Application of a Novel Endpoint Staging Framework: Proof of Concept in the AMBAR Study
Abstract
Background:
A theoretical endpoint staging framework was previously developed and published, aligning outcomes (i.e., memory) to the stage of Alzheimer’s disease (AD) in which a given outcome is most relevant (i.e., has the greatest risk of degradation). The framework guides the selection of endpoints measuring outcomes relevant within a target AD population. Here, a proof of concept is presented via post-hoc analyses of the Alzheimer Management by Albumin Replacement (AMBAR) Phase 2b clinical trial in patients with AD (NCT01561053, 2012).
Objective:
To evaluate whether aligning endpoints measuring cognition, function, and quality of life to hypothesized ‘target’ stages of AD yields magnitudes of treatment efficacy greater than those reported in the AMBAR full analysis set (FAS).
Methods:
Three endpoints were tested: ADAS-Cog 12, ADCS-ADL, and QoL-AD. The magnitude of treatment efficacy was hypothesized to be maximized in the target stages of mild, mild-to-moderate, and very mild AD, respectively, compared to the full analysis set (FAS) and non-target stages.
Results:
For ADAS-Cog 12, the magnitude of treatment efficacy was largest in the non-target stage (–4.0, p = 0.0760) compared to target stage and FAS. For ADCS-ADL and QoL-AD, the magnitude of treatment efficacy was largest in the target stage (14.2, p = 0.0003; 2.4, p < 0.0001, respectively) compared to non-target stage and FAS.
Conclusions:
Findings indicated that evaluating endpoints in the most relevant AD stage can increase the magnitude of the observed treatment efficacy. Evidence provides preliminary proof of concept for the endpoint staging framework.
INTRODUCTION
In the last decade, more than 200 clinical Alzheimer’s disease (AD) programs have been abandoned or have failed [1, 2]. Prior to 2003, only five therapies had been approved for the treatment of AD, all with only modest symptomatic improvement in people with overt dementia [3]. Efforts to develop a disease-modifying therapy (DMT) for AD have been largely unsuccessful; two DMTs (aducanumab and lecanemab) have been granted accelerated approval to date, with the clinical benefit of aducanumab yet to be confirmed in the upcoming confirmatory trial(s) [4]. Reasons hypothesized for the failure of AD trials include: 1) diagnostic inaccuracy of subjects enrolled into trials stemming from the sole use of historical clinical assessment-based criteria in the absence of biomarkers; 2) inadequate understanding of the heterogeneity of AD, which could include specific clinical stage of disease more likely to respond to particular treatments; 3) failure to select a relevant therapeutic target or dosing regimen; 4) the selected outcome measures (e.g., ADAS-Cog) are not sufficiently relevant or sensitive to detect treatment responses in the patient population under study [5, 6]; and 5) the endpoints selected and implemented in clinical trials do not appropriately measure the outcomes with the greatest risk of degradation within the stage of illness targeted by the trial.
A recent shift in the focus of randomized clinical trials (RCTs) to early-stage disease (i.e., prodromal AD and mild AD) means the examination of hypothesis four and five is crucial. Such early-stage AD RCTs employing endpoints with maximal sensitivity/relevance in later AD stages could mischaracterize therapeutic benefit [7, 8]. To date, the fifth hypothesis has not been extensively examined, although recent work by Jutten et al. emphasized the need to select the right cognitive outcome measure to accurately evaluate treatment efficacy in clinical trials [9]. Jutten et al. proposed a framework that provides recommendations for the selection of cognitive assessments for clinical trials in early AD based on elements or criteria such as target population (i.e., clinical disease stage), relevance of domains/items, and measurement quality (i.e., appropriate psychometric properties) [9]. That is, when an outcome measure (e.g., ADAS-Cog) is only clinically relevant (i.e., domain/item content is targeted to the context of use) and sensitive in a specific clinical AD stage, treatment efficacy could be underestimated or overestimated if this outcome measure is used in other AD stages.
This manuscript is the second part in our line of research testing the fifth hypothesis. In the first part of this line of research we developed a theoretical Endpoint Staging Framework [10]. This published framework (see Fig. 1a) provides a roadmap for the selection of outcomes based on the stage of disease under investigation, thereby, aligning core outcome(s) (i.e., memory, orientation, activities of daily living or quality of life [QoL]) to the stage of disease in which these outcomes are most clinically relevant (i.e., the stage in which the outcomes start to degrade or are at greatest risk of degradation) [10, 11]. In application, the framework was intended to guide the selection of endpoints (e.g., cognitive tools) measuring the outcomes (i.e., memory) that are known to be the most clinically relevant in the target population. For example, performance of activities of daily living (ADLs) is known to be at greatest risk of degradation in mild-to-moderate AD; instrumental ADLs (i.e., finances) are sensitive to cognitive decline that occurs in early AD and basic ADLs (i.e., toileting) degrade in later stages [12, 13]. We argue that selecting endpoints employing this framework should maximize measurement sensitivity and estimated magnitudes of treatment efficacy, under the assumption that a true treatment effect exists. This is consistent with current Food and Drug Administration (FDA) guidance, whereby outcome measures must assess concepts that are relevant to the context of use (i.e., target population) to be considered fit-for-purpose [14–16]. The framework was developed from an extensive review of the literature on the natural history of symptom expression, and findings ratified by clinical experts [10].
Fig. 1
Endpoint Staging Framework and Endpoint-to-Target Stage Matrix.
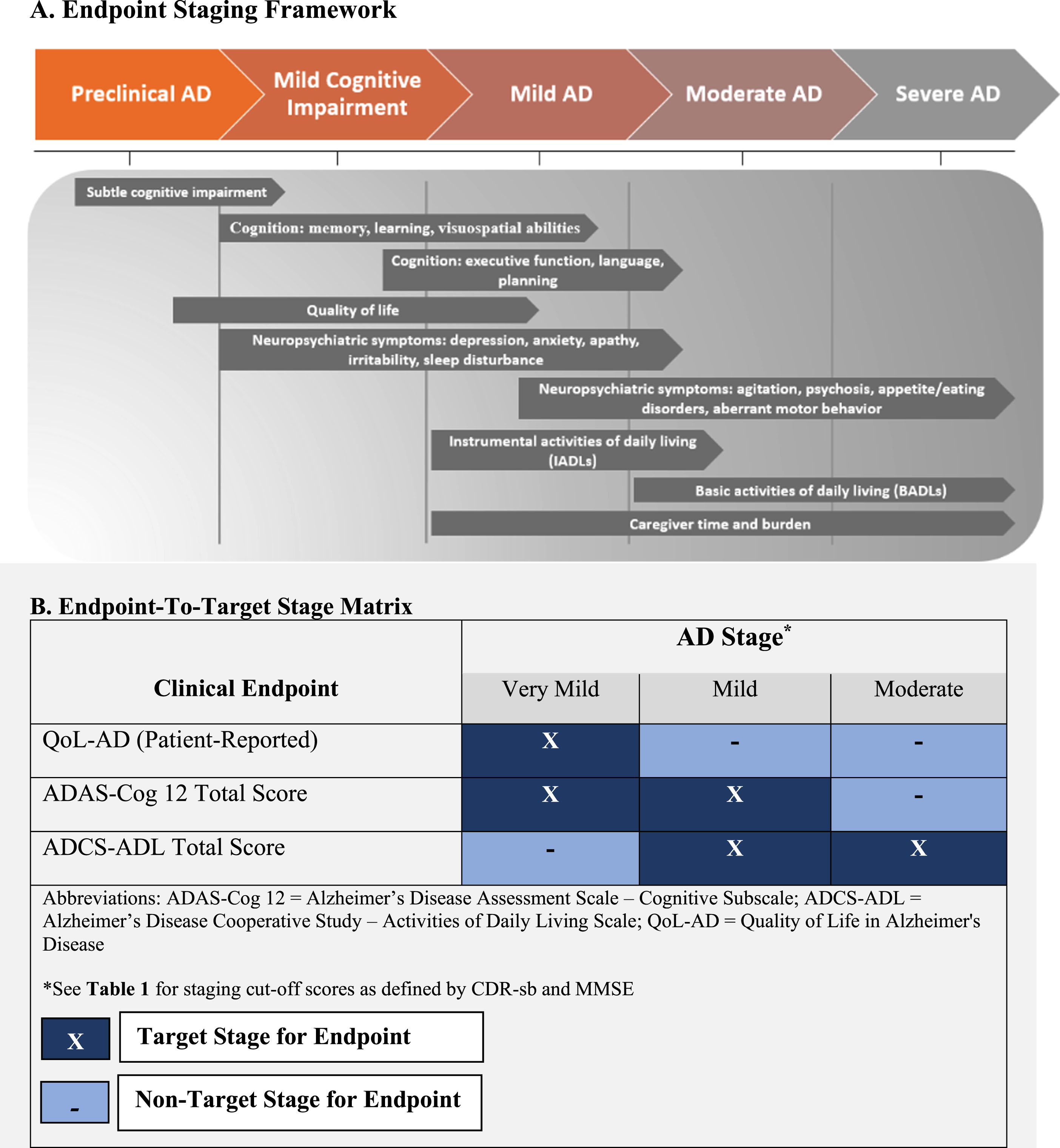
Here in the second part of our line of research we present an applied test of the theoretical Endpoint Staging Framework in a series of exploratory post-hoc analyses using data from the Alzheimer Management by Albumin Replacement (AMBAR) Phase 2b clinical trial [17]. Specifically, we examined whether testing treatment efficacy for endpoints measuring cognition, function, and QoL in the stages for which each endpoint was hypothesized to be most clinically relevant would yield estimates of treatment efficacy larger than those observed in the AMBAR full analysis set (FAS). The endpoints and disease stages (target versus non-target stages) defined to test the Endpoint Staging Framework are presented in Fig. 1b. The ‘target stage’ for each endpoint represents the stage of disease in which each of the endpoints are hypothesized to be most clinically relevant. For example, the ADCS-ADL (endpoint) was used to assess functional status (outcome). The ADCS-ADL accesses IADLs and BADLs and is both clinically relevant and sensitive to change in mild-to-moderate AD (Fig. 1a; [12, 18]). Accordingly, the ‘target stage’ for the ADCS-ADL endpoint was mild-to-moderate AD.
