Technologies for monitoring patients with Alzheimer’s disease: A systematic mapping study and taxonomy
Abstract
Alzheimer’s disease (AD) is an incurable disease and a type of dementia. About 55 million people around the world have AD. However, technologies have been used to assist in the healthcare of AD, supporting physicians in the palliative care of patients. This article presents a systematic mapping study (SMS) to identify articles that use technologies to monitor patients with AD in order to show an overview of the literature, identifying gaps and research opportunities in this field. The scientific contribution of this work is to identify monitoring technologies related to AD and highlight current trends on the subject. The paper uses the term technologies as hardware infrastructure and devices or systems without considering software technologies. In addition, this article proposes a taxonomy for the domain of technologies applied to AD patients. The SMS study was conducted in six databases, including articles from 1997 to 2021. An initial search resulted in 7,781 articles. After applying filter criteria, throwing automatic selection on databases, and manual assortment, 171 articles were selected. Subsequently, a second search was performed to reduce the list of articles and filter by the specific search objective of articles focused on technologies for monitoring with tracking, resulting in 74 works. The main results obtained are: (1) a relevant number of articles (43.42%) reported solutions used in sensor-based devices; (2) several works (33.33%) have the interaction focus on Position/Distance/Proximity/Location sensor type; (3) another group of articles has a secondary focus on Emergency help (18.97%). The results indicated the need for technologies to help caregivers monitor patients, in addition to evidence of research opportunities in palliative care and support for the daily activities of AD patients.
1.Introduction
According to the World Health Organization (WHO) [85], about 55 million people around the world have Alzheimer’s disease (AD) or another type of dementia. As reported by the Pan American Health Organization [55], Alzheimer’s is one of the top ten death-causing diseases in the world and one of the most prevalent diseases of dementia. AD is characterized as a syndrome – usually of a chronic or progressive nature – for which there is no cure or treatment, with deterioration of cognitive function (ability to process thoughts), which can be expected from normal aging. AD can affect memory, thinking, orientation, understanding, calculation, learning ability, language, and judgment, but consciousness is not affected.
The impairment of cognitive function is usually followed and sometimes preceded by the deterioration of emotional control, social behavior, or motivation. Although dementia mainly affects elderly people, the symptoms can start earlier, which influences the fact that there are almost 10 million new cases each year around the world [3]. AD is the most common form of dementia and may be considered for 60–70% of cases [4].
The total number of people with dementia is expected to reach 78 million in 2030 and 139 million in 2050. Much of this increment is attributable to the growing number of people with dementia living in low and middle-income countries [4]. The WHO has developed a global action plan proposed by 2025, in which the global production of dementia research should double the number of scientific publications, 50% of countries should routinely collect data on the leading indicators of dementia, and 75% of countries should have support for dementia care providers [84]. The alarming prevalence of Alzheimer’s disease and the absence of any effective treatment made this disease an important issue; it has been highlighted as a priority by the nations of the G8 for setting an ambition to identify a cure or a disease-modifying therapy for dementia by 2025 [21].
Dementia causes physical, psychological, social, and economic impacts, not only on patients but also on their caregivers, families, and society in general, as it is one of the main causes of disability and dependency among the elderly worldwide [85]. There is often a lack of awareness and understanding of dementia, resulting in stigmatization and barriers to diagnosis and care. The impact of the disease on caregivers, the family, and society, in general, can be physical, psychological, social, and economic [85]. Caregivers who assist individuals with dementia often feel stressed, frustrated with the amount of time required to support them, and emotionally challenged. In addition, cognitive function may progressively decrease over time in patients, in variations that fluctuate throughout the day or over the long term, as the neurological system is used [12]. Carer support and services should focus on preventing a decline in mental and physical health and improving social well-being in line with carers’ needs. Most people with dementia are cared for by family members or other unpaid carers without any additional support. Carers often face numerous financial, social, and psychological stressors. Contributing to increasing the cost of care for patients. In 2019, the global cost of dementia was estimated to be 1.3 trillion US$, and 61% of patients live in low and middle-income countries [26].
Alzheimer’s disease can only be detected currently after symptoms start to appear, but monitoring solutions are emerging with the use of electronic devices to care for these patients in the most diverse stages of the disease, therefore, technology presents possibilities for palliative care support AD patients. According to [12], research works on information and communication technologies11 (ICTs) for the treatment of dementia demonstrated how the successful incorporation of technology in everyday practices implies a set of judgments and attitudes of value about the best way to care for and make decisions for someone else.
Systematic reviews developed in the past years show a list of technologies that can be used to monitor people with Alzheimer’s disease. Some of them describe solutions for use in assisting people with Alzheimer’s and other dementias [32,77]. Others describe technologies used in practice for care [71]. The SMS found were developed between 2017 and 2019. There are few systematic reviews focused on technologies for Alzheimer’s disease that are recent and up-to-date.
The scientific contribution of this article is to classify how technologies are used to monitor patients with Alzheimer’s disease. The knowledge produced by this research was systematized in a taxonomy of technologies for monitoring patients with AD. The study was conducted from six search databases, including articles from 1997 to 2021. The paper uses the term technologies as hardware infrastructure and devices or systems (e.g., smart homes) without considering software technologies, such as mobile apps and machine learning algorithms. The article is divided into four sections. The first section introduces the theme, followed by Section 2, which defines the research methodology. Section 3 comments on the results for each research question. Finally, Section 4 assesses the article’s contributions and research gaps.
2.Methodology
This work applies the systematic mapping study (SMS) proposed by [58] as a methodology for conducting a literature review about technologies for monitoring patients with AD. The execution consists of four steps: 1) define research questions; 2) define the search process; 3) establish the criteria for filtering the results and 4) perform the analysis and organize the results.
2.1.Research questions
Table 1 shows the research questions, which consist of one General Question (GQ), two Research Questions (RQ), one Focal Question (FQ), and one Statistical question (SQ). The General Question addresses what kind of technologies are used. The Research Questions focus on the interaction of technologies and patients. The Statistical Question evaluates the distribution of articles’ publications per country. Finally, the Focal Questions focus on perceived trends.
Table 1
Research questions
ID | Question |
GQ | What technologies are being used in the care of patients with AD? |
RQ1 | What are the usage models between patients and technology? |
RQ2 | Has the application been tested by real patients? |
SQ | What is the distribution of articles by country? |
FQ | What are the perceived trends? |
2.2.Research process
The study of terms for the definition of the search string was based on: Alzheimer’s disease and technologies for patient care. The boolean search expression presented in Table 2 is divided into two sets of interest generating a search string base. These sets undergo disjunction and then are unified by boolean conjunctions. Synonyms and words are derived from a radical in order to obtain the most faithful results possible, respecting the relevance of variations in medical language. Singular and plural variations were used only when the database does not automatically consider them.
Table 2
Search terms
Main terms | Alternative terms |
Alzheimer | (alzheimer OR “Alzheimer’s Disease” OR “Alzheimer Patients” OR “Alzheimer’s Care”) AND |
Technologies | (care OR detect OR track OR monitoring OR “Assistive Technology” OR “Patient Monitoring” OR device OR smartphone OR smartphones OR mobile application OR mobile OR mHealth OR app) |
Table 3 shows databases selected for the research. PubMed and Journal of Medical Internet Research (JMIR) stand out as literature bases in the area of health and natural sciences, while the other bases are references in computer science.
Table 3
Databases used in the SMS
Database | Access URL |
ACM Digital Libray | https://dl.acm.org |
IEEE Xplore Digital Library | https://ieeexplore.ieee.org/Xplore/home.jsp |
Journal of Medical Internet Research (JMIR) | https://www.jmir.org/ |
PubMed Central | https://www.ncbi.nlm.nih.gov/pmc/ |
Science Digital Library | https://www.sciencedirect.com/ |
Springer Library | https://link.springer.com/ |
Table 4
Search string used in each database
Database | Filter option | Search string |
ACM Digital Library | Advanced Search (by Title and Abstract) | (alzheimer OR “Alzheimer’s Disease” OR “Alzheimer Patients” OR “Alzheimer’s Care”) AND (care OR detect OR track OR monitoring OR “Assistive Technology” OR “Patient Monitoring” OR device OR smartphone OR smartphones OR mobile application OR mobile OR mHealth OR app) |
IEEE Xplore Digital Library | Command Search (by Publication Title and Abstract) | NOT ((“Publication Title”: amyloid-β OR peptide OR β OR diagnosis OR genotypes OR review OR biomarkers OR magnetict ressonance OR Imaging OR Electromagnetic OR MRI OR fMRI OR molecular OR biomimetic OR hippocampal OR protein OR Electroencephalography OR Bioelectronics OR neural OR biomedical) AND (“Abstract”: amyloid-β OR peptide OR β OR diagnosis OR genotypes OR review OR biomarkers OR magnetict ressonance OR Imaging OR Electromagnetic OR MRI OR fMRI OR molecular OR biomimetic OR hippocampal OR protein OR Electroencephalography OR Bioelectronics OR neural OR biomedical)) AND ((“Publication Title”:alzheimer OR Alzheimer’s Disease OR Alzheimer Patients OR Alzheimer’s Care) AND (“Publication Title”: care OR detect OR track OR monitoring OR Assistive Technology OR Patient Monitoring OR device OR smartphone OR smartphones OR mobile application OR mobile OR mHealth OR app)) AND ((“Abstract”:alzheimer OR Alzheimer’s Disease OR Alzheimer Patients OR Alzheimer’s Care) AND (“Abstract”: care OR detect OR track OR monitoring OR Assistive Technology OR Patient Monitoring OR device OR smartphone OR smartphones OR mobile application OR mobile OR mHealth OR app)) |
Journal of Medical Internet Research (JMIR) | Article Search (by Title, Abstract and Keywords) | (alzheimer OR “Alzheimer’s Disease” OR “Alzheimer Patients” OR “Alzheimer’s Care”) AND (care OR detect OR track OR monitoring OR “Assistive Technology” OR “Patient Monitoring” OR device OR smartphone OR smartphones OR mobile application OR mobile OR mHealth OR app) |
PubMed Central | Advanced Search (by Title and Abstract) | ((((((((((((((((((alzheimer[Title/Abstract]) AND ((detect[Title/Abstract]) OR (track[Title/Abstract]) OR (monitoring[Title/Abstract]) OR (Assistive Technology[Title/Abstract]) OR (Patient Monitoring[Title/Abstract]) OR (device[Title/Abstract]) OR (smartphone[Title/Abstract]) OR (mobile[Title/Abstract]) OR (application[Title/Abstract]))) NOT (genetic)) NOT (biomarkers)) NOT (amyloid-β)) NOT (peptide)) NOT (genotypes)) NOT (resonance)) NOT (Electromagnetic)) NOT (MRI)) NOT (fMRI)) NOT (molecular)) NOT (biomimetic)) NOT (hippocampal)) NOT (protein)) NOT (Electroencephalography)) NOT (Bioelectronics)) NOT (neural)) NOT (biomedical) |
Science Digital Library | Advanced Search (by Title, abstract or author-specified keywords) + Publication title (by Engineering and Computer Science disciplines) | (alzheimer) AND (detect OR track OR monitoring OR “Assistive Technology” OR “Patient Monitoring” OR device OR smartphone) |
Springer Library | Discipline (by Computer Science) + Subdiscipline (by Information Systems Applications (incl. Internet) and User Interfaces and Human Computer Interaction) | (alzheimer OR “Alzheimer’s Disease” OR “Alzheimer Patients” OR “Alzheimer’s Care”) AND (detect OR track OR monitoring OR “Assistive Technology” OR “Patient Monitoring” OR device OR smartphone OR smartphones OR mobile application OR mobile OR mHealth OR app) |
In order to perform the most comprehensive research, the search string was adapted to be used in each database with their respective specifications. Table 4 presents the specific search string applied in each repository, respectively.
