Resting State Electrophysiological Profiles and Their Relationship with Cognitive Performance in Cognitively Unimpaired Older Adults: A Systematic Review
Abstract
Background:
Aging is a complex and natural process. The physiological decline related to aging is accompanied by a slowdown in cognitive processes, which begins shortly after individuals reach maturity. These changes have been sometimes interpreted as a compensatory sign and others as a fingerprint of deterioration.
Objective:
In this context, our aim is to uncover the mechanisms that underlie and support normal cognitive functioning in the brain during the later stages of life.
Methods:
With this purpose, a systematic literature search was conducted using PubMed, Scopus, and Web of Science databases, which identified 781 potential articles. After applying inclusion and exclusion criteria, we selected 12 studies that examined the brain oscillations patterns in resting-state conditions associated with cognitive performance in cognitively unimpaired older adults.
Results:
Although cognitive healthy aging was characterized differently across studies, and various approaches to analyzing brain activity were employed, our review indicates a relationship between alpha peak frequency (APF) and improved performance in neuropsychological scores among cognitively unimpaired older adults.
Conclusions:
A higher APF is linked with a higher score in intelligence, executive function, and general cognitive performance, and could be considered an optimal, and easy-to-assess, electrophysiological marker of cognitive health in older adults.
INTRODUCTION
Throughout the aging process, cognition and the brain itself change, both anatomically and functionally. Efficient synaptic transmission sits at the basis of normal cognitive functioning [1] and represents the foundational mechanism underlying neural oscillations, which in turn are known to be of critical relevance to cognitive performance [2]. Oscillations in the brain are the result of the coordinated electrophysiological activity of relatively large groups of neurons. They coordinate neural processing across the cortical surface by a phasic modulation of neuronal firing that largely depends on the amplitude of these oscillations [3]. The brain oscillations have been historically grouped into bands according to the frequency activity (i.e., the number of cycles per second), such as delta (1–4 Hz), theta (4–8 Hz), alpha (8–12 Hz), beta (12–30 Hz), low gamma (30–80 Hz), and high gamma (80–150 Hz).
The role of oscillatory activity during task performance has been largely studied and documented, since their amplitude is known to be modulated as a result of cognitive engagement in the task [4]. The metrics used in the characterization of brain oscillations include the calculation of the power spectral density, which means how the signal power is distributed over the different frequencies or bands, and the analyzing the statistical relationship between the activities of several brain areas under certain conditions called functional connectivity (FC). For instance, theta activity during memory paradigms, and in particular, frontal midline modulations of its power, have been systematically associated with increases in task difficulty and memory load [5, 6]. Similarly, alpha power has been also associated with cognitive effort during memory tasks [7, 8] but also with attentional allocation to the right and left during a somatosensory working memory task [9] and with distraction inhibition [10]. Even though a more profound review of this topic is out of the scope of the present manuscript, previous literature has systematically reported that modulations of each brain rhythm are associated with performance in several different cognitive tasks [7].
Contrarily, resting state activity represents the spontaneous modulations occurring in the brain activity while no specific task is being performed. Even though this activity has been traditionally misconceived as noise, it has been repeatedly shown to contain a significant amount of internal structure, exhibiting persistent patterns of long-range coordination between distant brain regions [11]. Furthermore, these patterns of resting state activity are known to be highly reproducible among different individuals, mental activity states and they have even been used to characterize different clinical and demographical groups [12]. Of note, different cognitive abilities have been shown to be consistently associated with these resting state patterns measured by functional magnetic resonance imaging (fMRI) [12]. In particular, a more intense activity (strength) during resting state in a specific network has traditionally been predictive of better performance in the cognitive domain associated to such network [12]. In fact, this greater activity at rest has been interpreted as a sign of the renormalization of patterns of oscillatory activity observed during ongoing cognitive processes [13]. That is to say, brain activity during active cognitive efforts would be directly linked to resting state activity, which should represent a major driver for cognitive research in resting state.
Interestingly, despite the fact that the relationship between resting state and cognitive abilities has been studied in the field of fMRI in a relatively consistent way, our understanding of this interaction from an electrophysiological point of view is by far scarcer. This gap in the literature is particularly striking concerning to study of aging, a stage in which cognitive change is particularly marked, as it has already been mentioned. fMRI has been widely used during the last few decades in research given its wide availability and extraordinary spatial resolution, despite its indirect estimation of brain activity based on the blood oxygen level dependent response (BOLD). However, magnetoencephalography (MEG) and electroencephalography (EEG) are techniques able to directly measure the result of the synaptic communication between neuron populations. Furthermore, M/EEG are able to track brain activity not only with good spatial resolution but also with incomparable temporal resolution up to the millisecond scale, orders of magnitude better compared to the nonetheless very useful fMRI technique. This difference makes these techniques able to capture the activity in a timescale much closer to the actual behavior of neural populations, and thus, can greatly contribute to increase our understanding of cognitive processing in the brain. This article aims to review and summarize the findings of previous literature studying the relationship between cognitive performance and resting-state electrophysiological activity in cognitively unimpaired older adults. Since wide literature utilizes the term “healthy aging” to refer to cognitively unimpaired older adults, even when the overall health status of the participants is not considered, we will use this term in our search. Nevertheless, in the interpretation of the results no assumptions will be made beyond the cognitive status of the individuals.
METHODS
Literature search
A systematic search of the literature was conducted on 27 November 2023 using PubMed, Scopus, and Web of Science databases according to the Patient, Intervention, Comparison, Outcome (PICO) search strategy [14] in order to systematize articles addressing the topic of interest. Keywords included in the literature search were:
Table 1 lists the key concepts and search terms employed in PubMed, Scopus, and Web of Science (June 2021).
Table 1
Search terms
Key concept | Search terms used |
Healthy aging | “Healthy*” OR “healthy aging” OR “healthy adults” OR “normal aging” OR “older adults” OR “elderly” OR “aging” OR “normal adult” |
Cognitive performance | “cognition” OR “neuropsychology” OR “cognitive markers” OR “cognitive functioning” OR “cognitive domains” OR “brief cognitive screening” OR “screening test” OR “cognitive battery” OR “neuropsychological evaluation” OR “Cognitive Assessment” OR “Screening Instrument” OR “Neurocognitive Tests” OR “cognitive test” OR “assess*” OR “screen*“OR “neuropsychological performance” OR “neuropsychological test” OR “neuropsych*” OR “cognitive screening” |
Resting state | “rsfc” OR “resting state” OR “resting-state” OR “resting” OR “ongoing activity” OR “task- free” OR “task free” |
Signal analysis | “power” OR “spectral density” OR “oscillations” OR “synchronization” OR “network” OR “topological” OR “graph” OR “complexity” OR “non-linear dynamic” OR “functional connectivity” |
Neuroimaging technique | “EEG”OR “electroence*”OR “MEG” OR “magnetoence*” OR “electrophysio*” |
Article’s inclusion and exclusion criteria
Articles included were limited to those published within the last twelve years (2011 to 2023) in journals indexed in the Journal Citation Reports (JCR), following a peer-review process, written in English, and in which study participants were human. The revision procedure included clinical studies, case report journal articles, and empirical studies.
