Genome-wide identification and characterization of long non-coding RNAs involved in grape berry ripening
Abstract
BACKGROUND:
Long non-coding ribonucleic acids (lncRNAs) have been linked to many important biological processes, including fruit ripening. “Fengzao” is an early-ripening bud mutant of “Kyoho” that matures nearly 30 days earlier. However, the molecular networks controlling early ripening in Fengzao are still poorly understood in comparison to those in Kyoho.
OBJECTIVE:
The aim of the study was to gain a better understanding of the regulatory role of lncRNAs in the early ripening of grape berries.
METHODS:
The RNA-sequencing approach and quantitative real-time polymerase chain reaction validation were employed to identify lncRNAs and profile their expression patterns during berry development.
RESULTS:
In total, 24726 lncRNAs were identified, of which 19699 were differentially expressed (DE-lncRNAs) between developmental stages. The target genes of these lncRNAs and their regulatory relationships were predicted. The oxidoreductase activity, plant–pathogen interaction, plant hormone signal transduction, and flavanol biosynthesis pathways were enriched in the target genes of DE-lncRNAs, and six key lncRNAs (TCONS_00221683, TCONS_00684459, TCONS_00022149, TCONS_00167247, TCONS_00258125, and TCONS_00261813) were identified that may regulate the early ripening of grape berries.
CONCLUSIONS:
The results contribute to the understanding of the role of lncRNAs in early ripening of grape berries and will provide new insights for molecular breeding of grapes.
1Introduction
Fruit ripening has received considerable scientific interest because of its uniqueness in plant biology and the fact that fruit is an important part of the human diet [1]. It is a complex and highly coordinated developmental process involving a series of changes at the physiological and biochemical levels [2]. Fruit ripening is regulated by thousands of genes that control progressive softening and/or lignification of pericarp layers, accumulation of sugars, acids and pigments, and the release of volatiles [2]. A deeper understanding of the fruit-ripening process is critical to crop improvement.
The common grape vine (Vitis vinifera L.) is one of the most widely cultivated fruiting plants in the world, and it has been grown for fruit and wine production for thousands of years. At present, most widely cultivated grape varieties in China are middle–late-ripening types, among which Kyoho is a typical representative cultivar. This cultivar has multiple advantages, including large berries, high production, and adaptation to environments with high temperatures and rainy weather [3]. Fengzao is a bud mutant of Kyoho that ripens in early July in Henan Province, China, which is nearly 30 days earlier than Kyoho [4]. The phenological, physiological, and molecular differences between Fengzao and Kyoho have been investigated in previous studies [5–7]. Histological and molecular analysis have shown that their genetic background is highly uniform [5]. RNA-sequencing (RNA-seq) analysis and MicroRNA (miRNA) analysis have demonstrated that the reactive oxygen species (ROS)-related genes and some key miRNAs are expressed differently in Fengzao and Kyoho [6–7]. In addition, a number of transcriptional analyses have been performed to investigate the ripening process in grapes at different developmental stages, across different tissues, and among different cultivars [8–10]. These studies have revealed a number of genes and pathways in grape berry ripening. However, the mechanisms by which molecular networks control early ripening in Fengzao are still poorly understood in comparison to those in Kyoho.
Long non-coding RNAs (lncRNAs) are those that are longer than 200 nt and lack a region for protein coding [11]. According to their distribution in the genome, lncRNAs can be divided into three types: long intergenic non-coding RNAs (lincRNAs), long non-coding natural antisense transcripts (lncNATs), and intronic RNAs [12]. They are involved in chromatin modification, epigenetic regulation, genomic imprinting, transcriptional control, and pre- and post-translational messenger RNA (mRNA) processing [13–14]. A vast number of lncRNAs have been identified and characterized in plants [15–16]. Functional studies have shown that lncRNAs play widespread roles in diverse biological processes, including fruit ripening, regulation of flowering time, and abiotic stress in plants [17–18]. It has been shown from RNA-sequencing analysis that many lncRNAs are significantly up- or down-regulated in tomato fruits of ripening mutants [16]. The silencing of two tomato intergenic lncRNAs resulted in an obvious delay in the ripening of wild-type fruit [19]. This indicates that lncRNAs might be essential regulators of tomato fruit ripening. The regulatory role of lncRNAs in fruit ripening in other plants has also been reported, such as Cucumis melo [20], Hippophae rhamnoides [21], and Fragaria pentaphylla [22]. However, to our knowledge, the way in which lncRNAs function during the ripening of grapes is still largely unknown.
To gain a better understanding of the regulatory role of lncRNAs in early-ripening grape berry, RNA-seq profiles taken during berry development in Kyoho and Fengzao were investigated. Differentially expressed lncRNAs during early-stage berry development were identified, and their corresponding target genes were predicted. The enrichment of the Gene Ontology (GO) terms and Kyoto Encyclopedia of Genes and Genomes (KEGG) pathways of the target genes and the differentially expressed mRNAs were also analyzed. The results strongly suggested that lncRNAs play an important role in grape berry ripening. The findings provide new insight into the understanding of grape berry development.
2Materials and methods
2.1Plant materials
Both Fengzao and Kyoho vines with the same age were hedgerow cultivated in the same vineyard with the same viticulture management practices on the farm of Henan University of Science and Technology, Luoyang, China. The berries of the Fengzao and Kyoho vines were collected at several developmental stages from five individual vines (grafting on the same rootstock), and ten berries were taken from each vine. A total of 30 berries were collected mixed as one replicate and immediately frozen in liquid nitrogen, then stored at –80°C until further use. Based on comprehensive consideration of the grape growth stages [23] and our previous results [4, 24], the berries were sampled at the E-L 33 (hard green berries), E-L 34 (starting to soften), E-L 35 (v
2.2RNA isolation, library construction, and sequencing
Total RNA was isolated from the berries according to the method of Rienth et al. [25]. The integrity of RNA was assessed with the RNA Nano 6000 Assay Kit of the Bioanalyzer 2100 system (Agilent Technologies, CA, USA). A total of 3μg RNA was used for preparation of each sample. Ribosomal RNA was removed by the Ribo-Zero™ rRNA Removal Kit (Illumina, USA). Sequencing libraries were generated using the rRNA-depleted RNA by NEBNext®Ultra™ Directional RNA Library Prep Kit for Illumina® (New England Biolabs, MA, USA) following the manufacturer’s recommendations. To preferentially select cDNA fragments of 150 to 200 bp in length, the library fragments were purified with the AMPure XP system (Beckman Coulter, Beverly, CA, USA). Then, USER Enzyme (New England Biolabs, MA, USA) was used with adaptor-ligated cDNA before polymerase chain reaction (PCR) analysis. The PCR products were purified using the AMPure XP system and library quality was assessed using the Agilent Bio analyzer 2100 system. The PCR was performed with Phusion High-Fidelity DNA Polymerase, universal PCR primers, and index primers. The libraries were sequenced on an Illumina HiSeq 2000 platform (Illumina, San Diego, CA, USA) and 100 bp paired ends were generated.
2.3Quality control, mapping, and transcriptome assembly
Raw reads in FASTQ format were first processed through in-house Perl scripts to remove reads containing more than 10% of undetermined bases (denoted as “N”). Then, sequencing adapters and low-quality reads were removed with Trimmomatic version 0.35 [26]. The FastQC tool (http://www.bioinformatics.babraham.ac.uk/projects/fastqc) was used to check the quality of trimmed reads, and all the subsequent analyses were based on these high-quality clean reads. The grape reference genome [27] and gene annotation files (v2.1) were downloaded from the grape genome database (http://www.genoscope.cns.fr). An index of the reference genome was built using Bowtie v2.0.6 [28] and all clean reads from each sample were aligned to the reference genome using HISAT2 v2.0.4 with the parameter “–rna-strandness RF” [29]. The distribution of known gene types was analyzed using HTSeq [30]. The mapped reads of each sample were assembled using Cufflinks v2.1.1 [31] using a reference-based approach with default parameters except “min-frags-per-transfrag = 0” and “–library-type”.
2.4Identification and filtering of lncRNA candidates
The above assembled transcripts for all samples were combined using the CuffCompare software [31]. Based on the structural and functional characteristics of lncRNA, we applied five filtering steps to identify lncRNA candidates from all the assembled transcripts. First, single-exon transcripts falling within 500 bp up- or downstream of any protein-coding gene were removed. Next, we filtered out any transcripts less than 200 bp. All resulting transcripts were then filtered based upon the expression level calculated by Cufflinks, with expression thresholds of 2 FPKM (reads per kilobase of transcript per million mapped reads) for single-exon transcripts and 0.5 FPKM for multiple-exon transcripts. The obtained lncRNA candidates were then compared with known grape lncRNAs (http://genomes.cribi.unipd.it/DATA/V2/V2.1/lncRNA/) using CuffCompare, and transcripts matched with known lncRNAs were retained and included in the final data set. Any transcripts sharing identity with known rRNAs, tRNAs, snRNAs, snoRNAs, pre-miRNAs, and pseudogenes were removed. Finally, when compared with known mRNAs using CuffCompare, three classes of lncRNAs, including lincRNAs, intronic lncRNAs, and antisense lncRNAs, were identified from the remaining transcripts according to the results of class_code (http://cole-trapnell-lab.github.io/cufflinks/cuffcompare/index.html).
To filter the potential protein-coding transcripts, the lncRNA transcripts found above were further processed using two tools for coding-potential analysis, Coding Potential Calculator (CPC) [32] and PfamScan [33]. The CPC assesses the extent and quality of the open reading frame (ORF) in a transcript and searches the sequences using a known protein sequence database to clarify the coding and non-coding transcripts. For CPC (v0.9-r2) analysis, the National Center for Biotechnology Information eukaryotic non-redundant protein database was used, and thee-value was set to 1×10–10. PfamScan (v1.3) with default parameters was used to identify the occurrence of any of the known protein family domains deposited in the Pfam database (release 31.0, both Pfam A and Pfam B). Transcripts with a CPC score >0 or with a Pfam hit were considered to have coding potential. Transcripts predicted to have coding potential by either of the tools were filtered out. The candidate lncRNAs without coding potential identified by both tools were considered as the final set of lncRNAs. The final data set was used for the following analyses.
2.5Quantification and differential expression analysis
The relative abundance of both candidate lncRNAs and coding genes in each sample was computed by calculating the FPKM using Cufflinks (v2.1.1) [31]. The FPKM is calculated based on the length of the fragments and read counts mapped to each fragment. Gene FPKMs were computed by summing the FPKMs of the transcripts in each gene group.
Differentially expressed lncRNAs and coding genes in comparison groups were identified using the Cuffdiff program [31]. Cuffdiff determines differential expression in digital transcripts or gene expression data using a model based on the negative binomial distribution. For biological replicates, transcripts or genes with a adjusted p value <0.05 were assigned as differentially expressed. For non-biological replicates, adjusted p value <0.05 and the absolute value of log2 (fold change) >1 were set as the thresholds for significantly differential expression.
2.6Target gene prediction and enrichment analysis
To explore the function of lncRNAs, we predicted their cis and trans target genes. This was carried out using an algorithm that searches for potential cis-target genes that are substantially near to lncRNAs (within 10kb upstream or downstream) using a genome browser and genome annotation. The function of the identified cis-target genes was then analyzed. The trans target genes of the lncRNAs can be predicted by correlation analysis or co-expression analysis between the lncRNAs and protein-coding genes. We calculated Pearson correlation coefficients between the lncRNAs and the coding genes. Coding genes with absolute correlation coefficients >0.95 were considered as trans target genes, and the function of these genes was analyzed through functional enrichment analysis to predict the main functions of the lncRNAs.
Gene Ontology enrichment analysis of differentially expressed genes, lncRNAs, and lncRNA target genes was performed using the R package “cluster Profiler” [34]. In this analysis, GO terms with a corrected p value <0.05 were considered significantly enriched by differentially expressed genes, lncRNAs, or lncRNA target genes. Similarly, pathways with p values <0.05 were considered significantly enriched by differentially expressed genes, lncRNAs, or lncRNA target genes.
2.7Systematic cluster analysis and co-expression network analysis
Cluster analysis was performed as described previously [6]. The WGCNA package [35–36] was used to test whether the expression of lncRNAs and mRNAs correlated with samples.
2.8Quantitative real-time PCR
To validate the expression of the differentially expressed lncRNAs and their roles in grape berry development, ten differently expressed lncRNAs and four mRNAs were chosen for quantitative real-time PCR (qPCR) analysis. Isolation of RNA, reverse transcription, and qPCR were performed as described previously [37]. The primer sequences are listed in Table 1.
