Step 10. Criteria weights are obtained in linguistic terms and the corresponding IVPFNs provided in Table 3. The aggregation of these IVPFNs is carried out by using Equation 17.
Table 3
Linguistic terms to rate the importance of criteria [41]
Linguistic Term | μL | μU | νL | νU |
Very important (VI) | 0.70 | 0.90 | 0.06 | 0.26 |
Important (I) | 0.54 | 0.74 | 0.22 | 0.42 |
Medium (M) | 0.38 | 0.58 | 0.38 | 0.58 |
Unimportant (U) | 0.22 | 0.42 | 0.54 | 0.74 |
Very unimportant (VU) | 0.06 | 0.26 | 0.70 | 0.90 |
Step 11. The calculation of Pythagorean fuzzy weighted sum values,
(22)
(23)
(24)
Step 12. The calculation of the Pythagorean fuzzy weighted product values,
(25)
(26)
Step 13.
(27)
Step 14.
4Application
4.1Selection ergonomic risk assessment method for emergency medical services
The choice of method to analyze the musculoskeletal loads arising from working postures is made according to the purposes of use, the characteristics of the work to be evaluated, the person who will use
the method, and the rources (7). Therefore, method selection is a multidimensional and slippery problem. First of all, a detaid literature review was conducted for the method section problem. Decision criteria were determined by literature review. In the decision criteria, the final decision was made by consulting five different anonymous experts. Experts were selected based on their experience in occupational health and safety practices in 112 emergency health services.
Within the scope of the study, four main criteria were determined. These are general and difficult to evaluate directly, so ten sub-criteria have been decided for the selection of the observation method. After the determination of the decision criteria, five experts were asked to evaluate the criteria and express their opinions on criteria-alternative scoring in a questionnaire for method selection.
Table 4 shows the criteria used to select the most appropriate observation method to analyze the musculoskeletal loads resulting from the working postures of 112 emergency health workers, and the references and definitions of these criteria.
Table 4
Criteria, sub-criteria and definitions
Main Criteria | Sub-criteria | References | Definition |
Entry Parameter (C1) | Reference (C1.1) | [7, 30, 59, 60] | The method is easy to use for implementers, has no data requirement is a simple and comprehensive method |
Versatility (C1.2) | [27, 30, 31, 59] | The method enables assessment of the whole body, including upper and lower organs | |
Data Range(C1.3) | [30, 31] | The number of angles and value range of the method is sufficient to define many various postures | |
Force Applied (C2) | Load (C2.1) | [30, 31, 59] | The method takes the force applied into account and load classification range is sufficient in identification of the load |
Repetition (C2.2) | [28, 30, 59, 61] | The method considers the frequency of repetition in the analysis of musculoskeletal load | |
Final Assessment (C3) | Risk Output (C3.1) | [7, 30] | Risk output of the method is related to the musculoskeletal load of the posture |
Prioritization (C3.2) | [7, 30, 59] | The method identifies the priorities for reducing the risks and load of musculoskeletal system disorders | |
Utility (C4) | Universality-Prevalence(C4.1) | [59, 61] | The sector is commonly used in the sector |
Repeatability (C4.2) | [7, 30, 61] | Results are consistent when the method is repeated in the same observer and with the same working posture | |
Flexibility (C4.3) | [59, 60] | The method is flexible enough to carry out multiple and complex tasks and comprehensive enough for a series of risk factors |
Step 1: This step includes defining the problem and the hierarchy construction of the goals, criteria, sub-criteria and alternatives. The decision hierarchy of the method selection problem is presented in Fig. 2.
Fig. 2
Decision hierarchy of method selection problem.
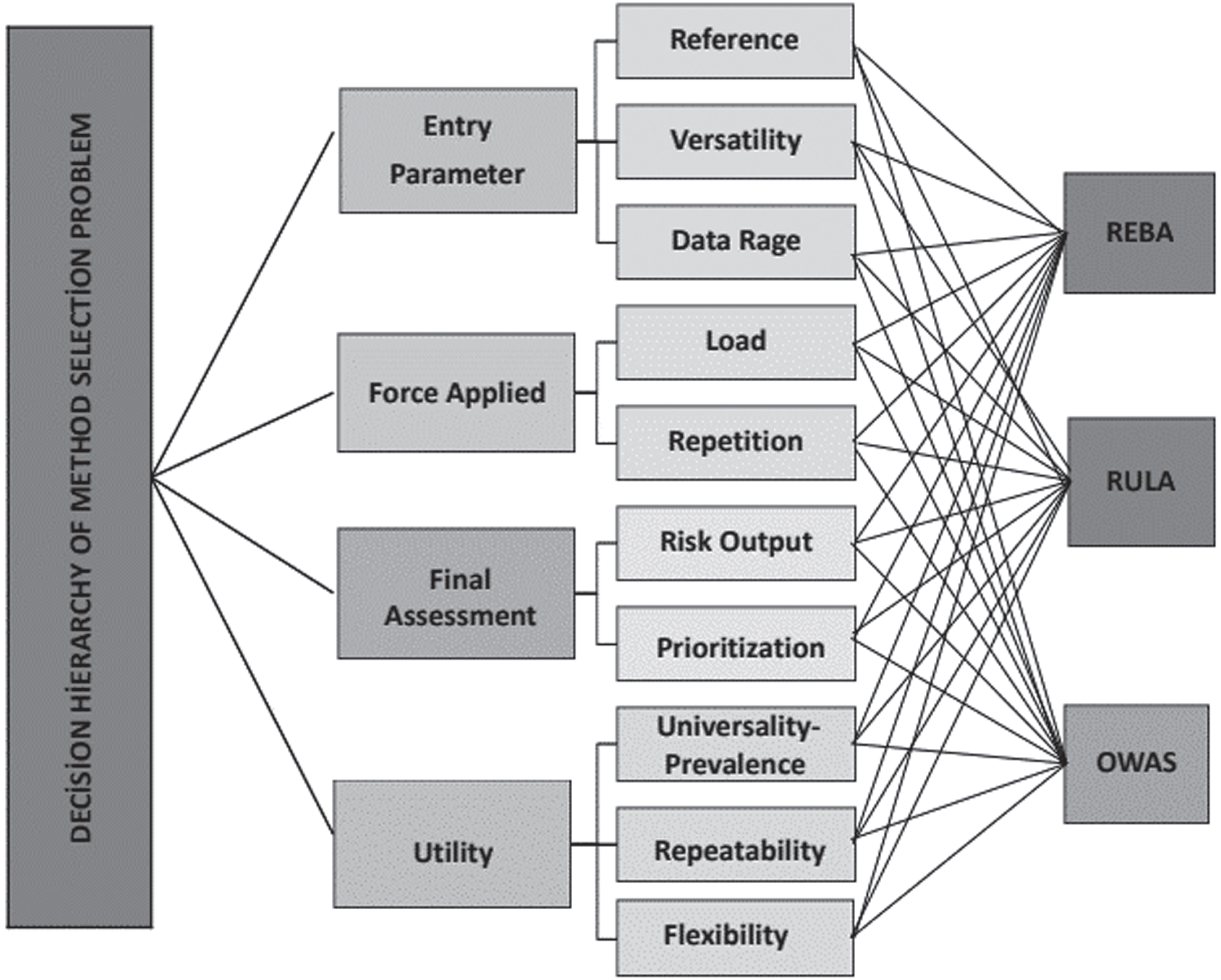
Step 2: İt is developed into a pairwise comparison matrix based on the inputs received from the experts panel using linguistic terms as given in Table 5 for main criteria and Appendix 2-3-4-5 for the sub-criteria, respectively.
