Artificial intelligence and ambient intelligence
Abstract
Ambient intelligence (AmI) is intrinsically and thoroughly connected with artificial intelligence (AI). Some even say that it is, in essence, AI in the environment. AI, on the other hand, owes its success to the phenomenal development of the information and communication technologies (ICTs), based on principles such as Moore’s law. In this paper we give an overview of the progress in AI and AmI interconnected with ICT through information-society laws, superintelligence, and several related disciplines, such as multi-agent systems and the Semantic Web, ambient assisted living and e-healthcare, AmI for assisting medical diagnosis, ambient intelligence for e-learning and ambient intelligence for smart cities. Besides a short history and a description of the current state, the frontiers and the future of AmI and AI are also considered in the paper.
1.Introduction
Intelligence refers to the ability to learn and apply knowledge in new situations [23]. Artificial is something made by human beings and ambience is something that surrounds us. We also tend to consider ambient intelligence (AmI) to be something artificial; the phenomena in natural AmI are the subjects of biology and sociology. The scope of this paper is in human-centric technologies, which require a close alignment of both humans and artificial intelligence (AI) interacting with the environment. Many AI technologies generated by computers originate from the idea of emulating neurological functions and human intelligence.
Figure 1 depicts some of the application areas of AmI; it shows some smart and intelligent systems surrounding the user deploying the AI technologies. In this sense AmI is not a particular technology, but essentially an experience of the user with respect to the service provided by those systems. The cost functions for optimizing the AmI solutions are usually related to improving the subjective human experience, which is only indirectly measurable. Therefore, a successful application of AI and AmI requires us to select the best objective/cost functions that meet the subjective human experience in AmI.
Fig. 1.
Application areas of ambient intelligence (own source).
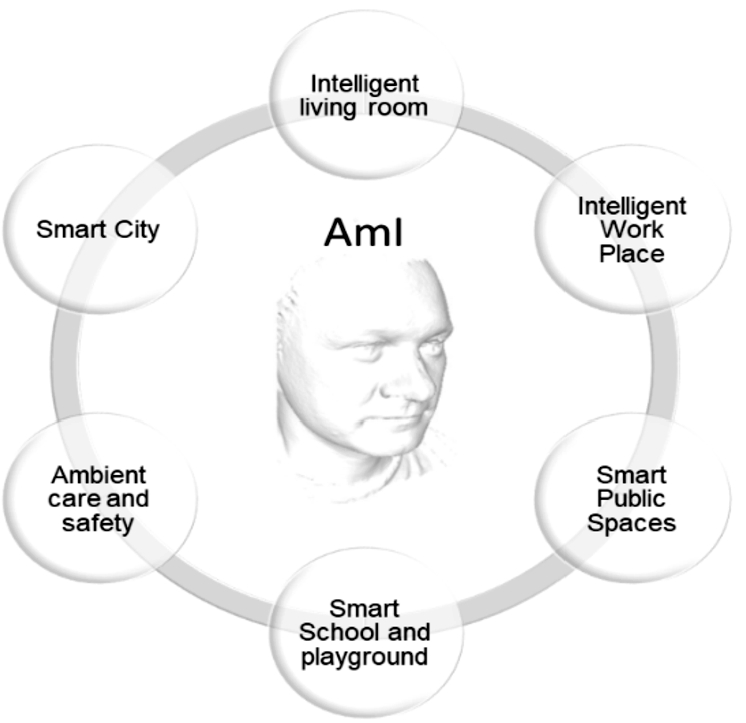
The progress in Artificial Intelligence (AI) and Ambient Intelligence (AmI) follows the rules and laws similar to those in the area of ICT, as described in Section 2. Many of these principles, such as Moore’s law, are exponential. Despite some signs indicating that progress is slowing down, rapid growth is likely to be maintained for AI and AmI in the foreseeable future.
Also, as discussed later in Section 3 about superintelligence [81], in recent research, AI has already reached and surpassed the level of human intelligence in several tasks, such as games, the so-called superintelligence [81] (see Section 3 for a more thorough description), whereas AmI is a seemingly less challenging task. On the other hand, through embodiment, embedding and interactions with humans, super-AmI might emerge even faster than superintelligence. Indeed, some researchers in the field prefer to use the terminology Computational Intelligence (CI) – for instance, in IEEE publications [40] – over artificial intelligence (AI). In this paper we will adopt the terminology “superintelligence” that is related to AI and AmI technologies alike.
The embedding of AI in the natural environment around a user requires the rapid development of advanced technologies in electrical and software engineering. Several advances have been made in the decades after the Handbook of Ambient Intelligence and Smart Environments [51] was published. We will describe some of the main developments and trends in enabling technologies in Section 4. We will also describe some ideas, including the services around the Internet of Things, enabled by the universal cloud connectivity provided by the emerging mobile radio standards [50].
While the communication links in the AmI network shown Fig. 1 are important, this paper will focus on the several main applications, i.e., the nodes of the AmI network, while leaving the communication issue in the AmI system to other review papers in this special issue.
The applications of AI in smart environments have encountered different challenges, for example, the task of recognizing the context that surrounds the user (detailed in several subsequent sections). The human centricity of AmI requires that the computational intelligence is closely bound to the needs and goals of the user and that the learning takes place in a rich living environment. The representation of meaning and knowledge of the environment require semantic models of entities, activities and their relations. In Section 5 we will give an overview of the software agent and knowledge-based technologies that are required to link computational intelligence to human-centric smart environments.
The subsequent sections deal with specific areas of AI closely related to AmI: ambient assisted living and e-healthcare in Section 6, AmI for assisting medical diagnosis in Section 7, AmI for e-learning in Section 8, and AmI for smart cities in Section 9. Finally, in Section 10, a discussion and summary will be provided.
2.Information society fosters AI and AmI
The main hypothesis is that AI and AmI are two closely related disciplines, both heavily dependent on the development of the information society, and both strongly influencing human development. To better understand the driving forces that support and proliferate the future development of AI and AmI, we briefly review the information-society laws, as one of the indicators of the past and the future.
An information society [27] is a society where the creation, distribution, use, integration and manipulation of information is a central economic, political and cultural activity. Its main drivers are digital information and communication technologies (ICTs), which have resulted in an information explosion and are profoundly changing all aspects of our society. The information society has succeeded the industrial society and might lead to the knowledge or intelligent society.
The explosive growth of information can be demonstrated with a variety of data. For example, the world’s technological capacity to store information grew during the period from 1986 to 2007 at a sustained annual rate of 25%. The world’s capacity to compute information grew from 3.0 × 108 MIPS in 1986 to 6.4 × 1012 MIPS in 2007, experiencing a growth rate of over 60% per year for the past two decades [39]. This progress resulted in a huge boost for human civilization, as demonstrated by the rapid growth in the number of Internet users (see Fig. 1).
The progress of the information society can also be presented through several information-society laws or computing laws [75]. There are several important laws shared by ICT, AI and AmI, for example, Moore’s law, Kryder’s law, Keck’s law and Makimoto’s law, among many others.
Moore’s law can be employed for the growth of the capabilities of electronic devices, e.g., chips, which is exponential; the number of transistors in a dense integrated circuit doubles about every 2 years. This has been valid for half a century [75,79]. Kryder’s law can be employed when, for example, disk capacity grows exponentially, even faster than Moore’s law [62]. Keck’s law (similar to Gilder’s law) can be employed when, for example, communication capabilities (actual traffic) grow exponentially. It was successfully used to predict the trends for data rates in optical fibres in the past four decades [37]. Makimoto’s law can be used, for example, for a 10-year cycle between research and standardization, meaning that one can predict future commercial capabilities by looking into today’s research facilities [75].
It is worth mentioning that there are many more related laws [28]. They present the core properties of progress in past decades and also provide good indications for the near future. The laws themselves, the number of associated rules and the consequences are fascinating. For example, the total production of semiconductor devices equates to one transistor per one metre of our galaxy’s diameter and billions of transistors per one star in our galaxy. The calculation is based on the data [75] that transistor production reached 2.5 × 1020 in 2014, which is 250 billion billion. In comparison, our Milky Way galaxy has a diameter between 100,000 and 180,000 light years, it contains 100–400 billion stars and 100 billion planets. Incidentally, there are also around 100 billion neurons in our heads, according to numerous reports.