The first search was performed in the ACM Digital Library repository with the string relating to the term Alzheimer and its variations and terms related to articles that contain technological solutions. The second search was in the IEEE Xplore Digital Library repository with the string relating the term Alzheimer and its variations, adding terms related to articles that contain technological solutions and removing terms related to Alzheimer’s in health and also specifying the fields in which the keywords should be found, which were “Publication Title” and “Abstract”. The string applied to perform the third search in the Journal of Medical Internet Research (JMIR) included Alzheimer’s and its variations, adding the terms related to technological solutions. The string applied to perform the fourth search in the PubMed Central repository included the term Alzheimer and its variations, terms related to articles that contain technological solutions, and keywords for removal of terms related to Alzheimer’s in the field of health. The search also specified the fields in which the keywords should be found: “Title” and “Abstract”. The fifth search in the Science Digital Library repository was carried out with the string relating to the term Alzheimer not including its variations, and terms related to technological solutions. Finally, the last search was performed in the Springer Library repository and was carried out with the string relating the term Alzheimer and its variations and terms related to technological solutions.
The selected articles were stored in the Mendeley22 tool and later exported for bibliometric analysis in VOSViewer.33
2.3.Results filter
Figure 1 presents the filtering process. The process search applied the string in databases for title, abstract, and keywords fields (when available, according to Table 4). The search resulted in articles from 1997 to 2021.
After querying the databases, the articles passed by a filter, considering exclusion criteria (EC). This review considered as a selected paper the studies that satisfied all EC. The EC allowed the elimination of any noise generated in the research. Table 5 shows the exclusion criteria.
EC1 excludes articles the string search does not match, as represented by Table 4. EC2 removes articles that are unavailable for a full reading. EC3 excludes articles not published in conferences, journals, or workshops. EC4 removes articles that are not in English. EC5 excludes works that are reviews or not articles. EC6 excludes studies that match the search string but do not investigate technologies for monitoring AD as the target objective. EC7 removes duplicated articles.
According to [44], there are different types of interaction focus that an application for AD can have: Health monitoring, Emergency help, Cognitive support, Therapy support, Training, ADL monitoring, Learning content and Mobility. The demand for applying EC8 and EC9 emerged in the last phase of this study based on interaction focus.
In addition, according to [44], Health monitoring interaction focus has two sub focuses which are tracking and study. Tracking uses data collected through monitoring to perform tasks with the analyzed data. In contrast, study only stores this data for further analysis. Filter EC9 was created based on EC8, with one more refinement, removing technologies aimed at monitoring that were not intended for tracking.
Initially, EC1, EC2, EC3, EC4, EC5, EC6, and EC7 were applied, resulting in 171 articles. The reading of the articles was performed based on the first two steps of [38]: 1) title reading, abstract, and introduction, followed by the titles of the sections and subsections, going through mathematical elements (if any) and conclusions; 2) analysis of figures, diagrams, and other illustrations. Secondly, the process search applied EC8 and EC9, resulting in 74 articles. Finally, the third step of [38] was applied, that is, the complete reading of the article, not generating exclusions, resulting in 74 articles.
Fig. 1.
Filtering process steps.
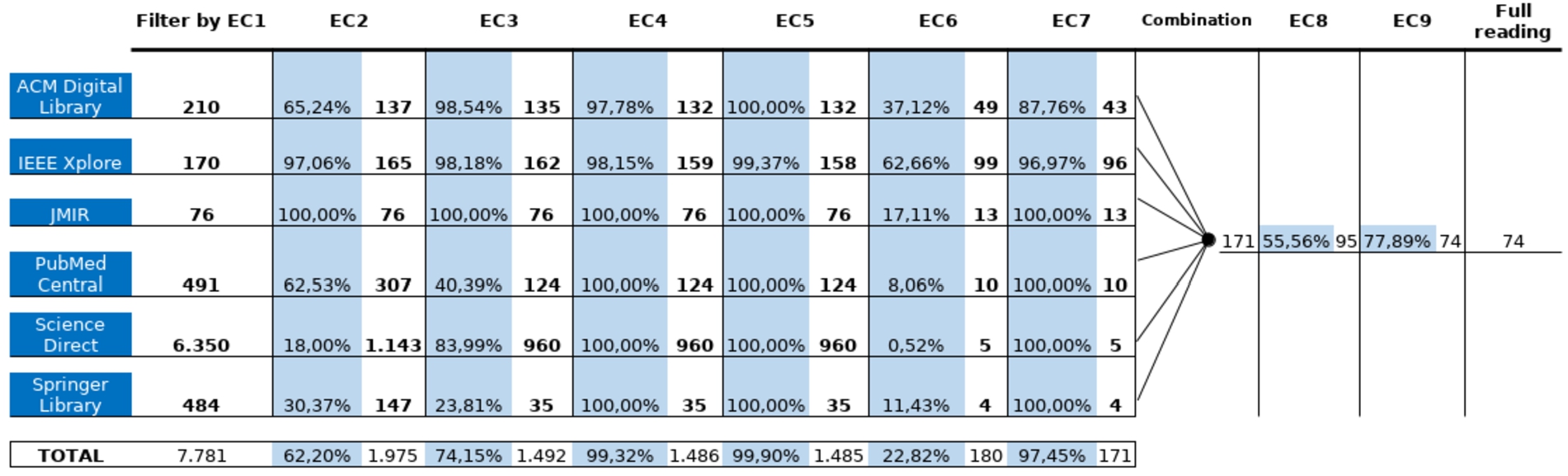
Table 5
SMS exclusion criteria
ID | Description |
EC1 | Publications not including solutions aimed to support AD patients |
EC2 | Full article unavailable |
EC3 | Articles not published in conference, journal, or workshop |
EC4 | Language other than English |
EC5 | Theses, dissertations, books, and reviews |
EC6 | Articles not related to the topic |
EC7 | Duplicate articles |
EC8 | Publications that do not mention the use of technologies for AD monitoring |
EC9 | Monitoring type is not tracking |
Fig. 2.
Taxonomy for technologies, tests, and approaches applied to Alzheimer’s patients. (Numbers represent ID field of Table 6.)
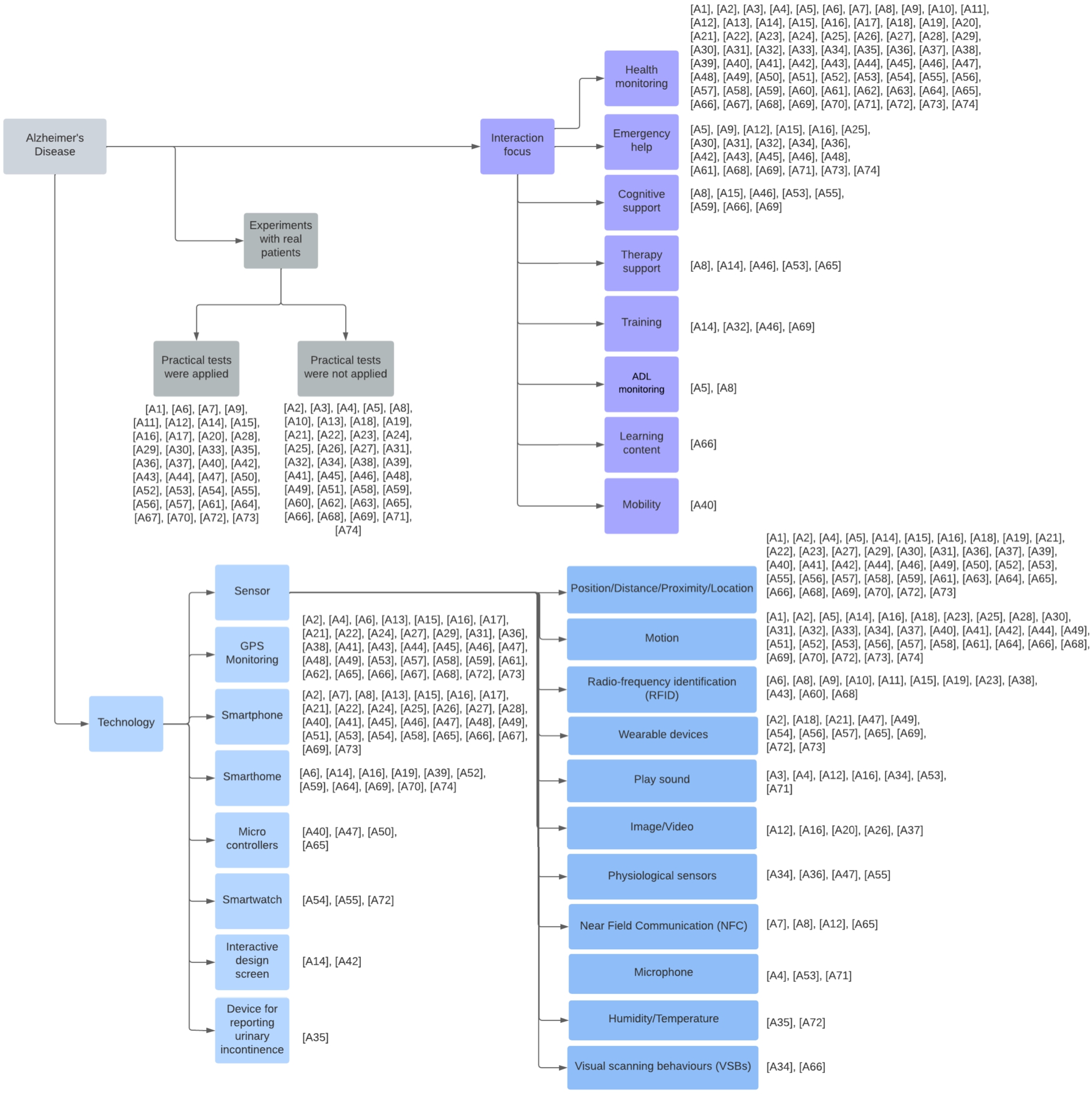
This mapping used the 74 selected articles to elaborate a taxonomy (Fig. 2) that organizes topics related to monitoring technologies for AD. The taxonomy organizes how the articles were filtered through three topics: Interaction Focus, Technology, and Experiments with real patients. The Interaction Focus topic shows the purpose of the interaction between the technological solution and the patient with AD. The Technology topic shows which type of technology is used. Finally, the topic Experiments with real patients shows whether the experiments to validate the use of technology were carried out with real patients with AD or using data created for testing. The topics were chosen according to the objective of this work to filter the types of technology interaction with patients, looking for articles that have as objective the interaction of the Health monitoring type. As articles can have more than one type of Interaction Focus and belong to more than one type of Technology, if necessary, works are cited in more than one type in the topics.
Table 6 shows the final articles selected after the execution of all steps of the search process, where the ID field is the identification of the article used in the figures with data analysis in Section 3.
3.Results
This section presents results about SMS obtained through analysis of General Question, Research Questions, Focal Question, and Specific Question.
