The Mediating Roles of Neurobiomarkers in the Relationship Between Education and Late-Life Cognition
Abstract
Background:
The mediating roles of neuropathologies and neurovascular damage in the relationship between early-life education and later-life cognitive function are unknown.
Objective:
To examine whether Alzheimer’s and neurovascular biomarkers mediate the relationships between education and cognitive functions.
Methods:
Data were from 537 adults aged 55–94 in the Alzheimer’s Disease Neuroimaging Initiative 3. We tested whether the relationships between education (continuous, years) and cognitive function (memory, executive functioning, and language composites) were mediated by neuroimaging biomarkers (hippocampal volumes, cortical gray matter volumes, meta-temporal tau PET standard uptake value ratio, and white matter hyperintensity volumes). Models were adjusted for age, race, sex/gender, cardiovascular history, body mass index, depression, and Apolipoprotein E-ɛ4 status.
Results:
Hippocampal volumes and white matter hyperintensities partially mediated the relationships between education and cognitive function across all domains (6.43% to 15.72% mediated). The direct effects of education on each cognitive domain were strong and statistically significant.
Conclusions:
Commonly measured neurobiomarkers only partially mediate the relationships between education and multi-domain cognitive function.
INTRODUCTION
In their Strategic Directions for Research, 2020–2025, the National Institute on Aging (NIA) identified that understanding “how social and economic factors throughout the lifespan affect health and well-being at older ages” is critical to informing interventions and policy decisions [1]. Education has long been identified as a protective factor against cognitive aging and Alzheimer’s disease and related dementias (ADRD) risk [2, 3]. Greater educational attainment is linked to higher levels of performance across cognitive domains, including semantic memory, executive functioning, and episodic memory, and findings are consistent across cross-sectional studies [4–6].
However, mechanisms accounting for the protective effect of education on cognitive performance are not fully understood, and longitudinal findings are mixed with some observing a continued protective effect of education against cognitive declines [7], others reporting null findings [8–10], and still others identifying a sharper decline in cognition among those with higher education as individuals progress to ADRD [11]. Education as it relates to late-life cognition is often viewed through a cognitive reserve lens [12, 13]. That is, higher experiential resources, such as education preserves cognitive functioning in the presence of brain atrophy and/or ADRD pathology potentially through differences in neural activation patterns and recruitment of brain networks [12–17]. However, in studies that incorporate biomarkers of brain atrophy or pathology, the relationships between education and cognition vary and may contradict the cognitive reserve hypothesis [11, 18, 19]. For example, Bauer and colleagues detected direct relationships between education and cognition, but higher education did not mitigate the relationship between ADRD biomarkers and cognition in a sample of individuals with varying levels of cognitive impairment [18]. Although education may not modify the relationships between ADRD biomarkers with cognition, it may preserve cognition by increasing neurogenesis early in life which could then increase brain reserve [12, 13, 20, 21]. In the presence of greater brain reserve (e.g., larger brain volumes), one may sustain more structural change before reaching a level of brain atrophy/pathology that correlates with notable cognitive declines or clinically significant symptoms of ADRD [13].
The pathway by which established ADRD biomarkers link education to cognitive functioning is somewhat unclear. Albeit mixed, the existing literature suggests that higher education is associated with larger global and regional (e.g., hippocampal) brain volumes and fewer neurofibrillary tangles (NFTs), particularly in cognitively healthy or preclinical ADRD stages, but education is less likely to be associated with white matter hyperintensity (WMH) volumes [22–26]. Several of these neuroimaging measures, including cerebral atrophy, presence of WMHs, and tau NFTs (measured as tau deposition from positron emission tomography (PET)) are associated with increased risk for cognitive impairment and ADRD [27–32]. NFTs found in Alzheimer’s disease (AD) are known to result from hyperphosphorylation of tau, with the abnormal accumulation of tau resulting in NFTs [33]. NFTs and hyperphosphorylated tau are typically first detected in the transentorhinal region of the mesial temporal lobe (Braak Stage I) [34] and correlate with cognitive symptoms later in the progression of AD [31, 35]. Prior studies related to this topic have been small but suggest that the relationship between education and cognitive function was not mediated by total brain nor hippocampal volumes [6] but other markers of brain structure and function (e.g., white matter integrity) may play a role[36].
Conflicting evidence of an ADRD biomarker pathway may also partially reflect heterogeneity in the cognitive domains assessed across studies or chance due to small and selected samples [6, 37]. The relationships between education and cognitive function may differ by domain as a function of biomarker-specific mediating pathways. Ischemic damage to the cerebrovasculature in the form of WMHs is more strongly associated with speed-dependent measures of perceptual-motor skills and executive functioning than measures of memory [38–41]. In contrast, greater hippocampal atrophy is more strongly associated with declines in episodic memory than in executive functioning [42, 43]. Language abilities are strongly linked to education, and certain tasks (e.g., word reading) may function as a proxy for education [44]. Although some language abilities (e.g., confrontation naming, word reading) are relatively well preserved in healthy aging and typical AD, others (e.g., verbal fluency) are susceptible to declines with age and preclinical ADRD [45, 46]. Therefore, we aimed to investigate whether ADRD biomarkers mediate the relationships between education and three cognitive domains (i.e., memory, executive functioning, and language).
MATERIALS AND METHODS
Study population
We used data from the third cohort of the Alzheimer’s Disease Neuroimaging Initiative (ADNI 3) database, collected from 2016 to 2021. Data were obtained from the ADNI database (http://adni.loni.usc.edu). The ADNI was launched in 2003 as a public-private partnership, led by Principal Investigator Michael W. Weiner, MD. The primary goal of ADNI has been to test whether serial magnetic resonance imaging (MRI), PET, other biological markers, and clinical and neuropsychological assessment can be combined to measure the progression of mild cognitive impairment (MCI) and early AD. For up-to-date information, see http://www.adni-info.org. ADNI 3 is a continuation of the initial ADNI multi-site study. ADNI 3 retained some participants from ADNI 2 and enrolled new participants (ages 55–90 years) including individuals who were cognitively healthy or were living with amnestic MCI or dementia. A complete description of the ADNI 3 study protocol was reported by Weiner and colleagues [47]. For the current study, only baseline data from newly enrolled ADNI 3 participants are used. From a total of 819 newly enrolled ADNI 3 participants who participated in neuroimaging, participants were excluded if they were missing information on education (n = 2), gray matter volume (n = 1), tau (n = 77), WMHs (n = 8), total brain volume (n = 2), cognitive domain measures (n = 8), or any covariates (n = 192) for a final analytic sample of 537 participants. Local Institutional Review Boards at each site approved ADNI study protocols. Participants or authorized representatives provided informedconsent.
Education (exposure)
Years of education was self-reported by the participant or study partner.
Neuroimaging measures (mediators)
The present study used processed neuroimaging values from the ADNI database. Detailed procedure on scan acquisition and optimization are provided elsewhere (www.adni.lon.i.usc.edu/pages/access/studyData.jsp). In brief, participants underwent whole-brain MRI scanning using 3.0- Tesla MRI. Scans of interest were the T1-weighted scan to measure whole and regional brain volumes and to provide spatial information for PET scans. ADNI MRI processing methods, including cortical reconstruction and volumetric segmentation, were based on previously published FreeSurfer techniques [48, 49] using FreeSurfer image analysis (version 5.1; http://surfer.nmr.mgh.harvard.edu/). Regional segmentations (e.g., the hippocampus) were identified according to an atlas defined by FreeSurfer. WMH volumes also required fluid-attenuation inversion recovery (FLAIR) sequences. The WMH measurement approach has been described in detail on the ADNI site (http://adni.loni.usc.edu/pages/access/studyData.jsp). In short, 1) non-brain structures were removed from the 3D T1 images prior to measurement using an automated atlas based method; 2) Preserved parallel relationships of the FLAIR image is then transformed to the 3DT1 image using the FLIRT method from the FSL tool box; 3) nonuniform correction of the 3D T1 is performed; 4) Next, the image is molded using a spatially smooth thin-plate spline interpolation; 5) Prior to WMH calculation, each 3D T1 image is non-linearly aligned to a common template atlas, and each of the accompanying images are transformed onto the same atlas; 6) estimation of WMH is then performed using a modified Bayesian probability structure based on a previously published method of histogram fitting [50]. Raw WMH volumes were log-transformed. Raw gray matter volumes (i.e., total cortical gray and bilateral hippocampal volumes) and log transformed WMH volumes were each adjusted for total brain volume by regressing each imaging measure on total brain volume. Each adjusted imaging measure was then z-standardized to a mean value of 0 and standard deviation of 1.