The studies considered describe the inclusion criteria to characterize the cognitively unimpaired older adults, considering as standard criteria: i) absence of a personal history of mental illness, psychiatric or neurological disorders, ii) no history of brain hemorrhage, concussion, skull fracture, brain surgery or brain tumor, and iii) no use of medication with an effect on the central nervous system.
In addition, articles were excluded when i) participants were younger than 50 years old, ii) with other medical conditions, iii) those whose evaluation was not conducted in the participant’s native language, iv) information regarding power spectral analysis or functional connectivity in EEG or MEG was not reported, and/or v) eyes closed recording was not included in the analysis.
Screening protocol
In order to avoid duplication, ensure the reliability of the process, and follow a standard systematic review protocol, this review was registered in PROSPERO (publication code: CRD42021279382). Selected articles were imported into COVIDENCE [15]. The revision process was conducted by two reviewers (BC and DLS), as recommended in the Preferred Reporting Items for Systematic Reviews and Meta-Analyses (PRISMA) guidelines for systematic reviews [16], attending to the above-mentioned exclusion and inclusion criteria. Briefly, the articles’ title’ and abstracts were initially screened. For those manuscripts selected in the first stage, full texts were obtained and subsequently reviewed. Disagreements were resolved in specific meetings to review each conflicting point (screening protocols and final selection are depicted in Fig. 2).
Quality assessment
Three independent reviewers (BC, LT, and SD) assessed the quality of the selected articles using the tool for cross-sectional studies using biomarker data (BIOCROSS) [17]. The specific assessment of the items was performed under the adaptation described in Torres-Simon et al. [18], as some of the original items could not be easily applied due to the nature of the specific research field studied. These adaptations did not alter the scale’s structure or the study’s aim for each item. This information included: neurophysiological technical specifications, research or data processing protocols modeling details. Furthermore, we incorporated additional specifications to improve quality standards within our study population, cognitively unimpaired older adults (for more details, see “Quality Assessment” in the Supplementary Material). Quality assessment was conducted over the 12 selected studies. No articles were excluded based on the quality assessment (The three reviewers’ final scores for the 12 articles for each quality item are reported in Supplementary Table 1) (See the Supplementary Material for further information).
Data extraction
The information extracted from each full text article reviewed is summarized in two tables according to the type of signal analysis performed (spectral analysis, Table 1; and connectivity analysis, Table 2).
In these tables the most relevant information was extracted for each of the articles following the same structure:
• Authors and publication date.
• Sample characteristics: including number of subjects and basic demographic information (age and distribution by sex).
• Methods: referring to the neuroimaging technique used in each article (MEG or EEG). and cognitive test (classified by cognitive process) were also included.
• Main findings: where main results, conclusions and limitations were reported.
Data synthesis
Due to the diversity in recording conditions and analysis methods utilized in the 12 selected articles, we employed the synthesis without meta-analysis (SWiM) guidelines described by Campbell et al. [19]. Regarding diversity in methods and conditions, data synthesis was conducted by attending to the main type of analysis employed (i.e., spectral analysis versus connectivity analysis) and the cognitive process involved.
Analyzing power spectral distribution is one of the most commonly utilized strategies in electrophysiology studies. The fundamental basis is the assumption that every oscillation can be decomposed into a sum of sine waves with different frequencies and amplitudes. Consequently, it is possible to estimate the contribution of various frequencies to the recorded brain activity. Several studies show that individual differences in peak frequencies have been linked to several individual characteristics, highlighting their potential as biomarkers for specific diseases. Also, in the last decades, dynamically studying the frequency content of brain signals has fostered great interest, revealing promising results in the field of aging. Analyzing the oscillatory components of brain signals is a key step to further study the “interaction” between brain regions, i.e., functional connectivity (FC).
Both analyses allowed us to categorize and compare the findings across different cognitive domains. In the case of connectivity studies, the division according to cognitive domains was not possible, considering the small number of studies. Figure 1 shows the number of papers included in each category.
Fig. 1
Data synthesis structure. Figure 1 classifies and quantifies the papers included in each category. *One of the studies analyzed the acquired signal with both methods (spectral analysis and functional connectivity).
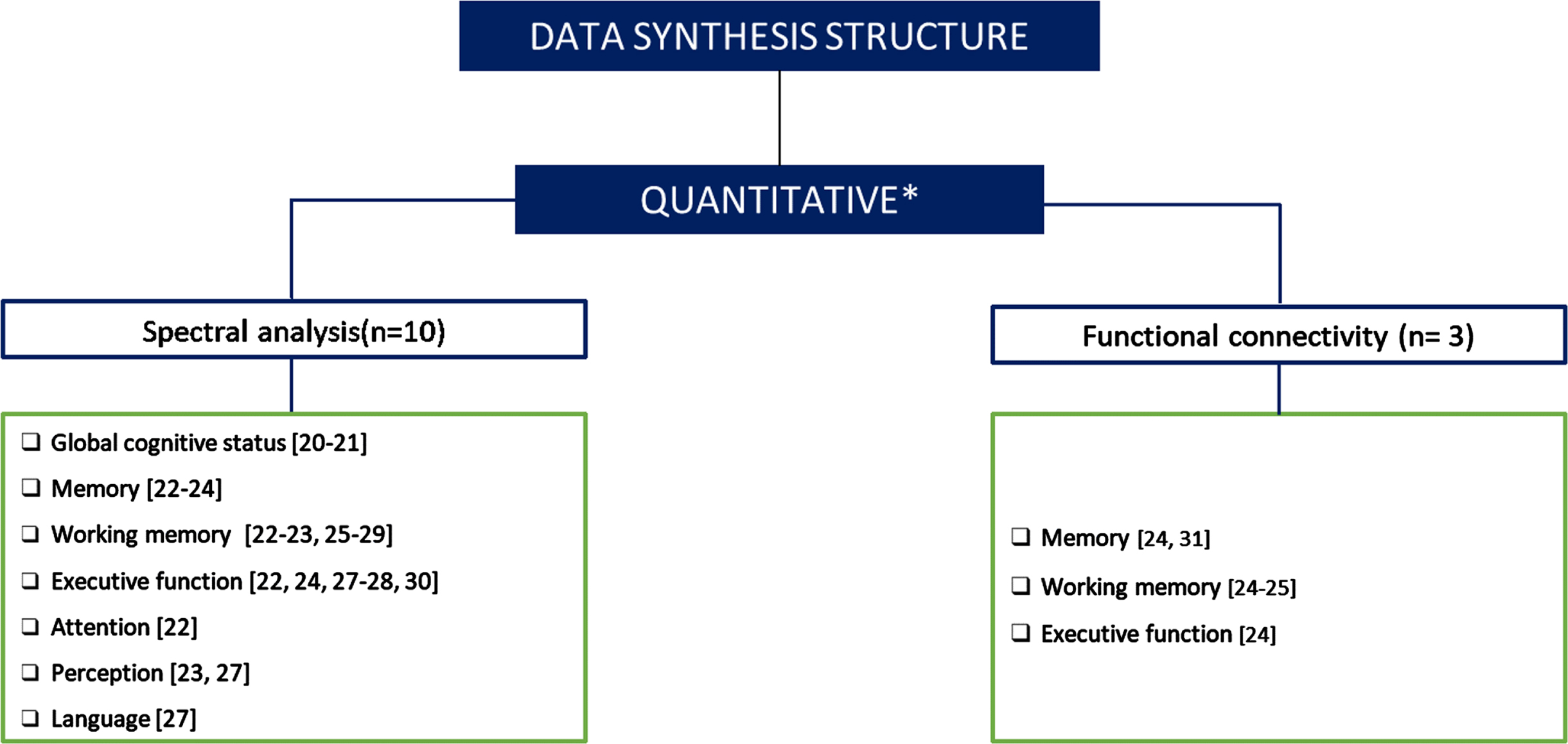
RESULTS
After conducting a literature search in the specified datasets, seven hundred eighty-one articles were imported for screening. After removing those duplicated and those irrelevant for this systematic review, 42 studies were assessed for eligibility. Thirty studies were excluded based on the exclusion criteria: twenty-four were excluded for incompatible study designs, and six were not included due to the age range studied. Finally, twelve studies with 3,437 participants were considered for analysis in the systematic review (see Fig. 2).