Table 1
The primers of lncRNAs and mRNAs used for qPCR verification
Primer name | Sequence (5′–3′) |
TCONS_00221683F | AGGATTTCGGGTTTAGCTAGGTC |
TCONS_00221683R | TTTGGTTGGCTTAAACACTTGTGA |
TCONS_00684459F | AACCCAAGCATGAACAAGCC |
TCONS_00684459R | ACTTGGGGTTTGGTTCGGTT |
TCONS_00022149F | TCCCACACCTCTACACTACCA |
TCONS_00022149R | CCCAATAGCCTAGCCAGCTT |
TCONS_00167247F | TGTCCCATGGGTAAGGTGGT |
TCONS_00167247R | ATCACCCACCCACCATCAAT |
TCONS_00258125F | TCCATGAAAGGAACCAAACTGC |
TCONS_00258125R | CATGAGAAAGTTGCACCCGC |
TCONS_00261813F | GAGGGAGCCATCTTGGATGTT |
TCONS_00261813R | GGGTTGTCAGTTGGGTTGAA |
TCONS_00109752F | TGCTTCATTGCCATGTTTGTTGA |
TCONS_00109752R | ATGCTAAGCAAGGCAAGATGAT |
TCONS_00719859F | TCTCCAGCCGGTGGTAGTAA |
TCONS_00719859R | GGGTGAAGACCGCACAAGTA |
TCONS_00745975F | CAGCCACTTCAACGCATCAA |
TCONS_00745975R | GCATGGACTTATGAATTACGAACCC |
TCONS_00519254F | GCATTTTTGCTGAGGACCCC |
TCONS_00519254R | AGAGCCCAGTGCACATTTCA |
VIT_07s0031g01710F | TTTGGGGTTTAGGGTTGCCT |
VIT_07s0031g01710R | AGTTCCTTGGATTGGGGCTG |
VIT_02s0033g01380F | TTGCCATTGACTACTGGCGT |
VIT_02s0033g01380R | GTGGTTAACGTCCCGGTGAT |
VIT_15s0046g00070F | TGTGTCAGAGCTTTGTGGGG |
VIT_15s0046g00070R | CCTCTGCATCCTCCTTGCTC |
VIT_14s0068g00680F | CCCCAGGAAAGGGTGACATC |
VIT_14s0068g00680R | CAGTTGGTTGTGCAGGAAGC |
Ubiquitin1F | GTGGTATTATTGAGCCATCCTT |
Ubiquitin1R | AACCTCCAATCCAGTCATCTAC |
3Results
3.1Statistics and sequencing quality assessment
Fruit samples were harvested at four (FZ1, FZ2, FZ3, and FZ4) and five (KY1, KY2, KY3, KY4, and KY5) developmental stages from the Fengzao and Kyoho vines, respectively. A total of 2094312898 clean reads were obtained from the sequencing libraries with an average of around 116 million reads for each library (Table 2). The cleaned reads had a Q20 percentage (percentage of sequences with sequencing error rate lower than 1%) of over 97%. The GC content of each library was between 48.49% and 53.21%. The reads of high quality were mapped to the grape reference genome. Approximately 59 million reads were uniquely aligned to the reference, accounting for 50% of the total reads. Around 76.6% of the uniquely mapped reads were assigned to known exons.
Table 2
Details of the sequencing results for each library
Sample name | Raw reads | Clean reads | Clean bases | Error rate (%) | Q20 (%) | Q30 (%) | GC content (%) |
FZ11 | 128589880 | 124049988 | 18.61G | 0.01 | 97.68 | 94.08 | 52.13 |
FZ12 | 97885788 | 94628514 | 14.19G | 0.01 | 97.38 | 93.48 | 52.07 |
FZ21 | 114023110 | 108835090 | 16.33G | 0.01 | 97.74 | 94.24 | 49.13 |
FZ22 | 122713506 | 119240346 | 17.89G | 0.01 | 97.61 | 93.95 | 50.82 |
FZ31 | 112404034 | 106015302 | 15.9G | 0.01 | 97.95 | 94.52 | 48.65 |
FZ32 | 157045718 | 153257780 | 22.98G | 0.01 | 97.92 | 94.58 | 51.13 |
FZ41 | 112580794 | 109495606 | 16.42G | 0.01 | 97.68 | 94.16 | 48.49 |
FZ42 | 111669038 | 108685332 | 16.3G | 0.01 | 97.76 | 94.28 | 50.86 |
KY11 | 127985418 | 123469514 | 18.52G | 0.01 | 97.19 | 92.85 | 52.37 |
KY12 | 132407132 | 128297430 | 19.24G | 0.01 | 97.3 | 93.1 | 53.21 |
KY21 | 141560320 | 136184936 | 20.43G | 0.01 | 97.62 | 94 | 48.82 |
KY22 | 107228096 | 104015392 | 15.6G | 0.01 | 97.46 | 93.62 | 51.77 |
KY31 | 125566036 | 122279408 | 18.34G | 0.01 | 97.56 | 93.85 | 49.47 |
KY32 | 125158980 | 121455268 | 18.22G | 0.01 | 97.44 | 93.57 | 50.45 |
KY41 | 106508874 | 103718700 | 15.56G | 0.01 | 97.21 | 92.99 | 49.21 |
KY42 | 104028882 | 100993530 | 15.15G | 0.01 | 97.04 | 92.66 | 50.86 |
KY51 | 120738168 | 115939420 | 17.39G | 0.01 | 97.52 | 93.83 | 49.33 |
KY52 | 116699660 | 113751342 | 17.06G | 0.01 | 97.5 | 93.72 | 50.5 |
3.2Identification and characterization of lncRNAs
After processing the assembled transcripts through five filtering steps, the lncRNAs were identified based on their structure and non-coding features. Of the 24726 lncRNAs identified, 9127 were intronic lncRNAs, 13731 were lincRNAs, and 1868 were lncNATs. These three types accounted for 36.9%, 55.5%, and 7.6% of total lncRNAs, respectively. There were also 5027 transcripts of uncertain coding potential (TUCPs).
We characterized the basic genomic features of the lncRNAs and mRNAs, including the length of the transcript and the ORF, the exon number, and the expression level. The full and ORF lengths of lncRNAs were shorter than them RNAs. The majority of lncRNAs (83.86%) were 200–300 bp, 13.96% were 301–1000 bp, and only 2.19% were >1000 bp in length. In contrast, 9.01% of mRNAs were 0–300 bp, 35.06% were 301–1000 bp, and 55.94% were >1000 bp in length (Fig. 1a). The ORF length of most lncRNAs (96.21%) was shorter than 100 bp, while only 18.01% of mRNA ORFs were shorter than 100 bp (Fig. 1b). In addition, we observed significant differences in the distribution of exon numbers between lncRNAs and mRNAs, with 96.54% of lncRNAs only containing one or two exons, while mRNAs had more exons and exon numbers distributed across a wider range (Fig. 1c). We then estimated the expression level of lncRNAs and mRNAs using FPKM the results showed that the overall expression levels of lncRNAs were lower than those of mRNAs (Fig. 1d).
Fig. 1
Characterization of genomic features of the lncRNAs and mRNAs. a, Length distribution of the transcripts; b, Open reading frame length of the transcripts; c, Exon number of the transcripts; d, FPKM distribution of the transcripts.
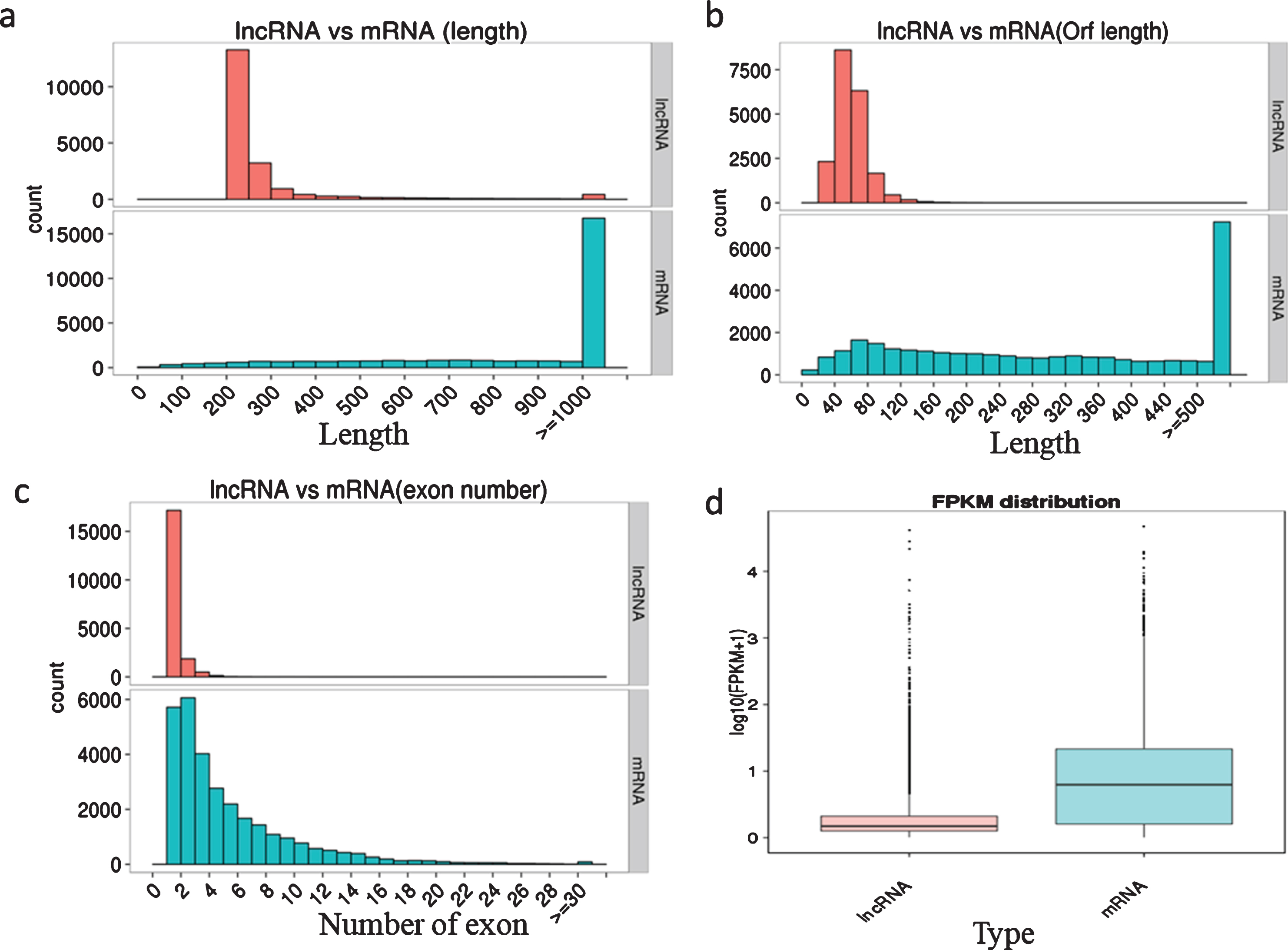
3.3Overall expression patterns between Fengzao and Kyoho
To better understand the overall expression patterns of the transcripts in the nine samples, clustering analysis of the expression patterns in both Fengzao and Kyoho across various developmental stages was performed using the R package “TCseq”. The TCseq package provides a unified suite for clustering analysis and visualization of time-course sequencing data. The clustering analysis revealed 20 clusters for both Fengzao and Kyoho (Fig. 2). The transcripts and corresponding clusters are shown in Table S1, and the number of transcripts in each cluster is shown in Table 3. As shown in Fig. 2, most clusters showed similar expression patterns between Fengzao and Kyoho. There were several interesting clusters, such as clusters 4, 5, 6, 8, 10, 18, and 19, which showed differential expression patterns between Fengzao and Kyoho. The expression levels of 3373 transcripts from cluster 10 (including 2075 lncRNAs, 1036 mRNAs, and 262 TUCPs), which accounted for 6.32% of the total transcripts, were higher in the FZ1 stage than in the other stages, and the expression levels of 3057 transcripts from cluster 5 (including 1515 lncRNAs, 1238 mRNAs, and 304 TUCPs), which accounted for 6.28% of the total transcripts, were higher in the FZ2 stage than in the other stages. The expression levels of transcripts from cluster 19 (2829 transcripts, including 1356 lncRNAs, 1114 mRNAs, and 359 TUCPs) and cluster 4 (9788 transcripts, including 4114 lncRNAs, 4488 mRNAs, and 1186 TUCPs) were higher in the KY2 and KY3 stages, respectively. The expression patterns of genes were therefore dramatically different in clusters 4, 5, 10, and 19 during the early developmental stages, and in clusters 6, 8, and 18 during later developmental stages (Fig. 2). Genes in clusters 4, 5, 10, and 19 may thus contribute to early fruit development and ripening in Fengzao.