Participants underwent PET imaging at their initial ADNI 3 visit. Tau PET data was obtained using the ligand [18F]-T807 (AV-1451). ADNI investigators applied a region of interest based approach to measure standard uptake value ratio in areas that represented Braak stages [47]. For the purposes of this study, we examined tau within the meta-temporal region of interest [51].
Cognitive scores (outcomes)
Participants completed a battery of neuropsychological tests and standardized, composite scores for memory, executive functioning, and language were previously developed by investigators using item-response theory methods and confirmatory factor analysis [45, 52, 53]. In brief, measures for the memory composite included the Rey Auditory Verbal Learning Test (RAVLT; including List B, immediate and delayed recalls, and recognition); Alzheimer’s Disease Assessment Scale-Cognitive subscale (ADAS-Cog) list learning, recall, and recognition; Logical Memory immediate and delayed recalls; and three word recall items from the Mini-Mental State Exam (MMSE) [52]. Measures for the executive functioning composite included Wechsler Adult Intelligence Scale (WAIS-R) Digit Symbol Substitution, Digit Span Backwards, Trails Making Test A & B, Category Fluency (Animals and Vegetables), and Clock Drawing [53]. Measures for the language composite included the Boston Naming Test, Animal Fluency, language items from the MMSE (i.e., object naming, sentence repetition, sentence reading, writing, and following a 3-step command), language items from the ADAS-Cog (i.e., commands, object naming, and ideational praxis), and language items from the Montreal Cognitive Assessment (i.e., phonemic fluency and sentence repetition) [45].
Covariates
Covariates considered to be possible confounders of the relationship between education and cognitive function were: age at the ADNI 3 baseline interview (continuous, years) sex/gender (male; female), self-reported race (considered as White or non-White due to insufficient sample sizes of individuals from distinct non-White backgrounds). We conceptualize racial identity as a social construct, developed and maintained to sustain a social hierarchy. It is relevant in this analysis because the historical and ongoing processes of structural racism create substantial race-based inequality in educational access and numerous other exposures that may influence brain aging. We also considered additional possible confounders of the mediator-outcome relationships: depressive symptoms within the past week (continuous score on the 15-item Geriatric Depression Scale), history of cardiovascular disease (a binary indicator of any self-reported cardiovascular disease, hypertension, stroke, or diabetes), body mass index (kg/m2, continuous), and APOE ɛ4 status (carriers versus non-carriers).
Statistical analysis
We fit linear regression models to assess the total effect (TE) of education on each cognitive outcome with each domain evaluated in separate models. We then used causal mediation analyses to estimate the neuroimaging measures as mediators between education and each cognitive domain [54, 55]. Each neuroimaging measure was included in separate models to estimate the natural direct effect (NDE) of education not mediated by that neuroimaging measure. The natural indirect effect (NIE) of education on cognitive functioning mediated by each neuroimaging measures was estimated by calculating the TE minus the NDE. We sequentially added covariates to assess each mediator. Model 1 included age, race, APOE ɛ4 status, and sex/gender as confounders. Model 2 additionally adjusted for cardiovascular disease history, depressive symptoms, and body mass index. See Fig. 1 for a depiction of the analytic model. All primary analyses were conducted using SAS 9.4, including PROC GENMOD and PROC CAUSALMED. We ran two secondary analyses. First, given that the relationships between education, biomarkers, and cognition may vary by cognitive status [11], we re-ran our primary mediation models excluding 55 individuals with dementia (n = 482). Second, we tested for multiple mediation using a regression-based approach in R [56]. For each outcome of interest, a model was run with all mediators and full covariates adjustment.
RESULTS
Descriptives
Participants were 73 years of age on average, 53% were women, the majority were White (91%), and highly educated, with 73% of participants completing at least a 4-year college degree (Table 1).
Table 1
ADNI 3 Descriptive Characteristics
N = 537 | |
Age, mean (sd) | 73 (8) |
Sex/gender, N (%) | |
Male | 252 (46.93) |
Female | 285 (53.07) |
Race, N (%) | |
White | 488 (90.88) |
Non-White* | 49 (9.12) |
Years of Education, N (%) | |
High school or less | 53 (9.87) |
Some college or associate | 94 (17.50) |
College degree | 132 (24.58) |
Graduate education | 258 (48.04) |
Health History, N (%) | |
No CVD factors | 303 (56.42) |
Any CVD, stroke, diabetes, or hypertension | 234 (43.58) |
Body Mass Index, mean (sd) | 27.53 (5.81) |
Depression, mean(sd) | 1.23 (1.54) |
APOE ɛ4, N (%) | |
Any | 191 (35.57) |
None | 346 (64.43) |
*Non-White participants consisted of 1 American Indian or Alaskan Native (0.19%) participant, 9 Asian (1.68%) participants, 30 Black or African American (5.59%) participants, and 9 multiracial (1.68%) participants. ADNI, Alzheimer’s Disease Neuroimaging Initiative; APOE ɛ4, Apolipoprotein E ɛ4 allele; CVD, cardiovascular disease; sd, standard deviation
Primary analysis
Education was associated with better performance on memory (est = 0.08, 95% CI: 0.06, 0.11), executive functioning (est = 0.10, 95% CI: 0.07, 0.14), and language composites (est = 0.11, 95% CI: 0.08, 0.14) in models adjusting for age, sex/gender, race, and APOE ɛ4 status. Additionally, higher education level was associated with higher hippocampal volume (est = 0.05, 95% CI: 0.01, 0.08) and gray matter volumes (est = 0.04, 95% CI: 0.00, 0.07) and lower tau (est = –0.03, 95% CI: –0.07, –0.00) and WMH burden (est = –0.05, 95% CI: –0.08, –0.02; Supplementary Tables 1–3).
Fig. 1
Hypothesized causal structure guiding choice of covariates in models to test mediation of the relationship between education and cognition by neuroimaging biomarkers. APOE4, Apolipoprotein E ɛ4 allele; BMI, body mass index.