In recent years there were several reports stating that the use of exponential laws in the information society, such as Moore’s or Keck’s law, is slowing down since we are approaching the physical limits. Although there are hard limitations in many processes, in particular for the exponential ones, we do not need to jump to conclusions. For example, consider the share of Internet users in the population: Fig. 2 not only indicates the rapid growth, but also the upper limit of 100%. However, even in the extreme scenario when the whole population uses the Internet, there could still be a growing number of devices and intelligent systems using the Internet, thus increasing the absolute number of overall users. This limit seems to be far above the total number of human users.
Fig. 2.
Internet users per 100 inhabitants 1997 to 2007; ICT Data and Statistics (IDS), International Telecommunication Union (ITU). Retrieved 25 May 2015. Archived 17 May 2015 at the Wayback Machine.
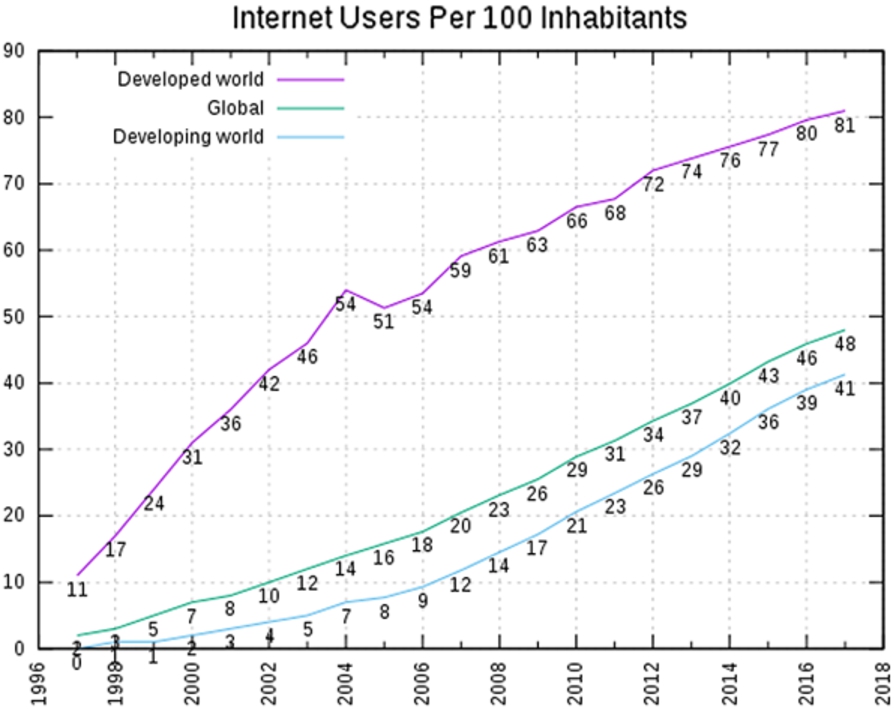
Similarly, warnings about the progress of ICs beginning to stall have been issued for years. But Moore’s law has been relatively stable over decades, with an annual gain in chip capacities of 50%, even though several partial Moore’s laws already ended [19]. What happened is that when one method to increase the chip’s performance started saturating, another one immediately followed, which successfully follows the overall prediction of Moore’s law. An example would be designing larger chips with more layers when the technology of one chip became stonewalled. Even though several limitations were already reached, there are several new possibilities to continue, following the predictive trends from Moore’s law for several decades, such as new technologies, including quantum computing or 3D connecting chips with sensors or sophisticated algorithms. There are always optimists and pessimists, but even in the worst scenario, the progress will just be slowing down, far from coming to an abrupt end.
What is certain is that IC technologies have significantly spurred human progress and that this progress will continue at a roughly similar pace in the decades to come. This progress has and will largely influence the progress of AI and AmI.
3.Relations between AI and AmI, super-AI and super-AmI
AI, also called machine intelligence (MI), is the intelligence demonstrated by machines, in contrast to the natural intelligence (NI) displayed by humans and other animals. AI, therefore, implements human cognitive functions such as perceiving, processing input from the environment and learning. Whenever intelligence is demonstrated by machines in the surrounding environment, it is referred to as ambient intelligence. Due to its focus on humans and the environment, AmI represents far more than just a huge number of AI application areas; rather it is a network of different areas (See Fig. 1).
Under the AmI framework, AI research can be regarded as AmI-oriented from its core since it is often characterized as the study of intelligent agents [78]. An intelligent agent therein is a system that perceives its environment and takes actions to improve its chance of success [59]. (See Section 5 for more details of intelligent agents.)
The birth year of AI is now generally accepted as 1956, with the Dartmouth conference, where John McCarthy defined the terminology of Artificial Intelligence. In Europe, several researchers recognize the paper [73] of Alan Turing, published in 1950, as the start of AI. However, while the concept was the same, the terminology in the 1950 paper was Machine Intelligence (MI). Whatever the case, Alan Turing is regarded by many as the founding father of computer science due to his seminal contributions, including the halting problem, the Turing test, the Turing machine, and the decoding of Hitler’s Enigma machine that helped to end the war more quickly and thus saved millions of human lives [58].
Whereas AI and AmI have seen their share of over-optimism and corresponding over-criticism, the overall, and the recent, progress corresponds to the exponential growth of the information-society laws. Each year there are scores of new achievements attracting worldwide attention in academia, gaming, industry and real life. For example, autonomous vehicles are constantly improving, and they are being introduced into more and more countries, whereas IoT, smart homes and smart cities are at the centre of current research.
AI and AmI are everywhere, and they are already making a big impact on our everyday lives. Together, they make around 100 trillion decisions a day. Smartphones, cars, banks, houses and cities all use artificial intelligence on a daily basis. We are probably aware of it when we use Siri, Google or Alexa to get directions to the nearest petrol station or to order a pizza. More often though, users are not aware that they are using intelligent services, e.g., when they make a purchase by credit card with a system in the background checking for potential fraud, or when an intelligent agent in a smart home regulates user-specific comfort.
How big and complex the research efforts in AI and AmI have become is even evident from a quick look at a basic teaching book for universities [59]. Each of the chapters in the book is represented in detail in several additional books and courses at top universities.
Similar progress can be seen from the trend at recent annual IJCAI conferences [41]: in 2009, 1291 papers were submitted to the IJCAI conference, in 2016 there were 2,294 papers in New York, 2,540 papers in Melbourne were reviewed in 2017, and 37% more appeared in Stockholm in 2018.
Amazing progress has been demonstrated, for example, in chess, where humans have been playing the game for 1500 years, and computers’ domination was first demonstrated in 1997. In 2018, AlphaZero learned only from the formal rules of the game, and just for 4 hours, and displayed superior performance to Stockfish, which won several championships. AlphaZero played the first 100-game match with 28 wins, 72 draws, and zero losses. One of the comments after this performance is attributed to the grandmaster Peter Heine Nielsen speaking to the BBC: “I always wondered how it would be if a superior species landed on Earth and showed us how they played chess. Now I know.”
When fed with huge numbers of examples and with fine-tuned parameters, AI methods and in particular deep neural networks (DNNs) regularly beat the best human experts in increasing numbers of artificial and real-life tasks [41], such as diagnosing tissue in several diseases. There are other everyday tasks, e.g., the recognition of faces from a picture, where DNNs recognize hundreds of faces in seconds, a result no human can match. Figure 3 demonstrates the progress of DNNs in visual tasks: around 2015 the visual recognition in specific domains was comparable to humans; now it has surpassed humans quite significantly in several visual tests. Of relevance to human-reserved applications, DNNs are currently starting to break the CAPTCHA test – a simple, yet a widely applied, method to differentiate between software agents and humans. These methods are directly applicable in the AmI fields, enabling rapid growth in particular areas like taking care of the elderly, smart devices, homes and cities. For example, in one of the core areas in AmI research, human-activity recognition, the application of deep-learning algorithms has been popular in recent years, see [40,51,82,83].
DNNs make it possible to solve several practical tasks in real life, such as detecting cancer [17,31,32,84] or Alzheimer’s [9,60,61]. Furthermore, DNN studies of facial properties can reveal several diseases, sexual orientation, IQ, and political preferences. When shown five photos of a man, a recent system was able to correctly select the man’s sexuality 91 percent of the time, while humans were able to perform the same task with less than 70% accuracy [46]. While these achievements might seem unrelated to the field of AmI, it is quite the contrary – one of the essential tasks of AmI is to detect the physical, mental, emotional and other states of a user. In real life, it is important to provide the user with the comfort required at a particular moment without demanding tedious instructions.