Fig. 2
Cluster analysis of the gene expression patterns in both Fengzao and Kyoho across various developmental stages. The Y axis represents deviation of the expression levels (log-transformed) of a gene at different developmental stages from the mean expression of the gene in consideration. The X-axis represents the sampling time points.
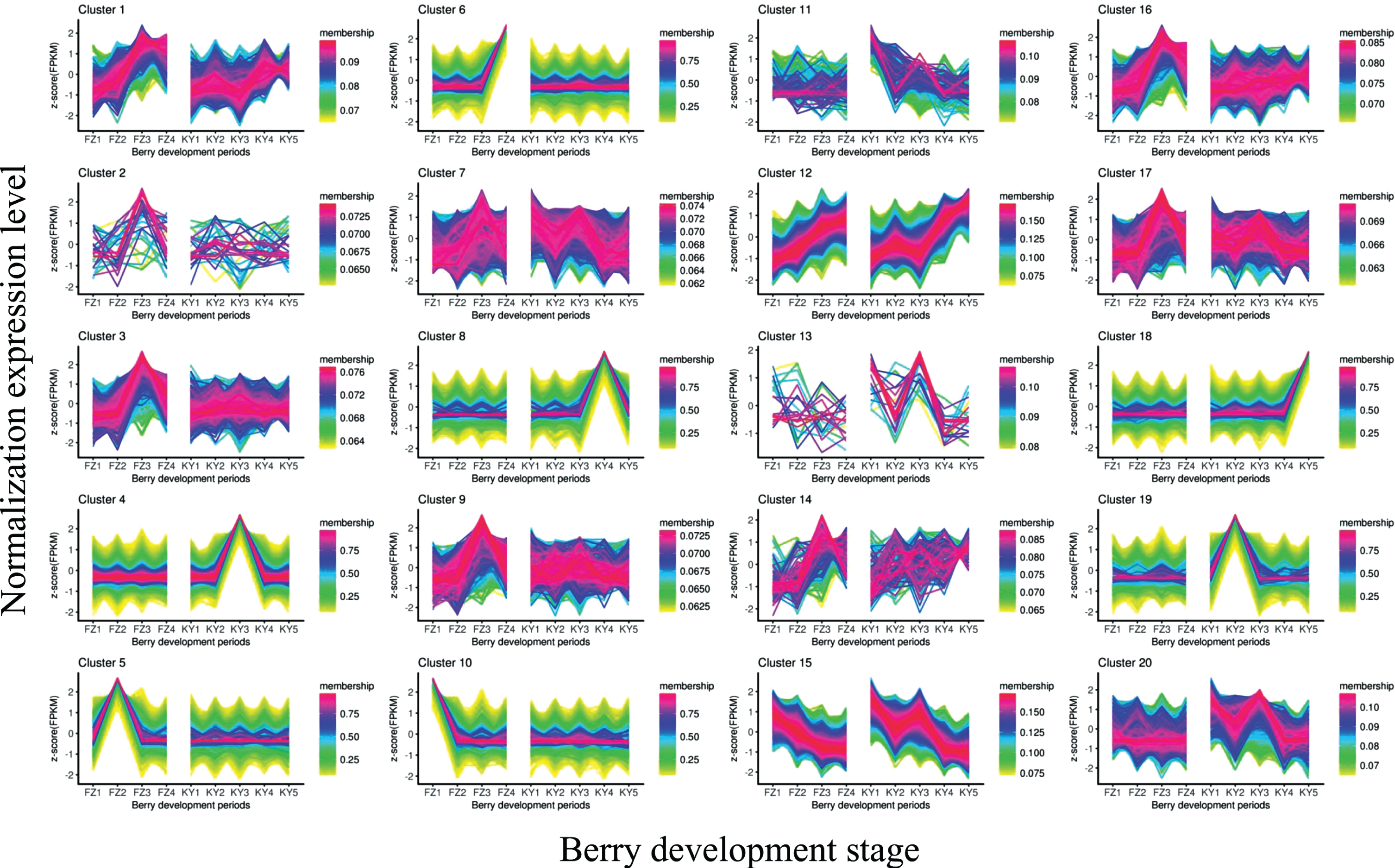
Table 3
The number of transcripts in each cluster
Cluster | lncRNA | mRNA | TUCP |
1 | 113 | 563 | 69 |
2 | 14 | 17 | 1 |
3 | 601 | 296 | 132 |
4 | 4114 | 4488 | 1186 |
5 | 1515 | 1238 | 304 |
6 | 3245 | 1008 | 463 |
7 | 129 | 509 | 75 |
8 | 1437 | 760 | 372 |
9 | 176 | 271 | 55 |
10 | 2075 | 1036 | 262 |
11 | 46 | 114 | 11 |
12 | 1004 | 4466 | 509 |
13 | 3 | 16 | 1 |
14 | 49 | 69 | 11 |
15 | 1074 | 4960 | 323 |
16 | 280 | 555 | 120 |
17 | 104 | 301 | 53 |
18 | 2178 | 1405 | 607 |
19 | 1356 | 1114 | 359 |
20 | 185 | 802 | 109 |
Both GO and KEGG enrichment analyses were performed in clusters 4, 5, 10, and 19. The GO term trihydroxystilbene synthase activity (GO:0050350) was enriched in cluster 10; 1,3-beta-D-glucan synthase activity (GO:0003843) was enriched in cluster 19; iron–sulfur cluster binding (GO:0051536), trihydroxystilbene synthase activity (GO:0050350), oxidoreductase activity (GO:0016671), carbohydrate binding (GO:0030246), and electron transfer activity (GO:0009055) were enriched in cluster 5; and 12 GO terms were enriched in cluster 4, the top five of which were microtubule motor activity (GO:0003777), double-stranded DNA binding (GO:0003690), motor activity (GO:0003774), hydroquinone:oxygen oxidoreductase activity (GO:0052716), and oxidoreductase activity (GO:0016722) (Fig. S1a).
The KEGG enrichment analysis revealed that flavonoid biosynthesis (ko00941), circadian rhythm-plant (ko04712), phenylalanine metabolism (ko00360), and the phosphatidylinositol signaling system (ko04070) were enriched in cluster 10; plant–pathogen interaction (ko04626) and non-homologous end-joining (ko03450) were enriched in cluster 19; photosynthesis (ko00195), carbon fixation in photosynthetic organisms (ko00710), circadian rhythm-plant (ko04712), carbon metabolism (ko01200), pentose phosphate pathway (ko00030), and flavonoid biosynthesis (ko00941) were enriched in cluster 5;and 11 terms were enriched in cluster4, the top five of which were phenylpropanoid biosynthesis (ko00940), DNA replication (ko03030), starch and sucrose metabolism (ko00500), fatty-acid biosynthesis (ko00061), and biotin metabolism (ko00780) (Fig. S1b).
3.4Differential expression analysis
The Cufflinks tool was used to quantify the relative abundance of transcripts by calculating FPKM, and Cuffdiff was used to conduct the differential expression analysis. A total of 54653 expressed transcripts were evaluated, including 29927 mRNAs, 19699 lncRNAs, and 5027 TUCPs. Of these transcripts, 2421 were significantly differentially expressed between Fengzao and Kyoho (adjusted p value <0.05 and the absolute value of log2 (fold change) <1). There were 1782 differentially expressed mRNAs (DE-mRNAs) among these 2421 transcripts, including 415 and 1367 mRNAs that were up- and down-regulated in Fengzao compared to Kyoho, respectively. Again, GO and KEGG enrichment analyses were performed to annotate these DE-mRNAs.
The GO analysis demonstrated that DE-mRNAs were enriched in microtubule motor activity (GO:0003777), flavonoid 3′,5′-hydroxylase (F3′5′H) activity (GO:0033772), hydrolase activity acting on glycosyl bonds (GO:0016798), hydrolase activity (GO:0016787), hydrolyzing O-glycosyl compounds (GO:0004553), motor activity (GO:0003774), oxidoreductase activity of oxygen (GO:0016709), and oxidoreductase activity (GO:0016705) (Fig. 3a). The KEGG enrichment analysis revealed that flavone and flavanol biosynthesis, galactose metabolism, DNA replication, phenylpropanoid biosynthesis, plant hormone signal transduction, flavonoid biosynthesis, starch and sucrose metabolism, and tropane, piperidine, and pyridine alkaloid biosynthesis were significantly enriched in DE-mRNAs between Fengzao and Kyoho (Fig. 3d, q value <0.1).
Fig. 3
The GO and KEGG enrichment analysis of DE-mRNAs and DE-lncRNAs targets in comparisons between two cultivars across developmental stages. Enrichment term is represented by colored dots (blue indicates high enrichment and red indicates low enrichment). a–c, GO terms in molecular categories from GO enrichment analysis of DE-mRNAs, cis targets of DE-lncRNAs and trans targets of DE-lncRNAs, respectively; d–f, KEGG terms of DE-mRNAs, cis targets of DE-lncRNAs and trans targets of DE-lncRNAs, respectively.
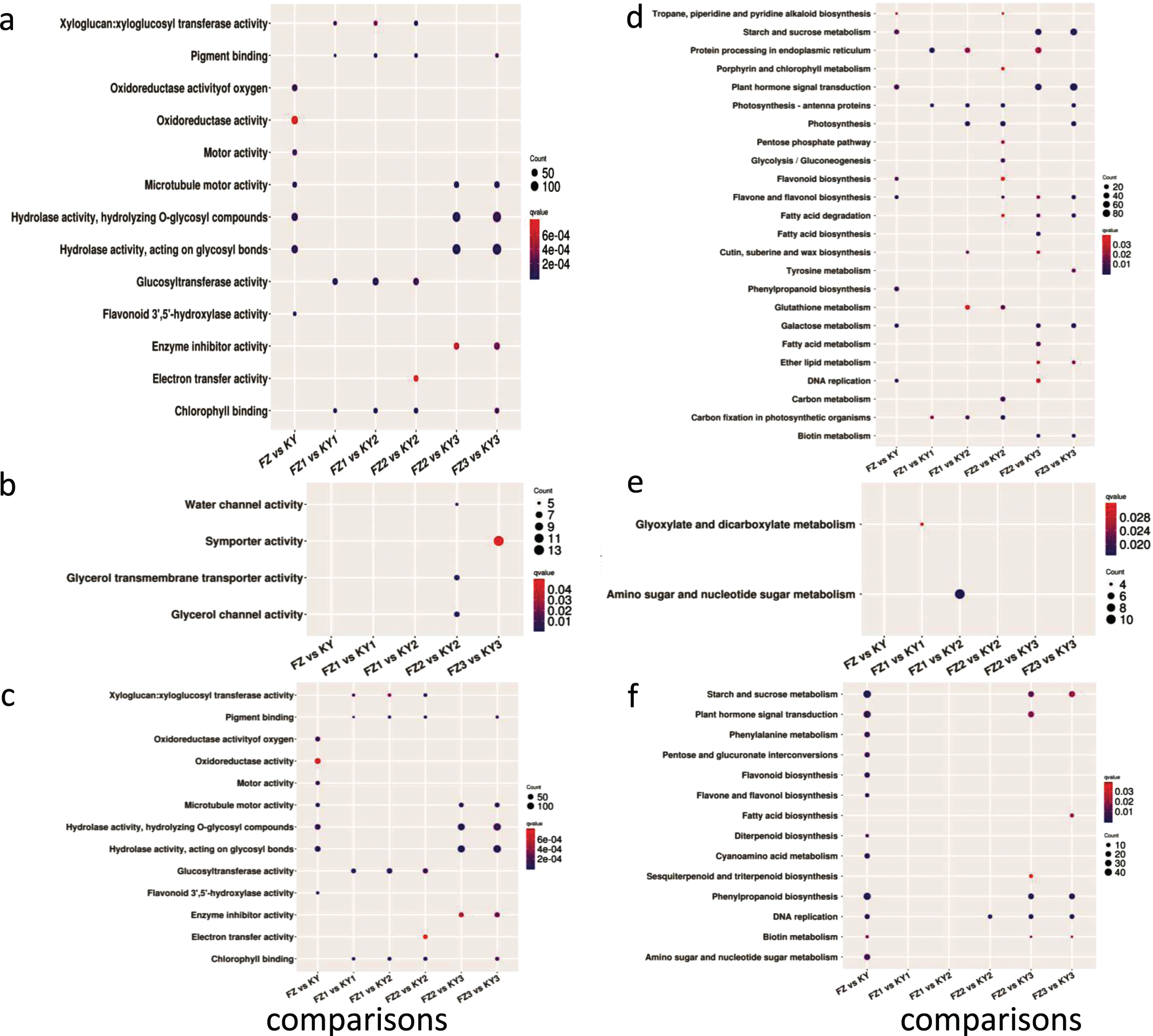
To further explore the genes contributing to early ripening in Fengzao, comparisons between the two cultivars across the developmental stages before ripening were made, and these comparisons included FZ1 vs KY1, FZ1 vs KY2, FZ2 vs KY2, FZ2 vs KY3, and FZ3 vs KY3. The number of differentially expressed mRNAs was 888 in FZ1 vs KY1, 1857 in FZ1 vs KY2, 1494 in FZ2 vs KY2, 3580 in FZ2 vs KY3, and 4833 in FZ3 vs KY3. Compared with KY1, 536 mRNAs were up-regulated and 352 mRNAs were down-regulated in FZ1. Compared with KY2, 1132 mRNAs were up-regulated and 725 mRNAs were down-regulated in FZ1, and 1010 mRNAs were up-regulated and 484 mRNAs were down-regulated in FZ2. Compared with KY3, 1255 mRNAs were up-regulated and 2325 mRNAs were down-regulated in FZ2, and 1566 mRNAs were up-regulated and 3267 mRNAs were down-regulated in FZ3 (adjusted p value <0.05, Table 4). Once more, GO and KEGG enrichment analyses were performed to annotate these DE-mRNAs in each comparison.