3.1.GQ – What technologies are being used in the care of patients with Alzheimer’s disease?
SMS filters took into consideration only the physical resources to search for technologies, as they all had as a secondary resource software for device orchestration and data validation. The technologies filtered by reading the articles were: Sensor, GPS Monitoring, Smartphone, Smarthome, Micro controllers, Smartwatch, Interactive design screen, and Device for reporting urinary incontinence. Table 7 shows the technology categories of each article.
More than one type of technology category is used in the majority of articles, such as the application developed by [76], based on the patient’s behavior and movement patterns using sensors and smartwatch, and an application developed by [1] in which they use sensors and smart home technologies to monitoring the environment and the patient’s movements.
According to [43], the devices utilized in the experiments were used in the least invasive way possible, so patients would not feel uncomfortable or intimidated, as a way to ensure greater acceptance and use by them.
Table 6
List of selected papers (sorted by year)
ID | Reference | Database | Country | Technology | Focus |
[A1] | Van Someren, 1997 [80] | IEEE | Netherlands | Sensor | Health monitoring |
[A2] | Ogawa et al., 2004 [53] | IEEE | Japan, United States | GPS Monitoring, Sensor, Smartphone | Health monitoring |
[A3] | Nishida et al., 2004 [51] | IEEE | Japan | Sensor | Health monitoring |
[A4] | Faria et al., 2008 [22] | IEEE | Portugal | GPS Monitoring, Sensor, Microphone, Sound | Health monitoring |
[A5] | Zhang et al., 2008 [89] | IEEE | United Kingdom | Sensor | Health monitoring, Emergency help |
[A6] | Chang et al., 2008a [16] | IEEE | Taiwan | Smarthome, Sensor, GPS Monitoring | Health monitoring |
[A7] | Chang, 2008 [14] | IEEE | Taiwan | Sensor, Smartphone | Health monitoring |
[A8] | Bravo et al., 2008 [11] | ACM | Spain, Mexico | Sensor, Smartphone | Health monitoring, Cognitive, Therapy |
[A9] | Chang et al., 2008b [15] | ACM | Taiwan | Sensor | Health monitoring, Emergency help |
[A10] | Naeem and Bigham, 2009 [50] | IEEE | United Kingdom | Sensor | Health monitoring |
[A11] | Chang et al., 2009 [18] | IEEE | Taiwan | Sensor | Health monitoring |
[A12] | Chang et al., 2009a [17] | IEEE | Taiwan | Sensor | Health monitoring, Emergency help |
[A13] | Geddes and Warwick, 2010 [24] | IEEE | United Kingdom | GPS Monitoring, Smartphone | Health monitoring |
[A14] | Spanoudakis et al., 2010 [70] | IEEE | France, Austria, Greece | Smarthome, Sensor, Interactive design screen | Health monitoring, Therapy support, Training |
[A15] | Vuong et al., 2011 [81] | ACM | Japan, United Kingdom | Smartphone, Sensor, GPS Monitoring | Health monitoring, Emergency help, Cognitive |
[A16] | Lapointe et al., 2012 [41] | ACM | Canada | Smarthome, Sensor, GPS Monitoring, Smartphone | Health monitoring, Emergency help |
[A17] | Memon et al., 2012 [46] | ACM | Japan | GPS Monitoring, Smartphone | Health monitoring |
[A18] | Kim et al., 2012 [39] | IEEE | United States | Sensor | Health monitoring |
[A19] | Kanai et al., 2012 [36] | IEEE | Japan | Smarthome, Sensor | Health monitoring |
[A20] | Romdhane et al., 2012 [66] | PubMed | France, United Kingdom | Sensor | Health monitoring |
[A21] | Paiva et al., 2012 [56] | Science | Portugal | GPS Monitoring, Sensor, Smartphone | Health monitoring |
[A22] | Yuce et al., 2012 [87] | Science | Turkey | GPS Monitoring, Sensor, Smartphone | Health monitoring |
[A23] | Belley et al., 2013 [8] | ACM | Canada | Sensor | Health monitoring |
[A24] | Yuce et al., 2013 [86] | IEEE | Turkey | Smartphone, GPS Monitoring | Health monitoring |
[A25] | Vuong et al., 2013 [82] | IEEE | Japan | Smartphone, Sensor | Health monitoring, Emergency help |
[A26] | Nogueira et al., 2013 [52] | IEEE | Brazil | Sensor, Smartphone | Health monitoring |
[A27] | Solanas et al., 2013 [69] | IEEE | Spain | Smartphone, Sensor, GPS Monitoring | Health monitoring |
[A28] | Moore et al., 2013 [48] | IEEE | Japan, Spain, UK | Sensor, Smartphone | Health monitoring |
[A29] | Tung et al., 2013 [78] | PubMed | Canada | GPS Monitoring, Sensor | Health monitoring |
[A30] | Chan et al., 2014 [13] | ACM | France | Sensor | Health monitoring, Emergency help |
[A31] | Schwarzmeier et al., 2014 [68] | IEEE | Germany | GPS Monitoring, Sensor | Emergency help, Health monitoring |
[A32] | Belley et al., 2014 [9] | ACM | Canada | Sensor | Health monitoring, Training, Emergency help |
[A33] | Stucki et al., 2014 [72] | JMIR | Sweden | Sensor | Health monitoring |
[A34] | Maglogiannis et al., 2014 [45] | ACM | Greece | Sensor | Health monitoring, Emergency help |
[A35] | Gong et al., 2015 [27] | ACM | United States | Device for reporting urinary incontinence, Sensor | Health monitoring |
[A36] | Koldrack et al., 2015 [40] | ACM | Germany | GPS Monitoring, Sensor | Health monitoring, Emergency help |
[A37] | Crispim-Junior et al., 2015 [20] | ACM | China, France | Sensor | Health monitoring |
[A38] | Mhamdi et al., 2015 [47] | IEEE | France, Morocco | GPS Monitoring, Sensor | Health monitoring |
[A39] | Rosner et al., 2015 [67] | IEEE | Romania | Smarthome, Sensor | Health monitoring |
[A40] | Oh et al., 2015 [54] | ACM | Japan | Mini Arduino, Sensor, Smartphone | Health monitoring, Mobility |
[A41] | Koldrack et al., 2015 [40] | IEEE | Germany | Sensor, Smartphone, GPS Monitoring | Health monitoring |
[A42] | Radziszewski et al., 2016 [64] | ACM | Canada | Sensor, Interactive design screen | Health monitoring, Emergency help |
[A43] | Wang et al., 2016 [83] | IEEE | Taiwan | GPS Monitoring, Sensor | Health monitoring, Emergency help |
[A44] | Ando et al., 2016 [6] | IEEE | Italy | GPS Monitoring, Sensor | Health monitoring |
[A45] | Helmy et al., 2016 [30] | IEEE | United States | Smartphone, GPS Monitoring | Health monitoring, Emergency help |
[A46] | Pirani et al., 2016 [59] | Science | India | Sensor, GPS Monitoring, Smartphone | Health monitoring, Therapy support, Training, Cognitive support, Emergency help |
[A47] | Tabakis et al., 2017 [74] | IEEE | Greece | GPS Monitoring, Sensor, Smartphone, Mini Arduino | Health monitoring |
[A48] | Helmy et al., 2017 [31] | IEEE | United States | Smartphone, GPS Monitoring | Health monitoring, Emergency help |
[A49] | Paul-Adrian et al., 2017 [57] | IEEE | Romania, Portugal | Sensor, GPS Monitoring, Smartphone | Health monitoring |
[A50] | Pratiarso et al., 2017 [62] | IEEE | Indonesia | Sensor, Mini Arduino | Health monitoring |
[A51] | Kammuller et al., 2017 [35] | IEEE | London | Smartphone, Sensor | Health monitoring |
[A52] | Muir-Hunter et al., 2017 [49] | PubMed | Canada | Smarthome, Sensor | Health monitoring |
[A53] | Kanno et al., 2018 [37] | IEEE | Brazil | Smartphone, GPS Monitoring, Microphone, Sensor | Health monitoring, Cognitive, Therapy |
[A54] | Amato et al., 2018 [5] | ACM | Italy | Smartphone, Smartwatch | Health monitoring |
[A55] | Aljehani et al., 2018 [2] | IEEE | Saudi Arabia | Smartwatch, Sensor | Health monitoring, Cognitive support |
[A56] | Pratiarso et al., 2018 [61] | IEEE | Indonesia | Sensor | Health monitoring |
[A57] | Gugliandolo et al., 2018 [29] | IEEE | Italy | GPS Monitoring, Sensor | Health monitoring |
[A58] | Rodrigues et al., 2018 [65] | IEEE | Portugal | Sensor, Smartphone, GPS Monitoring | Health monitoring |
[A59] | Zhang et al., 2018 [88] | IEEE | United States | Smarthome, Sensor, GPS Monitoring | Health monitoring, Cognitive support |
[A60] | Griggs et al., 2018 [28] | IEEE | Canada, Ireland | Sensor | Health monitoring |
[A61] | Jara et al., 2019 [33] | ACM | Ecuador | GPS Monitoring, Sensor | Emergency help, Health monitoring |
[A62] | Benitez et al., 2019 [10] | IEEE | Mexico | GPS Monitoring | Health monitoring |
[A63] | Garcia-Magarino et al., 2019 [23] | IEEE | Spain | Sensor | Health monitoring |
[A64] | Chavez et al., 2019 [19] | PubMed | Mexico | Smarthome, Sensor | Health monitoring |
[A65] | Surendran et al., 2019 [73] | Science | India | Sensor, Mini Arduino, GPS Monitoring, Smartphone | Therapy support, Health monitoring |
[A66] | Kalpana et al., 2020 [34] | IEEE | India | Sensor, GPS Monitoring, Smartphone | Health monitoring, Emergency help, Learning content |
[A67] | Pratama et al., 2020 [60] | IEEE | Indonesia | GPS Monitoring, Smartphone | Health monitoring |
[A68] | Baugbog et al., 2020 [7] | ACM | Philippines | Sensor,GPS Monitoring | Health monitoring, Emergency help |
[A69] | Tan et al., 2020 [75] | IEEE | United States | Smarthome, Sensor, Smartphone | Health monitoring, Emergency help, Cognitive support, Training |
[A70] | Lussier et al., 2020 [43] | JMIR | Canada | Smarthome, Sensor | Health monitoring |
[A71] | Li et al., 2020 [42] | JMIR | United States | Microphone, Sensor | Health monitoring, Emergency help |
[A72] | Thomas et al., 2020 [76] | JMIR | Canada, United States | GPS Monitoring, Sensor, Smartwatch | Health monitoring |
[A73] | Gimenez et al., 2020 [25] | Springer | United Kingdom | Smartphone, GPS Monitoring, Sensor | Health monitoring, Emergency help |
[A74] | Aissani et al., 2021 [1] | IEEE | Algeria | Smarthome, Sensor | Health monitoring, Emergency help |
A relevant number of articles use solutions with Sensors, that is 43.42%, while 23.03% use GPS monitoring, and 33.55% others (Smartphone, Smart home, Micro controllers, Smartwatch, Interactive design screen, Device for reporting urinary incontinence).
Table 8 shows that most sensors belong to the category of the Position/Distance/ Proximity/Location (33.33%), followed by the detection categories Movement/ Presence/Agitation (27,13%), Radio-frequency identification (RFID) (9.30%) and with the same percentage Wearable devices (9.30%).