Each year, more and more tasks are reported where humans are bypassed by AI (or computers). The phenomenon is termed “superintelligence,” often related to the singularity theory [47]. The seminal work for super-intelligence is quite probably Bostrom’s “Superintelligence: Paths, Dangers, Strategies” [13]. Another interesting, more technically oriented, viewpoint is presented in the book “Artificial Superintelligence: A Futuristic Approach” by R. V. Yampolskiy [81].
Superintelligence can be applied to a particular domain like chess, or to more general tasks when AI will likely challenge human society for supremacy in the world. This led to the world’s engineering leaders, like Elon Musk, Bill Gates and the late Stephen Hawking, to write the Open Letter on Artificial Intelligence in 2015, warning about the power, potential benefits, threats as well as pitfalls if AI is misused. The AI (and related fields, e.g., robotics) community reacted by establishing a StopKillerRobots movement that has 27 countries endorsing it. The European Parliament passed a resolution on September 12, 2018, calling for an international ban on lethal autonomous weapons systems.
Another milestone was the acceptance of the 4 Asilomar’s principles defined at The Future of Life Institute’s second BAI conference on the future of AI organized in January 2017 [3]. The opinion of the BAI 2017 attendees and the world-wide AI community is widely held: “a major change is coming, over unknown timescales but across every segment of society, and the people playing a part in that transition have a huge responsibility and opportunity to shape it for the best.” Therefore, a list of principles was designed to provide positive directions for future AI research.
In reality, AI has already provided useful tools that are employed every day by people all around the world. Its continued development, guided by the principles above, will offer amazing opportunities to help and empower people in the decades and centuries ahead. No wonder that AI is also the battleground for dominance among the world’s major powers [49]. However, there seems to be several obstacles to overcome before AI reaches overall superintelligence, among others the lack of multiple interactive reasoning [29] or general intelligence [59].
It is quite interesting that the term superintelligence was not immediately followed by the super-ambient-intelligence (SAmI). Indeed, many AmI tasks are in a way similar to optimization tasks, where for a long time computers have far surpassed human experts. Therefore, SAmI might appear even before the elementary superintelligence itself, whereas superintelligence will almost directly elevate SAmI. Not to mention that all bans, such as on autonomous weapons and the other superintelligence concepts and activities, are strongly connected to SAmI, and often much more. For example, when taking care of humans and dealing with the environment, the user must not only survive but feel as comfortable as possible, thus AmI must have in mind other criteria like energy consumption or safety. In addition, while hostile superintelligence in movies often escapes in the Web, in reality, SAmI would escape even more easily in the environment of smart devices, homes and cities. Unfortunately, no book about SAmI was published so far, somehow indicating that we might be sloppy when dealing with SI and SAmI.
4.Ambient intelligence
The first of several sections of the paper is used to define AI, the AmI background and the dilemmas faced. Indeed, AmI shares its progress with the progress of IC technologies and AI, spurring it on its own from time to time. For example, one is related to a side effect of the information society – an information overload because of computers and electronic devices being faster by a factor of a million to a billion when basic information tasks such as computation or storing information are concerned. As a result, we do not want to deal with electronic devices when everyday tasks are involved, for example, when controlling home appliances. We prefer to set guidelines and let the AmI take care of it – including the control and execution.
Ambient intelligence refers to electronic environments that are sensitive and responsive to the presence of people. The term was originally developed in the late 1990s by Eli Zelkha and his team at Palo Alto Ventures [2], and was later extended to the environment without people as well: “In an ambient intelligence world, devices work in concert to support people in carrying out their everyday life activities, tasks and rituals in an easy, natural way using information and intelligence that is hidden in the network connecting these devices (for example The Internet of Things).”
A modern definition was delivered by Juan Carlos Augusto and McCullagh [6]: “Ambient Intelligence is a multi-disciplinary approach which aims to enhance the way environments and people interact with each other. The ultimate goal of the area is to make the places we live and work in more beneficial to us. Smart Homes is one example of such systems, but the idea can also be used about hospitals, public transport, factories, and other environments.”
AmI is aligned with the concept of the “disappearing computer” [65,76]. The AmI field is very closely related to pervasive computing and ubiquitous computing, and also to context awareness, and human-centric computer interaction design. Among the most important properties of AmI systems we can find:
embedded: devices are integrated into the environment; they are “invisible”.
context-aware: they recognize a user, possibly also the current state of the user and the situational context.
personalized: they are often tailored to the user’s needs.
adaptive: AmI systems change their performance in response to changes in the user’s physical or mental state.
anticipatory: they can anticipate user’s desires without conscious mediation.
unobtrusive: discrete, not providing anything but the necessary information about the user to other devices and humans.
non-invasive: they do not demand any action from the user, rather they act on their own.
In the light of these features, several contributions related to the JAISE journal can be incorporated, for example:
These are seminal works contributing to the field of AmI, accompanied by several others [5].
In the following sections, several areas especially important for AI and AmI are analysed, starting with agents and the Semantic Web.
5.When multi-agent systems meet the semantic web: Representing agents’ knowledge in AmI scenarios
The concept of a software agent is based on representing an autonomous entity in a computer program that is embedded in a specific environment for the intelligent management of complex problems, probably interacting with other agents. This idea has given rise to the definition of multi-agent systems (MAS) [44,77,80]. These systems aim to coordinate the various actors within them and to integrate their particular goals into a common objective. MAS applications cover different situations: the management of problems whose elements are physically distributed; when the solution to solve a problem consists of using heterogeneous knowledge that is maintained by different agents, etc.
A traditional problem in developing MAS lies in finding a common way to represent the knowledge these agents share. One of the most commonly used alternatives in the past 10 years to solve this problem is the adoption of ontologies to express the domain information the agents are working on.
In a nutshell, an ontology allows us to formally define the terms and relationships that comprise the vocabulary of a domain along with a series of operators to combine both elements [36]. Once defined, the vocabulary is shared by all the agents and used by them to make specific statements about the domain. Thus, an agent using the ontology of that domain can interpret any statement made with that vocabulary.
With the rise of the Semantic Web, technologies to define and manage ontologies such as languages, standards, reasoners, etc. have increased considerably. The original idea of the Semantic Web concentrates on the services available on the Internet being carried out through intelligent agents that are capable of processing information on the Web, reasoning with it and interacting with other agents and services to satisfy the requirements imposed by users [10]. Currently, the combination of MAS and Semantic Web technologies is presented as an alternative to manage knowledge, not only at the Web layer but at a more general scale where systems and organizations need to understand their domain and reason about it to act intelligently [11].
Next, let us review some relevant papers published in JAISE that have dealt with the integration of MAS and Semantic Web technologies and the fields of AmI where such proposals have been applied.
Regarding the representation of knowledge in MAS for general purposes, one of the first works published in the journal is the paper by Daoutis et al. [22]. They propose augmenting perceptual data gathered from different sensors using ontologies that define concepts such as location, spatial relations, colours, etc. As a result, symbolic and meaningful information is obtained from the environment. Moreover, they use common-sense reasoning to reason about the elements in the environment. This knowledge-representation model is embedded into a smart environment called PEIS-HOME. The top level of this system is presented as an agent that can communicate with other artificial agents and humans on the conceptual level using the symbolic information represented by ontologies.
Another proposal by Nguyen et al. [52] describes a framework called PlaceComm to collect and share knowledge about the concept “place” as a key abstraction. An ontology named the Place-Based Virtual Community is developed to represent common concepts for any place: context, time, device, activity, service, etc. A MAS is used in this framework to gather different types of data, such as context and user information. The agents use the ontology to exchange information within the system. Several applications are presented as a proof of concept, as applications to guide the user in a place or to pose queries about the people in a place.
Following a similar approach, Pantsar-Syväniemi et al. [54] introduce an adaptive framework to develop smart-environment applications. This work provides generic ontologies to represent context, security and performance management, and therefore it is possible to reuse knowledge by separating these ontologies from domain ontologies. The ontologies are connected to applications through rules. To achieve adaptivity, the authors propose a context-aware micro-architecture based on three agents, i.e., context monitoring, context reasoning, and context-based adaptation agents.
In a more recent work by Ayala et al. [7], a MAS is introduced for managing the development of the Internet of Things (IoT) systems. The authors provide an agent platform called Sol to deal with IoT requirements such as the diversity of heterogeneous devices and communication among devices regardless of the technologies and protocols supported by such devices. The agents’ knowledge is represented by using an ontology named SolManagementOntology, which contains concepts such as service, agent profile, group of agents, etc. This ontology aims to represent the information of the IoT system that is managed and exchanged in the agent platform. As a proof of concept the authors show the performance of the Sol platform in an intelligent-museum scenario.