Table 4
The number of transcripts in each comparison
mRNA | lncRNA | TUCP | ||||
Up-regulated | Down-regulated | Up-regulated | Down-regulated | Up-regulated | Down-regulated | |
FZ vs KY | 415 | 1367 | 160 | 255 | 55 | 169 |
FZ1 vs KY1 | 536 | 352 | 111 | 67 | 29 | 44 |
FZ1 vs KY2 | 1132 | 725 | 128 | 162 | 40 | 94 |
FZ2 vs KY2 | 1010 | 484 | 85 | 124 | 40 | 69 |
FZ2 vs KY3 | 1255 | 2325 | 70 | 300 | 48 | 262 |
FZ3 vs KY3 | 1566 | 3267 | 107 | 297 | 75 | 259 |
The GO analysis demonstrated that xyloglucan:xyloglucosyl transferase activity, pigment binding, glucosyltransferase activity, and chlorophyll binding were enriched in all three comparisons of FZ1 vs KY1, FZ1 vs KY2, and FZ2 vs KY2. The DE-mRNAs in FZ2 vs KY3 and FZ3 vs KY3 were enriched in microtubule motor activity, hydrolase activity, hydrolyzing O-glycosyl compounds, hydrolase activity acting on glycosyl bonds, and enzyme inhibitor activity, and DE-mRNAs in FZ3 vs KY3 were also enriched in pigment binding and chlorophyll binding (Fig. 3a).
The KEGG enrichment analysis revealed that protein processing in endoplasmic reticulum, photosynthesis-antenna proteins, photosynthesis, glutathione metabolism, and carbon fixation in photosynthetic organisms were enriched in at least two comparisons of FZ1 vs KY1, FZ1 vs KY2, and FZ2 vs KY2. Furthermore, seven pathways were enriched in FZ2 vs KY3 and FZ3 vs KY3, the top five of which were starch and sucrose metabolism, plant hormone signal transduction, fatty-acid degradation, galactose metabolism, and biotin metabolism (Fig. 3d).
From the significant difference analysis of the 19699 lncRNAs, there were 415 differentially expressed lncRNAs (DE-lncRNAs) in FZ vs KY, including 160 and 255 lncRNAs that were up- and down-regulated in Fengzao compared to Kyoho, respectively. In the comparisons of the early ripening stages between Fengzao and Kyoho, there were 168, 290, 208, 370, and 403 DE-lncRNAs in FZ1 vs KY1, FZ1 vs KY2, FZ2 vs KY2, FZ2 vs KY3, and FZ3 vs KY3, respectively. Compared with KY1, 101 of the 168 lncRNAs were up-regulated and the other 67 lncRNAs were down-regulated in FZ1. Compared with KY2, 128 of the 290 lncRNAs were up-regulated and the other 162 lncRNAs were down-regulated in FZ1, and 85 of the 208 lncRNAs were up-regulated and the other 123 lncRNAs were down-regulated in FZ2. Compared with KY3, 70 of the 370 lncRNAs were up-regulated and the other 300 lncRNAs were down-regulated in FZ2, and 107 of the 403 lncRNAs were up-regulated and the other 296 lncRNAs were down-regulated in FZ3 (Table 4 and Table S2).
3.5Functional enrichment analysis of differentially expressed lncRNAs
The regulatory roles of lncRNAs on target genes were mediated by cis-and trans-acting mechanisms. To investigate the functions of lncRNAs, we first predicted the putative cis- and trans-regulatory target genes of lncRNAs. The cis targets of lncRNAs were sought within 10 kb upstream and downstream of the lncRNAs. The trans target genes of lncRNAs were predicted by correlation analysis or co-expression analysis between the lncRNAs and mRNAs. A total of 36689 cis-acting lncRNA–mRNA pairs were identified for the 10-kb flanking regions, including 16625 lncRNAs and 18062 mRNAs (Table S3). For the trans targets of lncRNAs, a total of 1131702 regulation pairs were identified, with 12579 lncRNAs and 4846 mRNAs (Table S4). Of the 20213 target mRNAs (including cis and trans) of the DE-lncRNAs, 5331 were overlapping with the DE-mRNAs (6990 in total), accounting for 76.27% of the total DE-mRNA.
To further predict the functions of DE-lncRNAs during early grape ripening, GO and KEGG analyses were performed using the cis and trans target genes of DE-lncRNAs between the two cultivars. Analysis of cis lncRNA targets revealed that there were no GO or KEGG terms significantly enriched in the comparison between Fengzao and Kyoho (corrected p value <0.05). Furthermore, we clustered the early developmental stages for Fengzao and Kyoho based on the GO (Fig. 3b) and KEGG (Fig. 3e) terms for cis-target genes of DE-lncRNAs. It is noteworthy that the GO terms glycerol channel activity, glycerol transmembrane transporter activity, and water channel activity were enriched in FZ2 vs KY2, and the GO term symporter activity was enriched in FZ3 vs KY3. From Fig. 3E, the KEGG terms glyoxylate and dicarboxylate metabolism, and amino sugar and nucleotide sugar metabolism, were enriched in FZ1 vs KY1 and FZ1 vs KY2, respectively.
For trans lncRNA targets, in the comparison between Fengzao and Kyoho, functional analysis illustrated that 12 GO terms were enriched, the top five of which were oxidoreductase activity, monooxygenase activity, heme binding, hydroquinone:oxygen oxidoreductase activity, and microtubule motor activity (Fig. 3c). We also performed the same analysis for the comparison between the two cultivars across the developmental stages before ripening. From this, there were two terms (motor activity and microtubule motor activity) that were enriched in FZ2 vs KY2, FZ2 vs KY3, and FZ3 vs KY3, and the other five terms (hydroquinone:oxygen oxidoreductase activity, monooxygenase activity, nutrient reservoir activity, oxidoreductase activity, and oxidoreductase activity, oxidizing metal ions) were enriched in FZ2 vs KY3 and FZ3 vs KY3 (Fig. 3c).
The KEGG analysis of trans lncRNA targets revealed that in the comparison between Fengzao and Kyoho there were 12 significant enriched KEGG terms, the top five of which were phenylpropanoid biosynthesis, starch and sucrose metabolism, flavone and flavonol biosynthesis, cyanoamino acid metabolism, and DNA replication. Furthermore, the DNA replication, phenylpropanoid biosynthesis, and starch and sucrose metabolism KEGG terms were enriched in the early-ripening-stage comparisons (Fig. 3f). These results indicate that pathways including starch and sucrose metabolism, flavone and flavonol biosynthesis, DNA replication, and phenylpropanoid biosynthesis are involved in the ripening of grapes.
3.6Co-expression network analysis of genes in grape berry development
To obtain a global view of the regulatory mechanisms and the key genes related to the early ripening of grape berries, mRNAs and lncRNAs were used to construct a gene co-expression network with the WGCNA package. In total, 29 co-expression modules were developed, and the correlation coefficient of each co-expression module with the samples was calculated (Fig. 4). The modules with the highest correlation coefficients with FZ1, FZ2, FZ3, KY1, KY2, and KY3 were MElightgreen (r = 0.85), MEpaleturquoise and MEsteelblue (r = 0.73), MEcyan (r = 0.67), MEviolet (r = 0.73), MEgrey60 (r = 0.88), and MEturquoise (r = 0.98), respectively.
Fig. 4
The relationships analysis between modules and samples by WGCNA (weighted gene co-expression network analysis). The numbers are module-sample weight correlation and corresponding p-values (in parentheses). The left panel shows the 29 modules. The color scale on the right shows module-sample correlation from –1 (green) to 1 (red).
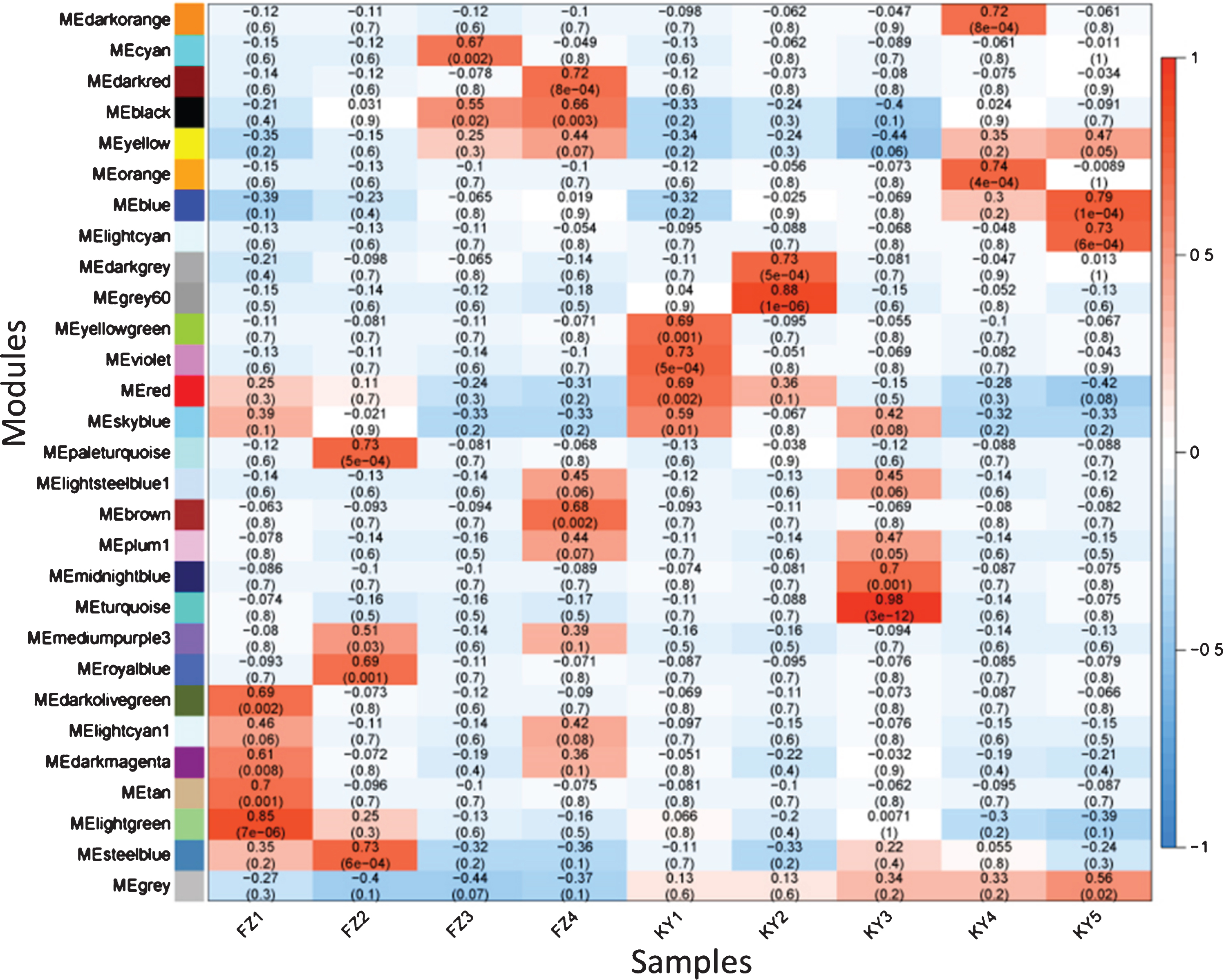
To gain a further understanding of the seven positive correlation modules, GO and KEGG analyses of the mRNAs and target genes of lncRNAs in these modules were conducted. The results showed that terms were enriched mainly in MEsteelblue, and the terms electron transfer activity and chlorophyll binding were enriched in GO analysis (Fig. 5a), while carbon fixation in photosynthetic organisms, carbon metabolism, photosynthesis, photosynthesis-antenna proteins, fructose and mannose metabolism, and the pentose phosphate pathway were enriched in KEGG analysis (Fig. 5b). These indicate that the FZ2 stage may play an important role in the early maturing of Fengzao. To identify the genes that play pivotal roles in essential biological processes, genes in the seven modules above were analyzed by connected sub networks (Fig. 6). We defined those with more than five edges as “hub genes”, and 31 hub genes (nine mRNAs and 22 lncRNAs) were screened out, including WRKY51 (VIT_07s0031g01710), alternative oxidase gene AOX1D (VIT_02s0033g01380), and glyceraldehyde-3-phosphate dehydrogenase gene GAPA-2 (VIT_14s0068g00680). Among these, GAPA-2 is a key enzyme gene in the glycolysis pathway, which is thought to play a role in the early ripening of pears [38].