The second category of technology most used in the works is GPS monitoring (23.03%) to assist caregivers in the monitoring of patients. The objective of the authors in the development of this type of resource was to track the patient to help the caregivers and parents in daily care.
Table 7
Categories of technologies used in the articles
Technology | Percentage | Papers |
Sensor | 43.42% | [A1], [A2], [A3], [A4], [A5], [A6], [A7], [A8], [A9], [A10], [A11], [A12], [A14], [A15], [A16], [A18], [A19], [A20], [A21], [A22], [A23], [A25], [A26], [A27], [A28], [A29], [A30], [A31], [A32], [A33], [A34], [A35], [A36], [A37], [A38], [A39], [A40], [A41], [A42], [A43], [A44], [A46], [A47], [A49], [A50], [A51], [A52], [A53], [A55], [A56], [A57], [A58], [A59], [A60], [A61], [A63], [A64], [A65], [A66], [A68], [A69], [A70], [A71], [A72], [A73], [A74] |
GPS Monitoring | 23.03% | [A2], [A4], [A6], [A13], [A15], [A16], [A17], [A21], [A22], [A24], [A27], [A29], [A31], [A36], [A38], [A41], [A43], [A44], [A45], [A46], [A47], [A48], [A49], [A53], [A57], [A58], [A59], [A61], [A62], [A65], [A66], [A67], [A68], [A72], [A73] |
Smartphone | 19.74% | [A2], [A7], [A8], [A13], [A15], [A16], [A17], [A21], [A22], [A24], [A25], [A26], [A27], [A28], [A40], [A41], [A45], [A46], [A47], [A48], [A49], [A51], [A53], [A54], [A58], [A65], [A66], [A67], [A69], [A73] |
Smarthome | 7.24% | [A6], [A14], [A16], [A19], [A39], [A52], [A59], [A64], [A69], [A70], [A74] |
Micro controllers | 2.63% | [A40], [A47], [A50], [A65] |
Smartwatch | 1.97% | [A54], [A55], [A72] |
Interactive design screen | 1.32% | [A14], [A52] |
Device for reporting urinary incontinence | 0.66% | [A35] |
Table 8
Categories of sensors used
Type of sensor | Percentage | Papers |
Position/Distance/ Proximity/Location | 33.33% | [A1], [A2], [A4], [A5], [A14], [A15], [A16], [A18], [A19], [A21], [A22], [A23], [A27], [A29], [A30], [A31], [A36], [A37], [A39], [A40], [A41], [A42], [A44], [A46], [A49], [A50], [A52], [A53], [A55], [A56], [A57], [A58], [A59], [A61], [A63], [A64], [A65], [A66], [A68], [A69], [A70], [A72], [A73] |
Movement/Presence/ Agitation | 27.13% | [A1], [A2], [A5], [A14], [A16], [A18], [A23], [A25], [A28], [A30], [A31], [A32], [A33], [A34], [A37], [A40], [A41], [A42], [A44], [A49], [A51], [A52], [A53], [A56], [A57], [A58], [A61], [A64], [A66], [A68], [A69], [A70], [A72], [A73], [A74] |
Radio-frequency identification (RFID) | 9.30% | [A6], [A8], [A9], [A10], [A11], [A15], [A19], [A23], [A38], [A43], [A60], [A68] |
Wearable devices | 9.30% | [A2], [A18], [A21], [A47], [A49], [A54], [A56], [A57], [A65], [A69], [A72], [A73] |
Sound | 5.43% | [A3], [A4], [A12], [A16], [A34], [A53], [A71] |
Image/Video | 3.88% | [A12], [A16], [A20], [A26], [A37] |
Vital Signs/Skin Conductance Electrodermal Activity | 3.10% | [A34], [A36], [A47], [A55] |
Near Field Communication (NFC) | 3.10% | [A7], [A8], [A12], [A65] |
Microphone | 2.33% | [A4], [A53], [A71] |
Humidity / Temperature | 1.55% | [A35], [A72] |
Visual scanning behaviours (VSBs) | 1.55% | [A34], [A66] |
3.2.RQ1 – What are the usage models between patients and technology?
The interaction between patient and technology can be classified according to the application focus: Health monitoring, Emergency help, Cognitive support, Therapy support, Training, ADL monitoring, Learning content, and Mobility. Health monitoring focuses on the monitoring of vital signs. Emergency help emphasizes warning caregivers of imminent danger. Cognitive support focuses on technology for patients to remember old memories, such as photos, videos, and music. Therapy support emphasizes the technology of exercise therapy to decrease memory loss. Training focuses on teaching how to do everyday tasks, as patients forget to do specific daily actions, i.e., tying shoelaces. ADL monitoring emphasizes the tracking of the patient’s activities. Learning content focuses on teaching new content to the patient. Mobility focuses on instigating physical movement.
Patients and their caregivers interact with the application. In this way, caregivers are considered family members or an employee of the nursing home the patient attends. Table 9 shows the results obtained, where Health monitoring is the main focus of the articles (63.79%), the secondary focus among the works is Emergency help (18.97%), and the third one is Cognitive support (6.03%) contributing to help with the main difficulties according to how the disease progresses over time, which is loss of memory.
Table 9
Evaluation of the usage models between patient and technology
Usage models | Percentage | Papers |
Health monitoring | 63.79% | [A1], [A2], [A3], [A4], [A5], [A6], [A7], [A8], [A9], [A10], [A11], [A12], [A13], [A14], [A15], [A16], [A17], [A18], [A19], [A20], [A21], [A22], [A23], [A24], [A25], [A26], [A27], [A28], [A29], [A30], [A31], [A32], [A33], [A34], [A35], [A36], [A37], [A38], [A39], [A40], [A41], [A42], [A43], [A44], [A45], [A46], [A47], [A48], [A49], [A50], [A51], [A52], [A53], [A54], [A55], [A56], [A57], [A58], [A59], [A60], [A61], [A62], [A63], [A64], [A65], [A66], [A67], [A68], [A69], [A70], [A71], [A72], [A73], [A74] |
Emergency help | 18.97% | [A5], [A9], [A12], [A15], [A16], [A25], [A30], [A31], [A32], [A34], [A36], [A42], [A43], [A45], [A46], [A48], [A61], [A68], [A69], [A71], [A73], [A74] |
Cognitive support | 6.03% | [A8], [A15], [A46], [A53], [A55], [A59], [A66], [A69] |
Therapy support | 4.31% | [A8], [A14], [A46], [A53], [A65] |
Training | 3.45% | [A14], [A32], [A46], [A69] |
ADL monitoring | 1.72% | [A5], [A8] |
Learning content | 0.86% | [A66] |
Mobility | 0.86% | [A40] |
In 6.96% of the selected papers, the focus of the devices is Cognitive support [17,22,37,37,41,42,45,51]. Therapy support is considered in 4.35% of the articles [2,40,45,45,74].
3.3.RQ2 – Has the application been tested through tests with patients?
In 51.35% of the articles, practical tests were not applied with patients, and 48.65% of the articles presented the prototype development and tests with fictitious data or data obtained from other scientific research. Table 10 shows types of article tests. Practical tests are considered essential, that is, without proper observation of sampling or statistical variables. However, performing a controlled and random test is one of the future works listed in several articles.
Table 10
Classification of the articles about experiments with real patients
Type of article test | Percentage | Papers |
Practical tests were applied | 48.65% | [A1], [A6], [A7], [A9], [A11], [A12], [A14], [A15], [A16], [A17], [A20], [A28], [A29], [A30], [A33], [A35], [A36], [A37], [A40], [A42], [A43], [A44], [A47], [A50], [A52], [A53], [A54], [A55], [A56], [A57], [A61], [A64], [A67], [A70], [A72], [A73] |
Practical tests were not applied | 51.35% | [A2], [A3], [A4], [A5], [A8], [A10], [A13], [A18], [A19], [A21], [A22], [A23], [A24], [A25], [A26], [A27], [A31], [A32], [A34], [A38], [A39], [A41], [A45], [A46], [A48], [A49], [A51], [A58], [A59], [A60], [A62], [A63], [A65], [A66], [A68], [A69], [A71], [A74] |
In turn, 74.32% of the articles realized tests had the support of health professionals during the execution of the experiments. The works in which tests were carried out with patients were mostly attended in nursing homes or care homes for people with disabilities.
3.4.SQ – What is the distribution of articles by country?
To identify the countries where the works were developed, all authors’ institutions of origin were considered in the classification. Table 11 shows the countries with the greatest contribution to the theme: the United States with 10 publications (11.63%), Canada with 9 publications (10.47%), and both Japan (8.14%) and United Kingdom (8.14%) with 7 publications. Of the 27 countries involved in the subject, 10 are on the list of the 20 countries with the highest Human Development Index (HDI) [63]. Therefore, the countries with the greatest scientific contribution to the subject are those with the greatest chances of prevention and better care for the disease [85].
Table 11
Participation of countries in publications
Country | Percentage | Papers |
United States | 11.90% | [A2], [A18], [A35], [A40], [A45], [A48], [A59], [A69], [A71], [A72] |
Canada | 10.47% | [A16], [A23], [A29], [A32], [A42], [A52], [A60], [A70], [A72] |
Japan | 8.14% | [A2], [A3], [A15], [A17], [A19], [A25], [A28] |
United Kingdom | 8.14% | [A5], [A10], [A13], [A15], [A20], [A28], [A73] |
Taiwan | 6.98% | [A6], [A7], [A9], [A11], [A12], [A43] |
France | 5.81% | [A14], [A20], [A30], [A37], [A38] |
Portugal | 4.65% | [A4], [A21], [A49], [A58] |
Spain | 4.65% | [A8], [A27], [A28], [A63] |
Germany | 3.49% | [A31], [A36], [A41] |
Greece | 3.49% | [A14], [A34], [A47] |
India | 3.49% | [A46], [A65], [A66] |
Indonesia | 3.49% | [A50], [A56], [A67] |
Italy | 3.49% | [A44], [A54], [A57] |
Mexico | 3.49% | [A8], [A62], [A64] |
Brazil | 2.33% | [A26], [A53] |
Romania | 2.33% | [A39], [A49] |
Turkey | 2.33% | [A22], [A24] |
Sweden | 1.16% | [A33] |
Saudi Arabia | 1.16% | [A55] |
Philippines | 1.16% | [A68] |
Netherlands | 1.16% | [A1] |
London | 1.16% | [A51] |
Ecuador | 1.16% | [A61] |
Algeria | 1.16% | [A74] |
Fig. 3.
Annual papers’ distribution according to the database.