Finally, it is worth mentioning the survey by Preuveneers and Novais [56] on software-engineering practices for the development of applications in AmI. In this survey the authors include relevant works on the representation of knowledge through ontologies in MAS along with their reasoning capabilities. They identify the adoption of these multi-agent systems as a compelling alternative for developing middleware solutions that are necessary in AmI applications.
Shifting now to works devoted to the use of MAS based on semantic technologies for specific AmI applications and services, the fields most frequently related to these works are those of human-centric applications, smart spaces, health, security and reputation.
One of the most productive areas of work has to do with human-centric applications. T. Bosse et al. [12] propose an agent-based framework to study human behaviour and its dynamics in a variety of domains, from driving activities to the assistance of naval operations. This framework is composed of several levels, ranging from the agent-interaction level to the individual agent-design level. In this work not only are human states (physical and mental) and behaviours represented using ontologies, but also the agents’ internal processes (beliefs, facts, actions) are defined in this manner. Another work focused on studying human interactions with the environment is that by Dourlens et al. [24]. Here, the authors utilize semantic agents in the form of Web services to compose ad-hoc architectures capable of acting with the environment. The agents are empowered with four abilities: perception, by using input Web services; understanding, by fusing different sources of information; decision, by reasoning on the available information; and action, by using output Web services. The agents’ knowledge (here called the Agent’s memory) is composed of three ontologies that represent events in the environment and their models, including meta-models to represent the relationships among the events. These ontologies are not only used to store agents’ knowledge, but also as part of the content of the agents’ communication language. The authors apply their system to daily-activities scenarios for monitoring and assisting elderly and disabled people. More specific work on the modelling of human activity within a smart home is presented by Ni et al. [53]. This model is composed of three ontologies to represent user information, smart-home context and activity daily living processes. Moreover, the authors adopt an upper-level ontology called DOLCE + DnS Ultralite to achieve the integration with domain-specific ontologies to offer interoperability with other smart-home applications. The concept of the agent is modelled to represent both the human user or the artificial agent’s profile and the static information.
The research line on smart spaces has also been studied as a practical field to apply the integration of MAS and Semantic Web technologies. For instance, Gravier et al. [35] study the management of semantic conflicts among agents in open environments. Such environments produce unpredictable and unreliable data, which may end by generating contradictory knowledge in the agents. In this work, the authors present an automatic approach to coping with conflicts in the OWL ontologies that represent the agents’ knowledge base. A different work by Stavropoulos et al. [63] focuses on monitoring and saving energy in the context of a smart university building. Rule-based agents are integrated into the middleware that monitors the use of energy in the International Hellenic University. These agents are capable of behaving both in a reactive and deliberative manner, based on the knowledge acquired from the middleware and represented using ontologies and rules. Finally, it is worth mentioning the work by Campillo-Sanchez et al. [15] where a smart space is simulated to test context-aware services for smartphones. The authors introduce the UbikMobile architecture that allows the design of simulated environments in a graphical manner. Following an agent-based social simulation approach, software agents are employed as users to perform automatic tests by interacting with the services developed for smartphones.
Health is another field of research where agents and Semantic Web technologies have been applied. As an example, Aziz et al. [8] have developed an agent-based system to prevent unipolar depression relapse. Several ontologies have been developed to model the depression process, including the recovery and relapse stages. Agents’ observations from sensors, agents’ belief base, and agents’ actions are all modelled using ontologies. Using this knowledge, a multi-agent system is built to monitor and reason about the depression status of the user. Another example can be found in the work of Kafali et al. [45], which is focused on the management of diabetes through an intelligent-agent platform called COMMODITY.
There are also some works related to security and reputation. Thus, Dovgan et al. [25] investigate the improvement of user-verification methods through an agent-based system. Firstly, sensor agents observe entry points and store the gathered data in an ontology. Then, a classification agent uses these data together with the users’ previous behaviours to detect any possible anomaly and raise the alarm. The ontology shared by these agents allows them to represent concepts such as access points, workers’ and non-workers’ profiles, different types of sensors (biometric, card reader, video sensors, etc.) as well as events, actions, types of anomaly, etc. Some examples of use, such as stolen-card and forged-fingerprint scenarios are shown as a proof of concept for the system. On the other hand, Venturini et al. [74] have developed a reputation system among intelligent agents, named CALoR (Context-Aware and Location Reputation System), which is mainly based on agents’ spatial and temporal interactions. The authors represent through ontologies the concepts related to these features of location and temporality. These ontologies also contain entities to represent information about recommendation protocols, including action fulfilment and recommendations received from other agents. The agents are implemented in the JADE platform [43] to show several tests of their reputation system when distance and temporal information are introduced in intelligent ambient systems.
6.Ambient assisted living and e-healthcare
This section describes one important application area in AmI. The communication link to the human-centric AmI system is important. However, for the sake of focusing, we only describe the AI technologies employed.
According to the United Nations [72], population aging is taking place in nearly all countries of the world. It is estimated in [71] that the global share of elderly people accounted for 11.7% in 2013 and will continue to grow, reaching 21.1% by 2050. Many of them choose to live at home, while others live in care centres for the elderly. However, there is a general shortage of caring personnel. Hence, a great need emerges for automatic machine-based assistance. Ambient assisted living and e-healthcare may offer intelligent services that give support to people’s daily lives.
An ambient-assisted-living system creates human-centric smart environments that are sensitive, adaptive and responsive to human needs, habits, gestures and emotions. The innovative interaction between the human and the surrounding environment makes ambient intelligence a suitable candidate for care of the elderly [83].
As shown in Fig. 4, when creating a human-centric smart environment for assisted living, two of the most fundamental tasks are to (a) classify normal daily activities; and (b) detect abnormal activities. Examples of ambient assisted living for the elderly include detecting anomalies like falling, robbery or fire at home, recognizing daily living patterns, and obtaining the statistics associated with various daily activities over time. Among all the activities, detecting a fall is one of the topics attracting the most attention, due to the associated risks such as bone fracture, stroke or even death. Triggering emergency help is often necessary, especially for people who live alone. Many different methods have been developed by exploiting different types of sensors, e.g., smart watches, sound sensors, wearable motion devices (gyroscopes, speedometers, accelerometers), visual, range and infrared (IR) cameras. Each sensor has its pros and cons, for example, a wearable device is straightforward; however, it requires regular charging and also the elderly can easily forget to wear them. For video-based technologies there are some privacy concerns, but they can be solved by near-real-time feature extraction followed by discarding the original data, or by replacing depth or IR data where only the person’s shape is visible. Figure 5 shows the graphical user interface (GUI) of an RGB-depth video-based activity-classification system for AmI assisted living [82].
Fig. 5.
GUI for a sample assisted-living system that recognizes typical activities of the elderly at home (from [82]).
![GUI for a sample assisted-living system that recognizes typical activities of the elderly at home (from [82]).](https://content.iospress.com:443/media/ais/2019/11-1/ais-11-1-ais180508/ais-11-ais180508-g005.jpg)
7.AmI for assisting medical diagnosis: Brain tumours and Alzheimer’s disease
This section describes another AmI application area that employs AI technologies for computer-assisted medical diagnosis and telemedicine. AmI in medicine can be the link between patients/subjects and medical doctors/personal spread over large distances in different parts of the world through a computer or a communication network. Apart from the communication link that plays an essential role in this application, AI technologies, especially deep machine-learning methods, play an important role and may provide doctors with an assisted diagnosis or a second opinion for many medical issues. Employing deep-learning methods in medical diagnostics already shows some promise that it could surpass what medical doctors can achieve. For example, by combining MRIs with a biomarker or molecular information in the training process, the diagnosis of brain-tumour types could possibly be done without a biopsy quite soon. Such an application of AmI also makes it possible to exploit medical expertise remotely in different regions in the world.
For example, machine-learning methods, especially deep-learning methods, have been used lately for automatically grading brain tumours and estimating their molecular sub-types without an invasive brain-tissue biopsy. Several deep-learning/machine-learning methods have been successfully exploited for brain-tumour gliomas. For example, some of the latest developments in machine-learning methods for grading gliomas have resulted in state-of-the-art results. In [31] 2D convolutional neural networks (CNNs) in combination with multimodal MRI information fusion was proposed, resulting in a test accuracy of 89.9% for gliomas with/without 1p19q, and of 90.87% for gliomas with low/high grade (see Fig. 6). In [17] a residual CNN was proposed in combination with several datasets along with data augmentation that yielded an IDH prediction accuracy of 87.6% on the test set. In [32] a 3D multi-scale CNN and fusion were used to exploit multi-scale features on MRIs with saliency-enhanced tumour regions that resulted in an 89.47% test accuracy for low/high-grade gliomas. Finally, [84] proposed using an SVM classifier on multimodal MRIs for predicting gliomas with extensive subcategories, like IDH wild, IDH mutant with TP53 wild/mutate subcategories and more with relatively good results.