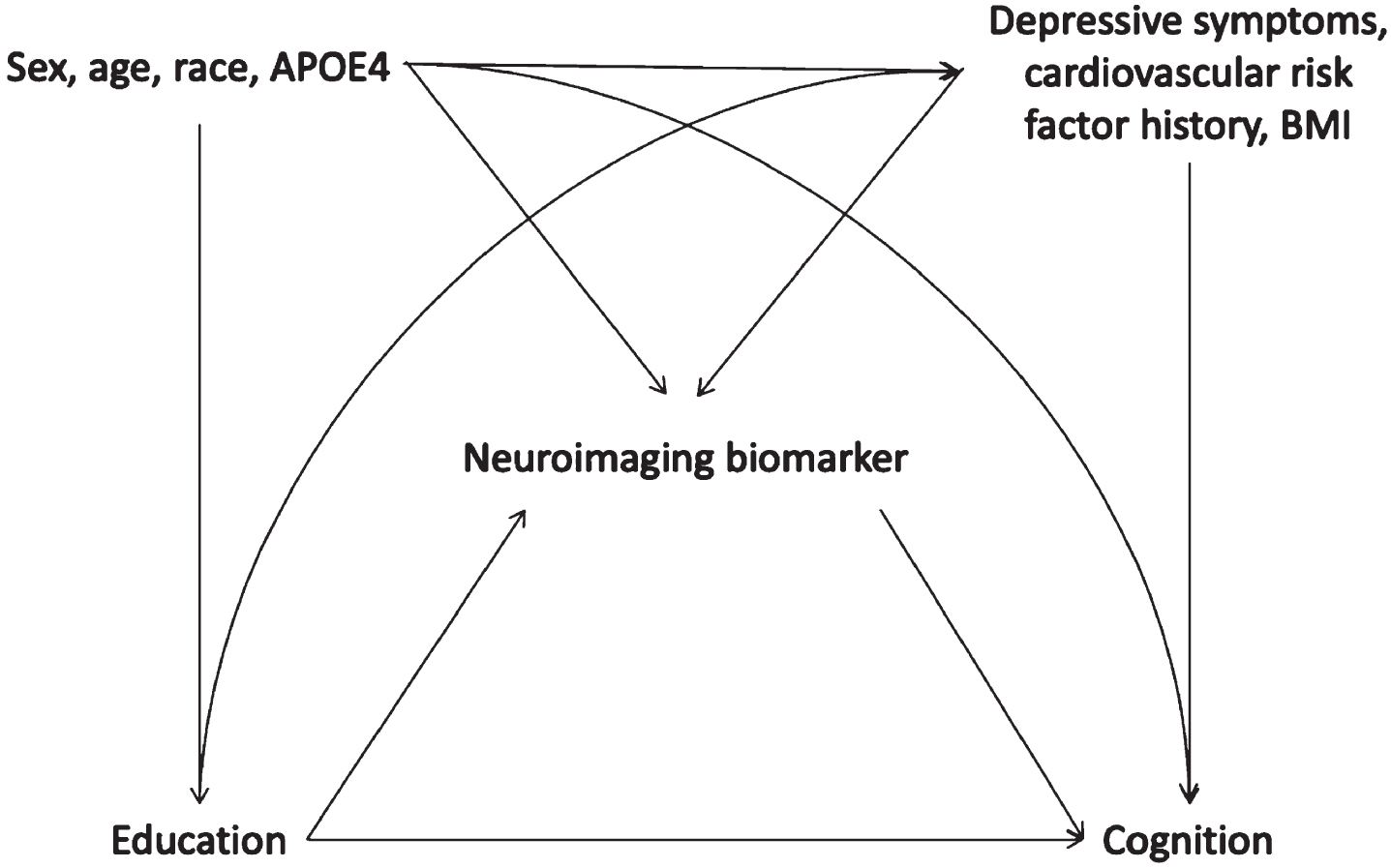
In fully adjusted models, hippocampal volume was estimated to mediate 15.7% (95% CI: 3.23, 28.22) of the relationship between education and memory and WMH volume to mediate 9.2% (95% CI: 1.73, 16.72) (Fig. 2 and Supplementary Table 1). Cortical gray matter volume was estimated to mediate 6.3% of the relationship between education and memory but the fully adjusted confidence interval (95% CI: –0.70, 13.27) included zero, consistent with no mediating role for gray matter volume. Likewise, presence of tau was estimated to mediate 14.3% of the relationship between education and memory but the confidence interval (95% CI: –0.30, 28.80) also included zero.
Hippocampal volume was estimated to mediate 14.5% (95% CI: 2.34, 26.64) of the effect of education on executive function and WMH to mediate 9.1% (95% CI: 1.18, 16.95) (Fig. 3 and Supplementary Table 2). Estimated mediation fractions were similar for cortical gray matter (11.9%, 95% CI: –0.24, 24.00) and tau (15.4%, 95% CI: –0.36, 31.21), although both CIs were wide and includedzero.
Fig.2
Direct and indirect effects between education, ADRD biomarkers, and memory in fully adjusted models. Fully adjusted models included the following covariates: age, sex/gender, race, apolipoprotein ɛ4 status (carriers and non-carriers), depressive symptoms (continuous score on the 15-item Geriatric Depression Scale), history of cardiovascular disease (a binary indicator of any self-reported cardiovascular disease, hypertension, stroke, or diabetes), and body mass index (kg/m2, continuous). ADRD, Alzheimer’s disease and related dementias; TE, total effect; NDE, natural direct effect; NIE, natural indirect effect; HV, hippocampal volumes; CGM, cortical gray matter volumes; WMH, white matter hyperintensities.
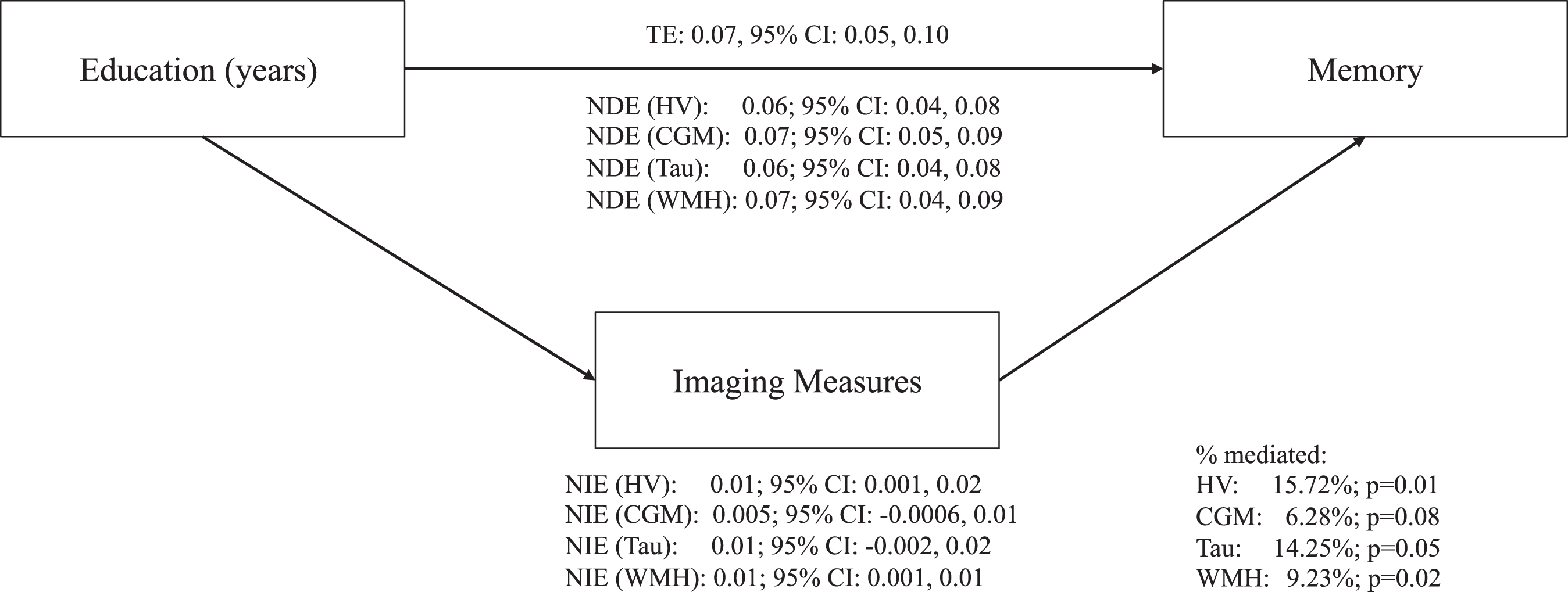
Fig.3
Direct and indirect effects between education, ADRD biomarkers, and executive functioning in fully adjusted models. Fully adjusted models included the following covariates: age, sex/gender, race, apolipoprotein ɛ4 status (carriers and non-carriers), depressive symptoms (continuous score on the 15-item Geriatric Depression Scale), history of cardiovascular disease (a binary indicator of any self-reported cardiovascular disease, hypertension, stroke, or diabetes), and body mass index (kg/m2, continuous). ADRD, Alzheimer’s disease and related dementias; TE, total effect; NDE, natural direct effect; NIE, natural indirect effect; HV, hippocampal volumes; CGM, cortical gray matter volumes; WMH, white matter hyperintensities.