Table 5
The aggregated pairwise comparison matrix for the main criteria
C1 | C2 | C3 | C4 | |||||||||||||
ML | MU | VL | VU | ML | MU | VL | VU | ML | MU | VL | VU | ML | MU | VL | VU | |
C1 | 0.500 | 0.500 | 0.500 | 0.500 | 0.385 | 0.523 | 0.385 | 0.523 | 0.359 | 0.484 | 0.429 | 0.554 | 0.000 | 0.000 | 0.411 | 0.547 |
C2 | 0.487 | 0.617 | 0.304 | 0.443 | 0.500 | 0.500 | 0.500 | 0.500 | 0.366 | 0.475 | 0.438 | 0.544 | 0.209 | 0.336 | 0.643 | 0.746 |
C3 | 0.429 | 0.554 | 0.359 | 0.484 | 0.438 | 0.544 | 0.366 | 0.475 | 0.500 | 0.500 | 0.500 | 0.500 | 0.617 | 0.728 | 0.240 | 0.376 |
C4 | 0.000 | 0.000 | 0.520 | 0.645 | 0.643 | 0.746 | 0.209 | 0.336 | 0.240 | 0.376 | 0.617 | 0.728 | 0.500 | 0.500 | 0.500 | 0.500 |
Step 3: The lower and upper values of the membership and non-membership for main and sub-criteria calculated as given in Appendix 6–10.
Step 4: Compute the interval multiplicative matrix estimated as given in Appendix 11–15.
Step 5: The determinacy value is computed as shown in Appendix 16–20.
Step 6: The matrix of weights before normalization is calculated in Appendix 21–25.
Step 7: It is estimated the normalized priority weights as depicted in Table 6.
Table 6
Priority weights of the main and sub-criteria
Weights | Local weights | global weights | ||
Entry Parameter (C1) | 0.169 | Reference (C1.1) | 0.265 | 0.04 |
Versatility (C1.2) | 0.412 | 0.07 | ||
Data Range (C1.3) | 0.323 | 0.05 | ||
Force Applied (C2) | 0.195 | Load (C2.1) | 0.435 | 0.08 |
Repetition (C2.2) | 0.565 | 0.11 | ||
Final Assessment (C3) | 0.347 | Risk Output (C3.1) | 0.427 | 0.15 |
Prioritization (C3.2) | 0.573 | 0.20 | ||
Utility (C4) | 0.289 | Universality-Prevalence (C4.1) | 0.309 | 0.09 |
Repeatability (C4.2) | 0.500 | 0.14 | ||
Flexibility (C4.3) | 0.191 | 0.06 |
According to Table 6, the most significant main criterion in selecting an ergonomic risk-evaluation method is the Final Assessment (0.347). In the main criteria, the most significant sub-criteria for the Entry Parameter is Versatility (0.412), Repetition for the Force Applied (0.565), Prioritization for the Final Assessment (0.573), and the Repeatability for Utility (0.5). The most significant 3 criteria in global weights are Prioritization (0.20), Risk Output (0.15) and Repeatability (0.14), respectively.
Step 8-9: In this step, we established the Pythagorean fuzzy decision matrix by criteria using the linguistic terms presented in Table 7 and the normalized decision matrix is given in Table 8.