Fig. 6.
A 2D convolutional network in combination with the multimodal information fusion of MRI for the grading of brain-tumour gliomas (from [31]).
![A 2D convolutional network in combination with the multimodal information fusion of MRI for the grading of brain-tumour gliomas (from [31]).](https://content.iospress.com:443/media/ais/2019/11-1/ais-11-1-ais180508/ais-11-ais180508-g006.jpg)
Another example of a machine-learning application is for the early detection of Alzheimer’s disease (AD), which could provide the possibility of early treatment that delays the progression of the disease. With the huge efforts made by the Alzheimer’s Disease Neuroimaging Initiative (http://adni.loni.usc.edu/about/) and their publicly available dataset, many research works have been proposed on the detection of AD. Among many others, some examples of the latest developments of deep-learning methods for AD detection include DeepAD [60], which resulted in a 98.84% accuracy on a relatively small dataset, by randomly partitioning the MRI dataset where each subject can contain several scans in a different time. The authors of [9] proposed a simple, yet effective, 3D convolutional network (3D-CNN) architecture and tested it on a larger dataset with a training/test set resulting in a 98.74% test accuracy. Further tests on using a subject-based training/test set partition strategy resulted in a 93.26% test accuracy for AD detection. These examples show that early AD detection is possible with the help of AI technologies, though much more research is still needed in this field.
8.Ambient intelligence for e-learning
Ambient intelligence has been developed as a new discipline that is targeted to facilitate the daily activities of all human beings, including a diversity of assessment schemes, interaction styles, pedagogical methods, supporting environments and tools, etc. of the involved learning activities and platforms [4,18,66,67].
Generally speaking, an AmI-assisted e-learning platform provides an adaptive and smart learning environment that can respond to the needs of each learner or learning group. The innovative interaction mechanisms and pedagogical methods between learners and their supporting e-learning platforms create ample opportunities for AmI techniques to carefully analyse and categorize the learners’ characteristics and learning needs together with the supporting environments, tools and other customizable system features to come up with a more effective or personalized e-learning platform. In this direction, there are many active research works on learning analytics [67], intelligent tutoring systems [66], collaborative learning platforms [30], etc.
Learning analytics (LA) [18,26] is an active research area for educators and researchers in the field of e-learning technologies. Essentially, it can help to better understand the process of learning and its environments through the measurement, collection and analysis of learners’ data and context. There are many existing LA techniques [26]; they are typically computationally expensive, thus making them impractical for estimating the learner’s real-time responses to course materials or live presentations on any mobile device with its relatively low computational power and memory size.
In addition to LA, mobile and sensing technologies have been developing surprisingly fast these days, with many new features. The usage of mobile devices, smartphones and tablets is increasing in all walks of life. Children and teenagers often find themselves busily engaged in various activities related to mobile devices, such as playing games on smartphones, exercising with the Microsoft Kinect [70], or learning through educational video clips that are streaming onto their tablets. Given the frequent use of technology, no matter whether it is inside or outside the classroom, there is an ever-pressing problem: e-Learning [4] seldom tailors itself to each child, thus making it more difficult to determine the individual’s true grasp or understanding of the material being taught. However, AmI or AI methods [11,31,35,38], specifically for facial feature detection and recognition techniques [16], are advancing very rapidly. With the combination of relevant AmI methods, mobile computing and sensing technologies in our daily living, it is possible to tackle this challenging problem of determining the individual’s actual progress and response to the involved learning materials, possibly delivered anytime and anywhere on mobile devices through next-generation e-learning systems.
In [16] Cantoni et al. give a precise overview of future e-learning systems, from both the technology- and user-centred perspectives. In particular, the visual component of the e-learning experience is emphasized as a significant feature for effective content development and delivery. Besides, the adoption of new interaction paradigms based on advanced multi-dimensional interfaces (including 1D/2D/3D/nD interaction metaphors) and perceptive interfaces (which are capable of acquiring explicit and implicit information about learners and their environment to allow the e-learning systems to “see”, “hear”, etc.) is presented as a promising direction towards more natural and effective learning experiences.
Most conventional e-learning systems utilize user feedback based on questionnaires to collect the relevant information, thus likely resulting in incomplete answers or misleading input. In [4] Asteriadis et al. present a specific facial recognition method for the analysis of learners’ responses to compile feedback related to the behavioural states of the learners (e.g., their levels of interest) in the context of reading an electronic document. This is achieved by using a non-intrusive scheme through employing a simple webcam installed on a desktop or laptop computer to detect and track the head, eye and hand movements [16], and provides an estimation of the level of interest and engagement for each individual learner using a neuro-fuzzy network [42] as an example of the AmI methods. Experiments reveal that the proposed e-learning system can effectively detect reading- and attention-related user states in a testing environment to monitor children’s reading performance.
Also, [66] explores the possible uses of intelligent image-processing techniques such as statistical learning approaches or machine-learning methods [14] with feature extraction and filtering mechanisms to analyse the learners’ responses to educational videos or other online materials. The analysis is based on snapshots of the learners’ facial expressions captured on the webcams of mobile devices such as tablet and laptop PCs. The captured images of the learners’ facial expressions and eye movements can be continuously and quickly analysed by the heuristic-based image-processing algorithm running on the mobile devices or desktop computers for classifying the responses of the learners as “attentive,” “distracted,” “dozing off” or “sleeping.” Fig. 7 shows the screenshots of an AmI-assisted e-learning system assessing the learners’ real-time responses on a tablet PC. The e-learning system uses the pupil detection of the learner to determine whether she is “attentive” (in the left-hand photograph) or being “distracted” (in the right-hand photograph) while viewing an educational video on a tablet PC. The captured images can also be sent to any cloud server for further analyses. Furthermore, the AmI-assisted image analyser running on the mobile devices can help to protect the learner’s eyesight by displaying an alert message when his/her eyes come too close to the webcam. Overall, this will help to create an AmI-assisted, customizable and effective e-learning environment for developing next-generation personalized and mobile learning systems.
Fig. 7.
Screenshots of an AmI-assisted e-learning system to assess the learner’s responses as attentive (left) and distracted (right) on a tablet PC (from [67]).
![Screenshots of an AmI-assisted e-learning system to assess the learner’s responses as attentive (left) and distracted (right) on a tablet PC (from [67]).](https://content.iospress.com:443/media/ais/2019/11-1/ais-11-1-ais180508/ais-11-ais180508-g007.jpg)
Besides facial analytics (FA), there are many AmI-assisted or intelligent tutoring systems [66] that employ AmI methods, including classification algorithms in machine learning or other statistical approaches to adapt the difficulty levels of the online materials and quizzes to each individual or group of learners based on the domain knowledge and/or the learner’s progress. An example is a semi-supervised learning approach [66] in which a concept-based classifier is co-trained with an explicit semantic analysis classifier to derive a common set of prerequisite rules based on a diverse set of online educational resources as the domain knowledge for intelligent tutoring systems. On the other hand, there are various research studies trying to apply one or several classifiers to analyse students’ performance in different courses for adaptive learning. In [48], a naïve Bayesian classifier is used to carefully examine students’ examination results on an open education platform.
Furthermore, there is some research work to integrate AmI approaches for collaborative learning activities. An example is the AmI-based multi-agent framework [30] that allows the development of learning applications based on the pedagogical Computer Supported Collaborative Learning (CSCL) approach and the AmI paradigm. The resulting framework combines different context-aware technologies such that the designed, developed and deployed application is dynamic, adaptive and easy-to-use by both course instructors and learners.