To clarify, the purpose of this manuscript was not to test efficacy or draw conclusions about any specific therapeutic benefit in AMBAR. The trial data was used to evaluate a proof of concept that AD endpoint target stages should theoretically be more sensitive than non-target stages. The manuscript was focused on evaluating a single question: if previously reported AMBAR efficacy evidence for the three endpoints considered here were re-estimated linking endpoint to corresponding clinically relevant AD stage, how would the previously reported results differ across target and non-target stages? We hypothesized that for all endpoints the magnitude of treatment efficacy would be greatest in the target stage, followed by the FAS, followed by the non-target stage, and that target and non-target stages would differ significantly in the observed magnitude of treatment efficacy. Should evidence support the conclusion that endpoints are more sensitive within a defined target stage then preliminary proof of the concept defined per the theoretical endpoint staging framework would be achieved [10]. Such a proof of concept would provide preliminary support for the implementation of the endpoint staging framework to improve prospective trial design.
MATERIALS AND METHODS
The AMBAR Trial Design
The AMBAR trial, described in detail elsewhere [19], is a phase 2b1 randomized, blinded, placebo-controlled clinical study (EudraCT#: 2011-001598-25; ClinicalTrials.gov ID: NCT01561053) to evaluate the efficacy and safety of plasma exchange with albumin (PE-A) in patients with mild-to-moderate AD.
Institutional Review Boards or Ethics Committees from the sites and the health authorities from both the United States and Spain approved the protocol. In Spain, this committee was part of the Research Center and Memory Clinic Fundació ACE, Institut Català de Neurociències Aplicades, and in the US this committee was part of the University of Pittsburgh Medical Center. A Data Safety Monitoring Committee met when approximately half of the patients were recruited due to the invasive nature of the study. When consenting to participate, the patient and a close relative or legal representative read the information sheet, agreed to participate in the trial, and signed the informed consent form.
Study population and intervention
A total of 347 eligible men and women aged 55 to 85 who had a probable diagnosis of AD dementia as defined by NINCDS-ADRDA criteria [20] and a baseline Mini-Mental State Examination (MMSE) score between 18 and 26, were enrolled. Twenty-five of the 347 randomized patients did not receive any planned treatment (18 in the PE-A treatment arms); therefore, these patients were excluded from the analysis.
Eligible patients were randomized into four arms (1 : 1:1 : 1): three PE-A treatment arms and one control (sham) arm (see Fig. 2), immunoglobulin (IVIG) infusion (10 g) every 4 months, or high dose albumin (40 g) alternated with IVIG infusion (20 g) every 4 months. The non-invasive (sham) procedure delivered to the placebo group consisted of a simulated PE to ensure blinding to treatment status. The total time from baseline to final visit was a maximum of 14 months.
Fig. 2
AMBAR Phase 2b Study Schema.
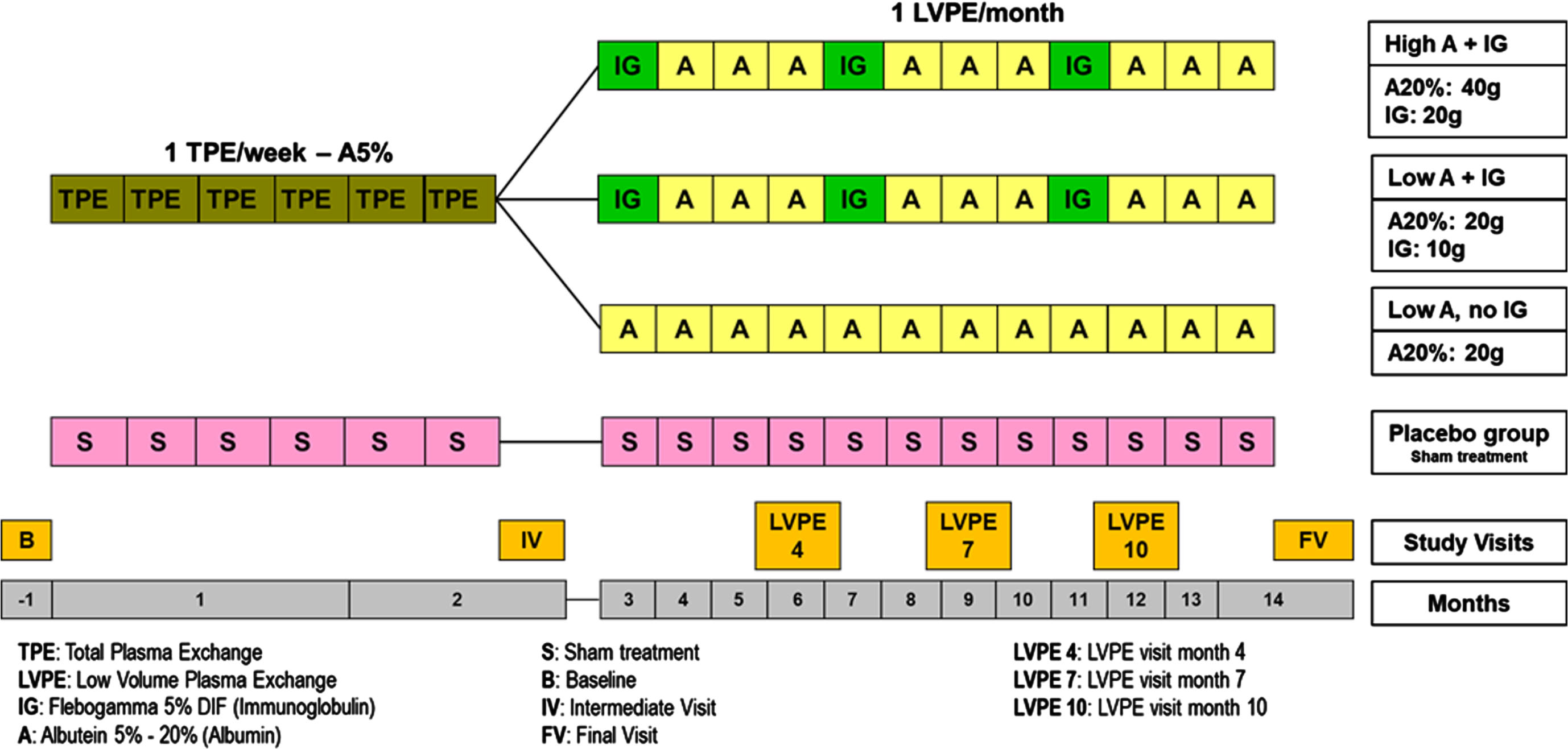
Outcome measures and endpoints
Co-primary endpoints were evaluated and included the Alzheimer’s Disease Assessment Scale-Cognitive Subscale (ADAS-Cog 12) and the Alzheimer’s Disease Cooperative Study–Activities of Daily Living (ADCS-ADL) total scores. In addition, the Quality of Life in Alzheimer’s Disease (QoL-AD), a secondary endpoint, was evaluated given the completeness of available data on this measure.
In these analyses, we examined stage-specific treatment efficacy on the total score change from baseline for the ADAS-Cog 12, the ADCS-ADL (composite of the IADLs and BADLs scores), and the QoL-AD as reported by the patient.
Disease stage definitions for post hoc analyses
For this proof-of-concept study, participant’s stage of disease at baseline (i.e., mild, moderate) was defined using a combination of MMSE and Clinical Dementia Rating – Sum of Boxes (CDR-sb) scores according to published cut-off values [21–23]. The cut-off values for these measures used to define AD severity at baseline are presented in Table 1.
Table 1
The MMSE and CDR-sb cut-off values used to define AD Severity
Measure | MCI/Very Mild | Mild AD | Moderate | Severe AD |
CDR-sb | 0.5–4.0 | 4.5–9.0 | 9.5–15.5 | 16.0–18.0 |
MMSE | ≥26 | 26–20 | 19–10 | ≤10 |
AD, Alzheimer’s disease; CDR-sb, Clinical Dementia Rating – Sum of Boxes; MCI, mild cognitive impairment; MMSE, Mini-Mental State Examination. Note, participants with ‘severe AD’ were not enrolled into the AMBAR trial; however, for completeness, the associated cut-off values are included here.
The MMSE is often used in clinical practice and research to determine dementia severity. There is empirical evidence to support the sensitivity of the MMSE in staging moderate AD; however, a growing body of evidence suggests the MMSE has both psychometric and diagnostic limitations in early stage disease (i.e., mild cognitive impairment (MCI) and mild AD) [24–27]. Specifically, the MMSE has been found to have large ceiling effects in MCI and mild dementia, thereby leading to greater misclassification of early-stage AD [25, 26]. Poor discrimination between MCI and mild AD has been demonstrated for the MMSE using data from clinical trials and observational studies [25, 28, 29]. In contrast, the CDR-sb has been found to accurately discriminate between cognitive impairment and early-stage disease, although it has been found to misclassify moderate disease [24, 30, 31].
Taken together, there is limited evidence to support the use of either instrument as a stand-alone tool to determine stages of AD across the continuum. While CDR-sb has been used as an endpoint in RCTs following the release of the FDAs 2013 guidance [32], the CDR global has also been used in RCTs for staging disease alongside the MMSE [33–35]. Therefore, in the absence of a ‘gold standard’ and to maximize stage classification accuracy using the available tools, cutoffs from both the MMSE and CDR-sb were used to define the target and non-target groups for these post-hoc analyses. To preserve classification accuracy of the target stages, subjects classified inconsistently across MMSE and CDR-sb were coded as members of the non-target stage for each endpoint.
Statistical analysis
Post-hoc analyses to test the proof of concept are described next. Note that AMBAR was not designed or powered to assess the proof of concept presented here; the powering of AMBAR has been described previously. Sample sizes reported and employed are those available in the data [17].