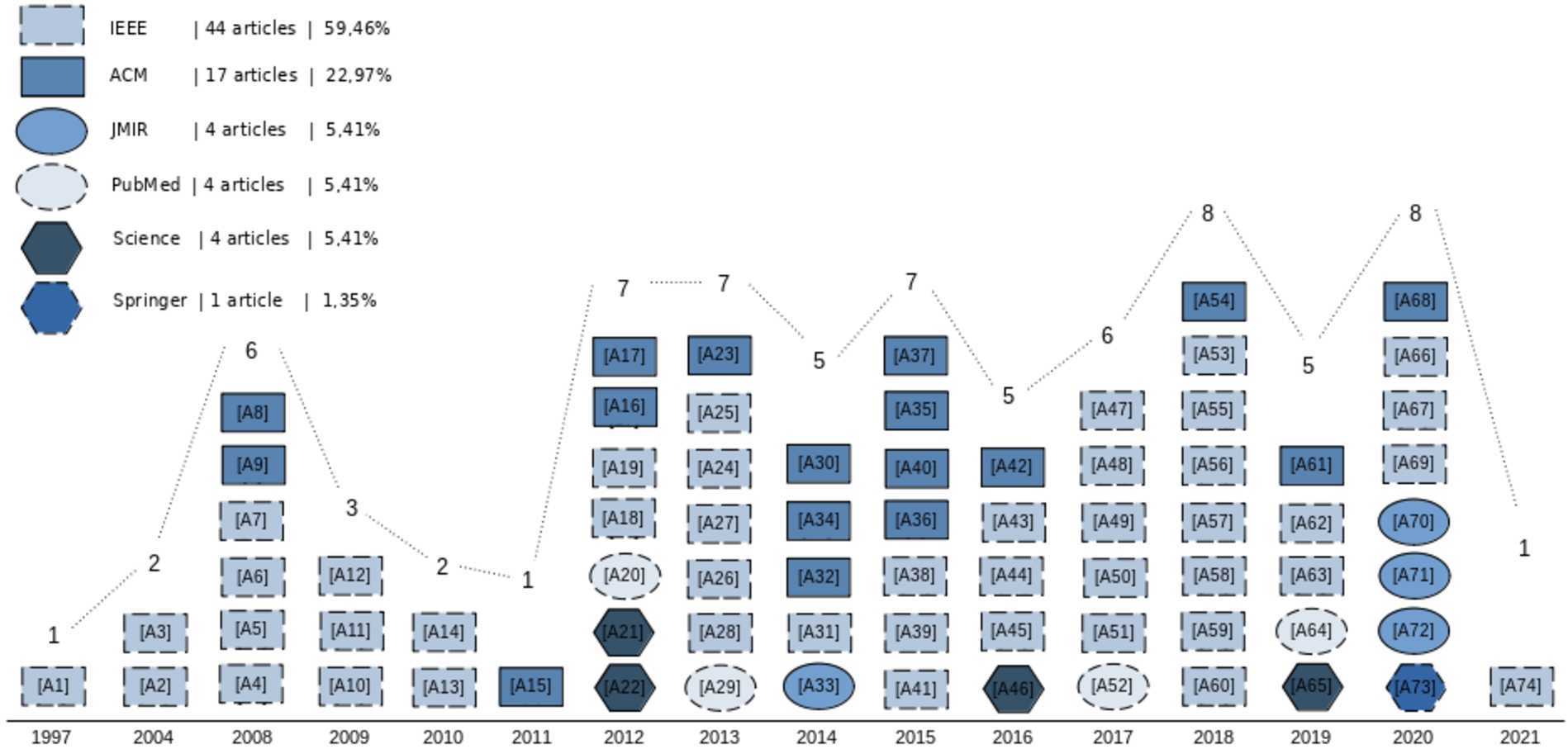
Fig. 4.
Cluster density map.
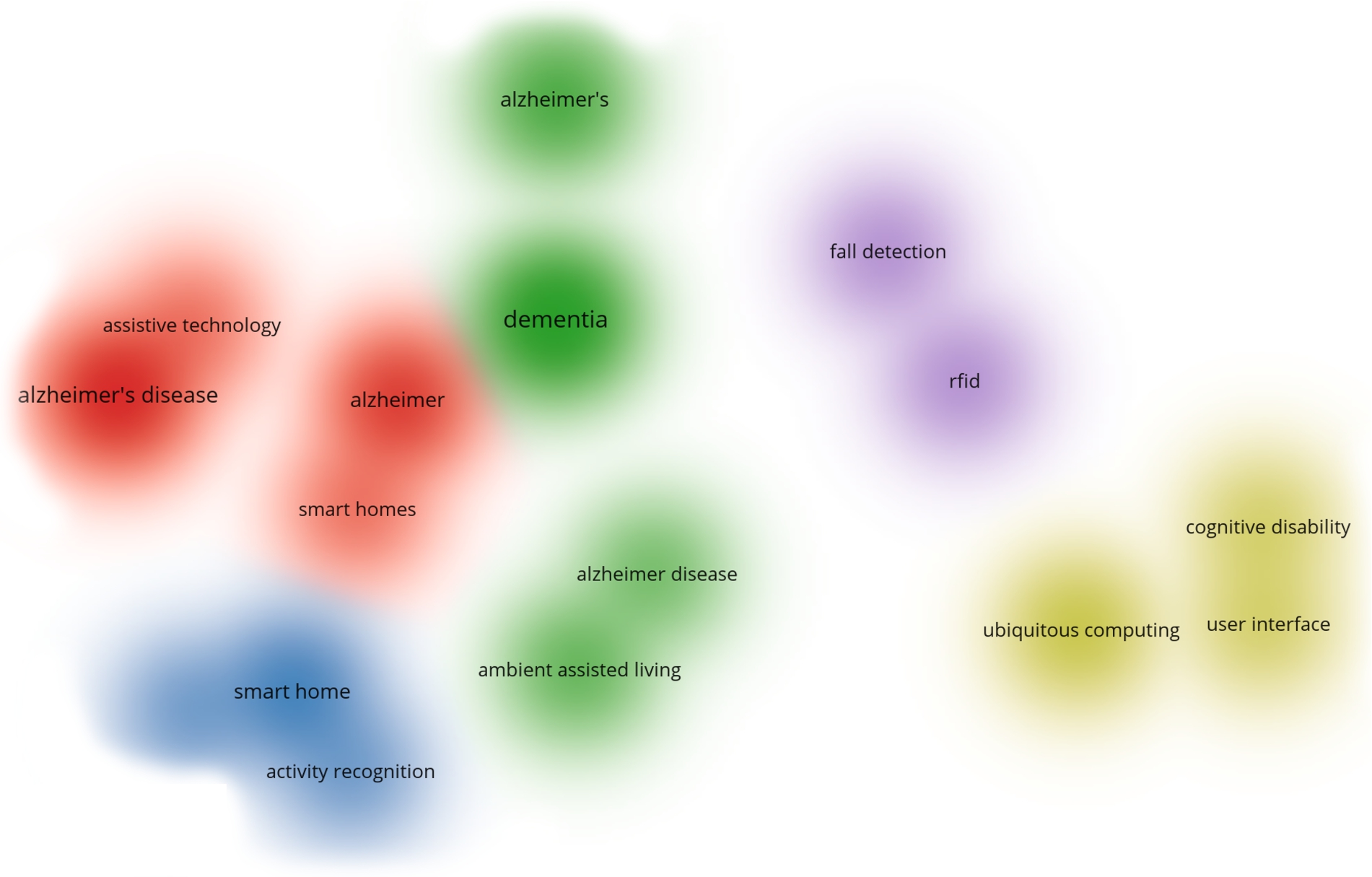
3.5.FQ – What are the trends perceived?
Figure 3 presents the frequency of publications on the subject, which shows that the research theme began to be explored in 1997, ending the last article in 2021. The majority of the selected papers (59.46% 44/74) were provided by the IEEE Xplore Digital Library, validating the efficiency of this database in the subject. Springer Library had just one article identified in the search process. Literary bases in the field of computing returned more relevant results than reference bases in the healthcare field.
Table 12
Description of identified clusters
ID | Description |
Red | The utilization of smart home devices with assisting technologies |
Blue | The utilization of smart home devices for activity recognition |
Green | Ambient assisted living for the care of patients with AD |
Purple | RFID devices used in solutions for patients’ fall detection |
Yellow | Solutions utilizes a user interface to interact with patients |
The frequency of “terms” was collected with the VOSViewer tool, containing the most found terms among the articles. Figure 4 represents the density of terms and the generation of clusters of interest, grouped by colors according to the proximity of the terms. The identified clusters are presented and characterized in Table 12. Five different clusters were identified. Red cluster represents the utilization of smart home devices with assisting technologies (4 terms). Blue cluster shows the utilization of smart home devices for activity recognition (2 terms). Cluster green represents ambient assisted living for the care of patients with AD (3 terms). Purple cluster represents RFID devices used in solutions for patients’ fall detection (2 terms). Yellow cluster shows solutions that utilize a user interface to interact with patients (2 terms). The more terms the cluster has, the more diverse and comprehensive the topic is among the articles.
Figure 5 presents an overview of the characteristics between terms of the same cluster or different clusters. The pairs and strength of connection in these networks are determined by factors such as the occurrence of the terms in all imported documents and the number of documents with the same data source or authorship [79]. This model represents an overlap of the chronological incidence of terms on the map of clusters. The characteristics of the research terms of this study are grouped in a cluster to the left of the image, represented by a green and yellow color, illustrating a recent scenario referring to the advance of studies in the area.
The tool detected that the most recent technologies being used for the solutions in this area are smart homes in ambient assisted living, represented by light green color, standing out in articles developed between 2018 and 2020. In contrast, technologies developed with user interfaces were used in articles belong 2010 and 2012, represented by the purple color. The trend is that technologies such as smart homes in ambient assisted living are increasingly being developed in new articles.
From 2012 to 2020 was the period with more publications of works on the subject of monitoring technologies for AD. However, there is a decrease from 2021 in the development of papers on the subject, resulting in one article demonstrating the trend of exchanging topics related to monitoring/tracking technologies for the use of technologies such as smart homes.
Fig. 5.
Overview of the relationship between terms.