9.Ambient intelligence for smart cities
Kevin Aston, cofounder and executive director of the Auto-ID Center at the Massachusetts Institute of Technology (MIT), first mentioned, in a presentation in 1999, the Internet of Things (IoT) to describe the network connecting objects in the physical world to the Internet. Since then, the rapid development of smart sensors like accelerometers, humidity or pressure sensors, and also high-speed wireless networks such as Wi-Fi or Zigbee have worked hand in hand with numerous AmI methods, including fuzzy-logic-based classifiers or machine-learning algorithms, to support many innovative smart-city applications. According to [69], there are many highly ranked and significant areas of smart-city applications involving various sensors and/or AmI methods, such as those below:
Smart Parking – monitoring the availability of parking spaces in the city;
Structural Health – monitoring the vibrations and material conditions in buildings, bridges and historical monuments;
Noise Urban Maps – monitoring the noises in bar areas and central zones in real time;
Smartphone Detection – detecting an iPhone or Android phone or, in general, any mobile device that works with a Bluetooth or Wi-Fi interface;
Electromagnetic Field Levels – measuring the energy radiated by cell stations and Wi-Fi routers;
Traffic Congestion – monitoring vehicles and pedestrian levels to optimize driving and walking routes;
Smart Lighting – Intelligent and weather-adaptive lighting in street lights;
Waste Management – detecting rubbish levels in containers to optimize rubbish-collection routes;
Smart Roads – Intelligent highways with warning messages and diversions according to climate conditions and unexpected events like accidents or traffic jams.
In addition, there are other aspects of smart-city applications, such as smart water involving portable water monitoring, the detection of water leakages along pipes or monitoring water-level variations in rivers, dams and reservoirs. Other examples of smart-city applications include smart metering that involves energy-consumption monitoring and management in smart grids or the measurement of water flow or pressure in water-transportation systems. In all these smart-city applications, fuzzy-logic-based classifiers, machine-learning or statistical approaches can be applied to learn the ‘regular’ patterns of changes in the flow rate, pressure, usage or another measured parameter, obtained from the smart sensors installed in the underlying application during the training phase. This may help to quickly identify any irregular pattern(s) of changes or movements occurring in the testing or deployment environment, possibly due to various causes such as disasters, faulty sensors, leakage, improper system operations, or the unexpected side effects of reactive processes, among others. In any case, this will help to alert the system administrator about any recovery procedure or pro-active maintenance to avoid any possible damage, risk or shutdown of the overall system.
For instance, deep-learning approaches [34] such as the multi-layer perceptron (MLP) neural network or the long-short-term memory (LSTM) model of the recurrent neural networks [1] are applied for the task of energy forecasting in some real-world applications involving smart meters installed in different smart buildings in a city of South East Asia. In addition, energy data sets obtained from the Global Energy Forecasting Competition [68] or other online sources are used to compare the performance of the involved AmI approaches for energy prediction. The resulting energy-prediction models can also be used to facilitate the concerned organizations in formulating effective energy-saving strategies to achieve a better energy efficiency for the whole building or enterprise. Figure 8 shows plots of the actual hourly energy consumption (highlighted in blue) versus the predicted hourly energy consumption (highlighted in orange/green) for a specific floor of a smart-building application using a deep-learning network from October of 2016 to May of 2017, in which the deep-learning network can achieve an averaged prediction accuracy of 71.7%.
Fig. 8.
Plots of the actual versus predicted hourly energy consumption for a specific floor of a smart-building application using a deep-learning network (from [1]).
![Plots of the actual versus predicted hourly energy consumption for a specific floor of a smart-building application using a deep-learning network (from [1]).](https://content.iospress.com:443/media/ais/2019/11-1/ais-11-1-ais180508/ais-11-ais180508-g008.jpg)
10.Discussion and summary
The progress of ICT and the information society has been exponential for the past 50 years and will continue at approximately the same pace for several decades to come if the ingenuity of researchers and designers manages to find new solutions to bypass the physical limitations of semiconductor technology.
The progress of AI, in general, and superintelligence, in particular, is even brighter. Even on only reasonably better hardware, new solutions will be created due to more advanced software algorithms and systems. Each year several killer AI applications appear, ones that most researchers doubted would be possible in the near future. The progress just amazes us.
The success of AmI relies heavily on the progress of the information society, ICT and AI. We humans are satisfied if the advanced technologies take care of us and do not want our attention or even additional activities besides giving occasional guidelines and orders. We like the invisible computer with the advanced AI hidden in the environment. Some devices like Google home are still present, but the hardware is just the microphone. What matters is the system connected, embedded and with all the other properties of AmI systems.
AmI is sharing its fate and fame with the field of AI – and the trend towards systems bypassing human capabilities is already here. These systems take care of our needs and desires, enable a better, safer, more comfortable life with a smaller environmental burden. Like superintelligence, super-ambient-intelligence (SAmI) is destined to develop as contemporary of superintelligence or even as the first pioneer, harvesting its physical attachment to the real world and constant interactions with humans.
However, the progress will likely not be straightforward as several challenges indicate in terms of design contexts and societal changes [64]. In addition, the AmI term problem is related to the distribution of the field, in particular the near-synonyms “pervasive computing” and “ubiquitous computing”. These terms are currently prevailing at least in the sense of conference attendees. At the same time, the concept, methods and approaches are the same.
Among most relevant AmI areas are the ones described in this paper: semantic web and agents, ambient assisted living, electronic and mobile healthcare, taking care for elderly, medical applications, e-learning, smart home and smart cities. The last two fields are related to IoT papers in this anniversary issue [20,33], while medical issues in this paper are complementing the e-medicine related anniversary paper [55].
The JAISE journal is the leading AmI publication integrating the AmI society around esteemed scientists like Aghajan and Augusto. Its tenth year is therefore of particular importance for this promising area of research and applications.
Hopefully, this special issue on AmI will provoke discussions and awareness, from AmI experts to the general public, and from media to government decision-makers, helping them to understand that the times are changing rapidly, and that new approaches and methods are coming faster than expected, even by the experts.
Acknowledgement
Andrés Muñoz would like to thank the Spanish Ministry of Economy and Competitiveness for its support under the project TIN2016-78799-P (AEI/FEDER, UE).
References
[1] | S.A. Ahmed, Applying Deep Learning for Energy Forecasting, M.Sc. Thesis, the University of Hong, Kong, August, 2017. |
[2] | M. Arribas-Ayllon, Ambient Intelligence: An innovation narrative, available at http://www.academia.edu/1080720/Ambient_Intelligence_an_innovation_narrative, 2003. |
[3] | Asilomar principles, available at https://futureoflife.org/2017/01/17/principled-ai-discussion-asilomar/, 2017. |