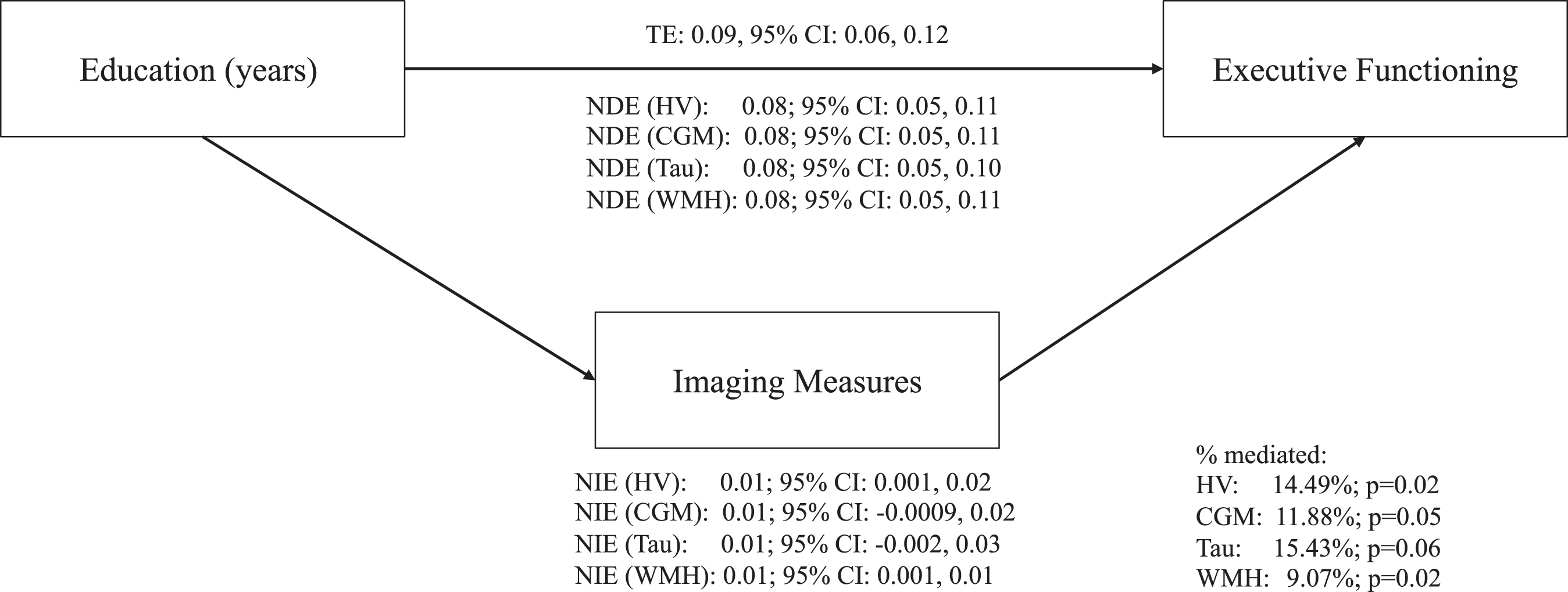
Fig.4
Direct and indirect effects between education, ADRD biomarkers, and language in fully adjusted models. Fully adjusted models included the following covariates: age, sex/gender, race, apolipoprotein ɛ4 status (carriers and non-carriers), depressive symptoms (continuous score on the 15-item Geriatric Depression Scale), history of cardiovascular disease (a binary indicator of any self-reported cardiovascular disease, hypertension, stroke, or diabetes), and body mass index (kg/m2, continuous). ADRD, Alzheimer’s disease and related dementias; TE, total effect; NDE, natural direct effect; NIE, natural indirect effect; HV, hippocampal volumes; CGM, cortical gray matter volumes; WMH, white matter hyperintensities.
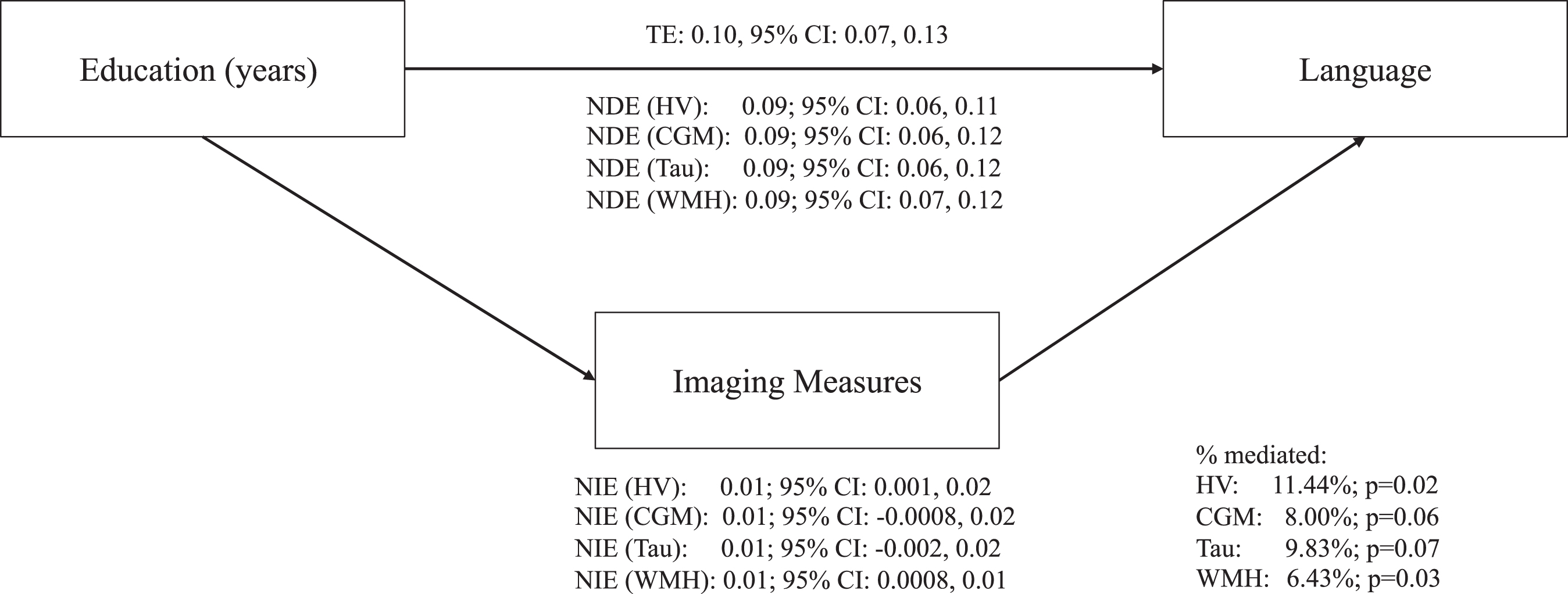
Hippocampal and WMH volumes were estimated to partially mediate the relationship of education with language in fully adjusted models (hippocampus: 11.4%, 95% CI: 1.91, 20.97; WMH: 6.4%, 95% CI: 0.75, 12.10) (Fig. 4 and Supplementary Table 3). Neither cortical gray matter nor tau significantly mediated the relationship between education with executive functioning after accounting for health risk factors in fully adjusted models (cortical gray matter: 8.0%, 95% CI: –0.38, 16.38; tau: 9.8%, 95% CI: –0.74,20.39).