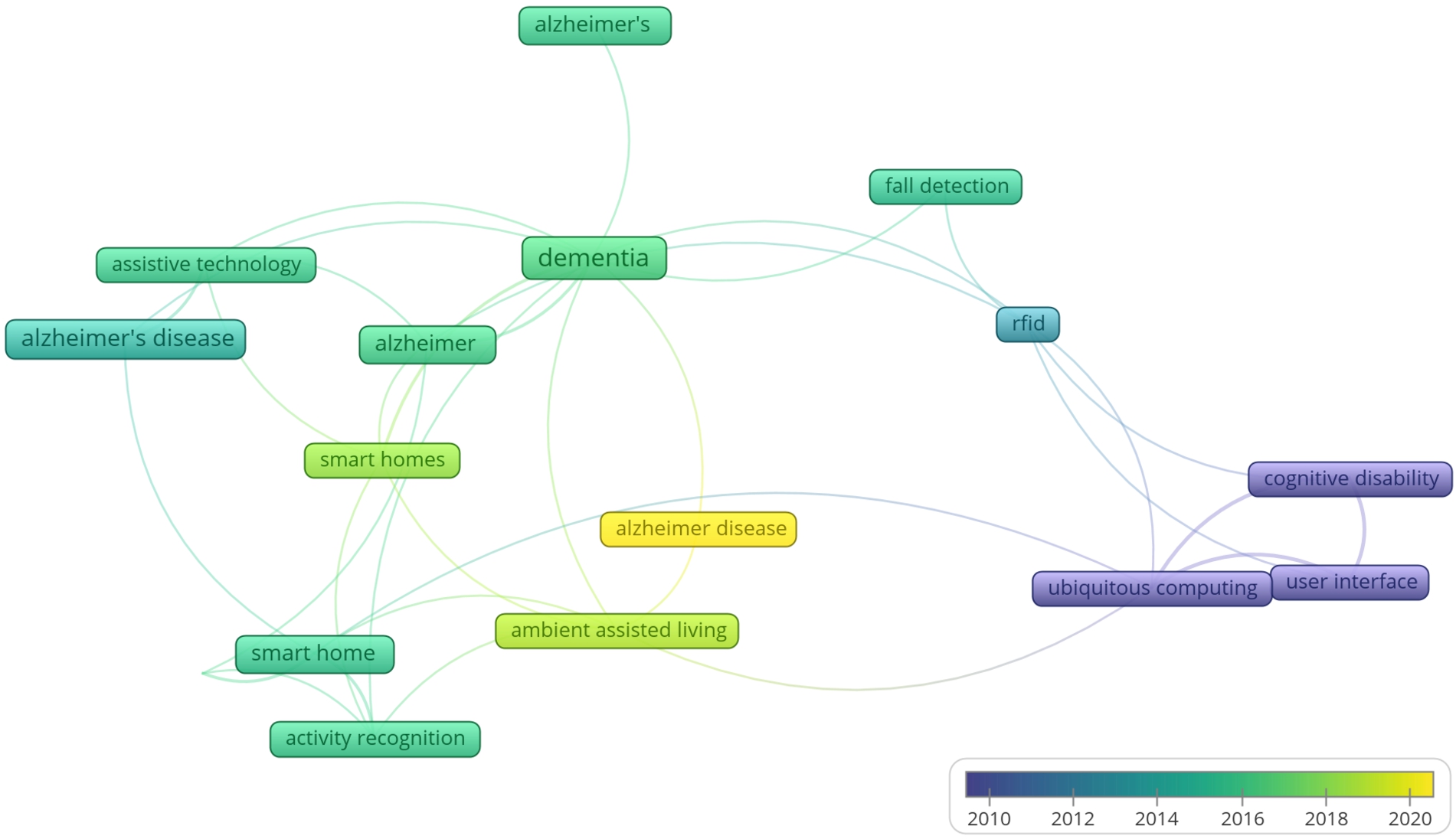
4.Final considerations
This work identified the current scenario in research regarding the use of monitoring technologies for AD. The SMS demonstrated and analyzed the articles selected in this study. The paper uses the term technologies as hardware infrastructure and devices or systems without considering software technologies.
The research identified Sensors as the most used technology category for project development, being related to 43.42% of the articles, followed by the use of GPS monitoring (23.03%), and others (33.55%).
The main focus of interaction between patients and technologies was Health monitoring (63.79%), the secondary focus among the works is Emergency help (18.97%), and the third one is Cognitive support (6.03%) contributing to help with the main difficulties according to how the disease progresses over time, which is loss of memory.
In those countries that promote studies in the area, they have greater chances of prevention and better care for the disease than in other countries. Simultaneously, it is necessary to encourage the adoption of more reliable applicability tests with more comprehensive and secure validations.
The results obtained through the VOSviewer tool corroborate the perception of current research and trends, in which the propensity is the more significant development of smart home devices.
Finally, despite attempts to mitigate risks, certain choices may have affected the outcome of this systematic mapping. The selection of databases is a risk factor. To seek better results, six databases were selected. However, the results presented that specific databases were ineffective, while the IEEE Xplore Digital Library was predominant. The search process, and exclusion criteria, in addition to the author’s assessment of relevance, also delimited the results, possibly excluding relevant articles. Therefore, we sought to minimize these risks following the methodologies of [38] and [58].
As a future work, it is intended to expand the study to compare the characteristics of the applications, mainly highlighting which patients’ needs have not yet been covered by current devices.
Notes
Acknowledgements
The authors would like to thank the Foundation for Research Support of the State of Rio Grande do Sul (FAPERGS), the Coordination for Improvement of Higher Education Personnel (CAPES) – Financing Code 001, the National Council for Scientific and Technological Development (CNPq), and the University of Vale do Rio dos Sinos (Unisinos), Brazil, for the support of the development of this work. The authors especially acknowledge the support of the Applied Computing Graduate Program (PPGCA), and the Mobile Computing Laboratory (Mobilab) of Unisinos.
Conflict of interest
None to report.
References
[1] | C. Aissani, Y.F. Akroun, M. Yazid and S. Bouchelaghem, Smart home danger prediction system to ensure people with Alzheimer’s disease safety, in: 2020 2nd International Workshop on Human-Centric Smart Environments for Health and Well-Being, IHSH 2020, (2021) , pp. 86–91. ISBN 9781665440844. doi:10.1109/IHSH51661.2021.9378728. |
[2] | S.S. Aljehani, R.A. Alhazmi, S.S. Aloufi, B.D. Aljehani and R. Abdulrahman, in: ICare: Applying IoT Technology for Monitoring Alzheimer’s Patients, 1st International Conference on Computer Applications and Information Security, ICCAIS 2018, (2018) , pp. 1–6. ISBN 9781538644263. doi:10.1109/CAIS.2018.8442010. |
[3] | A.A. ALZ, What is Alzheimer’s disease? (2021). Accessed: 2022-05-03. |
[4] | A.D.I. ALZINT, About dementia (2021). Accessed: 2022-05-04. |
[5] | F. Amato, S. Bianchi, S. Comai, P. Crovari, M.G.G. Pasquarelli, A. Imtiaz, A. Masciadri, M. Toldo and E. Yuyar, CLONE: A promising system for the remote monitoring of Alzheimer’s patients: An experimentation with a wearable device in a village for Alzheimer’s care, in: Proceedings of the 4th EAI International Conference on Smart Objects and Technologies for Social Good, Goodtechs ’18, Association for Computing Machinery, New York, NY, USA, (2018) , pp. 255–260. ISBN 9781450365819. doi:10.1145/3284869.3284906. |
[6] | B. Ando, S. Baglio, C.O. Lombardo and V. Marletta, A multisensor data-fusion approach for ADL and fall classification, IEEE Transactions on Instrumentation and Measurement 65: (9) ((2016) ), 1960–1967. doi:10.1109/TIM.2016.2552678. |
[7] | J.D.G. Baugbog, M.J.P. Manalo, J.M.M. Salonga, E.P. Naval and E.R. Zhuo, Wearable device equipped with door alert and mobile app for security and supervision, in: ACM International Conference Proceeding Series, (2020) , pp. 86–93. ISBN 9781450376853. doi:10.1145/3379310.3379314. |
[8] | C. Belley, S. Gaboury, B. Bouchard and A. Bouzouane, Activity recognition in smart homes based on electrical devices identification, in: ACM International Conference Proceeding Series, (2013) . ISBN 9781450319737. doi:10.1145/2504335.2504342. |
[9] | C. Belley, S. Gaboury, B. Bouchard and A. Bouzouane, A new system for assistance and guidance in smart homes based on electrical devices identification, in: ACM International Conference Proceeding Series, 2014-May, (2014) . ISBN 9781450327466. doi:10.1145/2674396.2674399. |
[10] | R.P. Benitez Cortes, R. Lopez Espinoza, P. Aguilar Navarrete, Y. Camacho Gonzalez, L.J. Benitez Ramirez and V.J. Torres Covarrubias, GPS devices to monitor cognitive impairment people: Technical implications of personal trackers for use in Mexico, in: Proceedings – 2019 International Conference on Inclusive Technologies and Education, CONTIE 2019, (2019) , pp. 40–44. ISBN 9781728154367. doi:10.1109/CONTIE49246.2019.00017. |
[11] | J. Bravo, R. Hervás, R. Gallego, G. Casero, M. Vergara, T. Carmona, C. Fuentes, S.W. Nava, G. Chavira and V. Villarreal, Enabling NFC technology to support activities in an Alzheimer’s day center, in: 1st International Conference on Pervasive Technologies Related to Assistive Environments, PETRA 2008, (2008) , pp. 1–5. ISBN 9781605580678. doi:10.1145/1389586.1389679. |
[12] | W. Burleson, C. Lozano, V. Ravishankar, J. Lee and D. Mahoney, An assistive technology system that provides personalized dressing support for people living with dementia: Capability study, Journal of Medical Internet Research 20: (5) ((2018) ), 1–20. doi:10.2196/medinform.5587. |
[13] | M. Chan, E. Campo, W. Bourennane, F. Bettahar and Y. Charlon, Mobility behavior assessment using a smart-monitoring system to care for the elderly in a hospital environment, in: ACM International Conference Proceeding Series 2014-May, (2014) , pp. 1–5. ISBN 9781450327466. doi:10.1145/2674396.2674397. |
[14] | W.C. Chang, CATPro: Context-aware task prompting system with multiple modalities for individuals with cognitive impairments, in: Proceedings of the 10th International ACM SIGACCESS Conference on Computers and Accessibility, Assets ’08, Association for Computing Machinery, New York, NY, USA, (2008) , pp. 301–302. ISBN 9781595939760. doi:10.1145/1414471.1414549. |
[15] | Y.-J. Chang, Y.-Y. Chu, C.-N. Chen and T.-Y. Wang, Mobile computing for indoor wayfinding based on bluetooth sensors for individuals with cognitive impairments, in: 2008 3rd International Symposium on Wireless Pervasive Computing, (2008) , pp. 623–627. doi:10.1109/ISWPC.2008.4556284. |
[16] | Y.J. Chang, C.N. Chen, L.D. Chou and T.Y. Wang, A novel indoor wayfinding system based on passive RFID for individuals with cognitive impairments, in: Proceedings of the 2nd International Conference on Pervasive Computing Technologies for Healthcare 2008, PervasiveHealth, (2008) , pp. 108–111. ISBN 9789639799158. doi:10.1109/PCTHEALTH.2008.4571043. |
[17] | Y.J. Chang, Y.-R. Chen, C.Y. Chang and T.Y. Wang, Video prompting and indoor wayfinding based on bluetooth beacons: A case study in supported employment for people with severe mental illness, in: Proceedings – 2009 WRI International Conference on Communications and Mobile Computing, CMC 2009, (2009) , pp. 137–141. ISBN 9780769535012. doi:10.1109/CMC.2009.134. |
[18] | Y.J. Chang, H.Y. Hsu, W.C. Chang, S.M. Peng, Y.R. Chen and T.Y. Wang, Context-aware task prompting: Sustaining of supported employment for people with severe mental illness, in: Proceedings – 2009 WRI International Conference on Communications and Mobile Computing, CMC 2009, (2009) , pp. 127–131. ISBN 9780769535012. doi:10.1109/CMC.2009.131. |
[19] | A. Chávez, G. Borrego, J.O. Gutierrez-Garcia and L.F. Rodríguez, Design and evaluation of a mobile application for monitoring patients with Alzheimer’s disease: A day center case study, International Journal of Medical Informatics 131: ((2019) ), 103972. doi:10.1016/j.ijmedinf.2019.103972. |
[20] | C.F. Crispim-Junior, Q. Ma, B. Fosty, R. Romdhane, F. Bremond and M. Thonnat, Combining multiple sensors for event detection of older people, in: Health Monitoring and Personalized Feedback Using Multimedia Data, (2015) , pp. 179–194. ISBN 9783319179636. doi:10.1007/978-3-319-17963-6_10. |
[21] | DHSCUK, G8 dementia summit: Global action against dementia – 11 December 2013 (2015). Accessed: 2022-05-03. |
[22] | S.M.M. Faria, T.R. Fernandes and F.S. Perdigoto, Mobile web server for elderly people monitoring, Proceedings of the International Symposium on Consumer Electronics, ISCE 1: (2) ((2008) ), 1–4. ISBN 9781424424221. doi:10.1109/ISCE.2008.4559490. |
[23] | I. Garcia-Magarino, M. Cardenas, J. Gomez-Sanz and J.L. Perez Diez, Framework-supported mechanism of testing algorithms for assessing memory and detecting disorientation from IoT sensors, in: IEEE 5th World Forum on Internet of Things, WF-IoT 2019 – Conference Proceedings, (2019) , pp. 899–904. ISBN 9781538649800. doi:10.1109/WF-IoT.2019.8767297. |