Fig. 2
Selection process overview. Figure 2 shows a flow chart of included and excluded articles through the screening process following the PRISMA presentation guidelines.
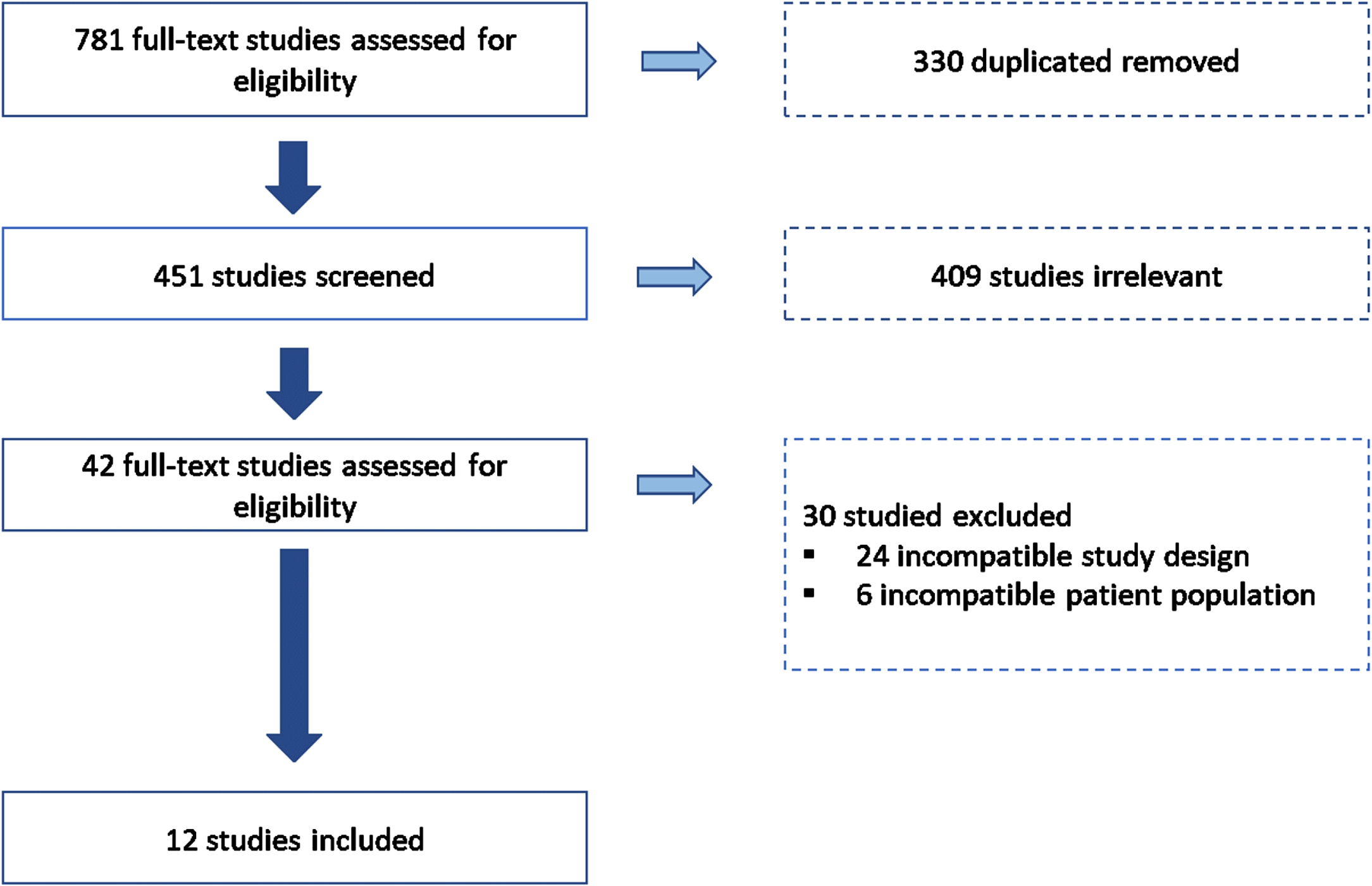
Inclusion and exclusion criteria across the studies
Before discussing neural oscillations and their relationship with cognitive performance, we briefly review the inclusion and exclusion criteria utilized within each of the 12 articles examined to define the samples and how each of them selects several characteristics associated with the definition of healthy aging. It is significant to mention that the majority of the sample was invited to get involved in the studies through fliers and advertisements in the community.
Regarding the selection criteria, 7/12 articles used screening tests to evaluate the subjects’ cognitive performance to select the sample (7/12). Other relevant aspects considered were: 5/12 required normal or corrected normal vision, 4/12 right-handedness, and 3/12 exclusively selected native speakers.
The studies show a pattern of factors to consider in selecting the sample. The essential criteria comprised the exclusion of individuals with: i) personal history of mental illness or psychiatric disorder (9/12), ii) neurological disorders (9/12), and iii) previous or other medical conditions (7/12). Other specifications also considered were: i) antecedents of traumatic brain injury (6/12), ii) the loss of consciousness for more than five minutes or in two or more episodes (5/12), and iii) history of drug or alcohol condition (5/12).
Spectral analysis
Described in Table 2.