Per trial statistical analysis plan (SAP), the original AMBAR efficacy models differed by endpoint. The Mixed-Effect Model for Repeated Measures (MMRM) was used to analyze longitudinal change from baseline across months 2, 6, 9, 12, and 14 for ADAS-Cog 12 total and ADCS-ADL total scores.
The MMRM accounted for the within-subject correlation of the repeated measures via an unstructured covariance matrix and included fixed effects of treatment (pooled PE-A treated versus Placebo), month, and the treatment by month interaction. Analysis of covariance (ANCOVA) was used to model a single change from baseline for QoL-AD total score (change from baseline to month 14) from the fixed effect of treatment (pooled PE-A treated versus Placebo). Per SAP, both the MMRM and ANCOVA included covariates of age, the endpoint-specific baseline score, and MMSE-based AD-severity (Mild: baseline MMSE 26-22; Moderate: baseline MMSE 21-18).
Proof of concept models
To reiterate, the goal of this proof of concept was to evaluate whether the observed treatment efficacy in change from baseline to month 14 would have a larger magnitude in the endpoint target stage than that reported in the original FAS analysis or the FAS adjusting for stage (assessed descriptively) or in the non-target stage (assessed descriptively and inferentially). If reproducing the AMBAR analyses on AMBAR data accounting for the hypothesized stage effect produced numerically larger efficacy estimates, then the preliminary proof of concept would be achieved. The goal was not to make claims regarding PE-A treatment efficacy specifically, but to report and compare magnitudes of observed treatment efficacy estimates for the purpose of testing the theoretical Endpoint Staging Framework.
To test the proof of concept, the AMBAR efficacy models were augmented by including the endpoint-specific stage variables defined in Fig. 1a and 1b [10, 11]. For each outcome, reference cell coding was used to define the target stage (coded 1) and reference (i.e., non-target) stage (coded 0) within each endpoint-specific stage variable.
For the MMRM modeling, longitudinal change from baseline in ADAS-Cog and ADCS-ADL, the endpoint-specific stage variable was added as a main effect and interaction with treatment, month, and treatment by month. The marginal mean change from baseline to each follow-up month within each treatment arm and the corresponding difference between treatment arms were estimated for the FAS adjusting for stage, the target stage and non-target stage. In addition, the difference in treatment efficacy between target and non-target stages was tested. Each of these contrasts were estimated as marginal means and marginal mean differences using SAS’ LSMESTIMATE function within the MIXED Procedure. This approach permitted evaluating the hypothesized effect of stage on the difference between treatment arms across month without the sample-size-reducing effect of stratification.
For the ANCOVA modeling, change from baseline to month 14 in QoL-AD the endpoint-specific stage variable was added as a main effect and interaction with treatment. The treatment efficacy within and the difference in efficacy between target and non-target stages was tested. Each of these contrasts were estimated as marginal means and marginal mean differences using SAS’ LSMESTIMATE function within the GLM Procedure.
Imbalance and missing data
Consistent with previous AMBAR evidence reporting, observed data were analyzed and no imputation procedure was used for missing data. Missing data were handled by the properties of the MMRM. The MMRM yields unbiased estimates in the presence of unbalanced data (e.g., the 3 : 1 PE-A treated to placebo allocation in AMBAR) and missing data under the assumption data are missing at random (MAR). A sensitivity analysis used the joint model to adjust estimates for potential bias arising from data missing not at random (MNAR). The joint model yields unbiased estimates under both MAR and MNAR by adjusting the MMRM for subject-specific study survival time [36].
All analyses were conducted in SAS Version 9.4 except the joint model sensitivity analysis which was conducted in R version 4.3.2 using the JM package.
RESULTS
Baseline characteristics
The average age of the study population (Table 2) was 69.0 years, consistent across pooled treatment and placebo arms (69.2 versus 68.4). The percentage of males was greater in the placebo arm (55%) compared to the pooled treatment arm (43%). The percentage of apolipoprotein E (APOE ɛ4) carriers was marginally greater for pooled treatment compared with the placebo arm (51.9% versus 44.2%). The mean time from diagnosis to enrolment was 2.4 years.
Table 2
Baseline demographics and clinical characteristics in AMBAR Trial
Characteristics | Placebo (n = 80) | Pooled PE-A Treated (n = 242) | Total (n = 322) |
Age (y), mean (SD) | 68.4 (8.4) | 69.2 (7.4) | 69.0 (7.7) |
Age group (n, %) | |||
<65 | 29 (36.3) | 65 (26.9) | 94 (29.2) |
65–75 | 33 (41.3) | 124 (51.2) | 157 (48.8) |
>75 | 18 (22.5) | 53 (21.9) | 71 (22.0) |
Sex (n, %) | |||
Male | 44 (55.0) | 104 (43.0) | 148 (46.0) |
Female | 36 (45.0) | 138 (57.0) | 174 (54.0) |
Time since AD diagnosis (y), mean (SD) | 2.5 (2.3) | 2.4 (2.4) | 2.4 (2.4) |
MMSE score, mean (SD) | 21.7 (2.6) | 21.6 (2.6) | 21.6 (2.6) |
CDR-sb score, mean (SD) | 5.1 (2.6) | 4.5 (2.1) | 4.6 (2.2) |
AD medication (n, %) | |||
CEIs | 56 (70.0) | 164 (67.7) | 220 (68.3) |
Memantine | 7 (8.8) | 36 (14.9) | 43 (13.4) |
CEIs + Memantine | 16 (20.0) | 40 (16.5) | 56 (17.4) |
None | 1 (1.3) | 2 (0.8) | 3 (0.9) |
APOE ɛ4 Status | |||
N | 77 | 231 | 308 |
Carriers (n, %) | 34 (44.2) | 120 (51.9) | 154 (50.0) |
Non-carriers (n, %) | 43 (55.8) | 111 (49.1) | 154 (50.0) |
CSF Aβ42 | |||
N | 71 | 226 | 297 |
pg/mL, median (IQR) | 551 (380–810) | 505 (431–700) | 515 (426–737) |
Abbreviations: AD, Alzheimer’s disease; BMI, body mass index; CEI, cholinesterase inhibitor; CSF, cerebrospinal fluid; IQR, Interquartile range; IVIG, intravenous immunoglobulin; MMSE, Mini-Mental State Examination; PE-A, plasma exchange with albumin replacement; SD, standard deviation.
Post-hoc analyses of endpoints
Full model results are presented in Supplementary Tables 1 and 2. For the sake of parsimony and consistency of reporting across all endpoints, only marginal means and mean contrasts for change from baseline to final visit (month 14) are reported. These are reported for the FAS adjusting for stage, within the target stage, within the non-target stage, and for the difference between target and non-target stages. Within Tables 4 and 5, results presented were obtained from a single model. The estimates for the FAS adjusting for stage (1a–1c) were derived from the two-way interaction between treatment and month; the target stage estimates (2a–2c) were obtained from the three-way interaction between stage, treatment and month, slicing on target stage; the non-target stage estimates (3a–3c) were obtained from the three-way interaction between stage, treatment and month, slicing on non-target stage; the difference in efficacy between target and non-target stage (4a) was obtained as the difference in stage-sliced three way interactions (2c minus 3c). The same was true for the ANCOVA presented in Table 6, except there was no effect of month and therefore no three-way interaction because the ANCOVA analyzed only a single change from baseline value (baseline to month 14).
Cognitive function
Original analysis in FAS
In the original FAS analysis, the ADAS-Cog 12 change from baseline to final visit (month 14) marginal mean was 2.8 for placebo and 1.2 for PE-A treatment. The difference between PE-A treatment and placebo groups in ADAS-Cog 12 total score change from baseline to final visit (month 14) was not statistically significant (–2.1 [95% CI: –4.4, 0.2], p = 0.063) [17].
Proof of concept model
Within the FAS adjusting for stage (target versus non-target), the PE-A treatment efficacy was statistically significant (–2.8, p = 0.0330; see Table 4 and Fig. 3). Treatment efficacy for ADAS-Cog 12 total score change from baseline to final visit (month 14) was not statistically significant in the target stage (mild AD; –1.6, p = 0.2284) nor in the non-target stage (moderate AD; –4.0, p = 0.0760). PE-A treatment efficacy did not significantly differ between the target and non-target stages (2.5, p = 0.3456; see Table 4 and Fig. 3). Note, the corresponding sample sizes for this analysis are presented in Table 3.
Fig. 3
ADAS-Cog 12 Total Score: Treatment Effect in FAS, Target, and Non-Target Stages.