Secondary analyses
Dementia-free sample: WMH volumes remained a significant mediator of the relationships between education and each cognitive composite in fully adjusted models (memory: 8.8%, 95% CI: 1.13, 16.41; executive functioning: 9.1%, 95% CI: 0.40, 17.84; and language; 5.9%, 95% CI: 0.12, 11.71). The relationships between education and each cognitive composite were not mediated by hippocampal volumes, cortical gray matter, or tau (Supplementary Tables 4–6).
Multiple mediation on overall sample: In fully adjusted multiple mediation analysis, ADRD biomarkers collectively mediated 21% (95% CI: 0.15, 0.28) of the proportion of the relationship between education and memory. The ADRD biomarkers collectively accounted for 25% (95% CI: 0.26, 0.31) and 20% (95% CI: 0.18,0.22) of the associations between education with executive functioning and language, respectively.
DISCUSSION
In the ADNI 3 cohort, higher education was associated with better cognitive functioning, an association partly mediated via larger hippocampal and smaller WMH volumes. Patterns were similar across cognitive domains of memory, executive functioning, and language. Our findings suggest major mechanisms via which education influences later life cognition are not captured in typical neuroimaging measures of brain health. Further, these results underscore the importance of education for later-life brain and cognitive health, suggesting that education continues to play a significant role in preserving brain integrity as one ages. Our findings were robust to control for multiple factors associated with poor brain health, including APOE ɛ4 status.
Our data support the notion that education may confer brain reserve as evidenced by lower ADRD biomarkers which were associated with better cognition. Given that ADRD biomarkers only partially mediated the relationship between education with cognitive functioning, education may largely operate through additional mechanisms, such as cognitive reserve which should be tested using longitudinal data [12, 13]. Existing literature corroborates a protective effect of education on cognitive function even in the presence of brain atrophy/pathology [16, 26, 57, 58], although findings are inconsistent [18].
Individual ADRD biomarkers were relatively similar in the extent to which they connected education to cognitive functioning with two out of four biomarkers (hippocampal and WMH volumes) reaching significance. Further, accounting for multiple ADRD biomarker pathways resulted in only slight increases in the percent of the relationships between education and each cognitive domain mediated, suggesting more overlap than independence across ADRD biomarkers. The hippocampus has a critical role in memory functioning and, to a lesser extent, has been associated with executive functioning and language [43]. Individuals with more years of education also had larger hippocampal volumes in a population of cognitively normal older adults [59] and across the lifespan [25]. Likewise, extensive training in spatial navigation was associated with larger posterior hippocampus volumes among London Taxi drivers, underscoring the connection between cognitive engagement and temporal lobe structure [60]. Education may trigger a feedback process, in which higher education preserves hippocampal volume and preserved hippocampal integrity promotes continued cognitive engagement. This in turn preserves hippocampal integrity and promotes plasticity.
Small vessel cerebrovascular damage (i.e., WMH volumes) also linked education to cognitive functioning with a nominally greater impact on memory, followed by executive functioning, and then language. Existing literature on WMHs indicates a stronger, negative relationship between white matter hyperintensities and executive functioning relative to memory [38–40]. Parks et al. [61] examined a mediational model of the relationships among WMHs and cognition in older adults and proposed an indirect effect of WMHs on episodic memory, one mediated by executive function and hippocampal volumes. With regards to education, there appears to be a negative effect of WMH on cognition particularity among those with lower education and not those with higher education with the same severity of WMH [62, 63].
Although point estimates suggest that NFTs in the temporal lobe and whole brain cortical gray matter volumes mediated a similar percentage of the association between education with cognition as hippocampal and WMH volumes, these relationships were not statistically significant. Larger samples are needed to precisely estimate the role of NFT and tau in mediating education effects on cognition, although our results rule out that these variables account for a large fraction of the effect.
Notably, when we excluded individuals with dementia, WMH volumes, but not hippocampal volumes, remained a robust mediator of the relationship between education and cognition. It is possible that the individuals with dementia were more likely to have an AD etiology which, when excluded, lowered our ability to detect mediation by hippocampal volumes to a greater extent than WMH volumes. Alternatively, by excluding a cognitive status group (i.e., individuals with dementia), other statistical challenges (e.g., a collider variable) may have been introduced [64]. This is possible given that, 1) higher education is linked to higher likelihood of dementia diagnosis due to greater access to healthcare and research studies, 2) neurobiomarkers are considered in dementia status/diagnosis, and similarly 3) dementia status/diagnosis is defined by cognitive status. Future studies with larger sample sizes are needed to test potential modification by cognitive status and the role of varying etiology (e.g., AD, vascular, mixed) among individuals with dementia.
In the present study, education was directly related with performance in each cognitive domain (memory, executive functioning, and language). Though not formally tested, the direct association of education with cognition was nominally largest for language, followed by executive functioning, then memory. Education may confer the most protection on the language domain which is consistent with other findings in the literature concerning the protective effects of education on cognition via preservation of white matter integrity [65]. However, it is more likely that the brain regions involved in language processing are less susceptible to changes from aging and ADRD pathology [34]. Additionally, education and specific language tests which measure crystallized abilities (e.g., vocabulary/semantic knowledge, a component of the ADNI language composite score) have a strong premorbid relationship. In fact, such crystallized language measures are often used to operationalize education and language [66]. Therefore, longitudinal data, separate analyses by cognitive status, and/or data breaking down the relationship between education with each language test (i.e., separating crystalized from fluency measures) would be necessary to clarify whether language abilities are uniquely protected by education in the face of ADRD pathology.
Limitations
ADNI 3 is a non-representative, highly educated sample of individuals who nearly all identify as White race/ethnicity. Generalizability to more diverse samples is unclear [67–69]. Future studies should incorporate more diverse samples. Educational attainment may be confounded by other social and economic factors that were not accounted for in this study. Multiple social factors such as low economic stability, lack of health care access, and increased stress are proposed to interact with one another in producing limited psychosocial resources or allostatic load [70], ultimately affecting later-life cognition and brain integrity [71, 72]. The ADRD biomarker mediation estimates in our study were relatively small. Given this limitation, we were also unable to conduct sensitivity analysis by other relevant demographic factors, such as sex/gender which may modify the associations between education, ADRD biomarkers, and cognition [73]. Finally, we examined cognition at one timepoint, so we are unable to determine whether ADRD biomarkers mediate the associations between education with rates of cognitive decline or conversion to dementia. Despite these limitations, our study has a number of strengths, including examination of a relatively large sample with data on multiple ADRD biomarkers derived from multimodal neuroimaging data. Additionally, cognitive outcomes were well-characterized with each cognitive domain reflecting a composite score; thus, improving stability and reducing the likelihood of a type I error [52]. Finally, our use of mediation analytic methods goes beyond simple regression to explain potential pathways by which education impacts late life cognition.
Conclusion
Among a predominantly White sample of middle-aged and older adults in the US, higher education was associated with better memory, executive functioning, and language domains. These relationships were partially attributable to larger hippocampal and smaller WMH volumes among those with higher education. Hippocampal volumes were a consistent mediator of the relationships between education with cognitive function across multiple domains. Our findings warrant further investigation into the relationships between education, ADRD biomarkers, and later-life cognitive functioning in more diverse and population-based cohorts.