Table 7
Aggregated decision matrix in the form of IVPFN
G1 | G2 | G3 | U1 | U2 | ||||||||||||||||
ML | MU | VL | VU | ML | MU | VL | VU | ML | MU | VL | VU | ML | MU | VL | VU | ML | MU | VL | VU | |
REBA | 0.678 | 0.828 | 0.102 | 0.252 | 0.678 | 0.828 | 0.102 | 0.252 | 0.660 | 0.810 | 0.120 | 0.270 | 0.516 | 0.666 | 0.264 | 0.414 | 0.552 | 0.702 | 0.228 | 0.378 |
RULA | 0.552 | 0.702 | 0.228 | 0.378 | 0.462 | 0.612 | 0.318 | 0.468 | 0.570 | 0.720 | 0.210 | 0.360 | 0.426 | 0.576 | 0.354 | 0.504 | 0.534 | 0.684 | 0.246 | 0.396 |
OWAS | 0.552 | 0.702 | 0.228 | 0.378 | 0.552 | 0.702 | 0.228 | 0.378 | 0.462 | 0.612 | 0.318 | 0.468 | 0.498 | 0.648 | 0.282 | 0.432 | 0.390 | 0.540 | 0.390 | 0.540 |
N1 | N2 | K1 | K2 | K3 | ||||||||||||||||
ML | MU | VL | VU | ML | MU | VL | VU | ML | MU | VL | VU | ML | MU | VL | VU | ML | MU | VL | VU | |
REBA | 0.624 | 0.774 | 0.156 | 0.306 | 0.606 | 0.756 | 0.174 | 0.324 | 0.678 | 0.828 | 0.102 | 0.252 | 0.606 | 0.756 | 0.174 | 0.324 | 0.624 | 0.774 | 0.156 | 0.306 |
RULA | 0.570 | 0.720 | 0.210 | 0.360 | 0.570 | 0.720 | 0.210 | 0.360 | 0.588 | 0.738 | 0.192 | 0.342 | 0.588 | 0.738 | 0.192 | 0.342 | 0.570 | 0.720 | 0.210 | 0.360 |
OWAS | 0.516 | 0.666 | 0.264 | 0.414 | 0.462 | 0.612 | 0.318 | 0.468 | 0.462 | 0.612 | 0.318 | 0.468 | 0.624 | 0.774 | 0.156 | 0.306 | 0.480 | 0.630 | 0.300 | 0.450 |
Table 8
Normalized decision matrix
G1 | G2 | G3 | U1 | U2 | ||||||||||||||||
ML | MU | VL | VU | ML | MU | VL | VU | ML | MU | VL | VU | ML | MU | VL | VU | ML | MU | VL | VU | |
REBA | 0.612 | 0.766 | 0.176 | 0.350 | 0.612 | 0.766 | 0.176 | 0.350 | 0.589 | 0.741 | 0.199 | 0.369 | 0.417 | 0.550 | 0.362 | 0.511 | 0.458 | 0.597 | 0.324 | 0.476 |
RULA | 0.492 | 0.636 | 0.324 | 0.476 | 0.409 | 0.548 | 0.418 | 0.561 | 0.504 | 0.648 | 0.304 | 0.459 | 0.341 | 0.469 | 0.453 | 0.593 | 0.442 | 0.579 | 0.343 | 0.494 |
OWAS | 0.492 | 0.636 | 0.324 | 0.476 | 0.492 | 0.636 | 0.324 | 0.476 | 0.405 | 0.543 | 0.418 | 0.561 | 0.401 | 0.534 | 0.381 | 0.527 | 0.318 | 0.447 | 0.488 | 0.625 |
N1 | N2 | K1 | K2 | K3 | ||||||||||||||||
ML | MU | VL | VU | ML | MU | VL | VU | ML | MU | VL | VU | ML | MU | VL | VU | ML | MU | VL | VU | |
REBA | 0.544 | 0.692 | 0.243 | 0.406 | 0.522 | 0.668 | 0.264 | 0.424 | 0.612 | 0.766 | 0.176 | 0.350 | 0.528 | 0.673 | 0.264 | 0.424 | 0.544 | 0.692 | 0.243 | 0.406 |
RULA | 0.494 | 0.637 | 0.304 | 0.459 | 0.489 | 0.632 | 0.304 | 0.459 | 0.526 | 0.672 | 0.284 | 0.441 | 0.511 | 0.655 | 0.284 | 0.441 | 0.494 | 0.637 | 0.304 | 0.459 |
OWAS | 0.445 | 0.584 | 0.362 | 0.511 | 0.392 | 0.528 | 0.418 | 0.561 | 0.409 | 0.548 | 0.418 | 0.561 | 0.544 | 0.692 | 0.243 | 0.406 | 0.413 | 0.550 | 0.399 | 0.544 |
Step 10: It can be seen the weighted sum model
Step 11: It can be seen the weighted product model
Step 12-13-14: İt is take the threshold value (λ) as 0.5. The weighted sum model and the weighted product models are combined with λ = 0.5. The Defuzzified weighted sum model, the defuzzified weighted product model and the results of each alternative can be seen in Table 9.
Table 9
Final score of each alternative
Alternatives | Defuzzified | Defuzzified | Final | Rank |
weighted | weighted | score | ||
sum model | product model | |||
REBA | 0.690 | 0.625 | 0.66 | 1 |
RULA | 0.613 | 0.568 | 0.59 | 2 |
OWAS | 0.566 | 0.525 | 0.55 | 3 |
According to Table 9, the highest score among three observation methods belongs to REBA (0.66).
4.2Sensitivity analysis
In this section, we conducted a sensitivity analysis to validate our framework. In the proposed approach, the weight of the main criteria plays a significant role in ranking the alternatives. That is why, first of all, the main criteria weights obtained from the PF-AHP are changed to test the accuracy of the results of the presented approach. For this purpose, six scenarios are considered as given in Table 10.
Table 10
Weights of the main criteria with different scenarios
![]() |
Figure 3 shows the effect of the main criteria weights on the perrmance scores of each alternative in the proposed method.
Fig. 3
Results of sensitivity analysis.