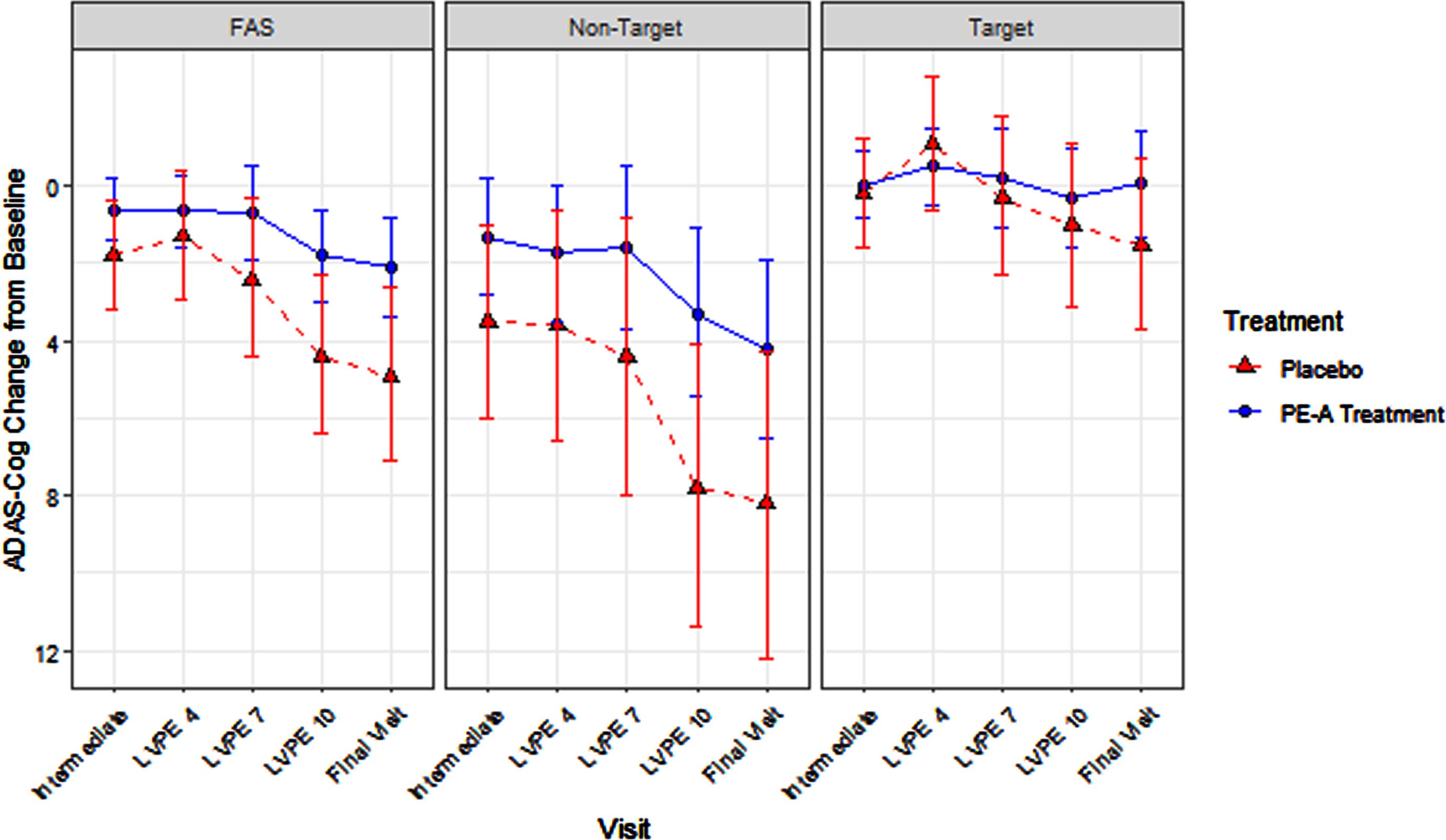
Table 3
Sample size for each post-hoc analysis, by endpoint and target and non-target stage
ADAS-Cog 12 | ADCS-ADL | QOL-AD1 | ||||
Visit | Non-Target | Target | Non-Target | Target | Non-Target | Target |
Intermediate Visit (Month 2) | 62 | 161 | 62 | 161 | – | – |
LVPE 4 (Month 6) | 56 | 144 | 55 | 144 | – | – |
LVPE 7 (Month 9) | 51 | 134 | 51 | 134 | – | – |
LVPE 10 (Month 12) | 48 | 126 | 48 | 126 | – | – |
Final Visit (Month 14) | 44 | 121 | 44 | 122 | 79 | 78 |
1Note that QOL-AD, in contrast to ADAS-Cog 12 and ADCS-ADL was modeled, per SAP/CSR as only change from baseline to final visit via ANCOVA. Therefore, there are no sample sizes reported for change from baseline to intermediate visit through LVPE 10.
Table 4
Marginal Mean-Based Effects for Cognition at Final Visit (ADAS-Cog 12)
EFFECT | LSM | 95% CI | p |
FAS Adjusted for Stage: Treatment Efficacy at Final Visit (Month 14) | |||
1a. PBO Change from Baseline | 4.9 | 2.6, 7.1 | <0.0001 |
1b. POOLED TX Change from Baseline | 2.1 | 0.8, 3.4 | 0.0020 |
1c. Treatment Efficacy (1b–1a) | –2.8 | –5.4, –0.2 | 0.0330 |
Target Stage: Treatment Efficacy at Final Visit (Month 14) | |||
2a. PBO Change from Baseline | 1.5 | –0.7, 3.7 | 0.1758 |
2b. POOLED TX Change from Baseline | –0.1 | –1.4, 1.3 | 0.9400 |
2c. Treatment Efficacy (2b–2a) | –1.6 | –4.1, 1.0 | 0.2284 |
Non-Target Stage Treatment Efficacy at Final Visit (Month 14) | |||
3a. PBO Change from Baseline | 8.2 | 4.3, 12.2 | 0.0001 |
3b. POOLED TX Change from Baseline | 4.2 | 1.9, 6.5 | 0.0004 |
3c. Treatment Efficacy (3b–3a) | –4.0 | –8.5, 0.4 | 0.0760 |
Difference Between Target and Non-Target Stage Treatment Efficacy at Final Visit (Month 14) | |||
4a. Treatment Efficacy Difference Across Endpoint Staging (2c–3c) | 2.5 | –2.7, 7.6 | 0.3456 |
TX, treatment; PBO, placebo; LSM, least squares means; 95% CI, 95% confidence limit; FAS, full analysis set.
Table 5
Marginal Mean-Based Effects for Functional Status at Final Visit (ADCS-ADL)
EFFECT | LSM | 95% CI | p |
FAS Adjusted for Stage: Treatment Efficacy at Final Visit (Month 14) | |||
1a. PBO Change from Baseline | –12.7 | –16.3, –9.0 | <0.0001 |
1b. POOLED TX Change from Baseline | –4.8 | –6.9, –2.7 | <0.0001 |
1c. Treatment Efficacy (1b–1a) | 7.9 | 3.7, 12.1 | 0.0002 |
Target Stage: Treatment Efficacy at Final Visit (Month 14) | |||
2a. PBO Change from Baseline | –21.6 | –28.3, –14.8 | <0.0001 |
2b. POOLED TX Change from Baseline | –7.4 | –11.2, –3.6 | 0.0002 |
2c. Treatment Efficacy (2b–2a) | 14.2 | 6.6, 21.8 | 0.0003 |
Non-Target Stage Treatment Efficacy at Final Visit (Month 14) | |||
3a. PBO Change from Baseline | –3.8 | –6.7, –0.9 | 0.0115 |
3b. POOLED TX Change from Baseline | –2.2 | –4.0, –0.4 | 0.0191 |
3c. Treatment Efficacy (3b–3a) | 1.6 | –1.8, 5.1 | 0.3519 |
Difference Between Target and Non-Target Stage Treatment Efficacy at Final Visit (Month 14) | |||
4a. Treatment Efficacy Difference Across Endpoint Staging (2c–3c) | 12.6 | 4.2, 20.9 | 0.0033 |
TX, treatment; PBO, placebo; LSM, least squares means; 95% CI, 95% confidence limit; FAS, full analysis set.
Inconsistent with the endpoint staging hypothesis, the magnitude of treatment efficacy was largest in the non-target stage (–4.0 [non-target]> –2.8 [FAS adjusting for stage]> –2.1 [original FAS]> –1.6 [target]), this is explored in the discussion and is hypothesized to be related to the item content and sensitivity of the ADAS-Cog 12.
Activities of daily living
Original analysis in FAS
In the original FAS analysis, the ADCS-ADL change from baseline to final visit (month 14) marginal mean was –6.7 for placebo and –3.2 for PE-A treatment. Treatment efficacy for the ADCS-ADL total score change from baseline to final visit (month 14) was statistically significant (mean difference: 3.5, p = 0.030), reflecting greater preservation of functional ability for PE-A treated patients compared to those receiving placebo [17].
Proof of concept model
PE-A treatment efficacy for the ADCS-ADL total score within the FAS adjusting for stage (target versus non-target) was statistically significant (7.9, p = 0.0002). The same was true in the target stage (mild-to-moderate AD; 14.2, p = 0.0003) but not in the non-target stage (very mild AD; 1.6, p = 0.3519). The difference in efficacy significantly differed between the target and non-target stages, with statistically significant superior preservation of function in the target stage (12.6, p = 0.0033; see Table 5 and Fig. 4) compared to the non-target stage. Note, the corresponding sample sizes for this analysis are presented in Table 3.
Fig. 4
ADCS-ADL Total Score: Treatment Effect in FAS, Target, and Non-Target Stages.