Table 2
Summary of studies included in power spectral analysis
Authors | N | Mean Age±SD (Range) | Sex (M/F) | Neuroimaging Technique | Resting State (Time) | Cognitive domain | Type of signal analysis | Main Findings | Quality Assessment Score- Classification |
Borhani et al. [25] | 43 | 71.6±7 | 20/23 | EEG (14 channels) | 60 s | Working memory (Short term memory STM) | Absolute power | •Reaction time: (+) δ (Left parietal site – P7) (–) reaction time in memory task(+) θ band (left parietal) Faster reaction time in memory task (no significant; p = 0.07) | 15.67 (VG) |
•Accuracy (Short- term memory task-STM) (–) β band over the right parietal site (+) accuracy STM.(–) α (–) β (Right parietal site) and (–) γ (right frontal) – Higher ability to correctly distinguish between target and distractor images in a STM. | |||||||||
Cesnaite et al. [30] | 1703 | 70±4.7 (60-80) | 823/880 | EEG (31 channels) | 5 min | Executive function (Trail Making Test, Stroop Test) | Absolute power | (+) APF (+) Better performance in EF. | 18.00 (VG) |
(+) α (–) speed of processingNo association between EM and EEG measures. | |||||||||
Choi et al. [20] | 496T3 = 162T2 = 179T1 = 155 | 67.84 ± 9.7763.05±8.3766.54±8.2474.34±9.31 | 165/33168/9464/11533/122 | EEG (2 channels; Fp1. Fp2) | 5 min | Global Cognitive status (MMSE scores) was divided by MMSE terciles:T3 (28≤MMSE≤30)T2 (25≤MMSE≤27)T1 (MMSE≤24) | Median frequencyAlpha Peak Frequency (APF)Alpha Theta Ratio (ATR) | Males: Differences in Alpha-Theta Ratio between T1-T2 (t = –5.19).Females: (+) Median frequency (+) MMSE.MMSE &EEG decreased with age and less education ((+) in females).(+) Median frequency (+) peak frequency (APF) and (+) Alpha-Theta Ratio (ATR) correlated with (+) orientation time and place. | 16.00 (VG) |
Clark et al. [26] | 550140160150100 | 33 (11–70)17±2.5 (11–20)24.6±2.8 (21–30)40.3±6 (31–50)58±5.7 (51–70) | Balanced | EEG (10 channels) | 2 min | Working Memory (Digit span, reverse digit span) | Alpha Peak Frequency (APF) | (–) Alpha peak frequency (APF) (+) Age: Stronger at anterior than posterior regions.(+) APF (+) working memory (independent of age).↑ 1 Hz in APF frontal is associated with ↑ 0.21 scores in reverse digit span. | 12.67 (G) |
Finnigan et al. [22] | 73 | 60.76 (56–70) | 25/48 | EEG (4 channels; CZ, FZ, PZ, and M1) | 4 min | Memory (Rey Auditory Verbal Learning test- RAVLT and Rivermead Behavioral Memory Test- RBMT immediate and delay recall)Intelligence (Raven’s Standard Progressive Matrices- RSPM)Working Memory (Digit span)Attention (Sustained Attention to respond task- SART) | Absolute power / Relative power | •In Fz:(+) θ relative power (+) List A Recall RAVLT (+) RSPM, (+) category fluency, and (–) SART reaction time. | 15.67 (VG) |
•In Pz:(+) θ band – (+) recall immediate and (+) delayed RBMT scores.(–) β band – (+) RSPM and (+) backward digit span scores. | |||||||||
Fleck et al. [24] | 66 | 67.15±9.16 (50–88) | 24/42 | EEG (19 channels) | 3 min | Memory (California Verbal Learning Test- CVLT II) Executive Function (Trail Making Test TMT) | Absolute power | •(–) posterior δ power – (+) Immediate memory and (+) delayed recall | 15.33 (VG)Authors |
•(–) posterior δ power – better performance in TMT A | |||||||||
•(–) β power at frontal and posterior electrode sites (+) CVLT II- immediate and delay recall (no significant at the 0.01 significance threshold). | |||||||||
Grandy et al. [23] | Interv 58 Young 30Old 28Control 27Young 15Old 12 | 25.3±3.1 (20–31)71.8±4.0 (65–80)24.8±2.3 (22–29)69.2±3.5 (66–79) | 13/1718/108/76/6 | EEG (64 channels) | 2 min | Perceptual speed. Choice reaction tasks (CRTs). Comparison tasks (CTs: Digits, letters, or lines)Working Memory (WM: numerical memory updating task)Memory (Episodic Memory- EM: word list task)Intelligence (Raven's Standard Progressive Matrices- RSPM) | Alpha peak frequency | In both groups: (+) APF (+) speed tasks (CRT, CT, digit symbol). In young adults: (+) APF (+) complex tasks (WM. EM, RSPM).APF: Lower in older adults relative to younger adults. APF with eyes closed is highly reliable and stable with regard to the rank order of individuals across a test- retest interval of 6.6 months. | 16.33 (VG) |
Kamal et al. [21] | 22 | 71.81±4.33 | 11/11 | EEG (31 channels) | 7 min | Global Cognitive status (MoCA scores)Memory (CVLT-II) | Absolute power | (–) θ power (+) Global cognitive performanceNo association between EM and rsEEG measures. | 12.00 (VG) |
Roca-Stappung et al. [27] | 27 | 67.2±6.70 (60–84) | 12/15 | EEG (19 channels) | 20 min | Intelligence (Wechsler Adult Intelligence Scale WAIS-III). Including too: | Absolute power / Relative power | (–) δ Absolute power (AP) (+) WAIS III index scores(+) WM index (–) δ (frontal site) (–) θ (fronto polar).Lateral prefrontal cortex (Left hemisphere) is activated during WM task.(+) alpha values – (+) cognition. (–) θ Relative power (RP) (+) verbal scores (Left temp. and bilateral central and parietal regions)(–) θ in frontal areas (+) perceptual organization index(+) β (+) verbal comprehension index, processing speed index, perceptual org. index.(+) β RP: (–) processing speed index(+) perceptual organization index. | 15.67 (VG) |
Stacey et al. [28] | 75Young 31Old 44 | 18–90 23.96±4.52 (18–30)71.47±6.49 (61–90) | 26/4910/2116/28 | EEG (128 channels) | 2.5 min per two times | Working memory (Digit span, Spatial span)Executive function (Trail Making Test TMT) | Alpha peak frequency / Absolute power | β power: Older > YoungAPF: Young > OlderEC: (+) APF Frontal (+) WM (no significant then of multiple comparison).Older: (+) central δ power (–) time in TMT. | 15.67 (VG) |
Trammell et al. [29] | 36Young 16Old 20 | –20,7±0.9 (20–19)72,9±2.5 (70–79) | 14/228/86/14 | EEG (19 channels) | 5 min per two times | Working memory (Short term Memory-STM)Intelligence (Raven’s Standard Progressive Matrices-RSPM) | Theta alpha RatioRelative powerAbsolute powerAlpha peak frequency | RP: relative PowerCz: TAR dependent upon cognitive performance only after accounting for age.With constant cognitive performance: young adults had greater θ and α than old adultsAge: (+) age (–) iPAFFZ: (–) Relative δ (+) age. Fz and Cz: (–) Relative θ (+) age.(+) α (+) cognitive ability in general (Aging: no sig.)(–) RSPM (+) TAR(Constant: Age, STM, and task)All sample: (+) STM (–) θ and (–) α(+)iAPF (+) RSPM.(+) δ RP (+) STM (Fz, Pz, Cz)Young: (+) TAR (+) STM(+) TAR (+)RSPM (no sig.).(+) α (+) RSPM(+) δ RP (+) STM (Fz)Older: (–) α (–) θ (+) RSPM(+) δ RP (+) STM (Pz). No sign. results in theta power and performance. | 16.00 (VG) |
Ten articles were included in this section, using EEG to record the physiological signal during resting state. Even though power spectral estimation is a relatively straight-forward measurement, a variety of different methodologies were used among the selected articles, including absolute power and relative power of the signal in each frequency band, the ratios between the power found in the theta and alpha band, the alpha peak frequency (APF), and the measure of distribution across the brain. We present below the results classified by the cognitive domain under study (see Fig. 3).