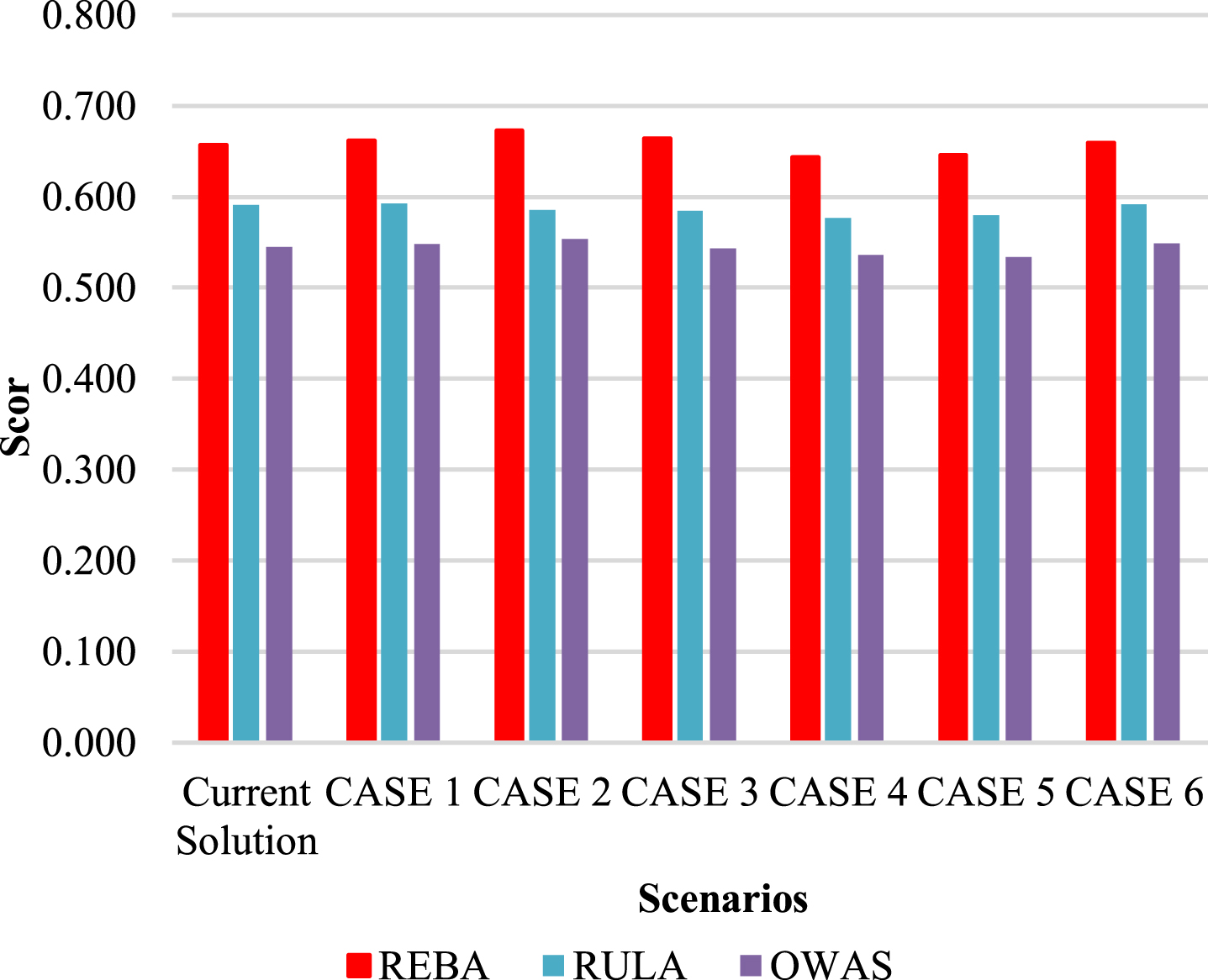
According to Fig. 3, the sensitivity analysis shows that the ranking of the alternatives is not sensitive to the changes, considering the weights of the main criteria. Thus, the presented model is robust in terms of the main criteria weights.
Secondly, the sensitivity analysis is performed by changing the threshold parameter (λ) in the process of the WASPAS method. Figure 4 shows the effect of λ parameter on the performance scores of each alternative in the presented PF-AHP&PF-WASPAS method.
According to Fig. 4, the result of the presented model is robust with different for the λ values.
Fig. 4
Final performance score with differentλ values for PF-AHP&PF-WASPAS model.
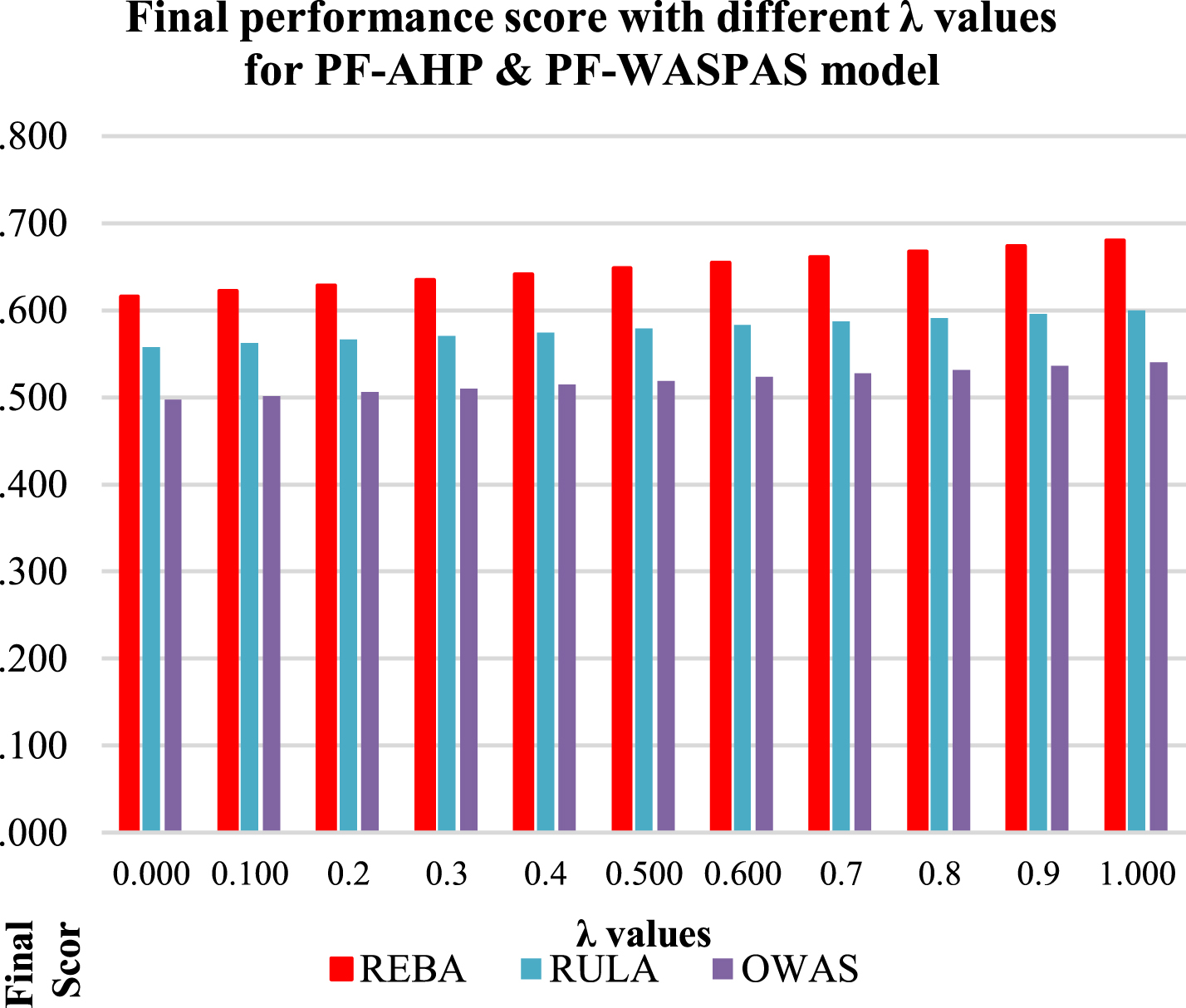
4.3Comparative analysis
In this section, the suggested PF-AHP&PF-WASPAS method AHP and F-AHP were compared. Table 11 displays the main criteria and sub-criteria weights calculated with AHP, F-AHP and PF-AHP. AHP, and F-AHP analysis also show that the most significant main criterion is Utility (0.40, 0.39, respectively). In terms of global weights, the most significant sub-criterion for AHP and F-AHP was found to be Repeatability (0.253, 0.250, respectively) and reliable results.
Table 11
Priority weights of the main and sub criteria
PF-AHP | AHP | F-AHP | PF-AHP | AHP | F-AHP | |||||
Main criteria | Weights | Sub-criteria | Local weights | Global weights | Local weights | Global weights | Local weights | Global weights | ||
Entry | ||||||||||
Parameter (C1) | 0.169 | 0.21 | 0.21 | Reference (C1.1) | 0.265 | 0.045 | 0.23 | 0.047 | 0.221 | 0.046 |
Versatility (C1.2) | 0.412 | 0.069 | 0.35 | 0.073 | 0.356 | 0.075 | ||||
Data Range (C1.3) | 0.323 | 0.055 | 0.42 | 0.087 | 0.423 | 0.089 | ||||
Force Applied (C2) | 0.195 | 0.24 | 0.24 | Load (C2.1) | 0.435 | 0.085 | 0.70 | 0.168 | 0.701 | 0.168 |
Repetition (C2.2) | 0.565 | 0.110 | 0.30 | 0.071 | 0.299 | 0.072 | ||||
Final Assessment (C3) | 0.347 | 0.15 | 0.16 | Risk Output (C3.1) | 0.427 | 0.148 | 0.55 | 0.084 | 0.553 | 0.088 |
Prioritization (C3.2) | 0.573 | 0.199 | 0.45 | 0.068 | 0.447 | 0.072 | ||||
Utility (C4) | 0.289 | 0.40 | 0.39 | Universality-Prevalence(C4.1) | 0.309 | 0.089 | 0.21 | 0.084 | 0.203 | 0.079 |
Repeatability (C4.2) | 0.500 | 0.144 | 0.63 | 0.253 | 0.641 | 0.250 | ||||
Flexibility (C4.3) | 0.191 | 0.055 | 0.16 | 0.065 | 0.155 | 0.060 |