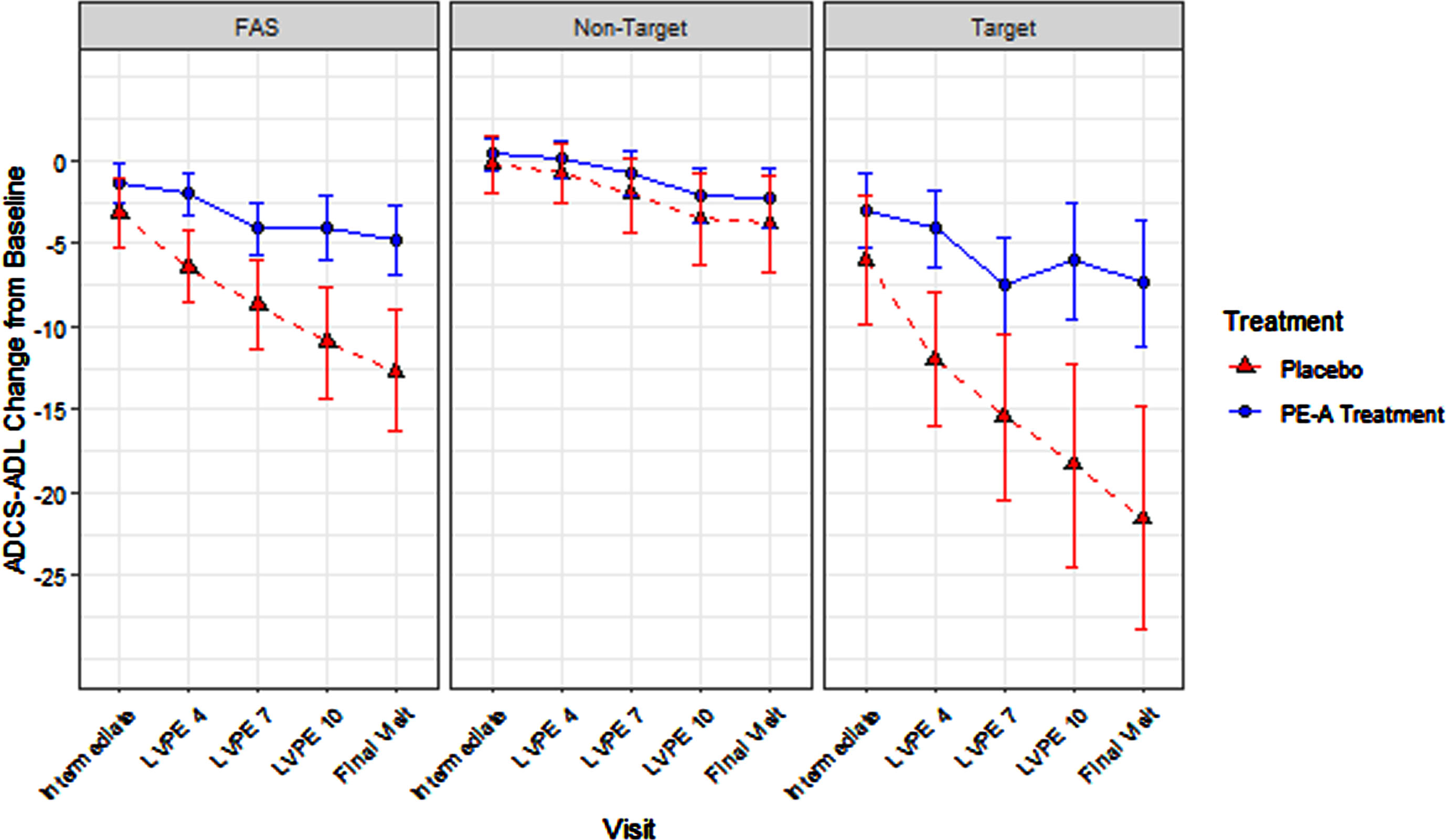
Consistent with the endpoint staging framework hypothesis, the magnitude of treatment efficacy was largest in the target stage (14.2 [target] > 7.9 [FAS adjusting for stage] > 3.5 [original FAS] > 1.6 [non-target]).
Quality of life
Original analysis in FAS
In the original FAS analysis, the QoL-AD change from baseline to final visit (month 14) marginal mean was 0.2 for placebo and 1.5 for PE-A treatment. Treatment efficacy for the QoL-AD total score change from baseline to final visit (month 14) was statistically significant (mean difference: 1.4, p = 0.024), indicating greater preservation of QoL in AD for patients receiving PE-A treatment compared with patients receiving placebo [37].
Proof of concept model
PE-A treatment efficacy for patient-reported QoL in the FAS adjusting for stage (target versus non-target) was statistically significant (1.5, p < 0.0001). Within the target stage (very mild AD) treatment efficacy was statistically significant (2.4, p < 0.0001) and was numerically smaller but significant (0.6, p = 0.0001) in the non-target stage (mild-to-moderate AD). The magnitude of the difference in treatment efficacy between target and non-target stages was significant (1.8, p < 0.0001; See Table 6 and Fig. 5). Additionally, in the target stage, the proportion of patients achieving the threshold for minimally important difference (MID, 3-point change [38–42]) was 39% for the PE-A treatment group and 20% for the placebo group. Note, the corresponding sample sizes for this analysis are presented in Table 3.
Table 6
Marginal Mean-Based Effects for Patient-Reported QoL at Final Visit (QoL-AD)
EFFECT | LSM | 95% CI | p |
FAS Adjusted for Stage: Treatment Efficacy at Final Visit (Month 14) | |||
1a. PBO Change from Baseline | 0.1 | –0.1, 0.3 | 0.4140 |
1b. POOLED TX Change from Baseline | 1.5 | 1.4, 1.7 | <0.0001 |
1c. Treatment Efficacy (1b–1a) | 1.5 | 1.3, 1.7 | <0.0001 |
Target Stage: Treatment Efficacy at Final Visit (Month 14) | |||
2a. PBO Change from Baseline | –0.6 | –0.8, –0.3 | 0.0001 |
2b. POOLED TX Change from Baseline | 1.8 | 1.6, 2.0 | <0.0001 |
2c. Treatment Efficacy (2b–2a) | 2.4 | 2.0, 2.7 | <0.0001 |
Non-Target Stage Treatment Efficacy at Final Visit (Month 14) | |||
3a. PBO Change from Baseline | 0.7 | 0.5, 1.0 | <0.0001 |
3b. POOLED TX Change from Baseline | 1.3 | 1.1, 1.5 | <0.0001 |
3c. Treatment Efficacy (3b–3a) | 0.6 | 0.3, 0.9 | 0.0001 |
Difference Between Target and Non-Target Stage Treatment Efficacy at Final Visit (Month 14) | |||
4a. Treatment Efficacy Difference Across Endpoint Staging (2c–3c) | 1.8 | 1.4, 2.2 | <0.0001 |
TX, treatment; PBO, placebo; LSM, least squares means; 95% CI, 95% confidence limit; FAS, full analysis set.
Fig. 5
QoL-AD: Treatment Effect in FAS, Target, and Non-Target Stages.
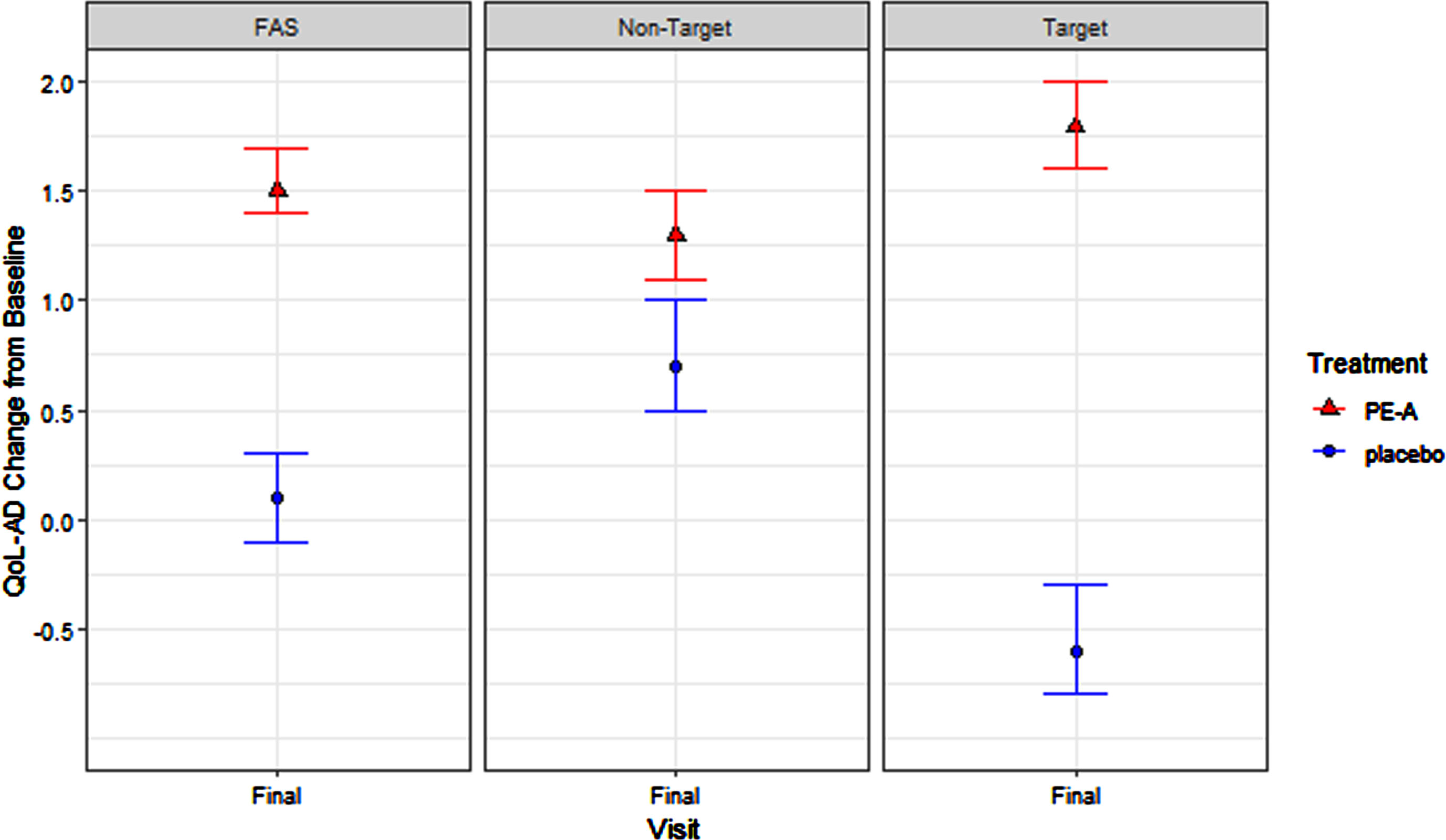
Consistent with the endpoint staging framework hypothesis, the magnitude of treatment efficacy was largest in the target stage (2.4 [target] > 1.5 [FAS adjusting for stage] > 1.4 [original FAS] > 0.6 [non-target]).
DISCUSSION
In this proof of concept, we conducted a series of post-hoc analyses using data from the AMBAR trial to test the hypothesis that optimal endpoints for measuring the benefits of treatment depend upon the stage of illness of the participants enrolled in the study. Thus, we posited that the accurate detection of a treatment effect and the magnitude of that effect strongly depends on aligning a measured endpoint with the appropriate stage of disease. Specifically, treatment efficacy was hypothesized to be maximized when using endpoints known to be clinically relevant within a given stage. Clinical relevance was defined as endpoints measuring outcomes at greatest risk of degradation within the AD stage of interest.