[24] | J. Geddes and K. Warwick, Cloud based global positioning system as a safety monitor for dementia patients, in: 2010 IEEE 9th International Conference on Cybernetic Intelligent Systems, CIS 2010, (2010) . ISBN 9781424490233. doi:10.1109/UKRICIS.2010.5898085. |
[25] | J.G. Giménez Manuel, J.C. Augusto and J. Stewart, AnAbEL: Towards empowering people living with dementia in ambient assisted living, in: Universal Access in the Information Society, (2020) . ISBN 1020902000. doi:10.1007/s10209-020-00760-5. |
[26] | S.R.W. Global status report World Health Organization, Global status report on the public health response to dementia (2021). Accessed: 2022-05-03. |
[27] | J. Gong, K.M. Rose, I.A. Emi, J.P. Specht, E. Hoque, D. Fan, S.R. Dandu, R.F. Dickerson, Y. Perkhounkova, J. Lach and J.A. Stankovic, Home wireless sensing system for monitoring nighttime agitation and incontinence in patients with Alzheimer’s disease, in: Proceedings – Wireless Health 2015, WH 2015, (2015) . ISBN 9781450338516. doi:10.1145/2811780.2822324. |
[28] | W.M. Griggs, R. Verago, J. Naoum-Sawaya, R.H. Ordonez-Hurtado, R. Gilmore and R.N. Shorten, Localizing missing entities using parked vehicles: An RFID-based system, IEEE Internet of Things Journal 5: (5) ((2018) ), 4018–4030. doi:10.1109/JIOT.2018.2864590. |
[29] | G. Gugliandolo, A. Sciva, G. Campobello, P.P. Capra, S. Marino, A. Bramanti, G. Di Lorenzo, P. Bramanti and N. Donato, A movement monitoring system for patients of neurodegenerative diseases, in: I2MTC 2018 – 2018 IEEE International Instrumentation and Measurement Technology Conference: Discovering New Horizons in Instrumentation and Measurement, Proceedings 2, (2018) , pp. 1–6. ISBN 9781538622223. doi:10.1109/I2MTC.2018.8409786. |
[30] | J. Helmy and A. Helmy, The Alzimio app for dementia, autism & Alzheimer’s: Using novel activity recognition algorithms and geofencing, in: 2016 IEEE International Conference on Smart Computing, SMARTCOMP 2016, (2016) . ISBN 9781509008988. doi:10.1109/SMARTCOMP.2016.7501720. |
[31] | J. Helmy and A. Helmy, Demo abstract: Alzimio: A mobile app with geofencing, activity-recognition and safety features for dementia patients, in: 2017 IEEE Conference on Computer Communications Workshops, INFOCOM WKSHPS 2017, (2017) , pp. 994–995. ISBN 9781538627846. doi:10.1109/INFCOMW.2017.8116527. |
[32] | M. Ienca, J. Fabrice, B. Elger, M. Caon, A. Scoccia Pappagallo, R.W. Kressig and T. Wangmo, Intelligent assistive technology for Alzheimer’s disease and other dementias: A systematic review, J Alzheimers Dis 56: (4) ((2017) ), 1301–1340. |
[33] | J.D. Jara, J. Lima, C. Valdez, M. Barbecho, J.P. Bermeo and D. Chacón, Design of a mobile panic button for older adults for monitoring through the ECU911 system, in: ACM International Conference Proceeding Series, (2019) . ISBN 9781450376259. doi:10.1145/3387168.3387195. |
[34] | S. Kalpana Devi, D. Amirthavarshini, R.S. Anbukani and S. Bhavatha Ranjanni, in: Personal Assistance for Alzheimer’s Patient, 4th International Conference on Computer, Communication and Signal Processing, ICCCSP 2020, (2020) , pp. 27–32. ISBN 9781728165097. doi:10.1109/ICCCSP49186.2020.9315250. |
[35] | F. Kammuller, J.C. Augusto and S. Jones, Security and privacy requirements engineering for human centric IoT systems using eFRIEND and Isabelle, in: Proceedings – 2017 15th IEEE/ACIS International Conference on Software Engineering Research, Management and Applications, SERA 2017, (2017) , pp. 401–406. ISBN 9781509057566. doi:10.1109/SERA.2017.7965758. |
[36] | H. Kanai, Introduction on a support system for awareness in an aware-environment enhanced group home, in: AwareRium, Proceedings – 2012 7th International Conference on Knowledge, Information and Creativity Support Systems, KICSS 2012, (2012) , pp. 165–168. ISBN 9780769548616. doi:10.1109/KICSS.2012.14. |
[37] | K.M. Kanno, E.A. Lamounier, A. Cardoso, E.J. Lopes and G.F. Mendes De Lima, Augmented reality system for aiding mild Alzheimer patients and caregivers, in: 25th IEEE Conference on Virtual Reality and 3D User Interfaces, VR 2018 – Proceedings, (2018) , pp. 593–594. ISBN 9781538633656. doi:10.1109/VR.2018.8446143. |
[38] | S. Keshav, How to read a paper, SIGCOMM Comput. Commun. Rev. 37: (3) ((2007) ), 83–84. doi:10.1145/1273445.1273458. |
[39] | D.J. Kim, M.H. Suk and B. Prabhakaran, Fault detection and isolation in motion monitoring system, in: Proceedings of the Annual International Conference of the IEEE Engineering in Medicine and Biology Society, EMBS, (2012) , pp. 5234–5237. ISBN 9781424441198. doi:10.1109/EMBC.2012.6347174. |
[40] | P. Koldrack, R. Henkel, F. Kruger, S. Teipel and T. Kirste, Supporting situation awareness of dementia patients in outdoor environments, in: Proceedings of the 2015 9th International Conference on Pervasive Computing Technologies for Healthcare, PervasiveHealth 2015, (2015) , pp. 245–248. ISBN 9781631900457. doi:10.4108/icst.pervasivehealth.2015.259288. |
[41] | J. Lapointe, B. Bouchard, J. Bouchard, A. Potvin and A. Bouzouane, Smart homes for people with Alzheimer’s disease: Adapting prompting strategies to the patient’s cognitive profile, in: Proceedings of the 5th International Conference on PErvasive Technologies Related to Assistive Environments, PETRA ’12, Association for Computing Machinery, New York, NY, USA, (2012) . ISBN 9781450313001. doi:10.1145/2413097.2413135. |
[42] | J. Li, B. Maharjan, B. Xie and C. Tao, A personalized voice-based diet assistant for caregivers of Alzheimer disease and related dementias: System development and validation, Journal of Medical Internet Research 22: (9) ((2020) ), 1–11. doi:10.2196/19897. |
[43] | M. Lussier, A. Aboujaoudé, M. Couture, M. Moreau, C. Laliberté, S. Giroux, H. Pigot, S. Gaboury, K. Bouchard, P. Belchior, C. Bottari, G. Paré, C. Consel and N. Bier, Using ambient assisted living to monitor older adults with Alzheimer disease: Single-case study to validate the monitoring report, JMIR Medical Informatics 8: (11) ((2020) ), 1–16. doi:10.2196/20215. |
[44] | S.D. Machado, J.E.d.R. Tavares, M.G. Martins, J.L.V. Barbosa, G.V. González and V.R.Q. Leithardt, Ambient intelligence based on IoT for assisting people with Alzheimer’s disease through context histories, Electronics 10: (11) ((2021) ). https://www.mdpi.com/2079-9292/10/11/1260. doi:10.3390/electronics10111260. |
[45] | I. Maglogiannis, Human centered computing for the development of assistive environments: The STHENOS project, in: ACM International Conference Proceeding Series, 2014-May, (2014) . ISBN 9781450327466. doi:10.1145/2674396.2674453. |
[46] | M.A. Memon, J. Tanaka and T. Kamba, Restrain from pervasive logging employing geo-temporal policies, in: APCHI’12 – Proceedings of the 2012 Asia Pacific Conference on Computer-Human Interaction, (2012) , pp. 201–208. ISBN 9781450314961. doi:10.1145/2350046.2350087. |
[47] | J. Mhamdi and S. El Abkari, Contriving an RFID system for Alzheimer patients tracking, in: Proceedings – 2015 3rd International Workshop on RFID and Adaptive Wireless Sensor Networks, RAWSN 2015 – In conjunction with the International Conference on NETworked sYStems, NETYS 2015, (2015) , pp. 23–28. ISBN 9781467380942. doi:10.1109/RAWSN.2015.7173273. |
[48] | P. Moore, F. Xhafa, L. Barolli and A. Thomas, Monitoring and detection of agitation in dementia: Towards real-time and big-data solutions, in: Proceedings – 2013 8th International Conference on P2P, Parallel, Grid, Cloud and Internet Computing, 3PGCIC 2013, (2013) , pp. 128–135. ISBN 9780769550947. doi:10.1109/3PGCIC.2013.26. |
[49] | S.W. Muir-Hunter and M. Montero-Odasso, The attentional demands of ambulating with an assistive device in older adults with Alzheimer’s disease, Gait and Posture 54: ((2017) ), 202–208. doi:10.1016/j.gaitpost.2017.03.011. |
[50] | U. Naeem and J. Bigham, Recognising activities of daily life through the usage of everyday objects around the home, in: 2009 3rd International Conference on Pervasive Computing Technologies for Healthcare – Pervasive Health 2009, PCTHealth 2009, (2009) . ISBN 9789639799424. doi:10.4108/ICST.PERVASIVEHEALTH2009.6059. |
[51] | Y. Nishida, S. Murakami, T. Hori and H. Mizoguchi, Minimally privacy-violative human location sensor by ultrasonic radar embedded on ceiling, in: SENSORS, 2004, Vol. 1: , IEEE, (2004) , pp. 433–436. doi:10.1109/ICSENS.2004.1426192. |
[52] | C. Nogueira, F.M. Bublitz, H.O. De Almeida, K.C. Gorgônio and A. Perkusich, Framework for developing applications for remote monitoring of people with dementia, in: International Conference on Wireless and Mobile Computing, Networking and Communications, (2013) , pp. 291–296. ISBN 9781479904280. doi:10.1109/WiMOB.2013.6673375. |
[53] | H. Ogawa, Y. Yonezawa, H. Maki and W.M. Caldwell, A mobile phone-based communication support system for elderly persons, in: Annual International Conference of the IEEE Engineering in Medicine and Biology – Proceedings, (2007) , pp. 3798–3801. ISBN 1424407885. doi:10.1109/IEMBS.2007.4353159. |
[54] | H. Oh and M.D. Gross, Awareable steps: Functional and fashionable shoes for patients with dementia, in: UbiComp and ISWC 2015 – Proceedings of the 2015 ACM International Joint Conference on Pervasive and Ubiquitous Computing and the Proceedings of the 2015 ACM International Symposium on Wearable Computers, (2015) , pp. 579–584. ISBN 9781450335751. doi:10.1145/2800835.2801666. |