Using the weights in Table 11, PF-WASPAS solutions were brought as Table 12. It is seen that results of 3 methods are almost the same. Thus, it is understood that the suggested PF-AHP&PF-WASPAS method yields consistent and reliable results.
Table 12
Final score of each alternative
PF-AHP&PF-WASPAS | AHP&PF-WASPAS | F-AHP&PF-WASPAS | ||||
Alternatives | Final score | Rank | Final score | Rank | Final score | Rank |
REBA | 0.66 | 1 | 0.65 | 1 | 0.65 | 1 |
RULA | 0.59 | 2 | 0.58 | 2 | 0.58 | 2 |
OWAS | 0.55 | 3 | 0.56 | 3 | 0.56 | 3 |
4.4Policy implications
In this study, a new method based on PF’s is proposed to specify the most appropriate observational ergonomic risk assessment method in the field of emergency health services. As a result of proposed method, it was found that REBA (0.66) was the most appropriate method for emergency health services among OWAS, RULA and REBA (Table 9). The result of this study is directly proportional to the ease of application in the field.
REBA is a postural analysis system sensitive to musculoskeletal risks in a variety of tasks, especially for the assessment of working postures in healthcare and other service sectors. We found that the cost-effectiveness ratio is good, the application is easy, and only pen and paper are sufficient for data collection. Even the most contradictory ergonomic aspects are determined from the individual score obtained after evaluating each part of the body (19).
Each technique has its own strengths and weaknesses depending on the assumptions made. However, unless some morbidity measures are analyzed, the best method to reflect the underlying risks for the tasks will remain unknown.
5Conclusion
This study suggests a two-step method to identify the most appropriate ergonomic risk assessment observation method for determining MSD loads of EMS workers. In the first step of the analysis, PF-AHP was used to identify the weights of the four main criteria and the sixteen sub-criteria that were used in the method selection. In the second stage, the PF-WASPAS method was developed to rank the methods. A sensitivity analysis and a comparative analysis were carried out to test the reliability of the suggested model. The sensitivity analysis was performed by altering the threshold parameter (λ) in the WASPAS method. In the comparative analysis, the method suggested in this study was compared with AHP and F-AHP.