Fig. 5
The GO and KEGG enrichment analysis of genes related to early-ripening of grape berry from co-expression network analysis. Enrichment term is represented by colored dots (blue indicates high enrichment and red indicates low enrichment). a, GO terms in molecular categories from GO enrichment analysis; b, KEGG terms.
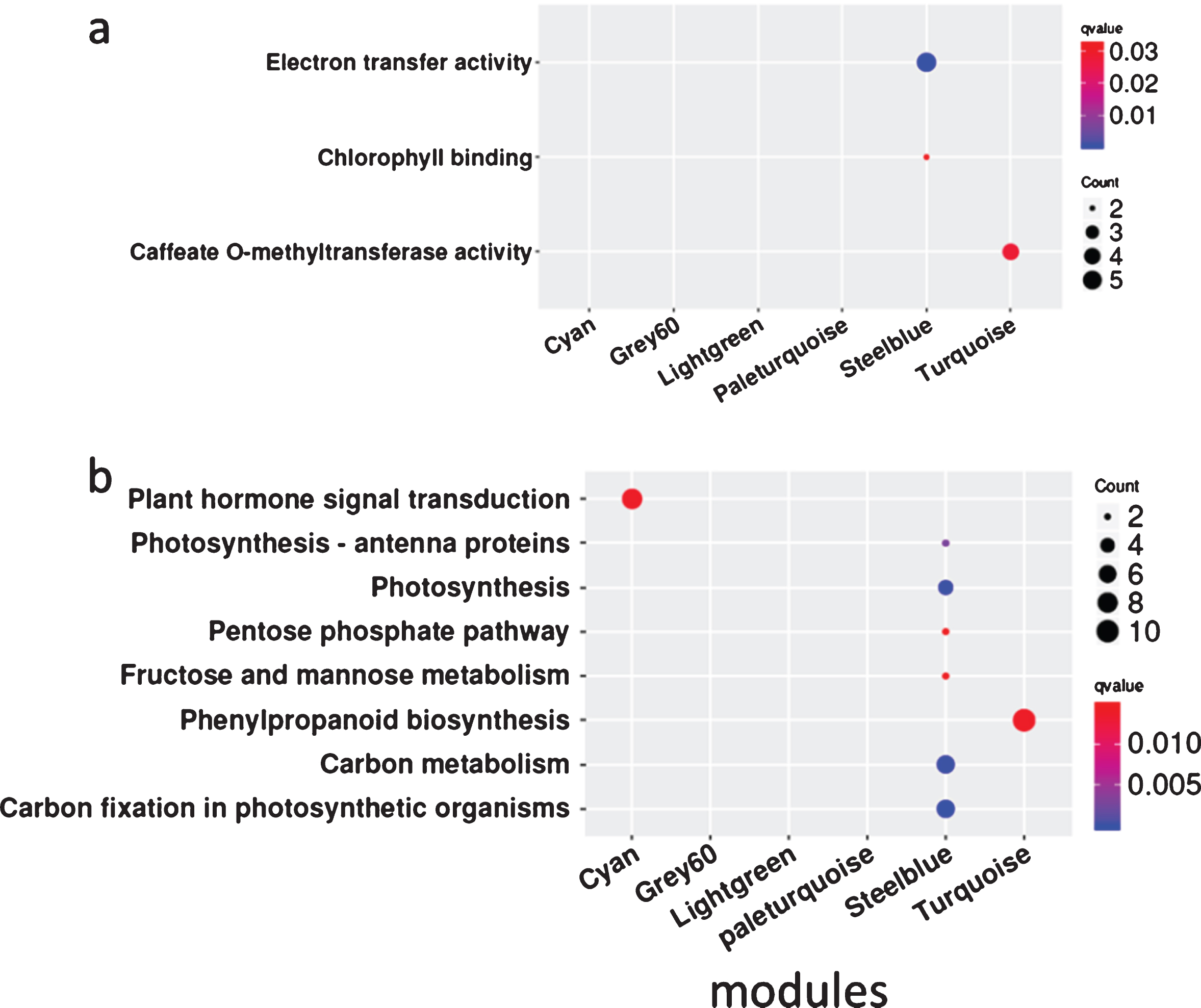
Fig. 6
Subnetwork analyses of important module genes in early development stages of grape berry. a, FZ1-lightgreen; b, FZ2-paleturquoise; c, FZ2-steelblue; d, FZ3-cyan; e, KY1-violet; f, KY2-grey60; g, KY3-turquoise.
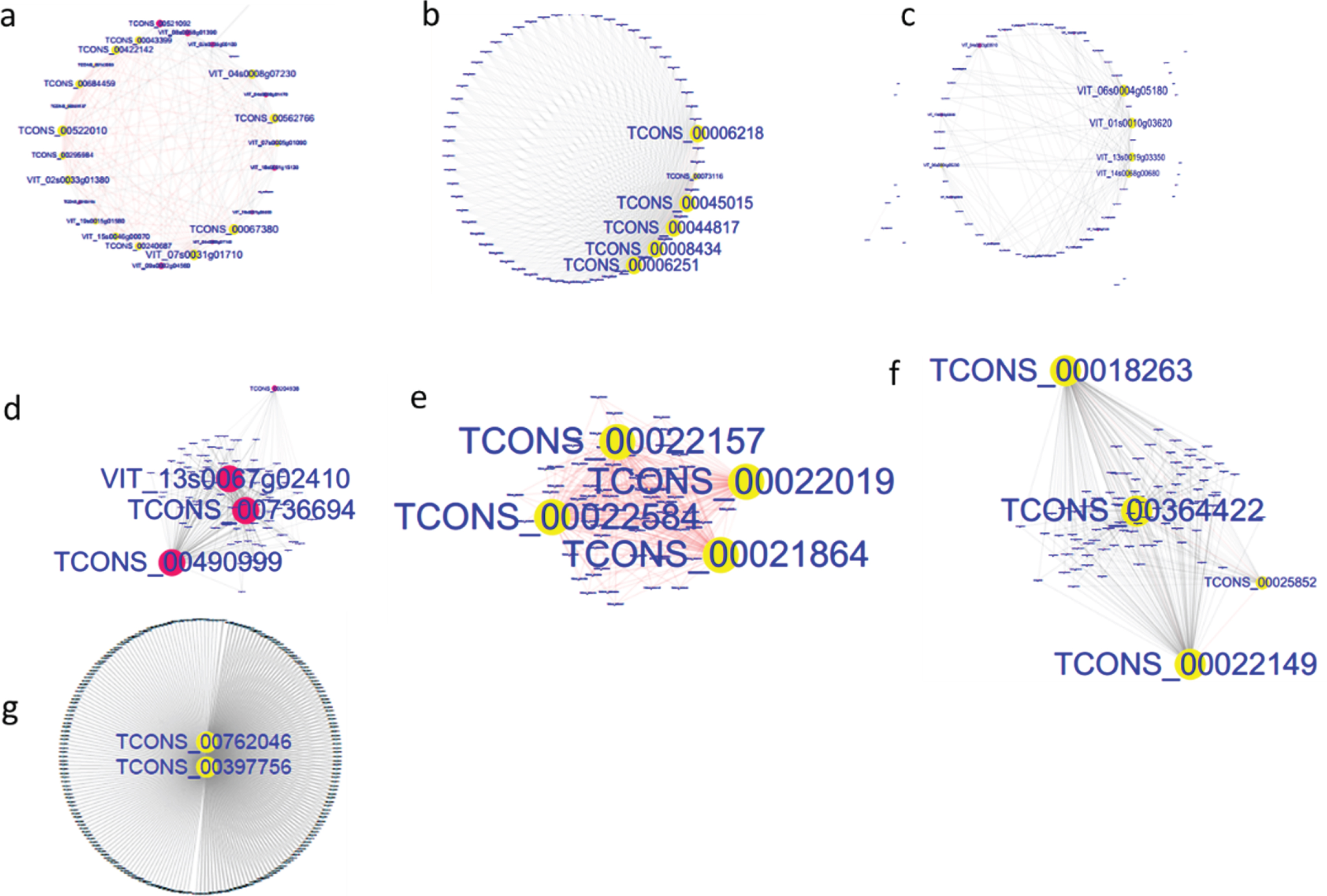
3.7Genes related to early ripening of grape berries
The lncRNAs that appeared in all three analyses, the DE-lncRNAs (FZ vs KY, FZ1 vs KY1, FZ1 vs KY2, FZ2 vs KY2, FZ2 vs KY3, and FZ3 vs KY3), TCseq analysis (clusters 4, 5, 10, and 19), and WGCNA analysis (modules MElightgreen, MEpaleturquoise, MEsteelblue, MEcyan, MEviolet, MEgrey60, and ME turquoise), were considered to be important lncRNAs in the early developmental stages of the grape berry. The Venn diagram in Fig. 7a shows that there are six overlapping lncRNAs: TCONS_00221683, TCONS_00684459, TCONS_00022149, TCONS_00167247, TCONS_00258125, and TCONS_00261813. These six lncRNAs are considered to play key roles in the early ripening of grape berries. Similar analysis was performed to obtain key mRNAs, and there were 86 overlapping mRNAs (Table S5) in the target genes of DE-lncRNAs, DE-mRNAs, TCseq analysis, and WGCNA analysis (Fig. 7b). Caffeate O-methyltransferase activity, monooxygenase activity, aspartic-type endopeptidase activity, and aspartic-type peptidase activity were significantly enriched in the GO analysis of the 86 mRNAs (Fig. 8), but none of the KEGG terms was enriched. By comparing the 86 overlapping mRNAs and hub genes in WGCNA, it was found that three mRNAs were present in both, and all were target genes of TCONS_00684459. These are WRKY1 (VIT_07s0031g01710), alternative oxidase gene AOX1D (VIT_02s0033g01380), and calcium-binding protein KIC-like gene CBP(VIT_15s0046g00070). These suggest that TCONS_00684459 may regulate the early ripening of grape berries by regulating the important target genes.
Fig. 7
Venn diagram showing the lncRNAs and mRNAs in this study among different analyses. a, lncRNAs among differential expression analysis, TCseq cluster analysis and WGCNA analysis; b, mRNAs between DE-mRNAs and targets of lncRNAs from DE-lncRNA, TCseq cluster analysis and WGCNA analysis.