ACKNOWLEDGMENTS
Data collection and sharing for this project was funded by the Alzheimer’s Disease Neuroimaging Initiative (ADNI) (National Institutes of Health Grant U01 AG024904) and DOD ADNI (Department of Defense award number W81XWH-12-2-0012). ADNI is funded by the NIA, the National Institute of Biomedical Imaging and Bioengineering, and through generous contributions from the following: AbbVie, Alzheimer’s Association; Alzheimer’s Drug Discovery Foundation; Araclon Biotech; BioClinica, Inc.; Biogen; Bristol-Myers Squibb Company; CereSpir, Inc.; Cogstate; Eisai Inc.; Elan Pharmaceuticals, Inc.; Eli Lilly and Company; EuroImmun; F. Hoffmann-La Roche Ltd and its affiliated company Genentech, Inc.; Fujirebio; GE Healthcare; IXICO Ltd.; Janssen Alzheimer Immunotherapy Research & Development, LLC.; Johnson & Johnson Pharmaceutical Research & Development LLC.; Lumosity; Lundbeck; Merck & Co., Inc.;Meso Scale Diagnostics, LLC.; NeuroRx Research; Neurotrack Technologies; Novartis Pharmaceuticals Corporation; Pfizer Inc.; Piramal Imaging; Servier; Takeda Pharmaceutical Company; and Transition Therapeutics. The Canadian Institutes of Health Research is providing funds to support ADNI clinical sites in Canada. Private sector contributions are facilitated by the Foundation for the National Institutes of Health (http://www.fnih.org). The grantee organization is the Northern California Institute for Research and Education, and the study is coordinated by the Alzheimer’s Therapeutic Research Institute at the University of Southern California. ADNI data are disseminated by the Laboratory for Neuro Imaging at the University of Southern California.
FUNDING
This work is supported by Advanced Psychometrics conference (NIA grant R13 AG030995). PAA is supported by funds from the Alzheimer’s Diseases and Related Dementias T32 training fellowships (NIH/NIA grant 5T32AG061892-04, T32AG020499-18). AMS is supported by funds from the NIH (K08AG075351, L30AG074401, U54CA267789). RC is supported in part by the NIA (4K00AG068431-02). CWE is supported by the NIA (F31AG062114).
CONFLICT OF INTEREST
The authors have no conflict of interest to report.
DATA AVAILABILITY
The data used to conduct the present analyses are available through the Alzheimer’s Disease Neuroimaging Initiative (ADNI) database (see http://adni.loni.usc.edu).
SUPPLEMENTARY MATERIAL
[1] The supplementary material is available in the electronic version of this article: https://dx.doi.org/10.3233/JAD-230244.
REFERENCES
[1] | National Institute on Aging ((2020) ) The National Institute on Aging: Strategic Directions for Research, 2020–2025. |
[2] | Caamano-Isorna F , Corral M , Montes-Martinez A , Takkouche B ((2006) ) Education and dementia: A meta-analytic study. Neuroepidemiology 26: , 226–232. |
[3] | Livingston G , Huntley J , Sommerlad A , Ames D , Ballard C , Banerjee S , Brayne C , Burns A , Cohen-Mansfield J , Cooper C , Costafreda SG , Dias A , Fox N , Gitlin LN , Howard R , Kales HC , Kivimäki M , Larson EB , Ogunniyi A , Orgeta V , Ritchie K , Rockwood K , Sampson EL , Samus Q , Schneider LS , Selbæk G , Teri L , Mukadam N ((2020) ) Dementia prevention, intervention, and care: 2020 report of the Lancet Commission. Lancet 396: , 413–446. |
[4] | Alley D , Suthers K , Crimmins E ((2007) ) Education and cognitive decline in older Americans: Results from the AHEAD sample. Res Aging 29: , 73–94. |
[5] | Opdebeeck C , Martyr A , Clare L ((2016) ) Cognitive reserve and cognitive function in healthy older people: A meta-analysis. Neuropsychol Dev Cogn B Aging Neuropsychol Cogn 23: , 40–60. |
[6] | Mungas D , Reed BR , Farias ST , Decarli C ((2009) ) Age and education effects on relationships of cognitive test scores with brain structure in demographically diverse older persons. Psychol Aging 24: , 116–128. |
[7] | Reas ET , Laughlin GA , Bergstrom J , Kritz-Silverstein D , Barrett-Connor E , McEvoy LK ((2017) ) Effects of sex and education on cognitive change over a 27-year period in older adults: The Rancho Bernardo Study. Am J Geriatr Psychiatry 25: , 889–899. |
[8] | Zahodne LB , Glymour MM , Sparks C , Bontempo D , Dixon RA , MacDonald SW , Manly JJ ((2011) ) Education does not slow cognitive decline with aging: 12-year evidence from the victoria longitudinal study. J Int Neuropsychol Soc 17: , 1039–1046. |
[9] | Early DR , Widaman KF , Harvey D , Beckett L , Park LQ , Farias ST , Reed BR , DeCarli C , Mungas D ((2013) ) Demographic predictors of cognitive change in ethnically diverse older persons. Psychol Aging 28: , 633–645. |
[10] | Wilson RS , Yu L , Lamar M , Schneider JA , Boyle PA , Bennett DA ((2019) ) Education and cognitive reserve in old age. Neurology 92: , e1041–e1050. |
[11] | Mungas D , Gavett B , Fletcher E , Farias ST , DeCarli C , Reed B ((2018) ) Education amplifies brain atrophy effect on cognitive decline: Implications for cognitive reserve. Neurobiol Aging 68: , 142–150. |
[12] | Stern Y , Arenaza-Urquijo EM , Bartres-Faz D , Belleville S , Cantilon M , Chetelat G , Ewers M , Franzmeier N , Kempermann G , Kremen WS , Okonkwo O , Scarmeas N , Soldan A , Udeh-Momoh C , Valenzuela M , Vemuri P , Vuoksimaa E , the Reserve, Resilience and Protective Factors PIA Empirical Definitions and Conceptual Frameworks Workgroup ((2020) ) Whitepaper: Defining and investigating cognitive reserve, brain reserve, and brain maintenance. Alzheimers Dement 16: , 1305–1311. |
[13] | Stern Y , Albert M , Barnes CA , Cabeza R , Pascual-Leone A , Rapp PR ((2023) ) A framework for concepts of reserve and resilience in aging. Neurobiol Aging 124: , 100–103. |
[14] | Stern Y ((2012) ) Cognitive reserve in ageing and Alzheimer’s disease. Lancet Neurol 11: , 1006–1012. |
[15] | Hoenig MC , Bischof GN , Hammes J , Faber J , Fliessbach K , van Eimeren T , Drzezga A ((2017) ) Tau pathology and cognitive reserve in Alzheimer’s disease. Neurobiol Aging 57: , 1–7. |
[16] | Hoenig MC , Bischof GN , Onur OA , Kukolja J , Jessen F , Fliessbach K , Neumaier B , Fink GR , Kalbe E , Drzezga A , van Eimeren T , Alzheimer’s Disease Neuroimaging Initiative ((2019) ) Level of education mitigates the impact of tau pathology on neuronal function. Eur J Nucl Med Mol Imaging 46: , 1787–1795. |
[17] | Stern Y , Zarahn E , Hilton HJ , Flynn J , DeLaPaz R , Rakitin B ((2003) ) Exploring the neural basis of cognitive reserve. J Clin Exp Neuropsychol 25: , 691–701. |
[18] | Bauer CE , Brown CA , Gold BT , Alzheimer’s Disease Neuroimaging Initiative ((2020) ) Education does not protect cognitive function from brain pathology in the ADNI 2 cohort. Neurobiol Aging 90: , 147–149. |