While we were limited to the endpoints and AD stages available within the AMBAR trial, the findings from these exploratory analyses offer preliminary proof of concept for the endpoint staging framework broadly.
The primary analysis on the FAS in AMBAR demonstrated statistically significant PE-A efficacy on QoL-AD and ADCS-ADL but not for ADAS-Cog 12 [17, 37].
In proof-of-concept models testing the endpoint staging framework, both the QoL-AD and the ADCS-ADL total score retained the significant treatment efficacy observed in the FAS. In addition, both performed as hypothesized, with the numerically largest and statistically significant treatment efficacy estimates observed in the target stage. Results for the ADAS-Cog 12 total score did not follow this pattern. That is, the numerically largest and statistically significant PE-A efficacy estimates were not observed within the target stage, and no difference was found in efficacy between the target and non-target stages. Given this finding, we propose that there are other potential contributors to effect suppression in cognition that warrant discussion.
The findings from this study suggest that two forms of heterogeneity could diminish detection of a true clinical benefit in AD trials, and that alternative study design solutions should be considered to address these forms of heterogeneity. One approach depends upon stage and matching the measures and endpoints to the most relevant stage of disease. The second approach depends upon the refinement of the measurement of endpoints. In this manuscript, we endeavored to address the effect of stage-dependent heterogeneity and the matching of endpoints to stages on the detection of efficacy for AD treatments. For measure-dependent heterogeneity, the refinement of measures may be required, as all tests of cognition are not equal within and across stage.
We know cognition encompasses various domains each exhibiting differential accelerated decline depending on AD stage (pre-clinical, MCI, mild AD, moderate AD, and severe AD) [43–45]. Recent evaluations have demonstrated increased accuracy in characterizing decline in preclinical to prodromal AD using alternative more targeted cognitive measures, such as the Free and Cued Selective Reminding test or Controlled Oral Word Association Test [46–48]. In contrast, the ADAS-Cog 12, widely used in clinical development programs regardless of stage, was developed to measure facets of cognition relevant to overt dementia where cognitive impairments are more severe. The potential misalignment between this endpoint and early stage AD is somewhat demonstrated by psychometric limitations such as significant floor effects in some items (i.e., delayed word recall; 10 errors) in mild AD [49]. Consequently, a cognition endpoint measuring cognition facets at highest risk of degradation in moderate AD, like the ADAS-Cog, may fail to detect treatment efficacy in early AD stages. This could offer some explanation to why the largest observed magnitude of treatment efficacy was seen in the non-target (moderate AD) stage in this analysis of the ADAS-Cog 12. Employing cognitive tools that robustly assess relevant facets of cognition (i.e., executive function) that start to degrade in the earlier disease stages compared with traditional tools, such as the ADAS-Cog, are likely to provide greater sensitivity to treatment effects in confirmatory trials of early-stage disease.
There have been tremendous advances in the use of neuroimaging and biomarkers as eligibility criteria and outcomes in AD trials, as well as for general clinical practice [50–53]. However, these advances have not been matched by advances in the measures of objective or subjective cognitive status and decline, or in measures of functional status, QoL, or neuropsychiatric symptoms. In many ways, the relevance and measurement issues presented here could be considered as consistent with recent clinical findings that demonstrated AD stage progression is associated with distinct elements and specific expressions of tau isoforms, and these stage-specific expressions have been proposed as stage-specific interventional targets [54]. However, while several drugs have demonstrated the clearance of amyloid from the brain, evidence of a corresponding slowing of cognitive and functional decline has not been robust [55].
The findings from this proof of concept support recent published work that concludes there is a need for the field to critically appraise the selection of outcome measures and endpoints for trials based on consensus-driven criteria or frameworks [9]. Here, we present preliminary support for the endpoint staging framework, that we hope can be used to aid in the selection of optimal outcomes, outcome measures, and endpoints for future AD trials.
Limitations
While these findings provide preliminary proof of concept, there are several limitations to this work. We conducted post-hoc analyses of a relatively small study that did not use biomarkers as part of the inclusion criteria. A prospective study designed to evaluate this hypothesis would require collection of multiple endpoints (cognition, function, QoL, emotional & mental health) in each disease stage (preclinical/MCI, mild, moderate) and sufficient powering of the study to detect any differences in change by endpoint and stage.
Second, several variables were not included as covariates (i.e., amyloid status, APOE status) in the primary AMBAR analysis, and, therefore, were not available for inclusion in these post-hoc analyses. These variables included APOE ɛ4 status (carrier versus non-carrier), amyloid status (positive versus negative), treatment received prior to enrolment, comorbidities (e.g., diabetes), and level of education. These variables are key contributors to observed heterogeneity in disease progression, as well as within-subject and between-subject variability in performance on outcome measures. While this specific limitation goes beyond the scope of the guidance provided by the Endpoint Staging Framework, it is important that these factors be considered for future stage-specific evaluation of outcomes in AD.
Subject loss-to-follow-up was a consideration in this analysis. Study attrition in clinical research and its potential corresponding biasing effect on estimates and inference remains a pervasive and vexing issue. AD RCTs are no exception, and neither was AMBAR. The rate of loss-to-follow-up within AMBAR was within the range commonly observed within AD trials: 20–30% [56]. While the MMRMs employed for the ADAS-Cog 12 and ADCS-ADL endpoints implicitly adjust for data missing at random (MAR), they are insufficient to adjust for informative missingness mechanisms broadly referred to as data missing not at random (MNAR). Survivor bias is a general example of the issues that can arise from MNAR data. A hypothetical example illustrating survivor bias would consist of a scenario wherein a subject experiences a dramatic deterioration in their AD status and can no longer participate in the study. Their departure from the study leaves the endpoint values preceding this deterioration event and omits the value that would have been observed had they remained. The unobserved endpoint value would have reflected a dramatic change in endpoint and could, depending on the severity of the endpoint deterioration, alter the analysis outcome.
To examine the potential biasing effect associated with AMBAR’s loss-to-follow-up, we conducted a sensitivity analysis using the joint model. The joint model simultaneously models the MMRM and the time to study drop-out for the analysis sample. The joint model links the MMRM to study survival via shared random effects, thereby adjusting for phenomena such as survivor bias [36]. The Cox model component of the joint model predicted time to study drop-out from planned treatment, age at baseline, endpoint baseline value, baseline AD severity, and AD target and non-target stage. None of these effects were significant predictors of time to study drop-out. Within the target stage, the joint model sensitivity analysis for the ADCS-ADL endpoint yielded a MAR- and MNAR-adjusted month 14 efficacy estimate of 13.71 (compared to 14.2 in the original model) and the associated p-value was <0.0001. Therefore, subject loss-to-follow-up was found to not bias the model results, regardless of mechanism (MAR or MNAR). A joint model was not fit for the ADAS-Cog 12 given the null results.
Note that because the original QoL-AD model replicated in this analysis employed an ANCOVA (as was the case for all non-primary endpoints), a joint model or pattern mixture model could not be used to examine the potential effect of MNAR data on the QoL-AD analysis. Given the pernicious nature of missing data and loss-to-follow-up in AD trials, it would behoove researchers to employ longitudinal models for all endpoints where possible, to permit testing the potential effect of MNAR bias on reported results. Finally, the authors note that this manuscript is not intended to promote the use of the methods employed here but rather to demonstrate what we believe would be observed if the Endpoint Staging Framework were used to prospectively link endpoints to the target stage of disease in an RCT. While such interaction terms could be employed in stage-heterogeneous trials, that is not the point of this manuscript. The manuscript is solely intended to provide a preliminary proof of concept that AD endpoints should be tailored to their context of use (here, stage) to maximize observed therapeutic benefit. Prospective trial design should carefully consider what AD stage is relevant for the clinical program, what stage-conversions may be observed post-baseline as a function of study duration, and then select the endpoints that are most relevant to these stages.
Conclusions
Taken together the findings from these exploratory post-hoc analyses provide preliminary support for the theoretical hypothesis that the optimal endpoints for measuring the benefits of treatment depend upon the stage of illness of the participants enrolled in the study. As hypothesized, the magnitude of the observed treatment efficacy in the function and QoL outcomes was numerically largest compared to all other estimates when evaluated in the most relevant stage of AD. From this, we posit that a failure to optimize the selection and evaluation of endpoints to measure clinical benefit in the stage of illness under study is one reason for the limited success of previous AD trials. We conclude that preliminary evidence supporting the use of the Endpoint Staging Framework to optimize outcome assessment in trials may exist, but that substantial additional work is needed.
AUTHOR CONTRIBUTIONS
Lauren Podger (Conceptualization; Formal analysis; Investigation; Methodology; Writing – original draft; Writing – review & editing); Walter F. Stewart (Conceptualization; Methodology; Writing – original draft; Writing – review & editing); Daniel Serrano (Conceptualization; Formal analysis; Methodology; Writing – original draft; Writing – review & editing); Richard B. Lipton (Conceptualization; Methodology; Writing – original draft; Writing – review & editing); David Gomez-Ulloa (Conceptualization; Methodology; Writing – original draft; Writing – review & editing); Nicolai D Ayasse (Formal analysis; Writing – original draft); Frederick B. Barnes (Methodology; Project administration; Writing – original draft; Writing – review & editing); E. Anne Davis (Conceptualization; Methodology; Writing – original draft; Writing – review & editing); M. Chris Runken (Conceptualization; Methodology; Writing – original draft; Writing – review & editing).
ACKNOWLEDGMENTS
We thank all the patients with Alzheimer’s disease and their families and caregivers who participated in the AMBAR trial, as well as all trial personnel, for their contribution.
The authors are indebted to the reviewers for their assistance, especially for the constructive dialogue on missing data.
The authors are indebted to Shauna McManus, MPH, who produced the figures for this manuscript.
The authors are indebted to Jack Wakefield, MSc, who assisted in reference formatting and editorial support.