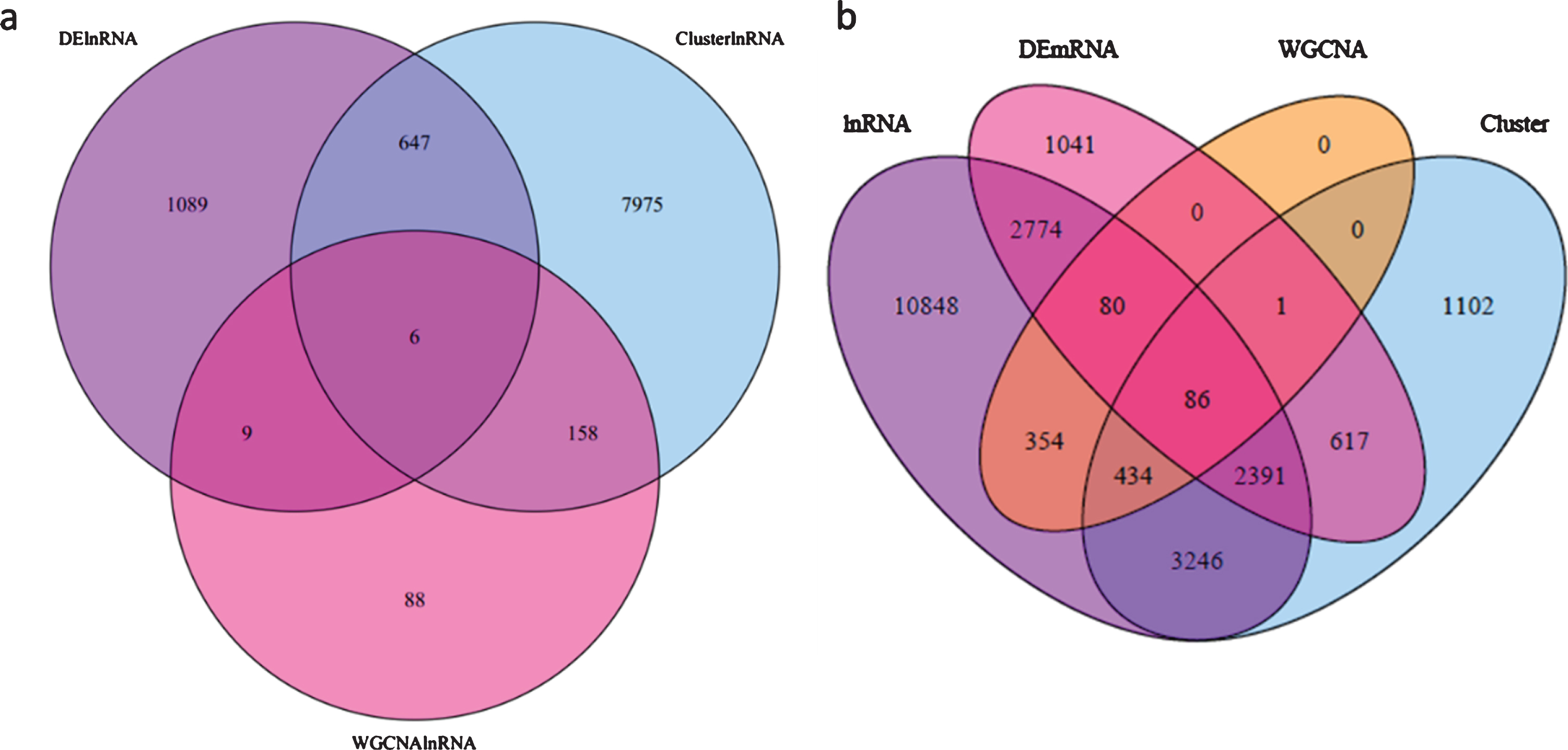
Fig. 8
GO terms in molecular categories from GO enrichment analysis of 86 overlapping mRNAs. Enrichment term is represented by colored dots (blue indicates high enrichment and red indicates low enrichment).
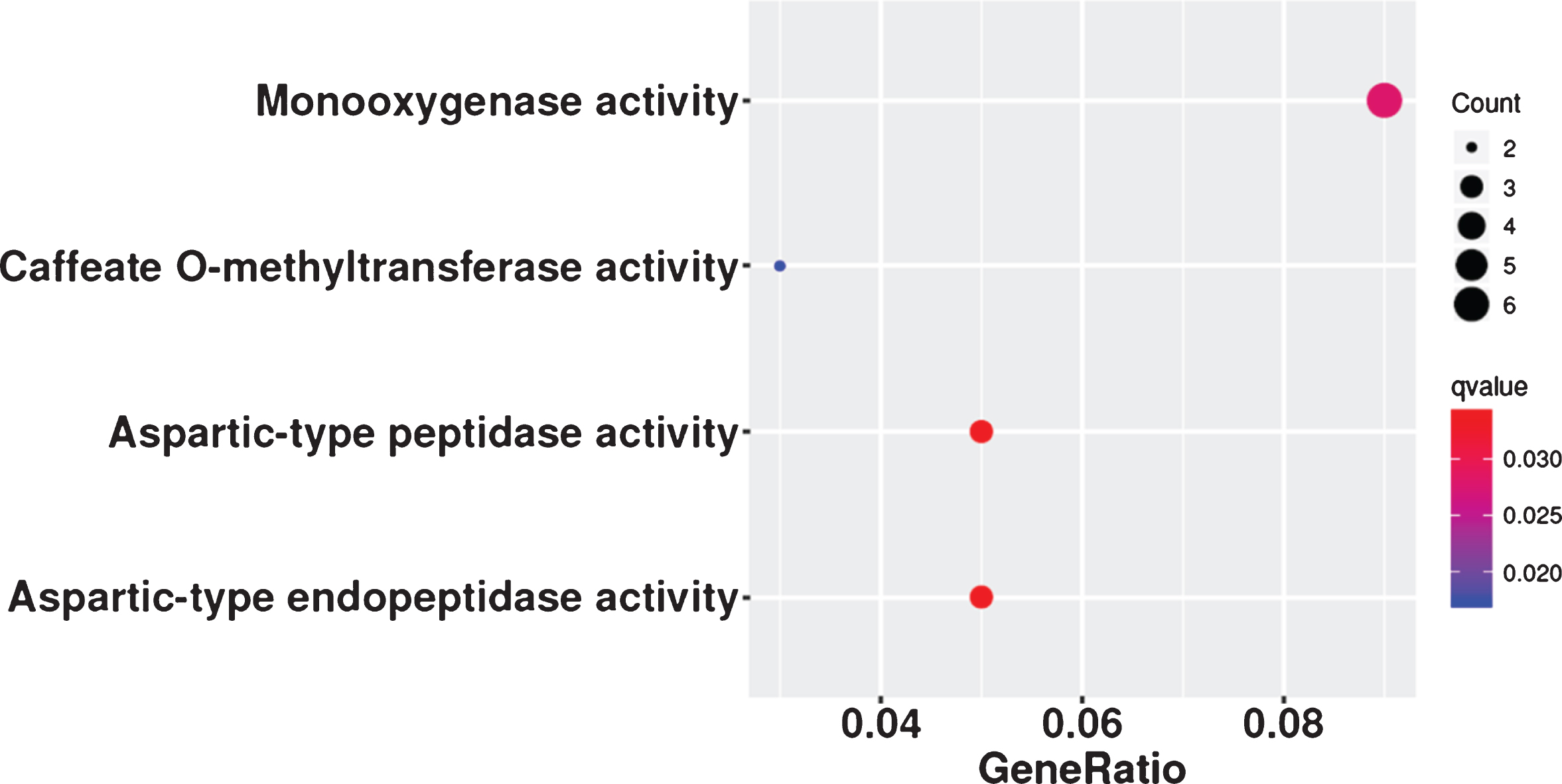
3.8qRT-PCR validation of differentially expressed genes
To assess the repeatability of the sequencing data, ten lncRNAs and four mRNAs were selected to determine their expression levels using qPCR. Six of the ten lncRNAs were those that were overlapping among DE-lncRNAs, TCseq analysis, and WGCNA analysis, and the remaining four were randomly selected from the DE-lncRNAs. The four mRNAs were GAPA-2 (VIT_14s0068g00680), WRKY51 (VIT_07s0031g01710), AOX1D (VIT_02s0033g01380), and CBP (VIT_15s0046g00070).
The validation tests were carried out on berry samples that were collected at the same developmental stages as those used in the RNA-seq experiment. Most tested lncRNAs showed similar expression trends in the qPCR data to those revealed by lncRNA-seq (Fig. 9). The expression of TCONS_00221683, TCONS_00022149, TCONS_00167247, and TCONS_00258125 in KY2 was higher than that in other stages in Kyoho and all stages detected in Fengzao, and the expression of TCONS_00261813 was the highest in KY3. The expression of TCONS_00684459 and its target genes (VIT_07s0031g01710, VIT_02s0033g01380, and VIT_15s0046g00070) was higher in FZ1 than in KY1. This result illustrates that our high-throughput data were reliable.
Fig. 9
qPCR validation of lncRNAs and mRNAs at different berry development stages in Fengzao and Kyoho. Relative quantity is based on the expression of the reference gene ubiquitin 1. X-axis indicates different stages and Y-axis indicates the expression of lncRNAs or mRNAs relative to reference gene. Data are mean±SD from three biological replicates. *, P < 0.05; **, P < 0.01 by student t test.
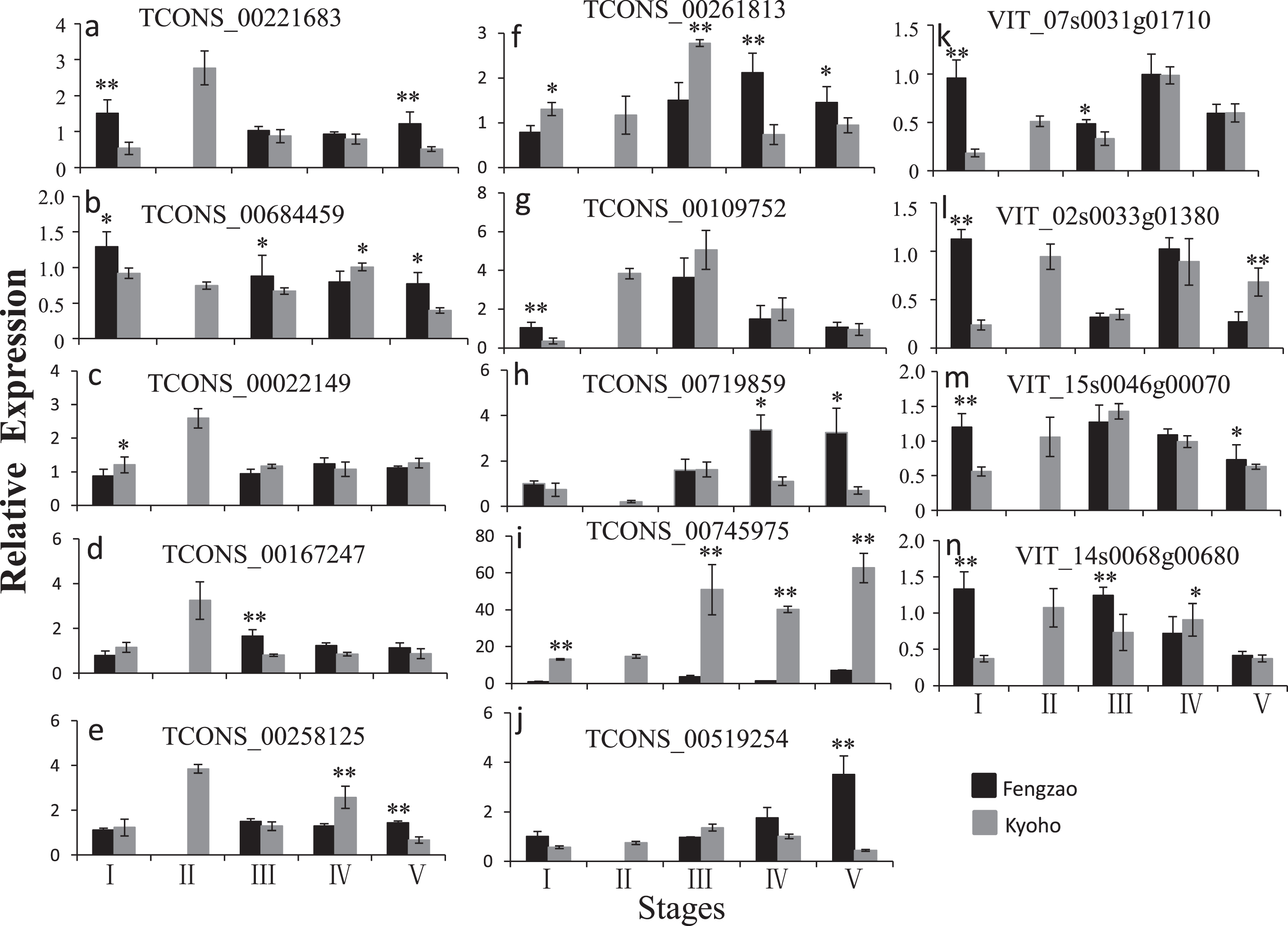
4Discussion
Recently, lncRNAs have been recognized as an important class of regulatory molecules. Plant lncRNAs are linked to biological processes such as gene silencing, regulation of flowering time, abiotic stress response, and fruit developmental pathways [39]. In previous studies, lncRNAs related to fruit development have been identified in tomato [16, 19], muskmelon [20], and strawberry [22], among others. However, the lncRNAs involved in the grape berry ripening process have remained largely unknown. To characterize the lncRNAs relevant to grape berry development, lncRNAs and their expression profiles across the key developmental stages of Kyoho and Fengzao were examined. A total of 24726 lncRNAs were identified, which included 9127 intronic lncRNAs, 13731 lincRNAs, and 1868 lncNATs. Furthermore, structural analysis showed that these lncRNAs were shorter than the mRNAs, and the lncRNAs expression levels were lower than those of the mRNAs (Fig. 1). The general features were consistent with previous studies [16, 40]. The TCseq clustering analysis classified lncRNAs into 20 clusters (Fig. 2), and the expression levels of lncRNAs varied at different developmental stages, indicating that lncRNAs play diverse roles during grape berry development. Interestingly, the expression levels of lncRNAs in clusters 4, 5, 10, and 19 changed dramatically during the early developmental stages, so they may contribute to early fruit development and ripening in Fengzao.