Fig. 3
Cognitive performance associated with alpha peak frequency (APF) in subjects without cognitive impairment. a) Various studies show a decrease in cognitive performance with increasing aging [32–34]; b) Interestingly, this pattern was also documented in the relationship between APF and age. c) Our review suggests that a higher APF is linked with a higher score in intelligence, executive function, and general cognitive performance and could be considered an optimal, and easy-to-assess, electrophysiological marker of cognitive health in older adults.
![Cognitive performance associated with alpha peak frequency (APF) in subjects without cognitive impairment. a) Various studies show a decrease in cognitive performance with increasing aging [32–34]; b) Interestingly, this pattern was also documented in the relationship between APF and age. c) Our review suggests that a higher APF is linked with a higher score in intelligence, executive function, and general cognitive performance and could be considered an optimal, and easy-to-assess, electrophysiological marker of cognitive health in older adults.](https://content.iospress.com:443/media/jad/2024/100-2/jad-100-2-jad231009/jad-100-jad231009-g003.jpg)
The memory domain was the main focus of five studies. Finnigan & Robertson [22] evaluated the immediate and delayed recall performance in the resting state with only four channels located over frontal, central, and parietal areas. They describe a positive association between immediate recall scores and theta band amplitude in frontal and parietal sites in relative and absolute power. A similar tendency was observed in delayed recall scores, where higher absolute theta power in parietal areas correlated with better performance. Fleck et al. [24] described a pattern in which decreases in delta and beta absolute power in posterior and frontal areas were related to better immediate and delayed recall performance despite evaluating the same cognitive domain. This might unveil a different association with memory depending on the area and the frequency band analysis. Furthermore, regarding the APF, Grandy et al. [23] reported an increase in APF power associated with episodic memory, commonly related in cognitive neuropsychology with delayed and immediate recall scores in young and older adults. However, two recent studies have found no significant relationship between the variables [21, 30].
Seven studies find results related to Working memory. Three studies showed that higher working memory scores were associated with higher APFs [23, 26, 28]. This result is particularly relevant considering these three previous studies’ large sample (683 subjects). The same tendency was observed related to delta relative power in frontal, parietal, and central areas, meaning that an increase in delta relative power is associated with better performance in short-term memory tasks [29]. However, Roca-Stappung et al. [27] and Borhani et al. [25], reported contradictory results, showing an inverse correlation between delta power, and working memory score and reaction time in the task, respectively. Similarly, lower values of alpha power [25, 29] beta power over parietal site [22, 25], and gamma power in parietal and frontal sites [25], respectively, have been recently reported in association to better working memory performance.
Regarding the executive function, the faster completion of the Trail Making Test (TMT) was associated with an increase in the delta power; however, the areas implicated in the task changed from posterior areas in TMT part A [24] to central areas in TMT part B [28]. In addition, Roca-Stappung et al. [27] reported a positive association between processing speed index and beta absolute power, and an inverse pattern emerged for beta relative power. On a different note, Finnigan & Robertson [25] describe that better performance in executive function (Verbal Fluency Test, VFT) was associated with more relative theta power over frontal areas. Also, one study shows a positive link between EF and APF, where an IAF performs better in EF [30]. In attentional processing, only one study found an inverse association between relative theta power during resting state and reaction times obtained during a sustained attention-to-response task in frontal areas [25].
Perception was studies in two articles, one focused on reaction times, and the other one on the perceptual organization index (derived from Picture Completion, Block Design, and Matrix Reasoning subtests). In the first case, the results suggested that higher APF was associated with better task performance speed, i.e., faster perceptual skills [23]. Similarly, in the second study, an increase in beta power (absolute and relative) was found to be predictive of higher perceptive index score [27]. The inverse tendency was observed for theta power over frontal areas [27].
The association between language and power spectral measures has been poorly described in the literature. Roca-Stappung et al. [27] described an inverse relationship between verbal scores in WAIS III and theta relative power over left temporal and bilateral central and parietal regions; as well as a positive association between beta-band power and scores in the verbal comprehension index.
Finally, two studies evaluated the relationship between global cognitive performance. Kamal et al. [21] describe a negative correlation between theta power and global cognitive performance evaluated with MoCA. On the other hand, Choi et al. [20] found significant differences between individuals scoring less than 25 points and those scoring between 25–27 points in Mini-Mental State Examination (MMSE) (adjusting by age and years of education). Their results suggest that median frequency, peak frequency, and alpha-theta ratio were higher in individuals scoring 25–27 points than in patients with lower scores in prefronal areas. They also found that higher performance in time and place orientation were associated with increased in the three measures.
Connectivity
Described in Table 3.
Table 3
Summary of studies included in functional connectivity analysis
Authors | N | Mean Age±SD (Range) | Sex (M/F) | Neuroimaging Technique | Resting State (Time) | Cognitive domain | Type of signal analysis | Main Findings | Quality Assessment Score- Classification |
Borhani et al. [25] | 43 | 71.6±7 | 20/23 | EEG (14 channels) | 60 s | Working memory (Short- term memory) | Coherence analysis | (+) α coherence between right parietal and left frontal sites (+) reaction time in WM task. (+) θ & (+) δ coherence between frontal and temporal sites (+) accuracy scores in memory retrieval. | 15.67 (VG) |
Chino et al. [31] | 261 | 65.96±8.48 (58-87) | 91/170 | MEG (306 channels) | 5 min | Memory (Weschler Memory Scale III- Logical memory) | Functional Connectivity Strength | (–) β strength FC in right occipital regions (+) delayed recall performance.(–) β strength between left frontal regions and right occipital regions (+) delayed recall performance | 17.00 (VG) |
Fleck et al. [24] | 66 | 67.15±9.16 (50-88) | 24/42 | EEG (19 channels) | 3 min | Working memory (Digit Span)Memory (California Verbal Learning Test- CVLT II) Executive Function (Verbal Fluency Test VFT, and the Trail Making Test TMT) | Coherence analysis | (+) Digit span forward (+) β frontal coherence (+) γ frontal coherence(+) Digit span sequencing (+) frontal β coherence(–) posterior γ coherence(+) Memory (–) Frontal posterior θ coherence(+) CVLT-False Positive scores (+) Frontal and posterior δ coherence(+) category fluency (+) frontal coherence in the δ.(+) Trail B scores (+) frontal coherence δ & β frequency bands(+) Trail A scores (+) θ coherence between frontal and posterior brain regions. | 15.33 (VG) |
Brain connectivity is a relatively new field compared with power spectrum analysis in brain activity research [35, 36]. Probably because of this, only three studies were identified that looked into brain connectivity during resting state in association with cognitive performance, using two different metrics: magnitude-squared coherence and partial directed coherence with EEG and MEG data. In the following lines, the main results of these studies are presented.