[19] | Mungas D , Fletcher E , Gavett BE , Widaman K , Zahodne LB , Hohman TJ , Mayeda ER , Dowling NM , Johnson DK , Tomaszewski Farias S ((2021) ) Comparison of education and episodic memory as modifiers of brain atrophy effects on cognitive decline: Implications for measuring cognitive reserve. J Int Neuropsychol Soc 27: , 401–411. |
[20] | Brown J , Cooper-Kuhn CM , Kempermann G , Van Praag H , Winkler J , Gage FH , Kuhn HG ((2003) ) Enriched environment and physical activity stimulate hippocampal but not olfactory bulb neurogenesis. Eur J Neurosci 17: , 2042–2046. |
[21] | Garthe A , Roeder I , Kempermann G ((2016) ) Mice in an enriched environment learn more flexibly because of adult hippocampal neurogenesis. Hippocampus 26: , 261–271. |
[22] | Almeida RP , Schultz SA , Austin BP , Boots EA , Dowling NM , Gleason CE , Bendlin BB , Sager MA , Hermann BP , Zetterberg H , Carlsson CM , Johnson SC , Asthana S , Okonkwo OC ((2015) ) Effect of cognitive reserve on age-related changes in cerebrospinal fluid biomarkers of Alzheimer disease. JAMA Neurol 72: , 699–706. |
[23] | Zahodne LB , Mayeda ER , Hohman TJ , Fletcher E , Racine AM , Gavett B , Manly JJ , Schupf N , Mayeux R , Brickman AM , Mungas D ((2019) ) The role of education in a vascular pathway to episodic memory: Brain maintenance or cognitive reserve? Neurobiol Aging 84: , 109–118. |
[24] | Dufouil C , Alperovitch A , Tzourio C ((2003) ) Influence of education on the relationship between white matter lesions and cognition. Neurology 60: , 831–836. |
[25] | Noble KG , Grieve SM , Korgaonkar MS , Engelhardt LE , Griffith EY , Williams LM , Brickman AM ((2012) ) Hippocampal volume varies with educational attainment across the life-span. Front Hum Neurosci 6: , 307. |
[26] | Vemuri P , Lesnick TG , Przybelski SA , Knopman DS , Machulda M , Lowe VJ , Mielke MM , Roberts RO , Gunter JL , Senjem ML , Geda YE , Rocca WA , Petersen RC , Jack CR , Jr. ((2016) ) Effect of intellectual enrichment on AD biomarker trajectories: Longitudinal imaging study. Neurology 86: , 1128–1135. |
[27] | Brickman AM ((2013) ) Contemplating Alzheimer’s disease and the contribution of white matter hyperintensities. Curr Neurol Neurosci Rep 13: , 415. |
[28] | Jack CR Jr Knopman DS , Jagust WJ , Shaw LM , Aisen PS , Weiner MW , Petersen RC , Trojanowski JQ ((2010) ) Hypothetical model of dynamic biomarkers of the Alzheimer’s pathological cascade. Lancet Neurol 9: , 119–128. |
[29] | Jack CR Jr Petersen RC , O’Brien PC , Tangalos EG ((1992) ) MR-based hippocampal volumetry in the diagnosis of Alzheimer’s disease. Neurology 42: , 183–188. |
[30] | Gomez-Isla T , Hollister R , West H , Mui S , Growdon JH , Petersen RC , Parisi JE , Hyman BT ((1997) ) Neuronal loss correlates with but exceeds neurofibrillary tangles in Alzheimer’s disease. Ann Neurol 41: , 17–24. |
[31] | Bennett DA , Schneider JA , Wilson RS , Bienias JL , Arnold SE ((2004) ) Neurofibrillary tangles mediate the association of amyloid load with clinical Alzheimer disease and level of cognitive function. Arch Neurol 61: , 378–384. |
[32] | Boyle PA , Yu L , Fleischman DA , Leurgans S , Yang J , Wilson RS , Schneider JA , Arvanitakis Z , Arfanakis K , Bennett DA ((2016) ) White matter hyperintensities, incident mild cognitive impairment, and cognitive decline in old age. Ann Clin Transl Neurol 3: , 791–800. |
[33] | Moloney CM , Lowe VJ , Murray ME ((2021) ) Visualization of neurofibrillary tangle maturity in Alzheimer’s disease: A clinicopathologic perspective for biomarker research. Alzheimers Dement 17: , 1554–1574. |
[34] | Braak H , Alafuzoff I , Arzberger T , Kretzschmar H , Del Tredici K ((2006) ) Staging of Alzheimer disease-associated neurofibrillary pathology using paraffin sections and immunocytochemistry.. Acta Neuropathol 112: , 389–404. |
[35] | Johnson SC ((2018) ) Brain imaging in dementia and antecedent conditions. In APA Handbook of Dementia Smith GE, Farias ST, eds. American Psychological Association, pp. 85–103. |
[36] | Vaque-Alcazar L , Sala-Llonch R , Valls-Pedret C , Vidal-Pineiro D , Fernandez-Cabello S , Bargallo N , Ros E , Bartres-Faz D ((2017) ) Differential age-related gray and white matter impact mediates educational influence on elders’ cognition.. Brain Imaging Behav 11: , 318–332. |
[37] | Chen Y , Qi D , Qin T , Chen K , Ai M , Li X , Li H , Zhang J , Mao H , Yang Y , Zhang Z ((2019) ) Brain network connectivity mediates education-related cognitive performance in healthy elderly adults. Curr Alzheimer Res 16: , 19–28. |
[38] | DeCarli C , Murphy DG , Tranh M , Grady CL , Haxby JV , Gillette JA , Salerno JA , Gonzales-Aviles A , Horwitz B , Rapoport SI , et al. ((1995) ) The effect of white matter hyperintensity volume on brain structure, cognitive performance, and cerebral metabolism of glucose in 51 healthy adults. Neurology 45: , 2077–2084. |
[39] | Birdsill AC , Koscik RL , Jonaitis EM , Johnson SC , Okonkwo OC , Hermann BP , Larue A , Sager MA , Bendlin BB ((2014) ) Regional white matter hyperintensities: Aging, Alzheimer’s disease risk, and cognitive function. Neurobiol Aging 35: , 769–776. |
[40] | Lockhart SN , Roach AE , Luck SJ , Geng J , Beckett L , Carmichael O , DeCarli C ((2014) ) White matter hyperintensities are associated with visual search behavior independent of generalized slowing in aging. Neuropsychologia 52: , 93–101. |
[41] | Kloppenborg RP , Nederkoorn PJ , Geerlings MI , van den Berg E ((2014) ) Presence and progression of white matter hyperintensities and cognition: A meta-analysis. Neurology 82: , 2127–2138. |
[42] | den Heijer T , van der Lijn F , Koudstaal PJ , Hofman A , van der Lugt A , Krestin GP , Niessen WJ , Breteler MM ((2010) ) A 10-year follow-up of hippocampal volume on magnetic resonance imaging in early dementia and cognitive decline. Brain 133: , 1163–1172. |
[43] | O’Shea A , Cohen RA , Porges EC , Nissim NR , Woods AJ ((2016) ) Cognitive aging and the hippocampus in older adults. Front Aging Neurosci 8: , 298. |
[44] | Manly JJ , Jacobs DM , Touradji P , Small SA , Stern Y ((2002) ) Reading level attenuates differences in neuropsychological test performance between African American and White elders. J Int Neuropsychol Soc 8: , 341–348. |
[45] | Choi SE, Mukherjee S, Gibbons LE, Sanders RE, Jones RN, Tommet D, Mez J, Trittschuh EH, Saykin A, Lamar M, Rabin L, Foldi NS, Sikkes S, Jutten RJ, Grandoit E, Mac Donald C, Risacher S, Groot C, Ossenkoppele R, Alzheimer’s Disease Neuroimaging Initiative, Crane PK ((2020) ) Development and validation of language and visuospatial composite scores in ADNI. Alzheimers Dement (N Y) 6: , e12072. |