[4] | S. Asteriadis, P. Tzouveli, K. Karpouzis and S. Kollias, Estimation of behavioral user state based on eye gaze and head pose – Application in an e-learning environment, Multimedia Tools Application 41: , ((2009) ), 469–493. doi:10.1007/s11042-008-0240-1. |
[5] | J.C. Augusto and H. Aghajan, Thematic issue: Computer vision for ambient intelligence preface, Journal of Ambient Intelligence and Smart Environments 3: (3) ((2011) ), 185. doi:10.3233/AIS-2011-0116. |
[6] | J.C. Augusto and P. McCullagh, Ambient intelligence: Concepts and applications, Computer Science and Information Systems 4: (1) ((2007) ), 1–27. doi:10.2298/CSIS0701001A. |
[7] | I. Ayala, M. Amor and L. Fuentes, The Sol agent platform: Enabling group communication and interoperability of self-configuring agents in the Internet of Things, Journal of Ambient Intelligence and Smart Environments 7: (2) ((2015) ), 243–269. |
[8] | A.A. Aziz, M.C. Klein and J. Treur, An integrative ambient agent model for unipolar depression relapse prevention, Journal of Ambient Intelligence and Smart Environments 2: (1) ((2010) ), 5–20. |
[9] | K. Bäckström, M. Nazari, I.Y.H. Gu and A.S. Jakola, An efficient 3D deep convolutional network for Alzheimer’s disease diagnosis using MR images, in: Proc. of 2018 IEEE 15th International Symposium on Biomedical Imaging (ISBI’18), (2018) , pp. 149–153. doi:10.1109/ISBI.2018.8363543. |
[10] | T. Berners-Lee, J. Hendler and O. Lassila, The semantic web, Scientific American 284: (5) ((2001) ), 34–43. doi:10.1038/scientificamerican0501-34. |
[11] | T. Bosse (ed.), Agents and Ambient Intelligence: Achievements and Challenges in the Intersection of Agent, Vol. 12: , IOS Press, Amsterdam. (2012) . |
[12] | T. Bosse, M. Hoogendoorn, M.C. Klein and J. Treur, An ambient agent model for monitoring and analysing dynamics of complex human behaviour, Journal of Ambient Intelligence and Smart Environments 3: (4) ((2011) ), 283–303. |
[13] | N. Bostrom, Superintelligence – Paths, Dangers, Strategies, Oxford University Press, Oxford, UK, (2014) . |
[14] | J. Brownlee, Machine Learning Mastery, available at http://machinelearningmastery.com/, last visited in 2018. |
[15] | P. Campillo-Sanchez, E. Serrano and J.A. Botía, Testing context-aware services based on smartphones by agent based social simulation, Journal of Ambient Intelligence and Smart Environments 5: (3) ((2013) ), 311–330. |
[16] | V. Cantoni, M. Cellario and M. Porta, Perspectives and challenges in e-learning: Towards natural interaction paradigms, Journal of Visual Languages and Computing 15: ((2004) ), 333–345. |
[17] | K. Chang, H.X. Bai, H. Zhou, C. Su, W.L. Bi, E. Agbodza, V.K. Kavouridis, J.T. Senders, A. Boaro, A. Beers, B. Zhang, A. Capellini, W. Liao, Q. Shen, X. Li, B. Xiao, J. Cryan, S. Ramkissoon, L. Ramkissoon, K. Ligon, P.Y. Wen, R.S. Bindra, W.J. Arnaout O, E.R. Gerstner, P.J. Zhang, B.R. Rosen, L. Yang, R.Y. Huang and J. Kalpathy-Cramer, Residual convolutional neural network for the determination of IDH status in low- and high-grade gliomas from MR imaging, Clin. Cancer Research 25: (5) ((2017) ), 1073–1081. doi:10.1158/1078-0432.CCR-17-2236. |
[18] | M.A. Chatti, A.L. Dychhoff, U. Schroeder and H. Thüs, A reference model for learning analytics, International Journal of Technology Enhanced Learning (IJTEL). Special Issue on “State-of-the-Art in TEL”, 4: (5) ((2012) ), 318–331. |
[19] | A.A. Chien and V. Karamcheti, Moore’s law: The first ending and a new beginning, Computer 46: (12) ((2013) ), 48–53. doi:10.1109/MC.2013.431. |
[20] | J. Chin, V. Callaghan and S.B. Allouch, The Internet of Things: Reflections on the past, present and future from a user centered and smart environments perspective, Journal of Ambient Intelligence and Smart Environments 11: (Tenth Anniversary Issue) ((2019) ), p. 1. |
[21] | D.J. Cook, J.C. Augusto and V.R. Jakkula, Ambient intelligence: Technologies, applications, and opportunities, Pervasive and Mobile Computing 5: (4) ((2009) ), 277–298. doi:10.1016/j.pmcj.2009.04.001. |
[22] | M. Daoutis, S. Coradeshi and A. Loutfi, Grounding commonsense knowledge in intelligent systems, Journal of Ambient Intelligence and Smart Environments 1: (4) ((2009) ), 311–321. |
[23] | Definition of INTELLIGENCE, [online], available at https://www.merriam-webster.com/dictionary/intelligence [accessed: 06-Jun-2018]. |
[24] | S. Dourlens, A. Ramdane-Cherif and E. Monacelli, Tangible ambient intelligence with semantic agents in daily activities, Journal of Ambient Intelligence and Smart Environments 5: (4) ((2013) ), 351–368. |
[25] | E. Dovgan, B. Kaluža, T. Tušar and M. Gams, Improving user verification by implementing an agent-based security system, Journal of Ambient Intelligence and Smart Environments 2: (1) ((2010) ), 21–30. |
[26] | A.L. Dyckhoff, D. Zielke, M. Bültmann, M.A. Chatti and U. Schroeder, Design and implementation of a learning analytics toolkit for teachers, Educational Technology & Society 15: (3) ((2012) ), 58–76. |
[27] | J. Feather, Information Society: A Study of Continuity and Change, 6th edn, in: Facet Publications (All Titles as Published), Facet Publishing, London, (2013) , ISBN-13: 978-1856048187. ISBN-10: 1856048187. |
[28] | M. Gams, Information society and the intelligent systems generation, Informatica 23: ((1991) ), 449–454. |
[29] | M. Gams, Weak Intelligence: Through the Principle and Paradox of Multiple Knowledge, in: Advances in the Theory of Computational Mathematics, Vol. 6: , Nova Science Publishers, New York, (2001) . ISBN-10: 1560728981. ISBN-13: 978-1560728986. |
[30] | Ó. García, R.S. Alonso, D.I. Tapia and J.M. Corchado, CAFCLA: An AmI-based framework to design and develop context-aware collaborative learning activities, in: Ambient Intelligence – Software and Applications. Advances in Intelligent Systems and Computing, A. van Berlo, K. Hallenborg, J. Rodríguez, D. Tapia and P. Novais, eds, Vol. 219: , Springer, Heidelberg, (2013) , pp. 41–48. doi:10.1007/978-3-319-00566-9_6. |
[31] | C. Ge, I.Y.H. Gu, A.S. Jakola and J. Yang, Deep learning and multi-sensor fusion for glioma classification using multistream 2D convolutional networks, in: Proc. of 40th Annual International Conference of the IEEE Engineering in Medicine and Biology Society (EMBC’18), (2018) , pp. 5894–5897. |
[32] | C. Ge, Q. Qu, I.Y.H. Gu and A.S. Jakola, 3D multiscale convolutional networks for glioma grading using MR images, in: Proc. of IEEE International Conference on Image Processing (ICIP’18), (2018) , pp. 141–145. |
[33] | C. Gomez, S. Chessa, A. Fleury, G. Roussos and D. Preuveneers, Internet of things for enabling smart environments: A technology-centric perspective, Journal of Ambient Intelligence and Smart Environments 11: (Tenth Anniversary Issue) ((2019) ), p. 1. |
[34] | I. Goodfellow, Y. Bengio and A. Courvil, Deep Learning, MIT Press, Cambridge, (2016) . |
[35] | C. Gravier, J. Subercaze and A. Zimmermann, Conflict resolution when axioms are materialized in semantic-based smart environments, Journal of Ambient Intelligence and Smart Environments 7: (2) ((2015) ), 187–199. |
[36] | T.R. Gruber, A translation approach to portable ontology specifications, Knowledge Acquisition 5: (2) ((1993) ), 199–220. doi:10.1006/knac.1993.1008. |
[37] | J. Hecht, Is Keck’s Law Coming to an End?, available at https://spectrum.ieee.org/semiconductors/optoelectronics/is-kecks-law-coming-to-an-end, 2016. |
[38] | C. Hennessey, B. Noureddin and P. Lawrence, A single camera eye-gaze tracking system with free head motion, in: Proceedings of the Symposium on Eye Tracking Research and Applications (ETRA’05), San Diego, CA, USA, (2006) , pp. 87–94. |
[39] | M. Hilbert and P. López, Science 332: (6025) ((2011) ), 60–65, available at martinhilbert.net/WorldInfoCapacity.html. doi:10.1126/science.1200970. |
[40] | IEEE Computational Intelligence Society, available at https://cis.ieee.org/ [accessed: 06-Jun-2018]. |
[41] | IJCAI Conference, available at https://ijcai-17.org, 2017. |
[42] | J. Jang, ANFIS: Adaptive-network-based fuzzy inference systems, IEEE Trans Syst. Man Cybernetics 23: (3) ((1993) ), 665–685. doi:10.1109/21.256541. |
[43] | JAVA Agent DEvelopment Framework, available at http://jade.tilab.com/ [accessed: 06-Jun-2018]. |
[44] | N.R. Jennings, K. Sycara and M. Wooldridge, A roadmap of agent research and development, Autonomous Agents and Multi-Agent Systems 1: (1) ((1998) ), 7–38. doi:10.1023/A:1010090405266. |