In our previous study, RNA-seq and miRNAs analyses were carried out on Fengzao and Kyoho during berry development, and the results indicated that superoxide dismutase (SOD) plays an important role in the early ripening of grape berries. The activity of the SOD enzyme in Fengzao was lower than that in Kyoho, except at the v
Pathogenesis-related (PR) proteins, which are generally considered as plant defense proteins, are believed to be involved in fruit ripening. Several differentially expressed genes that are related to disease-resistance proteins were observed in Fengzao and Kyoho during berry development, and the disease-resistance protein genes VIT_213s0067g01100 and tobacco mosaic virus resistance protein N-like gene VIT_200s0238g00060 were among the most differentially expressed genes detected in the study [6]. In this study, the KEGG term plant–pathogen interaction (ko04626) was enriched in cluster 19 of the TCseq analysis (Fig. S1b). Among the six overlapping lncRNAs, which appeared in the DE-lncRNAs, the TCseq analysis, and the WGCNA analysis (Fig. 7a), three (TCONS_00221683, TCONS_00022149, and TCONS_00167247) share the same target gene, VIT_03s0088g00910 (the basic form of PR protein 1), which is considered as a key gene in the early ripening of the Fengzao berry. Lijavetzky et al. [44] also observed the activation of pathogen-defense gene expression responses in the pericarp upon berry ripeness in “Muscat Hamburg”. Andthe role of PR proteins in the ripening of other fruits has also been demonstrated [45–46].
The only miRNA to be differentially expressed during all berry-development stages of Fengzao and Kyoho was miR159a [7]. The grape targets of miR159a include the GAMYB transcription factors MYB33, MYB65, and MYB101 [47], which participate in the signaling process induced by abscisic acid (ABA) accumulation in the presence of stress [48]. This suggests that the effect of miR159a on the development of grape berries is realized by a regulating-hormone signal pathway. Plant hormones are considered to be closely linked with fruit development and ripening [49]. Through the response of different hormones, the significant ripening regulations seem to be controlled primarily by ethylene, ABA, and auxin [50–52]. In this study, the KEGG term plant hormone signal transduction was enriched in DE-mRNAs, and the trans target genes of DE-lncRNAs were compared between two cultivars (Fig. 3d and 3f). There were 28, 57, and 80 DE-mRNAs that belonged to plant hormone signal transduction in the comparisons FZ vs KY, FZ2 vs KY3, and FZ3 vs KY3, respectively (Fig. 3d). Among the trans target genes of the DE-lncRNAs in FZ vs KY and FZ2 vs KY3, there were 41 and 25 target genes that belonged to plant hormone signal transduction, respectively (Fig. 3F). A similar result was observed in Cucumis melo, in which the target genes of DE-lncRNAs involved in fruit ripening were enriched plant hormone signal transduction terms [20], which is consistent with our results.
Other pathways have been demonstrated to be involved in fruit-ripening processes, for example cell-wall modification, oxidative stress, photosynthesis and glycolysis in pears [38], metabolic pathways, spliceosome, and biosynthesis of phenylpropanoids in oranges [53]. In this study, the DE-mRNAs and the target genes of DE-lncRNAs between Kyoho and its early-ripening bud Fengzao were found to be enriched in F3′5′H activity, flavanol biosynthesis, phenylpropanoid biosynthesis, and starch and sucrose metabolism pathways (Fig. 3d and Fig. 3f), which indicates that these pathways are involved in the early ripening of grape berries. The initiation of fruit ripening is mainly characterized by color change, hormone synthesis, and cell-wall dynamics [52]. Anthocyanins are secondary metabolites that play a substantial role in pigmentation and have many health benefits as antioxidants, including anti-tumor properties [54], and they accumulate in the early stage of fruit ripening [55–56]. In this study, three pathways related to anthocyanin synthesis (F3′5′H activity, flavanol biosynthesis, and phenylpropanoid biosynthesis) were enriched in DE-mRNAs and the target genes of DE-lncRNAs between Kyoho and Fengzao (Fig. 3d and 3f). These results showed that anthocyanin synthesis plays an important role in the early ripening of Fengzao, which is consistent with previous research about apple fruit development. Three different stages of apple development were used for transcriptome assembly using RNA-seq, and differentially expressed genes were involved in hormonal signaling pathways and anthocyanin biosynthesis pathways [57].
Combining differential expression analysis, TCseq analysis, and WGCNA analysis, it was found that six lncRNAs and 86 mRNAs may play key roles in the early ripening of Fengzao (Fig. 7). The six lncRNAs are TCONS_00221683, TCONS_00684459, TCONS_00022149, TCONS_00167247, TCONS_00258125, and TCONS_00261813. The 86 mRNAs not only contained genes in key hormone signal transduction pathways (VIT_03s0038g01150, VIT_05s0020g01070, VIT_04s0023g02350, and VIT_03s0038g01180), anthocyanin synthesis (VIT_06s0009g03140), starch and sucrose metabolism (VIT_12s0055g00310, VIT_06s0004g04330, and VIT_07s0289g00080) mentioned earlier, but also included methyltransferase (VIT_14s0066g01450, VIT_15s0048g02490, and VIT_15s0048g02450) and WRKY transcription factors (VIT_08s0058g01390, VIT_07s0031g01710, and VIT_04s0008g01470) (Table S2).
Methylation of DNA is an epigenetic modification that affects many biological processes and is widely presented in animals and plants. Some studies have revealed that DNA methylation plays a very important role in the growth and development of fruit [58–59]. In our previous study, different concentrations of azacitidine (5-azaC), an inhibitor of methyltransferase, were sprayed onto Kyoho grape berries, and the results showed that treatment with 100μM 5-azaC could cause berry ripening in Kyohoto occur 20 days earlier than in the control [60]. In this study, three genes (VIT_14s0066g01450, VIT_15s0048g02490, and VIT_15s0048g02450) related to methylation appeared to play important roles in the early ripening of Fengzao, which further confirms the role of methylation in the development and early ripening of grape berries.
The WRKY-family genes are generally considered to be stress tolerance regulators, but recent studies also implicate WRKY transcription factors in fruit development. It has been predicted that FvWRKY genes may operate in the ABA, indole-3-acetic acid, and sucrose signaling networks during strawberry fruit development [61]. The differentially expressed WRKY70 gene was found to be co-expressed with several DE-lncRNAs in the transcriptome of Cucumis melo fruit at four developmental stages, so it was speculated that WRKY70 might be a negative regulatory factor in the process of fruit development [20]. Of the 86 important mRNAs, three are WRKY transcription factors (Table S2), which indicates that WRKY plays a role in grape berry development. Further analysis of the 86 overlapping mRNAs and hub genes in WGCNA found that WRKY1 (VIT_07s0031g01710), alternative oxidase gene AOX1D (VIT_02s0033g01380), and calcium-binding protein KIC-like gene CBP (VIT_15s0046g00070) was present in both of them, and this shows that they are key genes in the early-ripening process of Fengzao. Interestingly, WRKY1, AOX1D, and CBP are all target genes of TCONS_00684459. Results from RNA-seq and qPCR showed that the expression of TCONS_00684459, WRKY1, AOX1D, and CBP was much higher in FZ1 than in other stages (Fig. 9). These results suggest that TCONS_00684459 may regulate the early ripening of grape berries by regulating the important target genes. However, the function of TCONS_00684459 and its regulatory role on targets needs to be explored in more depth.
5Conclusions
Fruit ripening is a well-regulated process in which many pathways and genes are probably involved. In this study, the oxidoreductase activity, plant–pathogen interaction, plant hormone signal transduction, flavanol biosynthesis, phenylpropanoid biosynthesis, and starch and sucrose metabolism pathways were found to be enriched in the target genes of DE-lncRNAs. Six key lncRNAs were identified (TCONS_00221683, TCONS_00684459, TCONS_00022149, TCONS_00167247, TCONS_00258125, and TCONS_00261813) that may regulate the early ripening of grape berries by regulating the important target genes. The results contribute to the understanding of the role of lncRNAs in the early ripening of grape berries and will provide new insights for molecular breeding of grapes.
Funding
This work was financially supported by Natural Science Foundation of China (NSFC: 31672106, U1904113), Zhongyuan Science and Technology Innovation Leaders (194200510007), National Key Research and Development Program of China (2018YFD1000105), Key Scientific Research Projects of Colleges and Universities in Henan Province (20A210009), and Research Funding for Young Academic Leaders of Henan University of Science and Technology (4026-13490004).
Conflict of interest
The authors have no conflict of interest to report.
Supplementary material
[1] The supplementary material is available in the electronic version of this article: https://dx.doi.org/10.3233/JBR-190518.
Acknowledgments
This work was financially supported by Natural Science Foundation of China (NSFC: 31672106, U1904113), Zhongyuan Science and Technology Innovation Leaders (194200510007), National Key Research and Development Program of China (2018YFD1000105), Key Scientific Research Projects of Colleges and Universities in Henan Province (20A210009), and Research Funding for Young Academic Leaders of Henan University of Science and Technology (4026-13490004). The Funding bodies were not involved in the design of the study and collection, analysis, and interpretation of data and in writing the manuscript.