[46] | Schretlen DJ , Buffington AL , Meyer SM , Pearlson GD ((2005) ) The use of word-reading to estimate “premorbid” ability in cognitive domains other than intelligence. J Int Neuropsychol Soc 11: , 784–787. |
[47] | Weiner MW , Veitch DP , Aisen PS , Beckett LA , Cairns NJ , Green RC , Harvey D , Jack CR Jr, Jagust W , Morris JC , Petersen RC , Salazar J , Saykin AJ , Shaw LM , Toga AW , Trojanowski JQ , Alzheimer’s Disease Neuroimaging Initiative ((2017) ) The Alzheimer’s Disease Neuroimaging Initiative 3: Continued innovation for clinical trial improvement. Alzheimers Dement 13: , 561–571. |
[48] | Hartig M, Truran-Sacrey D, Raptentsetsang S, Simonson A, Mezher A, Schuff N, Weiner M, UCSF FreeSurfer Methods, Alzheimer’s Disease Neuroimaging Initiative https://adni.bitbucket.io/reference/docs/UCSFFSX51/UCSF%20FreeSurfer%20Methods%20and%20QC_OFFICIAL.pdf. |
[49] | Reuter M , Schmansky NJ , Rosas HD , Fischl B ((2012) ) Within-subject template estimation for unbiased longitudinal image analysis. Neuroimage 61: , 1402–1418. |
[50] | DeCarli C , Miller BL , Swan GE , Reed T , Wolf PA , Garner J , Jack L , Carmelli D ((1999) ) Predictors of brain morphology for the men of the NHLBI twin study.. Stroke 30: , 529–536. |
[51] | Jack CR Jr Wiste HJ , Weigand SD , Therneau TM , Lowe VJ , Knopman DS , Gunter JL , Senjem ML , Jones DT , Kantarci K , Machulda MM , Mielke MM , Roberts RO , Vemuri P , Reyes DA , Petersen RC ((2017) ) Defining imaging biomarker cut points for brain aging and Alzheimer’s disease. Alzheimers Dement 13: , 205–216. |
[52] | Crane PK , Carle A , Gibbons LE , Insel P , Mackin RS , Gross A , Jones RN , Mukherjee S , Curtis SM , Harvey D , Weiner M , Mungas D , Alzheimer’s Disease Neuroimaging Initiative ((2012) ) Development and assessment of a composite score for memory in the Alzheimer’s Disease Neuroimaging Initiative (ADNI). Brain Imaging Behav 6: , 502–516. |
[53] | Gibbons LE , Carle AC , Mackin RS , Harvey D , Mukherjee S , Insel P , Curtis SM , Mungas D , Crane PK , Alzheimer’s Disease Neuroimaging Initiative ((2012) ) A composite score for executive functioning, validated in Alzheimer’s Disease Neuroimaging Initiative (ADNI) participants with baseline mild cognitive impairment. Brain Imaging Behav 6: , 517–527. |
[54] | VanderWeele TJ ((2014) ) A unification of mediation and interaction: A 4-way decomposition. Epidemiology 25: , 749–761. |
[55] | Valeri L , Vanderweele TJ ((2013) ) Mediation analysis allowing for exposure-mediator interactions and causal interpretation: Theoretical assumptions and implementation with SAS and SPSS macros. Psychol Methods 18: , 137–150. Erratum in: Psychol Methods. 2013;18(4):474. |
[56] | Shi B, Choirat C, Valeri L, Statistical Modeling with Multiple Mediators, https://bs1125.github.io/CMAverse/articles/multiple_mediators.html, Accessed May 2, 2023. |
[57] | Brickman AM , Siedlecki KL , Muraskin J , Manly JJ , Luchsinger JA , Yeung LK , Brown TR , DeCarli C , Stern Y ((2011) ) White matter hyperintensities and cognition: Testing the reserve hypothesis. Neurobiol Aging 32: , 1588–1598. |
[58] | Zhu W , Li X , Li X , Wang H , Li M , Gao Z , Wu X , Tian Y , Zhou S , Wang K , Yu Y ((2021) ) The protective impact of education on brain structure and function in Alzheimer’s disease. BMC Neurol 21: , 423. |
[59] | O’Shea DM , Langer K , Woods AJ , Porges EC , Williamson JB , O’Shea A , Cohen RA ((2018) ) Educational attainment moderates the association between hippocampal volumes and memory performances in healthy older adults. Front Aging Neurosci 10: , 361. |
[60] | Maguire EA , Gadian DG , Johnsrude IS , Good CD , Ashburner J , Frackowiak RS , Frith CD ((2000) ) Navigation-related structural change in the hippocampi of taxi drivers. Proc Natl Acad Sci U S A 97: , 4398–4403. |
[61] | Parks CM , Iosif AM , Farias S , Reed B , Mungas D , DeCarli C ((2011) ) Executive function mediates effects of white matter hyperintensities on episodic memory. Neuropsychologia 49: , 2817–2824. |
[62] | Eng CW , Gilsanz P , Fletcher EM , Kornak J , George KM , DeCarli CS , Barnes LL , Glymour MM , Whitmer RA ((2021) ) Education, white matter hyperintensities, and cognition in diverse cohorts of older adults. Alzheimers Dement 17: , (Suppl 10), e056709. |
[63] | Mortamais M , Portet F , Brickman AM , Provenzano FA , Muraskin J , Akbaraly TN , Berr C , Touchon J , Bonafe A , le Bars E , Menjot de Champfleur N , Maller JJ , Meslin C , Sabatier R , Ritchie K , Artero S ((2014) ) Education modulates the impact of white matter lesions on the risk of mild cognitive impairment and dementia. Am J Geriatr Psychiatry 22: , 1336–1345. |
[64] | Hernan MA , Monge S ((2023) ) Selection bias due to conditioning on a collider. BMJ 381: , 1135. |
[65] | Chen Y , Lv C , Li X , Zhang J , Chen K , Liu Z , Li H , Fan J , Qin T , Luo L , Zhang Z ((2019) ) The positive impacts of early-life education on cognition, leisure activity, and brain structure in healthy aging. Aging (Albany NY) 11: , 4923–4942. |
[66] | Schipolowski S , Wilhelm O , Schroeders U ((2014) ) On the nature of crystallized intelligence: The relationship between verbal ability and factual knowledge. Intelligence 46: , 156–168. |
[67] | Chin AL , Negash S , Xie S , Arnold SE , Hamilton R ((2012) ) Quality, and not just quantity, of education accounts for differences in psychometric performance between African Americans and white non-Hispanics with Alzheimer’s disease. J Int Neuropsychol Soc 18: , 277–285. |
[68] | Manly JJ , Byrd DA , Touradji P , Stern Y ((2004) ) Acculturation, reading level, and neuropsychological test performance among African American elders. Appl Neuropsychol 11: , 37–46. |
[69] | Gianattasio KZ , Bennett EE , Wei J , Mehrotra ML , Mosley T , Gottesman RF , Wong DF , Stuart EA , Griswold ME , Couper D , Glymour MM , Power MC , Alzheimer’s Disease Neuroimaging Initiative ((2021) ) Generalizability of findings from a clinical sample to a community-based sample: A comparison of ADNI and ARIC. Alzheimers Dement 17: , 1265–1276. |
[70] | Guidi J , Lucente M , Sonino N , Fava GA ((2021) ) Allostatic load and its impact on health: A systematic review. Psychother Psychosom 90: , 11–27. |
[71] | Ding X , Barban N , Mills MC ((2019) ) Educational attainment and allostatic load in later life: Evidence using genetic markers. Prev Med 129: , 105866. |
[72] | D’Amico D , Amestoy ME , Fiocco AJ ((2020) ) The association between allostatic load and cognitive function: A systematic and meta-analytic review. Psychoneuroendocrinology 121: , 104849. |
[73] | Koran MEI , Wagener M , Hohman TJ , Alzheimer’s Neuroimaging I ((2017) ) Sex differences in the association between AD biomarkers and cognitive decline. Brain Imaging Behav 11: , 205–213. |