As a result of the proposed model, it has been revealed that the most suitable alternative for EMS is the REBA method (0.66). Output results are listed as RULA (0.59) and OWAS (0.55). It transpires that the most significant main criterion is the Final Assessment (0.347). The mean weight of other alternatives was calculated as Utility (0.289), Force Applied (0.195) and Entry Parameter (0.169). The most significant sub-criteria are Versatility (0.412) for Entry Parameter; Repetition (0.565) for Force Applied; Prioritization (0.573) for Final Assessment; and Repeatability (0.5) for Utility. Global weights show that the most important 4 criteria are Prioritization (0.20), Risk Output (0.15), and Repeatability (0.14), respectively.
The contributions of this article can be summarized as follows: (1) the criteria and sub-criteria were determined by the Multi-Criteria Decision-Making method in the decision process of method selection in emergency health services, (2) While the PF-AHP method was developed for determining the criteria weights, the PF-WASPAS method ranked the REBA, RULA and OWAS methods. (3) The PF-AHP and PF-WASPAS methodology based on PFSs and the problem of ergonomic risk assessment method selection are examined for the first time as far as we know. (4) It is also the first study to address the problem of method selection for the field of emergency health services. These pioneering features reveal the contributions of this article to the literature.
In future studies, multiple experts can be involved in the ergonomic risk assessment method selection process by referring to the consensus-trust driven Bidirectional Feedback Mechanism for improving consensus in large group decision making [62–64]. The other fuzzy sets, such as hesitant fuzzy sets [65], neutrosophic fuzzy sets [66], or spherical fuzzy sets [67] can be deployed in order to handle uncertainty in decision making process. In addition, the best-worst method might be used instead of PF-AHP for the identification of weights. It is recommended to try newly developed methods such as EDAS, ARAS, MAIRCA and MARCOS.
Disclaimer
This article was produced from the first author’s unpublished doctoral thesis titled “Ergonomic Risk Assessment of Working Postures in 112 Emergency Health Services”.
Conflict of interest
None.
Funding disclosure
None.
Supplementary material
[1] The supplementary material is available in the electronic version of this article: https://dx.doi.org/10.3233/JIFS-222974.
References
[1] | European Agency for Safety and Health at Work (EU-OSHA). Trastornos Musculoesqueláticos. Available from: https://osha.europa.eu/es/themes/musculoskeletal-disorders, accessed on 20 December, 2021. |
[2] | WHO Scientific Group on the Burden of Musculoskeletal Conditions at the Start of the New Millennium. The burden of musculoskeletal conditions at the start of the new millennium.World Health Organ Tech Rep Ser. 2003;919:i- x,1-218, back cover. PMID: 14679827. |
[3] | European Agency for Safety and Health at Work (EU-OSHA), Work related MSDs prevalence costs and demographics in the EU report. Available from: https://osha.europa.eu/en, accessed on 20 December 2021. |
[4] | Kee D. , Comparison of OWAS, RULA and REBA for assessing potential work-related musculoskeletal disorders, International Journal of Industrial Ergonomics 83: ((2021) ), 103140. |
[5] | Deros B.M. , Daruis DD. , Thiruchelvam S. et al., Evaluation on ambulance design and musculoskeletal disorders risk factors among ambulance emergency medical service personnel, Iranian Journal of Public Health 45: (Supple 1) ((2016) ), 52–60. |
[6] | Roberts M.H. , Sim M.R. , Black O. et al., Occupational injury risk among ambulance officers and paramedics compared with other healthcare workers in Victoria, Australia: analysis of workers’ compensation claims from to , Occupational and Environmental Medicine 72: (7) ((2015) ), 489–495. |
[7] | Takala E.P. , Pehkonen I. , Forsman M. et al., Systematic evaluation of observational methods assessing biomechanical exposures at work, Scandinavian Journal of Work, Environment & Health 36: (1) ((2010) ), 3–24. |
[8] | Karhu O. , Kansi P. and Kuorinka I. , Correcting working postures in industry: a practical method for analysis, Applied Ergonomics 8: (4) ((1977) ), 199–201. |
[9] | McAtamney L. and Corlett E.N. , RULA: a survey method for the investigation of work-related upper limb disorders, Applied Ergonomics 24: (2) ((1993) ), 91–99. |
[10] | Hignett S. and McAtamney L. , Rapid entire body assessment (REBA), Applied Ergonomics 31: (2) ((2000) ), 201–205. |
[11] | Ilbahar E. , Karasan A. , Cebi S. et al., A novel approach to risk assessment for occupational health and safety using Pythagorean fuzzy AHP&fuzzy inference system, Safety Science 103: ((2018) ), 124–136. |
[12] | Shete P.C. , Ansari ZN and Kant R. , A Pythagorean fuzzy AHP approach and its application to evaluate the enablers of sustainable supply chain innovation, Sustainable Product and Consumption 23: ((2020) ), 77–93. |
[13] | Zadeh L.A. , Fuzzy sets, Information and Control 8: (3) ((1965) ), 338–353. |
[14] | Atanassov K.T , Intuitionistic fuzzy sets, Fuzzy Set System 20: (1) ((1986) ), 87–96. |
[15] | Yager R.R. , Pythagorean fuzzy subsets, In 2013 joint IFSA world congress and NAFIPS annual meeting (IFSA/NAFIPS) (2013), 57–61. IEEE. |