References
[1] | Giovannoni J . Molecular Biology Of Fruit Maturation And Ripening. Annu Rev Plant Biol. (2001) ;52: :725–49. |
[2] | Osorio S , Scossa F , Fernie A . Molecular regulation of fruit ripening. Front Plant Sci. (2013) ;4: :198. |
[3] | Li Y , Ma R , Xu Z , Wang J , Chen T , Chen F , et al. Identification and quantification of anthocyanins in Kyoho grape juice-making pomace, cabernet sauvignon grape winemaking pomace and their fresh skin. J Sci Food Agr. (2013) ;93: (6):1404–11. |
[4] | Guo DL , Zhang GH . A new early-ripening grape cultivar-‘Fengzao’. Acta Horticulturae. (2015) ;1082: :153–6. |
[5] | Guo DL , Yu YH , Xi FF , Shi YY , Zhang GH . Histological and Molecular Characterization of Grape Early Ripening Bud Mutant. Int J Genomics. 2016:5620106. |
[6] | Guo DL , Xi FF , Yu YH , Zhang XY , Zhang GH , Zhong GY . Comparative RNA-Seq profiling of berry development between table grape ‘Kyoho’ and its early-ripening mutant ’Fengzao’. BMC Genomics. (2016) ;17: (1):795. |
[7] | Guo DL , Li Q , Lv WQ , Zhang GH , Yu YH . MicroRNA profiling analysis of developing berries for ‘Kyoho’ and its early-ripening mutant during berry ripening. BMC Plant Biol. (2018) ;18: (1):285. |
[8] | Grimplet J , Deluc LG , Tillett RL , Wheatley MD , Schlauch KA , Cramer GR , et al. Tissue-specific mRNA expression profiling in grape berry tissues. BMC Genomics. (2007) ;8: (1):187. |
[9] | Pilati S , Perazzolli M , Malossini A , Cestaro A , Demattè L , Fontana P , et al. Genome-wide transcriptional analysis of grapevine berry ripening reveals a set of genes similarly modulated during three seasons and the occurrence of an oxidative burst at veraison. BMC Genomics. (2007) ;8: (1):428. |
[10] | Ni PY , Ji XR , Guo DL . Genome-wide identification, characterization, and expression analysis of GDSL-type esterases/lipases gene family in relation to grape berry ripening. Sci. Hortic. (2020) ;264: :109162. |
[11] | Chekanova JA . Long non-coding RNAs and their functions in plants. Curr Opin Plant Bio. (2015) ;27: :207–16. |
[12] | Ma L , Bajic VB , Zhang Z . On the classification of long non-coding RNAs. RNA Biol. (2013) ;10: (6):924–33. |
[13] | Mercer TR , Dinger ME , Mattick JS . Long non-coding RNAs: Insights into functions. Nat Rev Genet. (2009) ;10: (3):155–9. |
[14] | Forestan C , Aiese Cigliano R , Farinati S , Lunardon A , Sanseverino W , Varotto S . Stress-induced and epigenetic-mediated maize transcriptome regulation study by means of transcriptome reannotation and differential expression analysis. Sci Rep. (2016) ;6: (1):30446. |
[15] | Boerner S , Mcginnis KM . Computational identification and functional predictions of long noncoding RNA in zea mays. Plos One. (2012) ;7: (8):e43047. |
[16] | Zhu B , Yang Y , Li R , Fu D , Wen L , Luo Y , et al. RNA sequencing and functional analysis implicate the regulatory role of long non-coding RNAs in tomato fruit ripening. J Exp Bot. (2015) ;66: (15):4483–95. |
[17] | Zhang YC , Chen Y-Q . Long noncoding RNAs: New regulators in plant development. Biochem Bioph Res Co. (2013) ;436: (2):111–4. |
[18] | Liu X , Hao L , Li D , Zhu L , Hu S . Long non-coding RNAs and their biological roles in plants. Genom Proteom Bioinf. (2015) ;13: (3):137–47. |
[19] | Wang M , Zhao W , Gao L , Zhao L . Genome-wide profiling of long non-coding RNAs from tomato and a comparison with mRNAs associated with the regulation of fruit ripening. BMC Plant Biol. (2018) ;18: (1):75. |
[20] | Tian Y , Bai S , Dang Z , Hao J , Zhang J , Hasi A . Genome-wide identification and characterization of long non-coding RNAs involved in fruit ripening and the climacteric in Cucumis melo. BMC plant biol. (2019) ;19: (1):369. |
[21] | Zhang G , Chen D , Zhang T , Duan A , Zhang J , He C . Transcriptomic and functional analyses unveil the role of long non-coding RNAs in anthocyanin biosynthesis during sea buckthorn fruit ripening. DNA Res. (2018) ;25: (5):465–76. |
[22] | Bai L , Chen Q , Jiang L , Lin Y , Ye Y , Liu P , et al. Comparative transcriptome analysis uncovers the regulatory functions of long noncoding RNAs in fruit development and color changes of Fragaria pentaphylla. Hortic Res. (2019) ;6: (1):42. |
[23] | Coombe BG . Growth stages of the grapevine: Adoption of a system for identifying grapevine growth stages. Aust J Grape Wine Res. (1995) ;1: (2):104–10. |
[24] | Guo DL , Guo MX , Zhang GH . Comparisons of berry development characteristics between the early ripening bud mutants of grape and their parents. Plant Physiol J. (2014) ;50: (11):1733–41. |
[25] | Rienth M , Torregrosa L , Ardisson M , De Marchi R , Romieu C . Versatile and efficient RNA extraction protocol for grapevine berry tissue, suited for next generation RNA sequencing. Aust J Grape Wine Res. (2014) ;20: (2):247–54. |
[26] | Bolger AM , Lohse M , Usadel B . Trimmomatic: A flexible trimmer for Illumina sequence data. Bioinformatics. (2014) ;30: (15):2114–20. |
[27] | Jaillon O , Aury J-M , Noel B , Policriti A , Clepet C , Casagrande A , et al. The grapevine genome sequence suggests ancestral hexaploidization in major angiosperm phyla. Nature. (2007) ;449: (7161):463–7. |
[28] | Langmead B , Trapnell C , Pop M , Salzberg SL . Ultrafast and memory-efficient alignment of short DNA sequences to the human genome. Genome Biol. (2009) ;10: (3):25. |
[29] | Kim D , Langmead B , Salzberg SL . HISAT: a fast spliced aligner with low memory requirements. Nat Methods. (2015) ;12: (4):357–60. |
[30] | Anders S , Pyl PT , Huber W . HTSeq-a Python framework to work with high-throughput sequencing data. Bioinformatics. (2015) ;31: (2):166–9. |
[31] | Trapnell C , Williams BA , Pertea G , Mortazavi A , Kwan G , van Baren MJ , et al. Transcript assembly and quantification by RNA-Seq reveals unannotated transcripts and isoform switching during cell differentiation. Nat Biotechnol. (2010) ;28: (5):511–5. |
[32] | Kong L , Zhang Y , Ye ZQ , Liu XQ , Zhao SQ , Wei L , et al. Assess the protein-coding potential of transcripts using sequence features and support vector machine. Nucleic Acids Res. (2007) ;35: :345–9. |
[33] | Finn RD , Bateman A , Clements J , Coggill P , Eberhardt RY , Eddy SR , et al. Pfam: The protein families database. Nucleic Acids Res. (2014) ;42: :222–30. |
[34] | Young MD , Wakefield MJ , Smyth GK , Oshlack A . Gene ontology analysis for RNA-seq: accounting for selection bias. Genome Biol. (2010) ;11: (2):14. |
[35] | Langfelder P , Horvath S . WGCNA: an R package for weighted correlation network analysis. BMC bioinformatics. (2008) ;9: (1):559. |
[36] | Zhang B , Horvath S . A general framework for weighted gene co-expression network analysis. Stat Appl Genet Mol Biol. (2005) ;4: (1):17. |
[37] | Yu YH , Bai L , YU KK , Yang SD , Zhang GH , Guo DL . Grape (Vitis davidii) VdGATA2 functions as a transcription activator and enhances powdery mildew resistance via the active oxygen species pathway. Sci. Hortic. (2020) ;267: :109327. |
[38] | Liu X , Zhai R , Feng W , Zhang S , Wang Z , Qiu Z , et al. Proteomic analysis of ‘Zaosu’pear (Pyrus bretschneideri Rehd.) and its early-maturing bud sport. Plant Sci. (2014) ;224: :120–35. |
[39] | Liu M , Pirrello J , Chervin C , Roustan J-P , Bouzayen M . Ethylene control of fruit ripening: Revisiting the complex network of transcriptional regulation. Plant Physiol. (2015) ;169: (4):2380–90. |
[40] | Tang W , Zheng Y , Dong J , Yu J , Yue J , Liu F , et al. Comprehensive transcriptome profiling reveals long noncoding RNA expression and alternative splicing regulation during fruit development and ripening in kiwifruit (Actinidia chinensis). Front Plant Sci. (2016) ;7: :335. |
[41] | Xi FF , Guo LL , Yu YH , Wang Y , Li Q , Zhao HL , et al. Comparison of reactive oxygen species metabolism during grape berry development between ‘Kyoho’ and its early ripening bud mutant ‘Fengzao’. Plant Physiol Bioch. (2017) ;118: :634–42. |
[42] | Sunkar R , Kapoor A , Zhu J-K . Posttranscriptional induction of two Cu/Zn superoxide dismutase genes in Arabidopsis is mediated by downregulation of miR398 and important for oxidative stress tolerance. Plant Cell. (2006) ;18: (8):2051–65. |
[43] | Tang J , Chu C . MicroRNAs in crop improvement: Fine-tuners for complex traits. Nat plants. (2017) ;3: (7):17077. |
[44] | Lijavetzky D , Carbonell-Bejerano P , Grimplet Jrm , Bravo G , Flores P . Berry flesh and skin ripening features in vitis vinifera as assessed by transcriptional profiling. Plos One. (2012) ;7: :e39547. |
[45] | Clendennen SK , May GD . Differential gene expression in ripening banana fruit. Plant Physiol. (1997) ;115: (2):463–9. |
[46] | Goñi Ramos Ó , Sánchez Ballesta MT , Merodio C , Escribano MI . Ripening-related defense proteins in Annona fruit. (2010) ;55: (3):169–73. |
[47] | Pantaleo V , Szittya G , Moxon S , Miozzi L , Moulton V , Dalmay T , et al. Identification of grapevine microRNAs and their targets using high-throughput sequencing and degradome analysis. Plant J. (2010) ;62: (6):960–76. |
[48] | Reyes JL , Chua N-H . ABA induction of miR159 controls transcript levels of two MYB factors during Arabidopsis seed germination. Plant J. (2007) ;49: (4):592–606. |
[49] | Seymour GB , stergaard L , Chapman NH , Knapp S , Martin C . Fruit development and ripening. Annu Rev Plant biol. (2013) ;64: (1):219–41. |
[50] | Mcatee P , Karim S , Schaffer R , David K . A dynamic interplay between phytohormones is required for fruit development, maturation, and ripening. Front Plant Sci. (2013) ;4: :79. |
[51] | Kumar R , Khurana A , Sharma AK . Role of plant hormones and their interplay in development and ripening of fleshy fruits. J Exp Bot. (2014) ;65: (16):4561–75. |
[52] | Giovannoni JJ . Fruit ripening mutants yield insights into ripening control. Curr Opin Plant Bio. (2007) ;10: (3):283–9. |
[53] | Zhang YJ , Wang XJ , Wu JX , Chen SY , Chen H , Chai LJ , et al. Comparative transcriptome analyses between a spontaneous late-ripening sweet orange mutant and its wild type suggest the functions of ABA, sucrose and JA during citrus fruit ripening. Plos One. (2014) ;9: (12):e116056. |
[54] | Jaakola L . New insights into the regulation of anthocyanin biosynthesis in fruits. Trends Plant Sci. (2013) ;18: (9):477–83. |
[55] | Spinardi A , Cola G , Gardana CS , Mignani I . Variation of anthocyanins content and profile throughout fruit development and ripening of highbush blueberry cultivars grown at two different altitudes. Front Plant Sci. (2019) ;10: :1045. |
[56] | Kok D . Grape growth, anthocyanin and phenolic compounds content of early ripening Cv. Cardinal table grape (V. vinifera L.) as affected by various doses of foliar biostimulant applications with gibberellic acid. Erwerbs-Obstbau. (2018) ;60: (3):253–9. |
[57] | Onik J , Hu X , Lin Q , Wang Z . Comparative transcriptomic profiling to understand pre- and post-ripening hormonal regulations and anthocyanin biosynthesis in early ripening apple fruit. Molecules. (2018) ;23: (8):1908. |
[58] | Giovannoni J , Nguyen C , Ampofo B , Zhong S , Fei Z . The Epigenome and transcriptional dynamics of fruit ripening. Annu Rev Plant Biol. (2017) ;68: (1):61–84. |
[59] | Ma C , Jing C , Chang B , Yan J , Liang B , Liu L , et al. The effect of promoter methylation on MdMYB1 expression determines the level of anthocyanin accumulation in skins of two non-red apple cultivars. BMC Plant Biol. (2018) ;18: (1):108. |
[60] | Guo DL , Li Q , Zhao HL , Wang ZG , Zhang GH , Yu YH . The variation of berry development and DNA methylation after treatment with 5-azaC on ‘Kyoho’grape. Sci Hortic. (2019) ;246: :265–71. |
[61] | Zhou H , Li Y , Zhang Q , Ren S , Shen Y , Qin L , et al. Genome-wide analysis of the expression of WRKY family genes in different developmental stages of wild strawberry (Fragaria vesca) fruit. Plos One. (2016) ;11: (5):e0154312. |