[55] | P.A.H.O. PAHO, OMS revela principais causas de morte e incapacidade em todo o mundo entre 2000 e 2019 (2020). Accessed: 2022-05-03. |
[56] | S. Paiva and C. Abreu, Low cost GPS tracking for the elderly and Alzheimer patients, Procedia Technology 5: ((2012) ), 793–802. doi:10.1016/j.protcy.2012.09.088. |
[57] | G. Paul-Adrian, J. Sarraipa, F. Luis-Ferreira and R. Jardim-Goncalves, A GPS simulation framework on smartphones for elderly assistance applications, in: 2017 International Conference on Engineering, Technology and Innovation: Engineering, Technology and Innovation Management Beyond 2020: New Challenges, New Approaches, ICE/ITMC 2017 – Proceedings 2018-Janua, (2018) , pp. 1559–1563. ISBN 9781538607749. doi:10.1109/ICE.2017.8280068. |
[58] | K. Petersen, S. Vakkalanka and L. Kuzniarz, Guidelines for conducting systematic mapping studies in software engineering: An update, Information and Software Technology 64: ((2015) ), 1–18. doi:10.1016/j.infsof.2015.03.007. |
[59] | E.Z. Pirani, F. Bulakiwala, M. Kagalwala, M. Kalolwala and S. Raina, Android based assistive toolkit for Alzheimer, Procedia Computer Science 79: ((2016) ), 143–151. doi:10.1016/j.procs.2016.03.019. |
[60] | E.R. Pratama, F. Renaldi, F.R. Umbara and E.C. Djamal, Geofencing technology in monitoring of geriatric patients suffering from dementia and Alzheimer, in: 2020 3rd International Conference on Computer and Informatics Engineering, IC2IE 2020, (2020) , pp. 106–111. ISBN 9781728182476. doi:10.1109/IC2IE50715.2020.9274637. |
[61] | A. Pratiarso, A.H. Amirudin, M. Yuliana, P. Kristalina, I.G.P. Astawa and Arifin, Analysis of fingerprint-based indoor localization system on Alzheimer’s patient position tracking system, in: Proceedings – 2018 3rd International Conference on Information Technology, Information Systems and Electrical Engineering, ICITISEE 2018, (2018) , pp. 114–119. ISBN 9781538670828. doi:10.1109/ICITISEE.2018.8720972. |
[62] | A. Pratiarso, A.I. Imanuddin, M. Yuliana, P. Kristalina and I.G.P. Astawa, Implementation of Kalman filter method for tracking position of Alzheimer’s patients, in: 2017 International Conference on Smart Cities, Automation Intelligent Computing Systems (ICON-SONICS), (2017) , pp. 135–140. doi:10.1109/ICON-SONICS.2017.8267836. |
[63] | U.N.D. Programme, Human Development Indices and Indicators: 2018 Statistical Update (2018). Accessed: 2020-03-04. |
[64] | R. Radziszewski, V. Grégoire, H. Ngankam, D. Lorrain, H. Pigot and S. Giroux, An ambient assisted living nighttime wandering system for elderly, in: ACM International Conference Proceeding Series, (2016) , pp. 368–374. ISBN 9781450348072. doi:10.1145/3011141.3011171. |
[65] | D. Rodrigues, F. Luis-Ferreira, J. Sarraipa and R. Goncalves, Behavioural monitoring of Alzheimer patients with smartwatch based system, in: 2018 International Conference on Intelligent Systems (IS), (2018) , pp. 771–775. doi:10.1109/IS.2018.8710487. |
[66] | R. Romdhane, E. Mulin, A. Derreumeaux, N. Zouba, J. Piano, L. Lee, I. Leroi, P. Mallea, R. David, M. Thonnat, F. Bremond and P.H. Robert, Automatic video monitoring system for assessment of Alzheimer’s disease symptoms, Journal of Nutrition, Health and Aging 16: (3) ((2012) ), 213–218. doi:10.1007/s12603-012-0039-7. |
[67] | D. Rosner, D. Vasile, A. Aungurencei, S. Vasile and V. Tanasiev, DyGo – Household object interaction tracking for smart home environments, in: Proceedings – 2015 20th International Conference on Control Systems and Computer Science, CSCS 2015, (2015) , pp. 248–251. ISBN 9781479917792. doi:10.1109/CSCS.2015.138. |
[68] | A. Schwarzmeier, J. Besser, R. Weigel, G. Fischer and D. Kissinger, A compact back-plaster sensor node for dementia and Alzheimer patient care, in: 2014 IEEE Sensors Applications Symposium, SAS 2014 – Proceedings, (2014) , pp. 75–78. ISBN 9781479921799. doi:10.1109/SAS.2014.6798920. |
[69] | A. Solanas, A. Martinez-Balleste, P.A. Perez-Martinez, A.F.D.L. Pena and J. Ramos, m-Carer: Privacy-aware monitoring for people with mild cognitive impairment and dementia, IEEE Journal on Selected Areas in Communications 31: (9) ((2013) ), 19–27. doi:10.1109/JSAC.2013.SUP.0513002. |
[70] | N. Spanoudakis, B. Grabner, C. Kotsiopoulou, O. Lymperopoulou, V. Moser-Siegmeth, S. Pantelopoulos, P. Sakka and P. Moraitis, A novel architecture and process for Ambient Assisted Living – The HERA approach, in: Proceedings of the IEEE/EMBS Region 8 International Conference on Information Technology Applications in Biomedicine, ITAB, (2010) . ISBN 9781424465606. doi:10.1109/ITAB.2010.5687615. |
[71] | V. Sriram, C. Jenkinson and M. Peters, Informal carers’ experience and outcomes of assistive technology use in dementia care in the community: A systematic review protocol, Systematic Reviews 8: (1) ((2019) ), 158. doi:10.1186/s13643-019-1081-x. |
[72] | R.A. Stucki, P. Urwyler, L. Rampa, R. Müri, U.P. Mosimann and T. Nef, A web-based non-intrusive ambient system to measure and classify activities of daily living, Journal of Medical Internet Research 16: (7) ((2014) ). doi:10.2196/jmir.3465. |
[73] | D. Surendran and M. Rohinia, BLE bluetooth beacon based solution to monitor egress of Alzheimer’s disease sufferers from indoors, Procedia Computer Science 165: ((2019) ), 591–597. ISBN 9190807170. doi:10.1016/j.procs.2020.01.053. |
[74] | I.-M. Tabakis, M. Dasygenis and M. Tsolaki, A monitoring system for people living with Alzheimer’s disease, in: 2017 Panhellenic Conference on Electronics and Telecommunications (PACET), (2017) , pp. 1–4. doi:10.1109/PACET.2017.8259985. |
[75] | K. Tan, K. Sekhar, J. Wong, J. Holgado, M. Ameer and G. Vesonder, Alexa eldercare toolbox: A smarthome solution for the elderly, in: 2020 11th IEEE Annual Ubiquitous Computing, Electronics and Mobile Communication Conference, UEMCON 2020, (2020) , pp. 0806–0812. ISBN 9781728196565. doi:10.1109/UEMCON51285.2020.9298127. |
[76] | N.W.D. Thomas, Z. Beattie, J. Marcoe, K. Wright, N. Sharma, N. Mattek, H. Dodge, K. Wild and J. Kaye, An ecologically valid, longitudinal, and unbiased assessment of treatment efficacy in Alzheimer disease (the evaluate-ad trial): Proof-of-concept study, JMIR Research Protocols 9: (5) ((2020) ), 1–16. doi:10.2196/17603. |
[77] | B. Thordardottir, A. Malmgren Fänge, C. Lethin, D. Rodriguez Gatta and C. Chiatti, Acceptance and use of innovative assistive technologies among people with cognitive impairment and their caregivers: A systematic review, BioMed Research International 2019: ((2019) ), 9196729. doi:10.1155/2019/9196729. |
[78] | J.Y. Tung, R.V. Rose, E. Gammada, I. Lam, E.A. Roy, S.E. Black and P. Poupart, Measuring life space in older adults with mild-to-moderate Alzheimer’s disease using mobile phone GPS, Gerontology 60: (2) ((2014) ), 154–162. doi:10.1159/000355669. |
[79] | N.J. van Eck, L. Waltman and V. Manual, VOSviewer Manual (2018). Accessed: 2020-03-04. |
[80] | E.J.W. Van Someren, Actigraphic monitoring of movement and rest-activity rhythms in aging, Alzheimer’s disease, and Parkinson’s disease, IEEE Transactions on Rehabilitation Engineering 5: (4) ((1997) ), 394–398. doi:10.1109/86.650297. |
[81] | N.K. Vuong, S. Chan, C.T. Lau and K.M. Lau, Feasibility study of a real-time wandering detection algorithm for dementia patients, in: Proceedings of the International Symposium on Mobile Ad Hoc Networking and Computing (MobiHoc), (2011) , pp. 1–4. ISBN 9781450307802. doi:10.1145/2007036.2007050. |
[82] | N.K. Vuong, S.G.A. Goh, S. Chan and C.T. Lau, A mobile-health application to detect wandering patterns of elderly people in home environment, in: Proceedings of the Annual International Conference of the IEEE Engineering in Medicine and Biology Society, EMBS, (2013) , pp. 6748–6751. ISBN 9781457702167. doi:10.1109/EMBC.2013.6611105. |
[83] | P. Wang, C.S. Chen and C.C. Chuan, Location-aware fall detection system for dementia care on nursing service in Evergreen Inn of Jianan Hospital, in: Proceedings – 2016 IEEE 16th International Conference on Bioinformatics and Bioengineering, BIBE 2016, (2016) , pp. 309–315. ISBN 9781509038336. doi:10.1109/BIBE.2016.70. |
[84] | W.H.O. WHO, WHO global action plan on the public health response to dementia 2017–2025 (2017). Accessed: 2020-03-04. |
[85] | W.H.O. WHO, Dementia: 2021 statistical update (2021). Accessed: 2022-05-03. |
[86] | Y.K. Yuce and K.H. Gulkesen, CaregiverNet: A novel social support intervention for locating and securing wandering Alzheimer’s patients as soon as possible, in: 2013 9th International Wireless Communications and Mobile Computing Conference, IWCMC 2013, (2013) , pp. 1405–1411. ISBN 9781467324793. doi:10.1109/IWCMC.2013.6583762. |
[87] | Y.K. Yüce, K.H. Gülkesen and E.N. Barcin, Balancing autonomy and security over geotracking patients with Alzheimer’s using a personalized geotracking system with social support network, Procedia Computer Science 10: (Pasth) ((2012) ), 1064–1072. doi:10.1016/j.procs.2012.06.150. |
[88] | A. Zhang, K.J. Wang and Z.H. Mao, Design and realization of Alzheimer’s artificial intelligence technologies (AAIT) system, in: Proceedings – 2018 IEEE 6th International Conference on Future Internet of Things and Cloud, FiCloud 2018, (2018) , pp. 141–148. ISBN 9781538675038. doi:10.1109/FiCloud.2018.00028. |
[89] | S. Zhang, S. McClean, B. Scotney, X. Hong, C. Nugent and M. Mulvenna, Decision support for Alzheimer’s patients in smart homes, in: Proceedings – IEEE Symposium on Computer-Based Medical Systems, (2008) , pp. 236–241. ISBN 9780769531656. doi:10.1109/CBMS.2008.16. |