[16] | Gul M. , Application of Pythagorean fuzzy AHP and VIKOR methods in occupational health and safety risk assessment: the case of a gun and rifle barrel external surface oxidation and colouring unit, International Journal of Occupational Safety and Ergonomics 26: (4) ((2018) ), 705–718. |
[17] | Abdullah L. and Goh P. , Decision making method based on Pythagorean fuzzy sets and its application to solid waste management, Complex&Intelligent Systems 5: ((2019) ), 185–198. |
[18] | Radwan N.M. , Senousy M.B. , Alaa M.R. Neutrosophic AHP multi criteria decision making method applied on the selection of learning management system, Infinite Study (2016). |
[19] | Mathew M. , Chakrabortty R.K. and Ryan M.J. , A novel approach integrating AHP and TOPSIS under spherical fuzzy sets for advanced manufacturing system selection, Engineering Applications of Artificial Intelligence 96: ((2020) ), 103988. |
[20] | Kizielewicz B. and Baczkiewicz A. , Comparison of Fuzzy TOPSIS, Fuzzy VIKOR, Fuzzy WASPAS and Fuzzy MMOORA methods in the housing selection problem, Procedia Computer Science 192: ((2021) ), 4578–4591. |
[21] | Al-Barakati A. , Mishrab AR. , Mardani A. and Rani P. , An extended interval-valued Pythagorean fuzzy WASPAS method based on new similarity measures to evaluate the renewable energy sources, Applied Soft Computing 120: ((2022) ), 108689. |
[22] | Doormaal M.T.A.J. , Driessen A.P.A. , Landeweerd J.A. et al., Physical workload of ambulance assistants, Ergonomics 38: (2) ((1995) ), 361–376. |
[23] | Ferreira J. and Hignett S. , Reviewing ambulance design for clinical efficiency and paramedic safety, Applied Ergonomics 36: (1) ((2005) ), 97–105. |
[24] | Gentzler M. and Stader S. , Posture stress on firefighters and emergency medical technicians (EMTs) associated with repetitive reaching, bending, lifting, and pulling tasks, Work 37: (3) ((2010) ), 227–239. |
[25] | Verjans M. , Schütt A. , Schleer P. et al., Postural workloads on paramedics during patient transport, Cur Direct Bio Eng 4: (1) ((2018) ), 161–164. |
[26] | Kahya E. and Sakarya S. , Ambulans ÇalışanlarınınKas İskelet Sistemi RahatsızlıklarınınDeğerlendirilmesi, Ergoterapi ve Rehabilitasyon Dergisi(Evaluation of Musculoskeletal Disorders of Ambulance Workers, JOccup Ther Rehab), 8: (2) ((2020) ), 99–106. |
[27] | Genaidy A.M. , Al-Shedi A.A. and Karwowski W. , Postural stress analysis in industry, Applied Ergonomics 25: (2) ((1994) ), 77–87. |
[28] | Juul-Kristensen B. , Fallentin N. and Ekdahl C. , Criteria for classification of posture in repetitive work by observation methods: A review, International Journal of Industrial Ergonomics 19: (5) ((1997) ), 397–411. |
[29] | Denis D. , Lortie M. and Rossignol M. , Observation procedures characterizing occupational physical activities: critical review, International Journal of Occupational Safety and Ergonomics 6: (4) ((2000) ), 463–491. |
[30] | Roman-Liu D. , Comparison of concepts in easy-to-use methods for MSD risk assessment, Applied Ergonomics 45: (3) ((2014) ), 420–427. |
[31] | Sukadarin E.H. , Deros B.M. , Ghani J.A. et al., Postural assessment in pen-and-paper-based observational methods and their associated health effects: a review, International Journal of Occupational Safety and Ergonomics 22: (3) ((2016) ), 389–398. |
[32] | Kahraman C. , Cebi S. , Onar S.C. , Oztaysi B. , Tolga A.C. , Sari I.U. vd, Intelligent and Fuzzy Techniques in Big Data Analytics and Decision Making: Proceedings of the INFUS 2019 Conference, Istanbul, Turkey, July 23–25, Vol. 1029 (2019) Springer. |
[33] | Ilbahar E. and Kahraman C. , Retail store performance measurement using a novel interval-valued Pythagorean fuzzy WASPAS method, Journal of Intelligent & Fuzzy Systems 35: (3) ((2018) ), 3835–3846. |
[34] | Gul M. and Ak M.F. , A comparative outline for quantifying risk ratings in occupational health and safety risk assessment, }, J Cleaner Product 196: ((2018) ), 653–664. |
[35] | Senol M.B. , Adem A. and Dagdeviren M. , A fuzzy MCDM approach to determine the most influential logistics factors, J Polytechnic 22: (3) ((2019) ), 793–800. |
[36] | Yucesan M. and Kahraman G. , Risk evaluation and prevention in hydropower plant operations: A model based on Pythagorean fuzzy AHP, Energy Policy 126: ((2019) ), 343–351. |
[37] | Ozkan B. , Kaya İ. , Erdogan M. et al., Evaluating blockchain risks by using aMCDMmethodology based on pythagorean fuzzy sets, Intern Conf Intel Fuzzy Sys Springer, Cham. (2019), 935–943. |
[38] | Karasan A. , Ilbahar E. and Kahraman C. , A novel pythagorean fuzzy AHP and its application to landfill site selection problem, Soft Computing 23: (21) ((2019) ), 10953–10968. |
[39] | Karasan A. , Kaya İ. , Erdogan M. et al., Risk analysis of theautonomous vehicle driving systems by using pythagorean fuzzy AHP,International Conference on Intelligent and Fuzzy Systems Springer, Cham. ((2019) ), 926–934. |
[40] | Bolturk E. , Kahraman C. As/RS technology selection using interval-valued pythagorean fuzzy WASPAS, In International Conference on Intelligent and Fuzzy Systems, Springer, Cham (2019), 867–875. |
[41] | Ilbahar E. , Cebi S. , Kahraman C. Assessment of renewable energy alternatives with pythagorean fuzzy WASPAS method: a case study of Turkey, International conference on intelligent and fuzzy systems, Springer, Cham (2019), 888–895. |
[42] | Mohagheghi V. and Mousavi S.M. , A new framework for high-technology project evaluation and project portfolio selection based on Pythagorean fuzzy WASPAS, MOORA and mathematical modeling, Iranian Journal of Fuzzy Systems 16: (6) ((2019) ), 89–106. |