[45] | Ö. Kafali, S. Bromuri, M. Sindlar, T. van der Weide, E. Aguilar Pelaez, U. Schaechtle, A. Bruno, D. Zuffery, E. Rodriguez-Villegas, M.I. Schumacher and K. Stathis, Commodity 12: A smart e-health environment for diabetes management, Journal of Ambient Intelligence and Smart Environments 5: (5) ((2013) ), 479–502. |
[46] | M. Kosinski and Y. Wang, Deep neural networks are more accurate than humans at detecting sexual orientation from facial images, 2017, https://osf.io/zn79k/. |
[47] | R. Kurzweil, The Singularity Is Near: When Humans Transcend Biology, Vol. 26: , Penguin Books, (2006) . |
[48] | F. Liu, L.Q. Zhang, Y. Wang, F. Lu, F.W. Sun, S.G. Zhang, W.W.T. Fok, V. Tam and J. Yi, Application of naive Bayesian classifier for teaching reform courses examination data analysis in China open university system, in: Proceedings of the 2015 8th International Symposium on Computational Intelligence and Design (ISCID), Conference Center, Zhejiang University, Hangzhou, PRC, December 12–13, (2015) , pp. 25–29. |
[49] | Mail online, Science and technology, Vladimir Putin warns whoever cracks artificial intelligence will ’Rule the world’, available at http://www.dailymail.co.uk/sciencetech/article-4844322/Putin-Leader-artificial-intelligence-rule-world.html, 2017. |
[50] | E. Markakis, G. Mastorakis, C.X. Mavromoustakis and E. Pallis (eds), Cloud and Fog Computing in 5G Mobile Networks: Emerging Advances and Applications, The Institution of Engineering and Technology, London, (2017) . |
[51] | H. Nakashima, H. Aghajan and J.C. Augusto, in: Handbook of Ambient Intelligence and Smart Environments, New York, (2009) . |
[52] | T. Nguyen, S.W. Loke, T. Torabi and H. Lu, PlaceComm: A framework for context-aware applications in place-based virtual communities, Journal of Ambient Intelligence and Smart Environments 3: (1) ((2011) ), 51–64. |
[53] | Q. Ni, I.P. de la Cruz and A.B. García Hernando, A foundational ontology-based model for human activity representation in smart homes, Journal of Ambient Intelligence and Smart Environments 8: (1) ((2016) ), 47–61. doi:10.3233/AIS-150359. |
[54] | S. Pantsar-Syväniemi, A. Purhonen, E. Ovaska, J. Kuusijärvi and A. Evesti, Situation-based and self-adaptive applications for the smart environment, Journal of Ambient Intelligence and Smart Environments 4: (6) ((2012) ), 491–516. |
[55] | A. Prati, C. Shan and K. Wang, Sensors, vision and networks: From video surveillance to activity recognition and health monitoring, Journal of Ambient Intelligence and Smart Environments 11: (Tenth Anniversary Issue) ((2019) ), p. 1. |
[56] | D. Preuveneers and P. Novais, A survey of software engineering best practices for the development of smart applications in Ambient Intelligence, Journal of Ambient Intelligence and Smart Environments 4: (3) ((2012) ), 149–162. |
[57] | C. Ramos, J.C. Augusto and D. Shapiro, Ambient intelligence – The next step for artificial intelligence, IEEE Intelligent Systems 23: (2) ((2008) ), 15–18. doi:10.1109/MIS.2008.19. |
[58] | A. Revell and A. Turing, Alan Turing: Enigma: The Incredible True Story of the Man Who Cracked the Code, Computing Machinery and Intelligence, Mind, 1950, Paperback – August 10, 2017. |
[59] | S. Russel and P. Norvig, Artificial Intelligence: A Modern Approach, 3rd edn, (2014) , Pearson Education Limited, Upper Saddle River. ISBN-13:978-0136042594. |
[60] | S. Sarraf, G. Tofighi et al., DeepAD: Alzheimer’s disease classification via deep convolutional neural networks using MRI and fMRI, bioRxiv, available at https://www.biorxiv.org/content/early/2016/08/21/070441.full.pdf+html, 2016. doi:10.1101/070441. |
[61] | M. Scudellari, Eye Scans to Detect Cancer and Alzheimer’s Disease, available at https://spectrum.ieee.org/the-human-os/biomedical/diagnostics/eye-scans-to-detect-cancer-and-alzheimers-disease, 2017. |
[62] | G. Shroff, The Intelligent Web: Search, Smart Algorithms, and Big Data, Oxford University Press, London, (2015) . |
[63] | T.G. Stavropoulos, G. Koutitas, D. Vrakas, E. Kontopoulos and I. Vlahavas, A smart university platform for building energy monitoring and savings, Journal of Ambient Intelligence and Smart Environments 8: (3) ((2016) ), 301–323. doi:10.3233/AIS-160375. |
[64] | N. Streitz, D. Charitos, M. Kaptein and M. Böhlen, Grand challenges for ambient intelligence and implications for design contexts and smart societies, Journal of Ambient Intelligence and Smart Environments 11: (Tenth Anniversary Issue) ((2019) ), p. 1. |
[65] | N. Streitz and P. Nixon, Special issue on ‘The disappearing computer’, Communications of the ACM 48: (3), ((2005) ), 32–35. doi:10.1145/1047671.1047700. |
[66] | V. Tam, E.Y. Lam, S.T. Fung, A. Yuen and W.W.T. Fok, Enhancing educational data mining techniques on online educational resources with a semi-supervised learning approach, in: Proceedings of the IEEE International Conference on Teaching, Assessment and Learning for Engineering (TALE 2015), United International College, Zhuhai, PRC, December 10–12, (2015) , pp. 210–213. |
[67] | V. Tam, E.Y. Lam and Y. Huang, Facilitating a personalized learning environment through learning analytics on mobile devices, in: Proceedings of the IEEE International Conference on Teaching, Assessment and Learning for Engineering (TALE 2014), Wellington, New Zealand, December 8–10, (2014) , pp. 8–10. |
[68] | The Kaggle Development Team, Global Energy Forecasting Competition 2012 – Load Forecasting, available at https://www.kaggle.com/c/global-energy-forecasting-competition-2012-load-forecasting, last visited in May, 2016. |
[69] | The Libelium Development Team, Top 50 Sensor Applications for A Smarter World, available at http://www.libelium.com/resources/top_50_iot_sensor_applications_ranking, last visited in May, 2018. |
[70] | The Microsoft Kinect Development Team, Kinect for Windows, available at http://www.microsoft.com/en-us/kinectforwindows/, last visited in April, 2014. |
[71] | The United Nations, World population ageing 2013, Population Division, Department of Economic and Social Affairs (DESA), United Nations, 2013, pp. 1–95. |
[72] | The United Nations, World population prospects: The 2015 revision, key findings and advance tables, Population Division, Department of Economic and Social Affairs (DESA), United Nations, 2015, pp. 1-59. |
[73] | A.M. Turing, Computing machinery and intelligence, Mind 56: (236) ((1950) ), 433–460. doi:10.1093/mind/LIX.236.433. |
[74] | V. Venturini, J. Carbo and J.M. Molina, CALoR: Context-aware and location reputation model in AmI environments, Journal of Ambient Intelligence and Smart Environments 5: (6) ((2013) ), 589–604. |
[75] | R. Vetter (ed.), Computer laws revisited, Computer 46: (12) ((2013) ), 38–46. |
[76] | M. Weiser, The computer for the twenty-first century, Scientific American, 165: ((1991) ), pp. 94–104. Weiser, M. (1993). Hot topics: Ubiquitous. |
[77] | G. Weiss, Multiagent Systems: A Modern Approach to Distributed Artificial Intelligence, MIT Press, Cambridge, (1999) . |
[78] | G. Weiss, Multiagent Systems, 2nd edn, in: Intelligent Robotics and Autonomous Agents Series, MIT Press, Cambridge, (2013) . ISBN 13: 978-0262018890. |
[79] | J.M. Wilson, Computing, Communication, and Cognition, Three Laws that define the internet society: Moore’s, Gilder’s, and Metcalfe’s, available at http://www.jackmwilson.net/Entrepreneurship/Cases/Moores-Meltcalfes-Gilders-Law.pdf, 2012. |
[80] | M. Wooldridge, An Introduction to Multiagent Systems, John Wiley & Sons, New York, (2009) . |
[81] | R.V. Yampolskiy, Artificial Superintelligence: A Futuristic Approach, 1st edn, Chapman and Hall/CRC, Boca Raton, (2015) . |
[82] | Y. Yun and I.Y.H. Gu, Riemannian manifold-valued part-based features and geodesic-induced kernel machine for human activity classification dedicated to assisted living, Computer Vision and Image Understanding 161: ((2017) ), 65–76. doi:10.1016/j.cviu.2017.05.012. |
[83] | Y. Yun and I.Y.H. Gu, Visual information-based activity recognition and fall detection for assisted living and eHealthCare, in: Ambient Assisted Living and Enhanced Living Environments: Principles, Technologies and Control, C. Dobre, C. Mavromoustakis, N. Garcia, G. Mastorakis and R. Goleva, eds, Chapter 15, Elsevier, Amsterdam, (2017) , pp. 395–425. ISBN-13: 978-0-12-805195-5. doi:10.1016/B978-0-12-805195-5.00015-6. |
[84] | X. Zhang, Q. Tian et al., Radiomics strategy for molecular subtype stratification of lower-grade glioma: Detecting IDH and TP53 mutations based on multimodal MRI, Journal of Magnetic Resonance Imaging 48: (4) ((2018) ), 916–926. doi:10.1002/jmri.25960. |