Diverse strategies to assess memory were utilized. Fleck et al. [24] reported only a positive relationship between false positive recognition scores (as measured by the California Verbal Learning test) and delta coherence in frontal and posterior areas. That is, less false positive scores in the recognition task involved lower delta coherence in the above-mentioned regions. On the other hand, Chino et al. [31] describe a negative association between delayed recall performance and functional connectivity in beta band, over right occipital and left frontal regions.
Concerning working memory, very little is known regarding its relationship with resting state functional connectivity patterns. One study suggested a positive association between accuracy in working memory (Short term memory task) and delta and theta coherence between frontal and temporal areas [25]. Frontal areas involvement has been also highlighted by Fleck et al. [24] who reported a positive association between beta and gamma coherence in frontal areas and scores in digit span forward scores. However, this pattern is no longer followed by the results reported in the same study where gamma coherence over frontal areas was negatively associated with scores in digit span sequencing. Coherence over posterior brain regions showed in general negative associations in this study with the performance in the same digit span sequencing test for all delta, theta, beta, and gamma bands.
One study reported neural oscillations patterns in resting-state paradigms associated with executive function. Fleck et al. [24] found a positive relationship between executive function (using the VFT and TMT) and coherence measured in delta and beta bands over frontal regions, and also with coherence in the theta band between frontal and posterior brain regions.
DISCUSSION
Neuropsychological assessments are commonly used in healthcare facilities to differentiate between cognitive changes associated with aging and those that signal pathological states. Thus, establishing a correlate or biomarker of optimal cognitive functioning in the later stages of life could help unveil the underlying biological mechanisms. This could ultimately lead to the design of strategies (pharmacological or non-pharmacological) to protect and enhance cognitive performance at advanced age and improve quality of life. In this line, our purpose was to identify, considering the available literature, the electrophysiological fingerprints during resting state associated with cognitive performance cognitively unimpaired older adults, as reflected by the spectral and connectivity properties of brain activity in the different frequency bands. A thorough analysis shows one consistent resting-state brain oscillation pattern associated with cognitive skills, described below.
After examining the studies on power spectral analysis and cognition, the findings reveal a strong relationship between APF and cognitive capacity. Higher APF is associated with better performance in several cognitive domains, such as executive function, perception, and fluid intelligence [20, 23, 26, 28–30, 37]. A possible explanation of this finding is considering the APF as a state of cognitive preparedness [37]. According to Angelakis et al. [37], the APF predicts cognitive performance and reflects the brain state in healthy subjects. This idea was tested by comparing a group of traumatic brain injury (TBI) patients with healthy controls matched by age, sex, and education. Their results showed that TBI patients had lower APF in the post-test rest condition than the control group. Also, despite the evidence that APF decreases with age [39], this measure is considered a highly stable individual marker of central nervous system functioning [23], so that slowness of APF in the power spectrum could be a predictor of cognitive impairment in several cognitive domains [40], even before the dementia stage [41]. The consistent findings of the relationship between APF and cognitive performance in healthy older adults add to the reports on the role of APF as a potential alternative to assess the impact of intervention on lifestyle behaviors [42], making it a good candidate as a biological marker to assess healthy aging in different aspects.
Only three studies addressed the functional connectivity linked with cognitive performance. While differences in analytical approaches and the small number of studies limit the possibility of establishing general conclusions regarding stable and reliable patterns linking cognitive performance and functional connectivity, we observed a positive association between working memory and coherence across all frequency bands in frontal areas during eyes-closed conditions [24, 25]. This pattern was also observed using task paradigms in the theta and alpha band. Tóth et al. [43] found an increased theta FC strength between the frontal midline and temporal areas associated with better working memory performance. Similarly, Ariza et al. [44] showed that older adults require higher alpha synchronization between cortical brain regions to achieve a successful recognition. The similarity between resting state and task paradigms results could be explained by the high resources required in working memory execution by the association with other domains; however, this finding must be taken with discretion, considering the small sample, the different time analyzed, the methods used and the paradigms ofthe study.
Since cognitive aging is an extremely relevant phenomenon for modern societies and given the interplay between resting state activity and cognition, electrophysiology, which directly captures the result of neuronal activity, might represent a useful tool to delve into the mechanisms underlying cognitive decline in healthy aging. However, the pattern of association between neural oscillations and cognitive performance describing the lines above must be interpreted in light of their limitations. One aspect to consider is the different criteria used to define cognitive healthy aging across the studies. The World Health Organization [45], already in its 1946 Constitution, defined health as “a state of complete physical, mental and well-being and not merely the absence of disease or infirmity”. Later on, this definition was updated to reflect the capacity of individuals to reach their fullest potential, even in the presence of disease or where a complete state of well-being is not attainable [46]. Describing health in terms of physical and social functioning is particularly relevant when we talk about the aging population, where the absolute absence of disease appears utopian. Yet, the articles included in the review are based on exclusion criteria focused on mental and physical conditions such as history of mental illness, neurological disorders, and medical conditions. Seven studies consider the performance in screening tests as inclusion criteria, and only one considers the quality of life measure with a self-administered questionnaire that captures life satisfaction [27]. This could also partially explain some of the variability in the results observed in the literature and henceforth represent an important caveat that the field should seriously consider addressing in the future. There is a clear need to identify the best indicators of physical and social functioning and well-being in advanced age and to foster the standardization of the description of healthy aging across the scientific literature.
Another matter to regard is the scarce literature about the use of MEG in this field, despite its particularly appropriate use for studying neurocognitive processes. The hundreds of sensors used in MEG recordings have made it possible to identify and locate the source of brain oscillations [47]; due to the lack of interactions between the MEG signal and biological tissues, avoiding smearing and distortion outside of the scalp [48]. An improved signal-to-noise ratio and easier and more reliable source reconstruction process could also improve the consistency of future results.
An additional limitation that should be considered in future research is the use of cross-sectional designs. The cross-sectional studies provide only a snapshot of brain activity, and the picture can be enhanced by the development of longitudinal studies to track individual changes along the aging. Other aspects on the neurophysiological analysis level include the characterization of functional connectivity patterns and their identification as a potential neural marker for the diagnosis and discrimination of diseases such as Alzheimer’s disease [47]. In this sense, the development of studies focusing on cognitively unimpaired older adults and the use of techniques such as the MEG is a good spot to understand the brain patterns in healthy aging better. Along the same line, the number of participants recruited in the different studies is relatively small, and differences in age between groups limited the possibility of extrapolating the results in different populations.
Despite the difficulties described regarding the approach in characterizing of the general concept of healthy aging, this review sheds some light on the apparently inconsistent results of the electrophysiological fingerprints associated with cognitive performance during aging. The main conclusion of this review suggests that the APF could be considered an electrophysiological marker of healthy cognitive aging [49]. This is an easy-to-assess marker that could be used for monitoring deviations from central nervous system functioning [22], such is the case of the early stages of dementia, where the first pathological signs associated with the early stages of the disease appear decades before the cognitive decline [35], with the advantages that both measures could be registered during the resting-state condition, which is an easily replicable set-up, accessible to most populations. In this sense, assessing the resting state brain signal has wide appeal because this measure can be acquired in individuals whose cognitive ability or native language hinders testing with standard neuropsychological assessments, avoiding the cultural bias related to the cognitive test [50]. Furthermore, electrophysiological recordings, particularly using EEG, are relatively cheap and easy to transport, which makes this technology well-suited for most countries regardless of their economic power.