FUNDING
This study and the development of this manuscript were sponsored by Grifols SSNA.
CONFLICT OF INTEREST
Richard B. Lipton, MD, serves on the editorial boards of Neurology and Cephalalgia and is a senior advisor for Headache. He has received research support from the National Institutes of Health. He also receives support from the Migraine Research Foundation and the National Headache Foundation. He has reviewed for the National Institute on Aging and National Institute of Neurological Disorders and Stroke; serves as consultant, advisory board member, or has received honoraria or research support from AbbVie, Amgen, Biohaven, Dr. Reddy’s Laboratories, electroCore, Eli Lilly, eNeura Therapeutics, GlaxoSmithKline, Merck, Novartis, Teva, Vector, and Vedanta Research. He receives royalties from Wolff’s Headache, 8th edition (Oxford University Press, 2009), and Informa. He holds stock options in Biohaven and Ctrl M. Walter F. Stewart, PhD, serves as a consultant for Grifols and Amgen. Lauren Podger, MSc is an employee of OPEN Health Group. Daniel Serrano, PhD, and Nicolai D Ayasse, PhD, and Frederick B. Barnes, BSc are former employees of OPEN Health Group. Michael C. Runken, PharmD, and David Gomez-Ulloa, PharmD, are employees of Grifols. E Anne Davis, PharmD, is a former employee of Grifols.
DATA AVAILABILITY
The dataset analyzed in this study is the property of Instituto Grifols S.A. Data availability would occur at the discretion of Instituto Grifols S.A.
SUPPLEMENTARY MATERIAL
{ label (or @symbol) needed for fn } The supplementary material is available in the electronic version of this article: https://dx.doi.org/10.3233/JAD-231197.
REFERENCES
[1] | Anderson RM , Hadjichrysanthou C , Evans S , Wong MM ((2017) ) Why do so many clinical trials of therapies for Alzheimer’s disease fail? Lancet 390: , 2327–2329. |
[2] | Atri A ((2019) ) Current and future treatments in Alzheimer’s disease. Semin Neurol 39: , 227–240. |
[3] | Posner H , Curiel R , Edgar C , Hendrix S , Liu E , Loewenstein DA , Morrison G , Shinobu L , Wesnes K , Harvey PD ((2017) ) Outcomes assessment in clinical trials of Alzheimer’s disease and its precursors: Readying for short-term and long-term clinical trial needs. Innov Clin Neurosci 14: , 22–29. |
[4] | Knopman DS , Jones DT , Greicius MD ((2021) ) Failure to demonstrate efficacy of aducanumab: An analysis of the EMERGE and ENGAGE trials as reported by Biogen, December 2019. Alzheimers Dement 17: , 696–701. |
[5] | Gauthier S , Albert M , Fox N , Goedert M , Kivipelto M , Mestre-Ferrandiz J , Middleton LT ((2016) ) Why has therapy development for dementia failed in the last two decades? Alzheimers Dement 12: , 60–64. |
[6] | Mangialasche F , Solomon A , Winblad B , Mecocci P , Kivipelto M ((2010) ) Alzheimer’s disease: Clinical trials and drug development. Lancet Neurol 9: , 702–716. |
[7] | Cano SJ , Posner HB , Moline ML , Hurt SW , Swartz J , Hsu T , Hobart JC ((2010) ) The ADAS-cog in Alzheimer’s disease clinical trials: Psychometric evaluation of the sum and its parts. J Neurol Neurosurg Psychiatry 81: , 1363–1368. |
[8] | Wessels AM , Tariot PN , Zimmer JA , Selzler KJ , Bragg SM , Andersen SW , Landry J , Krull JH , Downing AM , Willis BA , Shcherbinin S , Mullen J , Barker P , Schumi J , Shering C , Matthews BR , Stern RA , Vellas B , Cohen S , MacSweeney E , Boada M , Sims JR ((2020) ) Efficacy and safety of lanabecestat for treatment of early and mild Alzheimer disease: The AMARANTH and DAYBREAK-ALZ randomized clinical trials. JAMA Neurol 77: , 199–209. |
[9] | Jutten RJ , Papp KV , Hendrix S , Ellison N , Langbaum JB , Donohue MC , Hassenstab J , Maruff P , Rentz DM , Harrison J , Cummings J , Scheltens P , Sikkes SAM ((2023) ) Why a clinical trial is as good as its outcome measure: A framework for the selection and use of cognitive outcome measures for clinical trials of Alzheimer’s disease. Alzheimers Dement 19: , 708–720. |
[10] | Lipton RB , Podger L , Stewart WF , Gomez-Ulloa D , Rodriguez WI , Runken MC , Barnes FB , Serrano D ((2022) ) Toward the optimized assessment of clinical outcomes in studies of novel treatments for Alzheimer’s disease. Expert Rev Neurother 22: , 863–873. |
[11] | Lipton R SW , Gomez-Ulloa D , Runken M , Barcelo M , Ayasse N , Serrano D ((2022) ) Effect of plasma exchange with albumin replacement on cognition in Alzheimer’s disease: A latent growth mixture model. Eur J Neurol 29: , 375–376. |
[12] | Galasko D , Bennett D , Sano M , Ernesto C , Thomas R , Grundman M , Ferris S ((1997) ) An inventory to assess activities of daily living for clinical trials in Alzheimer’s disease. The Alzheimer’s Disease Cooperative Study. Alzheimer Dis Assoc Disord 11: (Suppl 2), S33–39. |
[13] | Cahn-Weiner DA , Farias ST , Julian L , Harvey DJ , Kramer JH , Reed BR , Mungas D , Wetzel M , Chui H ((2007) ) Cognitive and neuroimaging predictors of instrumental activities of daily living. J Int Neuropsychol Soc 13: , 747–757. |
[14] | Food and Drug Administration, Early Alzheimer’s Disease: Developing Drugs for Treatment Guidance for Industry, https://www.fda.gov/media/110903/download, Accessed January 10, 2024. |
[15] | Food and Drug Administration, Patient-Focused Drug Development: Selecting, Developing, or Modifying Fit-for-Purpose Clinical Outcome Assessments Guidance for Industry, Food and Drug Administration Staff, and Other Stakeholders, https://www.fda.gov/media/159500/download, Accessed January 10, 2024. |
[16] | Food and Drug Administration, Patient-Focused Drug Development: Incorporating Clinical Outcome Assessments Into Endpoints for Regulatory Decision-Making Guidance for Industry, Food and Drug Administration Staff, and Other Stakeholders, https://www.fda.gov/media/166830/download, Accessed January 10, 2024. |
[17] | Boada M , López OL , Olazarán J , Núñez L , Pfeffer M , Paricio M , Lorites J , Piñol-Ripoll G , Gámez JE , Anaya F , Kiprov D , Lima J , Grifols C , Torres M , Costa M , Bozzo J , Szczepiorkowski ZM , Hendrix S , Páez A ((2020) ) A randomized, controlled clinical trial of plasma exchange with albumin replacement for Alzheimer’s disease: Primary results of the AMBAR Study. Alzheimers Dement 16: , 1412–1425. |
[18] | McKhann GM , Knopman DS , Chertkow H , Hyman BT , Jack CR Jr. , Kawas CH , Klunk WE , Koroshetz WJ , Manly JJ , Mayeux R , Mohs RC , Morris JC , Rossor MN , Scheltens P , Carrillo MC , Thies B , Weintraub S , Phelps CH ((2011) ) The diagnosis of dementia due to Alzheimer’s disease: Recommendations from the National Institute on Aging-Alzheimer’s Association workgroups on diagnostic guidelines for Alzheimer’s disease. Alzheimers Dement 7: , 263–269. |
[19] | Boada M , López O , Núñez L , Szczepiorkowski ZM , Torres M , Grifols C , Páez A ((2019) ) Plasma exchange for Alzheimer’s disease Management by Albumin Replacement (AMBAR) trial: Study design and progress. Alzheimers Dement (N Y) 5: , 61–69. |
[20] | McKhann G , Drachman D , Folstein M , Katzman R , Price D , Stadlan EM ((1984) ) Clinical diagnosis of Alzheimer’s disease: Report of the NINCDS-ADRDA Work Group under the auspices of Department of Health and Human Services Task Force on Alzheimer’s Disease. Neurology 34: , 939–944. |
[21] | Wattmo C , Wallin ÅK , Minthon L ((2013) ) Progression of mild Alzheimer’s disease: Knowledge and prediction models required for future treatment strategies. Alzheimers Res Ther 5: , 44. |
[22] | O’Bryant SE , Waring SC , Cullum CM , Hall J , Lacritz L , Massman PJ , Lupo PJ , Reisch JS , Doody R ((2008) ) Staging dementia using Clinical Dementia Rating Scale Sum of Boxes scores: A Texas Alzheimer’s research consortium study. Arch Neurol 65: , 1091–1095. |
[23] | Balsis S , Benge JF , Lowe DA , Geraci L , Doody RS ((2015) ) How do scores on the ADAS-Cog, MMSE, and CDR-SOB correspond? Clin Neuropsychol 29: , 1002–1009. |
[24] | Benoit JS , Chan W , Piller L , Doody R ((2020) ) Longitudinal sensitivity of Alzheimer’s disease severity staging. Am J Alzheimers Dis Other Demen 35: , 1533317520918719. |
[25] | Mitchell AJ ((2009) ) A meta-analysis of the accuracy of the mini-mental state examination in the detection of dementia and mild cognitive impairment. J Psychiatr Res 43: , 411–431. |
[26] | Franco-Marina F , García-González JJ , Wagner-Echeagaray F , Gallo J , Ugalde O , Sánchez-García S , Espinel-Bermúdez C , Juárez-Cedillo T , Rodríguez MA , García-Peña C ((2010) ) The Mini-mental State Examination revisited: Ceiling and floor effects after score adjustment for educational level in an aging Mexican population. Int Psychogeriatr 22: , 72–81. |