[43] | Yildiz A. , Ayyildiz E. , Taskin Gumus A. et al., A modified balanced scorecard based hybrid pythagorean fuzzy AHP-topsis methodology for ATM site selection problem, International Journal of Information Technology & Decision Making 19: (02) ((2020) ), 365–384. |
[44] | Ilbahar E. , Cebi S. and Kahraman C. , Prioritization of renewable energy sources using multi-experts Pythagorean fuzzy WASPAS, Journal of Intelligent & Fuzzy Systems 39: (5) ((2020) ), 6407–6417. |
[45] | Yucesan M. and Gul M. , Hospital service quality evaluation: an integrated model based on Pythagorean fuzzy AHP and fuzzy TOPSIS, Soft Computing 24: (5) ((2020) ), 3237–3255. |
[46] | Yazici E. , Ozcan E. , Alakas H.M. et al., Hidroelektrik SantrallardaBakım Strateji Optimizasyonu için Hiyerarşik Bir KararModeli Önerisi,, (A hierarchicaldecision model proposal for maintenance strategy optimization inhydroelectric power plants. Journal of Polytechnic) 1-1.Turkish, Politeknik Dergisi (2021). |
[47] | Erol E. , Ozcan E. and Eren T. , A hybrid decision model in the selection of occupational safety specialists in power generation plants, Journal of Turkish Operations Management 5: (1) ((2021) ), 615–629. |
[48] | Ayyildiz E. and Gumus A.T. , Pythagorean fuzzy AHP based risk assessment methodology for hazardous material transportation: an application in Istanbul, Environmental Science and Pollution Research 28: (27) ((2021) ), 35798–35810. |
[49] | Ayyildiz E. , Erdogan M. and Taskin A. , Gumus, A Pythagorean fuzzy number-based integration of AHP and WASPAS methods for refugee camp location selection problem: a real case study for Istanbul, Turkey, Neural Computing and Applications 33: (22) ((2021) ), 15751–15768. |
[50] | Zeng S. , Chen J. and Li X. , A hybrid method for Pythagorean fuzzy multiple-criteria decision making, International Journal of Information Technology & Decision Making 15: (02) ((2016) ), 403–422. |
[51] | Zhu J. and Li Y. , Pythagorean fuzzy Muirhead mean operators and their application in multiple-criteria group decision-making, Information 9: (6) ((2018) ), 142. |
[52] | Yager R.R. Properties and applications of Pythagorean fuzzy sets, In Imprecision and Uncertainty in Information Representation and Processing, Springer Cham, (2016), 119–136. |
[53] | Zhang X. and Xu Z. , Extension of TOPSIS to multiple criteria decision making with Pythagorean fuzzy sets, International Journal of Intelligent Systems 29: (12) ((2014) ), 1061–1078. |
[54] | Yager R.R. and Abbasov A.M. , Pythagorean membership grades, complex numbers, and decision making, International Journal of Intelligent Systems 28: (5) ((2013) ), 436–452. |
[55] | Zavadskas E.K. , Turskis Z. , Antucheviciene J. et al., Optimization of weighted aggregated sum product assessment, Elektronika ir elektrotechnika 122: (6) ((2012) ), 3–6. |
[56] | Zavadskas E.K. , Antucheviciene J. , Hajiagha S.H.R. et al., Extension of weighted aggregated sum product assessment with interval-valued intuitionistic fuzzy numbers (WASPAS-IVIF), Applied Soft Computing 24: ((2014) ), 1013–1021. |
[57] | Peng X. and Yang Y. , Fundamental properties of interval-valued Pythagorean fuzzy aggregation operators, International Journal of Intelligent Systems 31: (5) ((2016) ), 444–487. |
[58] | Kahraman C. , Sarı İ.U. , Onar S.C. et al., Fuzzy economic analysis methods for environmental economics, In Intelligence Systems in Environmental Management: Theory and Applications, Springer, Cham (2017), 315–346. |
[59] | David G.C. , Ergonomic methods for assessing exposure to risk factors for work-related musculoskeletal disorders, Occupational Medicine 55: (3) ((2005) ), 190–199. |
[60] | Li G. and Buckle P. , Current techniques for assessing physical exposure to work-related musculoskeletal risks, with emphasis on posture-based methods, Ergonomics 42: (5) ((1999) ), 674–695. |
[61] | Kilbom A. , Assessment of physical exposure in relation to work-related musculoskeletal disorders-what information can be obtained from systematic observations, Scandinavian Journal of Work, Environment&Health 20: ((1994) ), 30–45. |
[62] | Li Z. , Zhang Z. and Yu W. , Consensus reaching with consistency control in group decision making with incomplete hesitant fuzzy linguistic preference relations, Computers&Industrial Engineering 170: ((2022) ), 108311. |
[63] | Gai T. , Cao M. , Chiclana F. , Zhang Z. , Dong Y. , Herrera-Viedma E. , Wu J. Consensus-trust driven bidirectional feedback mechanism for improving consensus in social network large-group decision making, Group Decision and Negotiation (2022), 1–30. |
[64] | Wu J. , Cao M.S. , Chiclana F. , Dong Y.C. and Herrera-Viedma E. , An optimal feedback model to prevent manipulation behaviour in consensus under social network group decision making, IEEE Trans Fuzzy Syst 29: (7) ((2021) ), 1750–1763. |
[65] | Yu W. , Zhang Z. , Zhong Q. and Sun L. , Extended TODIM for multi-criteria group decision making based on unbalanced hesitant fuzzy linguistic term sets, Computers&Industrial Engineering 114: ((2017) ), 316–328. |
[66] | Bolturk E. and Kahraman C. , A novel interval-valued neutrosophic AHP with cosine similarity measure, Soft Computing 22: ((2018) ), 4941–4958. |
[67] | Gundogdu F.K. and Kahraman C. , A novel spherical fuzzy analytic hierarchy process and its renewable energy application, Soft Computing 24: (6) ((2020) ), 4607–4621. |