Altogether, our review provides the first approach to unveiling the mechanisms underlying and supporting normal cognitive functioning in the brain in the later stages of life. This understanding could be crucial for future global policymaking strategies aimed at preventing or treating cognitive impairment and promoting healthy aging.
AUTHOR CONTRIBUTIONS
Brenda Chino (Conceptualization; Methodology; Formal Analysis; Investigation; Writing-original Draft; Writing – Review & Editing; Visualization; Project administration; Funding acquisition); David López (Conceptualization; Formal Analysis; Methodology; Writing-original Draft); Sandra Doval (Formal Analysis; Methodology; Writing-original Draft; Funding acquisition); Lucía Torres- Simón: Methodology; Formal Analysis; Writing-original Draft; Funding acquisition); Jailsalmer de Frutos Lucas (Writing – Review & Editing); Lydia Giménez- Llort (Review; Editing; Supervision); Jonathan Zegarra (Writing- Review & Editing; Funding acquisition); Fernando Maestú (Resources; Writing – Review & Editing; Resources; Supervision).
ACKNOWLEDGMENTS
This work was supported by the predoctoral researchers grant from Universidad Complutense de Madrid (CT42/18-CT43/18 and CT63/19-CT64/19) cofounded by Santander bank, and National Council of Science, Technology and Technological Innovation (CONCYTEC, Perú) through the National Fund for Scientific and Technological Development (235-2018-FONDECYT). We thank the funding support from Universidad Señor de Sipán, Chiclayo, Perú.
FUNDING
The study was funded by Universidad Señor de Sipán, Chiclayo, Perú.
CONFLICT OF INTEREST
The authors have no conflict of interest to report.
DATA AVAILABILITY
No new data were created or analyzed during this study. Data sharing is not applicable to this article.
SUPPLEMENTARY MATERIAL
[1] The supplementary material is available in the electronic version of this article: https://dx.doi.org/10.3233/JAD-231009.
REFERENCES
[1] | Terry RD , Masliah E , Salmon DP , Butters N. , DeTeresa R. , Hill R. , Hansen L. A. , Katzman R. ((1991) ) Physical basis of cognitive alterations in Alzheimer’s disease: Synapse loss is the major correlate of cognitive impairment. Ann Neurol 30: , 572–580. |
[2] | Singer W ((2018) ) Neuronal oscillations: Unavoidable and useful? Eur J Neurosci 48: , 2389–2398. |
[3] | Jensen O , Spaak E , Zumer JM ((2014) ) Human brain oscillations: From physiological mechanisms to analysis and cognition. In Magnetoencephalography: From Signals to Dynamic Cortical Networks, Supek S, Aine C, eds. Springer, Berlin. |
[4] | Buzsáki G. ((2006) ) Rhythms of the brain. Oxford UniversityPress, New York. |
[5] | Gevins A , Smith ME ((2000) ) Neurophysiological measures of working memory and individual differences in cognitive ability and cognitive style. Cereb Cortex 10: , 829–839. |
[6] | Mitchell DJ , McNaughton N , Flanagan D , Kirk IJ ((2008) ) Frontal-midline theta from the perspective of hippocampal “theta”. Prog Neurobiol 86: , 156–185. |
[7] | Klimesch W , Doppelmayr M , Schwaiger J , Auinger P , Winkler T ((1999) ) “Paradoxical” alpha synchronization in a memory task. Brain Res Cogn Brain Res 7: , 493–501. |
[8] | Jensen O , Gelfand J , Kounios J , Lisman J ((2002) ) Oscillations in the alpha band (9–12Hz) increase with memory load during retention in a short-term memory task. Cereb Cortex 12: , 877–82. |
[9] | Hari R , Salmelin R ((1997) ) Human cortical oscillations: A neuromagnetic view through the skull. Trends Neurosci 20: , 44–49. |
[10] | Bonnefond M , Jensen O ((2012) ) Alpha oscillations serve to protect working memory maintenance against anticipated distracters. Curr Biol 22: , 1969–1974. |
[11] | Fox MD , Raichle ME ((2007) ) Spontaneous fluctuations in brain activity observed with functional magnetic resonance imaging. Nat Rev Neurosci 8: , 700–711. |
[12] | Stevens WD , Spreng RN ((2014) ) Resting-state functional connectivity MRI reveals active processes central to cognition. Wiley Interdiscip Rev Cogn Sci 5: , 233–245. |
[13] | Fingelkurts AA , Fingelkurts AA , Neves CF ((2010) ) . Natural world physical, brain operational, and mind phenomenal space-time. Phys Life Rev 7: , 195–249. |
[14] | Miller SA , Forrest JL ((2001) ) Enhancing your practice through evidence-based decision making: PICO, learning how to ask good questions. J Evid Base Dent Pract 1: , 136–141. |
[15] | Veritas Health Innovation. Covidence systematic review software. |
[16] | Moher D , Liberati A , Tetzlaff J , Altman DG; PRISMA Group ((2009) ) Preferred reporting items for systematic reviews and meta-analyses: The PRISMA statement. PLoS Med 6: , e1000097. |
[17] | Wirsching J , Graßmann S , Eichelmann F , Harms LM , Schenk M , Barth E , Berndzen A , Olalekan M , Sarmini L , Zuberer H , Aleksandrova K ((2018) ) Development and reliability assessment of a new quality appraisal tool for cross-sectional studies using biomarker data (BIOCROSS). BMC Med Res Methodol 18: , 122. |
[18] | Torres-Simón L , Doval S , Nebreda A , Llinas SJ , Marsh EB , Maestú F ((2022) ) Understanding brain function in vascularcognitive impairment and dementia with EEG and MEG: A systematic review. Neuroimage Clin 35: , 103040. |
[19] | Campbell M , McKenzie JE , Sowden A , Katikireddi SV , Brennan SE , Ellis S , Hartmann-Boyce J , Ryan R , Shepperd S , Thomas J , Welch V , Thomson H ((2020) ) Synthesis without meta-analysis (SWiM) in systematic reviews: Reporting guideline. BMJ 368: , l6890. |
[20] | Choi J , Ku B , You YG , Jo M , Kwon M , Choi Y , Jung S , Ryu S , Park E , Go H , Kim G , Cha W , Kim JU ((2019) ) Resting-state prefrontal EEG biomarkers in correlation with MMSE scores in elderly individuals. Sci Rep 9: , 10468. |
[21] | Kamal F , Campbell K , Taler V ((2022) ) Effects of the duration of a resting-state EEG recording in healthy aging and mild cognitive impairment. Clin EEG Neurosci 53: , 443–451. |
[22] | Finnigan S , Robertson IH ((2011) ) Resting EEG theta power correlates with cognitive performance in healthy older adults. Psychophysiology 48: , 1083–1087. |