[27] | Perneczky R , Wagenpfeil S , Komossa K , Grimmer T , Diehl J , Kurz A ((2006) ) Mapping scores onto stages: Mini-mental state examination and clinical dementia rating. Am J Geriatr Psychiatry 14: , 139–144. |
[28] | Arevalo-Rodriguez I , Smailagic N , Roqué IFM , Ciapponi A , Sanchez-Perez E , Giannakou A , Pedraza OL , Bonfill Cosp X , Cullum S ((2015) ) Mini-Mental State Examination (MMSE) for the detection of Alzheimer’s disease and other dementias in people with mild cognitive impairment (MCI). Cochrane Database Syst Rev 2015: , CD010783. |
[29] | Chapman KR , Bing-Canar H , Alosco ML , Steinberg EG , Martin B , Chaisson C , Kowall N , Tripodis Y , Stern RA ((2016) ) Mini Mental State Examination and Logical Memory scores for entry into Alzheimer’s disease trials. Alzheimers Res Ther 8: , 9. |
[30] | O’Bryant SE , Lacritz LH , Hall J , Waring SC , Chan W , Khodr ZG , Massman PJ , Hobson V , Cullum CM ((2010) ) Validation of the new interpretive guidelines for the clinical dementia rating scale sum of boxes score in the national Alzheimer’s coordinating center database. Arch Neurol 67: , 746–749. |
[31] | Julayanont P , DeToledo JC ((2022) ) Validity of the Clinical Dementia Rating Scale Sum of Boxes in staging and detection of cognitive impairment in Mexican Americans. J Geriatr Psychiatry Neurol 35: , 128–134. |
[32] | Food and Drug Administration, Guidance for Industry Alzheimer’s Disease: Developing Drugs for the Treatment of Early Stage Disease https://isctm.org/public_access/FDAGuidance_AD_Developing_Drugs_Early_Stage_Treatment.pdf, Accessed 10 January, 2024. |
[33] | Budd Haeberlein S , Aisen PS , Barkhof F , Chalkias S , Chen T , Cohen S , Dent G , Hansson O , Harrison K , von Hehn C , Iwatsubo T , Mallinckrodt C , Mummery CJ , Muralidharan KK , Nestorov I , Nisenbaum L , Rajagovindan R , Skordos L , Tian Y , van Dyck CH , Vellas B , Wu S , Zhu Y , Sandrock A ((2022) ) Two randomized phase 3 studies of aducanumab in early Alzheimer’s disease. J Prev Alzheimers Dis 9: , 197–210. |
[34] | Franzen S , Smith JE , van den Berg E , Rivera Mindt M , van Bruchem-Visser RL , Abner EL , Schneider LS , Prins ND , Babulal GM , Papma JM ((2022) ) Diversity in Alzheimer’s disease drug trials: The importance of eligibility criteria. Alzheimers Dement 18: , 810–823. |
[35] | van Dyck CH , Swanson CJ , Aisen P , Bateman RJ , Chen C , Gee M , Kanekiyo M , Li D , Reyderman L , Cohen S , Froelich L , Katayama S , Sabbagh M , Vellas B , Watson D , Dhadda S , Irizarry M , Kramer LD , Iwatsubo T ((2023) ) Lecanemab in early Alzheimer’s disease. N Engl J Med 388: , 9–21. |
[36] | Rizopoulos D ((2012) ) Joint Models for Longitudinal and Time-to-Event Data With Applications in R, CRC Press Boca Raton. |
[37] | Boada M , López OL , Olazarán J , Núñez L , Pfeffer M , Puente O , Piñol-Ripoll G , Gámez JE , Anaya F , Kiprov D , Alegret M , Grifols C , Barceló M , Bozzo J , Szczepiorkowski ZM , Páez A ((2022) ) Neuropsychological, neuropsychiatric, and quality-of-life assessments in Alzheimer’s disease patients treated with plasma exchange with albumin replacement from the randomized AMBAR study. Alzheimers Dement 18: , 1314–1324. |
[38] | Meeuwsen EJ , Melis RJ , Van Der Aa GC , Golüke-Willemse GA , De Leest BJ , Van Raak FH , Schölzel-Dorenbos CJ , Verheijen DC , Verhey FR , Visser MC , Wolfs CA , Adang EM , Olde Rikkert MG ((2012) ) Effectiveness of dementia follow-up care by memory clinics or general practitioners: Randomised controlled trial. BMJ 344: , e3086. |
[39] | Hoe J , Hancock G , Livingston G , Woods B , Challis D , Orrell M ((2009) ) Changes in the quality of life of people with dementia living in care homes. Alzheimer Dis Assoc Disord 23: , 285. |
[40] | Logsdon RG , Gibbons LE , McCurry SM , Teri L ((2002) ) Assessing quality of life in older adults with cognitive impairment. Psychosom Med 64: , 510–519. |
[41] | Spector A , Thorgrimsen L , Woods B , Royan L , Davies S , Butterworth M , Orrell M ((2003) ) Efficacy of an evidence-based cognitive stimulation therapy programme for people with dementia: Randomised controlled trial. Br J Psychiatry 183: , 248–254. |
[42] | Selwood A , Thorgrimsen L , Orrell M ((2005) ) Quality of life in dementia–a one-year follow-up study. Int J Geriatr Psychiatry 20: , 232–237. |
[43] | Hall CB , Lipton RB , Sliwinski M , Stewart WF ((2000) ) A change point model for estimating the onset of cognitive decline in preclinical Alzheimer’s disease. Stat Med 19: , 1555–1566. |
[44] | Hall C , Ying J , Kuo L , Lipton R ((2003) ) Bayesian and profile likelihood change point methods for modeling cognitive function over time. Comput Stat Data Anal 42: , 91–109. |
[45] | Grober E , Hall CB , Lipton RB , Zonderman AB , Resnick SM , Kawas C ((2008) ) Memory impairment, executive dysfunction, and intellectual decline in preclinical Alzheimer’s disease. J Int Neuropsychol Soc 14: , 266–278. |
[46] | Harrison JE , Rentz DM , Brashear HR , Arrighi HM , Ropacki MT , Liu E ((2018) ) Psychometric evaluation of the neuropsychological test battery in individuals with normal cognition, mild cognitive impairment, or mild to moderate Alzheimer’s disease: Results from a longitudinal study. J Prev Alzheimers Dis 5: , 236–244. |
[47] | Mortamais M , Ash JA , Harrison J , Kaye J , Kramer J , Randolph C , Pose C , Albala B , Ropacki M , Ritchie CW , Ritchie K ((2017) ) Detecting cognitive changes in preclinical Alzheimer’s disease: A review of its feasibility. Alzheimers Dement 13: , 468–492. |
[48] | Mura T , Proust-Lima C , Jacqmin-Gadda H , Akbaraly TN , Touchon J , Dubois B , Berr C ((2014) ) Measuring cognitive change in subjects with prodromal Alzheimer’s disease. J Neurol Neurosurg Psychiatry 85: , 363–370. |
[49] | Lowe DA , Balsis S , Benge JF , Doody RS ((2015) ) Adding delayed recall to the ADAS-cog improves measurement precision in mild Alzheimer’s disease: Implications for predicting instrumental activities of daily living. Psychol Assess 27: , 1234. |
[50] | Jack CR Jr. , Bennett DA , Blennow K , Carrillo MC , Dunn B , Haeberlein SB , Holtzman DM , Jagust W , Jessen F , Karlawish J , Liu E , Molinuevo JL , Montine T , Phelps C , Rankin KP , Rowe CC , Scheltens P , Siemers E , Snyder HM , Sperling R ((2018) ) NIA-AA Research Framework: Toward a biological definition of Alzheimer’s disease. Alzheimers Dement 14: , 535–562. |
[51] | Dubois B , Villain N , Frisoni GB , Rabinovici GD , Sabbagh M , Cappa S , Bejanin A , Bombois S , Epelbaum S , Teichmann M , Habert MO , Nordberg A , Blennow K , Galasko D , Stern Y , Rowe CC , Salloway S , Schneider LS , Cummings JL , Feldman HH ((2021) ) Clinical diagnosis of Alzheimer’s disease: Recommendations of the International Working Group. Lancet Neurol 20: , 484–496. |
[52] | Teunissen CE , Verberk IMW , Thijssen EH , Vermunt L , Hansson O , Zetterberg H , van der Flier WM , Mielke MM , Del Campo M ((2022) ) Blood-based biomarkers for Alzheimer’s disease: Towards clinical implementation. Lancet Neurol 21: , 66–77. |
[53] | Hampel H , Cummings J , Blennow K , Gao P , Jack CR Jr. , Vergallo A ((2021) ) Developing the ATX(N) classification for use across the Alzheimer disease continuum. Nat Rev Neurol 17: , 580–589. |
[54] | Wesseling H , Mair W , Kumar M , Schlaffner CN , Tang S , Beerepoot P , Fatou B , Guise AJ , Cheng L , Takeda S , Muntel J , Rotunno MS , Dujardin S , Davies P , Kosik KS , Miller BL , Berretta S , Hedreen JC , Grinberg LT , Seeley WW , Hyman BT , Steen H , Steen JA ((2020) ) Tau PTM profiles identify patient heterogeneity and stages of Alzheimer’s disease. Cell 183: , 1699–1713.e1613. |
[55] | Food and Drug Administration, FDA Grants Accelerated Approval for Alzheimer’s Drug, https://www.fda.gov/news-events/press-announcements/fda-grants-accelerated-approval-alzheimers-drug, Accessed January 10, 2024. |
[56] | Ritchie M , Gillen DL , Grill JD ((2023) ) Estimating attrition in mild-to-moderate Alzheimer’s disease and mild cognitive impairment clinical trials. Alzheimers Res Ther 15: , 203. |
Notes
1 Trial designed to determine effective PE-A dose and designated 2b by FDA; The Agencia Española de Medicamentos y Productos Sanitarios (AEMPS) designated AMBAR a Phase 3. Consequently, AMBAR is often referred to as a Phase 2b/3.