[23] | Grandy TH , Werkle-Bergner M , Chicherio C , Lövdén M , Schmiedek F , Lindenberger U ((2013) ) Individual alpha peak frequency is related to latent factors of general cognitive abilities. Neuroimage 79: , 10–18. |
[24] | Fleck JI , Kuti J , Brown J , Mahon JR , Gayda-Chelder C ((2016) ) Frontal-posterior coherence and cognitive function in older adults. Int J Psychophysiol 110: , 217–230. |
[25] | Borhani S , Zhao X , Kelly MR , Gottschalk KE , Yuan F , Jicha GA , Jiang Y ((2021) ) Gauging working memory capacity from differential resting brain oscillations in older individuals with a wearable device. Front Aging Neurosci 13: , 625006. |
[26] | Richard Clark C , Veltmeyer MD , Hamilton RJ , Simms E , Paul R , Hermens D , Gordon E ((2004) ) Spontaneous alpha peak frequency predicts working memory performance across the age span. Int J Psychophysiol 53: , 1–9. |
[27] | Roca-Stappung M , Fernández T , Becerra J , Mendoza-Montoya O , Espino M , Harmony T ((2012) ) Healthy aging: Relationship between quantitative electroencephalogram and cognition. Neurosci Lett 510: , 115–120. |
[28] | Stacey JE , Crook-Rumsey M , Sumich A , Howard CJ , Crawford T , Livne K , Lenzoni S , Badham S ((2021) ) Age differences in resting state EEG and their relation to eye movements and cognitive performance. Neuropsychologia 157: , 107887. |
[29] | Trammell JP , MacRae PG , Davis G , Bergstedt D , Anderson AE ((2017) ) The relationship of cognitive performance and the theta-alpha power ratio is age-dependent: An EEG study of short-term memory and reasoning during task and resting-state in healthy young and old adults. Front Aging Neurosci 9: , 364. |
[30] | Cesnaite E , Steinfath P , Jamshidi Idaji M , Stephani T , Kumral D , Haufe S , Sander C , Hensch T , Hegerl U , Riedel-Heller S , Röhr S , Schroeter ML , Witte AV , Villringer A , Nikulin VV ((2023) ) Alterations in rhythmic and non-rhythmic resting-state EEG activity and their link to cognition in older age. Neuroimage 268: , 119810. |
[31] | Chino B , Cuesta P , Pacios J , de Frutos-Lucas J , Torres-Simón L , Doval S , Marcos A , Bruña R , Maestú F ((2023) ) Episodicmemory dysfunction and hypersynchrony in brain functional networksin cognitively intact subjects and MCI: A study of 379 individuals. Geroscience 45: , 477–489. |
[32] | Ferreira D , Correia R , Nieto A , Machado A , Molina Y , Barroso J ((2015) ) Cognitive decline before the age of 50 can be detected with sensitive cognitive measures. Psicothema 27: , 216–222. |
[33] | Harada CN , Natelson Love MC , Triebel KL ((2013) ) Normal cognitive aging. Clin Geriatr Med 29: , 737–752. |
[34] | Salthouse TA ((2009) ) When does age-related cognitive decline begin? Neurobiol Aging 30: , 507–514. |
[35] | Maestú F , Cuesta P , Hasan O , Fernandéz A , Funke M , Schulz PE ((2019) ) The importance of the validation of M/EEG with current biomarkers in Alzheimer’s disease. Front Hum Neurosci 13: , 17. |
[36] | Sporns O ((2013) ) Structure and function of complex brain networks. Dialogues Clin Neurosci 15: , 247–262. |
[37] | López-Sanz D , Bruña R , Garcés P , Camara C , Serrano N , Rodríguez-Rojo IC , Delgado ML , Montenegro M , López-Higes R , Yus M , Maestú F ((2016) ) Alpha band disruption in the AD-continuum starts in the subjective cognitive decline stage: A MEG study. Sci Rep 6: , 37685. |
[38] | Angelakis E , Lubar JF , Stathopoulou S , Kounios J ((2004) ) Peak alpha frequency: An electroencephalographic measure of cognitive preparedness. Clin Neurophysiol 115: , 887–897. |
[39] | Knyazeva MG , Barzegaran E , Vildavski VY , Demonet JF ((2018) ) Aging of human alpha rhythm. Neurobiol Aging 69: , 261–273. |
[40] | Garcés P , Vicente R , Wibral M , Pineda-Pardo JÁ , López ME , Aurtenetxe S , Marcos A , de Andrés ME , Yus M , Sancho M , Maestú F , Fernández A ((2013) ) Brain-wide slowing ofspontaneous alpha rhythms in mild cognitive impairment. FrontAging Neurosci 5: , 100. |
[41] | Fernández A , Hornero R , Mayo A , Poza J , Gil-Gregorio P , Ortiz T ((2006) ) MEG spectral profile in Alzheimer’s disease and mildcognitive impairment. Clin Neurophysiol 117: , 306–314. |
[42] | de Frutos-Lucas J , López-Sanz D , Zuluaga P , Rodríguez-Rojo IC , Luna R , López ME , Delgado-Losada ML , Marcos A , Barabash A , López-Higes R , Maestú F , Fernández A ((2018) ) Physical activity effects on the individual alpha peak frequency of older adults with and without genetic risk factors for Alzheimer’s Disease: A MEG study. Clin Neurophysiol 129: , 1981–1989. |
[43] | Tóth B , Kardos Z , File B , Boha R , Stam CJ , Molnár M ((2014) ) Frontal midline theta connectivity is related to efficiency of WM maintenance and is affected by aging. Neurobiol Learn Mem 114: , 58–69. |
[44] | Ariza P , Solesio-Jofre E , Martínez JH , Pineda-Pardo JA , Niso G , Maestú F , Buldú JM ((2015) ) Evaluating the effect of aging on interference resolution with time-varying complex networks analysis. Front Hum Neurosci 9: , 250. |
[45] | World Health Organization (2024) Constitution. World Health Organization. https://www.who.int/about/accountability/governance/constitution. |
[46] | World Health Organization (2024). The 1st International Conference on Health Promotion, Ottawa, 1986. World Health Organization. https://www.who.int/about/accountability/governance/constitution. |
[47] | Maestú F , Solesio-Jofre E , Bajo R ((2019) ) Towards the understanding of healthy and pathological aging through MEG. In Magnetoencephalography: From Signals to Dynamic Cortical Networks, Supek S, Aine C, eds. Springer, Berlin. |
[48] | Baillet S ((2017) ) Magnetoencephalography for brain electrophysiology and imaging. Nat Neurosci 20: , 327–339. |
[49] | Drago V , Babiloni C , Bartrés-Faz D , Caroli A , Bosch B , Hensch T , Didic M , Klafki HW , Pievani M , Jovicich J , Venturi L , Spitzer P , Vecchio F , Schoenknecht P , Wiltfang J , Redolfi A , Forloni G , Blin O , Irving E , Davis C , Hårdemark HG , Frisoni GB ((2011) ) Disease tracking markers for Alzheimer’s disease at the prodromal (MCI) stage. J Alzheimers Dis 3: , 159–199. |
[50] | Rosazza C , Minati L ((2011) ) Resting-state brain networks: Literature review and clinical applications. Neurol Sci 32: , 773–785. |